- 1Department of Engineering Design and Mathematics, University of the West of England, Bristol, UK
- 2Bristol Urological Institute, Southmead Hospital, Bristol, UK
- 3School of Biosciences, Cardiff University, Cardiff, UK
- 4North Bristol NHS Trust, Southmead Hospital, Bristol, UK
- 5Department of Medical Microbiology, North Bristol NHS Trust, Southmead Hospital, Bristol, UK
- 6School of Clinical Sciences, University of Bristol, Bristol, UK
The urinary microbiome of healthy individuals and the way it alters with ageing have not been characterized and may influence disease processes. Conventional microbiological methods have limited scope to capture the full spectrum of urinary bacterial species. We studied the urinary microbiota from a population of healthy individuals, ranging from 26 to 90 years of age, by amplification of the 16S rRNA gene, with resulting amplicons analyzed by 454 pyrosequencing. Mid-stream urine (MSU) was collected by the “clean-catch” method. Quantitative PCR of 16S rRNA genes in urine samples, allowed relative enumeration of the bacterial loads. Analysis of the samples indicates that females had a more heterogeneous mix of bacterial genera compared to the male samples and generally had representative members of the phyla Actinobacteria and Bacteroidetes. Analysis of the data leads us to conclude that a “core” urinary microbiome could potentially exist, when samples are grouped by age with fluctuation in abundance between age groups. The study also revealed age-specific genera Jonquetella, Parvimonas, Proteiniphilum, and Saccharofermentans. In conclusion, conventional microbiological methods are inadequate to fully identify around two-thirds of the bacteria identified in this study. Whilst this proof-of-principle study has limitations due to the sample size, the discoveries evident in this sample data are strongly suggestive that a larger study on the urinary microbiome should be encouraged and that the identification of specific genera at particular ages may be relevant to pathogenesis of clinical conditions.
Introduction
The term “microbiome” refers to all microbiota in a defined microbial community (Dave et al., 2012). With molecular tools now developed to assess the composition and diversity of particular microbiomes far more accurately, and independently of culture methods, potential insights into the affiliation between humans and their associated microbiota in healthy and disease can be obtained. Bacteria constitute 90% of all cells in the human body, and this symbiotic relationship is crucial in maintaining health and for proper development of the host (Proctor, 2011). For example, maintaining a vaginal environment that is dominated by Lactobacillus species is associated with healthy pregnancy outcomes, lack of vaginal symptoms and reduced risk for acquiring several sexually transmitted pathogens (Marrazzo, 2011). In addition, studies of the gut have demonstrated a variety of functions for the resident microbiota in maintaining the host's health, including metabolic and trophic functions, as well as providing a protective barrier against pathogens (Guarner and Malagelada, 2003; Chervonsky, 2012).
The bladder was notably not included within the Human Microbiome Project. Historically urine has been considered sterile until reaching the urethra in healthy individuals, hence lacking in an associated microbiota (Fouts et al., 2012). However, urine (as a reflection of the bladder microbiota) from healthy individuals does contain extensive numbers of bacteria, which are not routinely cultivated by clinical microbiology laboratories, but can be identified by 16S rRNA gene sequencing (Nelson et al., 2010; Siddiqui et al., 2011; Wolfe et al., 2012).
The intestinal microbiota of healthy humans progressively develops in complexity from birth until adulthood, where a stable microbiome is established for the majority of an individual's adult life (Arumugam et al., 2011; Jalanka-Tuovinen et al., 2011; Durbán et al., 2012). Yet with aging, physiological changes (e.g., changes in diet, lifestyle, immune system function) induced by the ageing process will likely affect the abundance of an individual's bacteria at every bodily niche (Biagi et al., 2012). Therefore, as for the intestinal microbiota, it is important to understand how the ageing process shapes the microbiota within the healthy, ageing bladder as a platform for future studies (O'Toole, 2012).
The aim of this study was to capture the total urinary microbiota from a sample of healthy individuals of various ages by amplification of the 16S rRNA gene (surrogate marker for the presence of bacteria) with resulting amplicons analyzed by the 454 pyrosequencing high-density system. While bladder biopsies or suprapubic aspirates would provide the best quality material for capturing the bladder microbiota, this was not feasible for a pragmatic study in a healthy population (Wolfe et al., 2012). Accordingly, clean-catch mid-stream urine (MSU) samples were employed to characterize the urinary microbiota, as a reflection of the bladder microbiota. To enumerate the amount of bacteria within each sample and to discriminate contaminants as those that fall below a certain level in a comparable manner to standard urine microbiology tests, DNA within each sample was quantified by qPCR and compared to a known number of operon copies/ml of a urine sample spiked with Escherichia coli also quantified by qPCR. This strategy allowed us to relate the relative abundance of bacteria between donated samples and provide more informative data.
Materials and Methods
Sample Collection
Participants were recruited from people attending a secondary care urology clinic and informed consent was obtained from all. The UK South West Central National Research Ethics Service gave ethical approval for the study (NRES reference number 09/H0102/68). The inclusion criteria were as follows: no lower urinary tract symptoms, no history of urinary tract infection (cystitis, pyelonephritis, prostatitis) in the preceding year and no recent use (>1 month) of antibiotics for any indication. Volunteers were instructed to provide a clean-catch, mid-stream voided urine sample into a sterile container. Samples were immediately tested with a urinary dipstick to determine if they were positive for nitrites (Siemens Multistix 8 SG). The samples were anonymized in the order they were received by a local coding system (e.g., UWE01, UWE02 etc.), stored at 4°C and cultured within 4 h of sampling. An aliquot of the samples was frozen at −20°C for subsequent DNA extraction also.
Extraction of DNA from Urine and Quality Check
DNA was extracted by bead beating in a lysis buffer containing detergent followed by alcohol precipitation. A co-precipitant (sterile linear polyacrylamide, LPA) was included. All buffers were made up from 18.2 MΩ water and autoclaved. Blank extractions were used to confirm zero 16S rRNA gene background contamination in the reagents.
