- 1Zhejiang Province Key Laboratory of Quantum Technology and Device, Department of Physics, Interdisciplinary Centre for Quantum Information, Zhejiang University, Hangzhou, China
- 2Key Laboratory for Biomedical Engineering of Ministry of Education, College of Biomedical Engineering and Instrument Science, Zhejiang University, Hangzhou, China
- 3Zhejiang Provincial Key Laboratory for Cancer Molecular Cell Biology, Life Sciences Institute, Zhejiang University, Hangzhou, China
Spatiotemporal dynamics of small-molecule metabolites have gained increasing attention for their essential roles in deciphering the fundamental machinery of life. However, subcellular-level regulatory mechanisms remain less studied, particularly due to a lack of tools to track small-molecule metabolites. To address this challenge, we developed high-resolution stimulated Raman scattering (SRS) imaging of a genetically engineered model (GEM) to map metabolites in subcellular resolution. As a result, an unexpected regulatory mechanism of a critical metabolite, sterol, was discovered in yeast by amplifying the strength of vibrational imaging by genetic modulation. Specifically, isozymes of 3-hydroxy-3-methylglutaryl coenzyme A reductase (HMGR) were evident to promote ergosterol distribution to distinct subcellular locations, where ergosterol was enriched by a local HMGR-directed synthesis. The heterogeneity of this expression pattern thus provides new insights into sterol metabolism and related disease treatment strategies. These findings demonstrate SRS-GEM as a promising platform for new possibilities in investigating metabolic regulation, disease mechanisms, and biopharmaceutical research.
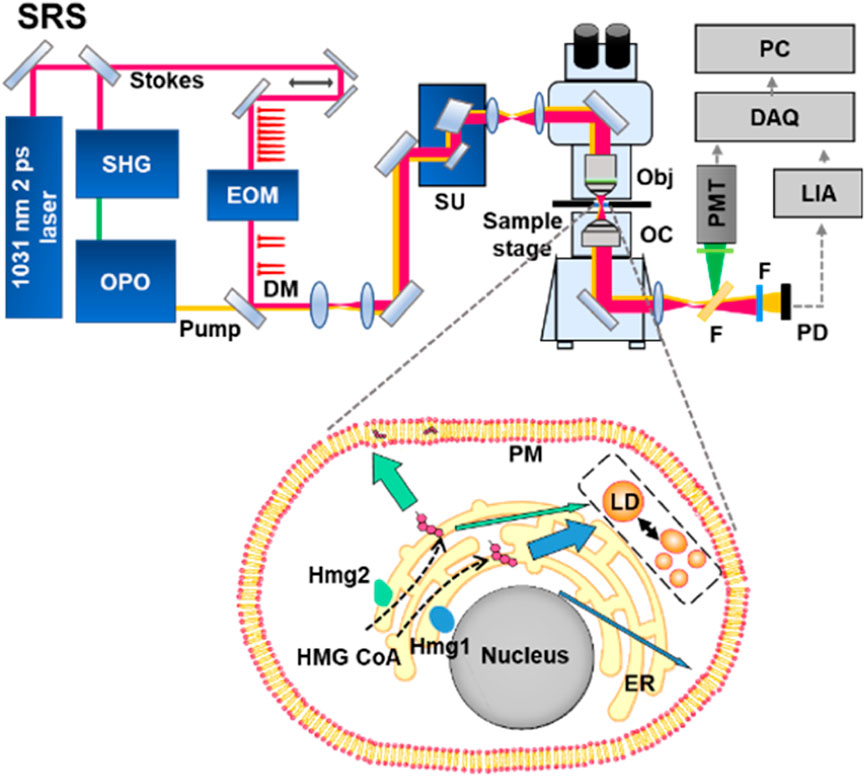
GRAPHICAL ABSTRACT | High-resolution stimulated Raman scattering (SRS) imaging of a genetically engineered model (GEM) enables metabolite imaging in a yeast model and uncovers an unexpected regulatory mechanism of sterol metabolism, providing new insights underpinning the distributional and functional importance of sterol in cells. SRS-GEM demonstrates a promising platform to explore unknown metabolic mechanisms beyond the reach of conventional approaches.
Introduction
Maintaining metabolite homeostasis is critical for the function and survival of cells (Hotamisligil, 2006; Gillies et al., 2008). Metabolic regulations can happen at multiple levels, from single-cell, tissue, and inter-organ to systematic levels. In recent decades, significant progress has been made in understanding the regulatory mechanisms of metabolites such as lipids and glucose at tissue or inter-organ levels by mass spectrometry or nuclear magnetic resonance spectroscopy (Cameron et al., 1993; Dezaki et al., 2008; Peckett et al., 2011). Changes in regulations of metabolite transport and distribution under specific physiological conditions have also been studied systematically (Kishida et al., 2004; Yamauchi and Rogers, 2018; Yutuc et al., 2020), indicating that the function of metabolites is highly dependent on their spatial distribution. For example, distinct spatial localization of sterols was observed between brain regions (Yutuc et al., 2020). Others have elucidated the differences in metabolic and transport dysfunction of cholesterol and sterol intermediates between different tissues in patients with atherosclerosis and cancer (Yamauchi and Rogers, 2018). However, these studies mainly focus on the tissue or inter-organ level, and the spatial regulation of metabolites at the subcellular level remains relatively unclear due to the challenges of tracking small molecules in situ with such a high spatial resolution.
Cholesterol, a vital metabolite with multiple functions (Porter et al., 1996), was selected to demonstrate metabolite imaging. Dysregulation of cholesterol homeostasis significantly impacts various diseases (Kaul, 2003; Vance et al., 2005; Riscal et al., 2019). Cells can obtain cholesterol either by de novo synthesis or by uptake. Notably, the intestinal cholesterol pool size is influenced mainly by endogenous sources (800–1,000 mg) since the diet contributes only 300–400 mg of cholesterol per day (Mok et al., 1979), indicating the importance of endogenous cholesterol synthesis. The enzyme 3-hydroxy-3-methylglutaryl coenzyme A reductase (HMGR) catalyzes one of the most critical steps in cholesterol biosynthesis. Therefore, HMGR has become an important drug target in the treatment of hypercholesterolemia (Grundy et al., 2019), cardiovascular diseases (Zhou and Liao, 2009), and cancer (Jung et al., 2021). It thus triggered the development of a series of statins (e.g., lovastatin, atorvastatin, and simvastatin). However, the long-term use of statins has shown adverse effects (Ramkumar et al., 2016), which are linked to suppression of the mevalonate pathway and membrane damage (Sakamoto and Kimura, 2013), presenting an urgent need to identify a more specific way to control cholesterol homeostasis.
A reliable and controlled biological model is required to address this question, for which a yeast model was adopted. Yeast is a eukaryotic cell model widely used for the mechanistic study of cell metabolism, including cholesterol. Like cholesterol in mammalian cells, ergosterol, the counterpart of cholesterol in yeast, is synthesized via an HMGR-mediated pathway (Daum et al., 1998). It is mainly found on the plasma membrane and in lipid droplets (LDs) as ergosterol ester (Choudhary and Schneiter, 2012). However, unlike mammalian cells, yeast expresses two HMGR genes, namely, HMG1 and HMG2 (Basson et al., 1986). These two isozymes were found to regulate the proliferation of the endoplasmic reticulum (ER) membrane differently (Koning et al., 1996). Moreover, these two isozymes are degraded by different degradation pathways (Burg and Espenshade, 2011). These differences suggest that there may be a functional difference between these two isozymes.
Furthermore, although two HMGR isozymes guide an equal amount of carbon in the biosynthesis of sterols (Casey et al., 1992), it is not clear whether they have relatively independent regulatory mechanisms. Answering these questions would provide opportunities to develop approaches to regulate sterol metabolism more accurately. However, considering the small size of yeast (∼5 µm in diameter), spatiotemporal dynamics of sterol metabolism in yeast are less studied, primarily due to lacking practical tools for high-resolution sterol imaging. Thus, new metabolite imaging methods are needed to synergistically work with biological models to address novel and essential questions in life science and biomedical sciences.
