- 1Environmental Science Centre, Qatar University, Doha, Qatar
- 2Department of Meteorology, COMSATS University Islamabad, Islamabad, Pakistan
- 3Department of Statistics, College of Arts and Science, Qatar University, Doha, Qatar
- 4College of Pharmacy, QU Health, Qatar University, Doha, Qatar
- 5Department of Engineering, University of New Brunswick, Saint John, NB, Canada
- 6Department of Building, Civil and Environmental Engineering, Concordia University, Montreal, QC, Canada
IoT-based Sensors networks play a pivotal role in improving air quality monitoring in the Middle East. They provide real-time data, enabling precise tracking of pollution trends, informed decision-making, and increased public awareness. Air quality and dust pollution in the Middle East region may leads to various health issues, particularly among vulnerable populations. IoT-based Sensors networks help mitigate health risks by offering timely and accurate air quality data. Air pollution affects not only human health but also the region’s ecosystems and contributes to climate change. The economic implications of deteriorated air quality include healthcare costs and decreased productivity, underscore the need for effective monitoring and mitigation. IoT-based data can guide policymakers to align with Sustainable Development Goals (SDGs) related to health, clean water, and climate action. The conventional monitor based standard air quality instruments provide limited spatial coverage so there is strong need to continue research integrated with low-cost sensor technologies to make air quality monitoring more accessible, even in resource-constrained regions. IoT-based Sensors networks monitoring helps in understanding these environmental impacts. Among these IoT-based Sensors networks, sensors are of vital importance. With the evolution of sensors technologies, different types of sensors materials are available. Among this carbon based sensors are widely used for air quality monitoring. Carbon nanomaterial-based sensors (CNS) and carbon nanotubes (CNTs) as adsorbents exhibit unique capabilities in the measurement of air pollutants. These sensors are used to detect gaseous pollutants that includes oxides of nitrogen and Sulphur, and ozone, and volatile organic compounds (VOCs). This study provides comprehensive review of integration of carbon nanomaterials based sensors in IoT based network for better air quality monitoring and exploring the potential of machine learning and artificial intelligence for advanced data analysis, pollution source identification, integration of satellite and ground-based networks and future forecasting to design effective mitigation strategies. By prioritizing these recommendations, the Middle East and other regions, can further leverage IoT-based systems to improve air quality monitoring, safeguard public health, protect the environment, and contribute to sustainable development in the region.
1 Introduction
The Middle East, a region known for its historical richness, cultural diversity, and economic significance, is also grappling with a pressing concern that affects the lives of millions of its inhabitants - poor air quality. As the region undergoes rapid urbanization, industrialization, and population growth, the importance of air quality monitoring in the Middle East has never been more evident. The Middle East is the conjunction of expanding urbanization, industrialization and agriculture along with inherited desertification. This provides a homegrown persistent source of air pollution affecting quality of life of residence by aggravating the health issues pertaining to respiratory functions irrespective of gender and age. Fine particulate matter (PM2.5) and pollutants such as nitrogen dioxide (NO2), Ozone (O3) and sulfur dioxide (SO2) pose serious health risks, particularly in densely populated urban areas (Dockery and Pope, 1994).
Vulnerable groups, such as children, the elderly, and individuals with preexisting health conditions, are at higher risk. Air pollution exacerbates respiratory ailments, leading to increased hospitalizations and premature deaths, burdening healthcare systems and affecting the overall wellbeing of communities (Kampa and Castanas, 2008). Beyond human health, air pollution takes a toll on the region’s unique ecosystems. It harms vegetation, disrupts fragile desert ecosystems, and affects biodiversity. Dust storms, a recurring phenomenon in the Middle East, carry pollutants that can travel vast distances, impacting regions far from their source (Awadh, 2023). Air pollutants like black carbon contribute to climate change by accelerating the melting of snow and ice in surrounding regions. As the region faces increasing temperatures and shifting climate patterns, air quality monitoring becomes essential for understanding and mitigating these changes (Lelieveld et al., 2015). Some common gaseous pollutants and PM alongwith their sources have been given in Table 1.
Monitoring air quality in the Middle East is not merely a response to a pressing problem; it is a beacon of hope for the region’s future. The deployment of advanced air quality monitoring networks, often integrated with Internet of Things (IoT) technology, offers several advantages (Alghamdi et al., 2015).
IoT-based sensor networks provide real-time data on air quality, enabling authorities to respond swiftly to pollution events and protect public health. Reliable air quality data informs policy and regulatory decisions, supporting efforts to reduce emissions and improve air quality standards. Accessible air quality information empowers citizens to make informed choices about outdoor activities, reducing personal exposure to pollution. Data collected from monitoring networks drive research on air quality and its effects, fostering innovation in pollution control technologies and sustainable urban planning.
2 Air quality in the middle east
The Middle East faces significant air quality challenges driven by rapid urbanization, industrialization, and natural factors. Air quality issues are exacerbated by unique regional characteristics, including arid climates and increasing dust storms, which introduce additional pollutants into the atmosphere (Goudie, 2014). Dust and fine particulate matter (PM10 and PM2.5) are major pollutants in the Middle East. Sources include natural dust storms, construction activities, and industrial emissions (Nisbet et al., 2019). Traffic emissions, industrial processes, and energy production contribute to elevated NO2 levels in urban areas (Duncan et al., 2016). SO2 emissions arise from industrial activities, including oil refining and power generation (Roy and Sardar, 2015). VOCs are released from transportation, petrochemical industries, and natural sources. They contribute to ozone formation and smog (Siddiqua et al., 2022). Ozone is a secondary pollutant formed through photochemical reactions involving VOCs, NOx, and sunlight.
IoT-based sensor networks offer crucial advantages in addressing air quality challenges in the Middle East. IoT sensors provide real-time data, enabling timely responses to pollution events, especially dust storms (Zheng et al., 2018). Distributed sensor networks offer comprehensive spatial coverage, essential for understanding pollution patterns across cities and regions (Saini et al., 2021). IoT sensors assist in identifying pollution sources, facilitating targeted mitigation efforts. Accessible air quality information empowers citizens to protect their health and advocate for pollution control measures. Reliable IoT-based data informs evidence-based policies and regulatory decisions, critical for improving air quality standards (Saini et al., 2021)
3 Sensors used for air quality monitoring
An air quality monitoring system can be considered comprehensive if it hosts a variety of sensors to monitor the air as well as weather. There is a large list of available options, but few are considered very much indispensable.
Gas sensors detect specific pollutants (e.g., NO2, CO, VOCs) through chemical reactions that change electrical conductivity, resistivity, or optical properties (Zheng et al., 2018). Gas sensors offer high precision and accuracy for targeted gases but may require calibration to maintain accuracy over time. However, regular maintenance and calibration is inevitable for quality assurance (Karagulian et al., 2019). Ozone (O3) is measured through fluctuations in electrical signals due to reactions triggered electrochemically (Gao et al., 2012). Like other sensors, this also demands frequent calibration and maintenance for ensuring quality of data. Temperature and Humidity sensors use thermistors and capacitors to convert changes in electrical flow into temperature and humidity values (Zheng et al., 2018).
