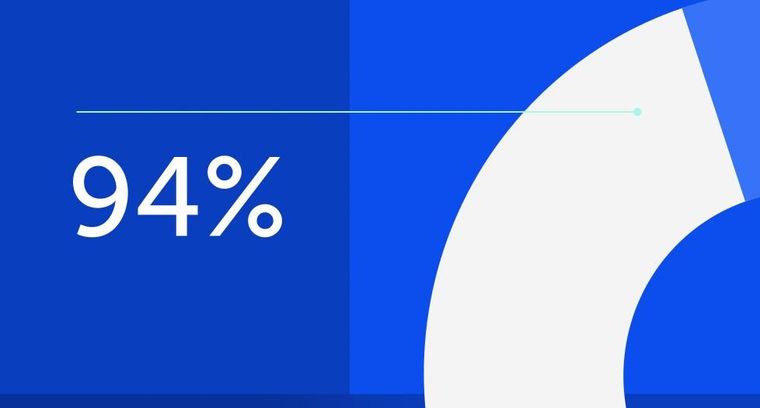
94% of researchers rate our articles as excellent or good
Learn more about the work of our research integrity team to safeguard the quality of each article we publish.
Find out more
REVIEW article
Front. Chem., 29 April 2021
Sec. Theoretical and Computational Chemistry
Volume 9 - 2021 | https://doi.org/10.3389/fchem.2021.662688
This article is part of the Research TopicComputer-Aided Drug Design: Drug Discovery, Computational Modelling, and Artificial IntelligenceView all 15 articles
Natural products are continually explored in the development of new bioactive compounds with industrial applications, attracting the attention of scientific research efforts due to their pharmacophore-like structures, pharmacokinetic properties, and unique chemical space. The systematic search for natural sources to obtain valuable molecules to develop products with commercial value and industrial purposes remains the most challenging task in bioprospecting. Virtual screening strategies have innovated the discovery of novel bioactive molecules assessing in silico large compound libraries, favoring the analysis of their chemical space, pharmacodynamics, and their pharmacokinetic properties, thus leading to the reduction of financial efforts, infrastructure, and time involved in the process of discovering new chemical entities. Herein, we discuss the computational approaches and methods developed to explore the chemo-structural diversity of natural products, focusing on the main paradigms involved in the discovery and screening of bioactive compounds from natural sources, placing particular emphasis on artificial intelligence, cheminformatics methods, and big data analyses.
Graphical Abstract. Assessment of the chemo-structural space of natural products using in silico tools.
The high structural and physicochemical diversity of natural products makes them a valuable source to discover and develop new bioactive compounds with different pharmaceutical, cosmetic, biotechnological, agrochemical, and food applications (Rayan et al., 2017). Success histories of natural product-based drugs have been reported in the pharmaceutical industry and include pilocarpine, quinine, morphine, and artemisinin (Newman and Cragg, 2016; Zhang L. et al., 2020). Natural products represent relevant importance in the discovery and development of new bioinspired bioactive compounds, and more than 50% of the developed drugs approved by the United States Food and Drug Administration (USFDA, 1981–2019) are derived or bioinspired from compounds obtained from natural sources (Newman and Cragg, 2020). Natural products are chemically complex and differ from synthetic compounds in different aspects; as an example, these structures contain a high percentage of oxygen as well as a larger fraction of sp3-hybridized atoms and chiral centers (Lee and Schneider, 2001; Feher and Schmidt, 2003; Rodrigues et al., 2016), and their chemical space is highly diverse, containing different structural scaffolds, when compared with synthetic compound libraries (Chen et al., 2018). Due to their unique features, their structures can provide an innovative solution for the design and synthesis of new bioactive compounds (Kumar et al., 2017; Silva et al., 2019; Bradley et al., 2020; Morais et al., 2020).
The systematic search for natural sources to obtain valuable compounds to develop products with commercial value and industrial purposes remains the most challenging task in bioprospecting (Skirycz et al., 2016; Roumpeka et al., 2017; Cubillos et al., 2019). The traditional approach to discover new bioactive compounds from natural sources includes sequential steps that are obtained from the biological material using ethnological knowledge, extraction, fractionation/isolation, chemical characterization, and, finally, the execution of the biological assays of the isolated or fractionated natural products (Zhang L. et al., 2020). Subsequent analyses include the lead compound optimization using chemical synthesis to perform structural modifications in order to improve their pharmacodynamic and pharmacokinetic properties and to increase their biological activities (Huffman and Shenvi, 2019). In contrast, bioprospecting strategies that use computational tools have been reported as efficient, low-cost, low-labor, and low-time approaches when compared to experimental methods that use solely in vitro and in vivo assays (Li and Vederas, 2009; Wingert and Camacho, 2018; Trujillo-Correa et al., 2019).
Despite natural products being continually explored in drug development programs, attracting the attention of scientific research efforts due to their pharmacophore-like structures, pharmacokinetic properties, and unique chemical space, the big pharma industry has focused on cutting-edge technologies that combine high-throughput screening and combinatory chemistry methods to obtain and evaluate synthetic compound libraries (Henninot et al., 2018; Batool et al., 2019). This decision is, in part, a consequence of the complex structures of natural products that impose limitations in synthetic routes and due to the time-consuming and laborious process involved in the isolation of a single chemical constituent, which often requires a significant amount of reagents and adequate infrastructure, obtaining low yields of purified target compounds (Huffman and Shenvi, 2019). Based on these limitations, the isolation and the characterization of compounds from natural sources have been indicated only for those with potential applications and desirable biological activities (Olivon et al., 2017). However, it has been suggested that the reduced new chemical entities found by the pharmaceutical industry that reach the final market could be due to the strategic decision to prioritize combinatorial synthetic libraries instead of natural product-based libraries (Over et al., 2013; Rodrigues, 2017). Currently, we are witnessing a resurgence of natural products in the development and research of novel bioactive compounds; besides, some structural scaffolds obtained from different classes of natural products, such as alkaloids, phenylpropanoids, polyketides, and terpenoids, have served as an inspiration to design new drug candidates (Thomford et al., 2018; Davison and Brimble, 2019; Galúcio et al., 2019; Li et al., 2019). Natural products remain inspiring the development of new drugs, cosmetics, and other bioactive compounds for human use (Newman and Cragg, 2020; Atanasov et al., 2021).
Recently, metabolomics and metabolic profiling approaches have explored novel taxonomic groups from the unique environment, providing opportunities for finding novel natural bioactive compounds, and some examples include bacteria (Kleigrewe et al., 2015; Gosse et al., 2019), cnidaria (Santacruz et al., 2020), marine sponge (Abdelhameed et al., 2020), insects (Klupczynska et al., 2020), and fungi (Oppong-Danquah et al., 2018). Special attention has been given to novel chemical entities that originated from marine environments due to their diverse and unique drug-like scaffolds (Shang et al., 2018) and physicochemical properties (Jagannathan, 2019) when compared with natural products of terrestrial origin, which make them a valuable source for exploration by the pharmaceutical and biotechnological industries. Advances in the experimental methods applied in metabolomic approaches coupled with computational methods have been useful to identifying new natural products with plausible biological activities as well as to understanding their molecular mechanisms of action (Atanasov et al., 2021).
Currently, artificial intelligence algorithms (Wolfe et al., 2018; Lima et al., 2020; Stokes et al., 2020) and omics-based technologies (Floros et al., 2016; Huang et al., 2017; Jones and Bunnage, 2017; Merwin et al., 2020) have emerged as approaches to characterize and select interesting chemo-structures with appropriate physicochemical properties and biological activities as well as to prioritize the isolation of natural compounds from biological sources (Chen et al., 2018; Wolfender et al., 2019), which open up new opportunities to explore their industrial applications. Combined with other in silico analyses, artificial intelligence and cheminformatics methods can screen a high diversity of chemo-structures isolated from natural sources or deposited in public databases (Chen and Kirchmair, 2020), analyzing their bioactivity, pharmacodynamics, and their pharmacokinetic properties, thus reducing the financial efforts involved in research programs that aim to find new chemical agents (Chen et al., 2018; Al Sharie et al., 2020; Medina-Franco and Saldívar-González, 2020).
In this review, we discuss the computational approaches and methods applied to explore the chemo-structural diversity of natural products, giving particular attention to the main paradigms involved in the discovery and screening of bioactive natural compounds with different industrial applications (e.g., herbicides, insecticides, etc.) that are beyond the discovery of new drugs. Here, we emphasize computational strategies that use artificial intelligence, cheminformatics, and big data analyses that have been developed in the last years. We also explore the limitations and biases of these methods and demonstrate practical applications to evaluate the chemical entities obtained from natural sources aiming at bioprospecting.
Virtual screening methods have innovated the discovery of new compounds with specific bioactivity, assessing in silico large structural libraries against a bioreceptor or biological system, thus favoring the reduction of financial efforts, infrastructure, and the time involved in the process of discovering new chemo-structures (Macalino et al., 2015). These methods apply sequential and hierarchical steps that aim at filtering and selecting compounds with desirable physicochemical, pharmacokinetic, and pharmacodynamic properties while discarding those that do not fit the desirable characteristics. A virtual screening workflow comprises two main computational tasks (Figure 1A): (1) the first one is the library preparation, which includes, among other computational tasks, obtaining the structures of the compounds, file conversion to readable formats, such as SMILES (simplified molecular-input line entry system), SDF (structure data file), and MOL2 (MDL Molfile) (Saldívar-González et al., 2020), conformer generation, and the correction of stereochemical and valence errors (Ropp et al., 2019); (2) the second one corresponds to the application of computational techniques to filter the desirable compounds (Gimeno et al., 2019). The final step corresponds to experimental validation using in vitro and in vivo assays, which include enzymatic inhibition assays and/or cell line inhibition (Spyrakis et al., 2019; Ye et al., 2019).
Figure 1. (A) Sequential steps applied in virtual screening workflows to select bioactive natural products. (B) Ligand- and structure-based virtual screening approaches and some of their associated computational methods.
Different computational methods have been developed over the years and implemented in virtual screening strategies (Tomar et al., 2018), applying knowledge of artificial intelligence (Gupta et al., 2013; Yang et al., 2018; Schaduangrat et al., 2019; Shoombuatong et al., 2019; Kong et al., 2020), molecular modeling (Semighini et al., 2011; Rampogu et al., 2018; Da Costa et al., 2019; Jin et al., 2020; Mascarenhas et al., 2020), statistics, and probability (Pire et al., 2015; Daina and Zoete, 2016; Blanco et al., 2018; Madzhidov et al., 2020; Cai et al., 2021). These methods, when combined with experimental approaches, increase the success to finding novel bioactive compounds (Kumar and Zhang, 2015; Coimbra et al., 2020; Gorgulla et al., 2020; Stokes et al., 2020). Two computational approaches are related to the virtual screening of compounds: (1) the ligand-based virtual screening (LBVS) and (2) structure-based virtual screening (SBVS) approaches (Figure 1B). Both computational approaches have been combined in virtual screening strategies that aim to identify novel bioactive compounds against a specific molecular target or a biological system (Da Costa et al., 2019; Galúcio et al., 2019; Wang et al., 2020).
The LBVS approach depends solely on the analyses of the intrinsic characteristics of the compound structure, such as the electronic, topological, physicochemical, and structural properties that are related to its molecular activity using, as a starting point, a set of compounds with experimentally proven biological activity (Hamza et al., 2012; Berenger et al., 2017; Garcia-Hernandez et al., 2019). Computational methods applied in the LBVS approach include structural-, three-dimensional (3D) shape-, and fingerprint-based similarity search methods, cheminformatics filters, machine learning algorithms, ligand-based pharmacophore modeling, and quantitative structure–activity relationship (QSAR) methods (Yan et al., 2016; Tahir et al., 2020). In contrast, the SBVS approach uses, as a starting point, information related to the molecular recognition of the ligand in the bioreceptor structure to design and discover new bioactive compounds. This information includes bioreceptor conformation, the ligand-binding affinity, intermolecular interactions, molecular surface charge, and the composition of the residue of the binding site (Gonczarek et al., 2018; Guedes et al., 2018; Yasuo and Sekijima, 2019; Maia E. H. B. et al., 2020). These methods require the elucidated 3D structure of the receptor and, preferably, in complex with the bioactive compound. The 3D structure informs the structural conformation and molecular binding site of the bioactive ligands. Among the computational methods applied in the SBVS approach, we can cite molecular docking, molecular dynamics simulation, and structure-based pharmacophore modeling (Wang et al., 2020). Currently, virtual screening methods are an integral part of the design and discovery process of new bioactive compounds, and their applications have become popular in the academia and industry (Kar and Roy, 2013).
The prediction of the pharmacokinetics and drug-likeness properties of chemical entities represents an important task for the discovery of structures with interesting biological activity (Mignani et al., 2018). In essence, drug-likeness represents a measure of the overall similarity of the analyzed compounds to a chemical space occupied by known drugs (Mignani et al., 2018; Jia et al., 2020).
The prediction of the chemical properties of compounds usually involves the application of a set of simple empirical chemical rules (Gfeller et al., 2014; Lagorce et al., 2015; Daina and Zoete, 2016). Over the years, different cheminformatics filters (also known as molecular filters) have been developed as useful tools to screen structures that have desirable pharmacokinetic and pharmacodynamic properties, low toxicity, and/or low promiscuity/reactivity in inhibition assays, thus guiding the decision-making process in the discovery of new chemical entities with pharmaceutical, cosmetic, agrochemical, and biotechnological interest (Huggins et al., 2011). The most commonly used filters are intended to remove from structural libraries the compounds with low cell membrane permeability or distribution. Among the well-known cheminformatics filters, we can cite those developed by Lipinski (Lipinski et al., 1997), Veber (Veber et al., 2002), and Jeffrey (Jeffrey and Summerfield, 2010). Some structural properties evaluated by these molecular filters predict some pharmacodynamic properties, such as compound promiscuity, i.e., their non-selectivity against a molecular target (Walters and Namchuk, 2003; Lovering, 2013). Some filters are based on the selection of a range of physicochemical and structural properties that are representative of specific pharmacokinetics (e.g., gastrointestinal absorption or penetration into the blood–brain barrier) and pharmacodynamic properties (e.g., specificity or promiscuity to a macromolecular target). These properties are selected using a statistical cutoff (e.g., 90th percentile limit) for each molecular descriptor that is representative to explain the interesting feature of the analyzed compounds (Daina and Zoete, 2016).
Since the first report of the chemical rules elected by Lipinski et al. (1997)—also known as the rule of five (RO5) and Pfizer rules—different chemical extensions to these chemical properties have been developed over the years to better define the “drug-like” features and bioavailability of compounds (Doak et al., 2014). More recently, hybrid methods that combine some counting schemes similar to Lipinski's rules with a set of functional groups identified as reactive, toxic, and problematic moieties have also been developed to eliminate promiscuous structures from the high-throughput screening assays (Walters and Murcko, 2002; Bruns and Watson, 2012). Filters have also been developed to screen fragment-based chemical libraries (rule of three, RO3) (Jhoti et al., 2013). Similar to filters developed for drugs, molecular filters have also been developed to select herbicide-, fungicide-, and insecticide-likeness due to their applications in the agrochemical industry (Tice, 2001; Avram et al., 2014).
