- 1Key Laboratory of Theory of TCM, Ministry of Education of China, Shandong University of Traditional Chinese Medicine, Jinan, China
- 2College of Intelligence and Information Engineering, Shandong University of Traditional Chinese Medicine, Jinan, China
The nature theory of Chinese herbal medicines (CHMs) is the core theory of traditional Chinese medicine (TCM). Cold-hot nature is an important part of CHM nature. It is found that the material basis of cold-hot nature is CHM ingredients. To test the scientific hypothesis that “CHMs with similar cold-hot nature should have similar material basis,” we explored an intelligent method for cold-hot nature identification of CHMs based on the feature similarity of CHM ingredients in this work. Sixty one CHMs were selected for cold-hot nature identification. High performance liquid chromatography (HPLC) was used to separate the chemical ingredients of CHMs and extract the feature information of CHM ingredients. A distance metric learning algorithm was then learned to measure the similarity of HPLC fingerprints. With the learned distance metric, cold-hot nature identification scheme (CHNIS) was proposed to build an identification model to evaluate the cold-hot nature of CHMs. A number of experiments were designed to verify the effectiveness and feasibility of the proposed CHNIS model. The total identification accuracy rate of 61 CHMs is 80.3%. The performance of the proposed CHNIS algorithm outperformed that of the compared classical algorithms. The experimental results confirmed our inference that CHMs with similar cold-hot nature had similar composition of substances. The CHNIS model was proved to be effective and feasible.
1 Introduction
The nature theory of Chinese herbal medicines (CHMs) is one of the core contents of traditional Chinese medicine (TCM), which has been concerned by scholars and research institutions for many years. There are four types as for the nature of CHMs: Cool, hot, and warm, which can be summarized as cold and hot nature (Ouyang et al., 2006; Gao and Chen, 2007). Especially, according to this theory, CHMs are used as drugs to treat diseases. Hot nature medicines are used to treat cold syndrome, and cold nature medicines are used to treat hot syndrome. The cold-hot nature theory has become an important principle for TCM treatment (Fu et al., 2017). Therefore, correctly identifying the nature of CHMs is crucial in TCM research.
The field of studying cold-hot nature of CHMs has attracted much attention, and yielded a lot of results. Some studies found that cold-hot nature of CHMs was closely related to energy metabolism, including ATPase activity and oxygen consumption (Mi et al., 2021; Su et al., 2022). CHMs with cold nature can significantly reduce the level of energy metabolism in normal rats (Mi et al., 2021), and CHMs with hot nature may regulate the energy metabolism of skeletal muscle by promoting the decomposition of muscle glycogen and increasing the activity of SDH enzyme, so as to produce more ATP (Huang et al., 2010). Some studies analyzed the cold-hot nature of CHMs according to their material basis (Fu et al., 2017; Wei et al., 2019a). Scientists proposed various scientific hypotheses to prove that the material basis of cold-hot nature was the composition of CHMs. Our group put forward the scientific hypothesis of “tri-element of property-effect-material” and carried out a lot of experimental work to verify it (Zhang, 2012). Many researches have demonstrated that cold-hot nature of CHMs is based on substance (Wei et al., 2019a). Xue’s group explored to extract the ingredient information of CHMs with chemical fingerprints, and build an identification model for nature prediction (Zhang, 2012). Some studies revealed the cold-hot nature of CHMs by bioinformatics methods (Liang et al., 2013; Fu et al., 2017). They concluded that cold nature CHMs possessed the tendency to impact cell growth, proliferation, had sedative function, associated with “mental and behavioural disorders” diseases, and hot nature CHMs were related to inflammation and immunity regulation, had cardio-protection function, associated with “endocrine, nutritional and metabolic diseases.” Although scientists have made some achievements in the field of cold-hot nature, the scientific connotation of cold-hot nature needs to be further explained. Our group is committed to revealing the scientific connotation of CHM nature with chemical fingerprint technique.
