- 1UNICAMP Materials Simulation Lab, Institute of Chemistry, Department of Physical-Chemistry, University of Campinas, Campinas, Brazil
- 2Química Teórica y Computacional, Department de Química Física i Analítica, Universitat Jaume I, Castellón de la Plana, Spain
In this work, first-principles calculations by using density functional theory at the GFN-xTB level, are performed to investigate the relative stability and structural, electronic, and magnetic properties of bimetallic Pt13−nNin (n = 0, 3, 6, 9, 13) nanoclusters by using corrected Hammer and Nørskov model. In addition, by employing the reaction path and the energetic span models, the energy profile and the turnover frequency are calculated to disclose the corresponding reaction mechanism of the water-gas shift reaction catalyzed by these nanoclusters. Our findings render that Ni causes an overall shrinking of the nanocluster’s size and misalignment of the spin channels, increasing the magnetic nature of the nanoclusters. Pt7Ni6 nanocluster is the most stable as a result of the better coupling between the Pt and Ni d-states. Pt4Ni9 maintains its structure over the reaction cycle, with a larger turnover frequency value than Pt7Ni6. On the other hand, despite Pt10Ni3 presenting the highest value of turnover frequency, it suffers a strong structural deformation over the completion of a reaction cycle, indicating that the catalytic activity can be altered.
1 Introduction
Catalysis plays a pivotal role in all strategies for establishing energy- and atom-efficient sustainable chemical technologies. One of the green chemistry goals is to find alternatives for the production of green fuels that either do not contribute to the increase of atmospheric CO2 concentration or reuse CO2 in the industrial chain. Hydrogen gas, H2(g), is a storable and green form of chemical energy with many practical applications (Zou and Zhang, 2015). The water-gas shift (WGS) reaction, CO(g) + H2O(g) → CO2(g) + H2(g), provides an important process for the H2(g) production (Yao et al., 2017), and also it is the main path for sequestrating CO(g), a toxic gas responsible for causing death from asphyxiation (Blumenthal, 2001). Nevertheless, the literature suggests that WGS can be employed for carbon-capturing, aiming to produce acetic acid from activated CO2(g) (Somiari and Manousiouthakis, 2017). To reach high reaction activity, the design of efficient catalysts is important (Beller et al., 2010; Jeong et al., 2013; Lin et al., 2013; Roşca et al., 2015; Zhang et al., 2016).
Heterogeneous catalytic processes generally include reactant molecular adsorption, dissociated atom reactions at the catalyst surface, and product molecular desorption. In particular, the reaction mechanism of the WGS reaction over metal surfaces might occur at least along eight steps to turn a complete cycle over the surface of the metal catalyst (Eqs 1–8), in which CO would be chemically activated first once it is adsorbed to the catalyst surface, followed by the H2O adsorption. Only then, the water hydrolysis would take place via an associative carboxylate mechanism, as shown in Figure 1 (Lian et al., 2015; Baraj et al., 2021).
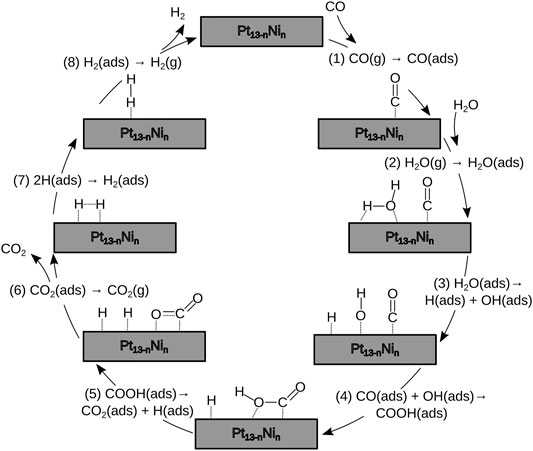
FIGURE 1. Associative carboxylate mechanism for the WGS reaction taking place over the nanocluster’s surface. The eight steps correspond to Eqs 1–8.
Designing new catalysts and understanding how they work is a time and resource-consuming process, necessitating an increasing reliance on tools that can predict catalyst performance. Computational techniques mainly employing density functional theory (DFT) methods have been used to study the structure, composition, and properties for the design of new catalysts based on metal alloys (Greeley, 2016). From a theoretical point of view, the d-band center model of Hammer and Nørskov (HN), developed more than 2 decades ago, is widely used for understanding and predicting catalytic activity on transition metal surfaces (Hammer and Nørskov, 1995, 2000; Hammer et al., 1996; Greeley et al., 2002; Xin et al., 2014) and offers a way to find a relationship between the catalytic activity and the characteristics of the electronic d-band profile (Morikawa et al., 1997). In the HN model, the center of the band of the d-states participating in the interaction between the surface atoms and an adsorbate molecule is approximated with a single state at energy ϵd. Such a model can be understood as a narrow d-band limit of the Newns-Anderson model (Anderson, 1961; Newns, 1969), and then suggests how prone the material is to work as a catalyst (Ínoğlu and Kitchin, 2010). The variation of the adsorption energy from one transition metal surface to another might be associated to the upward shift of ϵd with respect to the Fermi energy, i.e., the closer ϵd is to the Fermi level, the stronger the adsorption might be. However, studies have shown that relying solely on ϵd may be misleading; then, the d-band as a whole is used by calculating the d-bandwidth
Another way for assessing the performance of a catalyst is by obtaining its turnover frequency (TOF) by means of the energetic span (ES) model to calculate the energy profile of a reaction mechanism (Christiansen, 1953; Kozuch and Shaik, 2006, 2008; Uhe et al., 2010; Solel et al., 2019). Each reaction step might offer a degree of control for the entire mechanism, which can be used to identify the rate-determining step, known as XTOF. If the XTOF value belongs to an intermediate state, then it is called XTOF of a putative TOF determining intermediate (TDI, XTDI). On the other hand, if that belongs to a transition state, it is labeled as XTOF of a putative TOF determining transition state (TDTS, XTDTS). The values of both XTDI, and XTDTS range from 0 to 1, and the larger the value, the more important the corresponding stage is for the reaction mechanism. Thus, the TOF can be computed once the XTDI and XTDTS values are calculated. This strategy is applied for different reactions in Kozuch’s papers (Kozuch and Shaik, 2006; Kozuch and Shaik, 2008; Uhe et al., 2010; Solel et al., 2019). One key aspect of the ES model that differentiates it from the simple HN model is the possibility of assessing the temperature effects over the catalyzed mechanism. Such an approximation might help better describe the behavior of nanoclusters working as catalysts.
Metal nanoalloys, which are nanostructures consisting of one or more metal species, have received increased attention as their properties can be tuned by varying their composition and size. Nanoalloys exhibit a broad array of chemical ordering patterns depending on the interaction between the constituent metals. Then, they are attractive from a fundamental perspective and often show distinct catalytic properties and stability as compared to individual metals, which is typically ascribed to the coupling effect and/or synergism in the electronic and/or geometric structures that occur when the metals are combined (Ferrando et al., 2008; Tao, 2012; Yan et al., 2017; Ding et al., 2018; Añez et al., 2021; Reboul et al., 2021). Significant efforts have been put into the research field of nanocatalysis to explore the structure, surface composition, and atomic distribution for two metals systems, which are crucial for their properties and catalytic activities of bimetallic nanoalloys (Ferrando et al., 2008; Jellinek, 2008; Piotrowski et al., 2012; Cui et al., 2013; Zhao et al., 2013; Guedes-Sobrinho et al., 2015; Gilroy et al., 2016; Wang et al., 2016; Wu et al., 2019). However, an in-depth understanding of the processes involved in forming these alloy materials is somewhat limited, especially at the atomic level.