Compared to conventional biochemical ensemble measurements, imaging approaches provide broad possibilities for tracking biomolecules. Molecular spectroscopy-based imaging methods started to gain increasing attention for the advantages of label-free selective imaging of small biomolecules, such as glucose, amino acids, fatty acids, and cholesterol, with high sensitivity and spatial resolution at the sub-micron scale. Recently, stimulated Raman scattering (SRS) microscopy has shown the capability of tracking small molecules based on their intrinsic molecular vibrational properties (Freudiger et al., 2008; Yue et al., 2014; Zhang et al., 2014; Cheng and Xie, 2015). A SRS signal pinpoints specific chemical bonds by excitation with its vibrational energy, providing in situ concentration information and allowing high-speed imaging of various biomolecules at the subcellular level (Min et al., 2011; Chung and Potma, 2013; Cheng and Xie, 2015; Karanja et al., 2017).
The new spectroscopic dimension came with a price—the acquisition speed. In principle, spectroscopic imaging should acquire at Nyquist frequency over the spectroscopy dimension and the XYZ of spatial dimension, providing a complete spectrum at each voxel. However, such a time-consuming acquisition would preclude studying living systems. Thus, methods to break the physical acquisition speed limit have been developed, including frequency spectral multiplexing (Fu et al., 2012; Liao et al., 2015), matrix completion using Lissajous scan (Lin et al., 2018), and compressive sensing (Berto et al., 2017; Lin et al., 2021). Alternatively, molecular tracking was realized by focusing on characteristic spectral bands, such as isotopic labeling (Freudiger et al., 2008; Zhang et al., 2011; Wei et al., 2015) and Raman tags (Wei et al., 2014; Lee et al., 2015; Wei et al., 2016; Du et al., 2022), that have unique spectral peaks in the spectrally silent region of natural compounds. However, the additional complexity of these methods precluded the broad impact of the technique.
Here, we address the grand challenge by developing stimulated Raman imaging of a genetically engineered model (SRS-GEM) (Figures 1A–D). Although complicated, specific subcellular structures or locations are known to have a relatively stable composition in a model organism, allowing quantitative analysis based on the characteristic spectral peaks of the target molecule. Therefore, focusing on their distinctive bands with the proper excitation bandwidth would allow high-throughput tracking of specific metabolites. Demonstrated experimentally, high-selectivity imaging of subcellular metabolites reveals the functional differences of genes based on their spatial preferences.
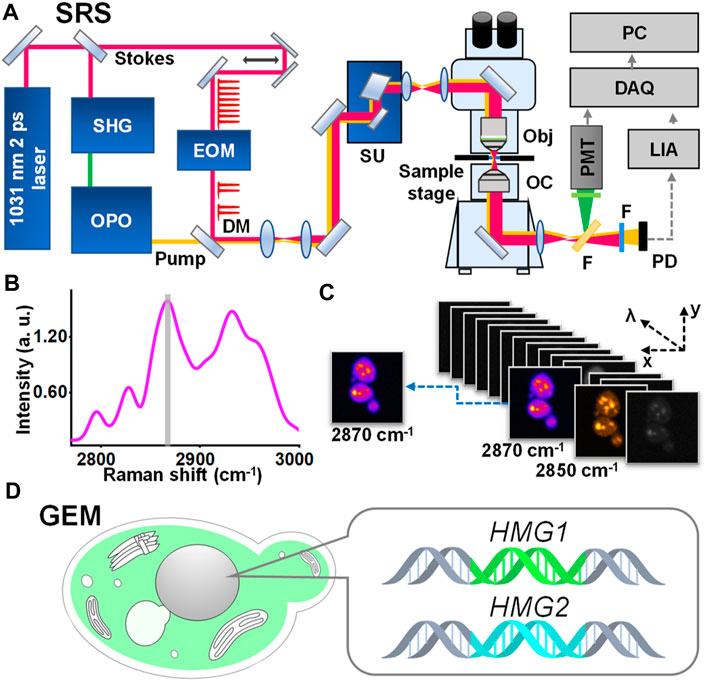
FIGURE 1. Schematic representation of SRS-GEM. (A) SRS platform. SHG, second harmonic generation; OPO, optical parametric oscillator; EOM, electro-optic modulator; DM, dichroic mirror; SU, scan unit; Obj, objective; OC, oil condenser; F, filter; PMT, photomultiplier; PD, photodiode; LIA, lock-in amplifier; DAQ, data acquisition; PC, personal computer. (B) Raman spectrum of ergosterol. The gray area highlights the characteristic peak selected for visualizing ergosterol. (C) Hyperspectral imaging of wildtype yeast. (D) Schematic representation of the GEM.
Unexpectedly, the Hmg1 and Hmg2 genes in the Saccharomyces cerevisiae model showed different spatial preferences for sterol synthesis. Interestingly, there is a significant difference in the subcellular distributions of Hmg1 and Hmg2, consistently correlated with their functions. Furthermore, the subcellular regulation of sterol and lipid metabolism plays a decisive role in controlling cell growth and survival. The SRS-GEM platform collects insights into subcellular metabolism regulation for life science, synthetic biology, and disease treatment.
Materials and methods
Wildtype and HMGR knocked-out yeast strains
All the yeast strains used in this study are isogenic to the prototrophic CEN.PK strain of the budding yeast S. cerevisiae (van Dijken et al., 2000). Single-gene deletion mutants, hmg1Δ and hmg2Δ, were constructed by replacing each open reading frame with an HYG cassette using homologous recombination. The mutants are selected against hygromycin B and verified by the polymerase chain reaction (PCR) (Supplementary Figure S1). Cells were cultured in a synthetic defined (SD) medium containing 0.17% yeast nitrogen base without amino acids and ammonium sulfate (Difco), 0.5% ammonium sulfate (Sigma), and 2% glucose.
Wildtype, hmg1Δ, and hmg2Δ cells were cultured in SD medium overnight and diluted to 0.1 of A600 in fresh SD medium. Then, 100 μM simvastatin was added to logarithmically growing cells. Cells were harvested and fixed after 6 h of simvastatin treatment. Yeast cells were fixed with 1% formaldehyde at room temperature for 15 min and quenched with 125 mM glycine. The cells were pelleted by centrifugation at 3,000 g for 5 min at 4°C, washed twice with cold sterile water, and re-suspended in water for microscopic imaging.
HMGR-GFP yeast strains
Yeast cells expressing Hmg1-GFP and Hmg2-GFP along with Mrh1-mCherry (a plasma membrane marker) or Nup157-mCherry (a nucleus marker) were cultured in synthetic glucose (SD) medium overnight and then diluted to 0.1 of A600 in fresh SD medium for another 6 h culture at 30°C. The localization of Hmg1-GFP and Hmg2-GFP in logarithmically growing cells was observed using a DeltaVision microscopy imaging system.
Specimen preparation for imaging
Agarose gel was used for cell imaging. To prepare the gel, 10 µL of melted 3% (w/v) agarose solution (agarose solution prepared by dissolving agarose in distilled water) was pipetted on a glass slide and then immediately covered with a coverslip. After the gel is solidified, the coverslip was gently removed by rotating. Strips of a double-sided tape were mounted around the gel to help with the sealing after the sample was mounted on the gel. Cells were pelleted and washed with 1 × PBS twice. A volume of 2 µL of the cells was transferred to the agarose gel and covered with another coverslip. Gentle pressure was applied to ensure the sample was completely sealed.