Particulate Matter (PM) sensors measure the concentration of airborne particles by capturing and counting particles using light scattering or light blocking techniques (Gao et al., 2015). PM sensors exhibit good accuracy for larger particles (PM10) but may have reduced accuracy for fine particles (PM2.5) due to variability in particle composition (Kelly et al., 2017). Regular maintenance, including cleaning of sensor components and calibration, is necessary to ensure accuracy.
3.1 Carbon based sensors
Advancement in nanotechnology and nanomaterials production has given vital solution in almost every field of life. Among these, development of smart sensor and biosensor has provided the alternatives for conventionally used analyzers and techniques for environmental pollutants sensing that has low sensitivity, high power consumption, and high cost (Alwin David et al., 2022; Gulati et al., 2022). The nanomaterial-based sensors are low cost, handy and have high sensitivity. The chemical sensor comprises of a transducer and an active layer of material that translates chemical information into electronic signal, such as change in frequency, voltage or current. There are different types of gas sensor based on their applications, measurement principle and performance. These are semiconductor, electrochemical, acoustic, catalytic and optical gas sensors. Nanomaterials based chemical sensors for detecting air pollutants are widely used (Gabal et al., 2020; Gulati et al., 2022). These sensors are lower cost monitoring devices that can efficiently measure gases and particle in ambient air. Due to large surface area-to-volume ratios their performance and sensitivity is high and these sensors are able to detect minute quantity of pollutants in air. Nanomaterial receptors include organic materials (polymers, bio-polymers, etc.), inorganic materials (carbon and metals) and hybrid materials. Among these carbon nanomaterials (CNM) have shown significant progress due to their thermal, optical, mechanical and electrochemical properties (Ibrahim et al., 2016; Zhang et al., 2019; Gabal et al., 2020; Dhall et al., 2021). These materials are carbon nanotubes (CNT), nanohorns, graphenes, fullerenes and carbon dots widely used in sensing technologies. Carbon based nanostructures like CNTs and graphene have capacity to detect extremely low concentrations of gases. The schematic diagram of the carbon nanomaterials based sensors if in Figure 1.
3.1.1 Carbon nanotubes (CNTs)
Graphene are extensively used in sensor manufacturing due to excellent performance in detecting variety of gaseous pollutants like VOCs, CO, CH4, NO2, NH3, H2S and SO2 (Mittal and Kumar 2014; Meyyappan, 2016; Schroeder et al., 2019; Zhang et al., 2019; Abdel-Karim et al., 2020; Gabal et al., 2020; Gulati et al., 2022). The structural characteristics of CNTs such as surface area, affinity of pollutants, high porosity, low density and functionalization made them more efficient and reliable. There are different types of CNTs based on their structure and applications.
The carbon atoms arranged in cylindrical tubes with diameters ranging from 1–100 nm, are classified as single-walled (SWCNTs) or multi-walled (MWCNTs) (Sinha and Yeow, 2005; Madani et al., 2013). These hexagonal rings regulate their properties and are synthesized using different methods. The commonly used methods are laser ablation, chemical vapor deposition (CVD) and arc discharge (Beg et al., 2010; Zhang et al., 2019). In the CNT based sensors, gases react at the surface of CNTs and changed sensor resistance that is measured as electrical signal. CNTs sensors provides high sensitivity at ambient temperatures. The combination of metal oxides and CNT further enhance the sensing capacity of the materials gases and these sensors can detect VOC in air. The structure of CNT and grephene is given in Figure 2.
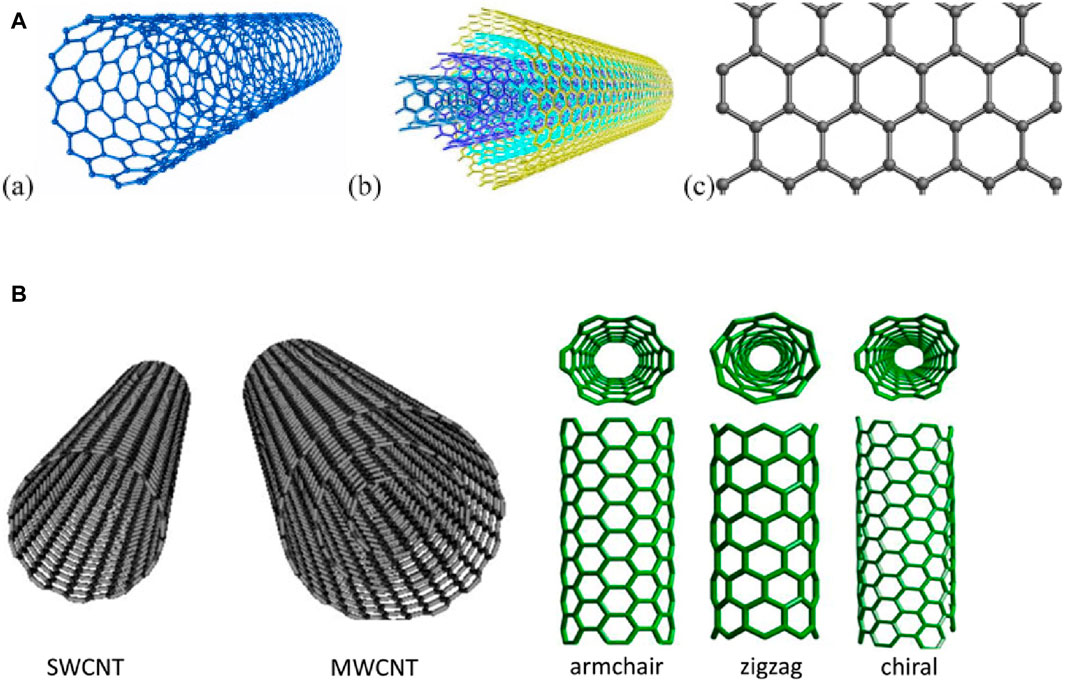
Figure 2. (A) SWCNT, (B) MWCNT and (C) graphene (Ouyang, 2019) (B). Different types of CNT in grey are models of either single-walled or multi-walled CNTs and in green are the various forms of SWCNTs (Madani et al., 2013).