Despite these molecular filters having been widely applied in virtual screening approaches to select natural products from large chemo-structural libraries (Thireou et al., 2018; Da Costa et al., 2019; Galúcio et al., 2019), caution must be taken to avoid remotion of the chemo-structures with appropriate bioavailability (Shultz, 2019). Most natural products break some chemical rules applied in molecular filtering; furthermore, some chemical classes of compounds, such as peptides and polyketides (e.g., macrolides), are located beyond the chemical limits determined by the rule of five (beyond the rule of five, bRO5) (Doak et al., 2014; Naylor et al., 2017; Rossi Sebastiano et al., 2018). Contrasting to the drug-likeness, the natural product-likeness concept has been developed to measure the overall molecular diversity of the natural product space, and it has been used as a selection criteria to screen substructures for the prioritization of combinatorial synthesis, aiming at novelty and the easy design of building blocks (Ertl et al., 2008; Jayaseelan et al., 2012). Currently, there are a great variety of cheminformatics programs that calculate these chemical properties that compose the cheminformatics filters, including the open-source programs Osiris DataWarrior [operating system (OS) compatibility: Linux/MS-Windows/Mac OS] (Sander et al., 2015) and RDKit (OS compatibility: Linux/MS-Windows/macOS) (Lovrić et al., 2019), and some commercial solutions, such as Instant JChem (OS compatibility: Linux/MS-Windows/macOS) (Instant JChem 21.4.0, 2021). Similar to these applications, the FAF-Drugs4 web server also predicts some chemical properties to screen structures from large compound libraries using some in-house cheminformatics filters, such as the Drug-Like Soft and Lead-Like Soft that predict compound similarity to drugs and leads, respectively (Miteva et al., 2006). Some databases also offer online tools to evaluate the drug-likeness and natural product-likeness (Sorokina and Steinbeck, 2019; Jia et al., 2020). Table 1 exhibits an overview of the main molecular filters applied to screen natural products from chemical libraries.
Table 1. Structural and physicochemical properties present in some cheminformatics filters applied in virtual screening.
Similarity search methods applied in the screening of natural products are based on the premise that molecules with similar structures have similar biological activities (Cereto-Massagué et al., 2015). These methods have been applied to evaluate natural compound similarities, their bioactivity (Muegge and Mukherjee, 2016), and potential molecular targets (Huang et al., 2018).
Molecular fingerprint-based methods use representations of chemical structures to allow the quantitative assessment of the pairwise similarity of compounds with computationally efficient calculations (Riniker and Landrum, 2013; Bajusz et al., 2015). Molecular fingerprints are binary representations (bits) of a chemical structure in which 1 (present) denotes the existence of a certain molecular feature and 0 (absent) denotes inexistence (Rácz et al., 2018). Figure 2A shows a schematic view of the binary representation of a molecular fingerprint of a compound structure. Molecular fingerprints can vary greatly concerning the applied molecular descriptors, and some of them are based solely on the chemical structure, such as topological distances and the presence/absence of functional groups (Cereto-Massagué et al., 2015). However, some molecular fingerprints use information from pharmacophore models, allowing the comparison of the ligand poses (pharmacophore fingerprints) (Wood et al., 2012). Some molecular fingerprints, such as SMILES fingerprint (SMIfp) (Schwartz et al., 2013), and structural interaction fingerprint (SIFt) (Deng et al., 2004), evaluate structural features related to intermolecular interactions, such as hydrophobic contacts, polar interactions, and hydrogen bond acceptors and donors (interaction fingerprints) (Desaphy et al., 2013). Considering that natural products are chemically complex and structurally different from the synthetic libraries, the analyses of their structures using molecular fingerprints can provide insights, evidencing some structural similarities (see example in Figure 2B) (Gu et al., 2013; Tao et al., 2015; Floros et al., 2016; Galúcio et al., 2019; Chávez-Hernández et al., 2020).
Figure 2. (A) Schematic representation of bits applied in the molecular fingerprints of chemical structures. (B) Fingerprint-based similarity of the natural compound bisdethiobis (methylthio)gliotoxin and the FDA-approved anticancer drug mitomycin (Galúcio et al., 2019). (C) Schematic view of the chemical space network and (D) hierarchical clustering that apply fingerprint-based descriptors to analyze natural compounds.
Molecular fingerprints offer a cost-efficient computational calculation to be implemented with other computational approaches. Molecular fingerprints have been widely applied in the representation of chemical space networks to evaluate the structural similarities of natural products (see example in Figure 2C) (Zhang et al., 2015) as well as in hierarchical clustering methods (Figure 2D) (Sánchez-Cruz and Medina-Franco, 2018). In chemical network representations, the nodes (vertices) represent the analyzed compounds and edges of the pairwise fingerprint similarity relationships calculated by a structural metric. The edge drawn between a pair of nodes uses a satisfying threshold criterion for the structural similarity value (e.g., a cutoff = 0.7) between the analyzed compounds (Maggiora and Bajorath, 2014; Kunimoto and Bajorath, 2018). The investigation of the chemical space of natural products is an intelligent way to identify some classes of compounds, their bioactivity, and the structural scaffolds present in known active compounds (Opassi et al., 2018). Due to the high diversity of the derived structures of natural products containing modified functional groups; different strategies have been applied to investigate their chemical space, which include the modeling of hypothetical structural modification (Skinnider et al., 2017) and the application of less restrictive similarity-based cutoffs (Pavadai et al., 2017).
Recently, machine learning algorithms using MACCS keys and Morgan molecular fingerprints have been used to differentiate natural products from synthetic molecules. The authors also used similarity maps to classify natural product substructures according to their similarity to natural or synthetic compounds (Chen et al., 2019). Galúcio et al. (2019) used fingerprint-based similarity to find correspondences between natural products and FDA-approved anticancer drugs, and the authors identified an interesting correspondence (see Figure 2B) between the bisdethiobis(methylthio)gliotoxin obtained from bacterial strain and the FDA-approved anticancer drug mitomycin.
Several programs and web servers have been developed to compute molecular fingerprints, and among them, we can cite ChemDes (web server) (Dong et al., 2015), ChemoPy (open-source Python package) (Cao et al., 2013), PaDEL (open-source Java program) (Yap, 2011), and jCompoundMapper (open-source Java program) (Hinselmann et al., 2011).
Structural similarity is a key concept in the discovery of new bioactive compounds from natural sources due to the assumption that similar compounds perform similar molecular activities. Different similarity and distance metrics have been applied to compare molecular fingerprints (Bajusz et al., 2015); some of them are available in cheminformatics tools, such as Konstanz Information Miner (KNIME) (Berthold et al., 2009), PyBel (O'Boyle et al., 2008), the Chemistry Development Kit (CDK) (Willighagen et al., 2017), and RDKit (Lovrić et al., 2019). Similarity metrics could use two-dimensional (2D) or 3D similarities of compounds, but studies have demonstrated that the 2D similarity coefficient neglects some important structural/functional features in the identification of the target compound (Gohlke et al., 2015; Kim et al., 2016).
Several similarities and distance metrics have been applied to compare the pairwise similarities of molecules and their substructures (Bajusz et al., 2015; O'Hagan and Kell, 2016; Rácz et al., 2018). Table 2 exhibits the main similarity coefficients and their dichotomous equations applied to compare molecular fingerprints, where a correspond to on bits (presence) in structure A, b is the number of the on bits in structure B, while c corresponds to bits that are on in both molecular structures. Differently from other similarity metrics, Tversky is an asymmetric coefficient that has two user-defined parameters, α and β. If α is set to 1 and β is set to 0, the Tversky coefficient will measure the substructural similarity between two molecules, where a Tversky value equal to 1 indicates that a given structural moiety is a substructure of the compared compound (Senger, 2009).
Tanimoto has been the most used similarity coefficient in fingerprint-based similarity in virtual screening strategies, and its results have been described, in some cases, as equivalent to other similarity metrics applied to compare two molecules, such as Soergel, Dice, and Cosine, while the similarity measures derived from Euclidean and Manhattan distances have been described as unsatisfactory (Bajusz et al., 2015; Rácz et al., 2018). However, the Tversky coefficient has been indicated to compare moieties of natural products or non-symmetrical scaffolds seeking to identify drug-like similarities (O'Hagan and Kell, 2016). Tanimoto and Tversky coefficient values range from 0 to 1, where values close to 1 correspond to a high similarity between the two analyzed molecules and values close to 0 represent a low similarity (Senger, 2009; Bajusz et al., 2015).
A pharmacophore model consists of a set of chemical groups with a specific 3D arrangement that are involved in biological activity against a specific molecular target (Schaller et al., 2020). The functional characteristics present in a pharmacophore model include hydrogen bond acceptors, hydrogen bond donors, hydrophobic groups, positive or negative ionizable groups, and coordination with metal ions (Vuorinen and Schuster, 2015; Schaller et al., 2020). The binding sites of ligands have physicochemical and spatial restrictions that impose limitations to the non-specific interaction of certain molecules, such as the physicochemical properties of the amino acid residue composition, the volume, and the shape of the cavity. These spatial restrictions dictate the binding mode of the ligands, thus allowing different molecules, even with different structures, to act against a specific bioreceptor due to the presence of the same pharmacophore model (Vuorinen and Schuster, 2015).
Pharmacophore modeling has been extensively applied in virtual screening, lead compound optimization strategies, and de novo drug design strategies (Akram et al., 2017; Azminah et al., 2019; Da Costa et al., 2019; El Kerdawy et al., 2019; Jade et al., 2020). Two computational approaches are distinguished in pharmacophore modeling: (1) ligand-based and (2) structure-based approaches. To predict the pharmacophore model, the ligand-based methods use 3D alignment to obtain the chemical information (e.g., shape, functional groups, etc.), shared by a set of active compounds, and select the functional groups that are relevant for the interaction of the ligand with the macromolecular target (Pal et al., 2019). In contrast, the structure-based approach uses the spatial information of the ligand complexed with the molecular target (e.g., ligand poses, conformations, etc.); thus, this approach is applied only in the presence of experimentally elucidated structures of the molecular targets (e.g., by X-ray crystallography) complexed with an active ligand (Jiang et al., 2020).
The ligand-based pharmacophore-based virtual screening comprises different stages: (1) selection of the active compounds validated experimentally; (2) generation of the 3D conformation of the ligands, followed by their structural alignment; (3) identification of the structural characteristics and functional groups involved in molecular recognition; (4) generation and validation of the pharmacophore model using a compound library as a testing dataset; and (5) screening of the natural product library (Figure 3).
Figure 3. An overview of pharmacophore-based virtual screening applied for natural product libraries.
In ligand-based pharmacophore modeling, the pharmacophore model is generated using a 3D alignment of the conformers of a set of bioactive compounds (training dataset). Then, active (true-positive compounds or hits) and inactive compounds (false-positive compounds or decoys) are used as a testing dataset to validate the pharmacophore model (Shahin et al., 2016; Pal et al., 2019). It is important to note that, despite the choice of strict pharmacophore models leading to the selection of compounds with better activities against the molecular target, it also could reduce the structural diversity of the analyzed natural products. In contrast, the choice of less restrictive models could retrieve a larger number of false-positive compounds (Schaller et al., 2020).
Pharmacophore modeling methods could be divided into two scoring function methods to predict the fitness of the analyzed compounds to the predicted pharmacophore models: the root of the mean square deviation (RMSD)-based and the overlay-based scoring function (Sanders et al., 2012). In RMSD-based methods, the distances between the functional groups of the compounds to the center of pharmacophore features are used to assess the fitness of the compounds concerning the predicted pharmacophore model. In contrast, the overlay-based methods use the radii of the functional groups and/or atoms to estimate the functional similarity of the structures with the pharmacophore model (Vuorinen and Schuster, 2015). Pharmacophore-based methods that apply RMSD-based scoring functions are better at predicting the ligand poses than the overlay-based scoring functions (Sanders et al., 2012). Nevertheless, the ratio of correctly predicted poses vs. incorrectly predicted poses is better obtained using overlay-based scoring functions (Sanders et al., 2012). Regarding structure-based pharmacophore modeling, the use of experimental structures to build the models must prioritize some structural features obtained from both methods; as an example, it has been demonstrated that a higher flexibility obtained in structures elucidated by nuclear magnetic resonance (NMR) spectroscopy helps to focus the models on the most essential interactions with the receptor due to the presence of structural flexibility of the complexes evidenced by the method. On the other hand, models obtained by X-ray crystallography had more pharmacophore elements compared to those obtained by NMR spectroscopy (Ghanakota and Carlson, 2017).
Pharmacophoric screening has been applied to screen compounds with cosmetic purposes using essential oils (Santana et al., 2018; Da Costa et al., 2019). Essential oils contain diverse classes of volatile and low-molecular-weight compounds with a broad spectrum of biological activities (Do Nascimento et al., 2020), and due to their reported repellent activities against mosquitos, these compounds have been investigated in virtual screening strategies (Santana et al., 2018; Thireou et al., 2018). Recently, a study performed an in silico analysis of 1,633 compounds from the essential oils of 71 botanical families by combining a structural similarity-based search method (ligand-based virtual screening) with a pharmacophore-based virtual screening (structure-based strategy). The authors used, as a reference, the structure of N,N-diethyl-meta-toluamide (DEET) complexed to the odorant-binding protein of Anopheles gambiae, and they found seven natural volatile compounds with potential repellent activity against mosquitos, such as p-cymen-8-yl, thymol acetate, carvacryl acetate, thymyl isovalerate, and p-anisyl hexanoate (Da Costa et al., 2019).
Currently, different programs generate pharmacophore models, differing in the algorithm applied to evaluate the conformational ligand flexibility as well as to perform the structural alignment. Some commercial programs applied to pharmacophore prediction include LigandScout (Wolber and Langer, 2005) and Molecular Operating Environment (MOE) (Molecular Operating Environment, 2019). Both programs apply ligand- and structure-based pharmacophore modeling and are compatible with the most used operating systems. Some open-source programs that use ligand-based pharmacophore prediction include Pharmer (https://sourceforge.net/projects/pharmer/) (Koes and Camacho, 2011) and Align-it (previously named Pharao; OS compatibility: OS X) (Taminau et al., 2008). Free-access web servers have also been developed to screen compounds using the structure-based pharmacophore approaches, such as Pharmit (http://pharmit.csb.pitt.edu/) (Sunseri and Koes, 2016) and PharmMapper (http://www.lilab-ecust.cn/pharmmapper/) (Liu et al., 2010).
The molecular shape acquired by a ligand is crucial to defining its affinity and selectivity against the protein binding site (Kortagere et al., 2009). Based on this assumption, the 3D shape-similarity search methods assume the premise that two compounds could be recognized by the same bioreceptor and then modulate their activity (Koes and Camacho, 2014; Kumar and Zhang, 2018). Shape-similarity methods can screen vast compound libraries against a reference ligand with known bioactivity (Ai et al., 2014; Koes and Camacho, 2014).
These methods are subdivided into two categories: (1) alignment-free methods that are usually computationally faster because they do not require overlapping the molecules or evaluating properties related to the surface (Seddon et al., 2019) and (2) alignment-based methods that are computationally costly since these methods superimpose molecular shapes and analyze surface properties, such as polarity and hydrophobicity (Fontaine et al., 2007; Kumar and Zhang, 2018). Different methods have been used in the representation of the 3D molecular shape of the ligands, such as Gaussian overlay-based methods (Cai et al., 2013), atomic distance-based methods (Ballester et al., 2009; Ballester, 2011; Bonanno and Ebejer, 2020), and surface-based methods (Karaboga et al., 2013; Cleves et al., 2019). The recognized molecular shapes are transformed into the 3D molecular fingerprints that are then compared using similarities or distance indexes, such as Tanimoto, Dice, and Tversky coefficients (Shin et al., 2015). Due to the complex structure of natural products, the identification of their molecular targets has been challenging even using computational tools; however, the 3D shape-based similarity search methods have emerged as an efficient strategy to predict the macromolecular targets of these compounds (Shin et al., 2015; Chen et al., 2020). Web servers that apply shape-similarity search methods include the SHAFTS (Liu et al., 2011) and USR-VS (Li et al., 2016). Some installable open-source programs include Shape-it (OS compatibility: Linux) (Grant et al., 1996), gWEGA (Yan et al., 2014), and OptiPharm (Puertas-Martín et al., 2019). Some commercial solutions include Shape TK (OS compatibility: Linux/MS-Windows/macOS) (Software O Scientific, 2008).
Shape-based similarity methods have been used in virtual screening workflows alone or combined with different computational techniques (Pavadai et al., 2017; Thireou et al., 2018). Pavadai et al. applied shape-based and fingerprint-based similarity search against natural product libraries to find new steroid-like natural products as antiplasmodial agents using, as a search key, fusidic acid. The hit compounds were filtered based on the predicted partition coefficient, logP, and the authors identified nine new compounds that inhibited parasite growth with IC50 values of <20 μM (Pavadai et al., 2017). Figure 4 exhibits an overview of the 3D shape-similarity search methods applied to identify compounds in chemical libraries with similar molecular shapes despite their different structures.
