- 1Chemical Development, Boehringer Ingelheim, Ridgefield, CT, United States
- 2Biotherapeutics Department, Boehringer Ingelheim, Ridgefield, CT, United States
- 3Medicinal Chemistry Department, Boehringer Ingelheim, Biberach, Germany
- 4Institute of Pharmacy, Christian-Albrechts-University of Kiel, Kiel, Germany
- 5Zymvol., Barcelona, Spain
- 6Gecco Biotech, Groningen, Netherlands
Introduction: Biocatalysis, particularly through engineered enzymes, presents a cost-effective, efficient, and eco-friendly approach to compound synthesis. We sought to identify ketoreductases capable of synthesizing optically pure alcohols or ketones, essential chiral building blocks for active pharmaceutical ingredients.
Methods: Using BioMatchMaker®, an in silico high-throughput platform that allows the identification of wild-type enzyme sequences for a desired chemical transformation, we identified a bacterial SDR ketoreductase from Thermus caliditerrae, Tcalid SDR, that demonstrates favorable reaction efficiency and desired enantiomeric excess.
Results: Here we present two crystal structures of the Tcalid SDR in an apo-form at 1.9 Å and NADP-complexed form at 1.7 Å resolution (9FE6 and 9FEB, respectively). This enzyme forms a homotetramer with each subunit containing an N-terminal Rossmann-fold domain. We use computational analysis combined with site-directed mutagenesis and enzymatic characterization to define the substrate-binding pocket. Furthermore, the enzyme retained favorable reactivity and selectivity after incubation at elevated temperature.
Conclusion: The enantioselectivity combined with the thermostability of Tcalid SDR makes this enzyme an attractive engineering starting point for biocatalysis applications.
Introduction
Biocatalysts, often in the form of carefully engineered enzymes, can make active pharmaceutical ingredient (API) synthesis more ecologically friendly, cost-effective and reduce the number of synthetic steps (Devine et al., 2018; Woodley, 2019; Wu et al., 2020; Buller et al., 2023). Despite clear advantages, broad implementation of enzymatic catalysis remains a challenge due to ambitious project timelines and resource constraints. In addition, time and cost constraints influence the degree of investment in protein engineering at early stages of development. These challenges are amplified at later stages where large scale manufacturing considerations come into play (France et al., 2023). Cost-effective methods to identify and engineer wild-type (WT) enzymes earlier reduce time pressure and ultimately enable broader implementation of biocatalysts. Computational approaches offer one option to expedite enzyme identification and engineering in the early stages of drug development (Kim et al., 2024).
With over 189 million sequence records available in the UniProt database, efficiently finding an enzyme starting point that is catalytically active in a target reaction can be a significant challenge (Bateman et al., 2021). Screening commercial libraries is a common approach to identify useful biocatalysts. Recently, we reported using such an approach to identify ketoreductases (KREDs) capable of catalyzing the synthesis of a challenging chiral ketone intermediate through a kinetic resolution process (Figure 1) (Wamser et al., 2022). Of the KREDs that were screened, the best enzyme demonstrated 34 – 45% yield of the desired ketone (out of a 50% maximum possible yield) and up to >99% enantiomeric excess (ee%) using only 2 – 4 wt% of enzyme in the reaction. In this work, we report the use of an orthogonal in-silico approach to identify alternative WT enzymes suitable for this reaction (Figure 1) that could serve as favorable protein engineering starting points.
We used BioMatchMaker® (BMM), an in-silico, physics-based, high-throughput platform to screen nearly half a million enzymes and tested the top 16 enzyme hits against the target reaction (Figure 1). Of the 16 enzymes tested, enzyme 15, a bacterial short-chain dehydrogenase (SDR) KRED from T. caliditerrae (UniProt: A0A7C5VFX3; Genbank: HHM67809.1), referred to in this study as Tcalid SDR, had the most favorable conversion rates and desired enantioselectivity.