3.1.2 Quantum Dots (QDs)
Inorganic nanoparticles with low fluorescence emission bands. QDs are made of very small number of atoms with distinct energy spectrum. QDs can be used as optical transducers in gas sensors. The flexible wavelength has made QDs more suitable for multi-detection of different analytes (Galstyan, 2021; Alwin David et al., 2022; Kumar et al., 2022; Luo et al., 2022). The sensing behavior of QDs depends upon the structure, composition, and synthesis method. QDs based sensors provides better surface area and charge transfer as compared to conventional devices. Due to their unique structural properties and broad absorption spectra, QDs can be excited by a single energy source. The graphene quantum dots and CdSe, CdSe/ZnS, CdTe, CdTe/CdS, and ZnS based QDs are widely used in sensors (Zhang et al., 2017; Galstyan, 2021; Geng et al., 2021; Lee et al., 2022; Luo et al., 2022). Due to zero-dimensional semiconductor nature of QDs with distinctive electrical and optical properties, QDs are also called “artificial” atoms (Galstyan, 2021; Alwin David et al., 2022; Kumar et al., 2022; Lee et al., 2022). There are two methods for development of semiconductor QDs for chemical gas sensing applications, i.e., epitaxial and colloidal. The ligand formation of the analytes surfaces restrict its reactivity with gases, this process decreases it efficiency, but it can controlled by treating QDs surfaces. The graphene QDs with SnO2 show extraordinary performance and high sensitivity with low detection limit against different gases like NO, CO, CH3COCH3 (Sun et al., 2020; Chen et al., 2021) while QDs with PbS and PbSbS showed excellent behavior against CO (Kumar et al., 2022). There are large number of studies that used QDs some examples of these sensors are given below in Table 2.
3.1.3 Metal oxide nanoparticles sensors (MoS)
Among the other nanomaterial sensors for environmental monitoring and gas sensing technology metal oxide nanoparticles sensors are emerged as exceptional performance. These nanostructures are usually one-dimensional (1D) and revolutionized electrochemical sensing. Likewise, CNT and QDs, MoS high sensitivity and selectivity is due to their surface to volume ratio. MoS based on tin, titanium, iron, zinc and cerium oxides are widely used for gas sensing. These sensors also show extraordinary performance in detecting volatile organic compounds (VOC) present in the air (Zhao and Yang, 2003; Fu and Liao, 2012; Gulati et al., 2022). The titanium dioxide (TiO₂) nanoparticles are usually used in photocatalytic activities but in nano sensor system it has emerged as a vital constituent. In air pollutant detection Tin oxide (SnO₂) is widely used due to its high sensitivity at low temperatures and cost-effectiveness (Dascalu et al., 2018; Shinde et al., 2022). ZnO nanorods exhibit impressive sensitivity to NH₃ in a wide range of temperatures (Tonezzer, 2019; Lupan et al., 2022). Additionally, the molybdenum disulfide (MoS2) modified with reduced graphene oxide (rGO) can also improve the detection sensitivity and selectivity in gas sensors (Niu et al., 2015; Sangeetha and Madhan, 2020; Chen et al., 2021; Rawat et al., 2021; Luo et al., 2022; Liu et al., 2023) provided a metal oxide based sensors framework for air quality monitoring. Summary of some commonly used metal oxide sensors have been given in Table 3.
4 Air quality monitoring networks
A large number of companies are designing and manufacturing sensors for Air quality monitoring using different materials. These sensors are categorized based on their performance, cost effectiveness as well as working conditions. A smart IAQ monitoring network works with on-the-go data transfer from sensors to server on cloud for subsequent IAQ processing and evaluation (Husain et al., 2007; Arar and Jung, 2022; Asghar et al., 2024). IoT based network also comprises of end equipment and communication exchange facilities. These components exchange information autonomously providing users with intellect, intense, and rapid response. A Schematic model of IoT based air quality network consists of user, cloud, internet, mobile network, sensors and data logger or hub/gateway (Figure 3). Although it is financially expensive to use cloud-based services, it can be the backbone for an efficient network dealing with big data analysis at operational level. This also can ensure security of the data with high level of possible encryption because end user interact with the network via variety of the devices such as smartphones, tablets, or laptops. These communication channels can be divided into three tiers of perception, network, and application (Figure 4). Perception tier is the core of the system consisting of air quality and weather sensors, and devices to record the information and send it into network (Gharaibeh et al., 2017). Network tier may consist of any communication protocol or network such cellular, LAN, WAN or wireless to efficiently disseminate the information retrieved from perception tier to user through application tier connecting various applications (Sai et al., 2019; Bousiotis et al., 2021; Buelvas et al., 2023).
4.1 IoT sensors network
IoT is extensively employed in air quality monitoring to gather real-time data from various sensors dispersed across urban areas and remote locations. These systems are instrumental in tracking key air pollutants, including particulate matter (PM), nitrogen dioxide (NO2), sulfur dioxide (SO2), volatile organic compounds (VOCs), and ozone (O3) (Saini et al., 2021).
IoT-based air quality monitoring systems offer several advantages over conventional networks. IoT sensors provide real-time, continuous monitoring of air quality, enabling rapid response to pollution events (Zheng et al., 2018). Distributed sensor networks offer comprehensive spatial coverage, facilitating a detailed understanding of pollution patterns across regions (Saini et al., 2021).
There have been examples of successful implementation of IoT based air quality monitoring networks in the world. China-Beijing’s comprehensive IoT-based air quality monitoring network includes over 35,000 sensors. It provides real-time data to citizens and authorities, enabling effective pollution control measures. London, UK runs the Breathe London project that utilizes IoT sensors to monitor air quality across the city. Data from over 100 fixed sensors and mobile monitoring units inform public health initiatives and policy decisions (Ma, 2021). Delhi - India’s air quality monitoring network relies on IoT sensors to track PM2.5 and PM10 levels. The data aids in issuing health advisories and implementing traffic control measures. Similarly, there are numerous examples of such IoT based networks to get inspired.
4.2 Data analysis and visualization
Collected IoT sensor data undergoes several stages of analysis and processing such as Preprocessing, Extraction, Modeling, and lastly Visualization. Raw data is cleaned, filtered, and transformed to remove noise and outliers, ensuring data quality (Khosravi et al., 2011). Relevant features are extracted from the data, which can include statistical measures, trends, or anomalies (Khosravi et al., 2011). Data is used to build models or algorithms that can predict future trends, detect anomalies, or provide insights (Chen et al., 2014). Data visualization plays a crucial role in making complex IoT data understandable and actionable. Line graphs, bar charts, and scatter plots are commonly used to visualize time-series data and relationships between variables (Hassan et al., 2020). Heat maps help represent data density and patterns, making it easier to identify hotspots or trends in spatial data. Mapping IoT data onto geographic information systems (GIS) helps visualize spatial trends and relationships.
Real-time reporting of IoT data is crucial for proactive and timely decision making. Real-time reporting enables immediate actions in response to critical events or anomalies, such as pollution spikes or security breaches (Bales et al., 2014). Real time reporting also brings in proactive maintenance allowing predictive maintenance, reducing downtime and maintenance costs for IoT devices and systems (Him et al., 2019). Most importantly real time reporting of an IoT network increases public awareness and empowers the public with up-to-the-minute information on air quality, traffic, and other factors that impact daily life.
