- 1Institutes for Systems Genetics, National Health Commission (NHC) Key Laboratory of Transplant Engineering and Immunology, West China Hospital, Sichuan University, Chengdu, China
- 2Department of Thoracic Surgery, Institute of Thoracic Oncology, West China Hospital, Sichuan University, Chengdu, China
- 3Department of Thyroid Surgery, West China Hospital, Sichuan University, Chengdu, China
- 4Department of Urology, Institute of Urology, West China Hospital, Sichuan University, Chengdu, China
- 5Division of Nephrology, West China Hospital, Sichuan University, Chengdu, China
- 6Renal Department and Institute of Nephrology, Sichuan Provincial People’s Hospital, University of Electronic Science and Technology of China, Sichuan Clinical Research Center for Kidney Diseases, Chengdu, China
Site-specific N-glycosylation characterization requires intact N-glycopeptide analysis based on suitable tandem mass spectrometry (MS/MS) method. Electron-transfer/higher-energy collisional dissociation (EThcD), stepped collision energy/higher-energy collisional dissociation (sceHCD), higher-energy collisional dissociation-product-dependent electron-transfer dissociation (HCD-pd-ETD), and a hybrid mass spectrometry fragmentation method EThcD-sceHCD have emerged as valuable approaches for glycoprotein analysis. However, each of them incurs some compromise, necessitating the systematic performance comparisons when applied to the analysis of complex clinical samples (e.g., plasma, urine, cells, and tissues). Herein, we compared the performance of EThcD-sceHCD with those previous approaches (EThcD, sceHCD, HCD-pd-ETD, and sceHCD-pd-ETD) in the intact N-glycopeptide analysis, and determined its applicability for clinical N-glycoproteomic study. The intact N-glycopeptides of distinct samples, namely, plasma from prostate cancer (PCa) patients, urine from immunoglobulin A nephropathy (IgAN) patients, human hepatocarcinoma cell line (HepG2), and thyroid tissues from thyroid cancer (TC) patients were analyzed by these methods. We found that EThcD-sceHCD outperformed other methods in the balance of depth and accuracy of intact N-glycopeptide identification, and sceHCD and EThcD-sceHCD have good complementarity. EThcD-sceHCD holds great potential for biomarker discovery from clinical samples.
Introduction
Glycosylation has been recognized as the most prevalent, structurally diverse, and multifunctional posttranslational modification of proteins (Moremen et al., 2012). It plays vital roles in physiopathological processes, including the progression of various cancers (Ohtsubo and Marth, 2006). Glycoproteomic (glycoprotein, glycosite, glycan, and intact glycopeptide) analysis of clinical samples can reveal tumor biomarkers and therapeutic targets (Zhang et al., 2020a; Cao et al., 2021). Among them, intact glycopeptide analysis can simultaneously obtain glycoprotein, glycosite, and glycan composition information. Hence, it has become the major method in the glycoproteomics study. However, the intact glycopeptide analysis is very challenging owing to the macroheterogeneity and microheterogeneity of glycosylation modification, low stoichiometry of intact glycopeptides, and different energy requirements to fragment the peptides and glycans (Pujić and Perreault, 2021). How to achieve the accurate and in-depth identification of intact glycopeptides is a critical problem in the clinical glycoproteomic study.
Over the past decades, a lot of researchers have come up with their own solutions. Efforts are mainly made in the following three areas: sample preparation, mass spectrometry analysis, and data processing. In sample preparation, many glycoproteins or intact glycopeptide enrichment materials or methods [e.g., hydrophilic interaction chromatography (HILIC), titanium dioxide chromatography, lectin affinity chromatography, boronate affinity chromatography, and hydrazide chemistry] were developed to avoid the interference of nonglycoprotein or nonglycopeptides (Palmisano et al., 2010; Sun et al., 2017; Yang et al., 2017; Zhang et al., 2017; Wu et al., 2018; Chen et al., 2019; Sun et al., 2019; Zhang et al., 2019; Zhang et al., 2020b). Besides, protein extraction and digestion will also affect the accuracy and depth of intact glycopeptide identification. In terms of mass spectrometry, varied fragmentation techniques for tandem mass spectrometry (MS/MS) analysis, such as electron-transfer/higher-energy collisional dissociation (EThcD), stepped collision energy/higher-energy collisional dissociation (sceHCD), higher-energy collisional dissociation-product-dependent electron-transfer dissociation (HCD-pd-ETD), have emerged as valuable approaches for clinical glycoproteomics (Saba et al., 2012; Ma et al., 2016; Yu et al., 2017). Yu’s team and Zhang’s team proved that EThcD is a powerful and effective tool for the analysis of intact N-glycopeptides and intact O-glycopeptides of human plasma in that EThcD produces glycan-attached c/z ions for the identification of both peptide backbone and glycosites as well as the glycan compositions (Yu et al., 2017; Zhang et al., 2018). However, each of these collision modes has advantages and disadvantages (Riley et al., 2020). In terms of data processing, the annotation of intact glycopeptide MS/MS data is very challenging, including the correct assignment of glycan composition, glycosite as well as the peptide backbone (Kawahara et al., 2021). Recently, many intact glycopeptides search engines have been developed based on modern MS techniques. These tools, especially Byonic, MSFragger-Glyco, pGlyco, StrucGP, O-pair, have gradually improved the identification depth or accuracy for glycoproteomics (Bern et al., 2012; Liu et al., 2017; Lu et al., 2020; Polasky et al., 2020; Shen et al., 2021). Facilitated by the HUPO Human Glycoproteomics Initiative (HGI), Kawahara et al. performed a comprehensive community-based evaluation of existing informatics solutions for large-scale intact glycopeptide analysis. Their study involved several high-performance search strategies and specified key variables that will help informatics decision making in glycoproteomics (Kawahara et al., 2021).
In this study, we focused on improving the depth and accuracy of intact glycopeptide identification from complex samples based on the advanced mass spectrometry analysis. In a recent work, we have presented a hybrid dissociation technique, EThcD-sceHCD, by integrating EThcD and sceHCD into a sequential glycoproteomic workflow (Zhang et al., 2021a; Zhang et al., 2021b). Our results have indicated that EThcD-sceHCD is able to achieve improved performance in the analysis of intact N/O-glycopeptides of a highly glycosylated protein (HIV-1 gp120) compared with other methods. Furthermore, we analyzed purified IgG subclasses (IgG1, IgG2, IgG3, and IgG4) from patients with chronic kidney disease using EThcD-sceHCD (Zhang et al., 2021a; Zhang et al., 2021b). When compared with EThcD and sceHCD, EThcD-sceHCD obtained more informative fragment ions, higher spectral quality, higher Byonic score, and more intact glycopeptide identifications. Does this approach also work well for complex clinical samples (such as plasma, urine, cells, and tissues)? Herein, we compared EThcD-sceHCD with previously reported methods, sceHCD, EThcD, HCD-pd-ETD, and sceHCD-pd-ETD, in the analysis of intact N-glycopeptides from complex clinical samples for the first time, and determined its applicability for clinical N-glycoproteomic study. The raw MS data have been deposited to the ProteomeXchange Consortium via the PRIDE partner repository with the dataset identifier PXD030288.