We then evaluated Tcalid SDR as a potential starting point for engineering with emphasis on understanding the enzyme’s structure-function landscape. The crystal structures of Tcalid SDR in the apo-form and complexed with NADP cofactor were determined and are presented herein (PDB IDs: 9FEB and 9FE6). Using these structures, a combination of computational site-directed mutagenesis and enzymatic activity analysis, we define a potential substrate-binding pocket that determines enantioselective catalysis. To further assess this enzyme as an engineering starting point, we determined the pH and temperature tolerance profiles and substrate promiscuity of this enzyme and selected mutants. Finally, we evaluated the performance of Tcalid SDR in an EasyMax reactor at 100 mL scale and found that the enzyme performed reasonably at scale, affording 64% conv and 89% ee.
Materials and methods
Protein identification
BioMatchMaker® (BMM) is an in silico high-throughput data platform developed at ZYMVOL that allows the identification of WT enzyme sequences capable of catalyzing a desired chemical transformation, even a new-to-nature transformation. We use BMM to identify sequences and ultimately select sequences to be tested. To do this, the BMM platform utilizes quantum mechanics to study energetically accessible substrate conformations, automatic homology modeling generation for enzymes under evaluation, ensemble enzyme conformer generation, model system sanitization, cofactor/ion enrichment evaluation, docking survey substrate exploration and post-docking analysis through MD simulations. MD simulation protocols used through the BMM platform are comparable to the MD simulation protocol previously reported in Pallara et al., 2022; Pallara et al., 2022). An initial search of public databases identified more than 450K enzyme sequences from bacteria, yeast and fungi with multi-label enzyme assignation “reductase”, “aldo-keto reductase”, “carbonyl reductase”, “dehydrogenase”, and others related to the enzyme function assigned to KREDs. The interaction between the substrate of interest and the 450K enzyme sequences identified for initial screening were studied using the BMM platform. While sequences with 3D crystallographic information were retained (130) for additional exploration, sequences lacking a realistic 3D representation underwent homology modeling techniques using proprietary software, BMM. To reduce complexity, only representative sequences from this pool were retained after clustering using a cutoff similarity threshold of 80%, leaving 11,423 representative (clustering centroid sequences) enzyme entities for modeling exploration. The homology models (HM) were profiled in terms of structural quality, the presence of required cofactor (NADPH or NADH) and sidechain orientation of catalytic residues, neglecting enzyme entries that were unable to satisfy quality of structural composition metrics as measured by z-scores (deviation of the total energy of the structure model with respect to a random distribution of random conformations) (Wiederstein and Sippl, 2007). A z-score value within the range of −3 to 3 defined a good quality structural classification. To increase structural quality classification robustness, additional parameters were investigated including the presence of cofactor atoms, correct protonation/hydrogenation state, the presence and location of binding site waters and the sidechain orientation of residues lying around cofactor atoms with a distance less than 8 Å. Each 3D model towards the substrate was created using AlphaFold in conjunction with a proprietary algorithm comparable to AlphaFill to introduce cofactors, ions and other ligands into the protein structural model (Jumper et al., 2021; Hekkelman et al., 2023). The models were further refined using MD simulations and previously described methods to allocate water molecules into the binding site (Seco et al., 2009). Before studying the affinity of each 3D model towards the substrate, an ensemble of conformations was generated to produce enzyme conformers that were biologically relevant in the presence of the cofactor while maximizing structural divergence (RMSD threshold lower than 8 Ả when compared to original starting model conformation). We use 20 to 50 conformers for each enzyme sequence to satisfy the minimal conformer requirement needed for a statistical analysis of the total conformation ensemble. With this analysis, BMM can quantify the binding site plasticity and capability of the enzyme system to adopt multiple geometries while interacting with the substrate. A total of 8972 sequences were subjected to conformational exploration. Computational docking studies with the target substrate, S-ketone ((S)-2-ethynyl-2-methylcyclohexan-1-one, CAS# not available), as well as its R-ketone ((R)-2-ethynyl-2-methylcyclohexan-1-one, CAS#: 2749965-88-4) counterpart were performed for each enzyme conformation (ensemble) using proprietary software that has been fine-tuned for the study of chemically relevant substrates. The averaged docking score was calculated for each substrate along the multiple enzyme conformers, retaining the best scored pair in terms of substrate-enzyme conformation (Table 1) as indicative of activity. The larger the docking score gap (in absolute value) for S-ketone and R-ketone substrate, the better the expected enzymatic resolution towards the affinity of one of the enantiomers.
