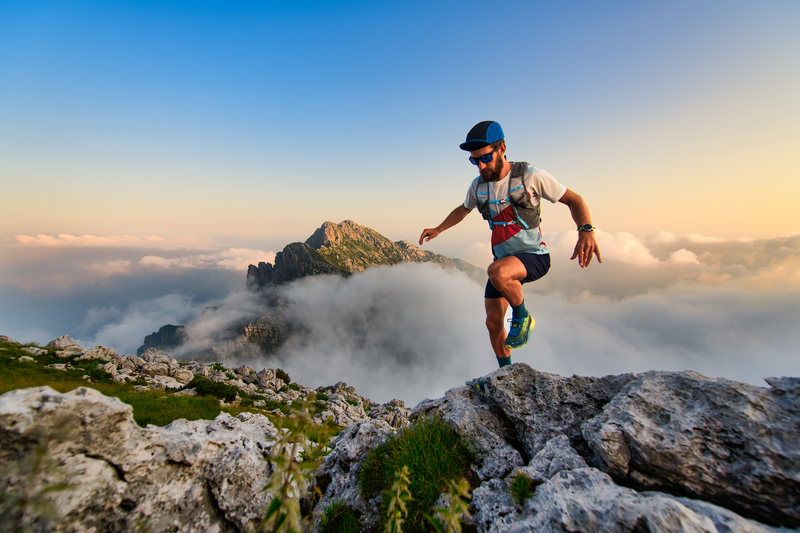
94% of researchers rate our articles as excellent or good
Learn more about the work of our research integrity team to safeguard the quality of each article we publish.
Find out more
PERSPECTIVE article
Front. Chem. , 12 February 2024
Sec. Inorganic Chemistry
Volume 12 - 2024 | https://doi.org/10.3389/fchem.2024.1057928
This article is part of the Research Topic Inorganic Chemistry, Polymer Chemistry, and Solid State Chemistry Editor's Pick 2024 View all 14 articles
Isotopes have been widely applied in a variety of scientific subjects; many aspects of isotopes, however, remain not well understood. In this study, I investigate the relation between the number of neutrons (N) and the number of protons (Z) in stable isotopes of non-radioactive elements and long-lived isotopes of radioactive elements at the double-linear scale (conventional Segrè chart) and the double-logarithmic scale. Statistical analyses show that N is a power-law function of Z for these isotopes: N = 0.73 × Z1.16. This power-law relation provides better predictions for the numbers of neutrons in stable isotopes of non-radioactive elements and long-lived isotopes of radioactive elements than the linear relation on the conventional Segrè chart. The power-law pattern reveled here offers empirical guidance for probing long-lived isotopes of unknown radioactive elements.
Isotopes are variants of elements that possess the same number of protons but differ in the number of neutrons (De Groot, 2004; Ellam, 2016). Several hundred isotopes have been detected in natural environments on Earth, while thousands of other isotopes are continuously created in various areas in the universe (White, 2014; McSween Jr and Huss, 2022). Since the first discovery by Frederick Soddy in 1913 (Soddy, 1913), isotopes have been widely applied in different subjects. For example, ecologists use isotopic signatures to study the exchanges of materials between life and environments (Michener and Lajtha, 2008), biologists investigate metabolic processes on the basis of intermolecular and intramolecular isotopic effects (Kohen and Limbach, 2005), and geochemists use the fingerprints that isotopes left in sedimentary records to reconstruct the evolutionary trajectories of life and environments on the ancient Earth (White, 2014).