From a theoretical point of view, studies have demonstrated that dispersion effects play an important role in the adsorption energy (Davis et al., 2015), leading to subtle changes in the adsorption process, particularly for Pt nanoclusters, which could modify the energetic description of the mechanism. Since the proposal of dispersion correction models such as the Grimme’s family of corrections (Grimme, 2004), many studies have been conducted to investigate the influence of such a description in different systems, from discrete organic molecules to periodic solid materials (Barone et al., 2009; Davis et al., 2015; Kovács et al., 2017). Those works mostly pointed out improvements in computational results when employing generalized gradient approximation (GGA) type functionals allied with dispersion corrections. Recently, Grimme et al. have developed a tight-binding (TB) method parameterized for most elements of the periodic table (Z = 1–86) entitled GFN-xTB (Grimme et al., 2017; Grimme, 2019). It allows a fast and accurate prediction of chemical structures for a variety of species, organic or inorganic materials, and even solid materials of periodic nature, which may offer an alternative path for exploratory analysis of new materials for a broad range of applications including catalysis. Nevertheless, the GFN-xTB presents a set of tools for quick assessment of reaction mechanisms, known as the reaction path (RP) model. The RP model works as a Gaussian biasing potential for both pushing the reactant towards the products and pulling the products towards the reactants (Grimme et al., 2019; Rasmussen and Jensen, 2020).
Previous studies render that both Pt and Ni pure systems are suitable catalysts for WGS reaction, but the former is prone to CO poisoning, and the latter tends to favor a methanation reaction pathway (Senanayake et al., 2011; Alijani and Irankhah, 2013; Ammal and Heyden, 2019; Nakhaei Pour and Tayyari, 2019; Palma et al., 2020). Regarding structural, magnetic, and electronic characteristics of bimetallic Pt13−nNin (n = 0–13) nanoclusters, some studies have shown that Ni does not provide any significant structural deformation but can weak the nanoalloy binding energy and increase its magnetic nature (Jia et al., 2013; Zhao et al., 2013). Concerning the WGS reaction, theoretical studies of PtNi nanoclusters with different atomic quantities of Pt and Ni have been published, exposing the possibility of using those nanoclusters as catalysts (de Souza Monteiro et al., 2008; Lin et al., 2011; Piotrowski et al., 2012; Zhao et al., 2013; Guedes-Sobrinho et al., 2015; Lian et al., 2015; Roduner and Jensen, 2015; Mokkath, 2018). Yet for the WGS reaction, previously computed adsorption energy values suggested that CO adsorption would occur more strongly than H2O on Pt3M (M = Cu, Mo, Ni, Rh) nanoclusters (Lian et al., 2015). However, to the best of our knowledge, many of those studies lack a description of the dispersion forces and mainly use small-sized nanoalloys having from 3 to 4 atoms when conducting an entire reaction cycle. Moreover, a full description spanning from the structural, electronic, and magnetic properties to the reaction mechanism of PtNi nanoalloys for the WGS reaction is scarce in the literature for systems with 13 atoms or more.
Thus, the purpose of this work is threefold. First, we aim to provide insights into the geometrical, electronic, and magnetic nature of Pt13−nNin (n = 0, 3, 6, 9, and 13) nanoclusters based on realistic HN models. The second goal is to rationalize the experimental results and understand the origin of the catalytic performance of these bimetallic nanoalloys for the WGS reaction by using the GFN-xTB method (Eqs 1–8). Finally, the energetic span model has been employed to analyze the influence of each step over the TOF. The results of these calculations can be considered a first-principles guided synthesis for pre-treatments to control the initial surface composition of Pt13−nNin nanoclusters as a model to explore the reaction mechanism for the WGS reaction. This paper contains three more sections. The next section is devoted to the computational methods and model systems. In section three, the results are presented and discussed. The main conclusions are summarized in the fourth and final section.
2 Computational Methods and Model Systems
2.1 Structural and Energetic Analysis
As one of the most stable structures of PtNi nanoclusters lies within 13 atoms regime and icosahedral shape (Watzky and Finke, 1997; Kaatz and Bultheel, 2019), we employed the third level of GFN-xTB family for quickly assessing all possible atomic arrangements within the Pt13−nNin composition (n = 0, 3, 6, 9, and 13). As these nanoclusters are known to have an icosahedral shape it is possible that a simple permutation of all atom positions would lead to symmetrically equivalent configurations, which can be discarded. The generation of all nanocluster structures for different compositions was carried out by an in-house package written in Python. Once the structures were relaxed, their local energy minima were confirmed by computing the hessian at the same theory level. Hence, by employing the Quantum-ESPRESSO (QE) (Giannozzi et al., 2017) package within the generalized gradient approximation (GGA) (Perdew et al., 1992) and using the Perdew-Burke-Ernzerhof (Perdew et al., 1996) functional with D3BJ correction (Grimme et al., 2010; Becke and Johnson, 2005; Johnson and Becke, 2005, 2006), we assessed if at least three samples for each nanocluster composition had a matching energy profile as that found by GFN-xTB (details can be found in the Supplementary Material). Finally, we selected those candidates with the minimal energy value for each composition group.
Concerning the nanocluster structure stability, we computed the binding energy per atom (Eb) as described in Eq. 9 and the excess energy (Eexc) as described in Eq. 10.
Etot, ni, and Ei are the total energy of the nanocluster, the number of times the ith atom appears for the m elements within the nanocluster formula, and its energy as an isolated atom, respectively.
2.2 Electronic Analysis
Through the analysis of the projected density of states (PDOS) we assessed the magnetic nature of the nanoclusters, as well as the d-band center (ϵd), and bandwidth
ϵd is taken as the energy average of the d-band for the electronically occupied fraction, comprising all the density of states (DOS) from negative infinity to the Fermi level (ϵf). For
To compute fd one can simply integrate the PDOS of the d-band of the metal atom assuming the sum of both spin channels, spin-up (α), and spin-down (β). Moreover, the HN model also proposed a corrected value (see Eq. 13), which considers the bandwidth to enhance the d-band center location with respect to the shifts observed in the bandwidth (Nørskov et al., 2014).
However, as already pointed out by previous studies (Bhattacharjee et al., 2016), the HN model suffers from not describing the spin polarization role in lowering the d-band center. Hence, an effective d-band center
ϵd↑, ϵd↓, fd↑, fd↓, represent the d-band center and fractional occupancy for the up, and down spin channels, respectively.
2.3 Reaction Path
The RP model works as a Gaussian biasing potential that pushes the reactants towards the products in a stepwise manner; every pushing step is followed by a pulling potential that forces the products back to the reactants. For each step, an optimization is made in order to minimize the total system energy (Etot) as given by Eq. 15.
in which
Under the description of the RP model, we analyze the different steps, 1–8, taking place over the nanoclusters’ surface for n = 3, 6, and 9. Since the RP model uses biasing parameters, we have optimized those values to perform the reaction path as already suggested in the literature (Rasmussen and Jensen, 2020). Herein, the values of kpush = 0.01 Eh, kpull = 0.02 Eh, and α = 0.03 Bohr−1 were employed for the RP model.
All posible adsorption sites were examined for each explored reaction by employing an in-house program written in Python. The routine was set to identify the best adsorption sites, i.e., which adsorption would lead to the lowest energy. Then, the values of the adsorption energy of CO at different sites (Pt, Ni, and PtNi, PtPt and NiNi bridge, and in a hollow position spanning three atoms at a time as Pt3, Pt2Ni, or PtNi2) for each cluster are calculated.