Initial values of −6.5 kcal/mol and −3.0 kcal/mol for S- and R-ketones respectively were applied, with negative results measured in terms of retained sequences. The docking score gap was lowered until a difference of 1.0 kcal/mol was achieved (compatible with associated docking score errors and error range of most common docking scoring functions), with larger docking scores towards S-ketone, but no significant results were found (Ramírez and Caballero, 2016; Velasquez-López et al., 2022). Due to the lack of discriminatory power of docking scores, we employed docking post-analysis using BMM that includes MD simulation in conjunction with additional metrics (interactions formed/broken, presence of structural waters, energetic profiles based on MM/PBSA, RMSD stability) (Monza et al., 2017). Lastly, we applied distance filters to discriminate between enzyme candidates. Briefly, the distance between the carbonyl substrate atom and reactive NAD(P)H cofactor atom was extracted for each ensemble substrate pair. Sequences whose averaged distance pairs for S-ketone was smaller than for R-ketone were retained. The distance threshold where differentiation was expected to be 3.5Ả units, with a difference in terms of distance 0.5 Ả when comparing the S-ketone versus its R-ketone counterpart. We postulated that this difference would preferentially select those substrate conformations that are compatible with a Near Attack Conformation (NAC) of the desired enzyme/substrate pair, being more preferential for S-ketone over the R-ketone under same enzyme conditions (Sadiq and Coveney, 2015).
Finally, enzyme sequences able to satisfy distance criteria were subjected to molecular mechanics minimization and short molecular dynamics (MD) simulations to further evaluate the substrate stability profile. Structures with an RMSD of 2.0 Å after minimization for S-ketone but >2.0 Å larger RMSD value for R-ketone were finally retained for visually inspection and prioritization based on different structural profiles observed along the analysis.
A set of 16 sequence diverse enzymes were proposed for experimental evaluation.
Cloning and expression
The synthetic gene encoding SDR oxidoreductase (A0A7C5VFX3), Tcalid SDR, was optimized for expression in Escherichia coli (Supplementary Table S1) and purchased from IDT (Integrated DNA Technologies). The gene was cloned by Golden Gate cloning in pBAD His-tag using BsaI (NEB, New England Biolabs) to obtain N-term fused His-tag enzyme.
To introduce single or double mutations a whole-plasmid PCR was performed using partially overlapping primers (IDT) (Supplementary Table S2) and Pfu-Ultra II Hotstart PCR Master Mix (Agilent Technologies). Template DNA was cleaved with DpnI (NEB). The plasmid was transformed into NEB 10 beta E. coli cells (NEB). The introduction of the mutations was confirmed by Sanger sequencing.
Expression was performed at 24°C for 20 h in NEB 10 beta E. coli cells in Terrific Broth media inducing the expression at O.D.600 0.5 with 0.02% arabinose (final concentration).
Cells were harvested by centrifugation and resuspended in 50 mM KPi buffer pH 7.5 150 mM NaCl, cell pellets solubilized in buffer in a ratio 1:15. Cell disruption was done by sonication for 8 min in water ice bath with the settings: 70% amplitude 5 s on and 10 s off. After that the cell lysate was centrifuged at 13000 g for 45 min at 4°C to obtain the cell free extract.
Purification of Tcalid SDR and mutants
The purification was performed using Ni-Sepharose resin (GE Healthcare) for metal-affinity chromatography (IMAC). Cell free extract was applied to pre-equilibrated resin. The resin was then washed with 50 mM KPi buffer pH 7.5 150 mM NaCl 10 mM imidazole, the enzyme was eluted in 50 mM KPi buffer pH 7.5 150 mM NaCl 500 mM imidazole and desalted in 100 mM NaPi buffer pH 7.5 150 mM NaCl and flash frozen. Enzyme purity was evaluated by SDS-PAGE and enzyme concentration was measured using ε 280 = 11.5 mM-1*cm-1 and molecular weight of 27.8 KDa. Expression, purification, and analysis of the mutant enzymes were performed as for the WT enzyme.