Urine samples (2 ml) were sedimented (2600 g, 5 min) and the pellet washed once in PBS. The pellet was resuspended in 850 μl lysis buffer (3% w/v sodium dodecyl sulphate in 50 mM tris, 5 mM EDTA, pH 8.0, 10 μg/ml RNase A) and transferred to a sterile bead beating tube containing 0.5 g 0.1 mm sterile glass beads. The sample was agitated at 6 m/s for 2 × 45 s. After centrifugation (16000 g, 10 min) 500 μl supernatant was transferred to a fresh tube and 350 μl 5 M ammonium acetate was added and the tubes held on ice for 5 min and centrifuged (16000 g, 5 min). The supernatant was held on ice for a further 5 min and centrifuged (16000 g, 5 min). This was followed by sequentially adding to the supernatant 10 μl 0.5% LPA and 850 μl iso-propyl alcohol. The sample was held at room temperature for 5 min and centrifuged (16000 g, 5 min). The pellet was washed in 500 μl 70% ethanol and re-dissolved in 400 μl TE buffer (10 mM Tris; HCl 1 mM EDTA pH 8.0). To this was added 1 ml 100% ethanol and after 5 min at room temperature the sample was centrifuged (16000 g, 5 min). The pellet was washed in 500 μl 70% ethanol, air dried and dissolved in 50 μl TE buffer. DNA extracts were stored at −80°C.
DNA was confirmed to be of sufficient quality for downstream PCR applications by 16S rRNA PCR by amplifying the V1-V3 hypervariable region of the bacterial 16S rRNA gene using primers 63F (5′ CAGGCCTAACACATGCAAGTC 3′) and 517R (5′ AGGCCTAACACATGCAAGTC 3′). All PCR amplifications were conducted in 50 μl volume containing 5 μl of DNA (10 to <1 ng per reaction depending on sample yield) according to manufacturers of the DNA polymerase (Moltaq 16S, Molzym, Bremen, Germany). The kit was supplied as a 2.5 × mastermix which contains dNTPS, Taq polymerase and MgCl2 (exact content is proprietary). An automated thermal cycler (BioRad, Hemel Hempstead, UK) was used for PCR amplification which was programmed for an initial denaturation of 94°C for 10 min, 40 cycles of denaturation (94°C for 30 s), annealing (60°C for 60 s) and extension (72°C for 120 s) and a final extension of (72°C for 10 min) as per manufacturer's instructions. The samples were verified on a 1.5% w/v agarose gel.
Quantification of Sample Bacterial Load by Quantitative PCR (qPCR) of 16S rRNA DNA
Sample bacterial load was quantified by 16S rRNA qPCR of the urine DNA extracts (Mastermix 16S, Molzym, Bremen, Germany). The kit reagent was supplied as a 2.5 × mastermix which contained dNTPs, primers (343F 5′ TCCTACGGGAGGCAGCAGT 3′ and 809R 5′ GGACTACCAGGGTATCTAATCCTGTT 3′), Taq polymerase and SYBR fluorescent dye in a PCR buffer (exact content is proprietary). A volume of 15 μl of this secondary mix was then added to 10 μl of a 1/10 dilution of respective urine DNA extract/E. coli DNA calibrator. The supplied Taq was non hot-start; this necessitated assay set-up on ice, and the use of the PCR cycler plate to be preheated (95°C) in order to minimize primer-dimer formation (verified by melt curve analysis). The amount of urine extract DNA template was 20 to <1 ng/reaction (it was not possible to accurately quantify the amount of DNA in all extracts since some concentrations were <1 ng/μl). The total urine DNA extract volume was 50 μl which originated from 2 ml urine, and 10 μl of a 1/10 dilution of extract was added. The qPCR was calibrated using DNA obtained from an enumerated E. coli liquid culture (OD 600 nm of 1.5) extracted by the urine DNA protocol.
In our hands linearity of the enumerated E. coli DNA extract was observed over 6 dilution decades from an initial 200 ng/reaction to 200 fg/reaction (equivalent enumerated culture 7 × 106 to 70 cfu per reaction, 4.9 × 107 to 490 operon copies per reaction using a copy value of 7 operons per CFU; Klappenbach et al., 2001). This yielded a urine assay range (obtained by calculation from extraction factorization) of 1.75 × 108 to 1.75 × 103 E. coli cfu/ml which is 1.25 × 109 to 1.25 × 104 operon copies/ml equivalent. The qPCR assay was susceptible to primer-dimer interference. Optimization experiments indicated that under the employed conditions a false signal originated in the negative sample (blank) and low copy sample extracts at 30 cycles. This yielded a practical detection limit of 104 cfu/ml or 7 × 104 operon copies/ml.
Sequencing and Analysis
FLX-titanium amplicon pyrosequencing (bTEFAP) of the VI-V3 regions of the 16S rRNA gene was performed by Research and Testing Laboratory (Lubbock, Tx, USA) and the tagged reads were denoized and chimeras removed prior to analysis in the host institution (Edgar et al., 2011). Once the reads were transferred they were analyzed by means of the bioinformatic software Mothur (http://www.mothur.org: version 1.28.1) using absolute numbers in each sample to minimize any artifacts which might be introduced due to re-sampling (Schloss et al., 2009). The reads were mapped to the Ribosomal Database Project (RDP) taxonomy by Mothur using a dissimilarity cutoff value of 0.03 and only operational taxonomic units (OTUs) with a minimum of 10 reads in any sample were used. The unique OTUs from this pipeline were used in the R statistical environment to determine clusters and heatmaps. The number of clusters was determined using partitioning around medoids (PAMK) in the package FPC in R. The 16S rRNA gene sequences are available for download from the European Nucleotide Archive (ENA) under accession number PRJEB4256.
Results
Sample Collection
MSU samples were collected by the clean-catch method from male (n = 6; age range 39–83 years of age) and female (n = 10; age range 26–90 years of age) donors. DNA extractions and PCR analysis of V1–V3 hypervariable region using 63F and 517R primers was performed to ensure samples were sufficient to be amplifiable by downstream PCR methods. Samples were confirmed to be of good quality DNA and were sent to the Research and Testing Laboratories (Lubbock, Texas) for 454 pyrosequencing with returned results as shown in Table 1.
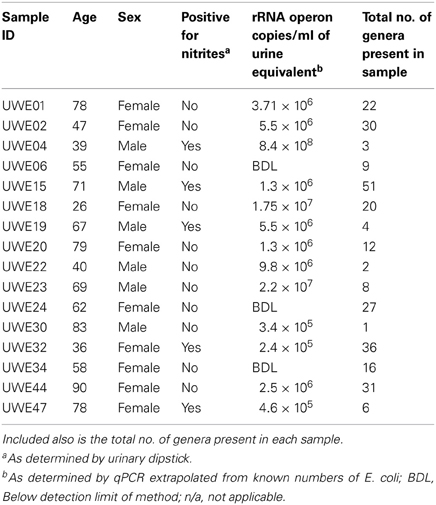
Table 1. Table listing the sex and age of each sample including the “equivalent” number of rRNA operon copies/ml.