Experimental section
Materials and chemicals
Dithiothreitol (DTT), iodoacetamide (IAA), sodium dodecyl sulfate (SDS), and ammonium bicarbonate (ABC) were obtained from Sigma-Aldrich (St. Louis, MO, USA). Protease inhibitor cocktail tablets were purchased from Roche Diagnostics (Sandhofer Strasse, Mannheim, Germany). Sequencing-grade trypsin and Lys-C were obtained from Promega (Madison, WI, USA). Trifluoroacetic acid (TFA), acetonitrile (ACN), and formic acid (FA) were purchased from Thermo Fisher Scientific (Waltham, MA, USA). Water used in the experiments was purified by a Milli-Q system (Millipore, Bedford, MA, United States). Zwitterionic HILIC (Zic-HILIC) was purchased from Fresh Bioscience (Shanghai, China). The C8 extraction disks were purchased from 3M Empore (St. Paul, MN, USA). Sterile cell culture plates were purchased from Corning (New York, NY, USA). Dulbecco’s modified Eagle medium (DMEM), fetal bovine serum (FBS), penicillin–streptomycin (PS) antibiotics, phosphate-buffered saline (PBS), and trypsin were obtained from Gibco (Waltham, MA, USA). All other chemicals and reagents of the best available grade were obtained from Sigma-Aldrich or Thermo Fisher Scientific.
Clinical sample collection
Diagnosis and pathological analyses of prostatic cancer (PCa) were performed in the Department of Urology, West China Hospital. Diagnosis and pathological analyses of thyroid cancer (TC) were performed in the Department of Thyroid Surgery, West China Hospital. Diagnosis and pathological analyses of Immunoglobulin A nephropathy (IgAN) were performed in the Renal Department and Institute of Nephrology, Sichuan Provincial People’s Hospital. All patients underwent tissue biopsy and were histopathologically diagnosed according to the criteria of the World Health Organization. Blood from PCa patients was collected in an ethylenediaminetetraacetic acid anticoagulant tube and centrifuged at 300 × g for 10 min at 4°C. Plasma was collected in 1-ml tubes and stored at –80°C until use. The midstream urine from IgAN patients was collected in an appropriate container and centrifuged at 1,000 × g for 10 min. The precipitate was discarded, and 10 ml of the supernatant was collected in a 50-ml tube and stored at −80°C until use. The thyroid tissue from thyroid cancer patients was collected within 30 min after the operation, cleaned with a sterile towel, transferred to sterile freezing vials, immersed in liquid nitrogen, and stored at −80°C until use. All experiments were performed following the guidelines of Chinese Medical Ethics Committee, and approved by the ethics committee at Sichuan Provincial People’s Hospital and West China Hospital. Informed consent was obtained for any experimentation with human subjects.
Cell culture
Human hepatocarcinoma cell line (HepG2) was obtained from the American Type Culture Collection (ATCC) and authenticated through Short Tandem Repeat (STR) profiling. HepG2 cells were cultured in DMEM supplemented with 5% FBS and 1% PS, and grown at 5% CO2 in a humidified incubator at 37°C.
Protein extraction
Cells were harvested when reaching 80% confluence and washed with ice-cold PBS three times. Then the cells were lysed by UA buffer (8 M urea, 0.1 M Tris, pH 8.5, 1% protease inhibitor cocktail) with sonication (SCIENTZ-IID, Ningbo Scientz Biotechnology Co., Ltd., Ningbo, China) at 4°C for protein extraction. The lysate was centrifuged at 13,000 × g for 20 min at 4°C. The supernatant was transferred to a clean tube and stored at −80°C. Approximately 80 mg of thyroid cancer tissues were homogenized in lysis buffer (8 M urea, 50 mM Tris, pH 8.5, 1% protease inhibitor cocktail) using a tissue lyser (speed = 6.5 m/s; time = 60 s; number of cycles = 3; Lifereal Biotechnology Co., Ltd., Hangzhou, China) followed by sonication at 4°C. The homogenate was centrifuged at 13,000 × g for 20 min at 4°C. The supernatant was then collected and stored at −80°C until use. Plasma was subpacked and stored at −80°C until use. Urine supernatant was concentrated using a 30-kDa ultrafiltration tube (Millipore) and stored at −80°C until use. Concentration of proteins extracted from cells, tissues, plasma, and urine was determined by Bradford assay (Thermo Fisher Scientific).
Reduction, alkylation, and digestion
Proteins extracted from plasma from PCa patients, urine from IgAN patients, HepG2 cells, and thyroid cancer tissues were processed and digested following the filter-aided sample preparation (FASP) protocol. Briefly, 500 µg of proteins was diluted with UA buffer and loaded onto a 30-kDa filter tube. After being centrifuged at 13,000 × g for 15 min at 25°C, the proteins were reduced by 20 mM DTT for 4 h at 37°C followed by alkylation with 50 mM IAA for 30 min at 25°C in the dark. The protein mixture was washed twice with 200 μl of UA buffer and four times with 200 μl of 50 mM ABC by centrifugation at 13,000 × g for 15 min at room temperature. Then 200 μl of ABC containing 10 μg of trypsin and 10 μg of Lys-C were added to each filter tube and incubated for 16 h at 37°C. Finally, the filter tubes were washed three times with 100 μl of water, and the flow-through fractions containing peptides were collected. The peptide concentration was determined using a quantitative colorimetric peptide assay kit (Thermo Fisher Scientific) based on the absorbance at 480 nm. The peptide mixtures were freeze dried and then stored at −80°C.
Intact N-glycopeptide enrichment
Intact N-glycopeptides were enriched by Zic-HILIC materials. Specifically, 10 mg of Zic-HILIC beads were washed three times for 10 min with 0.1% TFA and 70% ACN/0.2% TFA. Then 200 μg of tryptic peptides were dissolved in 70% ACN/0.2% TFA solution, added into the processed Zic-HILIC beads and rotated for 2 h at 37°C to enrich the intact N-glycopeptides. Last, the mixture was transferred to a 200-μl pipet tip packed with a layer of C8 membrane and washed twice with 70% ACN/0.2% TFA. Intact N-glycopeptides bound on the Zic-HILIC beads were eluted three times with 70 μl of 0.1% TFA. The pooled eluent was dried under vacuum for subsequent LC-MS/MS analysis.