Melting temperature
Enzyme apparent melting temperature (Tmapp) was measured using Thermofluor assay. Real-Time PCR thermocycler (CFX96-Touch, Bio-Rad) was used to measure the fluorescence emission of the melting curves profiles, in the assay the enzyme final concentration was 10 µM and SYPRO Orange dye (Merck) was 20x, total reaction volume was 25 µL.
Chemicals
Racemic, (R) or (S)-2-ethynyl-2-methylcyclohexan-1-one (CAS#: 65691-72-7) were previously reported (Wamser et al., 2022) and provided by Boehringer- Ingelheim. Acetophenone, (S)-1-phenylethan-1-ol, (R)-1-phenylethan-1-ol were purchased from Millipore Sigma, 4-phenylcyclohexanone was purchased from Fluorochem, cis-4-phenylcyclohexanol and trans-4-phenylcyclohexanol were purchased from Chempur. NADPH and NADH were purchased from Oriental Yeast Co., Ltd.
Enzymatic assays and scaleup
Reactions were performed in 1.5 mL Eppendorf vials containing 50 mM substrate, 20 mM NADH or 20 mM NADPH, 5 µM purified enzyme, in 100 mM NaPi pH 7.0 150 mM NaCl, with a total volume reaction 200 µL in triplicates. Reactions were incubated at 25°C for 2 h in orbital shaking at 135 rpm. For reaction performed using racemic 2-ethynyl-2-methylcyclohexan-1-one and acetophenone, reactions were stopped by adding 500 µL of ethyl acetate to prepare the samples for GC analysis. After the ethyl acetate addition, reactions were mixed by vortex and centrifuged at 10,000 g for 5 min. Supernatant containing ethyl acetate was dried over anhydrous MgSO4. Reaction mixtures were then transferred into GC vials for reaction conversion and enantioselectivity analysis. For reactions testing the effect of pH, an NaPi buffer was used for testing pH 6, 7, and 8 while a citrate buffer was used for pH 4 and 5. For reactions investigating thermal stability, enzyme solutions at 12.5 µM were first incubated at 30, 50, 70 and 90°C for 30 min with all other reaction conditions remaining the same before cooling to 25°C to perform the reactions. The scale-up EasyMax reaction was performed in a total volume of 100 mL with 50 mM (6.8 g/L) substrate, 0.2 mM NADH, 10 vol% lysate, 7 mg (1 wt% GDH-105 from Codexis), 30 mM glucose, 15 mM NaCl, and 100 mM NaPi pH 7, 25°C. After the reaction reached the desired conversions, ethyl acetate extractions were performed followed by Celite filtration to remove any protein present. The combined organic layer was concentrated under 35°C with 50 mbar to produce a mixture of R-ketone and S-alcohol. Reaction yield and enantiomeric ratio of R-ketone was calculated based on NMR and chiral GC analysis, respectively. For reactions with 4-phenylcyclohexanone, reactions were stopped by adding 200 µL of acetonitrile to denature the enzyme, charging an additional 200 µL of mix acetonitrile-water 50:50 (v:v), vortexed and centrifuged, transferred in HPLC vials and analyzed by HPLC. Enantiopure substrates ((R)-2-ethynyl-2-methylcyclohexan-1-one, (S)-2-ethynyl-2-methylcyclohexan-1-one, (S)-2-ethynyl-2-methylcyclohexan-1-ol, 4-phenylcyclohexanone and cis-4-phenylcyclohexanol) were used as standards to establish the absolute configuration of the alcohols and to obtain calibration curves to measure conversions and yields.