Figure 4. Applications of alignment-based 3D shape-similarity search methods to identify compounds with similar molecular shapes.
Machine learning (ML) is the computational practice of using intelligent algorithms to learn and make decisions in order to solve problems related to an amount of data. Artificial Intelligence has made important progress toward the acceleration of research and development of novel bioactive natural compounds with industrial applications. This approach has been widely applied in different steps related to the virtual screening strategies, for example to predict some pharmacokinetic properties (Wei et al., 2017; Qiang et al., 2018) [e.g., penetration of compounds into the blood–brain barrier (Zhang et al., 2017; Dai et al., 2021) and cell membrane (Wei et al., 2017; Wolfe et al., 2018)], compounds' side effects (Dimitri and Lió, 2017), their toxicity (Mayr et al., 2016; Pu et al., 2019; Zheng et al., 2020), molecular targets (Wang et al., 2013; Jeon et al., 2014), and their bioactivity (Li and Huang, 2012; Schaduangrat et al., 2019; Shoombuatong et al., 2019) [e.g., anti-tuberculosis (Gomes et al., 2017; Maia S. M. et al., 2020), anticancer (Charoenkwan et al., 2021), and insecticidal activities (Soares Rodrigues et al., 2021)] as well as to identify the pan-assay interference compounds (PAINS), i.e., highly reactive and promiscuous molecules that are often false positives in high-throughput screening assays (Jasial et al., 2018). In some cases, the ML algorithms have been reported with superior efficiency and, thus, are more suitable to predict hit compounds from chemical libraries than are the traditional QSAR methods (Tsou et al., 2020).
ML algorithms are trained using a large number of data that are used as a benchmark to accomplish a particular computational problem (Vamathevan et al., 2019). The main aim of an ML framework in virtual screening strategies is to generalize the results obtained from the training dataset to better evaluate the test dataset and, then, make the decision (Sieg et al., 2019; Vamathevan et al., 2019). ML algorithms applied in the LBVS approach aim to predict the bioactivity or pharmacodynamic/pharmacokinetic properties of molecules based on their similarity to known actives. Therefore, to evaluate the similarity of the molecules, these algorithms use, as datasets, molecular descriptors calculated from the compound structures (Li and Huang, 2012; Challa et al., 2020) using different molecular modeling and cheminformatics toolkits, such as RDKit (Lovrić et al., 2019) and CDK (Willighagen et al., 2017). Some chemo-structural and bioactivity information deposited in public databases, as well as experimental results, have also been used to train these algorithms (Martínez-Treviño et al., 2020). Molecular descriptors applied to evaluate the similarity of molecules include the physicochemical [cLogP, topological polar surface area (tPSA), molecular weight, etc.] and structural properties (rotatable bonds, aromatic rings, etc.) (Lo et al., 2018), molecular fingerprints (Zhang et al., 2018), functional groups, molecular shape (Bonanno and Ebejer, 2020), and pharmacophores (Sato et al., 2010); in the case of proteins and peptides, some molecular descriptors include amino acid sequence composition (Wei et al., 2017; Manavalan et al., 2018; Qiang et al., 2018). The choice of the molecular representation and the type of molecular descriptor determine the efficiency and the interpretability of the final results obtained by the ML algorithms (David et al., 2020; Jiménez-Luna et al., 2020). In structure-based strategies, ML algorithms have been used in scoring the functions of molecular docking methods, seeking rank compound libraries based on their predicted affinity against a molecular target, and discriminating between hits and decoy compounds. To reach these results, the ML algorithms are trained using the binding affinities of active molecules against protein targets (Wójcikowski et al., 2017; Li et al., 2020). Different open-source programs have been applied to develop machine learning models [e.g., scikit-learn (Pedregosa et al., 2011) and SciPy (Virtanen et al., 2020), both Python modules] and pipelines [e.g., KNIME (Berthold et al., 2009), a data analytics platform].
ML algorithms are classified into supervised and unsupervised learning (Figure 5). Supervised ML algorithms require a retrospective validation using a dataset of active and inactive compounds to better select the methods that are suitable to differentiate the bioactive molecules (Sieg et al., 2019). Supervised learning techniques are divided into two subgroups: (1) regression analysis and (2) classifier methods. The first one includes decision trees, artificial neural networks, support vector machines, and random forest methods. In contrast, the unsupervised algorithms recognize patterns in the dataset of compounds without the presence of inactive ones, thus trying to organize the data in a logical form. These methods have been used for exploratory analyses using clustering data (Patel et al., 2020). Unsupervised algorithms include clustering methods, such as the hidden Markov model, hierarchical clustering, and k-means (Vamathevan et al., 2019).
Figure 5. Classification of supervised and unsupervised learning techniques applied in virtual screening strategies.
Supervised ML algorithms have been widely applied to discover new bioactive natural products (Bilsland et al., 2015; Galúcio et al., 2019; Grisoni et al., 2019; Schaduangrat et al., 2019). Figure 6 exhibits a general overview of the computational steps involved in obtaining a validated supervised ML algorithm to predict the bioactivity of natural products. The first step to modeling a machine learning algorithm involves the preparation of a molecule dataset, i.e., obtaining the molecular structures/sequences that will be used in the algorithm using online databases, literature, or experimental data. This step also includes the correction of possible stereochemical and valence errors present in the molecular structures as well as the correction and conversions of the files to readable formats recognized by the cheminformatics programs. Then, the molecular properties are calculated using molecular modeling and cheminformatics toolboxes, extracted from online databases, or obtained from experimental results, then these descriptors are evaluated to compose the features of the ML model. Currently, different online databases have been developed with information regarding the structural and physicochemical properties of the molecular structure of natural products that could be used in the feature composition (Dunkel et al., 2006; Pilon et al., 2017; Pilón-Jiménez et al., 2019; Sorokina and Steinbeck, 2019). In this step, some statistical methods are applied to select the features, such as Kendall correlation, analysis of variance (ANOVA), and Spearman's test. Finally, the ML model is evaluated regarding its performance to discriminate the true and positive bioactive compounds. Several metrics have been applied to evaluate these models, such as the receiver operating characteristic (ROC) curve, enrichment factors, and mean squared error (R2) applied for linear regression methods. We do not intend to extend the discussion about the application and the choice of the most adequate method to select the feature composition or to evaluate ML models; thus, we recommend the readers to consult previous reviews (Hossin and Sulaiman, 2015; Rácz et al., 2019). In the present sessions, we will discuss the functioning of some ML algorithms most applied in virtual screening strategies focusing on the k-nearest neighbor, decision tree, random forest, artificial, and neural network.
Figure 6. A general overview of the computational steps involved in obtaining a validated supervised machine learning algorithm.
Decision tree algorithms are a supervised learning technique and their construction model is based on two steps: (1) selection of the features and (2) the building of the decision trees. This method is commonly represented by a tree, where the internal nodes represent the selected features (molecular descriptors), the branches represent the testing results of the molecule (decision criteria), and the leaf nodes represent the molecules (molecular structure) (Figure 7A). Compounds are classified based on the leaf nodes that are reached through a series of algorithm decisions (branches). Decision tree (DT) models are constructed focusing on the selection of the best test conditions to expand the extremities of the tree. Some test metrics, such as the information–gain ratio and entropy, are applied to select the best test classification for the algorithm (Lavecchia, 2015). Decision trees have been applied in different virtual screenings of natural products to predict their bioactivity and drug-likeness (Pereira et al., 2015; Wang et al., 2019). Random forest is an ensemble learning technique considered an improvement of the decision tree algorithms to correct the overfitting in the training set (Svetnik et al., 2003). Random forest algorithms generate a model composed of several randomly sampled decision trees from the original dataset obtaining its random features. Random forest models have been applied in virtual screening pipelines to predict compound drug-likeness, bioactivity (Svetnik et al., 2003; Zoffmann et al., 2019), and the pharmacokinetic profile (Dong et al., 2018).
Figure 7. Schematic overview of some of the machine learning algorithms applied in virtual screening. (A) Two-dimensional (2D) diagram of a single root tree of a decision tree algorithm and the general architecture of a random forest. (B) The architecture of a multilayer feed-forward and recursive artificial neural network. Zw refers to neurons of the hidden layers (internal); Zk and Zt, to the neurons of the input and output layers, respectively. (C) k-Nearest neighbor algorithm showing the learning technique to classify a new data represented by the 2D yellow point, which is classified as belonging to class A (gray triangles).
Artificial neural networks are the most studied learning techniques with widely diverse applications in the investigation of a compound's bioactivity (Lata et al., 2007; Liu et al., 2019, 2020; Stokes et al., 2020). Methods that apply neural networks mimic brain functioning and structure, building a model that reaches a decision based on previous experiences obtained from the training dataset (Jing et al., 2018). The architecture of an artificial neural network model comprises several units, named neurons which are connected to form a network arranged in different layers. Depending upon their position in the network, these layers are classified as output layers, input layers (external), and hidden layers (internal) (Zhang R. et al., 2020). A multilayer feed-forward neural network contains neurons connected only to those located in the following layers (Figure 7B), and this class is included in radial basis function networks, multilayer perceptrons, and self-organizing maps (Kohonen maps) (Lavecchia, 2015). In contrast, the recurrent neural networks contain feedbacks between the layers, i.e., interconnections between neurons from the same and consecutive layers; thus, their outputs are determined by the previous outputs and the current inputs (Figure 7B), which form a “memory” during the learning process.
The k-nearest neighbor is instance-based learning and is one of the simplest and intuitive ML algorithms applied to classify and rank compounds based on the nearest training examples present in the chemical space (analyzed feature composition) (Kauffman and Jurs, 2001; Medina-Franco et al., 2005). The algorithm compares the molecular descriptors of the query molecule with k-neighbors that have the smallest distance (k-value), where the k-value corresponds to the number of closest neighbors (a positive integer) and classifies them by majority votes of their closest neighbors (Figure 7C). The number of neighbors is the most important parameter for the model, deciding its complexity. k-nearest neighbor is a classifier algorithm; thus, irrelevant features can lead to disturbances in the compound classification. It is indicated to first preprocess the molecular descriptors to remove the irrelevant or the most correlated ones.
Despite the majority of the computational screening approaches using ML algorithms lacking experimental validations, we have some interesting successful studies that aimed to find and characterize novel natural products with experimentally validated biological activity (Rupp et al., 2010; Zhang et al., 2017; Nocedo-Mena et al., 2019; Patsilinakos et al., 2019; Lee et al., 2020; Liu et al., 2020). Recently, Reher et al. reported on the SMART 2.0, an NMR-based machine learning tool designed for the discovery and characterization of natural products. The tool was successfully applied to investigate the environmental extract of Symploca sp., a filamentous marine cyanobacterium, leading to the isolation and identification of a new chimeric macrolide named symplocolide A. The molecular structure of this novel natural product was confirmed by 1D/2D NMR and tandem liquid chromatography mass spectrometry (LC-MS2) analysis (Reher et al., 2020). Similarly, Lee et al. applied SMART 2.0 to prioritize the isolation and characterization of sesquiterpene lactones from the Eupatorium fortune plant. The isolated natural compounds were experimentally tested against five cancer cell lines and exhibited cytotoxic activities (Lee et al., 2020).
ML algorithms have been successfully applied to predict the bioactivity of compounds. Recently, Nocedo-Mena et al. (2019) combined machine learning, perturbation theory, and information fusion techniques to investigate the antibacterial activity of terpenes from the Cissus incisa plant, and the authors found that phytol and α-amyrin showed minimum inhibitory concentrations equal to 100 μg/ml against the carbapenem-resistant Acinetobacter baumannii and the vancomycin-resistant Enterococcus faecium. In another study, Liu et al. applied deep learning algorithms to find natural products with anti-osteoporosis activity. The selected hits successfully suppressed the osteoclastogenesis-related genes Rank, Tracp, Ctsk, and Nfatc1 in vitro (Liu et al., 2020). Some studies have also reported experimental validations of ML models to predict pharmacokinetic properties. Zhang et al. used a hybrid ML algorithm using support vector machine, probabilistic neural network, naive Bayes classifier, and random forest models combined with in vitro assays to predict the blood–brain barrier penetration of natural compounds from the Traditional Chinese Medicine database (TCMDB). The authors found an overall accuracy for experimental validation around 81% (Zhang et al., 2017).
Virtual screening approaches have been predictive, useful, and cost-effective in identifying novel bioactive compounds when compared with the traditional methods applied solely. However, despite their well-known success, these methods have limitations and their models are prone to biases (Sieg et al., 2019; Slater and Kontoyianni, 2019). It has been demonstrated that the presence of stereochemical and valence errors in the chemical data libraries could also induce investigators to choose unfeasible compounds (Williams and Ekins, 2011; Williams et al., 2012).
Biases, in essence, correspond to distortions from the true underlying relationship between the investigated objects. The investigation of the chemo-structural diversity of natural products and their bioactivity using similarity-based search methods is biased because it considers an assumption that the discovery of novel active compounds must consider the similarity of known active ones (Sieg et al., 2019). This assumption is susceptible to drive the decision-making process to erroneous directions and can reduce the structural diversity of new chemo-structures. Combining low time-consuming computational simulations and more realistic results also remains a challenge for some 3D similarity-based search algorithms, which, in general, require superimposing many conformation pairs of compounds from large chemical libraries, thus requiring high-performance computing (Yan et al., 2016).
Despite the chemical space being considered infinite, the pharmacological space of bioactive compounds of the “druggable human genome” is limited, and its exploration remains a difficult task even from a computational point of view (Opassi et al., 2018). This assumption has been proven to be true for other classes of bioactive compounds with industrial applications, such as pesticides and herbicides (Avram et al., 2014). Therefore, the exclusion of some compounds during the filtering process is comprehensive, but can also reduce the investigation of new chemical entities with specific bioactivity.
In pharmacophore-based virtual screening, the selection of inappropriate models, or very restricted ones, could eliminate an interesting structural diversity of natural compounds. However, the choice of less restrictive models could retrieve a larger number of false-positive compounds (Lans et al., 2020; Schaller et al., 2020). Based on these biases, a balanced choice between strict and loose criteria to select the pharmacophore model to filter natural products could be decided by prioritizing pharmacophore moieties better associated with a higher compound activity; thus, the information obtained from structure–activity analyses might be useful to decide on the most appropriate pharmacophore model to screen natural products (Qing et al., 2014). Regarding the limitation of ligand-based pharmacophore modeling methods, it has been reported that their dependence on structurally similar compounds reduces their application since compounds with high structural dissimilarities may not share the same binding mode (Schaller et al., 2020). Furthermore, few ligand-based methods consider the conformational flexibility of the macromolecular receptor in the determination of the pharmacophore model (Lans et al., 2020). In molecular docking, for example, the elimination of compounds with poor fitness could be biased due to the choice of wrong or inappropriate scoring functions, i.e., those that contain chemical information that contradicts the physical reality or that were not calibrated for the class of investigated molecules (Luo et al., 2017).
Supervised machine learning algorithms are also prone to biases, which can lead to a misleading interpretation of the final results obtained for chemical data libraries. It has been demonstrated that highly correlated training and testing datasets, i.e., containing chemical data too closely similar (e.g., same molecular scaffold with a high frequency between the datasets), could limit the applicability of the machine learning model, reaching false accuracies in its predictiveness (Wallach and Heifets, 2018; Sieg et al., 2019). Therefore, low training errors are insufficient to justify the choice of a machine learning model since the satisfactory predictive performance could be due to redundancy between the training and testing datasets rather than accuracy (Wallach and Heifets, 2018). It has also been demonstrated that some biased machine learning models could be obtained using a training dataset composed of active molecules that are easily differentiated from inactive ones by coarse properties, such as cLogP, the number of HBA, and molecular weight (Ripphausen et al., 2011). Based on these biases of machine learning models, it is necessary to investigate whether chemical data benchmarks contain design flaws that might lead to optimistic performances that are distorted from the chemical reality. Some computational methods have been developed to avoid overfitting in chemical datasets. Wallach and Heifets (2018) developed the asymmetric validation embedding (AVE) bias using Python language to predict the performance across common benchmarks and standard machine learning algorithms, and Ripphausen et al. (2011) developed a public compound database, named REPROVIS-DB, that contains information from successful ligand-based virtual screening strategies including experimentally confirmed hits, reference compounds, screening databases, and selection criteria.