LC-MS/MS analysis
The intact N-glycopeptides derived from plasma, urine, cells, and tissues were dissolved with 40 μl of loading buffer (0.1% FA in water), respectively. Peptide solution (2 μl) was loaded for LC-MS/MS analysis using an Orbitrap Fusion Lumos mass spectrometer (Thermo Fisher Scientific) with the Xcalibur software (version 4.3; Thermo Fisher Scientific). The intact N-glycopeptides were separated on a C18 column (ReproSil-Pur C18-AQ, 1.9 μm, 75 μm × 20 cm; Dr. Maisch) at a flow rate of 300 nl/min over a 78-min gradient (solvent A, 0.1% FA in water; solvent B, 0.1% FA in 80% ACN; 0–8 min, 5%–8% B; 8–58 min, 8%–22% B; 58–70 min, 22%–32% B; 70–71 min, 32%–90% B; and 71–78 min, 90% B), and detected in the data-dependent acquisition mode. Notably, five different MS/MS dissociation modes, EThcD, sceHCD, EThcD-sceHCD, HCD-pd-ETD, and sceHCD-pd-ETD were used for the fragmentation of intact N-glycopeptides. Each sample was detected in three technical replicates for every MS/MS dissociation method. The detailed parameters for the five dissociation modes were as follows.
The MS1 scan range, RF lens, exclusion duration, and MS2 first mass were set as 800–2,000 (m/z), 40%, 15 s, and 120 (m/z) in all the five modes. The MS1 and MS2 were acquired at an Orbitrap resolution of 60,000 and 30,000, respectively, in all the five methods except for sceHCD-MS/MS, where the Orbitrap resolution was set as 120,000 for MS1 acquisition and 15,000 for MS2 analysis. The precursor ion for MS2 analysis was isolated with an isolation window of 2.0 (m/z) in all methods except for the sceHCD cycle of EThcD-sceHCD-MS/MS, which used an isolation window of 1.6 (m/z).
For EThcD-MS/MS, the maximum injection time and AGC target were set as 50 ms and custom (2e5) in MS1, and 150 ms and custom (5e5) in MS2. EThcD normalized collision energy was 35%, and the cycle time was 3 s.
For sceHCD-MS/MS, the maximum injection time and AGC target were 100 ms and custom (2e5) in MS1, and 250 ms and custom (5e5) in MS2. Stepped collision energy HCD mode was turned on with an energy difference of ±10% (20%–30%–40%). The cycle time was 3 s.
For EThcD-sceHCD-MS/MS, the data acquisition for the EThcD-sceHCD-MS/MS was performed using an alternative fragmentation between EThcD and sceHCD modes in a duty cycle. In duty cycle 1 (EThcD), the maximum injection time and AGC target were 50 ms and custom (2e5) for MS1, and 150 ms and custom (5e5) in MS2. The EThcD normalized collision energy was set as 35%. The EThcD cycle time was 2 s. In duty cycle 2 (sceHCD), the maximum injection time and AGC target were auto and standard in MS1, and auto and custom (1e5) in MS2. Stepped collision mode was turned on with HCD normalized collision energies set as 20%–30%–40%. The sceHCD cycle time was 1 s.
For HCD-pd-ETD-MS/MS or sceHCD-pd-ETD-MS/MS, the product-dependent methods were constructed using two classic dissociation types as the triggered scan. AGC target and maximum injection time were custom (2e5) and 50 ms in MS1. Product-dependent triggering required at least one ion from the following list to be present in the top 20 most abundant peaks in a spectrum within 15 ppm tolerance: 138.0545, 204.0867, and 366.1396 (m/z). AGC target and maximum injection time were custom (5e5) and 150 ms in MS2. The ETD mode was turned on with an energy of 35%. Stepped collision energy mode (for sceHCD-pd-ETD) was turned on with an energy difference of ±10% (20%–30%–40%). The cycle time was 3 s.
Data analysis
The MS raw data files of intact N-glycopeptides were searched against the human Uniprot database (version 2015_03, 20,410 entries) using the Byonic software (version 3.6.0, Protein Metrics, Inc.). The mass tolerance for precursors and fragment ions were set as ±10 and ±20 ppm, respectively. The fixed modification was carbamidomethylation of cysteine, and variable modifications included oxidation of methionine and acetylation of protein N-terminal. Two missed cleavage sites were allowed for enzyme digestion. All other parameters were set at the default values. In addition, the 182 human N-glycans were specified as the N-glycosylation modification for all searches. Protein groups were filtered to 1% false discovery rates (FDRs) based on the number of hits obtained for searches against these databases. Stricter quality control criteria for intact N-glycopeptide identification were used, including a Byonic score over 200 and identification of at least seven amino acids.
Statistical analysis
Analysis of variance (ANOVA) was applied to the statistical comparison among five groups in the number of intact N-glycopeptides, N-glycan compositions, and N-glycoproteins identified based on different fragmentation modes for each sample. Student’s t-test was used for the binary statistical comparison. Data were shown as means ± standard deviation (SD), and a p-value <0.05 was considered significant.
Results and discussion
To characterize the site-specific N-glycosylation of proteins from complex clinical samples, the choice of suitable fragmentation mode is the key. Generally, HCD, the representative of beam-type collisional activation, and ETD, the typical electron-based dissociation, are two fundamental and commonly used MS/MS fragmentation methods for glycopeptide characterization. Because of their distinct activation mechanisms, HCD and ETD produce different fragments when applied to the dissociation of intact glycopeptides. HCD generates b/y-type fragments derived from the peptide backbone that lose part or all of the modified glycans due to the fragmentation of glycans by HCD, while ETD yields mainly c/z-type peptide backbone fragments retaining the intact glycan moieties (Riley et al., 2020). Hence, they share good complementarity to each other.
Based on the above two basic approaches, various fragmentation methods have been developed, including the optimized HCD (e.g., sceHCD) and the paired HCD and ETD (e.g., EThcD, HCD-pd-ETD, and sceHCD-pd-ETD) (Saba et al., 2012; Yu et al., 2017). Taking advantage of the stepped collision energies, sceHCD can provide better fragmentation for both glycans and peptide backbone as lower collision energies have been found to benefit glycan fragmentation, while higher collision energies aid in better dissociation of peptide backbone. Accordingly, previous reports suggest that sceHCD-MS/MS can generate the most abundant and informative fragment ions from both the peptide backbone and the attached glycan of an intact N-glycopeptide in one single spectrum compared with CID-, HCD-, ETD-, ETciD- and EThcD-MS/MS (Klein and Zaia, 2020; Riley et al., 2020). However, sceHCD-MS/MS cannot provide sufficient spectral evidence for accurate location of N/O-glycosite and identification of glycan composition in the case when more than one glycosite occurs within one peptide (Zhang et al., 2021b). EThcD-, HCD-pd-ETD-, and sceHCD-pd-ETD-MS/MS can produce a more complete fragmentation and a greater proportion of fragment ions of glycopeptides because the precursor ions are fragmented via both ETD and HCD, thereby enabling the glycosites and glycans to be unambiguously determined (Saba et al., 2012; Ma et al., 2016; Yu et al., 2017).