SRS microscopy
As for the microscope setup, we used an upright microscope (BX51WI, Olympus) coupled with a scanning galvanometer in X and Y directions (GVS002, Thorlabs) and a 60 × 1.2 NA water objective (Olympus, Japan). A picosecond laser (picoEmerald, Applied Physics & Electronics, Germany) supplied a synchronized pump beam (tunable wavelength 700–990 nm and 80 MHz repetition rate) and Stokes beam (fixed wavelength at 1,031 nm and 2-ps pulse width). The Stokes beam was modulated at 20 MHz using an electro-optic modulator. The forward-going pump and Stokes beams through the samples were collected using a high numerical aperture oil condenser (numerical aperture 1.4, Olympus). A short-pass dichroic mirror (650 nm cutoff, Thorlabs) was used to separate Raman photons and fluorescence. Fluorescence was acquired by using PMT (H7422-40, Hamamatsu, Japan) with a bandpass filter. A short-pass filter (1000 nm SP, Thorlabs, United States) was used to block the Stokes beam and transmit only the pump beam onto a large-area photodiode (10 mm × 10 mm) for the detection of the SRS signal. The detected photocurrent was sent into a lock-in amplifier. The extracted SRS and fluorescence signals were sent to the data acquisition unit. The final images were assembled in a home-built LabVIEW program during laser scanning at a rate of 10 μs per pixel.
Spectral excitation bandwidth simulation
Peak fitting of ergosterol was handled by Origin 2018. Gaussian functions with a spectral resolution of 2 cm-1 to 80 cm-1 were, respectively, established by MATLAB to simulate the beams’ convolution.
Image analysis
Images were processed and pseudo-colored by ImageJ. For SRS images, corresponding off-resonance images were taken at 2,100 cm−1 and subtracted from the on-resonance images, and the multi-spectral channels were assigned different colors. Quantification of metabolites was carried out by applying automatic local thresholding, analyzing particles, and profile measurements to the multi-spectral image stack. The results of the dual-color analysis were obtained from the intensity difference extracted by subtracting SRS intensity at 2,850 cm−1 in LDs and the plasma membrane based on SRS intensity at 2,870 cm-1 and then normalizing the intensity difference of different yeast treatments.
Quantification of plasma membrane ergosterol levels from SRS images
Background plus three standard deviations of the background were taken as the starting point of the plasma membrane. The maximum signal in the membrane was taken as the midpoint of the membrane. Finally, the sum of the whole membrane signal was calculated, as shown in Supplementary Figure S2 in the highlighted area. For cells where the maximum plasma membrane signal is difficult to be determined, the cell membrane width was replaced by the average membrane width of other cells. Specifically, six points were selected equidistantly throughout the whole membrane for analysis (Supplementary Figure S2A), and 4–5 cells were analyzed in each group.
Confocal Raman spectroscopy
The confocal Raman spectra of ergosterol, protein, and lipid were measured using ergosterol (powder), bovine serum albumin (powder), and olive oil as standard samples. Raman spectra were excited by a 785-nm laser source and obtained using the spectrometer (inVia Reflex, from Renishaw, Britain) with a spectral resolution of <1 cm-1.
Determination of ergosterol levels by liquid chromatography–mass spectrometry (LC–MS)
Yeast cells were collected and disrupted in 100% ice-cold methanol using glass beads for lipid extraction. Total yeast lipids were extracted with chloroform/methanol (2:1) (v/v), as described previously (Ye et al., 2013), and dried using a CentriVap Concentrator system (Labconco, United States). Lipids were re-suspended in an MS-grade buffer (isopropanol: acetonitrile: water = 2:1:1) and cleared with two rounds of centrifugation before injection. Ergosterol levels were quantitatively analyzed in a positive ion mode by multiple reaction monitoring (MRM) acquisition using a triple quadrupole mass spectrometer (Triple Quad 6500+, AB SCIEX) that is coupled with high-performance liquid chromatography. Ergosterol was monitored with a transition of the precursor ion to the production (m/z 379.400→69.400) and separated chromatographically on a C18 column (ACQUITY UPLC BEH C18 Column, 130 Å, 1.7 μm, 2.1 mm × 50 mm). The flow rate was set to 0.15 mL/min using the following buffer system and LC method: Buffer A: 5 mM ammonium acetate in 33.3% methanol, 33.3% acetonitrile, and 33.3% water; Buffer B: 5 mM ammonium acetate in 100% isopropanol. T = 0 min, 0% B; T = 1 min, 20% B; T = 3 min, 60% B; T = 13 min, 98% B; T = 13.10 min, 20% B; and T = 16 min, 20% B. The retention time was compared to the ergosterol standard. The area under each peak was quantitated using SCIEX OS software and re-inspected for accuracy.
Statistical analysis
For statistical analysis, a two-sample t-test was used to compare the metabolic difference between allelic types, including the ergosterol intensity, LD intensity, LD number, and cell area.
Result
SRS imaging enables label-free subcellular mapping of ergosterol
To tackle the challenges of metabolite imaging of the GEM, we developed a high-resolution, high-sensitivity SRS imaging platform (Figure 1A; Supplementary Figure S3). Briefly, a synchronously pumped optical parametric oscillator system provides picosecond pulse sequences at two different wavelengths for molecular vibrational excitation. These are fed into a home-built laser scanning microscope, followed by using a customized amplified detector and lock-in amplifier system. The technical advances include 1) a synchronized electro-optic modulator that locks to the laser repetition to ensure an accurate 2-pulse-on and 2-pulse-off modulation with 100% modulation depth; 2) a customized detector and lock-in amplifier with shot-noise limited detection, using the X channel (in-phase) signal to improve SNR by √2 of the R channel (amplitude), which also remove parasitic signals such as photothermal or cross-phase modulation; 3) a home-built microscope with a fixed objective lens to avoid a typical “dangling” arm, removing mechanical vibrations in the Z-direction critical for high-resolution imaging.
To demonstrate the chemical specificity of our system, a mathematical model was built to quantify and search for an optimal signal-to-background ratio (SBR, see Supplementary Figure S4). The Raman peak width of common small-molecule metabolites is about 25–40 cm-1, such as glucose (42 cm-1 at 1,373 cm-1) (Söderholm et al., 1999), ergosterol (38 cm-1 at 2,870 cm-1), and deuterated lipids (∼20 cm-1 at 2,103 cm-1) (Argov et al., 2008; Fu et al., 2013; Zhang et al., 2013; Zhang et al., 2013; Huang et al., 2020; Chen et al., 2021; Okotrub et al., 2022). The resulting SBR targeting ergosterol indicated high selectivity by <20 cm−1 narrowband excitations (Supplementary Figure S4D). However, a too narrow excitation band would result in a drastically low signal due to the multiphoton process of SRS. Thus, our system, with an excitation bandwidth of 14 cm-1, stands at an optimal balance between the signal level and selectivity.
We performed sterol imaging of the genetically modulated strains and cross-validated them by mass spectrometry (MS). Genetic modulation was constructed by knocking out HMGR, i.e., hmg1Δ and hmg2Δ strains (Supplementary Figures S1A, B), as cells cannot survive with simultaneous knockout (KO) of HMG1 and HMG2 (Basson et al., 1986). SRS-GEM images of ergosterol in wildtype showed strong signals in LDs and cellular membranes, while in the KO strains, significantly lower signals were observed (Figure 2A, at 2,870 cm-1). Furthermore, quantifying the SRS signal intensity, total ergosterol from hmg1Δ and hmg2Δ cells reduced significantly with p < 1 × 10−4 (Figure 2B). Importantly, the mass spectrometry measurement of ergosterol in a population of yeast cells showed an almost similar trend with p < 1 × 10−4 (Figure 2C). These results also demonstrate that SRS-GEM imaging allows quantitative measurement of ergosterol at the single-cell level.