Once the minimal energy paths were explored through the RP model, we computed the zero-point energy (ZPE) for energy corrections, and the transition structures were assessed by verifying they only presented one imaginary frequency.
2.4 Assessing the Catlytic Cycle
In a steady-state regime, the TOF can be computed as the net rate of product formation given by Eq 16 (Kozuch and Shaik, 2006, 2008):
the transition state (Ti), intermediate (Ij), and reaction energy (ΔErx) are given in kbT units, in which a specific temperature value can be set for assessing its effect over the TOF value, as shown by Eq. 17a, Eq. 17b, and Eq. 17c.
where E (Ti) and E (Ij) represent the energy of the ith and jth transition and intermediate states, respectively.
Despite the sum of all states found in a reaction cycle seen in the denominator term of Eq. 16, not all reaction steps contribute equally for the energy landscape of the reaction cycle, i.e., some transition states can be higher in energy than others, as some intermediates might be lower in energy. Therefore, it is possible to compute how each step contributes to the overall reaction energy profile as a transition state (Eq. 18) or intermediate (Eq. 19).
Values from 0 to 1 are computed for
That approximation can be useful for systems that present few rate-determining states and has been shown to give similar results as Eq. 16 (Kozuch and Shaik, 2006, 2008; Kozuch and Matrin, 2011; Solel et al., 2019).
That being said, we assessed each catalytic cycle by employing the ES model within a range of temperatures varying from 700 to 1000 K, with the temperature step of 5 K. As a final remark to verify the nanocluster’s capability of recovering its initial state, we assessed the RMSD for the nanocluster’s structure in each reaction step. In other words, by aligning only the metal atoms that compose the nanocluster, we verified the structural deformation that each nanocluster underwent after a complete reaction cycle.
The RMSD value was computed as shown by Eq 21, where the sum of the simple euclidean distances of each Cartesian coordinate of the target structure (β) with respect to the reference model (α) is assessed for each i atom. The initial nanocluster structure was chosen as the reference model, which returns an RMSD value of zero for the first step. The higher the RMSD value for the following steps, the greater the nanocluster deformation will be with respect to the initial nanocluster structure.
3 Results
3.1 Nanocluster Structures
The first step consisted of generating all unique structures for each nanocluster composition. Then, these structures were relaxed using the GFN-xTB method, and only positive frequencies were found after the optimization step; thus, structures with the lowest energy were selected to proceed to the next stages. A pool of nanocluster candidates was obtained for each composition (see Supplementary Table S1).
Figure 2 shows the relaxed nanoclusters and the corresponding electrostatic potential surface (EPS), respectively, where the number of Ni atoms is shown along with the related symmetry group. It is important to notice that the chosen structures under the GFN-xTB, which went to the DFT-D3BJ optimization, were all Ni-centered. Since only the lowest energy structures were selected, it is interesting that a low level of optimization sampled the Ni-centered structures in accordance with a previous study showing that those nanoclusters show lower energy than those Pt-centered (Zhao et al., 2013). The stability of the nanoclusters can be quantified directly from the values of Eb and Eexc. The former shows the binding strength among the atoms within the structure, and low values indicate that the atoms are bound strongly. The Eexc measures the energy associated with the alloying process, and low values imply more stable structures.
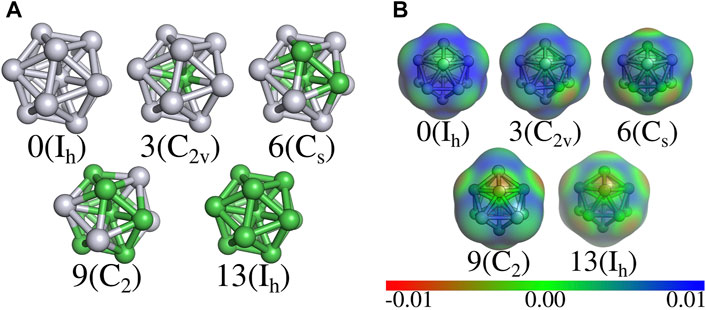
FIGURE 2. Optimized structures of the Pt13−nNin (n = 0, 3, 6, 9, 13) nanoclusters (A) and electrostatic potential surface (EPS), in a. u, of the optimized structures (B). Number of Ni atoms shown below each nanocluster structure along with the group symmetry in parenthesis. The icosahedral shape is kept for n = 0 and n = 13, as for n = 3, 6, and 9 Ni is conserved as the central atom. For n = 9 there are two Pt pair of atoms islanding at opposite nanocluster corners. For n = 3, 6, 9, and 13 an increasing number of red spots on the EPS can be observed as consequence of Ni doping process. Light gray and green colors represent platinum and nickel atoms, respectively.
As shown in Table 1, the Ni doping process increases the value of Eb from -3.26 eV to -2.81 eV; as for the alloying stability, the most stable structure is reached with Pt7Ni6 with -0.1961 eV. Yet, for the average atomic distances, there is a significant increase in Pt–Pt distance for the Pt4Ni9 composition due to the islanding of the Pt atoms into two pair groups over the structure vertices (Figure 2A). Concerning the nanocluster structure, a slightly shrinking can be observed when doping with Ni as the structures with n = 3, 6, and 9, which show a constant decrease of the average atomic distances for Pt–Ni. On the other hand, the average Ni–Ni atomic distances increase from 2.35 Å to 2.43 Å. Overall, the Ni-rich structures might cause the whole system to shrink as the average atomic distance value from Pt13 is 2.74 Å, and 2.43 Å for Ni13. A consequence of the nanocluster’s shrinking is the increase of charged spots over its surface, as shown in Figure 2B. Our findings agree with previous work (Zhao et al., 2013).
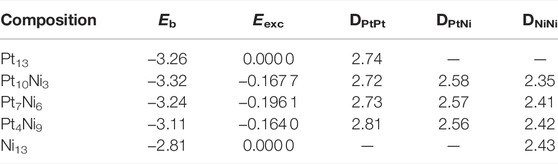
TABLE 1. Calculated values of Eb, Eexc in eV/atom and average atomic distances in Å for each nanocluster composition.
3.2 Electronic Analysis
The literature pointed out that Pt13−nNin nanoclusters have a stronger d-band coupling between Pt-5d and Ni-3d as the Ni content increases (Zhao et al., 2013). Hence, it is helpful to analyze the PDOS for the metal d-states to understand the nanocluster stability from an electronic perspective. As presented in Figure 3, for the nanoclusters with n ranging from 3 to 9, the resemblance of the Pt-5d and Ni-3d reaches a matching profile at n = 6. Nevertheless, for n = 3 and 9, there is a higher contribution from Pt and Ni to the overall DOS profile, respectively. Therefore, a weaker coupling between the Pt and Ni d-states is expected for n = 3 and 9, following a higher value of Eexc. In addition, an analysis of PDOS renders the presence of anisotropic spin-up (α) and spin-down (β) channels. This result suggests that a magnetic character for all nanocluster compositions could come from this misalignment because a noticeable shift towards a more magnetic character appears as a result of Ni doping: the β channel moves towards the Fermi level (Ef), which might cause a decrease in the β channel electronic population when compared to the channel as shown in Table 2.