Taxonomic Assignment of the Sequences
The 16S rRNA gene sequences were classified using the bioinformatics software Mothur and a dissimilarity cut-off of 0.03, into 663 OTUs. Upon further analysis, according to criteria stipulated in the methods, this was reduced to 234 OTUs and came from 10 phyla, 17 classes, 27 orders, 56 families, and 94 genera (Table A1). The microbiota of the 16 samples clustered into two groups as determined by the partitioning around mediods method (PAMK in R); those that were dominated by one phylum and those with more than one phylum (Figure A1). Of the monophyletic samples, three (UWE04, UWE22, and UWE30) were dominated by OTUs which clustered in the Firmicutes, while two (UWE19 and UWE47) were dominated by OTUs from the Proteobacteria. In the samples in which one phylum was present there was commonly only one OTU represented from that phylum; OTU525: genus Staphylococcus (in sample UWE04), OTU527: genus Lactobacillus (UWE22), OTU545: genus Aerococcus (UWE30), OTU582: genus Pseudomonas (UWE19), and OTU590: genus Enterobacter (UWE47) (Figure 1). The remaining samples had on average 5 phyla (range 2–8; Table A2).
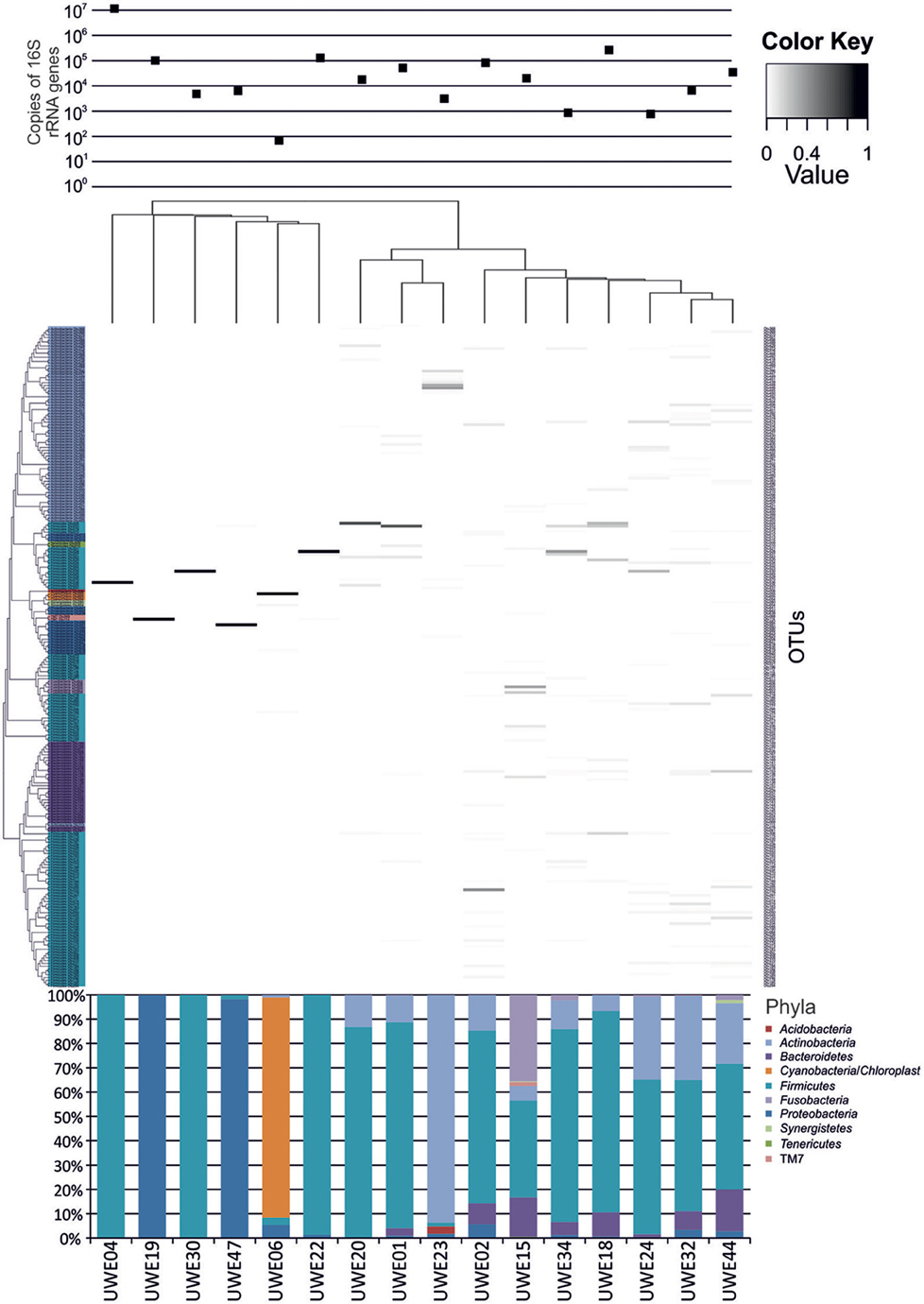
Figure 1. Heatmap showing the relative abundance of the OTUs per sample. The top panel presents the qPCR values for each sample while the bottom panel shows the percentage distribution of the OTUs' phyla for each sample.
Enumeration of 16S rRNA Genes by qPCR
To enable quantitative comparison between urine samples a qPCR assay of the 16S rRNA gene was done and compared to a known enumerated E. coli sample. Due to the inter species variation in rRNA operons per genome it was decided to express the urine bacterial load arbitrarily as operon copy/ml equivalent, using a copy value for E. coli of 7 operons per CFU (Klappenbach et al., 2001).
Table 1 shows the “equivalent” number of rRNA operon copies/ml of urine per patient and also the total number of different genera which were present within each sample. In 6 of the samples (5 female—UWE01, UWE02, UWE18, UWE20, and UWE44; and 1 male—UWE 22) the results of the urinary dipstick for the detection of nitrites as an indication of the presence of bacteria was negative but results from the qPCR suggest the bacterial count would likely be in numbers far greater than 1 × 105 cfu/ equivalent based on the copy value of E. coli of 7 operons per CFU. Equally two female samples were positive to nitrites (UWE32 and UWE44) however bacterial counts would likely be less than 1 × 105 cfu/ml equivalent, as determined by qPCR. Overall therefore 50% of samples could have been potentially misclassified based on the urinary dipstick analysis alone.
Diversity of Bacteria within Each Gender as Individuals
When considering the 16 samples collectively, the general trend is a more heterogeneous mix of bacterial genera in the female samples (range 6–36, average 21, median 21) than the male (range 1–8 plus one sample of 51, average 11.5, median 3.5) (Table 1; Figure 1). Analysis using the non-parametric Mann Whitney test indicates that the distribution of total genera is sex dependent (Z = 2.061, n1 = 10, n2 = 6, p = 0.042, two-sided). Three quarters of samples have greater than 50% of the total abundance of bacteria belonging to the phylum Firmicutes and this was true for both male and female samples. Female samples also generally had representative members of the phyla Actinobacteria and Bacteroidetes, which were generally absent from the male samples.