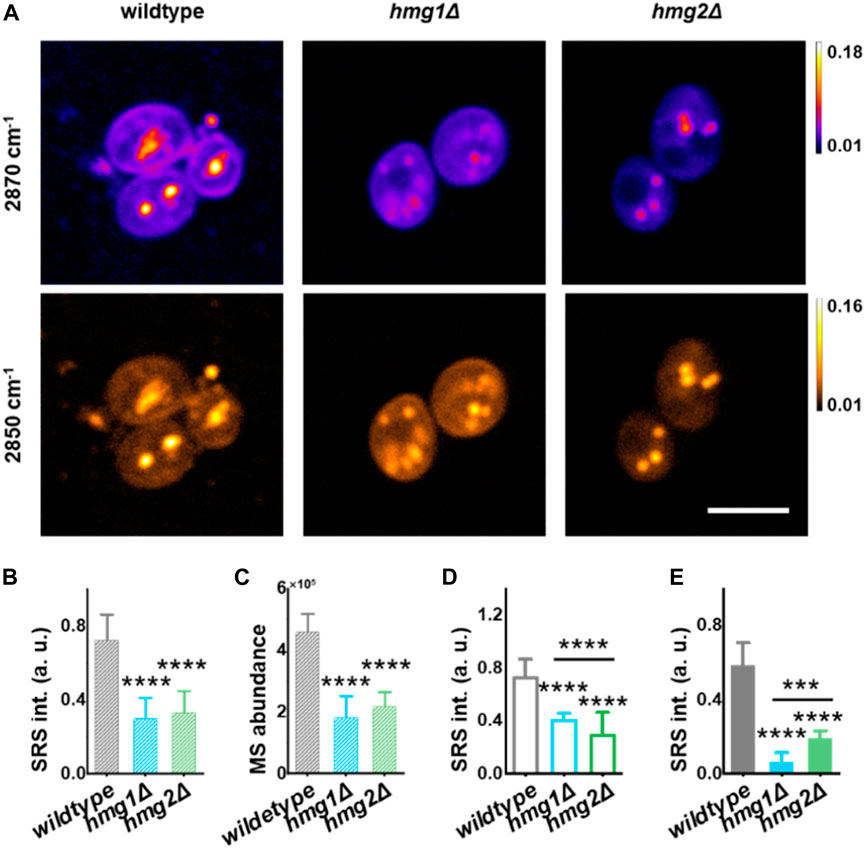
FIGURE 2. Regulation of ergosterol at the subcellular level by HMGR isozymes revealed by SRS-GEM. (A) Representative SRS images at 2,870 cm−1 and 2,850 cm-1 in S. cerevisiae strains. (B) Total ergosterol SRS intensity collected from a single S. cerevisiae cell obtained by dual-color unmixing analysis. (n = 10 for wildtype, n = 9 for hmg1Δ, and n = 14 for hmg2Δ). (C) Total ergosterol abundance extracted by mass spectrometry. MS, mass spectrometry. (D) Ergosterol signal from the plasma membrane extracted by dual-color unmixing analysis. (n = 30 for wildtype, n = 24 for hmg1Δ, n = 31 for hmg2Δ). (E) Ergosterol content in individual LD obtained by dual-color unmixing analysis (wildtype, n = 11; hmg1Δ, n = 16; hmg2Δ, n = 26). Error bars represent +SD. *, p < 0.05; **, p < 0.01; ***, p < 1 × 10−3; ****, p < 1 × 10−4. Scale bar: 5 μm.
HMG1 and HMG2 regulate ergosterol distribution differently
We noticed that ergosterol distribution is different between hmg1Δ and hmg2Δ. To study the subcellular distribution of ergosterol, we analyzed ergosterol in the plasma membrane and LDs in the SRS-GEM images of single yeast cells (Figure 2A). The SRS signal across the plasma membrane is defined by drawing a line from the extracellular, near-membrane position where the signal crosses the threshold (background +3 standard deviations) for the first time, and the highest SRS intensity positioned at the mid-point of the line (Supplementary Figures S2A, B). As expected, the membrane ergosterol level is the highest in the wildtype compared with other groups. Surprisingly, we found a significant difference in membrane ergosterol levels between the hmg1Δ and hmg2Δ groups (Figure 2D). Furthermore, while the hmg1Δ strain also showed reduction compared to the wildtype, the hmg2Δ strain showed a higher reduction than the hmg1Δ strain (Figure 2D, p < 1 × 10−4 between hmg1Δ and hmg2Δ). Interestingly, the ergosterol levels in LDs showed an opposite trend, while the ergosterol content in LDs showed a higher decrease in the hmg1Δ strain; a lower decrease was observed in the hmg2Δ strain (Figure 2E, p < 1 × 10−3 between hmg1Δ and hmg2Δ).
To validate these observations, we overexpressed either Hmg1 or Hmg2 in the KO strains (Supplementary Figures S1A, B) and compared subcellular ergosterol levels using SRS-GEM imaging of single yeast cells (Figure 3A). Both Hmg1 and Hmg2 overexpression (OE) resulted in increased ergosterol levels in the plasma membrane. Importantly, although overexpressing either Hmg1 or Hmg2 rescued the ergosterol level in the plasma membrane, Hmg2 OE in the hmg1Δ strain resulted in a membrane ergosterol level similar to wildtype. It suggests that HMG2 mainly contributes to ergosterol distribution in the plasma membrane (Figure 3B; Supplementary Figures S5A, B, p < 1 × 10−4). At the same time, both Hmg1 OE and Hmg2 OE rescued the ergosterol level in LDs (Figure 3C, p < 1 × 10−4). It is likely that ergosterol synthesized by HMG1 is mainly distributed to LDs, given that Hmg1 OE slightly rescued membrane ergosterol levels but resulted in a robust increase in ergosterol in LDs. These results suggest that HMG1 and HMG2 regulate ergosterol subcellular distribution differently.
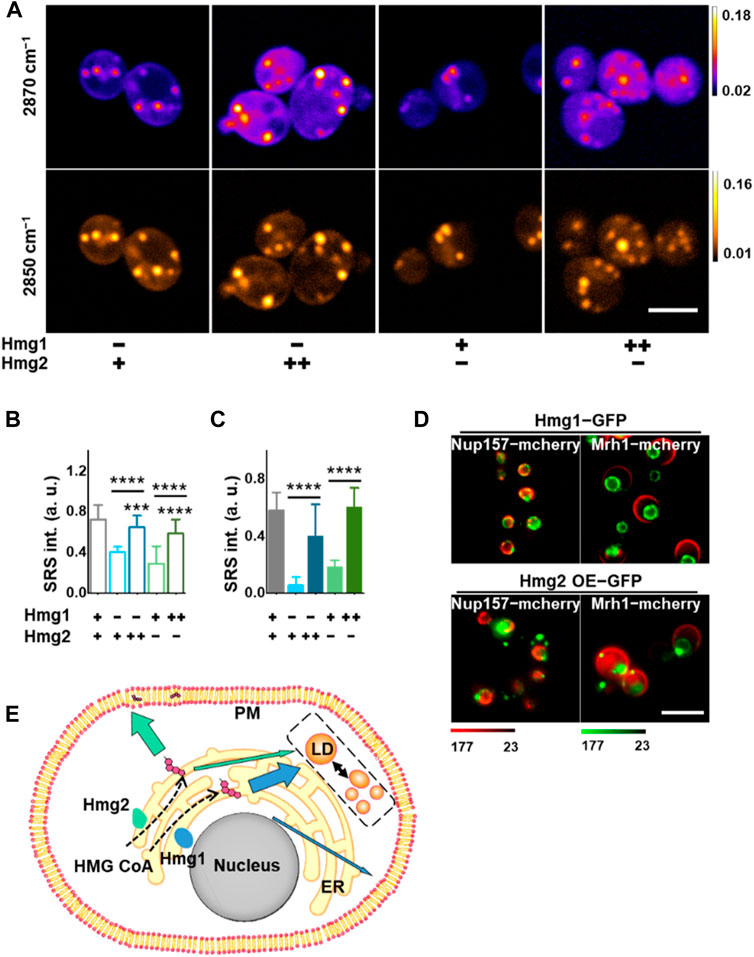
FIGURE 3. Distinctive subcellular pattern of HMGR isozymes for local ergosterol synthesis. (A) Representative SRS images at 2,870 cm−1 and 2,850 cm-1 in S. cerevisiae with different HMGR isozyme expressions. (B) Ergosterol signal from the plasma membrane after HMGR knockout or overexpression extracted by dual-color analysis. (n = 30, 24, 24, 30, and 30, respectively). Error bars: +SD. (C) Ergosterol content in individual LD after HMGR knockout or overexpression extracted by dual-color analysis (n = 11, 16, 26, 26, and 26, respectively). Error bars: +SD. (D) Confocal fluorescent images of yeast cells for HMGR isozyme distribution. Green: HMGR. Red: Nup157 for the nuclear membrane and Mrh1 for the plasma membrane. (E) Proposed regulatory mechanism for lipid and ergosterol metabolism. *, p < 0.05; **, p < 0.01; ***, p < 1 × 10−3; ****, p < 1 × 10−4. Scale bars, 5 μm. “−” stands for KO, “+” stands for the normal level, and “++” stands for OE.