Unfortunately, the dissociation efficiency of these methods is still limited, especially for the glycopeptide precursors with low charge density. Hence, we proposed a combined fragmentation mode, EThcD-sceHCD-MS/MS, which was implemented using an alternative fragmentation between EThcD and sceHCD mode in a duty cycle (Zhang et al., 2021b). This combined fragmentation strategy makes good use of both sceHCD and EThcD with higher dissociation efficiency and better spectra quality. Our previous studies have shown that EThcD-sceHCD can achieve improved performance for the analysis of intact glycopeptides from highly glycosylated proteins, HIV-1 gp120 and IgG subclasses (Zhang et al., 2021a; Zhang et al., 2021b). Herein, we applied this method to the analysis of intact N-glycopeptides from varying types of clinical samples and systematically compared with several other methods that are commonly used in glycoproteomics (i.e., sceHCD, EThcD, HCD-pd-ETD, and sceHCD-pd-ETD) to evaluate their performance in characterizing intact N-glycopeptides from the complex clinical samples.
To evaluate the usefulness and superiority of the EThcD-sceHCD method in complicated clinical samples, the following experiments were designed (Figure 1). Specifically, proteins extracted from plasma from PCa patients, urine from IgAN patients, HepG2 cells, and thyroid cancer tissues were digested by trypsin and Lys-C. The obtained peptides were enriched with Zic-HILIC materials. Then the enriched intact N-glycopeptides from plasma, urine, cells, and tissues were analyzed three times repeatedly using different fragmentation modes (i.e., EThcD, sceHCD, EThcD-sceHCD, HCD-pd-ETD, and sceHCD-pd-ETD) (Figure 1). All the MS raw data were searched by the Byonic software, and for all methods, the localized N-glycopeptide spectral matches (N-glycoPSMs) were filtered to get a Byonic score of over 200 and a peptide length of at least seven amino acids to ensure quality identifications. Statistical analysis was performed to systematically compare the number of identified N-glycoPSMs, N-glycans, intact N-glycopeptides, and N-glycoproteins among the five fragmentation modes (Supplementary Tables S1–S4).
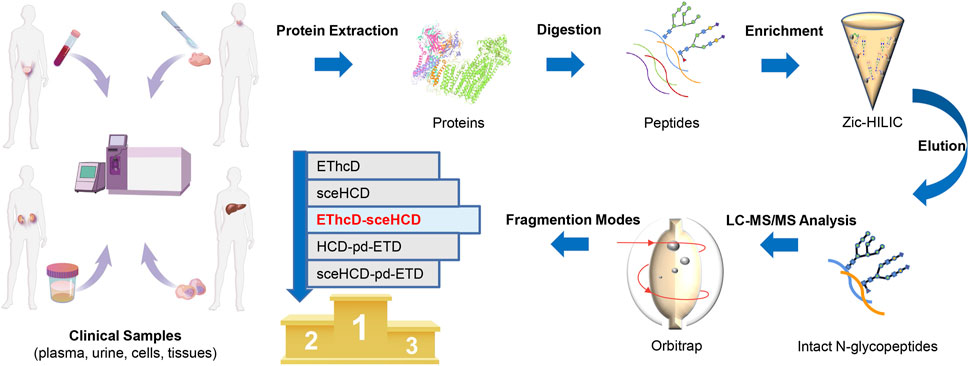
FIGURE 1. Schematic illustration of the workflow for human plasma, urine, cells, and tissue intact N-glycopeptides analysis using different dissociation methods.
In general, the five fragmentation methods produce abundant fragment ions with distinct features. For example, as shown in Figure 2, all methods can provide confident N-glycosite (N93) localization and abundant information about the N-glycan composition [HexNAc(5)Hex(6)NeuAc(3)] of an intact N-glycopeptide from human plasma alpha-1-acid glycoprotein 1. For EThcD-, EThcD-sceHCD-, HCD-pd-ETD-, and sceHCD-pd-ETD-MS/MS, the precursor ions or fragment ions were dissociated with both ETD and HCD. Specifically, the product-dependent mode was used to trigger a scan of specific ETD to fragment the glycopeptide upon the detection of glycopeptide-specific oxonium ions produced by HCD (Yin et al., 2013). Their spectra included glycan fragments, b/y/c/z-type peptide backbone fragments, and Y ions (y-type peptide backbone fragments attached by glycan) with few occurrences of glycan dissociation (Figures 2A,C–E). sceHCD-MS/MS spectrum contained b/y-type peptide backbone fragments without glycans, oxonium ions, and Y ions (Figure 2B).
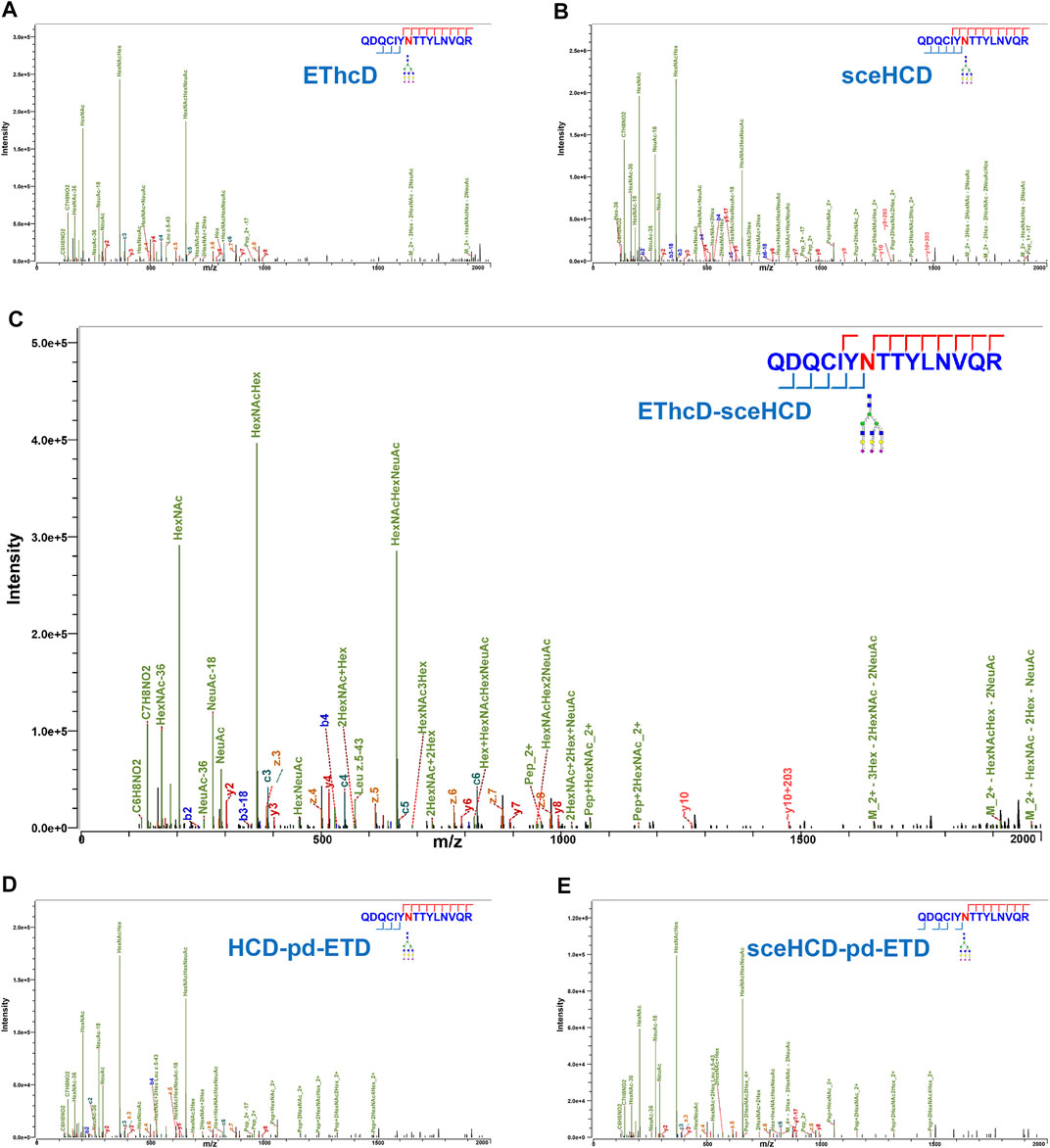
FIGURE 2. Comparison of electron-transfer/higher-energy collisional dissociation (EThcD), stepped collision energy/higher-energy collisional dissociation (sceHCD), EThcD-sceHCD, higher-energy collisional dissociation-product-dependent electron-transfer dissociation (HCD-pd-ETD), and sceHCD-pd-ETD spectra of alpha-1-acid glycoprotein 1 N-linked glycopeptide (N93) from human plasma.