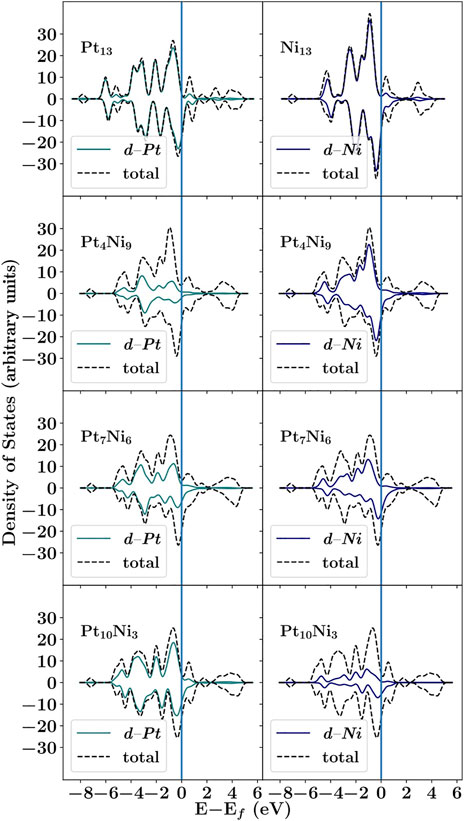
FIGURE 3. PDOS for each nanocluster composition. Pt and Ni d-state component is shown at the left and the right side, respectively.
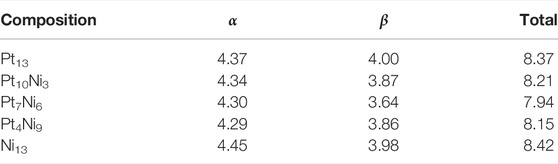
TABLE 2. Electronic population for each spin channel of the d-band averaged by atom for each Pt13−nNin (n = 0, 3, 6, 9, 13) nanocluster.
It is already known that Pt has significant magnetic nature (Paola et al., 2017; Albert and Rubin, 1964; Zhao et al., 2013; Du et al., 2014; Wang and Johnson, 2007). Also, experiments employing superconducting quantum interference device and x-ray magnetic circular dichroism have shown a magnetic behavior for Pt13 when supported inside zeolite pores (Roduner, 2014; Roduner and Jensen, 2015; Liu et al., 2006; Bartolomé et al., 2009), although these systems may differ from the perspective of the free nanoclusters in vacuum as discussed in the literature (Paola et al., 2017). Nonetheless, it is also reported that other metal nanoclusters from the third transition series exhibit magnetic character at a low size regime (Yamamoto et al., 2003; Roduner, 2014). Indeed, an analysis of the results shown in Table 3 renders that our computed values for the total magnetic moment (mT) are in close agreement with reported values in the literature (Bartolomé et al., 2009; Zhao et al., 2013; Du et al., 2014; Paola et al., 2017).
Despite the magnetic behavior of those nanoclusters, the total electronic population for the metal d-states is roughly conserved. As proposed by the HN model, the conservation of electronic population causes a narrowing of d-bandwidth
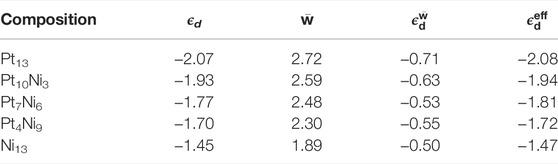
TABLE 4. Computed values in eV of d-band center (ϵd), bandwidth
The changes in the electronic distribution of nanoclusters suggest that Ni doping makes the nanocluster prone to a more effective d-states coupling with the σ-states from the adsorbates. As stated by the HN model, upshifting the ϵd towards the Fermi level of the catalyst surface could favor stronger adsorption (Kitchin et al., 2004). Yet, according to Sabatier principle there is a relationship between the catalyst activity and the strength a substrate adsorbs to a surface. It states that the binding energy between the catalyst’s surface and the substrate should not be too strong or too weak (Ooka et al., 2021). Hence, as the d-band center suggests how strong an adsorption would occur, it can be useful for indicating which nanocluster would show a better catalytic activity.
Considering the computed values of d-band center in Table 4, it could be suggested that the catalytic activity for the studied nanoclusters follows the order: (1) Ni13
3.3 Reaction Mechanisms
For a reaction mechanism in which CO and H2O will be adsorbed over a catalyst surface, a better d-σ coupling would lead to an easier activation of the reactants and a catalytic enhancement. In the following, we investigate the catalytic mechanism to determine which nanocluster composition is the favorable catalyst. By taking advantage of the RP model, we quickly assessed all the steps needed for the complete cycle of the WGS reaction through the carboxylate route (Eqs 1–8, see Figure 1). Despite the proposed mechanism shown in Eqs 1–8), steps 5 and 7 would be feasible to occur over a surface where there is enough room for the segregation process of hydrogen proton. However, in our calculations, we have observed that this step occurs in a concerted manner, in which the deprotonation process of COOH takes place as the H–H bond is formed.
The computed free energy profile for the complete reaction mechanism is shown in Figure 4. The steps from I0 to I1 and from I1 to I2 correspond to the adsorption processes of CO and H2O, respectively, while the steps I5 to I6, and from I6 to I7 correspond to the desorption processes of CO2 and H2, respectively. The WGS reaction then takes place from I2 to I5, and the transition steps are highlighted as TS1→3 (imaginary frequencies of TS are available in the SI). Figure 5 presents the structures from steps I2 to I5 along the values of some selected distances are also reported. As can be seen, for Pt10Ni3 and Pt4Ni9 nanoclusters, the TS3 step occurs in a roughly concerted manner, in which the hydrogen proton leaves the COOH as it orients itself towards the H–H formation. However, for Pt7Ni6, the hydrogen proton is slightly kept adsorbed above a Ni atom.
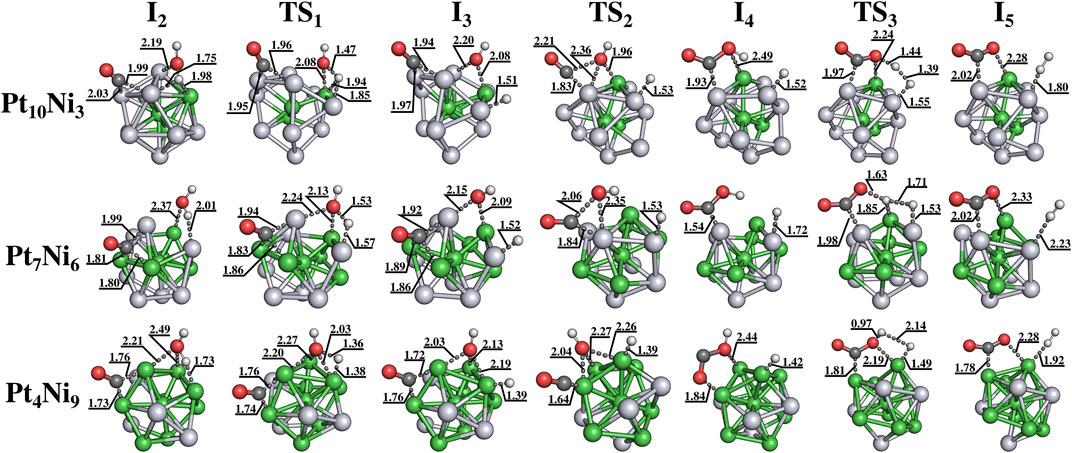
FIGURE 5. Structures of the stationary points from I2 to I5 for each nanocluster composition, Pt13−nNin for n = 3, 6, and 9. The interatomic distances are given in Å. Atoms were colored in white, red, dark gray, light gray and green for representing hydrogen, oxygen, carbon, platinum and nickel, respectively.
One key aspect to know about the catalytic mechanism is the influence that a specific step can have over the TOF values (Kozuch and Shaik, 2006). It might be possible that not only one particular pair of steps roles out the entire mechanism, thus it may be reasonable to observe the degree of control that each step has over the TOF (see Table 5). Interestingly, for n = 3 and 6, the TDTS is the TS3 step, but changes to TS2 for n = 9. As for the TDI, I3 is kept for all compositions. Indeed, the lowest energy step corresponds to where H2O has been split over the nanocluster’s surface. However, the most critical reaction step that imposes the inner catalytic energy barrier (δE) for n = 3 and 6 corresponds to the proton transfer occurring at TS3, as for n = 9 it is the carboxylic acid formation at TS2.