Analytical methods
For small-scale reactions, sample for gas-chromatography analysis were run on a GC-2014 Shimadzu system with column Beta DEX 22524348 30 mx 0.25 mmx 0.25 µm (Supelco). Program: 40°C equilibration time 1 min, to 90°C in 7.5 min, to 105°C in 15 min, to 150°C 2.5 min, to 40°C in 5.5 min. Retention times were: (S)-2-ethynyl-2-methylcyclohexan-1-one 12.6 min, (R)-2-ethynyl-2-methylcyclohexan-1-one 12.75 min, (S)-2-ethynyl-2-methylcyclohexan-1-ol 13.0 min. Acetophenone 15.5 min, (R)-1-phenylethan-1-ol 17.0 min, (S)-1-phenylethan-1-ol 17.35 min.
For the scale-up reaction, samples for gas-chromatography analysis were run on a HP 6890 GC with Supelco Beta DEX 225 (Part number 24348) 30 m x 0.25 mm x 0.25 µm column and FID detector. Program: 40°C equilibration time 1 min, ramp 6.8°C/min to 90°C, ramp 1°C/min to 105°C, ramp °C/min 18°C/min 150°C, ramp 27.3°C/min to 40°C, 0.5 min post run time. Retention times were: (S)-2-ethynyl-2-methylcyclohexan-1-one 14.7 min, (R)-2-ethynyl-2-methylcyclohexan-1-one 15.0 min, (S)-2-ethynyl-2-methylcyclohexan-1-ol 15.2 min.
Samples for HPLC analysis were run on a Vanquish system with column Gemini 3 µm NX-C18 110 Å LC Column 100 x 2 mm (Phenomenex). Program was isocratic 65% H2O pH 2.0 (using phosphoric acid), 35% acetonitrile flow 0.25 mL/min, 10 min total time. Retention times were: 4-phenylcyclohexanone; 7.3 min, cis-4-phenylcyclohexanol; 6.4 min, trans-4-phenylcyclohexanol; 5.4 min.
Protein sample for crystallization
The His (6x)-tagged purified protein was obtained from Gecco Biotech B.V in the following buffer: 50 mM Tris HCl, 150 mM NaCl, pH 7.5 at 10.4 mg/mL concentration.
Crystallization for obtaining apoprotein structure
Apoprotein crystals were obtained using the reservoir solution containing 0.1 M Tris pH 8.5, 0.3 M magnesium formate, with a 2:1 (protein:reservoir, 100 nL:50 nL) ratio at 20°C. Crystals were initially observed after a period of 13 days and underwent further growth for an additional week before being harvested. X-ray diffraction data were collected at beamline PXII-X10SA of Swiss Light Source (SLS) at Paul Scherrer Institute, Villigen, Switzerland.
Crystallization and soaking protocol for obtaining co-factor bound structure
Another batch of apoprotein crystals were obtained using the reservoir solution containing 0.1 M Tris pH 8.0, 0.2 M magnesium chloride, and 6% w/v PEG8000, with a 1:1 (protein: reservoir, 75 nL:75 nL) ratio at 20°C. These crystals are isomorphous to the other crystals above, grew to their maximal size within 4 days and were incubated in the reservoir buffer containing 20 mM NADP for 2 h at 20°C to obtain cofactor-bound protein. X-ray diffraction data were collected at the PETRA III Beamline (P14) at European Molecular Biology Laboratory (EMBL), Hamburg, Germany.
Crystallization trials were conducted in the 96-well SWISS SCI 3Drop plates via sitting drop vapor diffusion method. Crystals were cryopreserved in 30% ethylene glycol and subsequently flash-frozen in liquid nitrogen.
Structure determination and refinement
Datasets were processed using the autoPROC pipeline the XDS package v8.9. Diffraction or resolution limits were estimated via STARANISO (Tickle et al., 2024). ColabFold was used to obtain the structural model of a reductase tetramer, which was then used as an input to Phaser-MR to solve the phase problem via molecular replacement for the apoprotein structure (McCoy et al., 2007). For the co-factor bound structure, the newly determined apoprotein structure was used during molecular replacement. The final structures were determined after iterative rounds of manual model building and refinement via Coot and auto BUSTER, respectively (Emsley et al., 2010; Bricogne et al., 2024).
Data processing and refinement statistics for both the structures can be found in Supplementary Table S3. The final models of all crystal structures and their corresponding structure factors have been deposited in the Protein Data Bank (PDB). The PDB ID: 9FE6 for apoprotein, at resolution 1.9 Å, and PDB ID: 9FEBfor the NADP bound structure, at resolution 1.7 Å. Both structures have a single tetramer in the asymmetric unit.