The development of computational approaches for virtual screening has been incentivized by the presence of numerous biological and chemo-structural information of natural products deposited in public databases (Valli et al., 2013; Harvey et al., 2015; Pilon et al., 2017), as well as by the advances of computer processing and storage capacity (Walters, 2019). High scientific efforts to isolate and characterize natural products have increased the interest of the academia and industry to comprehensively organize this information using public databases to better explore these natural sources and also to contribute to our knowledge regarding their ethnobotanical information, biological activities, chemical structures, natural origin, and physicochemical properties. Herein, we do not intend to provide exhaustive information regarding these online databases with public access, but we will exhibit those with potential applications in virtual screening strategies of natural products.
NuBBEDB (https://nubbe.iq.unesp.br/portal/nubbe-search.html) provides information regarding chemo-structures obtained from Brazilian biodiversity (Valli et al., 2013). Currently, the database contains more than 2,200 structures of natural compounds obtained from different Brazilian biomes (Pilon et al., 2017). NuBBEDB contains the 3D structures of natural products in an MOL2 file format, which is compatible with the most widely used molecular modeling and cheminformatics programs.
The Comprehensive Marine Natural Products Database (CMNPD) (https://www.cmnpd.org/) is a comprehensive and curated marine natural products database that contains more than 32,000 structures (accessed on January 06, 2020) with different physicochemical and pharmacokinetic properties. Besides, it includes information regarding their biological activity, natural origin, and the geographical distribution of source organisms (Lyu et al., 2020). The database also contains the complete molecule datasets freely available for download (https://docs.cmnpd.org/downloads).
The natural product-likeness software suite NaPLeS (https://naples.naturalproducts.net/) is an MySQL database of natural products and an open-source web application that computes the natural product-likeness scores of large chemical libraries. Currently, the database contains 315,916 natural products from various public databases (Sorokina and Steinbeck, 2019).
The Universal Natural Product Database (UNaProd) (http://jafarilab.com/unaprod/index.php) is an online and public database of natural products used in Iranian traditional medicine. The database currently contains 2,696 compounds of botanical, animal, and mineral origins (accessed on January 06, 2020) (Naghizadeh et al., 2020).
The Natural Product Activity and Species Source Database (NPASS) (http://bidd.group/NPASS/index.php) provides biological activity results and information regarding the origin species of more than 35,032 natural products (accessed on January 06, 2020) (Zeng et al., 2018). The database also contains a structural compound library freely available for download in SDF and SMILES formats (http://bidd.group/NPASS/downloadnpass.html).
BIOFACQUIM (https://biofacquim.herokuapp.com/) is a free and public database of natural products isolated and characterized from Mexican biodiversity. Compounds from this database are also available in the ZINC database (Pilón-Jiménez et al., 2019). Currently, the database contains 423 natural compounds (accessed on January 08, 2020) which are identified by their respective names, accession codes, source organisms, in SMILE format, and references.
The Natural Products Atlas (https://www.npatlas.org/joomla/) is an open-access database of microbial natural products that contain 24,594 compound structures (accessed on January 07, 2020) and information related to their structure, IUPAC name, source organisms, and literature (van Santen et al., 2019). The database also contains information of other natural product databases, such as the Minimum Information about a Biosynthetic Gene Cluster (MIBiG) repository and the Global Natural Products Social Molecular Networking (GNPS) platform (van Santen et al., 2019).
The African Natural Products database (ANPDB) is a free database of natural products from different regions of the African continent (available at ANPDB|ANPDB (African-compounds.org) and contains ~4,500 structures (accessed on January 12, 2020). The available data content comprises sources covering the period from 1962 to 2019 (Ntie-Kang et al., 2017). The database also contains the 3D structures of natural products in SMILES and SDF formats available for non-commercial uses.
The Natural Products for Cancer Regulation (NPCARE) is a free online database (http://silver.sejong.ac.kr/npcare/) that provides more than 6,000 natural products and more than 2,000 extracts isolated from 1,952 different species including microorganisms, marine organisms, and plants, as well as information related to the action of these extracts and isolated natural compounds against the gene expression levels and cancer cell line inhibition (Choi et al., 2017). The database is an interesting source to discover potential anticancer compounds and to understand the anticancer molecular mechanisms underlying natural products.
StreptomeDB (http://www.pharmbioinf.uni-freiburg.de/streptomedb) is a free and online database used to explore natural products isolated or mutasynthesized from streptomycetes using an interactive phylogenetic analysis (Lucas et al., 2013; Moumbock et al., 2021). StreptomeDB 3.0 provides more than 6,500 natural products obtained from ~3,300 Streptomyces strains (Moumbock et al., 2021). These metabolites show interesting biological activities, such as antimicrobial, anticancer, and immunosuppressant properties. The compound structures are identified by their respective source organisms, references, biological role, and the routes of biosynthesis.
Natural products offer an interesting structural scaffold, helping to find new chemical entities with several industrial applications, thus offering innovative solutions to solve old worldwide problems, such as bacterial resistance against antibiotics (Smith et al., 2018; Newman and Cragg, 2020). However, the complex and highly diverse structure and the peculiar chemical space occupied by natural products have imposed pharmacokinetic and pharmacodynamic limitations, thus restricting their use for specific purposes by the pharmaceutical and cosmetic industries.
Several computational methods applied in virtual screening strategies have been developed over the years, thus increasing the rational explorations of natural sources aiming at the identification of specific bioactive compounds from large chemo-structural libraries. These computational strategies have also opened up new opportunities to discover new industrial applications of natural compounds justifying the financial and time efforts for their exploration. Natural products present a high structural diversity when compared with their synthetic counterparts, and their difference is, in part, due to the existing intricate biosynthetic pathways in living organisms that produce derived structures, containing modified functional groups, such as glycosylation and methylation. Based on these, the virtual screening strategies must investigate the chemical space of natural products, seeking to identify some classes of compounds with bioactivity or structural scaffolds present in known active molecules. Some of these screening strategies include applying less restrictive structural-based similarity cutoffs (Pavadai et al., 2017) and the modeling of hypothetically derived natural product structures (Skinnider et al., 2017). Regarding the application of molecular filters, some “bioactivity-likeness” criteria must be used with caution to avoid misleading screening or remotion of the important structural diversity of the compound libraries since the structural complexity of natural products situates them beyond the acceptable limits of some empirical rules determined by these filters.
Artificial intelligence algorithms employed in ligand-based approaches have demonstrated high success rates in finding interesting compounds with reduced computational time, and their combined uses with cheminformatics and molecular modeling methods have increased the efficiency of virtual screening strategies, allowing us to explore the highly diverse chemo-structural landscapes of natural products.
Here, we hope to encourage the use of these computational tools by experimental groups, helping researchers to familiarize themselves with their concepts and capabilities as well as alert them of some of the common biases faced by investigators during the investigation of natural sources using computational tools, citing some possible solutions. Finally, we indicate that the automatic process represented by virtual screening must be oriented by human expert decision to avoid misinterpretation or false findings, and also to select compounds based on their desirable features, such as commercial availability, low cost, and synthetic feasibility.
KS, RB, and JL: conceptualization. KS, LN, AL, and VD: investigation. KS, LN, VD, and RB: writing—original draft preparation. KS, AL, CN, RB, and JL: writing—review and editing. CN, RB, and JL: supervision. All authors have read and agreed to the published version of the manuscript.
We would like to thank the Conselho Nacional de Desenvolvimento Científico e Tecnológico (CNPq) and Pró-Reitoria de Pesquisa e Pós-Graduação (PROPESP/UFPA) for providing the financial support for the scientific research. KS was also grateful for the scholarship from the Brazilian funding agency Coordenação de Aperfeiçoamento de Pessoal de Nível Superior (CAPES, grant number: 88882.466102/2019-01). The funders had no role in the design of the study; in the collection, analyses, or interpretation of data; in the writing of the manuscript, or in the decision to publish the results.
RB was employed by company InsilicAll Ltda.
The remaining authors declare that the research was conducted in the absence of any commercial or financial relationships that could be construed as a potential conflict of interest.
Abdelhameed, R. F. A., Habib, E. S., Eltahawy, N. A., Hassanean, H. A., Ibrahim, A. K., Mohammed, A. F., et al. (2020). New cytotoxic natural products from the red sea sponge stylissa carteri. Mar Drugs 18:241. doi: 10.3390/md18050241
Ai, N., Welsh, W. J., Santhanam, U., Hu, H., and Lyga, J. (2014). Novel virtual screening approach for the discovery of human tyrosinase inhibitors. PLoS ONE 9:e112788. doi: 10.1371/journal.pone.0112788
Akram, M., Waratchareeyakul, W., Haupenthal, J., Hartmann, R. W., and Schuster, D. (2017). Pharmacophore modeling and in silico/in vitro screening for human cytochrome P450 11B1 and cytochrome P450 11B2 inhibitors. Front. Chem. 5:104. doi: 10.3389/fchem.2017.00104
Al Sharie, A. H., El-Elimat, T., Al Zu'bi YO Aleshawi, A. J., and Medina-Franco, J. L. (2020). Chemical space and diversity of seaweed metabolite database (SWMD): a cheminformatics study. J. Mol. Graph. Model. 100:107702. doi: 10.1016/j.jmgm.2020.107702
Atanasov, A. G., Zotchev, S. B., Dirsch, V. M., and Supuran, C. T. (2021). Natural products in drug discovery: advances and opportunities. Nat. Rev. Drug. Discov. 20, 200–216. doi: 10.1038/s41573-020-00114-z
Avram, S., Funar-Timofei, S., Borota, A., Chennamaneni, S. R., Manchala, A. K., and Muresan, S. (2014). Quantitative estimation of pesticide-likeness for agrochemical discovery. J. Cheminform. 6:42. doi: 10.1186/s13321-014-0042-6
Azminah, A., Erlina, L., Radji, M., Mun'im, A, Syahdi, R. R., and Yanuar, A. (2019). In silico and in vitro identification of candidate SIRT1 activators from Indonesian medicinal plants compounds database. Comput. Biol. Chem. 83:107096. doi: 10.1016/j.compbiolchem.2019.107096
Bajusz, D., Rácz, A., and Héberger, K. (2015). Why is Tanimoto index an appropriate choice for fingerprint-based similarity calculations? J. Cheminform. 7:20. doi: 10.1186/s13321-015-0069-3
Ballester, P. J. (2011). Ultrafast shape recognition: method and applications. Future Med. Chem. 3, 65–78. doi: 10.4155/fmc.10.280
Ballester, P. J., Finn, P. W., and Richards, W. G. (2009). Ultrafast shape recognition: evaluating a new ligand-based virtual screening technology. J. Mol. Graph. Model. 27, 836–45. doi: 10.1016/j.jmgm.2009.01.001
Batool, M., Ahmad, B., and Choi, S. (2019). A structure-based drug discovery paradigm. Int. J. Mol. Sci. 20:2783. doi: 10.3390/ijms20112783
Berenger, F., Vu, O., and Meiler, J. (2017). Consensus queries in ligand-based virtual screening experiments. J. Cheminform. 9:60. doi: 10.1186/s13321-017-0248-5
Berthold, M. R., Cebron, N., Dill, F., Gabriel, T. R., Kötter, T., Meinl, T., et al. (2009). KNIME–the Konstanz information miner. ACM SIGKDD Explor. Newsl. 11, 26–31. doi: 10.1145/1656274.1656280
Bilsland, A. E., Pugliese, A., Liu, Y., Revie, J., Burns, S., McCormick, C., et al. (2015). Identification of a selective G1-phase benzimidazolone inhibitor by a senescence-targeted virtual screen using artificial neural networks. Neoplasia (United States) 17, 704–715. doi: 10.1016/j.neo.2015.08.009
Blanco, J. L., Porto-Pazos, A. B., Pazos, A., and Fernandez-Lozano, C. (2018). Prediction of high anti-angiogenic activity peptides in silico using a generalized linear model and feature selection. Sci. Rep. 8:15688. doi: 10.1038/s41598-018-33911-z
Bonanno, E., and Ebejer, J. P. (2020). Applying machine learning to ultrafast shape recognition in ligand-based virtual screening. Front. Pharmacol. 10:1675. doi: 10.3389/fphar.2019.01675
Bradley, S. A., Zhang, J., and Jensen, M. K. (2020). Deploying microbial synthesis for halogenating and diversifying medicinal alkaloid scaffolds. Front. Bioeng. Biotechnol. 8:594126. doi: 10.3389/fbioe.2020.594126