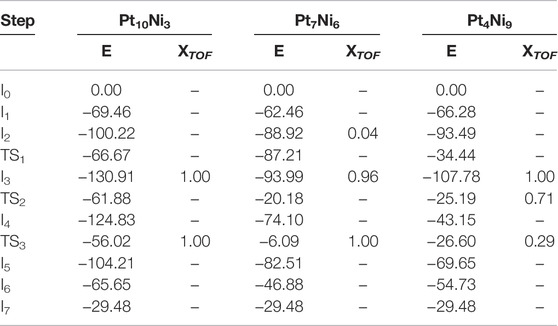
TABLE 5. Degree of TOF control for each nanocluster composition within n = 3, 6, and 9. Values of energy (E) given in kJ/mol, XTOF are dimensionless units.
Despite those changes seen for the TDTS for n = 9, it is important to notice that TS3 still imposes a resistance of almost 29% to the reaction process. In other words, between TS2 and TS3, one might seek strategies to change the catalytic process to reduce the TS2 energy barrier to improve the catalytic process of the mechanism where Pt4Ni9 has been applied. Not only the contribution values of each TDTS and TDI for each nanocluster composition were assessed, but also the TOF value for each system under different temperatures ranging from 750 to 1050 K. As shown in Figure 6, the expected catalytic activity of the studied nanoclusters would be arranged as Pt7Ni6 < Pt4Ni9 < Pt10Ni3. From the HN model, the catalytic activity would be placed as Pt4Ni9 < Pt7Ni6 < Pt10Ni3 for ϵd and
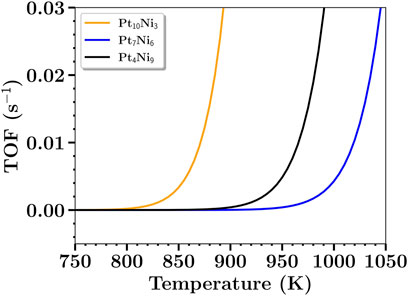
FIGURE 6. Calculated values of TOF for nanoclusters compositions of n = 3, 6, and 9 for a temperature window of 750–1050 K.
Another important feature of a catalyst is its ability to be recovered at the end of a reaction cycle, meaning that the catalyst will return to its initial structural condition. Hence, to understand whether the nanoclusters return to their original state, we investigated the structural changes of the nanoclusters in the catalytic cycle. In that regard, Table 6 summarizes the RMSD for each nanocluster composition concerning the first step I0. Thus, Pt10Ni3 is more affected from structural deformations than the other nanocluster compositions. Therefore, despite the suggested good catalytic performance of Pt10Ni3 according to the ES and corrected HN models, it might not hold once a few catalytic cycles have been completed.
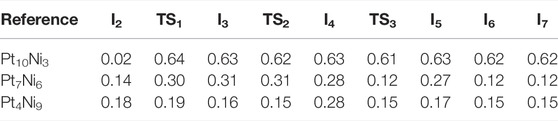
TABLE 6. Overall root-mean squared deviation (RMSD) of nanocluster structural changing for each reaction step, values given in Å.
4 Conclusion
In the present work, a theoretical study on the catalytic mechanisms for the WGS reaction at bimetallic nanoclusters Pt13−nNin (n = 3, 6, 9) is presented based on DFT calculations at the GFN-xTB level. The relative stability and structural, electronic, and magnetic properties of these nanoclusters are analyzed. The energy profile and the turnover frequency are calculated by means the reaction path and energetic span models. The main aims are the characterization of the most stable structures and putative adsorption sites on these bimetallic nanoclusters along the WGS reaction progress. The conclusions can be summarized as follows: (i) the Ni doping process provokes a slight decrease in the nanoalloys size, and a noticeable change in the magnetic property of the corresponding nanoalloy, (ii) Pt7Ni6 nanocluster is the most stable structure due to a most favorable coupling between the d-states coupling of Pt and Ni atoms. (iii) Pt10Ni3 nanocluster is the best catalyst followed by Pt4Ni9 and Pt7Ni6. (iv) however, despite the good catalyst performance expected from Pt10Ni3, it might not fulfill its promising catalytic behavior since a significant structural change happens after a complete reaction cycle; (v) Pt4Ni9 and Pt7Ni6 suffer a lower structural change, suggesting they would survive more cycle rounds keeping their catalytic activity, and (vi) due to its higher turnover frequency the Pt4Ni9 is expected to be a better catalyst than Pt7Ni6. We hope that the results in the current work encourage experimental studies focused on the shape and defect structure in Pt-Ni nanoalloys as catalysts for WGS reaction.
Data Availability Statement
The original contributions presented in the study are included in the article/Supplementary Material, further inquiries can be directed to the corresponding author.
Author Contributions
MB and MAS-M conceived the study. MB, MAS-M, and JA prepared the manuscript. MB conducted the computations. All authors contributed to this work, and approved the submitted version.
Funding
This work was founded by Conselho Nacional de Desenvolvimento Científico e Tecnológico - Brasil (CNPq) - grant number 142469/2018-1; 305792/2020-2; Fundação de Amparo à Pesquisa do Estado de São Paulo - FAPESP (2013/07296-2) (2016/23891-6) (2017/26105-4). This work was in part founded by the Coordenação de Aperfeioamento de Pessoal de Nível Superior - Brasil (CAPES) - Finance Code 001.
Conflict of Interest
The authors declare that the research was conducted in the absence of any commercial or financial relationships that could be construed as a potential conflict of interest.
Publisher’s Note
All claims expressed in this article are solely those of the authors and do not necessarily represent those of their affiliated organizations, or those of the publisher, the editors and the reviewers. Any product that may be evaluated in this article, or claim that may be made by its manufacturer, is not guaranteed or endorsed by the publisher.
Acknowledgments
The authors would like to kindly acknowledge Rafael Añez for his support and valuable discussions throughout this work. JA acknowledges Universitat Jaume I for project UJI-B2019-30, the Generalitat Valenciana for project AICO2020, and the Ministerio de Ciencia, Innovación y Universidades (Spain) for project PGC2018094417-B-I00, and for financially supporting this research. This work used computational resources of the “Centro Nacional de Processamento de Alto Desempenho em São Paulo” (CENAPAD-SP) and “Centro de Computação John David Rogers” (CCJDR-UNICAMP). The authors acknowledge the National Laboratory for Scientific Computing (LNCC/MCTI, Brazil) for providing HPC resources of the SDumont supercomputer, which have contributed to the research results reported within this work (URL: http://sdumont.lncc.br).