Diversity of Bacteria by Age as Individuals
Considering samples individually in the first instance, Figure 2 is a plot of the total number of genera identified per person against age. Statistical analysis using Spearman's correlation coefficient indicates that the number of genera and age are not significantly correlated in the sample (r = −0.029, p = 0.914, two-sided).
Considering the number of operons/ml as determined by qPCR for both routinely cultivated and not routinely cultivated individually, it can be seen in Figure 3 that the “total” number of bacteria is similar across the age for both groups. Note “not routinely cultivated” also includes those not reported individually by standard methods; please see Tables A3, A4 for more details on groupings. Further analysis using Spearman's rank correlation coefficient indicates that the sample relationship between operons/ml and age does not achieve statistical significance for genera not routinely cultivated (r = −0.464, p = 0.110, two-sided) or for routinely cultivated genera (r = −0.391, p = 0.187, two-sided).
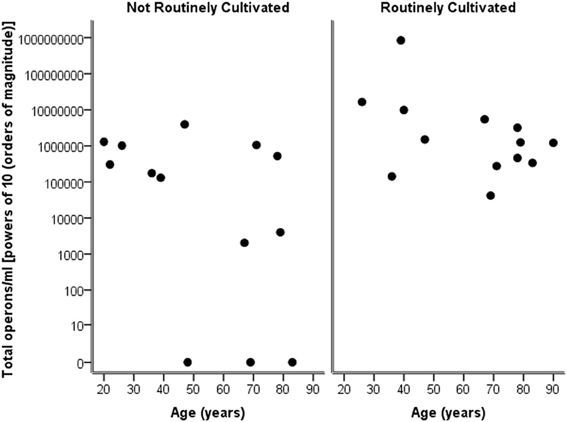
Figure 3. Plot of total operons/ml per person (powers of 10—order of magnitude) for each genus that is cultivated routinely by standard microbiological testing, and those not routinely cultivated (including those not individually identified in routine culture).
Breaking down the “total” number of bacteria per participant into the “total” numbers of bacteria for each genera against age, it can again be seen in Figure 4 that numbers are highly similar across the ages and for both routinely cultivated and not routinely cultivated bacteria.
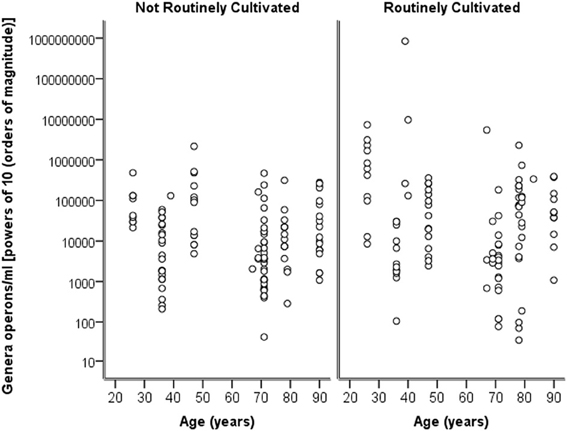
Figure 4. A plot of the genus specific count (operons/ml) on a logarithmic base ten scale against age for routinely cultivated and not routinely cultivated bacteria (including those not individually identified in routine culture).
Diversity of Bacteria by Age When Grouped
When samples are grouped into the following age ranges: 20–49 years, 50–69 years, and 70 plus years, irrespective of gender it is notable that the following genera appeared exclusive to the 70 plus age group, namely Jonquetella, Parvimonas, Proteiniphilum, and Saccharofermentans. Analysis using the non-parametric Mann Whitney test indicates that the distribution of the frequencies of this cluster of genera is age dependent (Z = 3.873, n1 = 6, n2 = 10, p < 0.001, two-sided).
Diversity of Bacteria by Age and Gender When Grouped
The sixteen samples were grouped within the following age ranges: 20–49 years, 50–69 years, and 70 plus years.
For females aged 20–49 (n = 3) the number of different genera identified was 48, with 30 of these having a predicted operons/ml of greater than the detection limit by qPCR (7 × 104 operons/ml equivalent) (Table 2). The average total operons/ml for all bacteria for the females within the age group 20–49 (n = 3) was 1.3 × 105. For females within the age group 50 to 69+ (n = 3) the number of genera present was 36. It was not however possible to predict operons/ml within this group for any of the samples, as the readings were below the detection limit of the qPCR method. Nonetheless, it does suggest that the bacterial counts were much lower than the other two groups who reached at least the detection limit. In the 70+ age group the number of different genera identified was 43 (n = 4). The average total operons/ml for all bacteria for the females in this age group (70+) was 3.3 × 104. Therefore in the 70+ female age group, there were approximately 75% fewer bacteria (operons/ml) than in the age group 20–49. Notably, the age groups 20–49 and 70+ had in common 17 genera that were present in both groups at numbers greater than the predicted 7 × 103 operons/ml (Table 2). A further 6 genera were common within these groups but detectable in greater numbers (>7 × 103 operons/ml) in the age group 20–49, than in the 70+ (Table 2).
The males presented a different picture, in that the numbers of genera present increased through the age groups (Table 3). However, despite the increase in number of genera within the male age group 70+, the average total number of bacteria was lower than the younger age groups with a predicted 4.9 × 107 operons/ml in the age group 20–49, compared with 9 × 104 operons/ml in the age group 50–69 and 1 × 105 operons/ml in the 70+ age group. Analysis using the Kruskal-Wallis test shows that these observed sample difference do not achieve statistical significance (H = 2.593, df = 2, p = 0.273).
Discussion
Understanding how the human microbiota develops and changes with ageing is essential for future studies investigating the effects of changes in the microbiota, and implications for maintaining a healthy host and whether any disease state results. Physiological changes that are induced by the ageing process, age-related events (for example morbidity, medication and lifestyle) and the reduction of functionality of the immune system or immunosenescence will inevitably modify the composition of the microbiota throughout the human body (Gruver et al., 2007). This change has been clearly demonstrated in the gut microbiota, sampling faeces, and the evidence provided here suggests it is likely an analogous situation occurs in the bladder and sampling urine (Tiihonen et al., 2010; Jalanka-Tuovinen et al., 2011; Biagi et al., 2012; O'Toole, 2012; Yatsunenko et al., 2012).