Bruns, R. F., and Watson, I. A. (2012). Rules for identifying potentially reactive or promiscuous compounds. J. Med. Chem. 55, 9763–72. doi: 10.1021/jm301008n
Cai, C., Gong, J., Liu, X., Gao, D., and Li, H. (2013). SimG: an alignment based method for evaluating the similarity of small molecules and binding sites. J. Chem. Inf. Model. 53, 2103–2115. doi: 10.1021/ci400139j
Cai, C., Wu, Q., Hong, H., He, L., Liu, Z., Gu, Y., et al. (2021). In silico identification of natural products from Traditional Chinese Medicine for cancer immunotherapy. Sci. Rep. 11:3332. doi: 10.1038/s41598-021-82857-2
Cao, D. S., Xu, Q. S., Hu, Q. N., and Liang, Y. Z. (2013). ChemoPy: freely available python package for computational biology and chemoinformatics. Bioinformatics 29, 1092–1094. doi: 10.1093/bioinformatics/btt105
Cereto-Massagué, A., Ojeda, M. J., Valls, C., Mulero, M., Garcia-Vallvé, S., and Pujadas, G. (2015). Molecular fingerprint similarity search in virtual screening. Methods 71, 58–63. doi: 10.1016/j.ymeth.2014.08.005
Challa, A. P., Beam, A. L., Shen, M., Peryea, T., Lavieri, R. R., Lippmann, E. S., et al. (2020). Machine learning on drug-specific data to predict small molecule teratogenicity. Reprod. Toxicol. 95, 148–58. doi: 10.1016/j.reprotox.2020.05.004
Charoenkwan, P., Chiangjong, W., Lee, V. S., Nantasenamat, C., Hasan, M. M., and Shoombuatong, W. (2021). Improved prediction and characterization of anticancer activities of peptides using a novel flexible scoring card method. Sci. Rep. 11:3017. doi: 10.1038/s41598-021-82513-9
Chávez-Hernández, A. L., Sánchez-Cruz, N., and Medina-Franco, J. L. (2020). Fragment library of natural products and compound databases for drug discovery. Biomolecules 10:1518. doi: 10.3390/biom10111518
Chen, Y., Garcia de Lomana, M., Friedrich, N. O., and Kirchmair, J. (2018). Characterization of the chemical space of known and readily obtainable natural products. J. Chem. Inf. Model. 58, 1518–1532. doi: 10.1021/acs.jcim.8b00302
Chen, Y., and Kirchmair, J. (2020). Cheminformatics in natural product-based drug discovery. Mol. Inform. 39:2000171. doi: 10.1002/minf.202000171
Chen, Y., Mathai, N., and Kirchmair, J. (2020). Scope of 3D shape-based approaches in predicting the macromolecular targets of structurally complex small molecules including natural products and macrocyclic ligands. J. Chem. Inf. Model. 60, 2858–75. doi: 10.1021/acs.jcim.0c00161
Chen, Y., Stork, C., Hirte, S., and Kirchmair, J. (2019). NP-scout: machine learning approach for the quantification and visualization of the natural product-likeness of small molecules. Biomolecules 9:43. doi: 10.3390/biom9020043
Choi, H., Cho, S. Y., Pak, H. J., Kim, Y., Choi, J. Y., Lee, Y. J., et al. (2017). NPCARE: database of natural products and fractional extracts for cancer regulation. J. Cheminform. 9:2. doi: 10.1186/s13321-016-0188-5
Cleves, A. E., Johnson, S. R., and Jain, A. N. (2019). Electrostatic-field and surface-shape similarity for virtual screening and pose prediction. J. Comput. Aided Mol. Des. 33, 865–86. doi: 10.1007/s10822-019-00236-6
Coimbra, J. R. M., Baptista, S. J., Dinis, T. C. P., Silva, M. M. C., Moreira, P. I., Santos, A. E., et al. (2020). Combining virtual screening protocol and in vitro evaluation towards the discovery of BACE1 inhibitors. Biomolecules 10:535. doi: 10.3390/biom10040535
Congreve, M., Carr, R., Murray, C., and Jhoti, H. (2003). A ‘Rule of Three' for fragment-based lead discovery? Drug Discov. Today 8, 876–877. doi: 10.1016/S1359-6446(03)02831-9
Cubillos, F. A., Gibson, B., Grijalva-Vallejos, N., Krogerus, K., and Nikulin, J. (2019). Bioprospecting for brewers: exploiting natural diversity for naturally diverse beers. Yeast 36, 383–398. doi: 10.1002/yea.3380
Da Costa, K. S., Galúcio, J. M., Da Costa, C. H. S., Santana, A. R., Dos Santos Carvalho, V., Do Nascimento, L. D., et al. (2019). Exploring the potentiality of natural products from essential oils as inhibitors of odorant-binding proteins: a structure- and ligand-based virtual screening approach to find novel mosquito repellents. ACS Omega 4, 22475–22486. doi: 10.1021/acsomega.9b03157
Dai, R., Zhang, W., Tang, W., Wynendaele, E., Zhu, Q., Bin, Y., et al. (2021). BBPpred: sequence-based prediction of blood-brain barrier peptides with feature representation learning and logistic regression. J. Chem. Inf. Model. 61, 525–34. doi: 10.1021/acs.jcim.0c01115
Daina, A., and Zoete, V. (2016). A BOILED-egg to predict gastrointestinal absorption and brain penetration of small molecules. ChemMedChem 11, 1117–1121. doi: 10.1002/cmdc.201600182
David, L., Thakkar, A., Mercado, R., and Engkvist, O. (2020). Molecular representations in AI-driven drug discovery: a review and practical guide. J. Cheminform. 12:56. doi: 10.1186/s13321-020-00460-5
Davison, E. K., and Brimble, M. A. (2019). Natural product derived privileged scaffolds in drug discovery. Curr. Opin. Chem. Biol. 52, 1–8. doi: 10.1016/j.cbpa.2018.12.007
Deng, Z., Chuaqui, C., and Singh, J. (2004). Structural interaction fingerprint (SIFt): a novel method for analyzing three-dimensional protein–ligand binding interactions. J. Med. Chem. 47, 337–344. doi: 10.1021/jm030331x
Desaphy, J., Raimbaud, E., Ducrot, P., and Rognan, D. (2013). Encoding protein–ligand interaction patterns in fingerprints and graphs. J. Chem. Inf. Model. 53, 623–637. doi: 10.1021/ci300566n
Dimitri, G. M., and Lió, P. (2017). DrugClust: a machine learning approach for drugs side effects prediction. Comput. Biol. Chem. 68, 204–210. doi: 10.1016/j.compbiolchem.2017.03.008
Do Nascimento, L. D., de Moraes, A. A. B., da Costa, K. S., Galúcio, J. M. P., Taube, P. S., Costa, C. M. L., et al. (2020). Bioactive natural compounds and antioxidant activity of essential oils from spice plants: new findings and potential applications. Biomolecules 10, 1–37. doi: 10.3390/biom10070988
Doak, B. C., Over, B., Giordanetto, F., and Kihlberg, J. (2014). Oral druggable space beyond the rule of 5: insights from drugs and clinical candidates. Chem. Biol. 21, 1115–1142. doi: 10.1016/j.chembiol.2014.08.013
Dong, J., Cao, D. S., Miao, H. Y., Liu, S., Deng, B. C., Yun, Y. H., et al. (2015). ChemDes: an integrated web-based platform for molecular descriptor and fingerprint computation. J. Cheminform. 7:60. doi: 10.1186/s13321-015-0109-z
Dong, J., Wang, N. N., Yao, Z. J., Zhang, L., Cheng, Y., Ouyang, D., et al. (2018). Admetlab: a platform for systematic ADMET evaluation based on a comprehensively collected ADMET database. J. Cheminform. 10:29. doi: 10.1186/s13321-018-0283-x
Dunkel, M., Fullbeck, M., Neumann, S., and Preissner, R. (2006). SuperNatural: a searchable database of available natural compounds. Nucleic Acids Res. 34, D678–D683. doi: 10.1093/nar/gkj132
El Kerdawy, A. M., Osman, A. A., and Zaater, M. A. (2019). Receptor-based pharmacophore modeling, virtual screening, and molecular docking studies for the discovery of novel GSK-3β inhibitors. J. Mol. Model. 25:171. doi: 10.1007/s00894-019-4032-5
Ertl, P., Roggo, S., and Schuffenhauer, A. (2008). Natural product-likeness score and its application for prioritization of compound libraries. J. Chem. Inf. Model. 48, 68–74. doi: 10.1021/ci700286x
Feher, M., and Schmidt, J. M. (2003). Property distributions: differences between drugs, natural products, and molecules from combinatorial chemistry. J. Chem. Inf. Comput. Sci. 43, 218–227. doi: 10.1021/ci0200467
Floros, D. J., Jensen, P. R., Dorrestein, P. C., and Koyama, N. (2016). A metabolomics guided exploration of marine natural product chemical space. Metabolomics 12:145. doi: 10.1007/s11306-016-1087-5
Fontaine, F., Bolton, E., Borodina, Y., and Bryant, S. H. (2007). Fast 3D shape screening of large chemical databases through alignment-recycling. Chem. Cent. J. 1:12. doi: 10.1186/1752-153X-1-12
Galúcio, J. M., Monteiro, E. F., de Jesus, D. A., Costa, C. H., Siqueira, R. C., Santos dos, G. B., et al. (2019). In silico identification of natural products with anticancer activity using a chemo-structural database of Brazilian biodiversity. Comput. Biol. Chem. 83:107102. doi: 10.1016/j.compbiolchem.2019.107102
Garcia-Hernandez, C., Fernández, A., and Serratosa, F. (2019). Ligand-based virtual screening using graph edit distance as molecular similarity measure. J. Chem. Inf. Model. 59, 1410–21. doi: 10.1021/acs.jcim.8b00820
Gfeller, D., Grosdidier, A., Wirth, M., Daina, A., Michielin, O., and Zoete, V. (2014). SwissTargetPrediction: a web server for target prediction of bioactive small molecules. Nucleic Acids Res. 42, W32–W38. doi: 10.1093/nar/gku293
Ghanakota, P., and Carlson, H. A. (2017). Comparing pharmacophore models derived from crystallography and NMR ensembles. J. Comput. Aided Mol. Des. 31, 979–993. doi: 10.1007/s10822-017-0077-7
Ghose, A. K., Viswanadhan, V. N., and Wendoloski, J. J. (1999). A knowledge-based approach in designing combinatorial or medicinal chemistry libraries for drug discovery. 1. A qualitative and quantitative characterization of known drug databases. J. Comb. Chem. 1, 55–68. doi: 10.1021/cc9800071
Gimeno, A., Ojeda-Montes, M., Tomás-Hernández, S., Cereto-Massagué, A., Beltrán-Debón, R., Mulero, M., et al. (2019). The light and dark sides of virtual screening: what is there to know? Int. J. Mol. Sci. 20:1375. doi: 10.3390/ijms20061375
Gohlke, B. O., Overkamp, T., Richter, A., Richter, A., Daniel, P. T., Gillissen, B., et al. (2015). 2D and 3D similarity landscape analysis identifies PARP as a novel off-target for the drug Vatalanib. BMC Bioinformatics 16:308. doi: 10.1186/s12859-015-0730-x
Gomes, M. N., Braga, R. C., Grzelak, E. M., Neves, B. J., Muratov, E., Ma, R., et al. (2017). QSAR-driven design, synthesis and discovery of potent chalcone derivatives with antitubercular activity. Eur. J. Med. Chem. 137, 126–138. doi: 10.1016/j.ejmech.2017.05.026
Gonczarek, A., Tomczak, J. M., Zareba, S., Kaczmar, J., Dabrowski, P., and Walczak, M. J. (2018). Interaction prediction in structure-based virtual screening using deep learning. Comput. Biol. Med. 100, 253–258. doi: 10.1016/j.compbiomed.2017.09.007
Gorgulla, C., Boeszoermenyi, A., Wang, Z. F., Fischer, P. D., Coote, P. W., Padmanabha Das, K. M., et al. (2020). An open-source drug discovery platform enables ultra-large virtual screens. Nature 580, 663–668. doi: 10.1038/s41586-020-2117-z
Gosse, J. T., Ghosh, S., Sproule, A., Overy, D., Cheeptham, N., and Boddy, C. N. (2019). Whole genome sequencing and metabolomic study of cave Streptomyces isolates ICC1 and ICC4. Front. Microbiol. 10:1020. doi: 10.3389/fmicb.2019.01020
Grant, J. A., Gallardo, M. A., and Pickup, B. T. (1996). A fast method of molecular shape comparison: a simple application of a Gaussian description of molecular shape. J. Comput. Chem. 17, 1653–1666. doi: 10.1002/(SICI)1096-987X(19961115)17:14<1653::AID-JCC7>3.0.CO;2-K
Grisoni, F., Merk, D., Friedrich, L., and Schneider, G. (2019). Design of natural-product-inspired multitarget ligands by machine learning. ChemMedChem 14, 1129–1134. doi: 10.1002/cmdc.201900097
Gu, J., Gui, Y., Chen, L., Yuan, G., Lu, H. Z. Z., and Xu, X. (2013). Use of natural products as chemical library for drug discovery and network pharmacology. PLoS ONE 8:e62839. doi: 10.1371/journal.pone.0062839
Guedes, I. A., Pereira, F. S. S., and Dardenne, L. E. (2018). Empirical scoring functions for structure-based virtual screening: applications, critical aspects, and challenges. Front. Pharmacol. 9:1089. doi: 10.3389/fphar.2018.01089
Gupta, S., Kapoor, P., Chaudhary, K., Gautam, A., Kumar, R., and Raghava, G. P. S. (2013). In silico approach for predicting toxicity of peptides and proteins. PLoS ONE 8:e73957. doi: 10.1371/journal.pone.0073957
Hamza, A., Wei, N. N., and Zhan, C. G. (2012). Ligand-based virtual screening approach using a new scoring function. J. Chem. Inf. Model. 52, 963–974. doi: 10.1021/ci200617d
Hao, G., Dong, Q., and Yang, G. (2011). A comparative study on the constitutive properties of marketed pesticides. Mol. Inform. 30, 614–622. doi: 10.1002/minf.201100020
Harvey, A. L., Edrada-Ebel, R., and Quinn, R. J. (2015). The re-emergence of natural products for drug discovery in the genomics era. Nat. Rev. Drug Discov. 14, 111–129. doi: 10.1038/nrd4510
Henninot, A., Collins, J. C., and Nuss, J. M. (2018). The current state of peptide drug discovery: back to the future? J. Med. Chem. 61, 1382–1414. doi: 10.1021/acs.jmedchem.7b00318
Hinselmann, G., Rosenbaum, L., Jahn, A., Fechner, N., and Zell, A. (2011). jCompoundMapper: an open source Java library and command-line tool for chemical fingerprints. J. Cheminform. 3:3. doi: 10.1186/1758-2946-3-3
Hossin, M., and Sulaiman, M. N. (2015). A review on evaluation metrics for data classification evaluations. Int. J. Data Min. Knowl. Manag. Process. 5, 1–11. doi: 10.5121/ijdkp.2015.5201
Huang, C., Yang, Y., Chen, X., Wang, C., Li, Y., Zheng, C., et al. (2017). Large-scale cross-species chemogenomic platform proposes a new drug discovery strategy of veterinary drug from herbal medicines. PLoS ONE 12:e0184880. doi: 10.1371/journal.pone.0184880
Huang, Y., You, Z., and Chen, X. (2018). A systematic prediction of drug-target interactions using molecular fingerprints and protein sequences. Curr. Protein Pept. Sci. 19, 468–478. doi: 10.2174/1389203718666161122103057
Huffman, B. J., and Shenvi, R. A. (2019). Natural products in the “Marketplace”: interfacing synthesis and biology. J. Am. Chem. Soc. 141, 3332–3346. doi: 10.1021/jacs.8b11297
Huggins, D. J., Venkitaraman, A. R., and Spring, D. R. (2011). Rational methods for the selection of diverse screening compounds. ACS Chem. Biol. 6, 208–217. doi: 10.1021/cb100420r
Instant JChem 21.4.0 (2021). ChemAxon. Available online at: http://www.chemaxon.com
Jade, D. D., Pandey, R., Kumar, R., and Gupta, D. (2020). Ligand-based pharmacophore modeling of TNF-α to design novel inhibitors using virtual screening and molecular dynamics. J. Biomol. Struct. Dyn. doi: 10.1080/07391102.2020.1831962. [Epub ahead of print].