Furthermore, we analyzed and compared the number of localized N-glycoPSMs, intact N-glycopeptides, and N-glycan compositions from four clinical samples (i.e., plasma from PCa patients, urine from IgAN patients, HepG2 cells, and tissues from TC patients) for all methods (Figure 3 and Supplementary Table S5). The average numbers of localized N-GlycoPSMs of four clinical samples for each method are summarized and presented in Figures 3A–D. The results clearly show that EThcD-sceHCD is the best (p < 0.01, ANOVA), followed by sceHCD, and the other three methods are equal in terms of the number of localized N-GlycoPSMs. Besides, the sceHCD method can provide quality fragmentation for both peptide and glycan moieties, whereas EThcD-sceHCD can generate more informative spectra including both EThcD products and sceHCD products by virtue of the flexibly alternate fragmentation of the precursor ions using EThcD (2 s) and sceHCD (1 s) mode in a duty cycle (3 s) (Zhang et al., 2021b). In contrast, the N-GlycoPSMs of EThcD, HCD-pd-ETD, and sceHCD-pd-ETD scans were much fewer than those of sceHCD or EThcD-sceHCD scans due to the less MS/MS acquisition resulting from the slower scan rate. Then, we compared the average numbers of N-glycan compositions and intact N-glycopeptides. Interestingly, for plasma sample, EThcD-sceHCD can outperform sceHCD (p < 0.001, t-test) and other methods (p < 0.001, t-test) in terms of the number of identified N-glycan compositions and intact N-glycopeptides (Figures 3E, I), while for urine, cell, and tissue samples, both EThcD-sceHCD and sceHCD obtain good peptide and glycan sequence coverage with comparable identification number of N-glycan compositions and intact N-glycopeptides (Figures 3F–H,J–L). This may be attributed to the higher complexity of plasma sample, especially the presence of numerous high-abundance proteins, which is not conducive to sceHCD and requires the improvement of fragmentation method for deeper coverage of intact N-glycopeptides particularly from low-abundance proteins. The above results indicated that EThcD-sceHCD has high scanning speed to generate sufficient number of spectra with superior fragmentation quality. Hence, it outperforms other methods in the balance of depth and accuracy of intact N-glycopeptide identification.
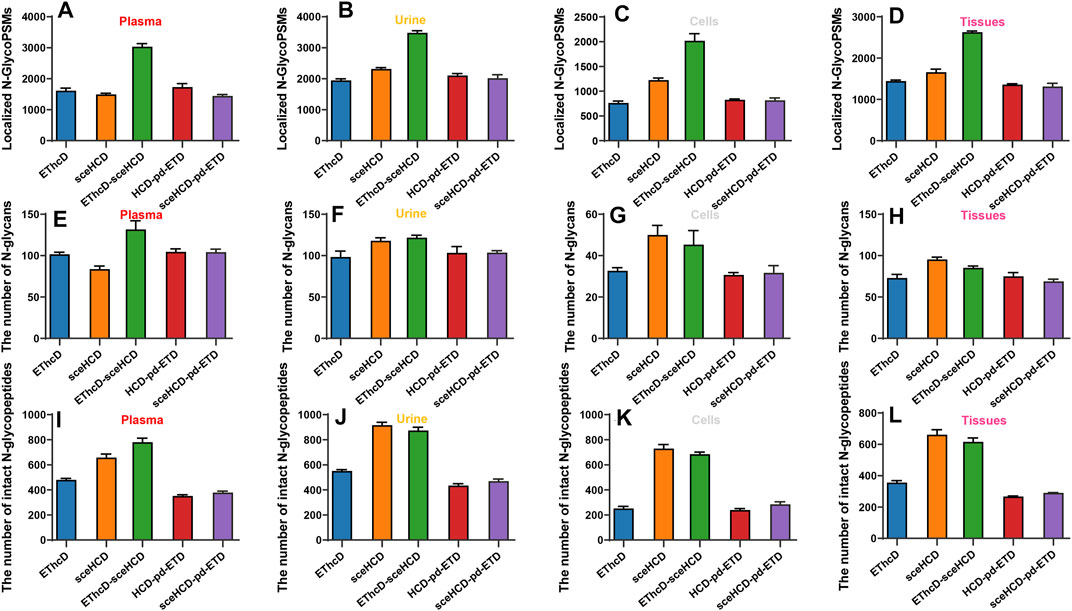
FIGURE 3. Comparison of the number of localized N-glycopeptide spectral matches (glycoPSMs), N-glycans, and intact N-glycopeptides from human plasma, urine, cells, and tissues.
To evaluate the types of intact N-glycopeptides identified by different methods, we analyzed intact glycopeptides from four clinical samples. As shown in Figure 4, a total of 1,549, 1949, 1,394, and 1,325 intact N-glycopeptides were identified from plasma, urine, cells, and tissues, respectively. However, only 289, 341, 148, and 194 intact N-glycopeptides were commonly identified by all five methods. Of note, sceHCD and EThcD-sceHCD can identify more unique intact N-glycopeptides. These results suggest good complementarity of the five methods, and combinations of different methods (e.g., the combination of sceHCD and EThcd-sceHCD) for the same sample analysis will greatly increase the depth of intact N-glycopeptide identification.