Supplementary Material
The Supplementary Material for this article can be found online at: https://www.frontiersin.org/articles/10.3389/fchem.2022.852196/full#supplementary-material
References
Albert, H. J., and Rubin, L. R. (1971). Magnetic Properties of the Platinum Metals and Their Alloys. Platin. Met. Rev. 8, 1–16. doi:10.1021/ba-1971-0098.ch001
Alijani, A., and Irankhah, A. (2013). Medium-temperature Shift Catalysts for Hydrogen Purification in a Single-Stage Reactor. Chem. Eng. Technol. 36, 209–219. doi:10.1002/ceat.201200151
Ammal, S. C., and Heyden, A. (2019). Understanding the Nature and Activity of Supported Platinum Catalysts for the Water-Gas Shift Reaction: From Metallic Nanoclusters to Alkali-Stabilized Single-Atom Cations. ACS Catal. 9, 7721–7740. doi:10.1021/acscatal.9b01560
Anderson, P. W. (1961). Localized Magnetic States in Metals. Phys. Rev. 124, 41–53. doi:10.1103/PhysRev.124.41
Añez, R., Cabral, L., da Silva, E. Z., Longo, E., Andrés, J., and San-Miguel, M. A. (2021). Unveiling the Ag–Bi Miscibility at the Atomic Level: a Theoretical Insight. Comput. Mater. Sci. 197, 110612. doi:10.1016/j.commatsci.2021
Bannwarth, C., Ehlert, S., and Grimme, S. (2019). GFN2-xTB-An Accurate and Broadly Parametrized Self-Consistent Tight-Binding Quantum Chemical Method with Multipole Electrostatics and Density-dependent Dispersion Contributions. J. Chem. Theor. Comput. 15, 1652–1671. doi:10.1021/acs.jctc.8b01176
Baraj, E., Ciahotný, K., and Hlinčík, T. (2021). The Water Gas Shift Reaction: Catalysts and Reaction Mechanism. Fuel 288, 119817. doi:10.1016/j.fuel.2020.119817
Barone, V., Casarin, M., Forrer, D., Pavone, M., Sambi, M., and Vittadini, A. (2009). Role and Effective Treatment of Dispersive Forces in Materials: Polyethylene and Graphite Crystals as Test Cases. J. Comput. Chem. 30, 934–939. doi:10.1002/jcc.21112
Bartolomé, J., Bartolomé, F., García, L. M., Roduner, E., Akdogan, Y., Wilhelm, F., et al. (2009). Magnetization ofPt13clusters Supported in a NaY Zeolite: A XANES and XMCD Study. Phys. Rev. B 80, 014404. doi:10.1103/PhysRevB.80.014404
Becke, A. D., and Johnson, E. R. (2005). A Density-Functional Model of the Dispersion Interaction. J. Chem. Phys. 123, 154101. doi:10.1063/1.2065267
Beller, M., Brückner, A., Büker, K., Caro, J., Claus, P., Demtröder, D., et al. (2010). Catalysis: A Key Technology for Sustainable Economic Growth. 3 edn. Frankfurt, Germany: German Catalysis Society.
Bhattacharjee, S., Waghmare, U. V., and Lee, S.-C. (2016). An Improved D-Band Model of the Catalytic Activity of Magnetic Transition Metal Surfaces. Sci. Rep. 6, 35916. doi:10.1038/srep35916
Blumenthal, I. (2001). Carbon Monoxide Poisoning. J. R. Soc. Med. 94, 270–272. doi:10.1177/01410768010940102910.1177/014107680109400604
Christiansen, J. A. (1953). The Elucidation of Reaction Mechanisms by the Method of Intermediates in Quasi-Stationary Concentrations (Academic Press), vol. 5 of Adv. Catal. 311–353. doi:10.1016/S0360-0564(08)60644-6
Cui, C., Gan, L., Heggen, M., Rudi, S., and Strasser, P. (2013). Compositional Segregation in Shaped Pt alloy Nanoparticles and Their Structural Behaviour during Electrocatalysis. Nat. Mater 12, 765–771. doi:10.1038/nmat3668
Davis, J. B. A., Baletto, F., and Johnston, R. L. (2015). The Effect of Dispersion Correction on the Adsorption of CO on Metallic Nanoparticles. J. Phys. Chem. A. 119, 9703–9709. doi:10.1021/acs.jpca.5b05710
de Souza Monteiro, R., Paes, L. W. C., Carneiro, de. M., Carneiro, J. W., and Aranda, D. A. G. (2008). Modeling the Adsorption of CO on Small Pt, Fe and Co Clusters for the Fischer-Tropsch Synthesis. J. Clust. Sci. 19, 601–614. doi:10.1007/s10876-008-0210-9
Di Paola, C., Pavan, L., D'Agosta, R., and Baletto, F. (2017). Structural Stability and Uniformity of Magnetic Pt13 Nanoparticles in NaY Zeolite. Nanoscale 9, 15658–15665. doi:10.1039/C7NR03533K
Ding, K., Cullen, D. A., Zhang, L., Cao, Z., Roy, A. D., Ivanov, I. N., et al. (2018). A General Synthesis Approach for Supported Bimetallic Nanoparticles via Surface Inorganometallic Chemistry. Science 362, 560–564. doi:10.1126/science.aau4414
Du, X., Liu, C., Zhang, S., Wang, P., Huang, S., and Tian, H. (2014). Structural, Magnetic and Electronic Properties of FenPt13−n Clusters with N=0-13: A First-Principle Study. J. Magnetism Magn. Mater. 369, 27–33. doi:10.1016/j.jmmm.2014.05.051
Ferrando, R., Jellinek, J., and Johnston, R. L. (2008). Nanoalloys: From Theory to Applications of Alloy Clusters and Nanoparticles. Chem. Rev. 108, 845–910. doi:10.1021/cr040090g
Giannozzi, P., Andreussi, O., Brumme, T., Bunau, O., Buongiorno Nardelli, M., Calandra, M., et al. (2017). Advanced Capabilities for Materials Modelling with Quantum ESPRESSO. J. Phys. Condens. Matter 29, 465901. doi:10.1088/1361-648x/aa8f79
Gilroy, K. D., Ruditskiy, A., Peng, H.-C., Qin, D., and Xia, Y. (2016). Bimetallic Nanocrystals: Syntheses, Properties, and Applications. Chem. Rev. 116, 10414–10472. doi:10.1021/acs.chemrev.6b00211
Greeley, J., Nørskov, J. K., and Mavrikakis, M. (2002). Electronic Structure and Catalysis on Metal Surfaces. Annu. Rev. Phys. Chem. 53, 319–348. doi:10.1146/annurev.physchem.53.100301.131630
Greeley, J. (2016). Theoretical Heterogeneous Catalysis: Scaling Relationships and Computational Catalyst Design. Annu. Rev. Chem. Biomol. Eng. 7, 605–635. doi:10.1146/annurev-chembioeng-080615-034413
Grimme, S. (2004). Accurate description of van der waals complexes by density functional theory including empirical corrections. J. Comput. Chem. 25, 1463–1473. doi:10.1002/jcc.20078
Grimme, S., Antony, J., Ehrlich, S., and Krieg, H. (2010). A Consistent and Accurate Ab Initio Parametrization of Density Functional Dispersion Correction (DFT-D) for the 94 Elements H-Pu. J. Chem. Phys. 132, 154104. doi:10.1063/1.3382344
Grimme, S., Bannwarth, C., and Shushkov, P. (20172009). A Robust and Accurate Tight-Binding Quantum Chemical Method for Structures, Vibrational Frequencies, and Noncovalent Interactions of Large Molecular Systems Parametrized for All Spd-Block Elements (Z = 1-86). J. Chem. Theor. Comput. 13, 1989–2009. doi:10.1021/acs.jctc.7b00118
