- 1Center for Life Nano and Neuro Science, Istituto Italiano di Tecnologia (IIT), Roma, Italy
- 2Department of Biochemical Sciences “Alessandro Rossi Fanelli”, Università “Sapienza”, Roma, Italy
- 3Department of Physics, Università “Sapienza”, Roma, Italy
In this study, we focused on the computational analysis of a selected single-point mutation identified by a NGS screening panel in the TET2 enzyme classified as “variant of uncertain clinical significance.” The mutation, namely Q1084P, occurs at the interface between TET2, an important epigenetic regulator, and NANOG, a transcription factor fundamental for hematopoietic cells differentiation. Notably, the mutation occurs in a protein region distant from the active site; moreover, the experimental structures of the interacting region of both proteins are unknown, making it difficult to validate the impact of TET2 mutation on its binding with NANOG. To address these challenges, we employed an integrated computational approach combining molecular docking, molecular dynamics simulations and protein-protein interaction prediction. Our findings indicate that the single-point mutation might effectively reduce the TET2-NANOG interaction, which would consequently impair cells differentiation and hematopoiesis process, consistent with the clinical presentation of pure red cell aplastic anemia. These results, along with the proposed computational method, provide insights for establishing clinical correlations between variants of uncertain significance and anemias in general, comprising common hematological problems widespread in the world population and for which dedicated NGS panels are still not available.
1 Introduction
In contemporary clinical practice, doctors increasingly use Next-Generation Sequencing (NGS) panels to perform comprehensive molecular profiling of somatic mutations from tissue biopsies. Mapping the genomic landscape of these mutations presents challenges, including the need to conduct extensive sequencing projects and compare results with publicly available registries that contain real-world clinical-genomic data. Databases like COSMIC (Tate et al., 2019) and STRING (Szklarczyk et al., 2023) consolidate somatic data from global sources, enabling researchers to access and analyze information about somatic mutations and their implications for many diseases, primarily including cancer (Sondka et al., 2024). The catalogue provides visualizations of mutation prevalence in relation to protein structures, offering insights into how molecular findings correlate with clinical significance, therapeutic options, and potential drug resistance.
Numerous somatic point mutations identified through NGS screenings, nevertheless, affect protein regions distant from active sites or within unstructured protein regions, therefore it is uncertain whether such mutations can be considered driver events that could lead to a loss of function of the protein. Therefore, these mutations are often classified as having “uncertain clinical significance,” which account for about 40% of total variants (Federici and Soddu, 2020). This is particularly true in the context of pathologies unrelated to cancer, for which NGS databases are not yet available. Indeed, single-point mutations that affect cell differentiation are commonly found in hematologic diseases, where clonal expansion of mutated progenitors of the bone marrow populations may lead to serious pathological conditions, spanning from myelodysplastic syndrome to leukemias or lymphomas. The hematopoietic system, especially red blood cell line, exhibits unique self-renewal capabilities and has the highest turnover rate of any cell type in the human body (Sender and Milo, 2021); it is thus particularly prone to expansion of hematopoietic stem and progenitor cells (HSPCs) carrying mutations. Key regulators of cell differentiation include epigenetic factors that influence DNA methylation/demethylation and chromatin remodeling.
Among these players, TET2 enzyme, belonging to TET (ten-eleven translocation) family of proteins, is an important epigenetic regulator responsible for DNA demethylation and cellular differentiation, and is one of the most mutated proteins in hematological malignancies (Delhommeau et al., 2009; Ko et al., 2010; Busque et al., 2012; Huang and Rao, 2014; Coltro et al., 2020; Jiang, 2020; Zhang et al., 2023; Guo et al., 2024). TET proteins, including TET1-3, are dioxygenases that convert 5-methylcytosine (5mC) into 5-hydroxymethylcytosine (5 hmC), 5-formyl cytosine (5fC), and 5-carboxyl cytosine (5caC) (Jiang, 2020; Zhang et al., 2023). This process generates substrates for base excision repair, replacing 5mC with C. TET2 mutations in its catalytic domain are prevalent in pathologies, found in about 10% of de novo acute myeloid leukemia (AML), 30% of myelodysplastic syndrome (MDS), and nearly 50% of chronic myelomonocytic leukemia cases. These mutations are associated with DNA hypermethylation, increased risk of MDS progression, and poor prognosis in AML (Zhang et al., 2023). The COSMIC database (cancer.sanger.ac.uk/cosmic/gene/analysis?ln=TET2) provides insights into various TET2 mutations across thousands of samples, many of them located in its catalytic region (Jiang, 2020; Guo et al., 2024).
However, many TET2 mutations thus identified occur outside its catalytic domain and affect regulatory regions involved in interactions with other proteins. To present a quantitative study of the mutations currently identified in TET2, we conducted an analysis on this aspect. By analyzing the coding point mutations from genome-wide screens (including whole exome sequencing) from the latest COSMIC release (https://cancer.sanger.ac.uk/cosmic), we produced the results shown in Figure 1A. In the pie chart in the top left corner, we show the distribution of mutation types, noting that many do not affect the protein sequence. Concentrating instead on mutations that impact the protein sequence, we present in the bottom distribution the probability of encountering a mutation across the entire sequence. As shown, this probability is distributed throughout the protein, although, as mentioned, mutations occurring in its catalytic domain are much more recognized in pathology. Indeed, looking at the pie chart shown in the top right corner, it is evident that only a minority (23.3%) of mutations (we also considered insertions and deletions) involve the catalytic domain. This result further highlights the urgency of studying the effects of mutations occurring outside this domain, and this work aims to be a first step in that direction. Partner proteins of TET2 are multifold, such as, for example, transcription factors (EBF1, NANOG, SPI1), histone modifiers (HDAC2) or regulatory proteins such as ASXL1, CXXC4 and many others (Zhang et al., 2023). Among these, transcription factors as the homeobox protein NANOG (Chambers et al., 2007; Silva et al., 2009) are responsible for recruiting TET2 to regulate target genes expression, facilitating DNA demethylation and differentiation processes (Costa et al., 2013; Zhang et al., 2023). A representative depiction of the protein-protein interactions involving TET2 can be found in Figure 1B. Here, using the STRING database (https://string-db.org/), we have highlighted the interactome of TET2, depicting known interactions with the highest possible confidence level. As shown and previously mentioned, TET2 is positioned at the center of a complex interaction network, and any impairment in one of these interactions could potentially trigger a cascading effect on cellular functionality. In particular, TET2 binds NANOG outside of its catalytic domain (Costa et al., 2013; Joshi et al., 2022): disruption of this interaction due to single-point mutations in their contact region could thus affect HSPCs differentiation, leading to an impaired hematopoiesis.