Isotopes are classified into two categories according to their stability: stable and radioactive isotopes. Stable isotopes of one element do not transform into other elements under natural conditions, while radioactive isotopes decay to other elements after certain time periods with specific half-lives (De Groot, 2004; Ellam, 2016). Among the known 118 elements on the periodic table, 80 of them have one or more stable isotopes and are called as non-radioactive elements (De Groot, 2004; Ellam, 2016). This non-radioactive category includes the elements with atomic number (Z) less than 83 except for two elements that have no stable isotopes (i.e., technetium with Z = 43 and promethium with Z = 61) (De Groot, 2004; Ellam, 2016). In contrast, nature creates neutrons and protons in an asymmetric manner in the nuclei of elements with atomic number larger than 83; these elements are also referred to as radioactive elements (Blatt and Weisskopf, 1991; Thomson, 2013). As the imbalance between neutrons and protons of a nucleus grows, its stability decreases; in this case, decay offers an chance for the nucleus to re-establish a balance between its neutrons and protons (Blatt and Weisskopf, 1991; Thomson, 2013). While radioactive elements eventually decay into other elements, their long-lived isotopes may have rather extended lifespans (Blatt and Weisskopf, 1991; Thomson, 2013). For example, the half life of 209Bi, an isotope of the radioactive element bismuth, is 2.01 × 1019 years, which is 1.5 × 109 times greater than the age of the universe (De Groot, 2004; Ellam, 2016).
Combining protons and neutrons in an arbitrary way does not necessarily generates a stable nucleus. A nucleus is unbounded when it is outside the valley of stability, which is defined by the neutron drip line and proton drip line (Hansen, 1993; Thoennessen, 2004). Substantial efforts have been dedicated to exploring the principles and mechanisms for the stability of isotopes. Since Myers, Światecki, Viola Jr., and Seaborg predicted that nuclei of superheavy elements occupy a region called as stability island on the Segrè chart (a plot arranging nuclides by proton number Z and neutron number N at the double-linear scale) (Myers and Swiatecki, 1966; Viola Jr and Seaborg, 1966), the concept of the “island of stability” has been a dominant paradigm in the study of superheavy nuclei. A variety of intriguing properties in the island of stability have been revealed. For example, it was discovered in the late 1940s that nuclei possessing a magic number (2, 8, 20, 28, 50, 82, or 126) of protons or neutrons exhibit much higher stability than other nuclei; this phenomenon then became the basis of the nuclear shell model (Wigner, 1937; Mayer, 1948; Haxel et al., 1949; Kanungo et al., 2002).
Here, I investigate the relation between N and Z in stable isotopes of non-radioactive elements and long-lived isotopes of radioactive elements at double-linear and double-logarithmic scales and show that N is a power-law function of Z in these isotopes. The power law refers to a functional relation between two variables in which one variable changes as a power of the other. On a double-logarithmic plot, the power law appears as a straight line, implying that the underlying regularity of this relation is independent of the specific scales one investigates (Clauset et al., 2009; Alstott et al., 2014). Statistical analyses in this work demonstrate that the power-law relation on the double-logarithmic Segrè chart provides more accurate predictions for N than the linear relation obtained on the conventional double-linear Segrè chart. These results offer new insights into the future searching for the long-lived isotopes of unknown radioactive elements.
The dataset on isotopes is from Nubase 2020 (Kondev et al., 2021). Except for technetium and promethium, each non-radioactive element has one or more stable isotopes; the numbers of neutrons in these isotopes usually are close to one another. For each non-radioactive element, I calculate the average number of neutrons in its stable isotopes and denote this mean value by
To investigate the relation between N and Z, I first divide the plot of N versus Z into two regions (Figure 1): (1) non-radioactive elements (
FIGURE 1. Relation of N versus Z in stable isotopes of non-radioactive elements and long-lived isotopes of radioactive elements at the (A) double-linear and (B) double-logarithmic scales. The N on the vertical axis represents either
FIGURE 2. Relation of N versus Z for (A) stable isotopes of non-radioactive elements (blue circles) and (B) long-lived isotopes of radioactive elements (green circles) at the double-linear scale. Panels (A) and (B) in this figure are the magnification of region (1) and region (2) in Figure 1A, respectively. The N on the vertical axis represents
FIGURE 3. Relation of N versus Z for (A) stable isotopes of non-radioactive elements (blue circles) and (B) long-lived isotopes of radioactive elements (green circles) at the double-logarithmic scale. Panels (A) and (B) in this figure are the magnification of region (1) and region (2) in Figure 1B, respectively. The N on the vertical axis represents
To evaluate the goodness of fit of the fitting formulas, I calculate the coefficients of determination (R2’s) and perform the Kolmogorov-Smirnov (KS) test (Massey Jr, 1951) and Cramér-von Mises (CM) two-sample test (Anderson, 1962). The metric R2 measures the fraction of variations in a dependent variable that can be explained by a fitting function; a larger value of R2 indicates a better fitting and therefore a more reliable model (Freund and Wilson, 2003). The KS statistic is defined as
Figures 1A, B show the linear regression for
TABLE 1. Coefficients of determination (R2’s), p-values of the Kolmogorov-Smirnov test (pKS’s), and p-values of the Cramér-von Mises two-sample test (pCM’s) of the best-fitting formulas obtained in region (1), region (2), and both regions (1) and (2) in Figure 1 at the double-linear (Figure 1A) and double-logarithmic (Figure 1B) scales. Region (1) and region (2) in Figure 1A correspond to panel (A) and panel (B) in Figure 2, respectively; region (1) and region (2) in Figure 1B correspond to panel (A) and panel (B) in Figure 3, respectively.