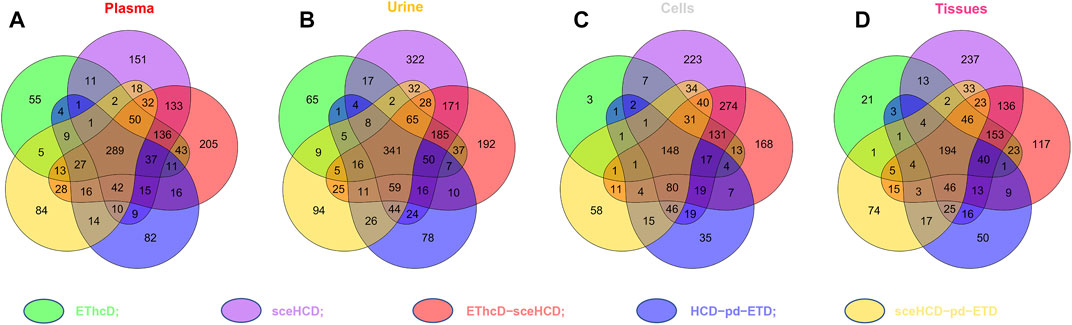
FIGURE 4. Comparison of the intact N-glycopeptides identified by different tandem mass spectrometry (MS/MS) fragmentation modes.
Based on the comprehensive and multilevel glycoproteomic information of varied clinical samples obtained in this work, we further constructed the glycoproteome databases of clinical samples, which may be potentially translated into clinical applications and provide important reference information for fundamental research. To illustrate the microheterogeneity and macroheterogeneity of glycoproteins, we chose alpha-1-acid glycoprotein 1 to illustrate because it has been used as a standard N-glycoprotein at a lot of glycoprotein studies. In this study, we also found that alpha-1-acid glycoprotein 1 in PCa patients’ plasma is a highly glycosylated protein (Figure 5). As a transport protein in the blood stream, it can bind various ligands and synthetic drugs, and then regulate their distribution and accumulation in the body (Fitos et al., 2006; Zsila and Iwao, 2007). Herein, three N-glycosites (N56, N93, and N103) and 48 intact N-glycopeptides were identified using the five methods (Supplementary Table S6). Specifically, N56, N93, and N103 were located at the α-helix, β-sheet, and loop of the protein and were modified with 15, 20, and 13 N-glycans, respectively. Nearly all N-glycans were sialylated complex type, and half of them were fucosylated. In addition, all the three N-glycosites were commonly modified by eight N-glycans [HexNAc(5)Hex(6)NeuAc(3), HexNAc(4)Hex(5)NeuAc(2), HexNAc(6)Hex(7)NeuAc(4), HexNAc(5)Hex(6)NeuAc(2), HexNAc(6)Hex(7)Fuc(1)NeuAc(4), HexNAc(5)Hex(6)Fuc(1)NeuAc(2), HexNAc(5)Hex(7)Fuc(1)NeuAc(2), HexNAc(5)Hex(6)Fuc(1)NeuAc(3)]. This result suggests that our methods can decipher the microheterogeneity and macroheterogeneity of glycoproteins from complex clinical samples. It is worth noting that five N-glycosites of alpha-1-acid glycoprotein have been found in some studies (Lee et al., 2016; Bollineni et al., 2018; Hwang et al., 2020; Keser et al., 2021; Virág et al., 2021). However, these studies mainly focus on the analysis of N-glycosylation of a recombinant expressed or purified or especially treated alpha-1-acid glycoprotein. To cover all N-glycosites of alpha-1-acid glycoprotein from complex plasma sample, special sample processing (high protein abundance removal, antibodies and affinity chromatography, acid precipitation, multienzyme digestion, etc.) or other mass-spectrometric techniques may also be required.
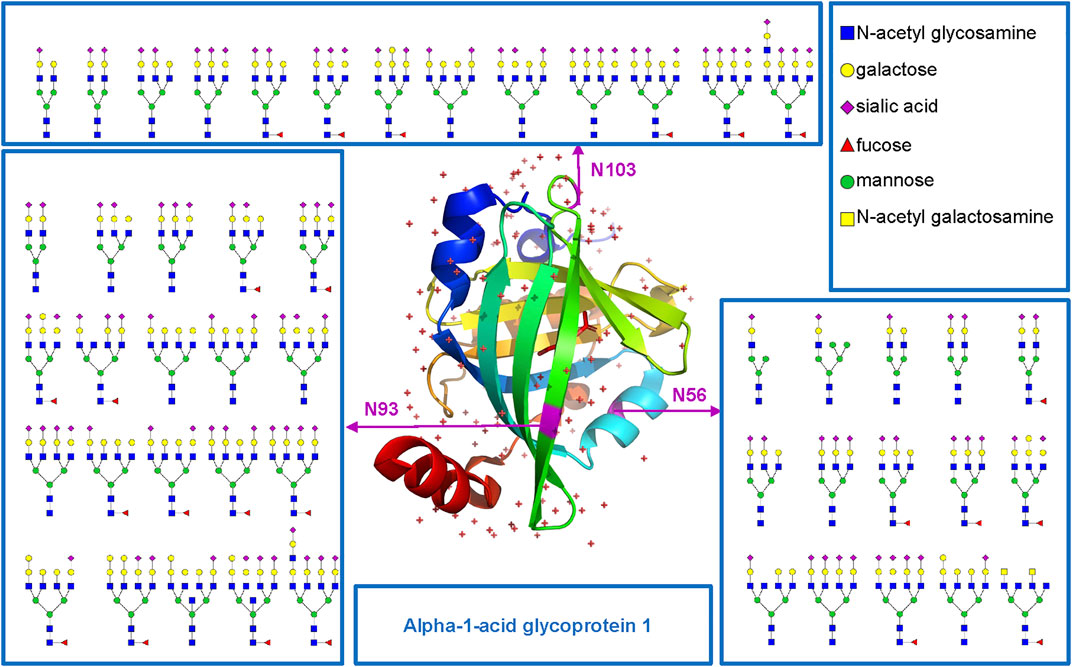
FIGURE 5. N-glycosites (N56, N93, and N103) and deduced N-glycans were mapped in the three-dimensional structure of the alpha-1-acid glycoprotein 1 (PDB code: 3KQ0) from human plasma.
Conclusion
This study, to the best of our knowledge, first conducted the comprehensive comparison among EThcD, sceHCD, EThcD-sceHCD-, HCD-pd-ETD, and sceHCD-pd-ETD in the intact N-glycopeptides analyses of clinical samples (plasma, urine, cells, and tissues). Based on the systematical comparison results, we conclude that EThcD-sceHCD is more suitable for the intact N-glycopeptides analysis of clinical samples because it outperforms other methods in the balance of depth and accuracy of intact N-glycopeptide identification. The combination of sceHCD and EThcD-sceHCD will greatly increase the depth of intact N-glycopeptide identification due to their good complementarity, offering more flexible choices for selecting a suitable fragmentation method in glycoproteomic study. These findings would drive glycoproteomic methodological development, guide the software development, and promote clinical application research.
Data Availability Statement
The datasets presented in this study can be found in online repositories. The names of the repository/repositories and accession number(s) can be found below: http://www.proteomexchange.org/, PXD030288.
Ethics Statement
The studies involving human participants were reviewed and approved by the ethics committee at Sichuan Provincial People’s Hospital and West China Hospital. All experiments were performed in accordance with the guidelines of the Chinese Medical Ethics Committee, and approved by the ethics committee at Sichuan Provincial People’s Hospital and West China Hospital. The patients/participants provided their written informed consent to participate in this study.