A number of studies have found that the material basis of the cold-hot nature of CHMs is chemical ingredients (Zhang, 2012; Liang et al., 2013; Wei et al., 2021a). The current research on medicinal nature concentrated on revealing the correlation between cold-hot nature and CHM ingredients. Generally, studies on correlation between cold-hot nature and CHM ingredients mainly included two parts: information representation and nature identification. Information representation was defined as the feature representation of CHM ingredients. Information representation applies chemical fingerprints (Zhang, 2012), molecular descriptors (Liang et al., 2013), metabolomics (Nie et al., 2015) and original effects (Liu et al., 2012) to extract the feature information of CHM ingredients. Chemical fingerprints, including ultraviolet spectrum, infrared spectrum, liquid chromatography, gas chromatography, have been widely used to analyze the ingredient information of CHMs. Molecular descriptors were usually used to extract the information of CHM compounds. Metabolomics applied zoological experiments to extract the ingredient information of CHMs. Original effects mainly included tranquilizing and activating blood, moistening the lung, invigorating the stomach and other attributes. Nature identification introduced machine learning algorithms or built classification algorithms for discriminating cold-hot nature of CHMs. Zhang (2012) utilized chemical fingerprints to analyze CHM ingredients and built nature identification models with machine learning algorithms (such as partial least square method, support vector machine) for nature identification. Our group explored similarity metric models based on chemical fingerprints of CHMs and built nature classification algorithms to classify cold-hot nature of CHMs. Long et al. (2011) and Wang et al. (2016) represented CHM compounds with molecular descriptors, and introduced classical classifiers for discriminating cold-hot nature, respectively. Nie et al. (2015) summarized Metabolomics information of CHMs and built a random forest model to predict the nature of unknown CHMs. Xue’s research group (Liu et al., 2012; Zhang et al., 2012) summarized original efficacy information of CHMs and applied machine learning algorithms for nature identification of CHMs.
As mentioned above, a number of research achievements have been made in the study of cold-hot nature. However, chemical fingerprint technology for nature identification has not been deeply explored. The previous studies focused on the UV thermal chromatogram (Wei et al., 2019b), but less on HPLC. HPLC has high resolution, which can separate complex chemical components to form a chromatogram composed of high and low peaks. Compared with UV spectrum, HPLC can better quantify and characterize the components of CHMs (Qi et al., 2011). It is possible to obtain higher accuracy of cold-hot nature identification by HPLC. Furthermore, most current studies introduced classical machine learning algorithms to establish nature identification models, resulting in low accuracy rates. Designing a special classification algorithm according to the ingredient information of CHMs may obtain higher accuracy rates. In this study, HPLC technology was used to analyze the ingredient information of CHMs. With the obtained HPLC of CHMs, the similarity of CHM ingredients was quantified as a distance metric. Finally, a special nature identification model was constructed to predict the cold-hot nature of CHMs.
2 Materials and methods
2.1 Chinese herbal medicine dataset
In this study, 61 representative CHMs with clear nature were selected from the classical “Chinese Materia Medica” and “Shen Nong’s Herbal Classic” (Wei et al., 2019a). In these CHMs, 30 CHMs were labeled as cold, and others were labeled as hot. The screening criteria are as follows: 1) Limited to traditional natural plant medicine; 2) CHMs with clear nature, clinically recognizing and no academic dispute. The 61 CHMs are listed in Table 1.
2.2 High performance liquid chromatography
In this study, HPLC was applied to represent the ingredient information of CHMs. The experimental methods of HPLC are as follows (Zhang, 2012).
Instruments and materials: Agilent 1100 high performance liquid chromatograph (DAD detector, binary high pressure gradient pump, column temperature box; Agilent); KQ-250E medical ultrasonic cleaner (Qunshan Ultrasonic Instrument Co., Ltd.); Mettler AE240 electronic balance (Mettler, Switzerland). Acetonitrile (Chromatographic pure, American TEDIA company); Wahaha pure water; Other reagents are analytically pure (Tianjin kemio Chemical Reagent Development Center).
Preparation of the test solution is as follows: accurately weigh about 0.5 g of the test medicinal powder (passing 40 mesh), put it in a conical flask with a stopper, accurately add 50 ml of 50% methanol, weigh the mass, and place it in a 60°C water bath for ultrasound extraction for 30 min, after the extraction is completed, let it cool, weigh again to determine the mass, supplement the lost mass with 50% methanol, shake well, filter, and take the continuous filtrate to obtain a 50% methanol extract. Chromatographic conditions is as follows: 1) Chromatographic column: Agilent xdb-c18 column [(4.6 mm) * 250 mm, 5 μm]. 2) Mobile phase: acetonitrile-water (3:97) → acetonitrile-water (100:0), linear gradient elution for 90 min. 3) Flow rate: 1.0 ml/min. 4) Injection volume: 20 ml. 5) Column temperature: 35°C.