The method of sampling is frequently debated in any study relating the microbiota of the urine (Nelson et al., 2010; Dong et al., 2011; Wolfe et al., 2012). The difficulties in getting a genuine “clean-catch” MSU sample representative of only organisms originating from the bladder is well-recognized and especially so for females, in which the urine has to pass through the distal urethra and/or for the elderly (both male and female), who have physical or other impairments (Franz and Hörl, 1999; Lifshitz and Kramer, 2000; Wilson and Gaido, 2004). Nonetheless, it is less intrusive than alternative approaches, such as bladder tissue biopsy or catheterization, and hence the most pragmatic and ethical approach for larger, longitudinal studies and clinical application and it was therefore our positive intention to collect samples in this way (Wolfe et al., 2012). However this is not withstanding potential urethral or perineal contamination which in a larger study could be considered by determining thresholds (operons/ml) in which to disregard bacteria that fall below this as potential contaminants. Further work could also include taking a urethral swab for comparison to the urine sample to identify with greater clarity what most likely resides truly in the urethra and has simply contaminated the urine sample.
Samples were collected from participants ranging in age from 26 to 90 years of age from both genders. Whilst these participants had no lower urinary tract symptoms and no history of urinary tract infection in the preceding year it is worth noting that the participants were visiting the hospital as an out-patient for other unspecified treatments and may not therefore be considered representative of the primary care population. Samples were also only included if antibiotics had not been taken for any indication in the preceding month. However a study by Dethlefsen and Relman (2011) suggests it may take greater than 2 months to return to a baseline microbiota or at least an altered stable composition. We also acknowledge that certain groups, for example the elderly are likely to have had repeated antibiotic exposure which will result in the possibility of greater dynamic change to the bladder microbiota in comparison to a different age group. Therefore the comparison of samples within age groups in a larger study will be essential.
Culture-independent molecular PCR methods were used to analyse all samples which included 454 pyrosequencing and quantitative PCR, as routine culture methods would not capture the entire urinary microbiota (Imirzalioglu et al., 2008; Wolfe et al., 2012). The sequencing results identified 94 different genera with only 31 likely to be routinely cultivated by standard culture methods. The remaining 63 are either not routinely cultivated or would not be reported individually by routine urine investigations in an NHS microbiology laboratory (Health Protection Agency, 2012). Therefore there is an important discrepancy between what is reported by United Kingdom National Health Service microbiology laboratories and what can actually be detected within urine using molecular methods.
To allow for direct, quantifiable comparisons of the bacterial abundance between each sample, each sample was measured by qPCR. This was to determine the number of copies of 16S rRNA gene against a spiked urine sample with a known number of Escherichia coli, also measured by qPCR to determine the number of copies of the 16S rRNA gene. We acknowledge this method has limitations, for example the assumption that all bacteria have the same copy number for the 16S rRNA gene leading to an over- or under-estimation for many bacteria. It does, however, allow us to directly compare the abundance of the different genera between samples and give us an indication at the very least of the number of colony forming units per ml of urine of bacteria.
In agreement with Fouts et al. (2012), the data presented here concurs that the different sexes have significantly different genera of bacteria present in their urine, different numbers of genera and that sequences in the main belong to the phylum Firmicutes for both male and female samples, as in the study by Siddiqui et al. (2011). The general lack of representatives from the phyla Actinobacteria and Bacteroidetes in the male samples within this study also seems typical in comparison to other studies (Nelson et al., 2010; Dong et al., 2011). The current study also describes the first observation of the genera Soehngenia detected within a sample originating from a human, in this instance urine, seen in four of the subjects, male (n = 1) and female (n = 3). The fastidious anaerobic bacteria from this genus would not be detected by standard methods (Parshina et al., 2003).
The correlation of the results of the urinary dipstick as a preliminary indication of bacterial presence of numbers greater than 1 × 105 cfu/ml has again been questioned within this study. Semeniuk and Church (1999) reported that approximately 20% of samples by urinary dipstick analysis would have been sent to the laboratory for microbiology testing and therefore not screened for bacteriuria. In this study, eight out of 16 samples could potentially have been misclassified by urinary dipstick analysis, either being called “negative” when actually positive on qPCR (n = 6), or “positive” when actually negative (n = 2). Additionally, by quantifying the total number of bacteria by qPCR methods, we have shown that the numbers of bacteria that are routinely cultivated are comparable in amount to the numbers not routinely cultivated and/or identified individually. This raises questions on the precision of methods used in routine microbiological investigation for reporting a total bacterial count. By inaccurately informing clinicians of the presence/absence and quantity of bacteria, there may be serious implications in any treatment plan of patients.
Whilst statistical significance was not achieved, there is marginal evidence that the numbers of genera decrease with age when individually considered, but that the total number of bacteria is independent of age. However the use of relatively small numbers of donors in the context of many different bacteria being identified, affects the ability of the study to make definitive conclusions.
It is clear that the bacterial composition in the urine collected within this study is highly variable regardless of sex or age. This makes it unlikely that a comparable bacterial community between individuals of the same age or gender exists, just as Siddiqui et al. (2011) suggest. Nevertheless, quantifying the samples by qPCR has allowed us directly to compare one sample to another, and thus group samples together according to their age and sex for collated comparisons. Dividing into arbitrary age groups, the data is suggestive of the existence of a “core” bladder microbiome, with variability in the amount of the “core” bacteria and flux of other bacteria with ageing, much like the gut microbiome (Arumugam et al., 2011; Jalanka-Tuovinen et al., 2011). The female samples perhaps best demonstrate this. Across all three age groups, the female samples have in common 23 genera, the “core” microbiome, with each age group additionally having their own distinct subset of genera. There are also a number of genera that occur within two age groups, suggesting a transitional period may occur. Unfortunately the qPCR method for enumerating/predicting bacterial counts was not valid for the samples within the 50–69 age group, as the samples were below the detection limit of the method. However, it is interesting that the age groups 20–49 and the 70+ have 32 genera in common; 17 were present at greater than 103 “E. coli equivalent” cfu/ml as determined by qPCR, so highly unlikely to be contaminants. Six of these genera were found in higher numbers in the age group 20–49 than the age group 70+, suggesting that these bacteria may remain present throughout life, but their quantity could be indicative of the age group.
Peters et al. (2009) have argued a link between earlier episodes of UTI in life, with the increasing prevalence of such conditions as interstitial cystitis (IC) and painful bladder syndrome (PBS). The data here could support the hypothesis that bladder colonization with specific genera in early child/adulthood (asymptomatic or symptomatic) might influence propensity to bladder pathology in later life as one factor in the multi-factorial basis of disease pathogenesis.