Author Contributions
YoZ and HY designed the research. WZe, SZ, TS, JC, YM, YiZ, YL, and JC performed the sample preparation, MS detection, and data analysis. WZh, TL, FL, and GL coordinated the acquisition, distribution, and quality evaluation of the clinical samples. WZe, YoZ, and HY wrote and revised the manuscript.
Funding
This work was funded by grants from the National Natural Science Foundation of China (31901038), Department of Science and Technology of Sichuan Province (2021YJ0479).
Conflict of Interest
The authors declare that the research was conducted in the absence of any commercial or financial relationships that could be construed as a potential conflict of interest.
Publisher’s Note
All claims expressed in this article are solely those of the authors and do not necessarily represent those of their affiliated organizations, or those of the publisher, the editors, and the reviewers. Any product that may be evaluated in this article, or claim that may be made by its manufacturer, is not guaranteed or endorsed by the publisher.
Supplementary Material
The Supplementary Material for this article can be found online at: https://www.frontiersin.org/articles/10.3389/fchem.2022.839470/full#supplementary-material
References
Bern, M., Kil, Y. J., and Becker, C. (2012). Byonic: Advanced Peptide and Protein Identification Software. Curr. Protoc. Bioinformatics Chapter 13, Unit 13.20. doi:10.1002/0471250953.bi1320s40
Bollineni, R. C., Koehler, C. J., Gislefoss, R. E., Anonsen, J. H., and Thiede, B. (2018). Large-scale Intact Glycopeptide Identification by Mascot Database Search. Sci. Rep. 8, 2117. doi:10.1038/s41598-018-20331-2
Cao, L., Huang, C., Cui Zhou, D., Hu, Y., Lih, T. M., Savage, S. R., et al. (2021). Proteogenomic Characterization of Pancreatic Ductal Adenocarcinoma. Cell 184, 5031–5052. doi:10.1016/j.cell.2021.08.023
Chen, Y., Sheng, Q., Hong, Y., and Lan, M. (2019). Hydrophilic Nanocomposite Functionalized by Carrageenan for the Specific Enrichment of Glycopeptides. Anal. Chem. 91, 4047–4054. doi:10.1021/acs.analchem.8b05578
Fitos, I., Visy, J., Zsila, F., Mády, G., and Simonyi, M. (2006). Selective Binding of Imatinib to the Genetic Variants of Human α1-acid Glycoprotein. Biochim. Biophys. Acta (Bba) - Gen. Subjects 1760, 1704–1712. doi:10.1016/j.bbagen.2006.08.015
Hwang, H., Jeong, H. K., Lee, H. K., Park, G. W., Lee, J. Y., Lee, S. Y., et al. (2020). Machine Learning Classifies Core and Outer Fucosylation of N-Glycoproteins Using Mass Spectrometry. Sci. Rep. 10, 318. doi:10.1038/s41598-019-57274-1
Kawahara, R., Chernykh, A., Alagesan, K., Bern, M., Cao, W., Chalkley, R. J., et al. (2021). Community Evaluation of Glycoproteomics Informatics Solutions Reveals High-Performance Search Strategies for Serum Glycopeptide Analysis. Nat. Methods 18, 1304–1316. doi:10.1038/s41592-021-01309-x
Keser, T., Tijardović, M., Gornik, I., Lukić, E., Lauc, G., Gornik, O., et al. (2021). High-Throughput and Site-specific N-Glycosylation Analysis of Human Alpha-1-Acid Glycoprotein Offers a Great Potential for New Biomarker Discovery. Mol. Cell Proteomics 20, 100044. doi:10.1074/mcp.ra120.002433
Klein, J. A., and Zaia, J. (2020). A Perspective on the Confident Comparison of Glycoprotein Site-specific Glycosylation in Sample Cohorts. Biochemistry 59, 3089–3097. doi:10.1021/acs.biochem.9b00730
Lee, J. Y., Lee, H. K., Park, G. W., Hwang, H., Jeong, H. K., Yun, K. N., et al. (2016). Characterization of Site-specific N-Glycopeptide Isoforms of α-1-Acid Glycoprotein from an Interlaboratory Study Using LC-MS/MS. J. Proteome Res. 15, 4146–4164. doi:10.1021/acs.jproteome.5b01159
Liu, M.-Q., Zeng, W.-F., Fang, P., Cao, W.-Q., Liu, C., Yan, G.-Q., et al. (2017). pGlyco 2.0 Enables Precision N-Glycoproteomics with Comprehensive Quality Control and One-step Mass Spectrometry for Intact Glycopeptide Identification. Nat. Commun. 8, 438. doi:10.1038/s41467-017-00535-2
Lu, L., Riley, N. M., Shortreed, M. R., Bertozzi, C. R., and Smith, L. M. (2020). O-pair Search with MetaMorpheus for O-Glycopeptide Characterization. Nat. Methods 17, 1133–1138. doi:10.1038/s41592-020-00985-5
Ma, C., Qu, J., Li, X., Zhao, X., Li, L., Xiao, C., et al. (2016). Improvement of Core-Fucosylated Glycoproteome Coverage via Alternating HCD and ETD Fragmentation. J. Proteomics 146, 90–98. doi:10.1016/j.jprot.2016.06.003
Moremen, K. W., Tiemeyer, M., and Nairn, A. V. (2012). Vertebrate Protein Glycosylation: Diversity, Synthesis and Function. Nat. Rev. Mol. Cell Biol 13, 448–462. doi:10.1038/nrm3383
Ohtsubo, K., and Marth, J. D. (2006). Glycosylation in Cellular Mechanisms of Health and Disease. Cell 126, 855–867. doi:10.1016/j.cell.2006.08.019
Palmisano, G., Lendal, S. E., Engholm-Keller, K., Leth-Larsen, R., Parker, B. L., and Larsen, M. R. (2010). Selective Enrichment of Sialic Acid-Containing Glycopeptides Using Titanium Dioxide Chromatography with Analysis by HILIC and Mass Spectrometry. Nat. Protoc. 5, 1974–1982. doi:10.1038/nprot.2010.167
Polasky, D. A., Yu, F., Teo, G. C., and Nesvizhskii, A. I. (2020). Fast and Comprehensive N- and O-Glycoproteomics Analysis with MSFragger-Glyco. Nat. Methods 17, 1125–1132. doi:10.1038/s41592-020-0967-9
Pujić, I., and Perreault, H. (2021). Recent Advancements in Glycoproteomic Studies: Glycopeptide Enrichment and Derivatization, Characterization of Glycosylation in SARS CoV2, and Interacting Glycoproteins. Mass. Spectrom. Rev. doi:10.1002/mas.21679