To test the effectiveness of predictions by the mathematical expressions in Table 1, I first use the fitting formulas for the dataset on stable isotopes of non-radioactive elements (i.e.,
TABLE 2. Average relative errors (AREs) and root mean square errors (RMSEs) of the predictions for N by the best-fitting formulas obtained in region (1), region (2), and both regions (1) and (2) in Figure 1 at the double-linear (Figure 1A) and double-logarithmic (Figure 1B) scales. The statistical results in the specific regions in Figure 1 from which the best-fitting formulas in this table are obtained is presented in Table 1. Region (1) and region (2) in Figure 1A correspond to panel (A) and panel (B) in Figure 2, respectively; region (1) and region (2) in Figure 1B correspond to panel (A) and panel (B) in Figure 3, respectively. The percentages in parentheses show how much the AREs or RMSEs of predictions for N in specific regions change when switching from linear relations to power laws; downward arrows indicate decreases.
To predict the N values of long-lived isotopes of unknown radioactive elements [region (3) in Figure 1B], I calculate the power-law formula between N and Z using all data [both regions (1) and (2) in Figure 1B] at the double-logarithmic scale; the relation of N versus Z for all data at the double-linear scale [both regions (1) and (2) in Figure 1A] is also computed for comparison. The AREs of the predictions for
As quantum systems, nuclei’s behaviors can be depicted with the nonrelativistic Schrödinger equation (Olavo, 1999; Schleich et al., 2013); within nuclei, how triple quarks gather to form protons and neutrons can be interpreted by quantum chromodynamics (QCD) (Marciano and Pagels, 1978; Greiner et al., 2007; Group et al., 2022). However, it is well known that describing the features of isotopes does not need to explicitly include quarks in theoretical models; instead, having protons and neutrons in these models is sufficient to predict a variety of properties of isotopes (Blatt and Weisskopf, 1991; Thomson, 2013). For example, the mass of a nucleus can be estimated from its number of protons and neutrons using the Weizsäcker mass formula (Weizsäcker, 1935), which is a refined form of the liquid drop model for the binding energy of nuclei (Gamow, 1930). Moreover, neutrons also affect the stability of nuclei and isotopes’ decay (Mueller and Sherrill, 1993; Pfützner et al., 2012). In a stable nucleus, valence neutrons are well bound; the corresponding wavefunction decays rapidly when the valence neutron is outside the limit of stability (Blatt and Weisskopf, 1991; Mueller and Sherrill, 1993). In contrast, within an unstable nucleus, valence neutrons are loosely bound and live in the classically forbidden region; the corresponding wavefunction possesses a long tail (Blatt and Weisskopf, 1991; Mueller and Sherrill, 1993). For a specific element (with a fixed number of protons), the lifespans of its isotopes are influenced by the number of neutrons (Thomson, 2013; Martin and Shaw, 2017). Therefore, the number of neutrons offers a window through which to predict the long-lived isotopes of unknown radioactive elements.