For both the male and female samples, the average total number of urinary bacteria measured by predicting operons/ml decreased with increasing age when considered in groups. Confirmation of this observation, and mechanisms responsible, would need to be addressed in a more substantial study but one possible reason is varying bacteria load according to sexual activity and therefore contamination of the urine from the urethra (Dong et al., 2011). However, for both the male and female samples within the age group 70+, four different genera unique to this age category were identified, namely bacteria of the genera Jonquetella, Parvimonas, Proteiniphilum, and Saccharofermentans. These bacteria are strict anaerobes and not routinely cultured, suggesting the possibility of specific bacteria more likely affecting the elderly age-groups but will need to be further explored in a wider study with greater numbers.
This study provides a catalogue of bacterial DNA identified in voided urine from a small cross-sectional sample of healthy adults. It additionally provides data giving an insight into the possibility of a “core” urinary microbiome, (which may potentially fluctuate in abundance with age) and provides data consistent with the concept of there being age and sex specific genera. The microbiological methods in current routine urine assessment are unlikely to identify many of these bacteria and certainly not accurately able to enumerate them, necessitating the need for alternative testing systems to be explored in due course. By quantifying the MSU sample with qPCR against an enumerated E. coli sample it has been possible to postulate “contaminant” bacteria as those with very few numbers (<103 cfu/ml or <7 × 103 operons/ml “E. coli equivalent”).
In conclusion, the discoveries evident in this sample data suggest there will be considerable scientific interest in a larger, longitudinal study on the urinary microbiome.
Conflict of Interest Statement
The authors declare that the research was conducted in the absence of any commercial or financial relationships that could be construed as a potential conflict of interest.
Acknowledgments
This work was principally supported by grant awarded from the Bristol Urological Institute Charitable Funds. Debbie A. Lewis was funded jointly by the Bristol Urological Institute and the University of the West of England, Bristol. We thank Adele Long, Jo Worthington, Richard Thompson, Nicola Morris, and Shona Nelson for their assistance in the research. Julian R. Marchesi wishes to acknowledge The Royal Society for funding (RG2010/R2/501628) the HIVE server which was used for the bioinformatic analysis.
References
Arumugam, M., Raes, J., Pelletier, E., Le Paslier, D., Yamada, T., Mende, D. R., et al. (2011). Enterotypes of the human gut microbiome. Nature 473, 174–180. doi: 10.1038/nature09944
Biagi, E., Candela, M., Fairweather-Tait, S., Franchechi, C., and Brigidi, P. (2012). Ageing of the human metaorganism: the microbial counterpart. Age 34, 247–267. doi: 10.1007/s11357-011-9217-5
Chervonsky, A. V. (2012). Intestinal commensals: influence on immune system and tolerance to pathogens. Curr. Opin. Immunol. 24, 255–260. doi: 10.1016/j.coi.2012.03.002
Dave, M., Higgins, P. D. R., Middha, S., and Rioux, K. (2012). The human gut microbiome: current knowledge, challenges, and future directions. Transl. Res. 160, 246–257. doi: 10.1016/j.trsl.2012.05.003
Dethlefsen, L., and Relman, D. A. (2011). Incomplete recovery and individualized responses of the human distal gut microbiota to repeated antibiotic perturbation. Proc. Natl. Acad. Sci. U.S.A. 108, 4554–4561. doi: 10.1073/pnas.1000087107
Dong, Q., Nelson, D. E., Toh, E., Diao, L., Gao, X., Fortenberry, J. D., et al. (2011). The microbial communities in male first catch urine are highly similar to those in paired urethral swab specimens. PLoS ONE 6:e19709. doi: 10.1371/journal.pone.0019709
Durbán, A., Abellán, J. J., Jiménez-Hernández, N., Latorre, A., and Moya, A. (2012). Daily follow-up of bacterial communities in the human gut reveals stable composition and host-specific patterns of Interaction. FEMS Microbiolol. Ecol. 81, 427–437. doi: 10.1111/j.1574-6941.2012.01368.x
Edgar, R. C., Hass, B. J., Clemente, J. C., Quince, C., and Knight, R. (2011). UCHIME improves sensitivity and speed of chimera detection. Bioinformatics 27, 2194–2200. doi: 10.1093/bioinformatics/btr381
Fouts, D. E., Pieper, R., Szpakowski, S., Pohl, H., Knoblach, S., Suh, M.-J., et al. (2012). Intergrated next-generation sequencing of the 16S rDNA and metaproteomics differentiate the healthy urine microbiome from asymptomatic bacteriuria in neuropathic bladder associated with spinal cord injury. J. Transl. Med. 10, 174. doi: 10.1186/1479-5876-10-174
Franz, M., and Hörl, W. H. (1999). Common errors in diagnosis and management of urinary tract infection. I: Pathophysiology and diagnostic techniques. Nephrol. Dial. Transplant. 11, 2746–2753. doi: 10.1093/ndt/14.11.2746
Gruver, A. L., Hudson, L., and Sempowski, G. D. (2007). Immunosenescence of ageing. J. Pathol. 211, 144–156. doi: 10.1002/path.2104
Guarner, F., and Malagelada, J.-R. (2003). Gut flora in health and disease. Lancet 361, 512–519. doi: 10.1016/S0140-6736(03)12489-0
Health Protection Agency. (2012). UK Standards for Microbiology Investigations. Investigation of Urine. B41, Issue no.7. Available online at: http://www.hpa.org.uk/webFile/HPAweb_C/1317132858791 (Accessed 28th, January 2012).