Riley, N. M., Malaker, S. A., Driessen, M. D., and Bertozzi, C. R. (2020). Optimal Dissociation Methods Differ for N- and O-Glycopeptides. J. Proteome Res. 19, 3286–3301. doi:10.1021/acs.jproteome.0c00218
Saba, J., Dutta, S., Hemenway, E., and Viner, R. (2012). Increasing the Productivity of Glycopeptides Analysis by Using Higher-Energy Collision Dissociation-Accurate Mass-product-dependent Electron Transfer Dissociation. Int. J. Proteomics 2012, 560391. doi:10.1155/2012/560391
Shen, J., Jia, L., Dang, L., Su, Y., Zhang, J., Xu, Y., et al. (2021). StrucGP: De Novo Structural Sequencing of Site-specific N-Glycan on Glycoproteins Using a Modularization Strategy. Nat. Methods 18, 921–929. doi:10.1038/s41592-021-01209-0
Sun, N., Wang, J., Yao, J., and Deng, C. (2017). Hydrophilic Mesoporous Silica Materials for Highly Specific Enrichment of N-Linked Glycopeptide. Anal. Chem. 89, 1764–1771. doi:10.1021/acs.analchem.6b04054
Sun, N., Wu, H., Chen, H., Shen, X., and Deng, C. (2019). Advances in Hydrophilic Nanomaterials for Glycoproteomics. Chem. Commun. 55, 10359–10375. doi:10.1039/c9cc04124a
Virág, D., Kremmer, T., Lőrincz, K., Kiss, N., Jobbágy, A., Bozsányi, S., et al. (2021). Altered Glycosylation of Human Alpha-1-Acid Glycoprotein as a Biomarker for Malignant Melanoma. Molecules (Basel, Switzerland) 26, 6003. doi:10.3390/molecules26196003
Wu, Q., Jiang, B., Weng, Y., Liu, J., Li, S., Hu, Y., et al. (2018). 3-Carboxybenzoboroxole Functionalized Polyethylenimine Modified Magnetic Graphene Oxide Nanocomposites for Human Plasma Glycoproteins Enrichment Under Physiological Conditions. Anal. Chem. 90, 2671–2677. doi:10.1021/acs.analchem.7b04451
Yang, W., Shah, P., Hu, Y., Toghi Eshghi, S., Sun, S., Liu, Y., et al. (2017). Comparison of Enrichment Methods for Intact N- and O-Linked Glycopeptides Using Strong Anion Exchange and Hydrophilic Interaction Liquid Chromatography. Anal. Chem. 89, 11193–11197. doi:10.1021/acs.analchem.7b03641
Yin, X., Bern, M., Xing, Q., Ho, J., Viner, R., and Mayr, M. (2013). Glycoproteomic Analysis of the Secretome of Human Endothelial Cells. Mol. Cell Proteomics 12, 956–978. doi:10.1074/mcp.m112.024018
Yu, Q., Wang, B., Chen, Z., Urabe, G., Glover, M. S., Shi, X., et al. (2017). Electron-Transfer/Higher-Energy Collision Dissociation (EThcD)-Enabled Intact Glycopeptide/Glycoproteome Characterization. J. Am. Soc. Mass. Spectrom. 28, 1751–1764. doi:10.1007/s13361-017-1701-4
Zhang, M., Liu, Y., Zhang, D., Chen, T., and Li, Z. (2017). Facile and Selective Enrichment of Intact Sialoglycopeptides Using Graphitic Carbon Nitride. Anal. Chem. 89, 8064–8069. doi:10.1021/acs.analchem.7b01556
Zhang, Y., Jing, H., Meng, B., Qian, X., and Ying, W. (2020). L-cysteine Functionalized Straticulate C3N4 for the Selective Enrichment of Glycopeptides. J. Chromatogr. A 1610, 460545. doi:10.1016/j.chroma.2019.460545
Zhang, Y., Jing, H., Wen, T., Wang, Y., Zhao, Y., Wang, X., et al. (2019). Phenylboronic Acid Functionalized C3N4 Facultative Hydrophilic Materials for Enhanced Enrichment of Glycopeptides. Talanta 191, 509–518. doi:10.1016/j.talanta.2018.09.016
Zhang, Y., Lin, T., Zhao, Y., Mao, Y., Tao, Y., Huang, Y., et al. (2020). Characterization of N-Linked Intact Glycopeptide Signatures of Plasma IgGs from Patients with Prostate Carcinoma and Benign Prostatic Hyperplasia for Diagnosis Pre-stratification. Analyst 145, 5353–5362. doi:10.1039/d0an00225a
Zhang, Y., Xie, X., Zhao, X., Tian, F., Lv, J., Ying, W., et al. (2018). Systems Analysis of Singly and Multiply O -glycosylated Peptides in the Human Serum Glycoproteome via EThcD and HCD Mass Spectrometry. J. Proteomics 170, 14–27. doi:10.1016/j.jprot.2017.09.014
Zhang, Y., Zheng, S., Mao, Y., Cao, W., Zhao, L., Wu, C., et al. (2021). Systems Analysis of Plasma IgG Intact N-Glycopeptides from Patients with Chronic Kidney Diseases via EThcD-sceHCD-MS/MS. Analyst 146, 7274–7283. doi:10.1039/d1an01657a
Zhang, Y., Zheng, S., Zhao, W., Mao, Y., Cao, W., Zeng, W., et al. (2021). Sequential Analysis of the N/O-Glycosylation of Heavily Glycosylated HIV-1 Gp120 Using EThcD-sceHCD-MS/MS. Front. Immunol. 12, 755568. doi:10.3389/fimmu.2021.755568
Keywords: N-glycosylation, clinical sample, glycoproteomics, mass spectrometry, electron-transfer/higher-energy collisional dissociation (EThcD)-stepped collision energy/higher-energy collisional dissociation (sceHCD)
Citation: Zeng W, Zheng S, Su T, Cheng J, Mao Y, Zhong Y, Liu Y, Chen J, Zhao W, Lin T, Liu F, Li G, Yang H and Zhang Y (2022) Comparative N-Glycoproteomics Analysis of Clinical Samples Via Different Mass Spectrometry Dissociation Methods. Front. Chem. 10:839470. doi: 10.3389/fchem.2022.839470
Received: 20 December 2021; Accepted: 20 January 2022;
Published: 24 February 2022.
Edited by:
Tara Louise Pukala, University of Adelaide, AustraliaReviewed by:
Heeyoun Hwang, Korea Basic Science Institute (KBSI), South KoreaHao Jiang, University of Dundee, United Kingdom
Copyright © 2022 Zeng, Zheng, Su, Cheng, Mao, Zhong, Liu, Chen, Zhao, Lin, Liu, Li, Yang and Zhang. This is an open-access article distributed under the terms of the Creative Commons Attribution License (CC BY). The use, distribution or reproduction in other forums is permitted, provided the original author(s) and the copyright owner(s) are credited and that the original publication in this journal is cited, in accordance with accepted academic practice. No use, distribution or reproduction is permitted which does not comply with these terms.
*Correspondence: Hao Yang, eWFuZ2hhb0BzY3UuZWR1LmNu; Yong Zhang, bmFua2FpMTk4OUBmb3htYWlsLmNvbQ==