Jagannathan, R. (2019). Characterization of drug-like chemical space for cytotoxic marine metabolites using multivariate methods. ACS Omega 4, 5402–5411. doi: 10.1021/acsomega.8b01764
Jasial, S., Gilberg, E., Blaschke, T., and Bajorath, J. (2018). Machine learning distinguishes with high accuracy between pan-assay interference compounds that are promiscuous or represent dark chemical matter. J. Med. Chem. 61, 10255–10264. doi: 10.1021/acs.jmedchem.8b01404
Jayaseelan, K. V., Moreno, P., Truszkowski, A., Ertl, P., and Steinbeck, C. (2012). Natural product-likeness score revisited: an open-source, open-data implementation. BMC Bioinformatics 13:106. doi: 10.1186/1471-2105-13-106
Jeffrey, P., and Summerfield, S. (2010). Assessment of the blood-brain barrier in CNS drug discovery. Neurobiol. Dis. 37, 33–37. doi: 10.1016/j.nbd.2009.07.033
Jeon, J., Nim, S., Teyra, J., Datti, A., Wrana, J. L., Sidhu, S. S., et al. (2014). A systematic approach to identify novel cancer drug targets using machine learning, inhibitor design and high-throughput screening. Genome Med. 6:57. doi: 10.1186/s13073-014-0057-7
Jhoti, H., Williams, G., Rees, D. C., and Murray, C. W. (2013). The “rule of three” for fragment-based drug discovery: where are we now? Nat. Rev. Drug Discov. 12:644. doi: 10.1038/nrd3926-c1
Jia, C. Y., Li, J. Y., Hao, G. F., and Yang, G. F. (2020). A drug-likeness toolbox facilitates ADMET study in drug discovery. Drug Discov. Today 25, 248–258. doi: 10.1016/j.drudis.2019.10.014
Jiang, S., Feher, M., Williams, C., Cole, B., and Shaw, D. E. (2020). AutoPH4: an automated method for generating pharmacophore models from protein binding pockets. J. Chem. Inf. Model. 60, 4326–4338. doi: 10.1021/acs.jcim.0c00121
Jiménez-Luna, J., Grisoni, F., and Schneider, G. (2020). Drug discovery with explainable artificial intelligence. Nat. Mach. Intell. 2, 573–584. doi: 10.1038/s42256-020-00236-4
Jin, Z., Wang, Y., Yu, X. F., Tan, Q. Q., Liang, S. S., Li, T., et al. (2020). Structure-based virtual screening of influenza virus RNA polymerase inhibitors from natural compounds: molecular dynamics simulation and MM-GBSA calculation. Comput. Biol. Chem. 85:107241. doi: 10.1016/j.compbiolchem.2020.107241
Jing, Y., Bian, Y., Hu, Z., Wang, L., and Xie, X. Q. S. (2018). Deep learning for drug design: an artificial intelligence paradigm for drug discovery in the big data era. AAPS J. 20:58. doi: 10.1208/s12248-018-0210-0
Jones, L. H., and Bunnage, M. E. (2017). Applications of chemogenomic library screening in drug discovery. Nat. Rev. Drug Discov. 16, 285–296. doi: 10.1038/nrd.2016.244
Kar, S., and Roy, K. (2013). How far can virtual screening take us in drug discovery? Expert Opin. Drug Discov. 8, 245–261. doi: 10.1517/17460441.2013.761204
Karaboga, A. S., Petronin, F., Marchetti, G., Souchet, M., and Maigret, B. (2013). Benchmarking of HPCC: a novel 3D molecular representation combining shape and pharmacophoric descriptors for efficient molecular similarity assessments. J. Mol. Graph. Model. 41, 20–30. doi: 10.1016/j.jmgm.2013.01.003
Kauffman, G. W., and Jurs, P. C. (2001). QSAR and k-nearest neighbor classification analysis of selective cyclooxygenase-2 inhibitors using topologically-based numerical descriptors. J. Chem. Inf. Comput. Sci. 41, 1553–1560. doi: 10.1021/ci010073h
Kim, S., Bolton, E. E., and Bryant, S. H. (2016). Similar compounds versus similar conformers: complementarity between PubChem 2-D and 3-D neighboring sets. J. Cheminform. 8:62. doi: 10.1186/s13321-016-0163-1
Kleigrewe, K., Almaliti, J., Tian, I. Y., Kinnel, R. B., Korobeynikov, A., Monroe, E. A., et al. (2015). Combining mass spectrometric metabolic profiling with genomic analysis: a powerful approach for discovering natural products from cyanobacteria. J. Nat. Prod. 78, 1671–1682. doi: 10.1021/acs.jnatprod.5b00301
Klupczynska, A., Plewa, S., Dereziński, P., Garrett, T. J., Rubio, V. Y., Kokot, Z. J., et al. (2020). Identification and quantification of honeybee venom constituents by multiplatform metabolomics. Sci. Rep. 10:21645. doi: 10.1038/s41598-020-78740-1
Koes, D. R., and Camacho, C. J. (2011). Pharmer: efficient and exact pharmacophore search. J. Chem. Inf. Model. 51, 1307–1314. doi: 10.1021/ci200097m
Koes, D. R., and Camacho, C. J. (2014). Shape-based virtual screening with volumetric aligned molecular shapes. J. Comput. Chem. 35, 1824–1834. doi: 10.1002/jcc.23690
Kong, W., Wang, W., and An, J. (2020). Prediction of 5-hydroxytryptamine transporter inhibitors based on machine learning. Comput. Biol. Chem. 87:107303. doi: 10.1016/j.compbiolchem.2020.107303
Kortagere, S., Krasowski, M. D., and Ekins, S. (2009). The importance of discerning shape in molecular pharmacology. Trends Pharmacol. Sci. 30, 138–147. doi: 10.1016/j.tips.2008.12.001
Kumar, A., and Zhang, K. Y. J. (2015). Hierarchical virtual screening approaches in small molecule drug discovery. Methods 71, 26–37. doi: 10.1016/j.ymeth.2014.07.007
Kumar, A., and Zhang, K. Y. J. (2018). Advances in the development of shape similarity methods and their application in drug discovery. Front. Chem. 6:315. doi: 10.3389/fchem.2018.00315
Kumar, D., Sharma, P., Singh, H., Nepali, K., Gupta, G. K., Jain, S. K., et al. (2017). The value of pyrans as anticancer scaffolds in medicinal chemistry. RSC Adv. 7, 36977–36999. doi: 10.1039/C7RA05441F
Kunimoto, R., and Bajorath, J. (2018). Combining similarity searching and network analysis for the identification of active compounds. ACS Omega 3, 3768–3777. doi: 10.1021/acsomega.8b00344
Lagorce, D., Sperandio, O., Baell, J. B., Miteva, M. A., and Villoutreix, B. O. (2015). FAF-Drugs3: a web server for compound property calculation and chemical library design. Nucleic Acids Res. 43, W200–W207. doi: 10.1093/nar/gkv353
Lans, I., Palacio-Rodríguez, K., Cavasotto, C. N., and Cossio, P. (2020). Flexi-pharma: a molecule-ranking strategy for virtual screening using pharmacophores from ligand-free conformational ensembles. J. Comput. Aided Mol. Des. 34, 1063–1077. doi: 10.1007/s10822-020-00329-7
Lata, S., Sharma, B., and Raghava, G. (2007). Analysis and prediction of antibacterial peptides. BMC Bioinformatics 8:263. doi: 10.1186/1471-2105-8-263
Lavecchia, A. (2015). Machine-learning approaches in drug discovery: methods and applications. Drug Discov. Today 20, 318–331. doi: 10.1016/j.drudis.2014.10.012
Lee, J., Park, J., Kim, J., Jeong, B., Choi, S. Y., Jang, H. S., et al. (2020). Targeted isolation of cytotoxic sesquiterpene lactones from Eupatorium fortunei by the NMR annotation tool, SMART 2.0. ACS Omega 5, 23989–23995. doi: 10.1021/acsomega.0c03270
Lee, M. L., and Schneider, G. (2001). Scaffold architecture and pharmacophoric properties of natural products and trade drugs: application in the design of natural product-based combinatorial libraries. J. Comb. Chem. 3, 284–289. doi: 10.1021/cc000097l
Li, F., Wang, Y., Li, D., Chen, Y., and Dou, Q. P. (2019). Are we seeing a resurgence in the use of natural products for new drug discovery? Expert Opin. Drug Discov. 14, 417–420. doi: 10.1080/17460441.2019.1582639
Li, G. H., and Huang, J. F. (2012). CDRUG: A web server for predicting anticancer activity of chemical compounds. Bioinformatics 28, 3334–3335. doi: 10.1093/bioinformatics/bts625
Li, H., Leung, K. S., Wong, M. H., and Ballester, P. J. (2016). USR-VS: a web server for large-scale prospective virtual screening using ultrafast shape recognition techniques. Nucleic Acids Res. 44, W436–W441. doi: 10.1093/nar/gkw320
Li, H., Sze, K. H., Lu, G., and Ballester, P. J. (2020). Machine-learning scoring functions for structure-based virtual screening. Wiley Interdiscipl. Rev. Comput. Mol. Sci. 11:e1478. doi: 10.1002/wcms.1478
Li, J. W. H., and Vederas, J. C. (2009). Drug discovery and natural products: end of an era or an endless frontier? Science 325, 161–165. doi: 10.1126/science.1168243
Lima, M. N. N., Borba, J. V. B., Cassiano, G. C., Mottin, M., Mendonça, S. S., Silva, A. C., et al. (2020). Artificial intelligence applied for the rapid identification of new antimalarial candidates with dual-stage activity. ChemMedChem 16, 1093–1103. doi: 10.1002/cmdc.202000685
Lipinski, C. A., Lombardo, F., Dominy, B. W., Feeney, P. J., Lombardo, F., Dominy, B. W., et al. (1997). Experimental and computational approaches to estimate solubility and permeability in drug discovery and development settings. Adv. Drug Deliv. Rev. 23, 3–25. doi: 10.1016/S0169-409X(96)00423-1
Liu, X., Jiang, H., and Li, H. (2011). SHAFTS: a hybrid approach for 3D molecular similarity calculation. 1. Method and assessment of virtual screening. J. Chem. Inf. Model. 51, 2372–2385. doi: 10.1021/ci200060s
Liu, X., Ouyang, S., Yu, B., Liu, Y., Huang, K., Gong, J., et al. (2010). PharmMapper server: a web server for potential drug target identification using pharmacophore mapping approach. Nucleic Acids Res. 38, W609–W614. doi: 10.1093/nar/gkq300
Liu, Z., Du, J., Fang, J., Yin, Y., Xu, G., and Xie, L. (2019). DeepScreening: a deep learning-based screening web server for accelerating drug discovery. Database 2019:baz104. doi: 10.1093/database/baz104
Liu, Z., Huang, D., Zheng, S., Song, Y., Liu, B., Sun, J., et al. (2020). Deep learning enables discovery of highly potent anti-osteoporosis natural products. Eur. J. Med. Chem. 210:112982. doi: 10.1016/j.ejmech.2020.112982
Lo, Y. C., Rensi, S. E., Torng, W., and Altman, R. B. (2018). Machine learning in chemoinformatics and drug discovery. Drug Discov. Today 23, 1538–1546. doi: 10.1016/j.drudis.2018.05.010
Lovering, F. (2013). Escape from Flatland 2: complexity and promiscuity. MedChemComm 4:515. doi: 10.1039/c2md20347b
Lovrić, M., Molero, J. M., and Kern, R. (2019). PySpark and RDKit: moving towards big data in cheminformatics. Mol. Inform. 38:1800082. doi: 10.1002/minf.201800082
Lucas, X., Senger, C., Erxleben, A., Grüning, B. A., Döring, K., Mosch, J., et al. (2013). StreptomeDB: a resource for natural compounds isolated from Streptomyces species. Nucleic Acids Res. 41, D1130–D1136. doi: 10.1093/nar/gks1253
Luo, Q., Zhao, L., Hu, J., Jin, H., Liu, Z., and Zhang, L. (2017). The scoring bias in reverse docking and the score normalization strategy to improve success rate of target fishing. PLoS ONE 12:e0171433. doi: 10.1371/journal.pone.0171433
Lyu, C., Chen, T., Qiang, B., Liu, N., Wang, H., Zhang, L., et al. (2020). CMNPD: a comprehensive marine natural products database towards facilitating drug discovery from the ocean. Nucleic Acids Res. 49, D509–D515. doi: 10.1093/nar/gkaa763
Macalino, S. J. Y., Gosu, V., Hong, S., and Choi, S. (2015). Role of computer-aided drug design in modern drug discovery. Arch. Pharm. Res. 38, 1686–1701. doi: 10.1007/s12272-015-0640-5
Madzhidov, T. I., Rakhimbekova, A., Kutlushuna, A., and Polishchuk, P. (2020). Probabilistic approach for virtual screening based on multiple pharmacophores. Molecules 25:385. doi: 10.3390/molecules25020385
Maggiora, G. M., and Bajorath, J. (2014). Chemical space networks: a powerful new paradigm for the description of chemical space. J. Comput. Aided Mol. Des. 28, 795–802. doi: 10.1007/s10822-014-9760-0
Maia, E. H. B., Assis, L. C., de Oliveira, T. A., da Silva, A. M., and Taranto, A. G. (2020). Structure-based virtual screening: from classical to artificial intelligence. Front. Chem. 8:343. doi: 10.3389/fchem.2020.00343
Maia, S. M., de Sousa, N. F., Rodrigues, G. C. S., Monteiro, A. F. M., Scotti, M. T., and Scotti, L. (2020). Lignans and neolignans anti-tuberculosis identified by QSAR and molecular modeling. Comb. Chem. High Throughput Screen. 23, 504–516. doi: 10.2174/1386207323666200226094940
Manavalan, B., Subramaniyam, S., Shin, T. H., Kim, M. O., and Lee, G. (2018). Machine-learning-based prediction of cell-penetrating peptides and their uptake efficiency with improved accuracy. J. Proteome Res. 17, 2715–2726. doi: 10.1021/acs.jproteome.8b00148
Martínez-Treviño, S. H., Uc-Cetina, V., Fernández-Herrera, M. A., and Merino, G. (2020). Prediction of natural product classes using machine learning and 13C NMR spectroscopic data. J. Chem. Inf. Model. 60, 3376–3386. doi: 10.1021/acs.jcim.0c00293
Mascarenhas, A. M. S., de Almeida, R. B. M., de Araujo Neto, M. F., Mendes, G. O., da Cruz, J. N., dos Santos, C. B. R., et al. (2020). Pharmacophore-based virtual screening and molecular docking to identify promising dual inhibitors of human acetylcholinesterase and butyrylcholinesterase. J. Biomol. Struct. Dyn. doi: 10.1080/07391102.2020.1796791. [Epub ahead of print].
Mayr, A., Klambauer, G., Unterthiner, T., and Hochreiter, S. (2016). DeepTox: toxicity prediction using deep learning. Front. Environ. Sci. 3:80. doi: 10.3389/fenvs.2015.00080
Medina-Franco, J. L., Golbraikh, A., Oloff, S., Castillo, R., and Tropsha, A. (2005). Quantitative structure-activity relationship analysis of pyridinone HIV-1 reverse transcriptase inhibitors using the k nearest neighbor method and QSAR-based database mining. J. Comput. Aided Mol. Des. 19, 229–242. doi: 10.1007/s10822-005-4789-8
Medina-Franco, J. L., and Saldívar-González, F. I. (2020). Cheminformatics to characterize pharmacologically active natural products. Biomolecules 10:1566. doi: 10.3390/biom10111566
Merwin, N. J., Mousa, W. K., Dejong, C. A., Skinnider, M. A., Cannon, M. J., Li, H., et al. (2020). DeepRiPP integrates multiomics data to automate discovery of novel ribosomally synthesized natural products. Proc. Natl. Acad. Sci. U.S.A. 117, 371–380. doi: 10.1073/pnas.1901493116
Mignani, S., Rodrigues, J., Tomas, H., Jalal, R., Singh, P. P., Majoral, J. P., et al. (2018). Present drug-likeness filters in medicinal chemistry during the hit and lead optimization process: how far can they be simplified? Drug Discov. Today 23, 605–615. doi: 10.1016/j.drudis.2018.01.010
Miteva, M. A., Violas, S., Montes, M., Gomez, D., Tuffery, P., and Villoutreix, B. O. (2006). FAF-Drugs: free ADME/tox filtering of compound collections. Nucleic Acids Res. 34, W738–W744. doi: 10.1093/nar/gkl065
Molecular Operating Environment (2019). Chemical Computing Group ULC. Montreal, QC: Molecular Operating Environment, 202.