Imirzalioglu, C., Hain, T., Chakraborty, T., and Domann, E. (2008). Hidden pathogens uncovered: metagenomic analysis of urinary tract infections. Andrologia 40, 66–71. doi: 10.1111/j.1439-0272.2007.00830.x
Jalanka-Tuovinen, J., Salonen, A., Nikkilä, J., Immonen, O., Kekkonen, R., Lahti, L., et al. (2011). Intestinal microbiota in healthy adults: temporal analysis reveals individual and common core and relation to intestinal symptoms. PLoS ONE 6:e23035. doi: 10.1371/journal.pone.0023035
Klappenbach, J. A., Saxman, P. R., Cole, J. R., and Schmidt, T. M. (2001). Rrndb: the ribosomal RNA operon copy number database. Nucleic Acid Res. 29, 181–184. doi: 10.1093/nar/29.1.181
Lifshitz, E., and Kramer, L. (2000). Outpatient urine culture – does collection technique matter? Arch. Intern. Med. 160, 2537–2540. doi: 10.1001/archinte.160.16.2537
Marrazzo, J. M. (2011). Interpreting the epidemiology and natural history of bacterial vaginosis: are we still confused? Anaerobe 17, 186–190. doi: 10.1016/j.anaerobe.2011.03.016
Nelson, D. E., Van Der Pol, B., Dong, Q., Revanna, K. V., Fan, B., Easwaran, S., et al. (2010). Characteristic male urine microbiomes associate with asymptomatic sexually transmitted infection. PLoS ONE 5:e14116. doi: 10.1371/journal.pone.0014116
O'Toole, P. W. (2012). Changes in the intestinal microbiota from adulthood through to old age. Clin. Microbiol. Infect. 18(Suppl. 4), 44–46. doi: 10.1111/j.1469-0691.2012.03867.x
Parshina, S. N., Kleerebezem, R., Sanz, J. L., Lettinga, G., Nozhevnikova, A. N., Kostrikina, N. A., et al. (2003). Soehngenia saccharolytica gen. nov., sp. nov. and Clostridium amygdalinum sp. nov., two novel anaerobic, benzaldehyde-converting bacteria. Int. J. Syst. Evol. Micr. 53, 1791–1799. doi: 10.1099/ijs.0.02668-0
Peters, K. M., Killinger, K. A., and Ibrahim, I. A. (2009). Childhood symptoms and events in women with interstitial cystitis/painful bladder syndrome. Urology 73, 258–262. doi: 10.1016/j.urology.2008.09.014
Proctor, L. M. (2011). The human microbiome project in 2011 and beyond. Cell Host Microbe. 10, 287–291. doi: 10.1016/j.chom.2011.10.001
Schloss, P. D., Westcott, S. L., Ryabin, T., Hall, J. R., Hartmann, M., Hollister, E. B., et al. (2009). Introducing mothur: open-source, platform-independent, community-supported software for describing and comparing microbial communities. Appl. Environ. Microbiol. 75, 7537–7541. doi: 10.1128/AEM.01541-09
Semeniuk, H., and Church, D. (1999). Evaluation of the leukocyte esterase and nitrate urine dipstick screening tests for detection of bacteriuria in women with suspected uncomplicated urinary tract infections. J. Clin. Microbiol. 37, 3051–3052.
Siddiqui, H., Nederbragt, A. J., Lagesen, K., Jeansson, S. L., and Jakobsen, K. S. (2011). assessing diversity of the female urine microbiota by high throughput sequencing of 16S rDNA amplicons. BMC Microbiol. 11:244. doi: 10.1186/1471-2180-11-244
Tiihonen, K., Ouwehand, A. C., and Rautonen, N. (2010). Human intestinal microbiota and healthy ageing. Ageing Res. Rev. 9, 107–116. doi: 10.1016/j.arr.2009.10.004
Wilson, M. L., and Gaido, L. (2004). Laboratory diagnosis of urinary tract infections in adult patients. Clin. Infect. Dis. 38, 1150–1158. doi: 10.1086/383029
Wolfe, A. J., Toh, E., Shibata, N., Rong, R., Kenton, K., Fitzgerald., et al. (2012). Evidence of uncultivated bacteria in the adult female bladder. J. Clin. Microbiol. 50, 1376–1383. doi: 10.1128/JCM.05852-11
Yatsunenko, T., Rey, F. E., Manary, M. J., Trehan, I., Dominguez-Bello, M. G., Contreras, M., et al. (2012). Human gut microbiome viewed across age and geography. Nature 486, 222–227.
Appendix
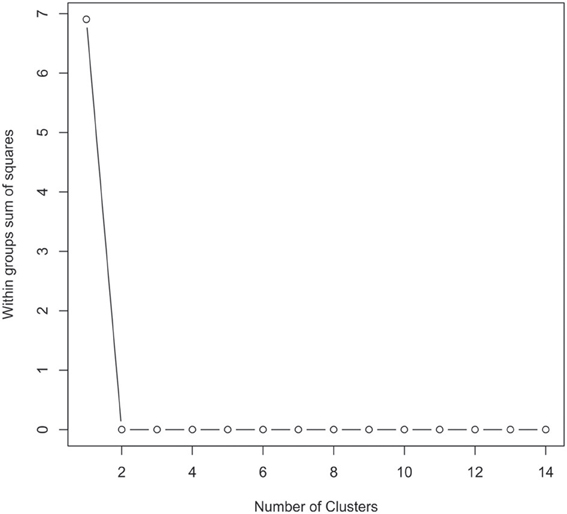
Figure A1. Graphical view of the results of the partitioning around mediods (PAMK in R) used to determine the number of clusters in the urinary microbiome datasets. In this instance two clusters were identified as being the optimal solution.
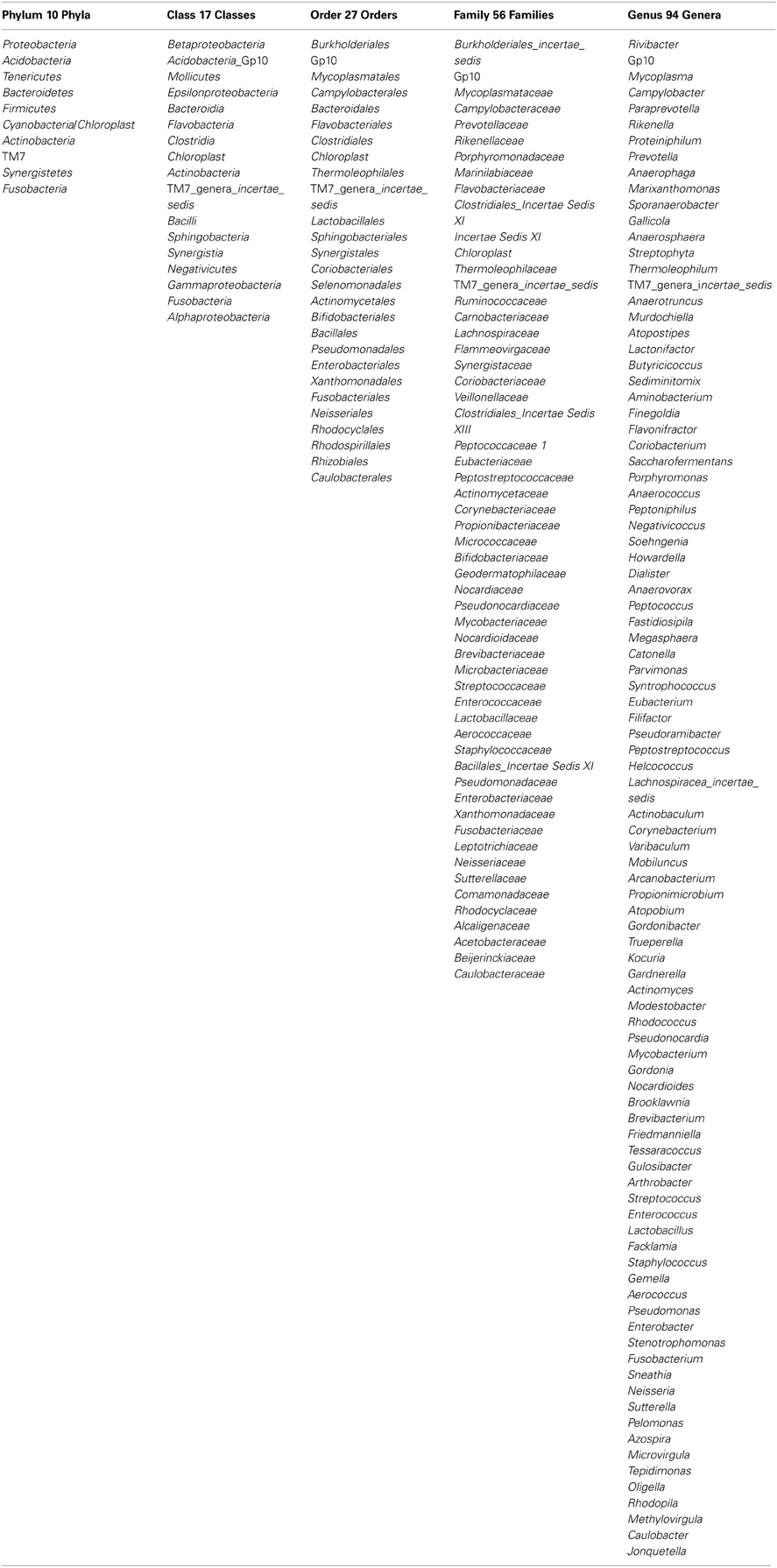
Table A1. The taxonomic groups identified for all the samples at the Phylum, Class, Order, Family, and Genus levels.