The test solution was determined according to the above chromatographic conditions, and the dad (diode array detector) was used for full wavelength scanning of 190–600 nm. Finally, each CHM was collected at 211 wavelengths of 190–400 nm, and the data at 6,524 retention time points were obtained. Because the amount of data was too large for further modeling and analysis, and the chromatographic data of the same CHM at adjacent wavelengths had great correlation, according to the characteristics of UV wavelength, the chromatographic data at representative wavelengths were selected from each CHM. In this work, the chromatographic data at wavelength of 210 nm were applied to study the nature identification model.
2.3 High performance liquid chromatography fingerprint similarity
In our previous studies, we proposed a scientific hypothesis that CHMs with similar nature hade similar material basis (Wei et al., 2021a). We have demonstrated this hypothesis with UV spectrum (Wei et al., 2019b; Wei et al., 2021b). In this study, we attempted to demonstrate this hypothesis by building a relationship between CHM ingredients and cold-hot nature. HPLC fingerprints were used to extract the ingredients information of CHMs. Therefore, we explored to reveal that CHMs with similar nature had similar HPLC fingerprints. This means that if the HPLC fingerprints of CHMs are similar, we consider they have the similar medicinal nature.
The similarity of HPLC fingerprints has been widely studied for the quality evaluation of CHMs (Mao, 2020). In this paper, the similarity of HPLC fingerprints was introduced to identify cold-hot nature of CHMs with unknown nature. According to the characteristics of HPLC fingerprints, we defined the similarity of HPLC fingerprints as semantic relevance and fingerprint similarity. Semantic relevance describes the consistency of nature labels, which means that HPLC fingerprints of two CHMs are semantically similar if they have the same labels (cold or hot) (Wei et al., 2018). Fingerprint similarity describes the similarity of ingredient information of CHMs, which means that CHM ingredients related to cold-hot nature are similar. We attempted to learn a Mahalanobis distance to evaluate the similarity of CHM ingredients, which preserved semantic relevance and fingerprint similarity. The smaller the Mahalanobis distance is, the more similar CHM ingredients are.
2.3.1 Distance metric learning
The CHM HPLC dataset is defined as
where superscript T denotes the transpose of a vector or a matrix, M is a positive semi-definite matrix, which can be decomposed intoM = AAT. Therefore, Eq. 1 can be rewritten as:
According to Eq. 2, learning
2.3.2 Similarity metric
In this study, a Mahalanobis distance was learned to measure the similarity of CHM ingredients. As mentioned above, the similarity of CHM ingredients was quantified as HPLC similarity, including semantic relevance and fingerprint similarity. Most distance metric learning algorithms mainly focused on semantic relevance among CHM fingerprints by learning a distance metric with a given pairwise constraints. Pairwise constraints divide the dataset into two parts, set of equivalence constraints and the set of inequivalence constraints. The set of equivalence constraints is defined as (
And the set of inequivalence constraints is defined as (
The semantic relevance represents the separability of cold-hot nature, which requires the feature representations of CHM fingerprints in the same class should be closer, and CHM fingerprints in different class should be far away. We built the semantic relevance by optimizing the formula as (Wei et al., 2020):
where
As mentioned above, fingerprint similarity represents the similarity of CHM HPLC fingerprints, which reflects the similarity of CHM ingredients. Inspired by feature similarity of pulmonary nodule images (Wei et al., 2016), we have built a patch alignment framework for the similarity of gas chromatography. In this study, we introduce this framework for quantifying the similarity of HPLC fingerprints. The patch alignment framework for HPLC fingerprint similarity metric is as follows:
Finally, the global alignment (Wei et al., 2021a) becomes:
Given the assumption of linearization that
Therefore, the transformation matrix
where
2.3.3 Projection learning
To solve transformation matrix
In this case, the optimal projections can be calculated by eigenvalue decomposition on matrix
2.4 Cold-hot nature identification scheme
In this study, a cold-hot nature identification scheme (CHNIS) based on similarity metric of HPLC was developed, described in Figure 1. For a CHM with unknown cold-hot nature, we firstly extracted the ingredient information of this CHM by HPLC fingerprints. We then calculated the similarity of HPLC fingerprints between this query CHM and CHMs with clear nature by learning the Mahalanobis distances. The learned Mahalanobis distances were arranged from smallest to largest in order of increasing distance metrics. The most similar
where
2.5 The cold-hot nature identification scheme based on similarity metric of High performance liquid chromatography
2.5.1 The cold-hot nature identification algorithm
Given a CHM HPLC dataset
1) Transformation matrix
2) The Mahalanobis distance
3) Similarity metric. Retrieving the r most similar CHMs corresponding to the r smallest Mahalanobis distances between the query CHM and the CHM dataset.