5 Air quality monitoring sensors networks in Middle Eastern countries
Geopolitical and socioeconomic importance of Middle East merits the sustainable existence of IoT based air quality monitoring networks in the region to work synergistically for equally good air quality of the region. Regionally integrated air quality monitoring networks will serve several stakeholders related to departments ranging from educational, research, policy, health, environment, tourism and many more. There are already a few successful examples of such IoT based air quality networks in the Middle East (Table 4). These networks play a vital role in assessing and managing air quality in the Middle East, helping to protect public health and the environment while informing policy decisions and regulatory actions.
Countries in the initial stages of implementing air quality networks at regional level must understand the responsibility and roles of the important stakeholders such as Government Agencies, Research Institutions, Environmental Organizations and public. Government agencies are often responsible for establishing and maintaining monitoring infrastructure, setting air quality standards, and regulating emissions. Research institutions contribute to data collection, analysis, and research on air quality, helping to improve monitoring techniques and policy recommendations (Hassan et al., 2020). Environmental organizations advocate for air quality improvements, raise public awareness, and sometimes operate independent monitoring stations (Sarigiannis et al., 2011). The role of the public is of the utmost importance to reach a sustainable clean environment reflected from their actions and awareness. Despite the importance of collaboration, several challenges hinder effective data sharing and cooperation in the Middle East which is linked to the unawareness or lack of understanding of the roles of stakeholders which usually leads to the limited accessibility to air quality data due to proprietary ownership, lack of standardized formats, and data restrictions by some stakeholders (Buelvas et al., 2023; Srishti et al., 2023). Data recorded by diverse sources often faces issues of interoperability when it converges in a network. Variability in data formats, measurement units, and quality control procedures among different monitoring stations hampers data integration and analysis (Din et al., 2019; Hassan et al., 2020). There is a lack of regulatory harmonization among the Middle Eastern countries due to several reasons. For instance, disparities in air quality regulations among Middle Eastern countries hinder regional cooperation and consistency in addressing cross-border pollution (Sarigiannis et al., 2011; Sarigiannis et al., 2017). Further, limited resources and funding for monitoring infrastructure and research impede the expansion and maintenance of monitoring networks.
The Middle East can consider Dubai Air Quality Monitoring Network (DAQMN) as an example to follow for establishing an air quality network embedded with IoT. DAQMN can be looked up as an air quality network covering various types of possible air pollution sources from urban, rural, industrial and natural. DAQMN is promoted as transparent source of information to both public and policymakers through real time dissemination of information through web and mobile platforms (Environment, 2024).
6 Effectiveness of IoT-based monitoring
IoT-based systems have brought significant advancements to air quality monitoring in the Middle East, leading to a deeper understanding of pollution trends, notable improvements, and positive changes in public awareness and policies. IoT-based systems provide real-time and precise data on air quality, allowing for the identification of pollution hotspots and the sources of contaminants (Him et al., 2019; Sai et al., 2019; Bousiotis et al., 2021; Saini et al., 2021; Buelvas et al., 2023; Asghar et al., 2024). Continuous monitoring has revealed seasonal and diurnal pollution patterns, aiding in the development of targeted mitigation strategies (Chen et al., 2015; Ammar et al., 2018; Din et al., 2019; Hassan et al., 2020; Saini et al., 2021; Saini et al., 2021; Buelvas et al., 2023). The data-driven approach has enabled governments to measure the effectiveness of pollution control measures and adapt policies accordingly.
Accessible air quality information through IoT platforms has empowered the Middle Eastern public to make informed decisions about outdoor activities and reduce personal exposure to pollution. Public pressure, driven by increased awareness, has catalyzed policy changes, leading to stricter emissions regulations, increased green initiatives, and support for cleaner transportation options (Zanella et al., 2014; Chen et al., 2015; Ammar et al., 2018; Čolaković and Hadžialić, 2018; Hassan et al., 2020; Saini et al., 2021; Kausar et al., 2023). IoT-based data has become integral to policy development, enabling evidence-based decision-making and fostering international cooperation in addressing air quality challenges (Sarigiannis et al., 2011; Sarigiannis et al., 2017).
7 Challenges and future directions
Implementing IoT-based systems for air quality monitoring in the Middle East faces several challenges such as infrastructure constraints, data privacy and security, and interoperability. Limited infrastructure in some areas may hinder the deployment of monitoring networks, particularly in remote or underdeveloped regions (Abomhara and Køien, 2014). Ensuring data privacy and security is crucial, given concerns related to data breaches and misuse of sensitive information (Miorandi et al., 2012). The lack of standardized protocols and data formats can hinder interoperability between different monitoring systems and devices (Al-Fuqaha et al., 2015). However, to overcome these challenges governments must invest in infrastructure development, including the expansion of wireless networks and power supply. This can facilitate the deployment of IoT-based systems in remote areas (Bales et al., 2014). To make this network sustainable, robust data encryption and access control mechanisms should be in place to protect sensitive information, addressing data privacy and security concerns (Zheng et al., 2018). Most importantly, a Regional Spatial Data Infrastructure (RSDI) or other data sharing protocols are necessary to capitalize the use of collected data from IoT network (Miorandi et al., 2012).
Use and development of low-cost air quality monitoring sensors should be encouraged at policy level to achieve high spatial and temporal coverage (Margaritis et al., 2021; Bousiotis et al., 2023; Buelvas et al., 2023; Nobell et al., 2023). Similarly, the use of artificial intelligence-based algorithms should be encouraged to process the collected data. This will enable real time availability of accurate reliable data for enhancing the scientific understanding of air pollution trends and sources for effective policy making (Chen et al., 2014).
8 Conclusion
An integrated air quality monitoring network based on Nanomaterial based sensors is an indispensable element for research, teaching, awareness and policy making to produce knowledgeable decisions in the vast interest of public of Middle East. Air quality in the Middle East is strongly impacted by dust. The following recommendations has been made based on this study.
➢ Integrated IoT-based sensor network systems with conventional air quality monitoring network will increase geospatial data coverage that will leads to better air quality management.
➢ The choice of sensor depends on factors such as the specific gases or particles to be detected, desired sensitivity and selectivity, environmental conditions, cost considerations, and integration requirements. Therefore, a comprehensive evaluation considering these factors is necessary to determine the most suitable sensor for a particular application.
➢ IoT-based monitoring will enhance understanding the impact of air quality of region’s ecosystems, environmental and climate change impacts.
➢ IoT-based data guides policy changes, aligning with Sustainable Development Goals (SDGs) related to health, clean water, and climate action.
➢ The use of new techniques like machine learning and artificial intelligence for advanced data analysis will improve pollution source identification and development of early warning system for extreme weather events like dust storms.
➢ The integration of satellite and UAV-based sensing technologies will complement ground-based networks and boost understanding of the pollution sources
➢ To tackle transboundary pollution sources within the region standardization of data formats and unified protocols are required to enhance data interoperability and collaboration.
➢ Public awareness and participation in air quality monitoring efforts is vital to foster responsible behaviors.