Grimme, S. (2019). Exploration of Chemical Compound, Conformer, and Reaction Space with Meta-Dynamics Simulations Based on Tight-Binding Quantum Chemical Calculations. J. Chem. Theor. Comput. 15, 2847–2862. doi:10.1021/acs.jctc.9b00143
Guedes-Sobrinho, D., Nomiyama, R. K., Chaves, A. S., Piotrowski, M. J., and Da Silva, J. L. F. (2015). Structure, Electronic, and Magnetic Properties of Binary PtnTM55-N (TM = Fe, Co, Ni, Cu, Zn) Nanoclusters: A Density Functional Theory Investigation. J. Phys. Chem. C 119, 15669–15679. doi:10.1021/acs.jpcc.5b02242
Hammer, B., Morikawa, Y., and Nørskov, J. K. (1996). CO Chemisorption at Metal Surfaces and Overlayers. Phys. Rev. Lett. 76, 2141–2144. doi:10.1103/PhysRevLett.76.2141
Hammer, B., and Nørskov, J. K. (2000). Theoretical Surface Science and Catalysis-Calculations and Concepts. In Impact of Surface Science on Catalysis (Academic Press), vol. 45 of Adv. Cat. 71–129. doi:10.1016/s0360-0564(02)45013-4
Hammer, B., and Nørskov, J. K. (1995). Electronic Factors Determining the Reactivity of Metal Surfaces. Surf. Sci. 343, 211–220. doi:10.1016/0039-6028(96)80007-0
İnoğlu, N., and Kitchin, J. R. (2010). New Solid-State Table: Estimating D-Band Characteristics for Transition Metal Atoms. Mol. Simulation 36, 633–638. doi:10.1080/08927022.2010.481794
Jellinek, J. (2008). Nanoalloys: Tuning Properties and Characteristics through Size and Composition. Faraday Discuss. 138, 11–35. doi:10.1039/B800086G
Jeong, D.-W., Jang, W.-J., Shim, J.-O., Han, W.-B., Roh, H.-S., Jung, U. H., et al. (2014). Low-temperature Water-Gas Shift Reaction over Supported Cu Catalysts. Renew. Energ. 65, 102–107. doi:10.1016/j.renene.2013.07.035
Jia, Q., Segre, C. U., Ramaker, D., Caldwell, K., Trahan, M., and Mukerjee, S. (2013). Structure-property-activity Correlations of Pt-Bimetallic Nanoparticles: a Theoretical Study. Electrochimica Acta 88, 604–613. doi:10.1016/j.electacta.2012.10.124
Johnson, E. R., and Becke, A. D. (2005). A post-Hartree-Fock Model of Intermolecular Interactions. J. Chem. Phys. 123, 024101. doi:10.1063/1.1949201
Johnson, E. R., and Becke, A. D. (2006). A post-Hartree-Fock Model of Intermolecular Interactions: Inclusion of Higher-Order Corrections. J. Chem. Phys. 124, 174104. doi:10.1063/1.2190220
Kaatz, F. H., and Bultheel, A. (2019). Magic Mathematical Relationships for Nanoclusters. Nanoscale Res. Lett. 14, 150. doi:10.1186/s11671-019-2939-5
Kitchin, J. R., Nørskov, J. K., Barteau, M. A., and Chen, J. G. (2004). Modification of the Surface Electronic and Chemical Properties of Pt(111) by Subsurface 3d Transition Metals. J. Chem. Phys. 120, 10240–10246. doi:10.1063/1.1737365
Kovács, A., Cz. Dobrowolski, J., Ostrowski, S., and Rode, J. E. (2017). Benchmarking Density Functionals in Conjunction with Grimme's Dispersion Correction for noble Gas Dimers (Ne2 , Ar2 , Kr2 , Xe2 , Rn2 ). Int. J. Quan. Chem. 117, e25358. doi:10.1002/qua.25358
Kozuch, S., and Martin, J. M. L. (2011). The Rate-Determining Step Is Dead. Long Live the Rate-Determining State!. ChemPhysChem 12, 1413–1418. doi:10.1002/cphc.201100137
Kozuch, S., and Shaik, S. (2006). A Combined Kinetic−Quantum Mechanical Model for Assessment of Catalytic Cycles: Application to Cross-Coupling and Heck Reactions. J. Am. Chem. Soc. 128, 3355–3365. doi:10.1021/ja0559146
Kozuch, S., and Shaik, S. (2008). Kinetic-Quantum Chemical Model for Catalytic Cycles: The Haber−Bosch Process and the Effect of Reagent Concentration. J. Phys. Chem. A. 112, 6032–6041. doi:10.1021/jp8004772
Lian, X., Guo, W., Shu, J., Zhang, X., Liu, Z., Zhang, Y., et al. (2015). A Density Functional Theory Study of the Water-Gas Shift Reaction Promoted by Pt-Based Catalysts. Theor. Chem. Acc. 134, 19. doi:10.1007/s00214-015-1624-8
Lin, J., Wang, A., Qiao, B., Liu, X., Yang, X., Wang, X., et al. (2013). Remarkable Performance of Ir1/FeOx Single-Atom Catalyst in Water Gas Shift Reaction. J. Am. Chem. Soc. 135, 15314–15317. doi:10.1021/ja408574m
Lin, Z. Z., Chen, X., Yin, C., Tang, H., Hu, Y. C., and Ning, X. J. (2011). Theoretical Prediction of the Growth and Surface Structure of Pt and Ni Nanoparticles. Epl 96, 66005. doi:10.1209/0295-5075/96/66005
Liu, X., Bauer, M., Bertagnolli, H., Roduner, E., van Slageren, J., and Phillipp, F. (2006). Structure and Magnetization of Small Monodisperse Platinum Clusters. Phys. Rev. Lett. 97, 253401. doi:10.1103/PhysRevLett.97.253401
Mokkath, J. H. (2018). Exotic Chemical Arrangements and Magnetic Moment Evolution of NixPt1-X (0≤x≤1) Nanoparticles. J. Magnetism Magn. Mater. 456, 269–273. doi:10.1016/j.jmmm.2018.02.048
Morikawa, Y., Mortensen, J. J., Hammer, B., and Nørskov, J. K. (1997). CO Adsorption and Dissociation on Pt(111) and Ni(111) Surfaces. Surf. Sci. 386, 67–72. doi:10.1016/S0039-6028(97)00337-3
Nakhaei Pour, A., and Tayyari, S. F. (2019). Water-gas-shift Reaction over Nickel Catalysts: DFT Studies and Kinetic Modeling. Struct. Chem. 30, 1843–1852. doi:10.1007/s11224-019-01294-0
Newns, D. M. (1969). Self-consistent Model of Hydrogen Chemisorption. Phys. Rev. 178, 1123–1135. doi:10.1103/physrev.178.1123
Norskov, J. K., Abild-Pedersen, F., Studt, F., and Bligaard, T. (2011). Density Functional Theory in Surface Chemistry and Catalysis. Proc. Natl. Acad. Sci. 108, 937–943. doi:10.1073/pnas.1006652108
Nørskov, J. K., Studt, F., Abild-Pedersen, F., and Bligaard, T. (2014). The Electronic Factor in Heterogeneous Catalysis, chap 8. John Wiley & Sons, 114–137. doi:10.1002/9781118892114.ch8
Ooka, H., Huang, J., and Exner, K. S. (2021). The Sabatier Principle in Electrocatalysis: Basics, Limitations, and Extensions. Front. Energ. Res. 9. doi:10.3389/fenrg.2021.654460
Palma, V., Ruocco, C., Cortese, M., Renda, S., Meloni, E., Festa, G., et al. (2020). Platinum Based Catalysts in the Water Gas Shift Reaction: Recent Advances. Metals 10, 866. doi:10.3390/met10070866
Perdew, J. P., Burke, K., and Ernzerhof, M. (1996). Generalized Gradient Approximation Made Simple. Phys. Rev. Lett. 77, 3865–3868. doi:10.1103/PhysRevLett.77.3865