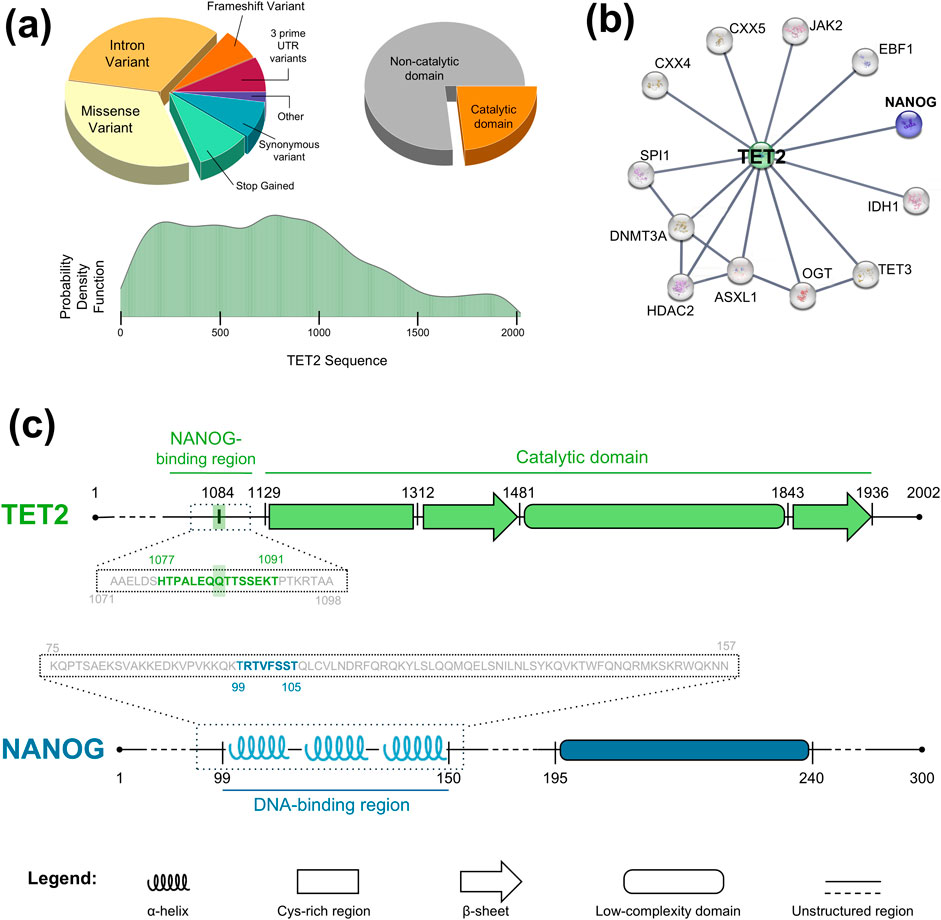
Figure 1. Structures of TET2 and NANOG proteins. (A) Distributions of coding point-mutations of TET2, from genome-wide screens extracted from COSMIC database. Top Left: Pie chart of mutation types. Bottom: Probability density function of encountering mutations for each TET2 residue. Top Right: Pie chart of any kind of mutation that occurs in the catalytic domain of TET2. (B) Interactome of TET2 protein (generated with STRING database) in which the link between TET2 and NANOG is highlighted. (C) Upper panel: Schematic representation of TET2 structure, based on AlphaFold prediction (Jumper et al., 2021) and protein structures deposited on Protein Data Bank (e.g. 4NM6, 5D9Y). TET2 is an important enzyme involved in DNA demethylation, influencing gene expression and contributing to cellular differentiation (Zhang et al., 2023). It is divided in an unstructured part (residues 1–1,128), where the NANOG interaction is known to happen (Costa et al., 2013; Joshi et al., 2022), and a catalytic domain composed by a Cys-rich region (residues 1,129–1,311), two beta-sheets (res. 1,312–1,480 and 1843–1,935) and one low-complexity domain (res. 1,481–1,842). The 1,084 residue of interest belongs to the unstructured part and to the NANOG-binding domain. For molecular dynamics simulations, we extracted a 28 residues-long peptide around 1,084 (dotted box region), from residue 1,071 to 1,098, and then we further narrowed down the peptide of interest around residues 1,077–1,091 (green bold residues, where 1,084 is highlighted). Lower panel: NANOG structure. This is a homeobox protein that maintains pluripotency in embryonic stem cells by suppressing differentiation factors. Its structure comprises a structured part (composed of three alpha-helices, namely H1, H2 and H3, residues 99–149, that bind to DNA), experimentally solved by NMR (PDB code 2KT0) and X-ray crystallography (PDB code 4RBO), a low-complexity domain with unknown structure (residues 195–239) and several unstructured regions. In this study, we assumed that the interaction region of NANOG with TET2 occurs mostly within the structured region (dotted box region); other important transcription factors (as Sox2 (Yesudhas et al., 2019) or Oct4 (Hayashi et al., 2015)) have been experimentally shown to bind here. The 99-105 sequence highlighted in blue is found from the peak of the HybridPBRpred webserver prediction curve (see Figure 3B).
In this study, we focused on a specific clinical case of refractory anemia, classified as Pure Red Cell Aplastic Anemia (PRCAA) where a NGS panel from a bone marrow biopsy identified a Q1084P substitution in TET2, with a heterozygous variant allele frequency (VAF) of 49%. This mutation had been shown to co-occur with mutations in other relevant genes (e.g., ASXL1, RUNX1, SETBP1, NRAS, SRSF2), all related to leukemias or myelodysplastic disorders (Jankowska et al., 2009); here, nevertheless, it was identified as the sole mutation of interest and was therefore classified as of “variant of uncertain clinical significance.” Our study aims to gain new insights into TET2 mutations in the NANOG-interacting region, located outside of TET2 catalytic domain (Joshi et al., 2022) (Figure 1) where, besides Q1084P, at least other 60 mutations have been reported. No structural data on this interaction interface is currently available: AlphaFold (Jumper et al., 2021) predicts TET2 region involved in NANOG interaction as unstructured, and the NANOG region interacting with TET2 remains largely unknown. To address these issues, here we employed a thorough computational approach based on the combination of molecular docking, extensive molecular dynamics, and the prediction of protein-protein interaction probabilities. This allowed to identify the most representative configurations of TET2 and NANOG and to hypothesize a possible binding mode between them, thereby highlighting the molecular mechanisms through which the single-point mutation of interest could impair this association. As such, we potentially identified a molecular etiology that links the clinical onset of anemia to the single-point mutation detected by a NGS panel that is originally dedicated to the detection of somatic mutations of leukemia. We thus present a computational tool that may provide correlations between specific clinical onsets of unknown causes and mutations located in protein regions distant from active sites or within unstructured areas.
2 Materials and methods
2.1 Dataset
The peptide constituting the examined region of TET2 was extracted from the whole structure as modeled by Alphafold2 (Jumper et al., 2021; Varadi et al., 2024). In particular, we selected the residues 1,071–1,098 from the whole protein sequence, narrowing the subsequent analyses to its central part 1,077–1,091 (Figure 1C). We selected this region of the TET2 protein to investigate a linear protein segment centered around the position of the mutation of interest, i.e. Q1084P (shaded green area of Figure 1C), in this study. Additionally, this region of TET2 is known to be involved in the interaction with NANOG. To explore the conformational space accessible to this region, molecular dynamics simulations were performed, from which equilibrium structural conformations were extracted. Peripheral residues (at the ends of a 15-amino-acid segment centered around the mutation, gray residues of Figure 1C) were included in the simulation to mimic the influence of the rest of the protein on the conformations accessible to the central peptide. However, these residues were subsequently excluded from further analyses.
The NANOG NMR structure was downloaded from Protein Data Bank (Berman et al., 2000), labeled with the code 2KT0. Although an X-ray crystallography structure of this protein is available in the PDB (code: 4RBO, Hayashi et al., 2015), we selected the NMR structure because it provides data for a larger number of residues. Specifically, the NMR structure includes residues 75–157, while the X-ray structure only covers residues 94–162.