By prioritizing these recommendations, the Middle East can further leverage IoT-based systems to improve air quality monitoring, safeguard public health, protect the environment, and contribute to sustainable development in the region.
Author contributions
IS: Conceptualization, Data curation, Funding acquisition, Methodology, Project administration, Writing–original draft, Writing–review and editing. MS: Investigation, Methodology, Writing–original draft. ET: Formal Analysis, Methodology, Writing–review and editing. MK: Investigation, Methodology, Writing–review and editing. MA: Data curation, Formal Analysis, Investigation, Writing–original draft. SA: Formal Analysis, Validation, Visualization, Writing–review and editing. HR: Investigation, Methodology, Writing–review and editing, Validation. ZA: Methodology, Visualization, Writing–review and editing. ZC: Conceptualization, Validation, Visualization, Writing–review and editing.
Funding
The author(s) declare that financial support was received for the research, authorship, and/or publication of this article. The funding for this work is provided by Qatar University by IRCC grant # IRCC-2023-152.
Conflict of interest
The authors declare that the research was conducted in the absence of any commercial or financial relationships that could be construed as a potential conflict of interest.
Publisher’s note
All claims expressed in this article are solely those of the authors and do not necessarily represent those of their affiliated organizations, or those of the publisher, the editors and the reviewers. Any product that may be evaluated in this article, or claim that may be made by its manufacturer, is not guaranteed or endorsed by the publisher.
References
Abdel-Karim, R., Reda, Y., and Abdel-Fattah, A. (2020). Review—nanostructured materials-based nanosensors. J. Electrochem. Soc. 167 (3), 037554. doi:10.1149/1945-7111/ab67aa
Abomhara, M., and Køien, G. M. (2014). “Security and privacy in the Internet of Things: current status and open issues,” in 2014 international conference on privacy and security in mobile systems (PRISMS), USA, 11-14 May 2014 (IEEE).
Al-Fuqaha, A., Guizani, M., Mohammadi, M., Aledhari, M., and Ayyash, M. (2015). Internet of things: a survey on enabling technologies, protocols, and applications. IEEE Commun. Surv. tutorials 17 (4), 2347–2376. doi:10.1109/comst.2015.2444095
Alghamdi, M. A., Almazroui, M., Shamy, M., Redal, M. A., Alkhalaf, A. K., Hussein, M. A., et al. (2015). Characterization and elemental composition of atmospheric aerosol loads during springtime dust storm in western Saudi Arabia. Aerosol Air Qual. Res. 15 (2), 440–453. doi:10.4209/aaqr.2014.06.0110
Alwin David, S., Rajkumar, R., Karpagavinayagam, P., Fernando, J., and Vedhi, C. (2022). Chapter 25 - sustainable carbon nanomaterial-based sensors: future vision for the next 20years. Carbon Nanomaterials-Based Sensors. Elsevier, 429–443. doi:10.1016/B978-0-323-91174-0.00011-1
Ammar, M., Russello, G., and Crispo, B. (2018). Internet of Things: a survey on the security of IoT frameworks. J. Inf. Secur. Appl. 38, 8–27. doi:10.1016/j.jisa.2017.11.002
Arar, M., and Jung, C. (2022). Analyzing the perception of indoor air quality (IAQ) from a survey of new townhouse residents in Dubai. Sustainability 14 (22), 15042. doi:10.3390/su142215042
Asghar, N., Hussain, A., Nguyen, D. A., Ali, S., Hussain, I., Junejo, A., et al. (2024). Advancement in nanomaterials for environmental pollutants remediation: a systematic review on bibliometrics analysis, material types, synthesis pathways, and related mechanisms. J. Nanobiotechnology 22 (1), 26. doi:10.1186/s12951-023-02151-3
Awadh, S. M. (2023). Impact of North African sand and dust storms on the Middle East using Iraq as an example: causes, sources, and mitigation. Atmosphere 14 (1), 180. doi:10.3390/atmos14010180
Bales, E., Nikzad, N., Ziftci, C., Quick, N., Griswold, W., and Patrick, K. (2014) Personal pollution monitoring: mobile real-time air-quality in daily life.
Beg, S., Rizwan, M., Sheikh, A. M., Hasnain, M. S., Anwer, K., and Kohli, K. (2010). Advancement in carbon nanotubes: basics, biomedical applications and toxicity. J. Pharm. Pharmacol. 63 (2), 141–163. doi:10.1111/j.2042-7158.2010.01167.x
Bousiotis, D., Allison, G., Beddows, D. C. S., Harrison, R. M., and Pope, F. D. (2023). Towards comprehensive air quality management using low-cost sensors for pollution source apportionment. npj Clim. Atmos. Sci. 6 (1), 122. doi:10.1038/s41612-023-00424-0
Bousiotis, D., Singh, A., Haugen, M., Beddows, D. C. S., Diez, S., Murphy, K. L., et al. (2021). Assessing the sources of particles at an urban background site using both regulatory instruments and low-cost sensors – a comparative study. Atmos. Meas. Tech. 14 (6), 4139–4155. doi:10.5194/amt-14-4139-2021
Buelvas, J., Múnera, D., Tobón V, D. P., Aguirre, J., and Gaviria, N. (2023). Data quality in IoT-based air quality monitoring systems: a systematic mapping study. Water, Air, and Soil Pollut. 234 (4), 248. doi:10.1007/s11270-023-06127-9
Chen, F., Deng, P., Wan, J., Zhang, D., Vasilakos, A. V., and Rong, X. (2015). Data mining for the internet of things: literature review and challenges. Int. J. Distributed Sens. Netw. 11 (8), 431047. doi:10.1155/2015/431047
Chen, M., Mao, S., and Liu, Y. (2014). Big data: a survey. Mob. Netw. Appl. 19, 171–209. doi:10.1007/s11036-013-0489-0
Chen, X., Behboodian, R., Bagnall, D., Taheri, M., and Nasiri, N. (2021). Metal-organic-Frameworks: low temperature gas sensing and air quality monitoring. Chemosensors 9, 316. doi:10.3390/chemosensors9110316
Chen, Z.-L., Wang, D., Wang, X.-Y., and Yang, J.-H. (2021). Enhanced formaldehyde sensitivity of two-dimensional mesoporous SnO2 by nitrogen-doped graphene quantum dots. Rare Met. 40 (6), 1561–1570. doi:10.1007/s12598-020-01636-6
Chirmata, A., Leghrib, R., and Ichou, I. A. (2017). Implementation of the air quality monitoring network at Agadir City in Morocco. J. Environ. Prot. 8 (4), 540–567. doi:10.4236/jep.2017.84037
Čolaković, A., and Hadžialić, M. (2018). Internet of Things (IoT): a review of enabling technologies, challenges, and open research issues. Comput. Netw. 144, 17–39. doi:10.1016/j.comnet.2018.07.017
Dascalu, I., Somacescu, S., Hornoiu, C., Calderon-Moreno, J. M., Stanica, N., Stroescu, H., et al. (2018). Sol-gel Zn, Fe modified SnO2 powders for CO sensors and magnetic applications. Process Saf. Environ. Prot. 117, 722–729. doi:10.1016/j.psep.2018.06.010
Dhall, S., Mehta, B. R., Tyagi, A. K., and Sood, K. (2021). A review on environmental gas sensors: materials and technologies. Sensors Int. 2, 100116. doi:10.1016/j.sintl.2021.100116
Din, I. U., Guizani, M., Hassan, S., Kim, B. S., Khan, M. K., Atiquzzaman, M., et al. (2019). The internet of things: a review of enabled technologies and future challenges. IEEE Access 7, 7606–7640. doi:10.1109/access.2018.2886601
Dockery, D. W., and Pope, C. A. (1994). Acute respiratory effects of particulate air pollution. Annu. Rev. public health 15 (1), 107–132. doi:10.1146/annurev.publhealth.15.1.107
Duncan, B. N., Lamsal, L. N., Thompson, A. M., Yoshida, Y., Lu, Z., Streets, D. G., et al. (2016). A space-based, high-resolution view of notable changes in urban NOx pollution around the world (2005–2014). J. Geophys. Res. Atmos. 121 (2), 976–996. doi:10.1002/2015jd024121
Fezari, M., Boumaza, M. S., Al-Dahoud, A., and Al-Dahoud, A. (2015). “WSN for AIR quality monitoring in annaba city,” in Proceedings of the 7th International Conference on Information Technology ICIT, Amman, Jordan, 24-25 Sept. 2020 (IEEE).