Morais, T. R., Conserva, G. A. A., Varela, M. T., Costa-Silva, T. A., Thevenard, F., Ponci, V., et al. (2020). Improving the drug-likeness of inspiring natural products - evaluation of the antiparasitic activity against Trypanosoma cruzi through semi-synthetic and simplified analogues of licarin A. Sci. Rep. 10:5467. doi: 10.1038/s41598-020-62352-w
Moumbock, A. F. A., Gao, M., Qaseem, A., Li, J., Kirchner, P. A., Ndingkokhar, B., et al. (2021). StreptomeDB 3.0: an updated compendium of streptomycetes natural products. Nucleic Acids Res. 49, D600–D604. doi: 10.1093/nar/gkaa868
Muegge, I., and Mukherjee, P. (2016). An overview of molecular fingerprint similarity search in virtual screening. Expert Opin. Drug Discov. 11, 137–148. doi: 10.1517/17460441.2016.1117070
Naghizadeh, A., Hamzeheian, D., Akbari, S., Mohammadi, F., Otoufat, T., Asgari, S., et al. (2020). UNaProd: a universal natural product database for materia medica of iranian traditional medicine. Evid. Based Complement. Altern. Med. 2020, 1–14. doi: 10.1155/2020/3690781
Naylor, M. R., Bockus, A. T., Blanco, M. J., and Lokey, R. S. (2017). Cyclic peptide natural products chart the frontier of oral bioavailability in the pursuit of undruggable targets. Curr. Opin. Chem. Biol. 38, 141–147. doi: 10.1016/j.cbpa.2017.04.012
Newman, D. J., and Cragg, G. M. (2016). Natural products as sources of new drugs from 1981 to 2014. J. Nat. Prod. 79, 629–661. doi: 10.1021/acs.jnatprod.5b01055
Newman, D. J., and Cragg, G. M. (2020). Natural products as sources of new drugs over the nearly four decades from 01/1981 to 09/2019. J. Nat. Prod. 83, 770–803. doi: 10.1021/acs.jnatprod.9b01285
Nocedo-Mena, D., Cornelio, C., Camacho-Corona M del R, Garza-González, E., Waksman de Torres, N., Arrasate, S., et al. (2019). Modeling antibacterial activity with machine learning and fusion of chemical structure information with microorganism metabolic networks. J. Chem. Inf. Model. 59, 1109–1120. doi: 10.1021/acs.jcim.9b00034
Ntie-Kang, F., Telukunta, K. K., Döring, K., Simoben, C. V., Moumbock, A. F. A., Malange, Y. I., et al. (2017). NANPDB: a resource for natural products from northern African Sources. J. Nat. Prod. 80, 2067–2076. doi: 10.1021/acs.jnatprod.7b00283
O'Boyle, N. M., Morley, C., and Hutchison, G. R. (2008). Pybel: a Python wrapper for the OpenBabel cheminformatics toolkit. Chem. Cent. J. 2:5. doi: 10.1186/1752-153X-2-5
O'Hagan, S., and Kell, D. B. (2016). MetMaxStruct: a Tversky-similarity-based strategy for analysing the (sub)structural similarities of drugs and endogenous metabolites. Front. Pharmacol. 7:266. doi: 10.3389/fphar.2016.00266
Olivon, F., Allard, P. M., Koval, A., Righi, D., Genta-Jouve, G., Neyts, J., et al. (2017). Bioactive natural products prioritization using massive multi-informational molecular networks. ACS Chem. Biol. 12, 2644–2651. doi: 10.1021/acschembio.7b00413
Opassi, G., Gesù, A., and Massarotti, A. (2018). The hitchhiker's guide to the chemical-biological galaxy. Drug Discov. Today 23, 565–574. doi: 10.1016/j.drudis.2018.01.007
Oppong-Danquah, E., Parrot, D., Blümel, M., Labes, A., and Tasdemir, D. (2018). Molecular networking-based metabolome and bioactivity analyses of marine-adapted fungi co-cultivated with phytopathogens. Front. Microbiol. 9:2072. doi: 10.3389/fmicb.2018.02072
Oprea, T. I. (2000). Property distribution of drug-related chemical databases. J. Comput. Aided Mol. Des. 14, 251–264. doi: 10.1023/A:1008130001697
Over, B., Wetzel, S., Grütter, C., Nakai, Y., Renner, S., Rauh, D., et al. (2013). Natural-product-derived fragments for fragment-based ligand discovery. Nat. Chem. 5, 21–28. doi: 10.1038/nchem.1506
Pal, S., Kumar, V., Kundu, B., Bhattacharya, D., Preethy, N., Reddy, M. P., et al. (2019). Ligand-based pharmacophore modeling, virtual screening and molecular docking studies for discovery of potential topoisomerase I inhibitors. Comput. Struct. Biotechnol. J. 17, 291–310. doi: 10.1016/j.csbj.2019.02.006
Patel, L., Shukla, T., Huang, X., Ussery, D. W., and Wang, S. (2020). Machine learning methods in drug discovery. Molecules 25:5277. doi: 10.3390/molecules25225277
Patsilinakos, A., Artini, M., Papa, R., Sabatino, M., BoŽović, M, Garzoli, S., et al. (2019). Machine learning analyses on data including essential oil chemical composition and in vitro experimental antibiofilm activities against Staphylococcus species. Molecules 24:890. doi: 10.3390/molecules24050890
Pavadai, E., Kaur, G., Wittlin, S., and Chibale, K. (2017). Identification of steroid-like natural products as antiplasmodial agents by 2D and 3D similarity-based virtual screening. MedChemComm 8, 1152–1157. doi: 10.1039/C7MD00063D
Pedregosa, F., Varoquaux, G., Gramfort, A., Michel, V., Thirion, B., Grisel, O., et al. (2011). Scikit-learn: machine learning in Python. J. Mach. Learn. Res. 12, 2825–2830.
Pereira, F., Latino, D., and Gaudêncio, S. (2015). QSAR-assisted virtual screening of lead-like molecules from marine and microbial natural sources for antitumor and antibiotic drug discovery. Molecules 20, 4848–4873. doi: 10.3390/molecules20034848
Pilon, A. C., Valli, M., Dametto, A. C., Pinto, M. E. F., Freire, R. T., Castro-Gamboa, I., et al. (2017). NuBBE DB: an updated database to uncover chemical and biological information from Brazilian biodiversity. Sci. Rep. 7:7215. doi: 10.1038/s41598-017-07451-x
Pilón-Jiménez, B., Saldívar-González, F., Díaz-Eufracio, B., and Medina-Franco, J. (2019). BIOFACQUIM: a mexican compound database of natural products. Biomolecules 9:31. doi: 10.3390/biom9010031
Pire, D. E. V., Blundell, T. L., and Ascher, D. B. (2015). pkCSM: predicting small-molecule pharmacokinetic and toxicity properties using graph-based signatures. J. Med. Chem. 58, 4066–4072. doi: 10.1021/acs.jmedchem.5b00104
Pu, L., Naderi, M., Liu, T., Wu, H. C., Mukhopadhyay, S., and Brylinski, M. (2019). EToxPred: a machine learning-based approach to estimate the toxicity of drug candidates 11 Medical and Health Sciences 1115 Pharmacology and Pharmaceutical Sciences 03 Chemical Sciences 0305 Organic Chemistry 03 Chemical Sciences 0304 Medicinal and Biomolecular Chemistry. BMC Pharmacol. Toxicol. 20:2. doi: 10.1186/s40360-018-0282-6
Puertas-Martín, S., Redondo, J. L., Ortigosa, P. M., and Pérez-Sánchez, H. (2019). OptiPharm: an evolutionary algorithm to compare shape similarity. Sci. Rep. 9:1398. doi: 10.1038/s41598-018-37908-6
Qiang, X., Zhou, C., Ye, X., Du, P., Su, R., and Wei, L. (2018). CPPred-FL: a sequence-based predictor for large-scale identification of cell-penetrating peptides by feature representation learning. Brief. Bioinform. doi: 10.1093/bib/bby091. [Epub ahead of print].
Qing, X., Lee, X. Y., De Raeymaeker, J., Tame, J. R., Zhang, K. Y., De Maeyer, M., et al. (2014). Pharmacophore modeling: advances, limitations, and current utility in drug discovery. J. Receptor Ligand Channel Res. 7, 81–92. doi: 10.2147/JRLCR.S46843
Rácz, A., Bajusz, D., and Héberger, K. (2018). Life beyond the Tanimoto coefficient: similarity measures for interaction fingerprints. J. Cheminform. 10:48. doi: 10.1186/s13321-018-0302-y
Rácz, A., Bajusz, D., and Héberger, K. (2019). Multi-level comparison of machine learning classifiers and their performance metrics. Molecules 24:2811. doi: 10.3390/molecules24152811
Rampogu, S., Son, M., Baek, A., Park, C., Rana, R. M., Zeb, A., et al. (2018). Targeting natural compounds against HER2 kinase domain as potential anticancer drugs applying pharmacophore based molecular modelling approaches. Comput. Biol. Chem. 74, 327–338. doi: 10.1016/j.compbiolchem.2018.04.002
Rayan, A., Raiyn, J., and Falah, M. (2017). Nature is the best source of anticancer drugs: indexing natural products for their anticancer bioactivity. PLoS ONE 12:e0187925. doi: 10.1371/journal.pone.0187925
Reher, R., Kim, H. W., Zhang, C., Mao, H. H., Wang, M., Nothias, L. F., et al. (2020). A convolutional neural network-based approach for the rapid annotation of molecularly diverse natural products. J. Am. Chem. Soc. 142, 4114–4120. doi: 10.1021/jacs.9b13786
Riniker, S., and Landrum, G. A. (2013). Similarity maps–a visualization strategy for molecular fingerprints and machine-learning methods. J. Cheminform. 5:43. doi: 10.1186/1758-2946-5-43
Ripphausen, P., Wassermann, A. M., and Bajorath, J. (2011). REPROVIS-DB: a benchmark system for ligand-based virtual screening derived from reproducible prospective applications. J. Chem. Inf. Model. 51, 2467–2473. doi: 10.1021/ci200309j
Rodrigues, T. (2017). Harnessing the potential of natural products in drug discovery from a cheminformatics vantage point. Org. Biomol. Chem. 15, 9275–9282. doi: 10.1039/C7OB02193C
Rodrigues, T., Reker, D., Schneider, P., and Schneider, G. (2016). Counting on natural products for drug design. Nat. Chem. 8, 531–541. doi: 10.1038/nchem.2479
Ropp, P. J., Spiegel, J. O., Walker, J. L., Green, H., Morales, G. A., Milliken, K. A., et al. (2019). Gypsum-DL: an open-source program for preparing small-molecule libraries for structure-based virtual screening. J. Cheminform. 11:34. doi: 10.1186/s13321-019-0358-3
Rossi Sebastiano, M., Doak, B. C., Backlund, M., Poongavanam, V., Over, B., Ermondi, G., et al. (2018). Impact of dynamically exposed polarity on permeability and solubility of chameleonic drugs beyond the rule of 5. J. Med. Chem. 61, 4189–4202. doi: 10.1021/acs.jmedchem.8b00347
Roumpeka, D. D., Wallace, R. J., Escalettes, F., Fotheringham, I., and Watson, M. (2017). A review of bioinformatics tools for bio-prospecting from metagenomic sequence data. Front. Genet. 8:23. doi: 10.3389/fgene.2017.00023
Rupp, M., Schroeter, T., Steri, R., Zettl, H., Proschak, E., Hansen, K., et al. (2010). From machine learning to natural product derivatives that selectively activate transcription factor PPARγ. ChemMedChem 5, 191–194. doi: 10.1002/cmdc.200900469
Saldívar-González, F. I., Huerta-García, C. S., and Medina-Franco, J. L. (2020). Chemoinformatics-based enumeration of chemical libraries: a tutorial. J. Cheminform. 12:64. doi: 10.1186/s13321-020-00466-z
Sánchez-Cruz, N., and Medina-Franco, J. L. (2018). Statistical-based database fingerprint: Chemical space dependent representation of compound databases. J. Cheminform. 10:55. doi: 10.1186/s13321-018-0311-x
Sander, T., Freyss, J., von Korff, M., Rufener, C., von Korff, M., and Rufener, C. (2015). DataWarrior: an open-source program for chemistry aware data visualization and analysis. J. Chem. Inf. Model. 55, 460–473. doi: 10.1021/ci500588j
Sanders, M. P. A., Barbosa, A. J. M., Zarzycka, B., Nicolaes, G. A. F., Klomp, J. P. G., de Vlieg, J., et al. (2012). Comparative analysis of pharmacophore screening tools. J. Chem. Inf. Model. 52, 1607–1620. doi: 10.1021/ci2005274
Santacruz, L., Hurtado, D. X., Doohan, R., Thomas, O. P., Puyana, M., and Tello, E. (2020). Metabolomic study of soft corals from the Colombian Caribbean: PSYCHE and 1H-NMR comparative analysis. Sci. Rep. 10:5417. doi: 10.1038/s41598-020-62413-0
Santana, I. B., Leite, F. H. A., and Santos Junior, M. C. (2018). Identification of lutzomyia longipalpis odorant binding protein modulators by comparative modeling, hierarchical virtual screening, and molecular dynamics. J. Chem. 2018, 1–10. doi: 10.1155/2018/4173479
Sato, T., Honma, T., and Yokoyama, S. (2010). Combining machine learning and pharmacophore-based interaction fingerprint for in silico screening. J. Chem. Inf. Model. 50, 170–185. doi: 10.1021/ci900382e
Schaduangrat, N., Nantasenamat, C., Prachayasittikul, V., and Shoombuatong, W. (2019). ACPred: a computational tool for the prediction and analysis of anticancer peptides. Molecules 24:1973. doi: 10.3390/molecules24101973
Schaller, D., Šribar, D., Noonan, T., Deng, L., Nguyen, T. N., Pach, S., et al. (2020). Next generation 3D pharmacophore modeling. WIREs Comput. Mol. Sci. 10:e1468. doi: 10.1002/wcms.1468
Schwartz, J., Awale, M., and Reymond, J. L. (2013). SMIfp (SMILES fingerprint) chemical space for virtual screening and visualization of large databases of organic molecules. J. Chem. Inf. Model. 53, 1979–1989. doi: 10.1021/ci400206h
Seddon, M. P., Cosgrove, D. A., Packer, M. J., and Gillet, V. J. (2019). Alignment-free molecular shape comparison using spectral geometry: the framework. J. Chem. Inf. Model. 59, 98–116. doi: 10.1021/acs.jcim.8b00676
Semighini, E. P., Resende, J. A., De Andrade, P., Morais, P. A. B., Carvalho, I., Taft, C. A., et al. (2011). Using computer-aided drug design and medicinal chemistry strategies in the fight against diabetes. J. Biomol. Struct. Dyn. 28, 787–796. doi: 10.1080/07391102.2011.10508606
Senger, S. (2009). Using tversky similarity searches for core hopping: finding the needles in the haystack. J. Chem. Inf. Model. 49, 1514–1524. doi: 10.1021/ci900092y
Shahin, R., Swellmeen, L., Shaheen, O., Aboalhaija, N., and Habash, M. (2016). Identification of novel inhibitors for Pim-1 kinase using pharmacophore modeling based on a novel method for selecting pharmacophore generation subsets. J. Comput. Aided Mol. Des. 30, 39–68. doi: 10.1007/s10822-015-9887-7
Shang, J., Hu, B., Wang, J., Zhu, F., Kang, Y., Li, D., et al. (2018). Cheminformatic insight into the differences between terrestrial and marine originated natural products. J. Chem. Inf. Model. 58, 1182–1193. doi: 10.1021/acs.jcim.8b00125
Shin, W. H., Zhu, X., Bures, M., and Kihara, D. (2015). Three-dimensional compound comparison methods and their application in drug discovery. Molecules 20, 12841–12862. doi: 10.3390/molecules200712841
Shoombuatong, W., Schaduangrat, N., Pratiwi, R., and Nantasenamat, C. (2019). THPep: a machine learning-based approach for predicting tumor homing peptides. Comput. Biol. Chem. 80, 441–451. doi: 10.1016/j.compbiolchem.2019.05.008
Shultz, M. D. (2019). Two decades under the influence of the rule of five and the changing properties of approved oral drugs. J. Med. Chem. 62, 1701–1714. doi: 10.1021/acs.jmedchem.8b00686
Sieg, J., Flachsenberg, F., and Rarey, M. (2019). In need of bias control: evaluating chemical data for machine learning in structure-based virtual screening. J. Chem. Inf. Model. 59, 947–961. doi: 10.1021/acs.jcim.8b00712
Silva, G. N. S., da, Primon-Barros, M., Macedo, A. J., and Gnoatto, S. C. B. (2019). Triterpene derivatives as relevant scaffold for new antibiofilm drugs. Biomolecules 9:58. doi: 10.3390/biom9020058
Skinnider, M. A., Dejong, C. A., Franczak, B. C., McNicholas, P. D., and Magarvey, N. A. (2017). Comparative analysis of chemical similarity methods for modular natural products with a hypothetical structure enumeration algorithm. J. Cheminform. 9:46. doi: 10.1186/s13321-017-0234-y