The modeling of the mutation Q1084P was performed using Pymol (Schrödinger and DeLano, 2020), selecting the lowest energy rotamer for the inserted residue. Therefore, among the rotamers available in terms of the steric hindrance of the structure, we selected the rotamer most frequent in terms of occurrence in protein structures (Desantis et al., 2024; Miotto et al., 2023).
2.2 Molecular dynamics and molecular docking simulations
We used Gromacs 2021 to conduct all molecular dynamics simulations (Abraham et al., 2015). The system topologies were generated using the CHARMM-27 force field (Brooks et al., 2009). Initially, each molecular system underwent energy minimization with the steepest descent algorithm. Following this, thermalization was carried out through sequential simulations in both NVT and NPT ensembles, consisting of two 0.1 ns-long runs with a 2 fs time-step. The system’s temperature was maintained at 300 K using a v-rescale thermostat (Bussi et al., 2007), and pressure was kept constant at 1 bar via the Parrinello-Rahman barostat (Parrinello and Rahman, 1980). A 1.2 nm cut-off was applied for short-range non-bonded interactions, while the Particle Mesh Ewald method (Cheatham et al., 1995) was employed to handle long-range electrostatic interactions. We used the TIP3P model for water molecules (Jorgensen et al., 1983). All the molecular dynamics protocol was already used in our previous works (Di Rienzo et al., 2023a; Parisi et al., 2023). In each simulation, we extracted one frame each 1 ns. The conformations extracted from the molecular dynamics simulation of the peptide derived from TET2 were analyzed using principal component analysis (PCA) on the C-alpha coordinates (see Results). By applying a clustering approach to the first two principal components, we identified the five representative structures of the peptide that best capture the explored conformational space. Similarly, three representative conformations were selected from the 20 provided by the NMR structure of NANOG. The molecular docking simulations were performed using the Hdock webserver (Yan et al., 2020), with standard options. For each combination of the 5 TET2-derived peptide structures and the 3 NANOG structures, we conducted an independent run.
2.3 Statistical analysis
All the analyses of the protein structures and of the molecular dynamics frames were conducted with in-house R script relying on bio3d package (Grant et al., 2006). Root Mean Square Deviation (RMSD) with respect to starting structure is defined as following:
where M is the total mass, mi is the mass of the single atom and ri is its position vector. It has been calculated on the protein backbone atoms after structural alignment on the backbone atoms. Root Mean Square Fluctuations (RMSF) is defined as:
and it has been calculated on all the weighted atoms and averaged per residue.
The intermolecular contact between two residues has been established if the distance between at least one of their couple of atoms is lower than 4 Å (Di Rienzo et al., 2023b).
The Principal Component Analyses (PCA) were performed on the 3D coordinate of the protein α-carbon atom.
3 Results
In this section, we initially present the structural details of the two proteins involved: the enzyme TET2 and the transcription factor NANOG. We selected a 28-residue long peptide from TET2 sequence and we explored its conformational space through molecular dynamics simulation; this region comprises the mutation of interest (Q1084P) and constitutes the part involved in the interaction with NANOG. Later, we analyzed the variability of the NANOG experimental NMR structures, where we hypothesized that the binding would take place. In such a manner, we selected the most representative structures of both molecular partners. We then identified a possible binding mode between NANOG and TET2 by investigating the protein-binding propensity of NANOG using HybridPBRpred webserver (Zhang et al., 2020), through molecular docking simulation and extensive molecular dynamics. Finally, we found that the Q1084P residue substitution would impair a physiological association between proteins TET2 and NANOG, that could correlate with the clinical onset of anemia presented.
3.1 Exploring the conformational space of the TET2-derived peptide and NANOG
TET2 is an enzyme involved in DNA demethylation, influencing gene expression and contributing to cellular differentiation (Zhang et al., 2023). Whereas its experimental structure is not known, AlphaFold (Jumper et al., 2021) predicts it to be divided in an unstructured part (residues 1–1,128), where the NANOG interaction was previously demonstrated (Costa et al., 2013; Joshi et al., 2022), and a catalytic domain composed by a Cys-rich region (residues 1,129–1,311), two beta-sheets (res. 1,312–1,480 and 1843–1935) and one low-complexity domain (res. 1,481–1842) (Figure 1C). The 1,084 residue of interest belongs to the unstructured part and to the NANOG-binding domain; interestingly, this region is highly conserved among species, indicating its significant importance.
In order to extrapolate the most representative structure assumed by this specific region, we extracted a 28-residues long peptide centered in residue 1,084 of TET2 (dotted box of Figure 1C) and we performed a 150-ns long molecular dynamics simulation of such a system. The obtained results are reported in Figure 2. The Root Mean Square Deviation (RMSD) shown by the simulation with respect to the initial configuration highlights, after the initial equilibration, a substantial stability of the simulation (Figure 2A). Moreover, we calculated the Root Mean Square Fluctuations (RMSF) for each residue as an indicator of the residue motility with respect to an average position (Figure 2B). In general, our results show that the peptide is characterized by quite high mobility, as expected. Peripheral residues (red bars in Figure 2B), displaying the highest RMSF values, have been removed from the structure. Indeed, we wanted to study solely the central residues, but we retained the lateral residues in the simulation to preserve, to some extent, the influence that the rest of the structure has on the peptide’s conformations. Therefore, our focus was on the central 15-amino-acid region around position 1,084 (green bar in Figure 2B, corresponding to residues 1,077–1,091).
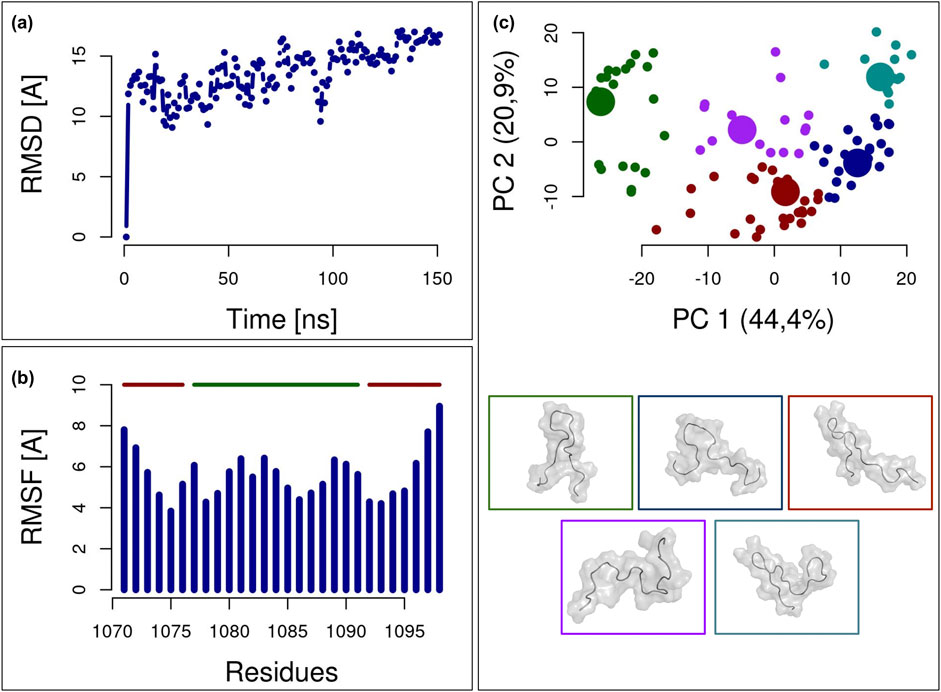
Figure 2. Analysis of the molecular dynamics simulation of the TET2-derived peptide. (A) Root Mean Square Deviation (RMSD) of each frame of the simulation relative to the initial configuration. The vertical dashed line marks the beginning of the equilibrium range, from which peptide structures are extracted for analysis. (B) Root Mean Square Fluctuation (RMSF) of each residue during the equilibrium time interval. The 15-residue-long central peptide is highlighted with a solid green line at the top, while the side residues are highlighted with a solid red line at the top. Additional side residues are included to simulate the presence of the entire protein during the exploration of the configuration space. (C) Scatter plot of the equilibrium frames in the space of the two principal components as determined by Principal Component Analysis (PCA) of the atom coordinate during the simulation. The frames are clustered into five groups, each labelled with a specific color. The centroid of each cluster, represented by a larger point, is depicted with a molecular image in the lower part of this panel.