Fu, Y., and Liao, H. (2012). Simulation of the interannual variations of biogenic emissions of volatile organic compounds in China: impacts on tropospheric ozone and secondary organic aerosol. Atmos. Environ. 59, 170–185. doi:10.1016/j.atmosenv.2012.05.053
Gabal, E., Chatterjee, S., Ahmed, F. K., and Abd-Elsalam, K. A. (2020). “7 - carbon nanomaterial applications in air pollution remediation,” in Carbon nanomaterials for agri-food and environmental applications. K. A. Abd-elsalam (China: Elsevier), 133–153.
Galstyan, V. (2021). “Quantum dots: perspectives in next-generation chemical gas sensors” ‒ A review. Anal. Chim. Acta 1152, 238192. doi:10.1016/j.aca.2020.12.067
Gao, M., Cao, J., and Seto, E. (2015). A distributed network of low-cost continuous reading sensors to measure spatiotemporal variations of PM2. 5 in Xi'an, China. Environ. Pollut. 199, 56–65. doi:10.1016/j.envpol.2015.01.013
Gao, R., Ballard, J., Watts, L., Thornberry, T., Ciciora, S., McLaughlin, R., et al. (2012). A compact, fast UV photometer for measurement of ozone from research aircraft. Atmos. Meas. Tech. 5 (9), 2201–2210. doi:10.5194/amt-5-2201-2012
Geng, X., Li, S., Mawella-Vithanage, L., Ma, T., Kilani, M., Wang, B., et al. (2021). Atomically dispersed Pb ionic sites in PbCdSe quantum dot gels enhance room-temperature NO2 sensing. Nat. Commun. 12 (1), 4895. doi:10.1038/s41467-021-25192-4
Gharaibeh, A., Salahuddin, M. A., Hussini, S. J., Khreishah, A., Khalil, I., Guizani, M., et al. (2017). Smart cities: a survey on data management, security, and enabling technologies. IEEE Commun. Surv. Tutorials 19 (4), 2456–2501. doi:10.1109/comst.2017.2736886
Goudie, A. S. (2014). Desert dust and human health disorders. Environ. Int. 63, 101–113. doi:10.1016/j.envint.2013.10.011
Gulati, S., Lingam B, H. N., Kumar, S., Goyal, K., Arora, A., and Varma, R. S. (2022). Improving the air quality with Functionalized Carbon Nanotubes: sensing and remediation applications in the real world. Chemosphere 299, 134468. doi:10.1016/j.chemosphere.2022.134468
Haiduk, Y., Khort, A., Lapitskaya, V., Kuznetsova, T., Moskovskikh, D., Savitsky, A., et al. (2022). WO3–graphene–Cu nanocomposites for CO, NO2 and acetone gas sensors. Nano-Structures Nano-Objects 29, 100824. doi:10.1016/j.nanoso.2021.100824
Hassan, R., Qamar, F., Hasan, M. K., Aman, A. H. M., and Ahmed, A. S. (2020). Internet of Things and its applications: a comprehensive survey. Symmetry 12 (10), 1674. doi:10.3390/sym12101674
Him, L. C., Poh, Y. Y., and Pheng, L. W. (2019). “Iot-based predictive maintenance for smart manufacturing systems,” in 2019 Asia-Pacific Signal and Information Processing Association Annual Summit and Conference (APSIPA ASC), USA, 18-21 Nov. 2019 (IEEE).
Howes, J., and Abdel-Rehiem, A. (2012). “Powering an EGYPTIAN air quality information. geoENV III—geostatistics for environmental applications,” in Proceedings of the Third European Conference on Geostatistics for Environmental Applications held in Avignon, France, November 22–24, 2000, France, November 22–24, 2000 (Springer Science and Business Media).