The subcellular location of HMGRs may regulate ergosterol fate
The aforementioned findings triggered us to investigate the mechanism behind the subcellular regulation of ergosterol by different HMGR isozymes because HMGRs are found to be expressed on the ER membranes (Koning et al., 1996; Gatta et al., 2015). We tracked the expression profiles of HMG1 and HMG2 using GFP fusion proteins (method in Supplementary Figure S5C). To validate their locations, the nuclear and plasma membranes were labeled by expressing Nup157-mCherry and Mrh1-mCherry, respectively. From the imaging results (Figure 3D), interestingly, we found a distinctive subcellular expression pattern where HMG1 is almost exclusively expressed on the peri-nuclear side of ER. In contrast, HMG2 is primarily expressed on the peri-membrane side.
Based on the aforementioned complementary observations, we suggest an unrecognized regulatory mechanism of sterol synthesis and trafficking, driven by the subcellular enrichment of critical enzymes (Figure 3E). HMG1 and HMG2 are expressed differently to promote subcellular enrichment of the rate-limiting step of ergosterol synthesis. Specifically, HMG1 is mainly expressed on the peri-nuclear ER, which drives ergosterol synthesis and storage in LDs. HMG2, on the other hand, is expressed mainly on the peri-membrane ER, which mediates more efficient synthesis and incorporation of ergosterol into the plasma membrane.
HMG1 and HMG2 regulate LD properties differently
In addition to changing the content and distribution of ergosterol, we found that modulating the expression of HMGR also changed the LD properties in yeast. To image lipids, a prominent peak at 2,850 cm-1 in the Raman spectrum of olive oil was used (Supplementary Figure S6A), which arises mainly from the CH2 symmetric stretch mode. The spectral width of this peak is 22 cm-1, which matches the FWHM of the pump and Stokes beams. By SRS-GEM imaging at 2,850 cm-1, prominent LD features were observed (Figures 2A, 3A; Supplementary Figure S6B). To quantify different LD properties, SRS signals from LDs were isolated by applying a threshold (Supplementary Figure S6C, Experimental Section).
From quantification, we found that the size of LDs decreased significantly after HMG2 KO (Supplementary Figure S6D). Although the size of LDs was not rescued, Hmg1 OE in hmg2Δ resulted in an increased number of LDs (Supplementary Figure S6E). On the other hand, Hmg2 OE in hmg1Δ rescued the LD size (Supplementary Figure S6D). Interestingly, the LDs found in the Hmg2 OE hmg1Δ are closer to the plasma membrane than those found in the Hmg1 OE hmg2Δ (Supplementary Figure S6F, p < 1 × 10−4), indicating a local regulation of LD metabolism by HMGR isozymes. Collectively, these results demonstrate distinctive functional roles of HMG1 and HMG2.
HMG1 and HMG2 affect the yeast cell size and survival, respectively
Ergosterol distribution is essential for cell growth and survival. Therefore, it is likely that spatial regulation of ergosterol synthesis will cause different physiological consequences. Because the complete knockout of both HMG1 and HMG2 is lethal, we adopted a potent HMGR inhibitor, simvastatin, to study this process. As a treatment of hypercholesterolemia, simvastatin competitively binds to HMG-coenzyme A with HMGR (Maciejak et al., 2013), reducing the synthesis of cholesterol (or ergosterol in yeast). We found that the cell size and viability reduced significantly after simvastatin treatment in the wildtype strain (Figures 4A–C). Interestingly, the cell size reduction by simvastatin treatment was only observed in the hmg2Δ strain (Figure 4B, p < 1 × 10−2), suggesting HMG1 may be essential for regulating the cell size. On the other hand, cell viability is suppressed most significantly in the hmg1Δ strain by simvastatin (Figures 4C–E), suggesting HMG2 may be essential for maintaining cell survival.
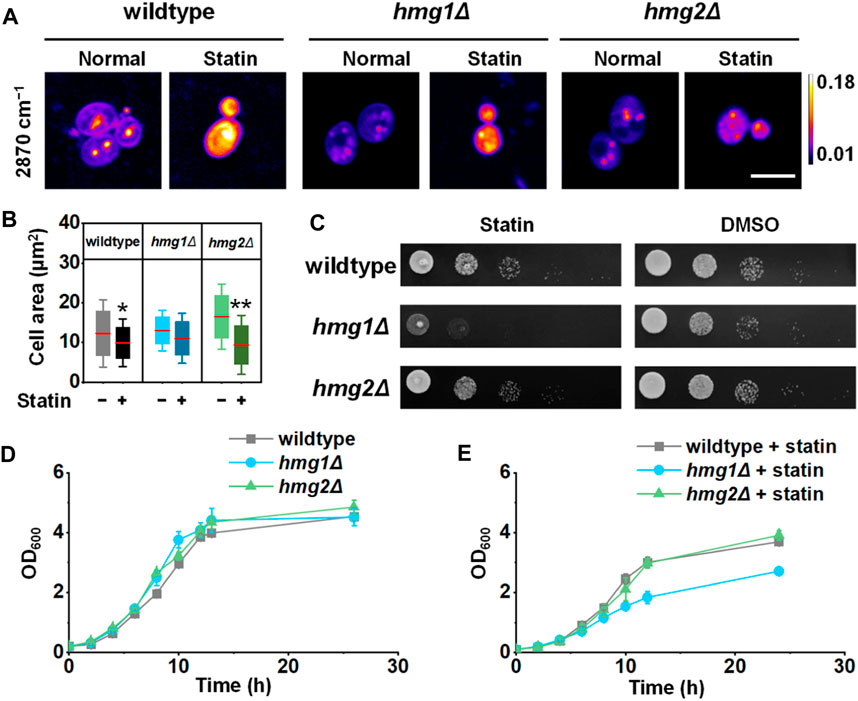
FIGURE 4. Effects of two HMGR isozymes on the cell size and survival rate. (A) Representative SRS images of ergosterol after simvastatin treatment (100 mM, 6 h). (B) Quantification of the cell area (n = 37, 40, 27, 49, 27, and 40). (C) Viability of S. cerevisiae after simvastatin treatment compared to the DMSO control. (D, E) Growth curves of S. cerevisiae with wildtype and simvastatin treatment, respectively, (n = 3 for each group). Error bars: ±SD. *, p < 0.05; **, p < 0.01; ***, p < 1 × 10−3; ****, p < 1 × 10−4. Scale bar: 5 μm. “−” stands for untreated and “+” stands for treated.