4) Cold-hot nature classification. Calculating the ratio of the weight of the cold CHMs to the total weight of CHMs retrieved.
2.6 Performance evaluation
In this subsection, numerous experiments were built to assess the feasibility of the constructed CHNIS for cold-hot nature classification. We compared the identification performance of our scheme with that of other classical schemes, including retrieval system (RS) (Wei et al., 2019b), Pearson correlation coefficient (PCC) (Wei et al., 2021b), large margin nearest neighbor (LMNN) (Weinberger et al., 2009), information-theoretic metric learning (ITML) (Davis et al., 2007) and extreme learning machine (ELM) (Wei et al., 2021c). RS and PCC were also applied for cold-hot nature classification of CHMs in our studies. LMNN and ITML were classical distance metric learning models. ELM was used for cold-hot-neutral nature prediction of CHM compounds. All experiments of performance assessment were carried out in the environment of CHM HPLC dataset. The application predicted the cold-hot nature of a CHM by studying the similar CHMs with clear nature. We firstly tested the ingredient information of CHMs by HPLC fingerprints. Secondly, we proposed a CHNIS to classify the cold-hot nature of CHMs. Finally, extensive experiments were constructed to verify the feasibility of our proposed scheme.
In the experiments, extrapolation evaluation and stability evaluation were used to test the performance of our CHNIS. Extrapolation evaluation describes the extent to which cold CHMs can be computed based on the retrieved similar CHMs. Extrapolation evaluation divided the CHM dataset into training dataset and test dataset and calculated the probability that the nature of each test CHM belongs to cold. A Receiver Operating Characteristic (ROC) curve was depicted with varying the threshold of the cold probabilities. The area under the ROC curve (AUC) and classification accuracy (ACC) were introduced to evaluate the performance of our proposed CHNIS. The ACC value can be calculated as,
In Eq. 10,
The second evaluation method, stability evaluation, represents the proportion of retrieved CHMs that are semantically relevant to the query CHMs. Leave-one-out method was used to analyze the stability evaluation in the whole CHM HPLC fingerprints. Each time, one CHM was selected as the query sample, and remaining 60 CHMs were used as the training samples. The cold probability of each CHM can be obtained according to the calculated
3 Results
3.1 Parameter configurations
In our experiments, several parameters in CHNIS were analyzed to classify the cold-hot nature of CHMs. The tuning parameter
In this study, the stability evaluation was performed to configure the parameters for the optimal CHNIS model. AUC and ACC values were computed to analyze the performance of our CHNIS with varying the parameters (
In this study, we investigated the effect of parameter
Furthermore, the number of retrieved CHMs r in CHNIS was configured for evaluating the identification performance of our model. The value of parameter r was test within the range [1, 3, 5, 7, 10, 12, 15, 20]. Figure 4 shows the AUC and ACC curves with different parameter r. From this figure, AUC and ACC curves fluctuate with different r values, which means that the performance of our CHNIS tends to change slightly with the increase of r. Comprehensively analyzing the AUC and ACC curves, our CHNIS achieves optimal performance r = 5. In this experiment, the tradeoff parameter
3.2 Performance evaluation
Several experiments were constructed to demonstrate the feasibility of our proposed CHNIS model for classifying cold-hot nature of CHMs. We compared the classification performance of our CHNIS with that of classifiers applied in our nature studies (RS, PCC, and ELM) and some classical distance metric learning models (i.e., LMNN, ITML). RS and PCC were utilized as comparative references to analyze the similarity of HPLC fingerprints.