After selecting the central 15 residues and the frames following system equilibration, a principal component analysis (PCA) was performed on the protein’s C-alpha coordinates. The conformational space explored by the peptide during the molecular dynamics simulation is illustrated in Figure 2C, where each frame is depicted within the space defined by the two principal components. To identify the most representative structures of the peptide conformations during the simulation, we performed k-means clustering: this approach allowed us to divide the simulation frames into five groups and select the centroids of each cluster. These centroid structures represent the set of possible conformations the peptide can adopt in water and were subsequently used for docking with the partner protein NANOG. Molecular representations of these five structures are also shown in the lower panel of Figure 2C.
NANOG is a transcription factor that maintains pluripotency in embryonic stem cells by suppressing differentiation factors (Chambers et al., 2007; Silva et al., 2009). Its structure comprises a structured part (composed of three alpha-helices, namely H1, H2 and H3, residues 99–149), a low-complexity domain with an unknown structure (residues 195–239) and several unstructured regions, as shown in Figure 1C. The structured part is responsible for DNA interaction, as the helices H2 and H3 form a helix–turn–helix motif. H3 is the recognition helix, inserted in the major groove of DNA, and is responsible for bases recognition, whereas additional base contacts are formed by the N-terminus that reaches into the minor groove. The region comprising H1 and H2 has been observed to be the one involved in binding other important transcription factors such as Sox2 (Yesudhas et al., 2019) or Oct4 (Hayashi et al., 2015): thus, the region comprising H1 and H2 might be the most likely structural determinant for interaction with TET2. Hence, we hypothesized that the interaction with TET2 occurs within the H1-H2 structured region (dotted box of Figure 1C), experimentally determined by NMR and available in the PDB (code 2KT0). To generate a set of structures for docking analysis, we performed a Principal Component Analysis (PCA) on the C-alpha coordinates obtained from the NMR experiment (Figure 3A). Each of these 20 structures was then projected into the space defined by the first two principal components. A k-means clustering into three groups was subsequently applied to identify the centroid structures that were then used for the subsequent docking analysis.
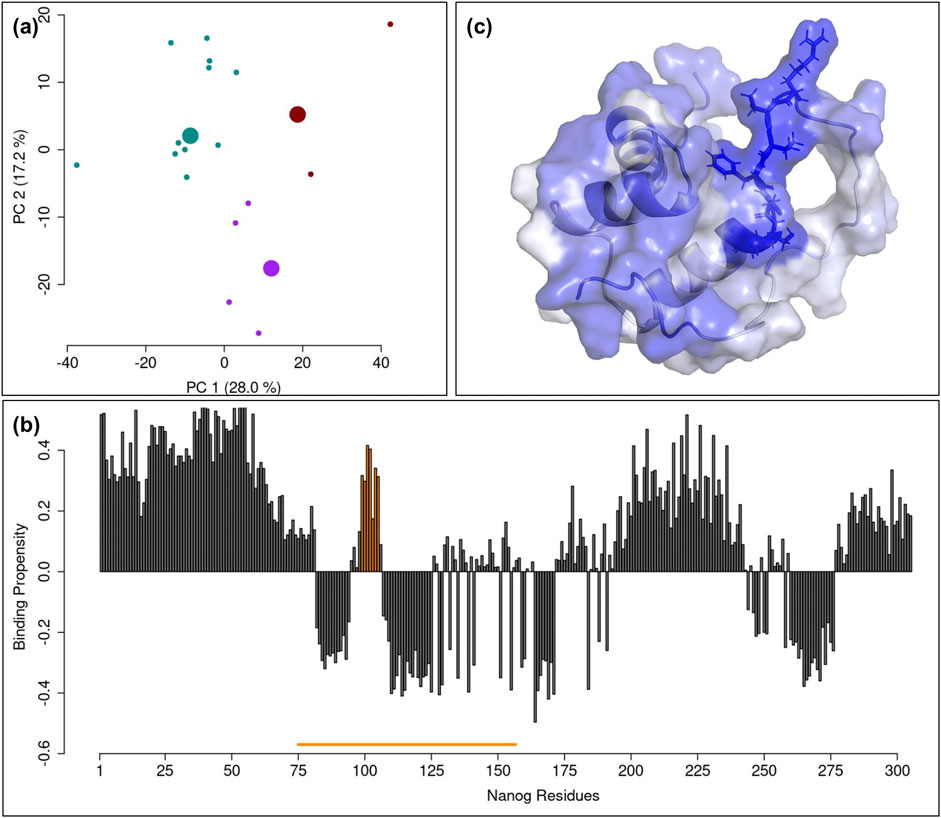
Figure 3. Analysis of NANOG NMR structure. (A) Scatter plot of the NMR frames in the space of the two principal components as determined by Principal Component Analysis (PCA) of the atom coordinate in the various NMR conformation. The 20 frames are clustered into three groups, each labelled with a specific color. The centroid of each cluster is represented by a larger point. (B) Barplot of the protein-protein binding propensity regarding each NANOG residue, as predicted by HybridPBRpred webserver. The solid orange line in the bottom represents the fraction of NANOG structure experimentally determined in NMR. The peak highlighted in orange corresponds to the binding propensity bars of the residues of NANOG taken into consideration (positions 99–105). (C) Molecular representation of the NMR NANOG structure: each residue is colored according to the score reported in (B). The blue dotted box is centered on the interacting segment of NANOG and the side chains of the residues are visible.
The use of the HybridPBRpred software (Zhang et al., 2020), allowed us to identify the regions of NANOG most likely to interact with other proteins. This software, which combines predictions for both ordered and disordered regions of proteins, provided the results shown in the bar plot in Figure 3B, where the binding propensity value for each residue is displayed. Notably, when focusing on the structured region, highlighted by a yellow line underneath, there is a pronounced propensity peak corresponding to residues 99–105 of NANOG. Indeed, the molecular representation in Figure 3C shows the structured part of NANOG protein, colored according to the binding propensity as predicted by HybridPBRpred.