Skirycz, A., Kierszniowska, S., Méret, M., Willmitzer, L., and Tzotzos, G. (2016). Medicinal bioprospecting of the Amazon rainforest: a modern Eldorado? Trends Biotechnol. 34, 781–790. doi: 10.1016/j.tibtech.2016.03.006
Slater, O., and Kontoyianni, M. (2019). The compromise of virtual screening and its impact on drug discovery. Expert Opin. Drug Discov. 14, 619–637. doi: 10.1080/17460441.2019.1604677
Smith, P. A., Koehler, M. F. T., Girgis, H. S., Yan, D., Chen, Y., Chen, Y., et al. (2018). Optimized arylomycins are a new class of Gram-negative antibiotics. Nature 561, 189–194. doi: 10.1038/s41586-018-0483-6
Soares Rodrigues, G. C., Maia, M, dos, S A. B., Costa Barros, R. P., Scotti, L., Cespedes-Acuña, C. L., et al. (2021). Computer-assisted discovery of compounds with insecticidal activity against Musca domestica and Mythimna separata. Food Chem. Toxicol. 147:111899. doi: 10.1016/j.fct.2020.111899
Software O Scientific (2008). OpenEye Scientific Software. Available online at: http://www.eyesopen.com/products/applications/filter.html
Sorokina, M., and Steinbeck, C. (2019). NaPLeS: a natural products likeness scorer—web application and database. J. Cheminform. 11:55. doi: 10.1186/s13321-019-0378-z
Spyrakis, F., Bellio, P., Quotadamo, A., Linciano, P., Benedetti, P., D'Arrigo, G., et al. (2019). First virtual screening and experimental validation of inhibitors targeting GES-5 carbapenemase. J. Comput. Aided Mol. Des. 33, 295–305. doi: 10.1007/s10822-018-0182-2
Stokes, J. M., Yang, K., Swanson, K., Jin, W., Cubillos-Ruiz, A., Donghia, N. M., et al. (2020). A deep learning approach to antibiotic discovery. Cell 180, 688–702.e13. doi: 10.1016/j.cell.2020.01.021
Sunseri, J., and Koes, D. R. (2016). Pharmit: interactive exploration of chemical space. Nucleic Acids Res. 44, W442–W448. doi: 10.1093/nar/gkw287
Svetnik, V., Liaw, A., Tong, C., Culberson, J. C., Sheridan, R. P., and Feuston, B. P. (2003). Random forest: a classification and regression tool for compound classification and QSAR modeling. J. Chem. Inf. Comput. Sci. 43, 1947–1958. doi: 10.1021/ci034160g
Tahir, R. A., Hassan, F., Kareem, A., Iftikhar, U., and Sehgal, S. A. (2020). Ligand-based pharmacophore modeling and virtual screening to discover novel CYP1A1 inhibitors. Curr. Top. Med. Chem. 19, 2782–2794. doi: 10.2174/1568026619666191112104217
Taminau, J., Thijs, G., and De Winter, H. (2008). Pharao: pharmacophore alignment and optimization. J. Mol. Graph. Model. 27, 161–169. doi: 10.1016/j.jmgm.2008.04.003
Tao, L., Zhu, F., Qin, C., Zhang, C., Chen, S., Zhang, P., et al. (2015). Clustered distribution of natural product leads of drugs in the chemical space as influenced by the privileged target-sites. Sci. Rep. 5:9325. doi: 10.1038/srep09325
Thireou, T., Kythreoti, G., Tsitsanou, K. E., Koussis, K., Drakou, C. E., Kinnersley, J., et al. (2018). Identification of novel bioinspired synthetic mosquito repellents by combined ligand-based screening and OBP-structure-based molecular docking. Insect Biochem. Mol. Biol. 98, 48–61. doi: 10.1016/j.ibmb.2018.05.001
Thomford, N., Senthebane, D., Rowe, A., Munro, D., Seele, P., Maroyi, A., et al. (2018). Natural products for drug discovery in the 21st century: innovations for novel drug discovery. Int. J. Mol. Sci. 19:1578. doi: 10.3390/ijms19061578
Tice, C. M. (2001). Selecting the right compounds for screening: does Lipinski's Rule of 5 for pharmaceuticals apply to agrochemicals? Pest Manag. Sci. 57, 3–16. doi: 10.1002/1526-4998(200101)57:1<3::AID-PS269>3.0.CO;2-6
Tomar, V., Mazumder, M., Chandra, R., Yang, J., and Sakharkar, M. K. (2018). “Small molecule drug design,” in Encyclopedia of Bioinformatics and Computational Biology: ABC of Bioinformatics, Vol. 1–3, eds S. Ranganathan, M. Gribskov, K. Nakai, and C. B. Schönbach (Oxford: Academic Press), 741–760. doi: 10.1016/B978-0-12-809633-8.20157-X
Trujillo-Correa, A. I., Quintero-Gil, D. C., Diaz-Castillo, F., Quiñones, W., Robledo, S. M., and Martinez-Gutierrez, M. (2019). In vitro and in silico anti-dengue activity of compounds obtained from Psidium guajava through bioprospecting. BMC Complement. Altern. Med. 19:298. doi: 10.1186/s12906-019-2695-1
Tsou, L. K., Yeh, S. H., Ueng, S. H., Chang, C. P., Song, J. S., Wu, M. H., et al. (2020). Comparative study between deep learning and QSAR classifications for TNBC inhibitors and novel GPCR agonist discovery. Sci. Rep. 10:16771. doi: 10.1038/s41598-020-73681-1
Valli, M., dos Santos, R. N., Figueira, L. D., Nakajima, C. H., Castro-Gamboa, I., Andricopulo, A. D., et al. (2013). Development of a natural products database from the biodiversity of Brazil. J. Nat. Prod. 76, 439–444. doi: 10.1021/np3006875
Vamathevan, J., Clark, D., Czodrowski, P., Dunham, I., Ferran, E., Lee, G., et al. (2019). Applications of machine learning in drug discovery and development. Nat. Rev. Drug Discov. 18, 463–477. doi: 10.1038/s41573-019-0024-5
van Santen, J. A., Jacob, G., Singh, A. L., Aniebok, V., Balunas, M. J., Bunsko, D., et al. (2019). The natural products atlas: an open access knowledge base for microbial natural products discovery. ACS Cent. Sci. 5, 1824–1833. doi: 10.1021/acscentsci.9b00806
Veber, D. F., Johnson, S. R., Cheng, H. Y. Y., Smith, B. R., Ward, K. W., and Kopple, K. D. (2002). Molecular properties that influence the oral bioavailability of drug candidates. J. Med. Chem. 45, 2615–2623. doi: 10.1021/jm020017n
Virtanen, P., Gommers, R., Oliphant, T. E., Haberland, M., Reddy, T., Cournapeau, D., et al. (2020). SciPy 1.0: fundamental algorithms for scientific computing in Python. Nat. Methods 17, 261–272. doi: 10.1038/s41592-019-0686-2
Vuorinen, A., and Schuster, D. (2015). Methods for generating and applying pharmacophore models as virtual screening filters and for bioactivity profiling. Methods 71, 113–134. doi: 10.1016/j.ymeth.2014.10.013
Wallach, I., and Heifets, A. (2018). Most ligand-based classification benchmarks reward memorization rather than generalization. J. Chem. Inf. Model. 58, 916–932. doi: 10.1021/acs.jcim.7b00403
Walters, W. P. (2019). Virtual chemical libraries. J. Med. Chem. 62, 1116–1124. doi: 10.1021/acs.jmedchem.8b01048
Walters, W. P., and Murcko, M. A. (2002). Prediction of ‘drug-likeness.’ Adv. Drug Deliv. Rev. 54, 255–271. doi: 10.1016/S0169-409X(02)00003-0
Walters, W. P., and Namchuk, M. (2003). Designing screens: how to make your hits a hit. Nat. Rev. Drug Discov. 2, 259–266. doi: 10.1038/nrd1063
Wang, L., Ma, C., Wipf, P., Liu, H., Su, W., and Xie, X. Q. Q. (2013). TargetHunter: an in silico target identification tool for predicting therapeutic potential of small organic molecules based on chemogenomic database. AAPS J. 15, 395–406. doi: 10.1208/s12248-012-9449-z
Wang, Y., Jafari, M., Tang, Y., and Tang, J. (2019). Predicting Meridian in Chinese traditional medicine using machine learning approaches. PLoS Comput. Biol. 15:e1007249. doi: 10.1371/journal.pcbi.1007249
Wang, Z., Sun, H., Shen, C., Hu, X., Gao, J., Li, D., et al. (2020). Combined strategies in structure-based virtual screening. Phys. Chem. Chem. Phys. 22, 3149–3159. doi: 10.1039/C9CP06303J
Wei, L., Xing, P., Su, R., Shi, G., Ma, Z. S., and Zou, Q. (2017). CPPred-RF: a sequence-based predictor for identifying cell-penetrating peptides and their uptake efficiency. J. Proteome Res. 16, 2044–2053. doi: 10.1021/acs.jproteome.7b00019
Williams, A. J., and Ekins, S. (2011). A quality alert and call for improved curation of public chemistry databases. Drug Discov. Today 16, 747–750. doi: 10.1016/j.drudis.2011.07.007
Williams, A. J., Ekins, S., and Tkachenko, V. (2012). Towards a gold standard: regarding quality in public domain chemistry databases and approaches to improving the situation. Drug Discov. Today 17, 685–701. doi: 10.1016/j.drudis.2012.02.013
Willighagen, E. L., Mayfield, J. W., Alvarsson, J., Berg, A., Carlsson, L., Jeliazkova, N., et al. (2017). The chemistry development kit (CDK) v2.0: atom typing, depiction, molecular formulas, and substructure searching. J. Cheminform. 9:33. doi: 10.1186/s13321-017-0220-4
Wingert, B. M., and Camacho, C. J. (2018). Improving small molecule virtual screening strategies for the next generation of therapeutics. Curr. Opin. Chem. Biol. 44, 87–92. doi: 10.1016/j.cbpa.2018.06.006
Wójcikowski, M., Ballester, P. J., and Siedlecki, P. (2017). Performance of machine-learning scoring functions in structure-based virtual screening. Sci. Rep. 7:46710. doi: 10.1038/srep46710
Wolber, G., and Langer, T. (2005). LigandScout: 3-D pharmacophores derived from protein-bound ligands and their use as virtual screening filters. J. Chem. Inf. Model. 45, 160–169. doi: 10.1021/ci049885e
Wolfe, J. M., Fadzen, C. M., Choo, Z. N., Holden, R. L., Yao, M., Hanson, G. J., et al. (2018). Machine learning to predict cell-penetrating peptides for antisense delivery. ACS Cent. Sci. 4, 512–520. doi: 10.1021/acscentsci.8b00098
Wolfender, J. L., Litaudon, M., Touboul, D., and Queiroz, E. F. (2019). Innovative omics-based approaches for prioritisation and targeted isolation of natural products–new strategies for drug discovery. Nat. Prod. Rep. 36, 855–868. doi: 10.1039/C9NP00004F
Wood, D. J., Vlieg, J, de Wagener, M., and Ritschel, T. (2012). Pharmacophore fingerprint-based approach to binding site subpocket similarity and its application to bioisostere replacement. J. Chem. Inf. Model. 52, 2031–2043. doi: 10.1021/ci3000776
Yan, X., Li, J., Gu, Q., and Xu, J. (2014). gWEGA: GPU-accelerated WEGA for molecular superposition and shape comparison. J. Comput. Chem. 35, 1122–1130. doi: 10.1002/jcc.23603
Yan, X., Liao, C., Liu, Z., Hagler, T. A., Gu, Q., and Xu, J. (2016). Chemical structure similarity search for ligand-based virtual screening: methods and computational resources. Curr. Drug Targets 17, 1580–1585. doi: 10.2174/1389450116666151102095555
Yang, H., Sun, L., Li, W., Liu, G., and Tang, Y. (2018). In silico prediction of chemical toxicity for drug design using machine learning methods and structural alerts. Front. Chem. 6:30. doi: 10.3389/fchem.2018.00030
Yap, C. W. (2011). PaDEL-descriptor: an open source software to calculate molecular descriptors and fingerprints. J. Comput. Chem. 32, 1466–1474. doi: 10.1002/jcc.21707
Yasuo, N., and Sekijima, M. (2019). Improved method of structure-based virtual screening via interaction-energy-based learning. J. Chem. Inf. Model. 59, 1050–1061. doi: 10.1021/acs.jcim.8b00673
Ye, W. L., Zhang, L. X., Guan, Y. D., Xue, W. W., Chen, A. F., Cao, Q., et al. (2019). Virtual screening and experimental validation of eEF2K inhibitors by combining homology modeling, QSAR and molecular docking from FDA approved drugs. New J. Chem. 43, 19097–19106. doi: 10.1039/C9NJ02600B
Zeng, X., Zhang, P., He, W., Qin, C., Chen, S., Tao, L., et al. (2018). NPASS: natural product activity and species source database for natural product research, discovery and tool development. Nucleic Acids Res. 46, D1217–D1222. doi: 10.1093/nar/gkx1026
Zhang, B., Vogt, M., Maggiora, G. M., and Bajorath, J. (2015). Design of chemical space networks using a Tanimoto similarity variant based upon maximum common substructures. J. Comput. Aided Mol. Des. 29, 937–950. doi: 10.1007/s10822-015-9872-1
Zhang, H., Liu, W., Liu, Z., Ju, Y., Xu, M., Zhang, Y., et al. (2018). Discovery of indoleamine 2,3-dioxygenase inhibitors using machine learning based virtual screening. MedChemComm 9, 937–945. doi: 10.1039/C7MD00642J
Zhang, L., Song, J., Kong, L., Yuan, T., Li, W., Zhang, W., et al. (2020). The strategies and techniques of drug discovery from natural products. Pharmacol. Ther. 216:107686. doi: 10.1016/j.pharmthera.2020.107686
Zhang, R., Li, X., Zhang, X., Qin, H., and Xiao, W. (2020). Machine learning approaches for elucidating the biological effects of natural products. Nat. Prod. Rep. 38, 346–361. doi: 10.1039/d0np00043d
Zhang, X., Liu, T., Fan, X., and Ai, N. (2017). In silico modeling on ADME properties of natural products: classification models for blood-brain barrier permeability, its application to traditional Chinese medicine and in vitro experimental validation. J. Mol. Graph. Model. 75, 347–354. doi: 10.1016/j.jmgm.2017.05.021
Zheng, S., Wang, Y., Liu, W., Chang, W., Liang, G., Xu, Y., et al. (2020). In silico prediction of hemolytic toxicity on the human erythrocytes for small molecules by machine-learning and genetic algorithm. J. Med. Chem. 63, 6499–6512. doi: 10.1021/acs.jmedchem.9b00853
Keywords: machine learning, big data, natural products, bioprospecting, cheminformatics, virtual screening, drug discovery, chemical data
Citation: Santana K, do Nascimento LD, Lima e Lima A, Damasceno V, Nahum C, Braga RC and Lameira J (2021) Applications of Virtual Screening in Bioprospecting: Facts, Shifts, and Perspectives to Explore the Chemo-Structural Diversity of Natural Products. Front. Chem. 9:662688. doi: 10.3389/fchem.2021.662688
Received: 01 February 2021; Accepted: 25 February 2021;
Published: 29 April 2021.
Edited by:
Fei Ye, Zhejiang Sci-Tech University, ChinaReviewed by:
José L. Medina-Franco, National Autonomous University of Mexico, MexicoCopyright © 2021 Santana, do Nascimento, Lima e Lima, Damasceno, Nahum, Braga and Lameira. This is an open-access article distributed under the terms of the Creative Commons Attribution License (CC BY). The use, distribution or reproduction in other forums is permitted, provided the original author(s) and the copyright owner(s) are credited and that the original publication in this journal is cited, in accordance with accepted academic practice. No use, distribution or reproduction is permitted which does not comply with these terms.
*Correspondence: Kauê Santana, a2F1ZS5jb3N0YUB1Zm9wYS5lZHUuYnI=
†ORCID: Kauê Santana orcid.org/0000-0002-2735-8016
Lidiane Diniz do Nascimento orcid.org/0000-0003-1370-4472
Anderson Lima e Lima orcid.org/0000-0002-8451-9912
Vinícius Damasceno orcid.org/0000-0003-2263-2124
Rodolpho C. Braga orcid.org/0000-0003-3814-3464
Jerônimo Lameira orcid.org/0000-0001-7270-1517
Disclaimer: All claims expressed in this article are solely those of the authors and do not necessarily represent those of their affiliated organizations, or those of the publisher, the editors and the reviewers. Any product that may be evaluated in this article or claim that may be made by its manufacturer is not guaranteed or endorsed by the publisher.
Research integrity at Frontiers
Learn more about the work of our research integrity team to safeguard the quality of each article we publish.