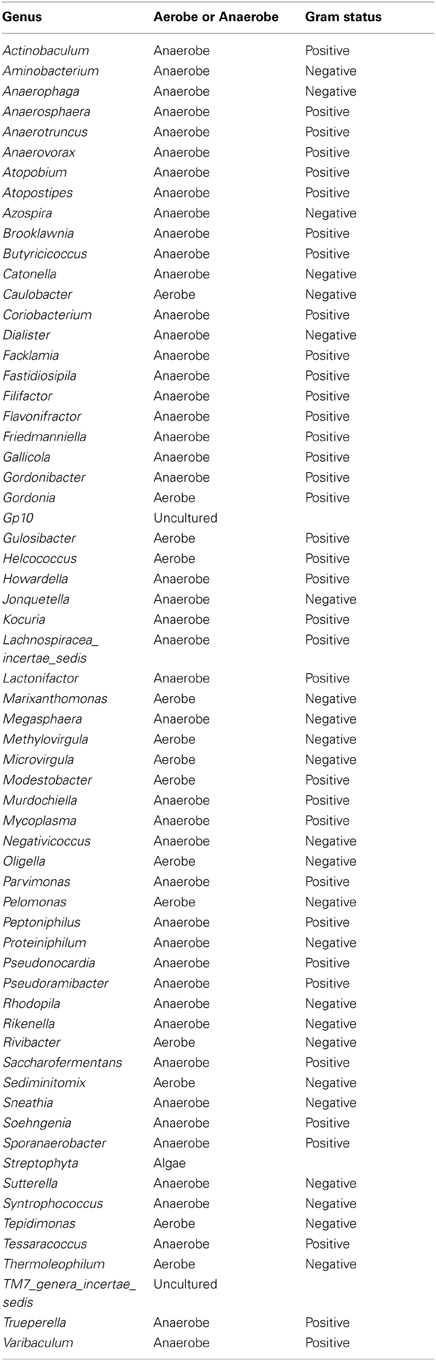
Table A3. Table listing all the bacteria identified in this study that are not routinely cultivated or reported individually by standard methods described by the Health Protection Agency in routine investigations of urine (“Health Protection Agency, 2012”).
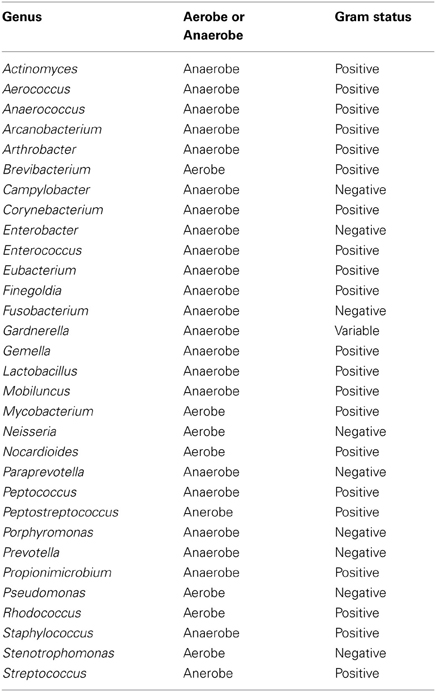
Table A4. Table listing all bacteria identified in this study that can be routinely cultivated and identified individually by standard methods described by the Health Protection Agency in routine investigations of urine (“Health Protection Agency, 2012”).
Keywords: bladder microbiome, pyrosequencing, urinary microbiome, bladder disease, microbiological methods
Citation: Lewis DA, Brown R, Williams J, White P, Jacobson SK, Marchesi JR and Drake MJ (2013) The human urinary microbiome; bacterial DNA in voided urine of asymptomatic adults. Front. Cell. Infect. Microbiol. 3:41. doi: 10.3389/fcimb.2013.00041
Received: 24 February 2013; Accepted: 25 July 2013;
Published online: 15 August 2013.
Edited by:
Alain Stintzi, Ottawa Institute of Systems Biology, CanadaReviewed by:
David Nelson, Indiana University Bloomington, USAValerio Iebba, ‘Sapienza’ University of Rome, Italy
Copyright © 2013 Lewis, Brown, Williams, White, Jacobson, Marchesi and Drake. This is an open-access article distributed under the terms of the Creative Commons Attribution License (CC BY). The use, distribution or reproduction in other forums is permitted, provided the original author(s) or licensor are credited and that the original publication in this journal is cited, in accordance with accepted academic practice. No use, distribution or reproduction is permitted which does not comply with these terms.
*Correspondence: Julian R. Marchesi, School of Biosciences, Cardiff University, Museum Avenue, Cardiff CF10 3AX, UK e-mail:bWFyY2hlc2lqckBjYXJkaWZmLmFjLnVr;
Marcus J. Drake, Bristol Urological Institute, Southmead Hospital, Bristol BS10 5NB, UK e-mail:bWFyY3VzLmRyYWtlQGJ1aS5hYy51aw==