Husain, L., Dutkiewicz, V. A., Khan, A. J., and Ghauri, B. M. (2007). Characterization of carbonaceous aerosols in urban air. Atmos. Environ. 41 (32), 6872–6883. doi:10.1016/j.atmosenv.2007.04.037
Ibrahim, R. K., Hayyan, M., AlSaadi, M. A., Hayyan, A., and Ibrahim, S. (2016). Environmental application of nanotechnology: air, soil, and water. Environ. Sci. Pollut. Res. 23 (14), 13754–13788. doi:10.1007/s11356-016-6457-z
Isaac, N. A., Pikaar, I., and Biskos, G. (2022). Metal oxide semiconducting nanomaterials for air quality gas sensors: operating principles, performance, and synthesis techniques. Microchim. Acta 189 (5), 196. doi:10.1007/s00604-022-05254-0
Jassim, M. S., and Coskuner, G. (2017). Assessment of spatial variations of particulate matter (PM 10 and PM 2.5) in Bahrain identified by air quality index (AQI). Arabian J. Geosciences 10, 19–14. doi:10.1007/s12517-016-2808-9
Kampa, M., and Castanas, E. (2008). Human health effects of air pollution. Environ. Pollut. 151 (2), 362–367. doi:10.1016/j.envpol.2007.06.012
Karagulian, F., Barbiere, M., Kotsev, A., Spinelle, L., Gerboles, M., Lagler, F., et al. (2019). Review of the performance of low-cost sensors for air quality monitoring. Atmosphere 10 (9), 506. doi:10.3390/atmos10090506
Kausar, A. I., Ahmad, T., Zhu, H., Shahzad, H., and M. H. Eisa, (2023). "Exigency for the control and upgradation of indoor air quality—forefront advancements using nanomaterials.". Pollutants 3, 123–149. doi:10.3390/pollutants3010011
Kelly, K., Whitaker, J., Petty, A., Widmer, C., Dybwad, A., Sleeth, D., et al. (2017). Ambient and laboratory evaluation of a low-cost particulate matter sensor. Environ. Pollut. 221, 491–500. doi:10.1016/j.envpol.2016.12.039
Khosravi, A., Nahavandi, S., Creighton, D., and Atiya, A. F. (2011). Comprehensive review of neural network-based prediction intervals and new advances. IEEE Trans. neural Netw. 22 (9), 1341–1356. doi:10.1109/tnn.2011.2162110
Kumar, U., Yang, Y.-H., Deng, Z.-Y., Lee, M.-W., Huang, W.-M., and Wu, C.-H. (2022). In situ growth of ternary metal sulfide based quantum dots to detect dual gas at extremely low levels with theoretical investigations. Sensors Actuators B Chem. 353, 131192. doi:10.1016/j.snb.2021.131192
Lee, K. S., Shim, J., Lee, J. S., Lee, J., Moon, H. G., Park, Y. J., et al. (2022). Adsorption behavior of NO2 molecules in ZnO-mono/multilayer graphene core–shell quantum dots for NO2 gas sensor. J. Industrial Eng. Chem. 106, 279–286. doi:10.1016/j.jiec.2021.11.003
Lelieveld, J., Evans, J. S., Fnais, M., Giannadaki, D., and Pozzer, A. (2015). The contribution of outdoor air pollution sources to premature mortality on a global scale. Nature 525 (7569), 367–371. doi:10.1038/nature15371
Li, H., Li, M., Kan, H., Li, C., Quan, A., Fu, C., et al. (2019). Surface acoustic wave NO2 sensors utilizing colloidal SnS quantum dot thin films. Surf. Coatings Technol. 362, 78–83. doi:10.1016/j.surfcoat.2019.01.100
Li, Q., Huang, N., Cui, Y., Lin, J., Zhao, C., and Ding, L. (2022). Synthesis of porous rod-like In2O3 nanomaterials and its selective detection of NO at room temperature. J. Alloys Compd. 902, 163632. doi:10.1016/j.jallcom.2022.163632
Liu, H., Zhang, S., Cheng, Q., Wang, L., and Wang, S. (2023). A mini review on the recent progress of MoS2-based gas sensors. Catal. Lett. 154, 1375–1384. doi:10.1007/s10562-023-04436-6
Luo, J., Li, C., Yang, Q., Yan, L., Zhang, B., Tao, R., et al. (2022). Facile fabrication of MoS2 nanoflowers/SnO2 colloidal quantum dots nanocomposite for enhanced NO2 sensing at room temperature. IEEE Sensors J. 22 (7), 6295–6302. doi:10.1109/jsen.2022.3151068
Lupan, C., Mishra, A. K., Wolff, N., Drewes, J., Krüger, H., Vahl, A., et al. (2022). Nanosensors based on a single ZnO:Eu nanowire for hydrogen gas sensing. ACS Appl. Mater. Interfaces 14 (36), 41196–41207. doi:10.1021/acsami.2c10975
Ma, Q. (2021) New insights into understanding urban traffic emissions using novel mobile air quality measurements in the Breathe London pilot study. Cambridge: University of.
Madani, S. Y., Mandel, A., and Seifalian, A. M. (2013). A concise review of carbon nanotube's toxicology. Nano Rev. 4 (1), 21521. doi:10.3402/nano.v4i0.21521
Margaritis, D., Keramydas, C., Papachristos, I., and Lambropoulou, D. (2021). Calibration of low-cost gas sensors for air quality monitoring. Aerosol Air Qual. Res. 21 (11), 210073. doi:10.4209/aaqr.210073
Meyyappan, M. (2016). Carbon nanotube-based chemical sensors. Small 12 (16), 2118–2129. doi:10.1002/smll.201502555
Miorandi, D., Sicari, S., De Pellegrini, F., and Chlamtac, I. (2012). Internet of things: vision, applications and research challenges. Ad hoc Netw. 10 (7), 1497–1516. doi:10.1016/j.adhoc.2012.02.016
Mittal, M., and Kumar, A. (2014). Carbon nanotube (CNT) gas sensors for emissions from fossil fuel burning. Sensors Actuators B Chem. 203, 349–362. doi:10.1016/j.snb.2014.05.080
Mofarrah, A., and Husain, T. (2010). A holistic approach for optimal design of air quality monitoring network expansion in an urban area. Atmos. Environ. 44 (3), 432–440. doi:10.1016/j.atmosenv.2009.07.045
Nisbet, E. G., Manning, M., Dlugokencky, E., Fisher, R., Lowry, D., Michel, S., et al. (2019). Very strong atmospheric methane growth in the 4 years 2014–2017: implications for the Paris Agreement. Glob. Biogeochem. Cycles 33 (3), 318–342. doi:10.1029/2018gb006009
Niu, Y., Wang, R., Jiao, W., Ding, G., Hao, L., Yang, F., et al. (2015). MoS2 graphene fiber based gas sensing devices. Carbon 95, 34–41. doi:10.1016/j.carbon.2015.08.002
Nobell, S., Majumdar, A., Mukherjee, S., Chakraborty, S., Chatterjee, S., Bose, S., et al. (2023). Validation of in-field calibration for low-cost sensors measuring ambient particulate matter in Kolkata, India. Aerosol Air Qual. Res. 23 (11), 230010. doi:10.4209/aaqr.230010
Omidvarborna, H., Baawain, M., and Al-Mamun, A. (2018). Ambient air quality and exposure assessment study of the Gulf Cooperation Council countries: a critical review. Sci. Total Environ. 636, 437–448. doi:10.1016/j.scitotenv.2018.04.296
Ouyang, J. (2019). Applications of carbon nanotubes and graphene for third-generation solar cells and fuel cells. Nano Mater. Sci. 1 (2), 77–90. doi:10.1016/j.nanoms.2019.03.004