3.2 Molecular docking and molecular dynamics simulations: hypothesizing the structural details of the TET2-NANOG interactions
After selecting the five structures of the TET2-derived peptide and the three structures of NANOG, we carried out a docking analysis to identify a plausible binding conformation between the two proteins. We utilized the Hdock webserver (Yan et al., 2020) to simulate the interaction between each peptide structure and each NANOG configuration, resulting in 15 docking simulations. From these, we chose the top 10 poses from each simulation, leading to a total of 150 potential docking poses. Each of these predicted complexes was then analyzed in detail to filter out any poses that did not meet all the necessary criteria for interaction. First, we examined the scores predicted by Hdock. The distribution of these scores is depicted by the cyan curve in Figure 4A, where it should be noted that a lower score indicates a stronger interaction. Consequently, we selected only those poses with scores below −175. The choice of the threshold is based on the value of the top quartile of the probability distribution shown in Figure 4A (specifically −174.03). In this way we ensured the selection of the docking poses that belong to the top 25% of poses with the best Hdock scores. Thereafter, we analyzed how many of the NANOG residues with a high binding propensity, as defined in the previous section, were involved in the interaction. Specifically, for each pose, we examined the percentage of residues 99–105 that were in contact with the peptide. The distribution of these percentages is shown in Figure 4B. Again, we filtered out poses where less than 43% of these key residues were engaged in the interaction, selecting the top 25% of poses with the highest number of NANOG residues in contact. Lastly, we examined how many of the 15 residues forming the core of the peptide were interacting with NANOG (See Figure 4C). In this case, we required that at least 67% of the peptide residues be involved in the interaction, filtering out any poses that did not meet this criterion. Also here, this choice was made in order to select the top 25% of poses with the highest number of selected TET2 residues in contact. Among the 150 poses evaluated, only one met all these conditions, and this pose was subsequently selected as the potential binding mode between these two proteins. Thus, this entire computational protocol led us to the construction of an interaction complex between these two proteins.
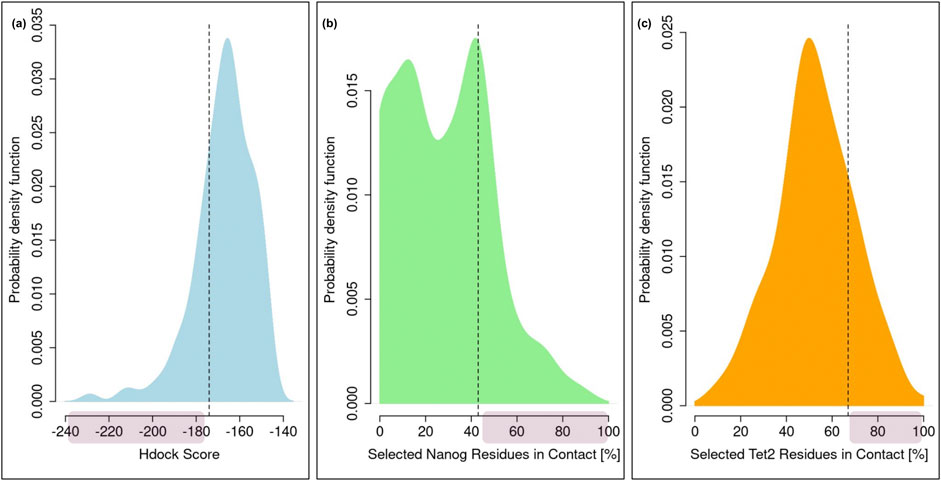
Figure 4. Analysis of the TET2-NANOG Molecular Docking. (A) Probability distribution of the Hdock scores characterizing all the docking poses. (B) Probability distribution of the percentage of high-propensity NANOG residues involved in each docking pose. (C) Probability distribution of the percentage of central peptide residues involved in each docking pose. In all the three panels, a red shaded area indicates the region where the poses are extracted.
As previously mentioned, the next question we addressed was whether the Q1084P mutation could impair this physiological interaction. We thus conducted extensive molecular dynamics simulations (1 µs-long each) using the docking complex as starting structure for both the wild-type and mutated forms of TET2 in order to single out possible significant variations. The results of the analyses conducted on the two molecular dynamics simulations are presented in Figure 5. Specifically, panel 5.a shows the time evolution of the RMSD for both simulations, highlighting that both simulations reach an overall stability, even if the mutant exhibits a higher variability with respect to the starting structure. Notably, the WT complex shows greater stability than the mutated complex in terms of both the number of intermolecular contacts and the compactness at the interface (Figures 5B–D). In particular, panel 5.b displays the distribution of the number of contacts in each equilibrium frame for both simulations. It is evident that the green curve (WT) is statistically higher than the red curve (mutated), indicating a stronger interconnection at the interface. Interestingly, part of this difference can be directly attributed to the behavior of residue 1,084 at the interface. As shown in panel 5.c, the distribution of intermolecular contacts involving residue 1,084 reveals that, when a glutamine is substituted with a proline in this position, the number of contacts made by this residue is significantly lower compared to the WT case. This reduction in the number of contacts likely weakens the binding strength. This hypothesis is further supported by the observation that the two molecular partners are farther apart in the mutated complex during the simulation. Specifically, panel 5.d, which presents the distribution of distances between the centroids of the two molecular partners in each frame, clearly shows that the WT complex is characterized by a shorter distance (∼1.5 nm) compared to the mutated complex (∼2.3 nm).
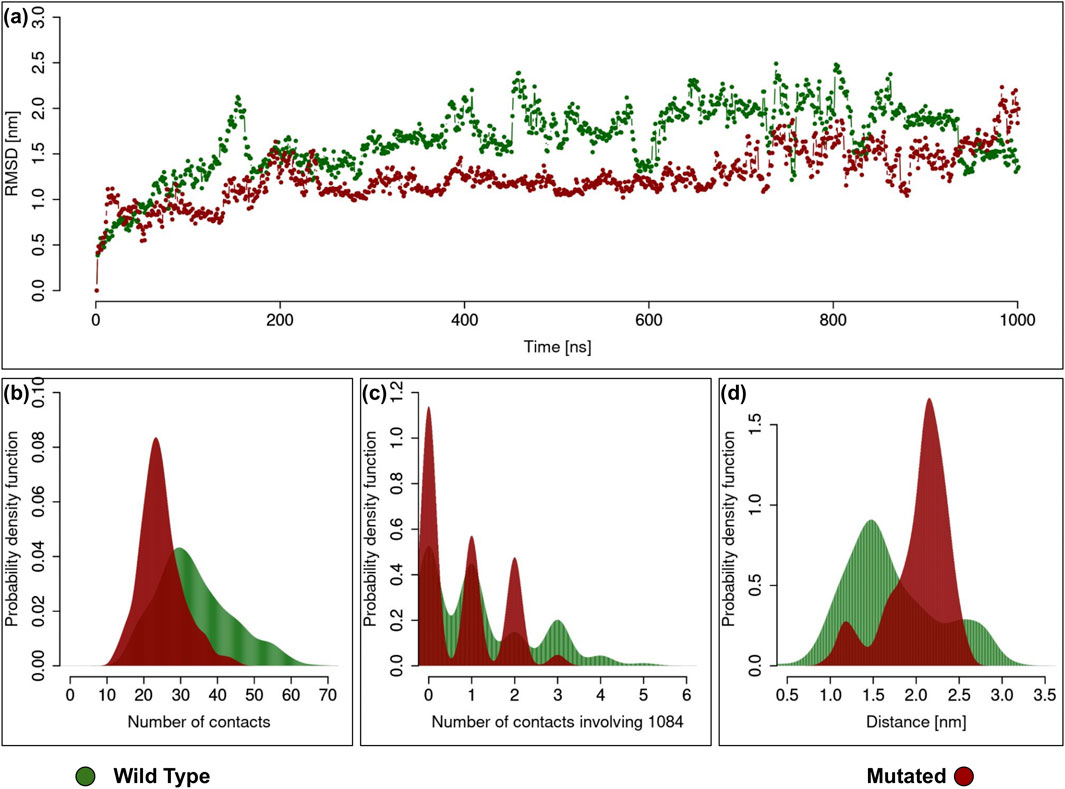
Figure 5. Analysis of the TET2-NANOG Complex Molecular Dynamics Simulation. In all the plots the green curve and the red curve regard the simulation of the wild type and mutated version of TET2, respectively. (A) Root Mean Square Deviation (RMSD) of each frame of the simulation relative to the initial configuration, both for wild type and mutated TET2. (B) Probability distribution of the number of intermolecular contacts between the two proteins, both for wild type and mutated version of TET2. (C) Probability distribution of the number of intermolecular contacts between the two proteins involving 1,084, both for wild type and mutated version of TET2. (D) Probability distribution of the distance between the center of mass of the two proteins, both for wild type and mutated version of TET2.