Table 2 shows the performance comparison of extrapolation evaluation between CHNIS and other models. Extrapolation evaluation experiments randomly selected 40 CHMs as the training dataset and the remaining CHMs as test dataset. In training dataset, the number of cold CHMs and hot CHMs were about 20, respectively. According to the comparison of identification performance, we draw the following conclusions. Firstly, the identification performance of our scheme CHNIS for cold-hot nature is better than that of the comparison algorithms, which means that CHNIS, comprehensively considering semantic relevance and fingerprint similarity, has the best performance for identifying cold-hot nature. Therefore, our scheme can better mine the ingredient information of CHMs to identify the cold-hot nature. Secondly, distance metric learning algorithms (including ITML, LMNN, RS) are more accurate in nature identification than PCC and ELM. This illustrates that it is more effective for nature identification with similarity metric of CHM ingredients, and also demonstrates the hypothesis that CHMs with similar medicinal nature have similar material basis. Thirdly, ELM with HPLC is poor in predicting cold-hot nature. Finally, the extrapolation evaluation experiments demonstrate the feasibility of our scheme.
Stability evaluation experiments were preformed to compare the identification performance between CHNIS and other algorithms. Table 3 displays the comparison results, which draws a similar conclusion to Table 2. We reach the following conclusions. Firstly, the performance of our scheme CHNIS outperforms that of the comparison models. Secondly, distance metric learning algorithms used in this study can better mine the ingredients information of CHMs than ELM and PCC in predicting cold-hot nature. Thirdly, our CHNIS has the best stability evaluation. Finally, extrapolation evaluation and stability evaluation experiments comprehensively demonstrate the effectiveness and feasibility of our CHNIS.
3.3 Nature identification examples
Leave-one-out method was utilized to present the examples of nature identification. Two query CHMs, including Rhei Radix et Rhizoma (cold) and Asari Radix et Rhizoma (hot), were chosen as the instances to interpret the principle of nature identification. Table 4 reports two retrieval CHM instances returned by our CHNIS model. In this table, query CHMs are showed in the second row and top k = 7 similar reference CHMs are listed in other rows. The similar reference CHMs were arranged in the order of monotonically increasing Mahalanobis distance. Rhei Radix et Rhizoma was selected as a representative query cold medicine for research. The retrieved CHMs were six reference CHMs with cold nature and one reference CHM with hot nature. The cold nature probability was calculated as 92.1%, which meant that the query CHMs was probably cold. Asari Radix and Rhizoma was selected as a representative query hot medicine for research. The retrieved CHMs were six reference CHMs with hot nature and one reference CHM with cold nature. The cold nature probability was calculated as 6.9%, which meant that the query CHMs was probably hot. The identification examples indicate that there is a correlation between CHM ingredients and cold-hot nature.
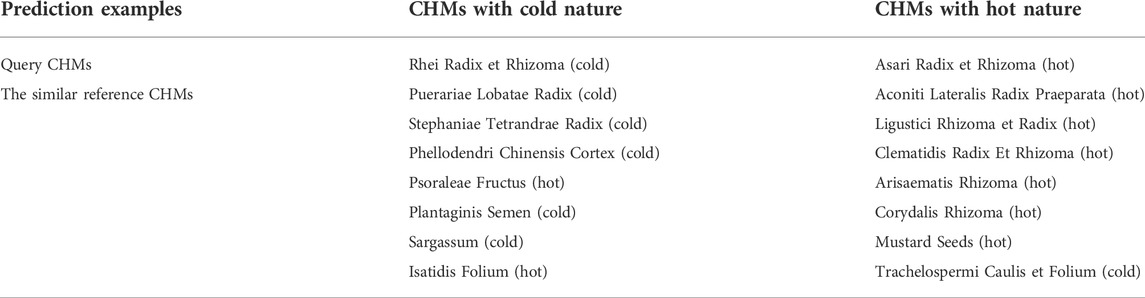
TABLE 4. The nature identification examples. The top k = 7 similar CHMs are arranged in the order of monotonically increasing Mahalanobis distance. Cold/hot nature labels are denoted in the brackets.