Perdew, J. P., Chevary, J. A., Vosko, S. H., Jackson, K. A., Pederson, M. R., Singh, D. J., et al. (1992). Atoms, Molecules, Solids, and Surfaces: Applications of the Generalized Gradient Approximation for Exchange and Correlation. Phys. Rev. B 46, 6671–6687. doi:10.1103/physrevb.46.6671
Piotrowski, M. J., Piquini, P., and Da Silva, J. L. F. (2012). Platinum-Based Nanoalloys PtnTM55-N (TM = Co, Rh, Au): A Density Functional Theory Investigation. J. Phys. Chem. C 116, 18432–18439. doi:10.1021/jp302844f
Rasmussen, M. H., and Jensen, J. H. (2020). Fast and Automatic Estimation of Transition State Structures Using Tight Binding Quantum Chemical Calculations. Peerj Phys. Chem. 2, e15. doi:10.7717/peerj-pchem.15
Reboul, J., Li, Z. Y., Yuan, J., Nakatsuka, K., Saito, M., Mori, K., et al. (2021). Synthesis of Small Ni-Core-Au-Shell Catalytic Nanoparticles on TiO2 by Galvanic Replacement Reaction. Nanoscale Adv. 3, 823–835. doi:10.1039/d0na00617c
Roduner, E., and Jensen, C. (2015). Magnetic Properties and the Superatom Character of 13-atom Platinum Nanoclusters. Magnetochemistry 1, 28–44. doi:10.3390/magnetochemistry1010028
Roduner, E. (2014). Nanoscopic Materials. Dorchester, United Kingdom: The Royal Society of Chemistry. doi:10.1039/9781847557636
Roşca, D. A., Fernandez-Cestau, J., Morris, J., Wright, J. A., and Bochmann, M. (2015). Gold(III)-CO and Gold(III)-CO2 Complexes and Their Role in the Water-Gas Shift Reaction. Sci. Adv. 1, e1500761. doi:10.1126/sciadv.1500761
Senanayake, S. D., Evans, J., Agnoli, S., Barrio, L., Chen, T.-L., Hrbek, J., et al. (2011). Water-Gas Shift and CO Methanation Reactions over Ni-CeO2(111) Catalysts. Top. Catal. 54, 34–41. doi:10.1007/s11244-011-9645-6
Solel, E., Tarannam, N., and Kozuch, S. (2019). Catalysis: Energy Is the Measure of All Things. Chem. Commun. 55, 5306–5322. doi:10.1039/C9CC00754G
Somiari, I., and Manousiouthakis, V. (2017). Coproduction of Acetic Acid and Hydrogen/power from Natural Gas with Zero Carbon Dioxide Emissions. Aiche J. 64, 860–876. doi:10.1002/aic.15966
Tao, F. (2012). Synthesis, Catalysis, Surface Chemistry and Structure of Bimetallic Nanocatalysts. Chem. Soc. Rev. 41, 7977–7979. doi:10.1039/C2CS90093A
Uhe, A., Kozuch, S., and Shaik, S. (2010). Automatic Analysis of Computed Catalytic Cycles. J. Comput. Chem. 32, 978–985. doi:10.1002/jcc.21669
Vojvodic, A., Nørskov, J. K., and Abild-Pedersen, F. (2014). Electronic Structure Effects in Transition Metal Surface Chemistry. Top. Catal. 57, 25–32. doi:10.1007/s11244-013-0159-2
Wang, L.-L., and Johnson, D. D. (2007). Density Functional Study of Structural Trends for Late-Transition-Metal 13-atom Clusters. Phys. Rev. B 75, 235405. doi:10.1103/PhysRevB.75.235405
Wang, L., Yang, Y., Wang, N., and Huang, S. (2016). Theoretical Investigation of Structural, Electronic and Magnetic Properties for Pt Ni55− (N= 0-55) Nanoparticles. Comput. Mater. Sci. 117, 15–23. doi:10.1016/j.commatsci.2016.01.016
Watzky, M. A., and Finke, R. G. (1997). Nanocluster Size-Control and "Magic Number" Investigations. Experimental Tests of the "Living-Metal Polymer" Concept and of Mechanism-Based Size-Control Predictions Leading to the Syntheses of Iridium(0) Nanoclusters Centering about Four Sequential Magic Numbers. Chem. Mater. 9, 3083–3095. doi:10.1021/cm9704387
Wu, C. H., Liu, C., Su, D., Xin, H. L., Fang, H.-T., Eren, B., et al. (2019). Bimetallic Synergy in Cobalt-Palladium Nanocatalysts for CO Oxidation. Nat. Catal. 2, 78–85. doi:10.1038/s41929-018-0190-6
Xin, H., Vojvodic, A., Voss, J., Nørskov, J. K., and Abild-Pedersen, F. (2014). Effects Ofd-Band Shape on the Surface Reactivity of Transition-Metal Alloys. Phys. Rev. B 89, 115114. doi:10.1103/PhysRevB.89.115114
Yamamoto, Y., Miura, T., Nakae, Y., Teranishi, T., Miyake, M., and Hori, H. (2003). Magnetic Properties of the noble Metal Nanoparticles Protected by Polymer. Physica B: Condensed Matter 329-333, 1183–1184. doi:10.1016/s0921-4526(02)02102-6
Yan, Y., Du, J. S., Gilroy, K. D., Yang, D., Xia, Y., and Zhang, H. (2017). Intermetallic Nanocrystals: Syntheses and Catalytic Applications. Adv. Mater. 29, 1605997. doi:10.1002/adma.201605997
Yao, S., Zhang, X., Zhou, W., Gao, R., Xu, W., Ye, Y., et al. (2017). Atomic-layered Au Clusters on α-MoC as Catalysts for the Low-Temperature Water-Gas Shift Reaction. Science 357, 389–393. doi:10.1126/science.aah4321
Zhang, F., Zhao, P., Niu, M., and Maddy, J. (2016). The Survey of Key Technologies in Hydrogen Energy Storage. Int. J. Hydrogen Energ. 41, 14535–14552. doi:10.1016/j.ijhydene.2016.05.293
Zhao, F., Liu, C., Wang, P., Huang, S., and Tian, H. (2013). First-principles Investigations of the Structural, Electronic, and Magnetic Properties of Pt13−nNin Clusters. J. Alloys Comp. 577, 669–676. doi:10.1016/j.jallcom.2013.06.175
Keywords: water-gas shift reaction, bimetallic (Pt/Ni) nanoclusters, DFT, density functional theory, heterogeneous catalysis, first-principles calculations
Citation: Barrionuevo MVF, Andrés J and San-Miguel MA (2022) A Theoretical Study on the Structural, Electronic, and Magnetic Properties of Bimetallic Pt13−nNin (N = 0, 3, 6, 9, 13) Nanoclusters to Unveil the Catalytic Mechanisms for the Water-Gas Shift Reaction. Front. Chem. 10:852196. doi: 10.3389/fchem.2022.852196
Received: 10 January 2022; Accepted: 21 February 2022;
Published: 25 March 2022.
Edited by:
Mauricio Chagas Da Silva, UMR7019 Laboratoire de Physique et Chimie Théoriques, FranceReviewed by:
Hélio Anderson Duarte, Federal University of Minas Gerais, BrazilBastien Casier, UMR7019 Laboratoire de Physique et Chimie Théoriques, France
Copyright © 2022 Barrionuevo, Andrés and San-Miguel. This is an open-access article distributed under the terms of the Creative Commons Attribution License (CC BY). The use, distribution or reproduction in other forums is permitted, provided the original author(s) and the copyright owner(s) are credited and that the original publication in this journal is cited, in accordance with accepted academic practice. No use, distribution or reproduction is permitted which does not comply with these terms.
*Correspondence: Juan Andrés, andres@qfa.uji.es; Miguel Angel San-Miguel, smiguel@unicamp.br