Taken together, these findings suggest that the Q1084P mutation may destabilize the TET2-NANOG complex, potentially resulting in impaired TET2 enzyme activity.
4 Discussion and conclusion
The aim of this study was to explore the binding between TET2 and NANOG proteins by computational methods, starting from results of a NGS screening on a clinical case of refractory anemia, classified as pure red cell aplastic anemia on histopathological basis. The screening spotted an isolated missense substitution (c.3251 A > C) leading to Q1084P mutation on TET2 protein in a region demonstrated to interact with NANOG transcription factor (Costa et al., 2013; Joshi et al., 2022). In the lack of structural data that may confirm a direct cause to effect relationship that explains the pathological effect of the mutation, we combined molecular docking, molecular dynamics simulations and protein-protein interaction prediction in order to demonstrate the possible destabilization of TET2-NANOG interface as a consequence of the mutation.
Residue 1,084 is located outside of the TET2 catalytic domain (shown in Figure 1C) and belongs to an unstructured region. We performed a 150-ns molecular dynamics simulation on the TET2 peptide centered around 1,084 (Figure 2) and performed principal component analysis on its C-alpha coordinates, thus finding the conformational space explored by the peptide and identifying the five most representative structures of the peptide through k-means clustering (Figure 2C). For NANOG, we hypothesized that its binding region belonged to the structured domain (Figure 1), a region already exploited by other transcription factors, and whose experimental structure is known from NMR (PDB code 2KT0) and X-ray crystallography (PDB code 4RBO). While this could represent a major limitation of the present work, the HybridPBRpred software showed a peak in binding propensity with other proteins in this region (Figure 3B): this assumption thus appeared to be justified. Again, we performed a PCA on C-alpha coordinates of this region and used k-means to find the three most representative structures of the NANOG binding domain.
By using the Hdock webserver (Yan et al., 2020), we then performed the molecular docking between the five structures of TET2 peptide and three structures of NANOG to identify a plausible binding conformation between the two. Amongst the possible 150 docking simulations, we filtered one conformation with the highest binding propensity, thus finding the putative docking complex (Figure 4). We finally performed 1 µs-long molecular dynamics simulations of the docking complex for both the wild-type and mutated forms (Q1084P) of TET2 (Figure 5A). We found that the WT complex shows greater stability than the mutated complex in terms of both the number of intermolecular contacts and the compactness at the interface (Figures 5B–D): both distributions indicate that the number of contacts between the wild-type protein and NANOG is higher compared to the mutated protein. These results suggest that the Q1084P mutation in TET2 significantly hinders its interaction with NANOG. This assumption is further supported by the fact that the amino acids involved have distinct chemical and structural properties: proline is a unique amino acid known for disrupting secondary structures such as alpha-helices due to its cyclic ring, which restricts peptide chain flexibility; in contrast, glutamine is more flexible and polar, with a side chain capable of forming hydrogen bonds. Replacing glutamine with proline results in the loss of two hydrogen bonds, with an energetic cost of approximately 3 kcal/mol. This alteration can significantly impact the local protein structure, especially in alpha-helices or flexible regions, which in turn may affect its function and stability.
The consequence of such a mutation is likely to interfere with the proper interaction of the transcription factor NANOG with TET2. Transcription factors like the homeobox protein NANOG bind to TET2 to regulate target gene expression, thus enabling cell differentiation processes: disruption of this interaction due to single-point mutations in their contact region could therefore affect HSPCs differentiation, leading to an impairment of the hematopoiesis process due to the clonal expansion of cells carrying this TET2 somatic mutation.
On these bases, we hypothesized that the reported mutation can be linked to the observed refractory anemia (or PRCAA) in the clinical case of interest, which involved the single-point mutation Q1084P of “uncertain clinical significance,” as classified by a NGS panel dedicated to the screening of possible somatic mutations at the origin of leukemias. Possible limitations of this work include the hypothesis that the contact region between TET2 and NANOG lies in the H1-H2 zone of NANOG (a hypothesis, however, that seems plausible as this region is the one recognized by other important transcription factors (Yesudhas et al., 2019; Hayashi et al., 2015)), and that Q1084P mutation of TET2 would play an important role in TET2-NANOG binding, an assumption that may not be true for other variants for which, however, correspondence to clinical had not been found yet.
To date, a deep investigation of potential clinical correlations between TET2 mutations and hematological diseases from a molecular interaction analysis perspective has not yet been explored. Such an approach could significantly advance our understanding of the cause-and-effect relationships underlying hematological disorders, as NGS often provides information that is not immediately linked to structural determinants of the target protein. The refractory anemia (PRCAA) observed in the clinical case here reported falls within this category of diseases and the current analysis could be potentially expanded to many anemias of unknown origin, which are widespread in the global population, with only a small percentage being diagnosed at the molecular level (Kassebaum et al., 2014; Chaparro and Suchdev, 2019; Sankar and Oviya, 2024).
In summary, our current computational analyses pave the way for the development of a novel computational tool aimed at facilitating experimental validation and uncovering potential correlations between single-point mutations in proteins—particularly those in regions far from active sites or within unstructured domains—and specific clinical outcomes of unclear origin. In other words, such computational analysis could be carried out or even implemented in current NGS database analysis in order to suggest the clinicians novel diagnostic pathways within the group of “uncertain clinical significance” cases. Looking ahead, future efforts will focus on experimentally investigating the interactions between NANOG and TET2, which may affirm our computational predictions. Expanding this approach to include systematic analyses of NGS databases could significantly enhance our understanding, bridging observed mutations in somatic cell lines with specific clinical scenarios by keeping in mind that there are variants that are not necessarily related to malignant transformations but lead to severe function impairment. This integration could be further augmented by AI methodologies, driving deeper insights and connections in the field.
Data availability statement
The raw data supporting the conclusions of this article will be made available by the authors, without undue reservation.
Ethics statement
Written informed consent was obtained from the individual(s) for the publication of any potentially identifiable images or data included in this article.