Results
Computational screening for enantioselective KREDs
We used BioMatchMaker® (BMM®), an in silico high-throughput platform to identify publicly disclosed enzyme sequences predicted to catalyze our desired reaction (Figure 1). The BMM® algorithm uses a staged approach that combines sequence analysis, homology modeling, and docking to identify enzymes predicted to be compatible with a desired reaction, in this case stereospecific reduction of an S-ketone.
Because the algorithm relies heavily on docking and detailed evaluation of the docked poses (i.e., distance between reactive atoms), the enzyme structure is critically important. Of the unranked top-16 enzymes selected by BMM®, none had structural data available in the public domain necessitating the use of homology models for each of these enzymes (Table 1). Although it has been demonstrated that high-quality homology models can rival crystal structures in terms of docking scores (Novoa et al., 2010), structural ensembles were generated for each homology model to further enrich the docking results.
The higher the quality of the 3D models (measured in terms of Z-score), the better the ensemble of conformations generated and more accurate and reliable the predictive capability of BMM. The ensemble computational strategy employed with the use of BMM has been previously proven to be effective and allows identification of enzyme-substrate interaction (Mohammadi et al., 2022) The ensemble approach was critical to identify enantioselective enzyme candidates because of the ability to consider simultaneous events when studying different pair enzyme/substrate interactions.
We postulated the better the docking scores of the ensemble of conformations towards one enantiomer, the higher the enzymatic conversion. To support this, docking scores and distance threshold gaps need to be considered simultaneously. While multiple enzyme/substrate pairs could not be discriminated through docking divergence alone (maximum difference of 0.3 docking score units, Table 1, Supplementary Figure S1), the distance separation method offered a more robust discrimination. However, combining the distance threshold with the distance difference cutoff of at least 0.5 Å between enantiomers per enzyme conformation, we were able to discriminate between stereoisomers in most enzyme conformations. In applying this method across the ensemble, the accumulated effect of multiple enzyme conformers with multiple substrate conformers (either from S- or R-ketone), the enantioselectivity became more pronounced.
Using multiple conformers allowed better discrimination between stereoisomers than using one single enzyme representation, even using the crystal structure as the single pose tested. When re-docking the enantiomer ketone substrate to each crystallographic form (especially to co-factor bound), no significant differences were found when comparing the two substrate stereoisomers. One possible reason that docking scores alone were unable to discriminate between the stereoisomers is the relatively large pocket size (300 Å3) in relation the small size of the substrate (∼19 Å3). This finding re-enforces the idea that multiple enzyme co-factor bound conformers are needed for determining enantioselectivity (Supplementary Figure S2). Importantly, the crystal structure was highly similar to the lowest energy homology model of enzyme 15, the RMSD ranged from 0.86 to 2.05 Å depending on the chains used for comparison.
Coupled together, these findings suggest that enzyme 15, a bacterial short-chain dehydrogenase (SDR) KRED from T. caliditerrae (Tcalid SDR), along with the other 15 selected enzymes, may be capable of catalyzing the desired reaction but might also have a narrow margin for promoting the reaction in a stereospecific manner given the relatively small substrate, a finding that has been observed previously (Noey et al., 2015).
Chemical conversion and stability of selected hits
All 16 hits were expressed in E. coli, purified, and tested for their performance as biocatalysts in the KRED promoted kinetic resolution of racemic ketone. Percent conversion (c%) and enantiomeric excess (ee%) based on product analysis were measured at 30-min in the reaction (Figure 2). Of the enzymes tested, enzyme 15 obtained the best results within a 30-min reaction, demonstrating 63% conversion while achieving 100 ee% (Figure 2). Enzyme 2 performed similarly and reached an ideal conversion of 50% and an ee% of 88; however, this enzyme displayed undesired reactivity to the R-ketone, resulting in a lower ee%. Enzyme 14 had an ee% of 100 but displayed very low conversion to product (0.2%). Of the 16 selected hits, enzymes 1, 4, 6, 8, 11, 12 and 16 appeared to have little or no activity in a reaction time of 30 min.