Patel, C., Mandal, B., Jadhav, R. G., Ghosh, T., Dubey, M., Das, A. K., et al. (2022). S, N Co-doped carbon dot-functionalized WO3 nanostructures for NO2 and H2S detection. ACS Appl. Nano Mater. 5 (2), 2492–2500. doi:10.1021/acsanm.1c04174
Rawat, S., Bamola, P., Dwivedi, C., and Sharma, H. (2021). Two dimensional MoS2 gas sensor to detect carbon monoxide (CO). Mater. Today Proc. 45, 4841–4843. doi:10.1016/j.matpr.2021.01.297
Roy, P., and Sardar, A. (2015). SO2 emission control and finding a way out to produce sulphuric acid from industrial SO2 emission. J. Chem. Eng. Process Technol. 6 (2), 1000230. doi:10.4172/2157-7048.1000230
Sai, K. B. K., Subbareddy, S. R., and Luhach, A. K. (2019). IOT based air quality monitoring system using MQ135 and MQ7 with machine learning analysis. Scalable Comput. Pract. Exp. 20 (4), 599–606. doi:10.12694/scpe.v20i4.1561
Saini, J., Dutta, M., and Marques, G. (2021). Sensors for indoor air quality monitoring and assessment through Internet of Things: a systematic review. Environ. Monit. Assess. 193 (2), 66. doi:10.1007/s10661-020-08781-6
Sangeetha, M., and Madhan, D. (2020). Ultra sensitive molybdenum disulfide (MoS2)/graphene based hybrid sensor for the detection of NO2 and formaldehyde gases by fiber optic clad modified method. Opt. Laser Technol. 127, 106193. doi:10.1016/j.optlastec.2020.106193
Sarigiannis, D. A., Karakitsios, S. P., Gotti, A., Liakos, I. L., and Katsoyiannis, A. (2011). Exposure to major volatile organic compounds and carbonyls in European indoor environments and associated health risk. Environ. Int. 37 (4), 743–765. doi:10.1016/j.envint.2011.01.005
Sarigiannis, D. A., Kontoroupis, P., Nikolaki, S., Gotti, A., Chapizanis, D., and Karakitsios, S. (2017). Benefits on public health from transport-related greenhouse gas mitigation policies in Southeastern European cities. Sci. Total Environ. 579, 1427–1438. doi:10.1016/j.scitotenv.2016.11.142
Schroeder, V., Savagatrup, S., He, M., Lin, S., and Swager, T. M. (2019). Carbon nanotube chemical sensors. Chem. Rev. 119 (1), 599–663. doi:10.1021/acs.chemrev.8b00340
Shinde, R. S., Khairnar, S. D., Patil, M. R., Adole, V. A., Koli, P. B., Deshmane, V. V., et al. (2022). Synthesis and characterization of ZnO/CuO nanocomposites as an effective photocatalyst and gas sensor for environmental remediation. J. Inorg. Organomet. Polym. Mater. 32 (3), 1045–1066. doi:10.1007/s10904-021-02178-9
Siddiqua, A., Hahladakis, J. N., and Al-Attiya, W. A. K. (2022). An overview of the environmental pollution and health effects associated with waste landfilling and open dumping. Environ. Sci. Pollut. Res. 29 (39), 58514–58536. doi:10.1007/s11356-022-21578-z
Sinha, N., and Yeow, J. T. W. (2005). Carbon nanotubes for biomedical applications. IEEE Trans. NanoBioscience 4 (2), 180–195. doi:10.1109/tnb.2005.850478
Srishti, S., Agrawal, P., Kulkarni, P., Gautam, H. C., Kushwaha, M., and Sreekanth, V. (2023). Multiple PM low-cost sensors, multiple seasons’ data, and multiple calibration models. Aerosol Air Qual. Res. 23 (3), 220428. doi:10.4209/aaqr.220428
Sun, Y., Yang, H., Zhao, Z., Suematsu, K., Li, P., Yu, Z., et al. (2020). Fabrication of ZnO quantum dots@SnO2 hollow nanospheres hybrid hierarchical structures for effectively detecting formaldehyde. Sensors Actuators B Chem. 318, 128222. doi:10.1016/j.snb.2020.128222
Tonezzer, M. (2019). Selective gas sensor based on one single SnO2 nanowire. Sensors Actuators B Chem. 288, 53–59. doi:10.1016/j.snb.2019.02.096
Wang, Y., Liu, L., Sun, F., Li, T., Zhang, T., and Qin, S. (2021). Humidity-insensitive NO2 sensors based on SnO2/rGO composites. Front. Chem. 9, 681313. doi:10.3389/fchem.2021.681313
Yaacoub, E., Kadri, A., Mushtaha, M., and Abu-Dayya, A. (2013). “Air quality monitoring and analysis in Qatar using a wireless sensor network deployment,” in 2013 9th International Wireless Communications and Mobile Computing Conference (IWCMC), USA, 1-5 July 2013 (IEEE).
Zanella, A., Bui, N., Castellani, A., Vangelista, L., and Zorzi, M. (2014). Internet of things for smart cities. IEEE Internet Things J. 1 (1), 22–32. doi:10.1109/jiot.2014.2306328
Zhang, B., Li, M., Song, Z., Kan, H., Yu, H., Liu, Q., et al. (2017). Sensitive H2S gas sensors employing colloidal zinc oxide quantum dots. Sensors Actuators B Chem. 249, 558–563. doi:10.1016/j.snb.2017.03.098
Zhang, Y.-n., Niu, Q., Gu, X., Yang, N., and Zhao, G. (2019). Recent progress on carbon nanomaterials for the electrochemical detection and removal of environmental pollutants. Nanoscale 11 (25), 11992–12014. doi:10.1039/c9nr02935d
Zhao, J., and Yang, X. (2003). Photocatalytic oxidation for indoor air purification: a literature review. Build. Environ. 38 (5), 645–654. doi:10.1016/s0360-1323(02)00212-3
Zheng, T., Bergin, M. H., Johnson, K. K., Tripathi, S. N., Shirodkar, S., Landis, M. S., et al. (2018). Field evaluation of low-cost particulate matter sensors in high-and low-concentration environments. Atmos. Meas. Tech. 11 (8), 4823–4846. doi:10.5194/amt-11-4823-2018
Keywords: carbon nanotubes, nanomaterials, air quality, sensors, IoT sensors network
Citation: Shahid I, Shahzad MI, Tutsak E, Mahfouz MMK, Al Adba MS, Abbasi SA, Rathore HA, Asif Z and Chen Z (2024) Carbon based sensors for air quality monitoring networks; middle east perspective. Front. Chem. 12:1391409. doi: 10.3389/fchem.2024.1391409
Received: 25 February 2024; Accepted: 26 April 2024;
Published: 20 May 2024.
Edited by:
Asim Jilani, King Abdulaziz University, Saudi ArabiaReviewed by:
Lakshmi Narayanan Mosur Saravana Murthy, Intel, United StatesMuhammad Bilal Shakoor, University of the Punjab, Pakistan
Majid Nazeer, Hong Kong Polytechnic University, Hong Kong SAR, China
Copyright © 2024 Shahid, Shahzad, Tutsak, Mahfouz, Al Adba, Abbasi, Rathore, Asif and Chen. This is an open-access article distributed under the terms of the Creative Commons Attribution License (CC BY). The use, distribution or reproduction in other forums is permitted, provided the original author(s) and the copyright owner(s) are credited and that the original publication in this journal is cited, in accordance with accepted academic practice. No use, distribution or reproduction is permitted which does not comply with these terms.
*Correspondence: Imran Shahid, aXNoYWhpZEBxdS5lZHUucWE=