Author contributions
CT: Conceptualization, Data curation, Investigation, Visualization, Writing–original draft, Writing–review and editing. RP: Data curation, Visualization, Writing–original draft, Writing–review and editing. AP: Data curation, Writing–original draft. CB: Data curation, Writing–original draft. DL: Data curation, Writing–original draft. DP: Data curation, Writing–original draft. EP: Data curation, Writing–original draft. FS: Data curation, Writing–original draft. IC: Data curation, Writing–original draft. MA: Data curation, Writing–original draft. MI: Data curation, Writing–original draft. AB: Conceptualization, Funding acquisition, Supervision, Writing–original draft, Writing–review and editing. LD: Conceptualization, Data curation, Formal Analysis, Investigation, Methodology, Visualization, Writing–original draft, Writing–review and editing.
Funding
The author(s) declare financial support was received for the research, authorship, and/or publication of this article. This research was founded by grants from ERC-2019-Synergy Grant (ASTRA, n. 855923), from EIC-2022-PathfinderOpen (ivBM-4PAP, n. 101098989) and from Project “National Center for Gene Therapy and Drugs based on RNA Technology” (CN00000041) financed by NextGeneration EU PNRR MUR-M4C2-Action 1.4- Call “Potenziamento strutture di ricerca e creazione di “campioni nazionali di R&S,” CUP J33C22001130001 (to CT); from the National Center for Gene Therapy and Drugs Based on RNA Technology, funded in the framework of the National Recovery and Resilience Plan (NRRP), Mission 4 “Education and Research,” Component 2 “From Research to Business,” Investment 1.4 “Strengthening research structures for supporting the creation of National Centres, national R&D leaders on some Key Enabling Technologies,” funded by the European Union - Next Generation EU, Project CN00000041, CUP B93D21010860004 (to RP).
Conflict of interest
The authors declare that the research was conducted in the absence of any commercial or financial relationships that could be construed as a potential conflict of interest.
Generative AI statement
The author(s) declare that no Generative AI was used in the creation of this manuscript.
Publisher’s note
All claims expressed in this article are solely those of the authors and do not necessarily represent those of their affiliated organizations, or those of the publisher, the editors and the reviewers. Any product that may be evaluated in this article, or claim that may be made by its manufacturer, is not guaranteed or endorsed by the publisher.
References
Abraham, M. J., Murtola, T., Schulz, R., Páll, S., Smith, J. C., Hess, B., et al. (2015). GROMACS: high performance molecular simulations through multi-level parallelism from laptops to supercomputers. SoftwareX 1–2, 19–25. doi:10.1016/j.softx.2015.06.001
Berman, H. M., Westbrook, J., Feng, Z., Gilliland, G., Bhat, T. N., Weissig, H., et al. (2000). The protein Data Bank. Nucleic Acids Res. 28, 235–242. doi:10.1093/nar/28.1.235
Brooks, B. R., Brooks, C. L., Mackerell, A. D., Nilsson, L., Petrella, R. J., Roux, B., et al. (2009). CHARMM: the biomolecular simulation program. J. Comput. Chem. 30, 1545–1614. doi:10.1002/jcc.21287
Busque, L., Patel, J. P., Figueroa, M. E., Vasanthakumar, A., Provost, S., Hamilou, Z., et al. (2012). Recurrent somatic TET2 mutations in normal elderly individuals with clonal hematopoiesis. Nat. Genet. 44, 1179–1181. doi:10.1038/ng.2413
Bussi, G., Donadio, D., and Parrinello, M. (2007). Canonical sampling through velocity rescaling. J. Chem. Phys. 126, 014101. doi:10.1063/1.2408420
Chambers, I., Silva, J., Colby, D., Nichols, J., Nijmeijer, B., Robertson, M., et al. (2007). Nanog safeguards pluripotency and mediates germline development. Nature 450, 1230–1234. doi:10.1038/nature06403
Chaparro, C. M., and Suchdev, P. S. (2019). Anemia epidemiology, pathophysiology, and etiology in low- and middle-income countries. Ann. N. Y. Acad. Sci. 1450, 15–31. doi:10.1111/nyas.14092
Cheatham, T. E. I., Miller, J. L., Fox, T., Darden, T. A., and Kollman, P. A. (1995). Molecular dynamics simulations on solvated biomolecular systems: the Particle Mesh Ewald method leads to stable trajectories of DNA, RNA, and proteins. J. Am. Chem. Soc. 117, 4193–4194. doi:10.1021/ja00119a045
Coltro, G., Mangaonkar, A. A., Lasho, T. L., Finke, C. M., Pophali, P., Carr, R., et al. (2020). Clinical, molecular, and prognostic correlates of number, type, and functional localization of TET2 mutations in chronic myelomonocytic leukemia (CMML)—a study of 1084 patients. Leukemia 34, 1407–1421. doi:10.1038/s41375-019-0690-7
Costa, Y., Ding, J., Theunissen, T. W., Faiola, F., Hore, T. A., Shliaha, P. V., et al. (2013). NANOG-dependent function of TET1 and TET2 in establishment of pluripotency. Nature 495, 370–374. doi:10.1038/nature11925
Delhommeau, F., Dupont, S., Valle, V. D., James, C., Trannoy, S., Massé, A., et al. (2009). Mutation in TET2 in myeloid cancers. N. Engl. J. Med. 360, 2289–2301. doi:10.1056/NEJMoa0810069
Desantis, F., Miotto, M., Milanetti, E., Ruocco, G., and Di Rienzo, L. (2024). Computational evidences of a misfolding event in an aggregation-prone light chain preceding the formation of the non-native pathogenic dimer. Proteins 92 (7), 797–807. doi:10.1002/prot.26672
Di Rienzo, L., Miotto, M., Desantis, F., Grassmann, G., Ruocco, G., and Milanetti, E. (2023a). Dynamical changes of SARS-CoV-2 spike variants in the highly immunogenic regions impact the viral antibodies escaping. Proteins Struct. Funct. Bioinforma. 91, 1116–1129. doi:10.1002/prot.26497
Di Rienzo, L., Miotto, M., Milanetti, E., and Ruocco, G. (2023b). Computational structural-based GPCR optimization for user-defined ligand: implications for the development of biosensors. Comput. Struct. Biotechnol. J. 21, 3002–3009. doi:10.1016/j.csbj.2023.05.004
Federici, G., and Soddu, S. (2020). Variants of uncertain significance in the era of high-throughput genome sequencing: a lesson from breast and ovary cancers. J. Exp. and Clin. Cancer Res. 39, 46. doi:10.1186/s13046-020-01554-6
Grant, B. J., Rodrigues, A. P. C., ElSawy, K. M., McCammon, J. A., and Caves, L. S. D. (2006). Bio3d: an R package for the comparative analysis of protein structures. Bioinformatics 22, 2695–2696. doi:10.1093/bioinformatics/btl461
Guo, L., Hong, T., Lee, Y.-T., Hu, X., Pan, G., Zhao, R., et al. (2024). Perturbing TET2 condensation promotes aberrant genome-wide DNA methylation and curtails leukaemia cell growth. Nat. Cell Biol. 26, 2154–2167. doi:10.1038/s41556-024-01496-7