Discussion
The spatial regulation of metabolic mechanisms demonstrates the importance of imaging technologies in tracking biomolecules. With the resolution beyond the optical diffraction limit, localization microscopy techniques have broad applications in biology and medicine. However, fluorescence-based imaging relies on fluorophores to selectively tag target molecules, presenting problems when tracking small molecules such as cholesterol or sterol. Furthermore, the amount of dye needed for the super-resolution fluorescence technique increases quadratically with the fold improvement of resolution. Nevertheless, lipid-tracking dyes are usually based on hydrophobicity and, thus, low in selectivity (Yen et al., 2010). Therefore, SRS-GEM provides label-free imaging of mapping small biological molecules and avoids the various problems caused by labeling.
Based on our hypothesized molecular mechanism, HMG1 primarily drives ergosterol distribution to LDs, an energy storage unit for a cell. In contrast, HMG2 mainly drives ergosterol distribution to the plasma membrane, where sterol-rich membrane structures, such as lipid rafts, are formed. It is known that lipid rafts are essential for pro-survival signal transduction (George and Wu, 2012). Therefore, the physiological functions of HMG1 and HMG2 may be related to the final fate of ergosterol.
We note that protein isoforms often show different metabolic activities, which accompany specific regional distribution at tissue levels. For example, mammalian cells express sterol regulatory element-binding protein (SREBP) isoforms, namely, SREBP-1a, SREBP-1c, and SREBP-2, which show different efficiencies for regulating fatty acid and cholesterol synthesis. Furthermore, SREBP-1c and SREBP-2 are expressed in most tissues, whereas SREBP-1a is mainly expressed in specific tissues and cells, showing a difference in spatial preferences (Shimano et al., 1997). Moreover, lactate dehydrogenase (LDH) has five isoforms, among which LDH-1 and LDH-5 show differential distributions in the brain (Laughton et al., 2000). Our study revealed the regulation of cellular metabolism by different protein isoforms at the subcellular level with a much higher spatial resolution. These results present the importance of precise regulation of ergosterol synthesis and distribution at the subcellular level.
Both ergosterol synthesis and transport are energy-intensive processes (Hu et al., 2017). Therefore, the proposed mechanism here is likely a result of balancing the energy consumption from the synthesis and transport of ergosterol to enhance the efficiency. In previous studies, Koning et al. reported that Hmg2 OE results in the proliferation of the ER membrane (Koning et al., 1996), which will extend toward the plasma membrane. Interestingly, other proteins mediating ergosterol transport, such as ERG28 (Mo et al., 2004), are co-localized in the proliferating ER membranes (Federovitch et al., 2008), suggesting one function of the proliferating ER membrane is to transport ergosterol. More recently, a similar phenomenon has been observed in regulating nucleus–vacuole junctions (NVJs), a multifunctional platform for lipid synthesis. HMG1 is found to be enriched in the NVJ under acute cellular glucose restriction (Hariri et al., 2018; Rogers et al., 2021). Such enrichment enhanced the efficiency of the mevalonate pathway. Together, these studies further support our hypothesis that subcellular enrichment of HMGR is essential for more efficient sterol synthesis and transport.
Mammalian cells express only one HMGR, so to translate our findings into the mammalian system, we suggest that mammalian HMGR might bind to the peri-nuclear and peri-membrane ER through interactions with different protein complexes or unique post-translational modifications. It indicates that the ER properties at different subcellular locations are essential in achieving subcellular-level cholesterol synthesis regulation. Future studies to explore such a mechanism in the mammalian system and design approaches to control the expression of HMGR at specific subcellular locations will be critical. Overall, these findings present a fundamental mechanism for the precise regulation of subcellular sterol synthesis, potentially inspiring therapeutic approaches for cholesterol-related diseases. Finally, we have demonstrated that SRS-GEM imaging of metabolites at the subcellular level is an enabling technology to provide new insights into metabolic processes.
Data availability statement
The original contributions presented in the study are included in the article/Supplementary Material; further inquiries can be directed to the corresponding authors.
Author contributions
DZ, HJL, and CY conceived the idea and designed experiments. YoZ and DZ constructed the imaging platform. YoZ, YiZ, and WF performed experiments and analyzed data. DZ, HJL, and YoZ wrote the manuscript with input from all authors.
Funding
This work was supported by the National Natural Science Foundation of China (Research Fund for International Young Scientists, 32050410293), the Fundamental Research Funds for the Central Universities (2020QNA5027), and MOE Frontier Science Center for Brain Science and Brain-Machine Integration of Zhejiang University, Zhejiang University 100-Young Professor startup fund to HJL; the National Natural Science Foundation of China (General Program, 12074339) to DZ; and the National Natural Science Foundation of China (92057102) and a research fund from the Zhejiang Provincial Key Laboratory for Cancer Molecular Cell Biology to CY.
Conflict of interest
The authors declare that the research was conducted in the absence of any commercial or financial relationships that could be construed as a potential conflict of interest.
Publisher’s note
All claims expressed in this article are solely those of the authors and do not necessarily represent those of their affiliated organizations, or those of the publisher, the editors, and the reviewers. Any product that may be evaluated in this article, or claim that may be made by its manufacturer, is not guaranteed or endorsed by the publisher.
Supplementary material
The Supplementary Material for this article can be found online at: https://www.frontiersin.org/articles/10.3389/fchem.2023.1166313/full#supplementary-material
References
Argov, N., Wachsmann-Hogiu, S., Freeman, S. L., Huser, T., Lebrilla, C. B., and German, J. B. (2008). Size-dependent lipid content in human milk fat globules. J. Agric. Food Chem. 56, 7446–7450. doi:10.1021/jf801026a
Basson, M. E., Thorsness, M., and Rine, J. (1986). Saccharomyces cerevisiae contains two functional genes encoding 3-hydroxy-3-methylglutaryl-coenzyme A reductase. Proc. Natl. Acad. Sci. U. S. A. 83, 5563–5567. doi:10.1073/pnas.83.15.5563
Berto, P., Scotté, C., Galland, F., Rigneault, H., and de Aguiar, H. B. (2017). Programmable single-pixel-based broadband stimulated Raman scattering. Opt. Lett. 42, 1696–1699. doi:10.1364/ol.42.001696