3.4 Overall identification performance
In this study, the overall performance of our CHNIS was assessed with evaluation indices, including confusion matrix, recall, precision and F-score. All evaluation indices were calculated by leave-one-out method. The confusion matrix for nature identification of 61 CHMs is shows in Table 5. The prediction accuracy rate of cold CHMs is 83.3% (25/30), while the classification accuracy rate of hot CHMs is 77.4% (24/31). Therefore, the total identification accuracy rate of 61 CHMs is 80.3% (49/61). Our scheme has higher prediction accuracy for cold CHMs, but lower prediction accuracy for hot CHMs. The recall, precision and F-score of nature prediction of 61 CHMs are showed in Table 6. From Tables 5, 6, we find that our scheme is effective in the prediction of cold-hot nature with HPLC fingerprints. CHM ingredients are closely related to cold-hot nature.
4 Discussion
HPLC is an important analytical method in the research of CHM ingredients in recent years. HPLC can quantitatively and qualitatively analyze the ingredients of CHMs. Our group previously used UV spectrum to analyze the ingredients of CHMs, and established a nature identification model for classifying cold-hot nature. Table 7 displays the performance comparison of cold-hot nature evaluation of related studies. All related studies are carried out in the environment of 61 representative CHM dataset. The experimental results show that HPLC has a higher prediction accuracy rate, which means that HPLC can better extract the characteristics of cold-hot nature of CHM ingredients.
HPLC fingerprints present a challenge to classical classification algorithms, which leads to a low classification accuracy. The same conclusion is also obtained in the traditional similarity metric methods, such as Pearson correlation. These methods perhaps suffer from high dimensionality of data. Furthermore, the classical algorithms are all general classification algorithms. These methods may not be able to mine the characteristic information of CHM ingredients, which makes the identification accuracy of cold-hot nature low.
According to the hypothesis that CHMs with the same cold-hot nature have the similar material basis, a distance metric learning method is introduced to quantify the similarity of CHM ingredients as distance metric, and build a cold-hot nature identification scheme for understanding material basis of CHMs. Distance metric learning methods, such as LMNN and ITML, only focus on the semantic of relevance of CHM ingredients without considering fingerprint similarity. Experiments indicate that semantic relevance alone cannot represent all the similarity measures. Here we combine semantic relevance and fingerprint similarity to represent the similarity of CHM ingredients. This model can better mine the information of CHM ingredients. Experiments find that fingerprint similarity can improve the performance of the model. We assume fingerprint similarity is an important part of similarity measurement.
As a classical classifier, ELM used in our study is a general classifier, which does not consider the characteristics of CHM fingerprints. This results in low nature prediction accuracy because of the small samples and high dimensionality of CHM fingerprints in this study. Our CHNIS is built for cold-hot nature identification base on the hypothesis that CHMs with the same cold-hot nature have similar material basis. Compared with classical classifiers, our CHNIS not only models the class separability of fingerprints, but considers the fingerprint similarity. The experimental results also show that our model achieves good classification rate.
However, there are some limitations to our study. First, this research only analyzes CHM ingredients with HPLC fingerprints. Other chemical fingerprints are not taken into account in this study. Multi-fingerprints technology perhaps improves the nature identification accuracy. Therefore, multi-fingerprints fusion algorithm for cold-hot nature identification of CHMs is the focus of follow-up attention. Second, we define the similarity of CHM HPLC as a distance metric. The HPLC fingerprints are small sample and high dimension, which makes classifiers difficult to perform. On the basis of such characteristics, we are committed to designing the forecasting models in the future. Third, this study focuses on building a similar model to identify cold-hot nature of CHMs by CHM HPLC characteristics. The information of CHM ingredients has not been thoroughly mined. In the future, we will explore other chemical fingerprints to extract the information of CHM ingredients for cold-hot nature classification.
5 Conclusion
In this study, a CHNIS for cold-hot nature identification of CHMs is proposed. CHM HPLC fingerprints are applied to extract the information of CHM ingredients. Based on CHM HPLC fingerprints, effective experiments demonstrate that the performance of our scheme outperforms that of the comparative classifiers in classifying cold-hot nature. The overall identification accuracy of 61 CHMs reached 80.3%. According to the experimental results, we find that CHM ingredients are closely related to the cold-hot nature of CHMs. Furthermore, we demonstrate the feasibility of scientific hypothesis that CHMs with the same cold-hot nature have similar material basis.
Data availability statement
The raw data supporting the conclusion of this article will be made available by the authors, without undue reservation.
Author contributions
GW conceived and designed the project, RJ and ZK analyzed the Chinese medicine data sets. CJ assisted in revising the manuscript. ZW collected data and provided expert knowledge. All authors read and approved the final manuscript.