Statistical analyses in this study show that power laws exist between the number of neutrons and the number of protons in both stable isotopes of non-radioactive elements and long-lived isotopes of radioactive elements. Power laws have been identified in a variety of natural systems, such as the evolutionary processes of life over geological time scales (Raup, 1986; Shang, 2024), the distributions of amino acids and expressed genes in various organisms and tissues (Furusawa and Kaneko, 2003; Mora and Bialek, 2011), and the degradation rate versus age of organic matter in ecosystems (Middelburg, 1989; Shang, 2023). However, the specific reasons for many observed power-law patterns are not well understood (Clauset et al., 2009; Alstott et al., 2014). Similarly, why the coefficient and exponent in the power-law relation between N and Z (Figure 1B, Figure 3; Table 1) take those specific values remain unknown. Moreover, the underlying physical mechanisms responsible for the emergence of these power laws may not be readily interpreted by our current knowledge of nucleons. It is well known that the binding energy of a nucleus derives from the strong interaction, which is described by QCD (Marciano and Pagels, 1978; Greiner et al., 2007; Group et al., 2022). Nevertheless, the (low-energy) QCD is not at the stage where we can use it to obtain comprehensive understating of nucleons (Marciano and Pagels, 1978; Greiner et al., 2007; Group et al., 2022). From the perspective of statistical mechanics, power laws are usually attributed to self-organized criticality, a concept that was originally suggested by Bak, Tang, and Wiesenfeld (Bak et al., 1987). Self-organized criticality refers to the phenomenon that the internal interactions of a system organize itself into states where power laws appear (Bak et al., 1987, 1988). However, studies have shown that power-law patterns are an emergent property of self-organized criticality and do not necessarily originate from the latter (Solow, 2005; Clauset et al., 2009; Marković and Gros, 2014). Whether the power-law pattern observed in this study (Figure 1B, Figure 3; Table 1) originates from certain mechanisms related to self-organized criticality in nuclei requires further investigation.
The power laws shown in this work provide better prediction for the number of neutrons in stable isotopes of non-radioactive elements or long-lived isotopes of radioactive elements than the linear relation on the Segrè chart (Tables 1, 2). The power law, N = 0.73 × Z1.16, which is obtained using all data (i.e., both
Studies have suggested that the neutron-proton asymmetry significantly influences the stability of a nucleus; as the value of Z increases, the stability of a nucleus decreases due to the growth of Coulomb repulsion (Blatt and Weisskopf, 1991; Martin and Shaw, 2017). This implies that the power-law pattern presented here probably will disappear when Z exceeds a certain large, critical value. Actually, power laws observed in natural systems often vanish at some critical points (Schroeder, 2009; Bak, 2013). Although this appears daunting, no available clue shows that the disappearance of the power-law pattern occurs immediately when Z ≥ 119. Therefore, the mathematical formula, N = 0.73 × Z1.16, may still provide empirical guidance for probing the long-lived isotopes of unknown radioactive elements. Future discovery of new radioactive elements will offer further validation for the predictive ability of the power-law relation revealed in this study.
The original contributions presented in the study are included in the article/Supplementary Material, further inquiries can be directed to the corresponding author.
HS conceived the project, performed the analysis, and wrote the manuscript.
I thank editor Zoltan Kovacs for securing reviewers and Valeriia Starovoitova and Zoltan Szucs for thoughtful and constructive comments on the manuscript. I am grateful for the financial support from the Open Access Article Processing Charge Award Fund of the University of Oregon Libraries.
The author declares that the research was conducted in the absence of any commercial or financial relationships that could be construed as a potential conflict of interest.
All claims expressed in this article are solely those of the authors and do not necessarily represent those of their affiliated organizations, or those of the publisher, the editors and the reviewers. Any product that may be evaluated in this article, or claim that may be made by its manufacturer, is not guaranteed or endorsed by the publisher.
Alstott, J., Bullmore, E., and Plenz, D. (2014). Powerlaw: a Python package for analysis of heavy-tailed distributions. PloS One 9 (1), e85777. doi:10.1371/journal.pone.0085777
Anderson, T. W. (1962). On the distribution of the two-sample Cramér-von Mises criterion. Ann. Math. Statistics 33, 1148–1159. doi:10.1214/aoms/1177704477
Bak, P. (2013). How nature works: the science of self-organized criticality. Cham: Springer Science & Business Media.