Burg, J. S., and Espenshade, P. J. (2011). Regulation of HMG-CoA reductase in mammals and yeast. Prog. Lipid Res. 50, 403–410. doi:10.1016/j.plipres.2011.07.002
Cameron, J. S., Moro, F., and Simmonds, H. A. (1993). Gout, uric acid and purine metabolism in paediatric nephrology. Pedia 7, 105–118. doi:10.1007/bf00861588
Casey, W. M., Keesler, G. A., and Parks, L. W. (1992). Regulation of partitioned sterol biosynthesis in Saccharomyces cerevisiae. J. Bacteriol. 174, 7283–7288. doi:10.1128/jb.174.22.7283-7288.1992
Chen, X., Cui, S., Yan, S., Zhang, S., Fan, Y., Gong, Y., et al. (2021). Hyperspectral stimulated Raman scattering microscopy facilitates differentiation of low-grade and high-grade human prostate cancer. J. Phys. D. 54, 484001. doi:10.1088/1361-6463/ac2175
Cheng, J. X., and Xie, X. S. (2015). Vibrational spectroscopic imaging of living systems: An emerging platform for biology and medicine. Science 350, aaa8870. doi:10.1126/science.aaa8870
Choudhary, V., and Schneiter, R. (2012). Pathogen-Related Yeast (PRY) proteins and members of the CAP superfamily are secreted sterol-binding proteins. Proc. Natl. Acad. Sci. U. S. A. 109, 16882–16887. doi:10.1073/pnas.1209086109
Chung, C. Y., and Potma, E. O. (2013). Biomolecular imaging with coherent nonlinear vibrational microscopy. Annu. Rev. Phys. Chem. 64, 77–99. doi:10.1146/annurev-physchem-040412-110103
Daum, G., Lees, N. D., Bard, M., and Dickson, R. (1998). Biochemistry, cell biology and molecular biology of lipids of Saccharomyces cerevisiae. Yeast 14, 1471–1510. doi:10.1002/(sici)1097-0061(199812)14:16<1471:aid-yea353>3.0.co;2-y
Dezaki, K., Sone, H., and Yada, T. (2008). Ghrelin is a physiological regulator of insulin release in pancreatic islets and glucose homeostasis. Pharmacol 118, 239–249. doi:10.1016/j.pharmthera.2008.02.008
Du, J., Wang, H., and Wei, L. (2022). Bringing vibrational imaging to chemical biology with molecular probes. ACS Chem. Biol. 17, 1621–1637. doi:10.1021/acschembio.2c00200
Federovitch, C. M., Jones, Y. Z., Tong, A. H., Boone, C., Prinz, W. A., and Hampton, R. Y. (2008). Genetic and structural analysis of Hmg2p-induced endoplasmic reticulum remodeling in Saccharomyces cerevisiae. Mol. Biol. Cell 19, 4506–4520. doi:10.1091/mbc.e07-11-1188
Freudiger, C. W., Min, W., Saar, B. G., Lu, S., Holtom, G. R., He, C., et al. (2008). Label-free biomedical imaging with high sensitivity by stimulated Raman scattering microscopy. Science 322, 1857–1861. doi:10.1126/science.1165758
Fu, D., Holtom, G., Freudiger, C., Zhang, X., and Xie, X. S. (2013). Hyperspectral imaging with stimulated Raman scattering by chirped femtosecond lasers. J. Phys. Chem. B 117, 4634–4640. doi:10.1021/jp308938t
Fu, D., Lu, F. K., Zhang, X., Freudiger, C., Pernik, D. R., Holtom, G., et al. (2012). Quantitative chemical imaging with multiplex stimulated Raman scattering microscopy. J. Am. Chem. Soc. 134, 3623–3626. doi:10.1021/ja210081h
Gatta, A. T., Wong, L. H., Sere, Y. Y., Calderón-Noreña, D. M., Cockcroft, S., Menon, A. K., et al. (2015). A new family of StART domain proteins at membrane contact sites has a role in ER-PM sterol transport. eLife 4, e07253. doi:10.7554/elife.07253
George, K. S., and Wu, S. (2012). Lipid raft: A floating island of death or survival. Toxicol. Appl. Pharmacol. 259, 311–319. doi:10.1016/j.taap.2012.01.007
Gillies, R. J., Robey, I., and Gatenby, R. A. (2008). Causes and consequences of increased glucose metabolism of cancers. J. Nucl. Med. 49 (2), 24S–42S. doi:10.2967/jnumed.107.047258
Grundy, S. M., Stone, N. J., Bailey, A. L., Beam, C., Birtcher, K. K., Blumenthal, R. S., et al. (2019). 2018 AHA/ACC/AACVPR/AAPA/ABC/ACPM/ADA/AGS/APhA/ASPC/NLA/PCNA guideline on the management of blood cholesterol. J. Am. Coll. Cardiol. 73, e285–e350. doi:10.1016/j.jacc.2018.11.003
Hariri, H., Rogers, S., Ugrankar, R., Liu, Y. L., Feathers, J. R., and Henne, W. M. (2018). Lipid droplet biogenesis is spatially coordinated at ER-vacuole contacts under nutritional stress. EMBO Rep. 19, 57–72. doi:10.15252/embr.201744815
Hotamisligil, G. S. (2006). Inflammation and metabolic disorders. Nature 444, 860–867. doi:10.1038/nature05485
Hu, Z., He, B., Ma, L., Sun, Y., Niu, Y., and Zeng, B. (2017). Recent advances in ergosterol biosynthesis and regulation mechanisms in Saccharomyces cerevisiae. Indian J. Microbiol. 57, 270–277. doi:10.1007/s12088-017-0657-1
Huang, K. C., Li, J., Zhang, C., Tan, Y., and Cheng, J. X. (2020). Multiplex stimulated Raman scattering imaging cytometry reveals lipid-rich protrusions in cancer cells under stress condition. iScience 23, 100953. doi:10.1016/j.isci.2020.100953
Jung, Y. Y., Ko, J. H., Um, J. Y., Chinnathambi, A., Alharbi, S. A., Sethi, G., et al. (2021). LDL cholesterol promotes the proliferation of prostate and pancreatic cancer cells by activating the STAT3 pathway. J. Cell. Physiol. 236, 5253–5264. doi:10.1002/jcp.30229
Karanja, C. W., Hong, W., Younis, W., Eldesouky, H. E., Seleem, M. N., and Cheng, J. X. (2017). Stimulated Raman imaging reveals aberrant lipogenesis as a metabolic marker for azole-resistant Candida albicans. Anal. Chem. 89, 9822–9829. doi:10.1021/acs.analchem.7b01798
Kaul, D. (2003). Cholesterol-receptor-mediated genomics in health and disease. Trends. Mol. Med. 9, 442–449. doi:10.1016/j.molmed.2003.08.010
Kishida, T., Kostetskii, I., Zhang, Z., Martinez, F., Liu, P., Walkley, S. U., et al. (2004). Targeted mutation of the MLN64 START domain causes only modest alterations in cellular sterol metabolism. J. Biol. Chem. 279, 19276–19285. doi:10.1074/jbc.m400717200
Koning, A. J., Roberts, C. J., and Wright, R. L. (1996). Different subcellular localization of Saccharomyces cerevisiae HMG-CoA reductase isozymes at elevated levels corresponds to distinct endoplasmic reticulum membrane proliferations. Mol. Biol. Cell 7, 769–789. doi:10.1091/mbc.7.5.769
Laughton, J. D., Charnay, Y., Belloir, B., Pellerin, L., Magistretti, P. J., and Bouras, C. (2000). Differential messenger RNA distribution of lactate dehydrogenase LDH-1 and LDH-5 isoforms in the rat brain. Neuroscience 96, 619–625. doi:10.1016/s0306-4522(99)00580-1
Lee, H. J., Zhang, W., Zhang, D., Yang, Y., Liu, B., Barker, E. L., et al. (2015). Assessing cholesterol storage in live cells and C. elegans by stimulated Raman scattering imaging of phenyl-diyne cholesterol. Sci. Rep. 5, 7930. doi:10.1038/srep07930
Liao, C. S., Wang, P., Wang, P., Li, J., Lee, H. J., Eakins, G., et al. (2015). Spectrometer-free vibrational imaging by retrieving stimulated Raman signal from highly scattered photons. Sci. Adv. 1, e1500738. doi:10.1126/sciadv.1500738
Lin, H., Lee, H. J., Tague, N., Lugagne, J. B., Zong, C., Deng, F., et al. (2021). Microsecond fingerprint stimulated Raman spectroscopic imaging by ultrafast tuning and spatial-spectral learning. Nat. Commun. 12, 3052. doi:10.1038/s41467-021-23202-z
Lin, H., Liao, C. S., Wang, P., Kong, N., and Cheng, J. X. (2018). Spectroscopic stimulated Raman scattering imaging of highly dynamic specimens through matrix completion. Light Sci. Appl. 7, 17179. doi:10.1038/lsa.2017.179