Funding
This work was supported by the national key basic research development program (973 Program) (No. 2007CB512600); National Natural Science Foundation of China (No. 81473369); Key research and development plan of Shandong province (No. 2016CYJS08A01-1); Shandong Province TCM science and technology development plan project (2019-0037).
Conflict of interest
The authors declare that the research was conducted in the absence of any commercial or financial relationships that could be construed as a potential conflict of interest.
Publisher’s note
All claims expressed in this article are solely those of the authors and do not necessarily represent those of their affiliated organizations, or those of the publisher, the editors and the reviewers. Any product that may be evaluated in this article, or claim that may be made by its manufacturer, is not guaranteed or endorsed by the publisher.
References
Davis, J. V., Kulis, B., Jain, P., Sra, S., and Dhillon, I. S. (2007). “Information-theoretic metric learning,” in Proc. of the International Conference on Machine Learning, Corvallis, Oregon, USA, 209–216.
Fu, X., Mervin, L. H., Li, X., Yu, H., Li, J., Mohamad Zobir, S. Z., et al. (2017). Toward understanding the cold, hot, and neutral nature of Chinese medicines using in silico mode-of-action analysis. J. Chem. Inf. Model. 57, 468–483. doi:10.1021/acs.jcim.6b00725
Gao, J., and Chen, C. (2007). Discussion on scientific connotation of four natures of Chinese materia medica. Acta Univ. Tradit. Med. Sin. Pharmacol. Shanghai. 21, 16–18. doi:10.16306/j.1008-861x.2007.06.018
Huang, L., Peng, S., Hu, Q., Meng, X., Yu, R., Liu, H., et al. (2010). Effect on energy metabolism factors in rat skeletal muscle by hot Chinese drugs. Chin. J. Tradit. Med. Pharm. 25, 228–230. CNKI:SUN:BXYY.0.2010-02-021.
Liang, F., Li, L., Wang, M., Niu, X., Zhan, J., He, X., et al. (2013). Molecular network and chemical fragment-based characteristics of medicinal herbs with cold and hot properties from Chinese medicine. J. Ethnopharmacol. 148, 770–779. doi:10.1016/j.jep.2013.04.055
Liu, W. H., Li, Y., Ji, Y. J., Wang, P., Zhang, Y. Q., and Xue, F. Z. (2012). Partial least squares in the discrimination of traditional Chinese herbal medicine property. J. Shandong Univer. Heal. Sci.) 50, 151–154. CNKI:SUN:SDYB.0.2012-01-036.
Long, W., Liu, P., Xiang, J., Pi, X., Zhang, J., and Zou, Z. (2011). A combination system for prediction of Chinese Materia Medica properties. Comput. Methods Programs Biomed. 101, 253–264. doi:10.1016/j.cmpb.2011.01.006
Mao, C. (2020). Study on quality evaluation standard of the flower of chrysanthemum morifolium Ramat based on the correlation of ingredients and efficacy. Beijing: China Academy of Chinese Medical Sciences. doi:10.27658/d.cnki.gzzyy.2020.000040
Mi, W., Yang, C., Ke, Y., Wang, M., Shan, Z., Li, Y., et al. (2021). Discussion on cold and heat properties of Chrysanthemi Flos in normal rats based on substance and energy metabolism. Chin. Tradit. Herb. Drugs. 52, 799–807. doi:10.7501/j.issn.0253-2670.2021.03.023
Nie, B., Hao, Z. L., Gui, B., Wang, Z., Du, J. Q., Wang, G. L., et al. (2015). The research for metabolomics discriminant method for cold and hot property of traditional Chinese medicine based on random forest. J. Jiangxi Univ. Tradit. Chin. Med. 27, 82–86. CNKI:SUN:XYXB.0.2015-02-033.
Ouyang, B., Wang, Z., and Wang, P. (2006). Researech thinking and method of modern study on four properties theory of Chinese materia medica. J. Beijing Univ. Tradit. Chin. Med. 29, 592–594. doi:10.3321/j.issn:1006-2157.2006.09.004
Qi, F., Rong, R., and Xue, F. (2011). Application of the Bayesian netw ork in Chinese herbal medicine property recognition. J. Shandong Univ. Heal. Sci.) 49, 147–152. CNKI:SUN:SDYB.0.2011-05- 034.