Bak, P., Tang, C., and Wiesenfeld, K. (1987). Self-organized criticality: an explanation of the 1/f noise. Phys. Rev. Lett. 59 (4), 381–384. doi:10.1103/physrevlett.59.381
Bak, P., Tang, C., and Wiesenfeld, K. (1988). Self-organized criticality. Phys. Rev. A 38 (1), 364–374. doi:10.1103/physreva.38.364
Blatt, J. M., and Weisskopf, V. F. (1991). Theoretical nuclear physics. Massachusetts, United States: Courier Corporation.
Clauset, A., Shalizi, C. R., and Newman, M. E. (2009). Power-law distributions in empirical data. SIAM Rev. 51 (4), 661–703. doi:10.1137/070710111
De Groot, P. A. (2004). Handbook of stable isotope analytical techniques, volume 1. Amsterdam, Netherlands: Elsevier.
Furusawa, C., and Kaneko, K. (2003). Zipf’s law in gene expression. Phys. Rev. Lett. 90 (8), 088102. doi:10.1103/physrevlett.90.088102
Gamow, G. (1930). Mass defect curve and nuclear constitution. Proc. R. Soc. Lond. Ser. A, Contain. Pap. a Math. Phys. Character 126 (803), 632–644. doi:10.1098/rspa.1930.0032
Greiner, W., Schramm, S., and Stein, E. (2007). Quantum chromodynamics. Cham: Springer Science & Business Media.
Group, P. D., Workman, R., Burkert, V., Crede, V., Klempt, E., Thoma, U., et al. (2022). Review of particle physics. Prog. Theor. Exp. Phys. 2022 (8), 083C01. doi:10.1093/ptep/ptac097
Hansen, P. (1993). Nuclear structure at the drip lines. Nucl. Phys. A 553, 89–106. doi:10.1016/0375-9474(93)90617-7
Haxel, O., Jensen, J. H. D., and Suess, H. E. (1949). On the “magic numbers” in nuclear structure. Phys. Rev. 75 (11), 1766. doi:10.1103/physrev.75.1766.2
Hergert, H. (2020). A guided tour of ab initio nuclear many-body theory. Front. Phys. 8, 379. doi:10.3389/fphy.2020.00379
Kanungo, R., Tanihata, I., and Ozawa, A. (2002). Observation of new neutron and proton magic numbers. Phys. Lett. B 528 (1-2), 58–64. doi:10.1016/s0370-2693(02)01206-6
Kohen, A., and Limbach, H. H. (2005). Isotope effects in chemistry and biology. Florida, United States: CRC Press.
Kondev, F., Wang, M., Huang, W., Naimi, S., and Audi, G. (2021). The NUBASE2020 evaluation of nuclear physics properties. Chin. Phys. C 45 (3), 030001. doi:10.1088/1674-1137/abddae
Marciano, W., and Pagels, H. (1978). Quantum chromodynamics. Phys. Rep. 36 (3), 137–276. doi:10.1016/0370-1573(78)90208-9
Marković, D., and Gros, C. (2014). Power laws and self-organized criticality in theory and nature. Phys. Rep. 536 (2), 41–74. doi:10.1016/j.physrep.2013.11.002
Massey, F. J. (1951). The Kolmogorov-Smirnov test for goodness of fit. J. Am. Stat. Assoc. 46 (253), 68–78. doi:10.1080/01621459.1951.10500769
Mayer, M. G. (1948). On closed shells in nuclei. Phys. Rev. 74 (3), 235–239. doi:10.1103/physrev.74.235
Michener, R., and Lajtha, K. (2008). Stable isotopes in ecology and environmental science. New Jersey, United States: John Wiley & Sons.