Maciejak, A., Leszczynska, A., Warchol, I., Gora, M., Kaminska, J., Plochocka, D., et al. (2013). The effects of statins on the mevalonic acid pathway in recombinant yeast strains expressing human HMG-CoA reductase. BMC Biotechnol. 13, 68. doi:10.1186/1472-6750-13-68
Min, W., Freudiger, C. W., Lu, S., and Xie, X. S. (2011). Coherent nonlinear optical imaging: Beyond fluorescence microscopy. Annu. Rev. Phys. Chem. 62, 507–530. doi:10.1146/annurev.physchem.012809.103512
Mo, C., Valachovic, M., and Bard, M. (2004). The ERG28-encoded protein, Erg28p, interacts with both the sterol C-4 demethylation enzyme complex as well as the late biosynthetic protein, the C-24 sterol methyltransferase (Erg6p). Biochim. Biophys. Acta Biomembr. 1686, 30–36. doi:10.1016/j.bbalip.2004.08.001
Mok, H. Y., von Bergmann, K., and Grundy, S. M. (1979). Effects of continuous and intermittent feeding on biliary lipid outputs in man: Application for measurements of intestinal absorption of cholesterol and bile acids. J. Lipid Res. 20, 389–398. doi:10.1016/s0022-2275(20)40622-4
Okotrub, K. A., Shamaeva, D. V., and Surovtsev, N. V. (2022). Raman spectra of deuterated hydrocarbons for labeling applications. J. Raman Spectrosc 53, 297–309. doi:10.1002/jrs.6279
Peckett, A. J., Wright, D. C., and Riddell, M. C. (2011). The effects of glucocorticoids on adipose tissue lipid metabolism. Metab 60, 1500–1510. doi:10.1016/j.metabol.2011.06.012
Porter, J. A., Young, K. E., and Beachy, P. A. (1996). Cholesterol modification of hedgehog signaling proteins in animal development. Science 274, 255–259. doi:10.1126/science.274.5285.255
Ramkumar, S., Raghunath, A., and Raghunath, S. (2016). Statin therapy: Review of safety and potential side effects. Acta Cardiol. Sin. 32, 631–639. doi:10.6515/acs20160611a
Riscal, R., Skuli, N., and Simon, M. C. (2019). Even cancer cells watch their cholesterol. Mol. Cell. 76, 220–231. doi:10.1016/j.molcel.2019.09.008
Rogers, S., Hariri, H., Wood, N. E., Speer, N. O., and Henne, W. M. (2021). Glucose restriction drives spatial reorganization of mevalonate metabolism. eLife 10, e62591. doi:10.7554/elife.62591
Sakamoto, K., and Kimura, J. (2013). Mechanism of statin-induced rhabdomyolysis. J. Pharmacol. Sci. 123, 289–294. doi:10.1254/jphs.13r06cp
Shimano, H., Shimomura, I., Hammer, R. E., Herz, J., Goldstein, J. L., Brown, M. S., et al. (1997). Elevated levels of SREBP-2 and cholesterol synthesis in livers of mice homozygous for a targeted disruption of the SREBP-1 gene. J. Clin. Invest. 100, 2115–2124. doi:10.1172/jci119746
Söderholm, S., Roos, Y. H., Meinander, N., and Hotokka, M. (1999). Raman spectra of fructose and glucose in the amorphous and crystalline states. J. Raman Spectrosc 30, 1009–1018. doi:10.1002/(sici)1097-4555(199911)30:11<1009:aid-jrs436>3.0.co;2-#
Van Dijken, J. P., Bauer, J., Brambilla, L., Duboc, P., Francois, J. M., Gancedo, C., et al. (2000). An interlaboratory comparison of physiological and genetic properties of four Saccharomyces cerevisiae strains. Enzyme Microb. Technol. 26, 706–714. doi:10.1016/s0141-0229(00)00162-9
Vance, J. E., Hayashi, H., and Karten, B. (2005). Cholesterol homeostasis in neurons and glial cells. Semin. Cell Dev. Biol. 16, 193–212. doi:10.1016/j.semcdb.2005.01.005
Wei, L., Hu, F., Chen, Z., Shen, Y., Zhang, L., and Min, W. (2016). Live-cell bioorthogonal chemical imaging: Stimulated Raman scattering microscopy of vibrational probes. Accounts Chem. Res. 49, 1494–1502. doi:10.1021/acs.accounts.6b00210
Wei, L., Hu, F., Shen, Y., Chen, Z., Yu, Y., Lin, C.-C., et al. (2014). Live-cell imaging of alkyne-tagged small biomolecules by stimulated Raman scattering. Nat. methods 11, 410–412. doi:10.1038/nmeth.2878
Wei, L., Shen, Y., Xu, F., Hu, F., Harrington, J. K., Targoff, K. L., et al. (2015). Imaging complex protein metabolism in live organisms by stimulated Raman scattering microscopy with isotope labeling. ACS Chem. Biol. 10, 901–908. doi:10.1021/cb500787b
Yamauchi, Y., and Rogers, M. A. (2018). Sterol metabolism and transport in atherosclerosis and cancer. Front. Endocrinol. 9, 509. doi:10.3389/fendo.2018.00509
Ye, C., Bandara, W. M., and Greenberg, M. L. (2013). Regulation of inositol metabolism is fine-tuned by inositol pyrophosphates in Saccharomyces cerevisiae. J. Biol. Chem. 288, 24898–24908. doi:10.1074/jbc.m113.493353
Yen, K., Le, T. T., Bansal, A., Narasimhan, S. D., Cheng, J. X., and Tissenbaum, H. A. (2010). A comparative study of fat storage quantitation in nematode Caenorhabditis elegans using label and label-free methods. PLoS One 5, e12810. doi:10.1371/journal.pone.0012810
Yue, S., Li, J., Lee, S. Y., Lee, H. J., Shao, T., Song, B., et al. (2014). Cholesteryl ester accumulation induced by PTEN loss and PI3K/AKT activation underlies human prostate cancer aggressiveness. Cell Met. 19, 393–406. doi:10.1016/j.cmet.2014.01.019
Yutuc, E., Angelini, R., Baumert, M., Mast, N., Pikuleva, I., Newton, J., et al. (2020). Localization of sterols and oxysterols in mouse brain reveals distinct spatial cholesterol metabolism. Proc. Natl. Acad. Sci. U. S. A. 117, 5749–5760. doi:10.1073/pnas.1917421117
Zhang, D., Slipchenko, M. N., and Cheng, J. X. (2011). Highly sensitive vibrational imaging by femtosecond pulse stimulated Raman loss. J. Phys. Chem. Lett. 2, 1248–1253. doi:10.1021/jz200516n
Zhang, D., Slipchenko, M. N., Leaird, D. E., Weiner, A. M., and Cheng, J.-X. (2013). Spectrally modulated stimulated Raman scattering imaging with an angle-to-wavelength pulse shaper. Opt. express 21, 13864–13874. doi:10.1364/oe.21.013864
Zhang, D., Wang, P., Slipchenko, M. N., and Cheng, J. X. (2014). Fast vibrational imaging of single cells and tissues by stimulated Raman scattering microscopy. Acc. Chem. Res. 47, 2282–2290. doi:10.1021/ar400331q
Keywords: stimulated Raman scattering (SRS) microscopy, metabolite imaging, biophysics, cholesterol, genetic engineering, HMGCoA reductase, yeast
Citation: Zhang Y, Zhou Y, Fang W, Zhu H, Ye C, Zhang D and Lee HJ (2023) Spatial sterol metabolism unveiled by stimulated Raman imaging. Front. Chem. 11:1166313. doi: 10.3389/fchem.2023.1166313
Received: 15 February 2023; Accepted: 13 March 2023;
Published: 29 March 2023.
Edited by:
Youhui Lin, Xiamen University, ChinaCopyright © 2023 Zhang, Zhou, Fang, Zhu, Ye, Zhang and Lee. This is an open-access article distributed under the terms of the Creative Commons Attribution License (CC BY). The use, distribution or reproduction in other forums is permitted, provided the original author(s) and the copyright owner(s) are credited and that the original publication in this journal is cited, in accordance with accepted academic practice. No use, distribution or reproduction is permitted which does not comply with these terms.
*Correspondence: Cunqi Ye, eWVjdW5xaUB6anUuZWR1LmNu; Delong Zhang, ZGx6aGFuZ0B6anUuZWR1LmNu; Hyeon Jeong Lee, aGpsZWVAemp1LmVkdS5jbg==
†These authors have contributed equally to this work