Hayashi, Y., Caboni, L., Das, D., Yumoto, F., Clayton, T., Deller, M. C., et al. (2015). Structure-based discovery of NANOG variant with enhanced properties to promote self-renewal and reprogramming of pluripotent stem cells. Proc. Natl. Acad. Sci. 112 (15), 4666–4671. doi:10.1073/pnas.1502855112
Huang, Y., and Rao, A. (2014). Connections between TET proteins and aberrant DNA modification in cancer. Trends Genet. 30, 464–474. doi:10.1016/j.tig.2014.07.005
Jankowska, A. M., Szpurka, H., Tiu, R. V., Makishima, H., Afable, M., Huh, J., et al. (2009). Loss of heterozygosity 4q24 and TET2 mutations associated with myelodysplastic/myeloproliferative neoplasms. Blood 113, 6403–6410. doi:10.1182/blood-2009-02-205690
Jiang, S. (2020). Tet2 at the interface between cancer and immunity. Commun. Biol. 3, 667. doi:10.1038/s42003-020-01391-5
Jorgensen, W. L., Chandrasekhar, J., Madura, J. D., Impey, R. W., and Klein, M. L. (1983). Comparison of simple potential functions for simulating liquid water. J. Chem. Phys. 79, 926–935. doi:10.1063/1.445869
Joshi, K., Liu, S., Breslin Sj, P., and Zhang, J. (2022). Mechanisms that regulate the activities of TET proteins. Cell. Mol. Life Sci. 79, 363. doi:10.1007/s00018-022-04396-x
Jumper, J., Evans, R., Pritzel, A., Green, T., Figurnov, M., Ronneberger, O., et al. (2021). Highly accurate protein structure prediction with AlphaFold. Nature 596, 583–589. doi:10.1038/s41586-021-03819-2
Kassebaum, N. J., Jasrasaria, R., Naghavi, M., Wulf, S. K., Johns, N., Lozano, R., et al. (2014). A systematic analysis of global anemia burden from 1990 to 2010. Blood 123, 615–624. doi:10.1182/blood-2013-06-508325
Ko, M., Huang, Y., Jankowska, A. M., Pape, U. J., Tahiliani, M., Bandukwala, H. S., et al. (2010). Impaired hydroxylation of 5-methylcytosine in myeloid cancers with mutant TET2. Nature 468, 839–843. doi:10.1038/nature09586
Miotto, M., Di Rienzo, L., Grassmann, G., Desantis, F., Cidonio, G., Gosti, G., et al. (2023). Differences in the organization of interface residues tunes the stability of the SARS-CoV-2 spike-ACE2 complex. Front. Mol. Biosci. 10, 1205919. doi:10.3389/fmolb.2023.1205919
Parisi, G., Piacentini, R., Incocciati, A., Bonamore, A., Macone, A., Rupert, J., et al. (2023). Design of protein-binding peptides with controlled binding affinity: the case of SARS-CoV-2 receptor binding domain and angiotensin-converting enzyme 2 derived peptides. Front. Mol. Biosci. 10, 1332359. doi:10.3389/fmolb.2023.1332359
Parrinello, M., and Rahman, A. (1980). Crystal structure and pair potentials: a molecular-dynamics study. Phys. Rev. Lett. 45, 1196–1199. doi:10.1103/PhysRevLett.45.1196
Sankar, D., and Oviya, I. (2024). Multidisciplinary approaches to study anaemia with special mention on aplastic anaemia (Review). Int. J. Mol. Med. 54, 95. doi:10.3892/ijmm.2024.5419
Schrödinger, L., and DeLano, W. (2020). PyMOL. Available at: http://www.pymol.org/pymol.
Sender, R., and Milo, R. (2021). The distribution of cellular turnover in the human body. Nat. Med. 27, 45–48. doi:10.1038/s41591-020-01182-9
Silva, J., Nichols, J., Theunissen, T. W., Guo, G., van Oosten, A. L., Barrandon, O., et al. (2009). Nanog is the gateway to the pluripotent ground state. Cell 138, 722–737. doi:10.1016/j.cell.2009.07.039
Sondka, Z., Dhir, N. B., Carvalho-Silva, D., Jupe, S., Madhumita, , McLaren, K., et al. (2024). COSMIC: a curated database of somatic variants and clinical data for cancer. Nucleic Acids Res. 52, D1210–D1217. doi:10.1093/nar/gkad986
Szklarczyk, D., Kirsch, R., Koutrouli, M., Nastou, K., Mehryary, F., Hachilif, R., et al. (2023). The STRING database in 2023: protein–protein association networks and functional enrichment analyses for any sequenced genome of interest. Nucleic Acids Res. 51 (D1), D638–D646. doi:10.1093/nar/gkac1000
Tate, J. G., Bamford, S., Jubb, H. C., Sondka, Z., Beare, D. M., Bindal, N., et al. (2019). COSMIC: the catalogue of somatic mutations in cancer. Nucleic Acids Res. 47, D941–D947. doi:10.1093/nar/gky1015
Varadi, M., Bertoni, D., Magana, P., Paramval, U., Pidruchna, I., Radhakrishnan, M., et al. (2024). AlphaFold Protein Structure Database in 2024: providing structure coverage for over 214 million protein sequences. Nucleic Acids Res. 52, D368–D375. doi:10.1093/nar/gkad1011
Yan, Y., Tao, H., He, J., and Huang, S.-Y. (2020). The HDOCK server for integrated protein–protein docking. Nat. Protoc. 15, 1829–1852. doi:10.1038/s41596-020-0312-x
Yesudhas, D., Anwar, M. A., and Choi, S. (2019). Structural mechanism of DNA-mediated Nanog–Sox2 cooperative interaction. RSC Adv. 9 (14), 8121–8130. doi:10.1039/C8RA10085C
Zhang, J., Ghadermarzi, S., and Kurgan, L. (2020). Prediction of protein-binding residues: dichotomy of sequence-based methods developed using structured complexes versus disordered proteins. Bioinformatics 36, 4729–4738. doi:10.1093/bioinformatics/btaa573
Keywords: anemia, TET2, nanog, single-point mutations, molecular dynamics, molecular docking, protein-protein binding
Citation: Testi C, Piacentini R, Perrone A, Bartoli C, Leso D, Pavia D, Pistolesi E, Scipione F, Cotronea I, Adinolfi Falcone M, Ierani M, Boffi A and Di Rienzo L (2025) Exploring the NANOG-TET2 interaction interface. Effects of a selected mutation and hypothesis on the clinical correlation with anemias. Front. Chem. Biol. 3:1517163. doi: 10.3389/fchbi.2024.1517163
Received: 25 October 2024; Accepted: 16 December 2024;
Published: 06 January 2025.
Edited by:
Andrea Ilari, National Research Council (CNR), ItalyReviewed by:
Vikash Kumar Dubey, Indian Institute of Technology (BHU), IndiaPatrizio Di Micco, National Research Council (CNR), Italy
Copyright © 2025 Testi, Piacentini, Perrone, Bartoli, Leso, Pavia, Pistolesi, Scipione, Cotronea, Adinolfi Falcone, Ierani, Boffi and Di Rienzo. This is an open-access article distributed under the terms of the Creative Commons Attribution License (CC BY). The use, distribution or reproduction in other forums is permitted, provided the original author(s) and the copyright owner(s) are credited and that the original publication in this journal is cited, in accordance with accepted academic practice. No use, distribution or reproduction is permitted which does not comply with these terms.
*Correspondence: Claudia Testi, Y2xhdWRpYS50ZXN0aUBpaXQuaXQ=; Roberta Piacentini, cm9iZXJ0YS5waWFjZW50aW5pQHVuaXJvbWExLml0; Lorenzo Di Rienzo, bG9yZW56by5kaXJpZW56b0BpaXQuaXQ=