Middelburg, J. J. (1989). A simple rate model for organic matter decomposition in marine sediments. Geochimica Cosmochimica Acta 53 (7), 1577–1581. doi:10.1016/0016-7037(89)90239-1
Mora, T., and Bialek, W. (2011). Are biological systems poised at criticality? J. Stat. Phys. 144, 268–302. doi:10.1007/s10955-011-0229-4
Mueller, A. C., and Sherrill, B. M. (1993). Nuclei at the limits of particle stability. Annu. Rev. Nucl. Part. Sci. 43 (1), 529–583. doi:10.1146/annurev.ns.43.120193.002525
Myers, W. D., and Swiatecki, W. J. (1966). Nuclear masses and deformations. Nucl. Phys. 81 (1), 1–60. doi:10.1016/0029-5582(66)90639-0
Olavo, L. (1999). Foundations of quantum mechanics: non-relativistic theory. Phys. A Stat. Mech. its Appl. 262 (1-2), 197–214. doi:10.1016/s0378-4371(98)00395-1
Pfützner, M., Karny, M., Grigorenko, L., and Riisager, K. (2012). Radioactive decays at limits of nuclear stability. Rev. Mod. Phys. 84 (2), 567–619. doi:10.1103/revmodphys.84.567
Raup, D. M. (1986). Biological extinction in Earth history. Science 231 (4745), 1528–1533. doi:10.1126/science.11542058
Ring, P., and Schuck, P. (2004). The nuclear many-body problem. Cham: Springer Science & Business Media.
Schleich, W. P., Greenberger, D. M., Kobe, D. H., and Scully, M. O. (2013). Schrödinger equation revisited. Proc. Natl. Acad. Sci. 110 (14), 5374–5379. doi:10.1073/pnas.1302475110
Schroeder, M. (2009). Fractals, chaos, power laws: minutes from an infinite paradise. Massachusetts, United States: Courier Corporation.
Shang, H. (2023). A generic hierarchical model of organic matter degradation and preservation in aquatic systems. Commun. Earth Environ. 4 (16), 16. doi:10.1038/s43247-022-00667-4
Shang, H. (2024). Scaling laws in the evolutionary processes of marine animals over the last 540 million years. Geosystems Geoenvironment 3 (1), 100242. doi:10.1016/j.geogeo.2023.100242
Solow, A. R. (2005). Power laws without complexity. Ecol. Lett. 8 (4), 361–363. doi:10.1111/j.1461-0248.2005.00738.x
Thoennessen, M. (2004). Reaching the limits of nuclear stability. Rep. Prog. Phys. 67 (7), 1187–1232. doi:10.1088/0034-4885/67/7/r04
Viola, V., and Seaborg, G. (1966). Nuclear systematics of the heavy elements — II Lifetimes for alpha, beta and spontaneous fission decay. J. Inorg. Nucl. Chem. 28 (3), 741–761. doi:10.1016/0022-1902(66)80412-8
Weizsäcker, C. v. (1935). Zur theorie der kernmassen. Z. für Phys. 96 (7-8), 431–458. doi:10.1007/BF01337700
Keywords: non-radioactive elements, radioactive elements, stable isotopes, long-lived isotopes, unknown isotopes, power laws, double-logarithmic scale, Segrè chart
Citation: Shang H (2024) Probing long-lived radioactive isotopes on the double-logarithmic Segrè chart. Front. Chem. 12:1057928. doi: 10.3389/fchem.2024.1057928
Received: 30 September 2022; Accepted: 09 January 2024;
Published: 12 February 2024.
Edited by:
Zoltan Kovacs, University of Texas Southwestern Medical Center, United StatesReviewed by:
Valeriia Starovoitova, International Atomic Energy Agency, AustriaCopyright © 2024 Shang. This is an open-access article distributed under the terms of the Creative Commons Attribution License (CC BY). The use, distribution or reproduction in other forums is permitted, provided the original author(s) and the copyright owner(s) are credited and that the original publication in this journal is cited, in accordance with accepted academic practice. No use, distribution or reproduction is permitted which does not comply with these terms.
*Correspondence: Haitao Shang, aHRzaGFuZy5yZXNlYXJjaEBnbWFpbC5jb20=
†ORCID: Haitao Shang, orcid.org/0000-0002-5731-4559
Disclaimer: All claims expressed in this article are solely those of the authors and do not necessarily represent those of their affiliated organizations, or those of the publisher, the editors and the reviewers. Any product that may be evaluated in this article or claim that may be made by its manufacturer is not guaranteed or endorsed by the publisher.
Research integrity at Frontiers
Learn more about the work of our research integrity team to safeguard the quality of each article we publish.