Su, F., Bai, C., Zhang, W., Yang, J., Hu, J., Sun, Y., et al. (2022). Study on drug properties of Arisaematis Rhizoma and Arisaema Cum Bile based on substance and energy metabolism in normal and cold/heat syndrome model rats. China J. Chin. Mat. Med. 2022, 1–9. doi:10.19540/j.cnki.cjcmm.20220524.402
Wang, M., Li, L., Yu, C., Yan, A., Zhao, Z., Zhang, G., et al. (2016). Classification of mixtures of Chinese herbal medicines based on a self-organizing map (SOM). Mol. Inf. 35, 109–115. doi:10.1002/minf.201500115
Wei, G., Cao, H., Ma, H., Qi, S., Qian, W., and Ma, Z. (2018). Content-based image retrieval for lung nodule classification using texture features and learned distance metric. J. Med. Syst. 42, 13. doi:10.1007/s10916-017-0874-5
Wei, G., Fu, X., He, X., Qiu, P., Yue, L., Rong, R., et al. (2021a). Cold-hot nature identification based on GC similarity analysis of Chinese herbal medicine ingredients. RSC Adv. 11, 26008–26015. doi:10.1039/D1RA04189D
Wei, G., Fu, X., and Wang, Z. (2019a). Multisolvent similarity measure of Chinese herbal medicine ingredients for cold-hot nature identification. J. Chem. Inf. Model. 59, 5065–5073. doi:10.1021/acs.jcim.9b00682
Wei, G., Fu, X., and Wang, Z. (2021c). Nature identification of Chinese herbal medicine compounds based on molecular descriptors. J. AOAC Int. 104, 1754–1759. doi:10.1093/jaoacint/qsab002
Wei, G., Fu, X., and Wang, Z. (2019b). Similarity measurement of Chinese medicine ingredients for cold-hot nature identification. TMR Mod. Herb. Med. 2, 183–191.
Wei, G., Fu, X., Wang, Z., and Zhou, H. (2021b). Cold-hot nature identification of Chinese medicine based on an ultraviolet chemical fingerprint. Spectroscopy 36, 23–29.
Wei, G., Ma, H., Qian, W., and Qiu, M. (2016). Similarity measurement of lung masses for medical image retrieval using kernel based semisupervised distance metric. Med. Phys. 43, 6259–6269. doi:10.1118/1.4966030
Wei, G., Zhang, F., Fu, X., and Wang, Z. (2020). Similarity measurement of traditional Chinese medicine components for cold-hot nature discrimination. Data analy. kowl. Discov. 41, 75–83. doi:10.11925/infotech.2096-3467.2019.0974
Weinberger, K. Q., Blitzer, J., and Saul, L. K. (2009). Distance metric learning for large margin nearest neighbor classification. J. Mach. Learn. Res. 10, 207–244. doi:10.1007/s10845-008-0108-2
Zhang, X. X., Li, Y., Ji, Y. J., Wang, P., Zhang, Y. Q., and Xue, F. Z. (2012). Discrimination of properties of Chinese traditional medicine with principal component analysis-linear discriminant analysis. J. Shandong Univ. Heal. Sci.) 50, 143–146. doi:10.6040/j.issn.1671-7554.2012.01.033
Keywords: Chinese herbal medicines, nature identification, HPLC, similarity metric, cold-hot nature
Citation: Wei G, Jia R, Kong Z, Ji C and Wang Z (2022) Cold-hot nature identification of Chinese herbal medicines based on the similarity of HPLC fingerprints. Front. Chem. 10:1002062. doi: 10.3389/fchem.2022.1002062
Received: 24 July 2022; Accepted: 05 September 2022;
Published: 20 September 2022.
Edited by:
Zheng Han, Shanghai Academy of Agricultural Sciences, ChinaCopyright © 2022 Wei, Jia, Kong, Ji and Wang. This is an open-access article distributed under the terms of the Creative Commons Attribution License (CC BY). The use, distribution or reproduction in other forums is permitted, provided the original author(s) and the copyright owner(s) are credited and that the original publication in this journal is cited, in accordance with accepted academic practice. No use, distribution or reproduction is permitted which does not comply with these terms.
*Correspondence: Guohui Wei, Ym1pZTUzMEAxNjMuY29t; Zhenguo Wang, emhlbmd1b3dAMTI2LmNvbQ==