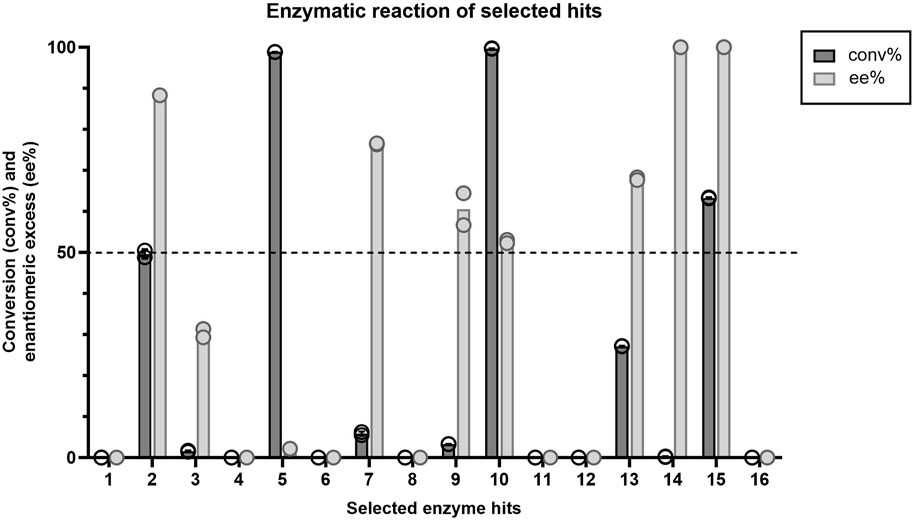
Figure 2. Average conversion (%) and enantiomeric excess (%) values (n=2) for each enzyme hit within the target kinetic resolution reaction. Dashed line indicates the targeted conversion (50%) within this reaction. Symbols represent the values of individual experiments.
To assess enzyme stability of the 16 hits, we measured apparent melting temperature (Tmapp) using a Thermofluor assay (Table 2). Enzyme 2 had the highest melting temperature (71°C). We were unable to obtain a melting temperature when testing enzyme stability up to 80°C for enzymes 1, 8 and 15. Of the 16 enzymes, Tcalid SDR (enzyme 15) was established as the best candidate for further characterization due to its high ee% at 30 min while maintaining a conversion close to 50%.
Tcalid SDR structure
The structure of apo and NADP-bound structure of Tcalid SDRwere determined at 1.9 and 1.7 Å, respectively. The enzyme adopts a tetrameric quaternary structure with 222-symmetry. Both structures contain a tetramer in the asymmetric unit. Each sub-unit of the tetramer consists of a α/β/α-fold barrel, where the parallel β-sheet (β1-β7 strands) is in the center of the six helices (α1-α5 and α8) representing the typical nucleotide binding Rossmann fold (Rao and Rossmann, 1973) (Figure 3A). The N-terminal region (residues 1-25 based on the modified protein sequence and residues 1-4 in the WT sequence) is poorly defined by electron density likely due to flexibility in all chains. Interestingly, additional electron density is present for the linker peptides ‘LVPR’ and ‘GLVPR’ in the apo and co-factor bound structures, respectively. These peptides represent the thrombin cleavage site and are visible near the N-termini of chain A and chain D and are referred to as ‘Chain E’ in each of the structures (Figure 3B). The poor electron density in the N-terminus of the chains makes it difficult to assess whether the chain E is a part of chain A or chain D. In addition, two magnesium ions with an octahedral hydration sphere were observed at the center of the tetramer near the C-terminus of the sub-units (Figure 3B). The location and the functional relevance of the magnesium ions has been described and investigated before for SDR family of proteins (Ji et al., 2021; Niefind et al., 2003). Tcalid SDR belongs to the classical family of SDRs known for the presence of N-terminal motif – TGxxxGxG, where ‘x’ can be any amino acid (Kallberg et al., 2002). This motif in Tcalid SDR has the sequence ‘TGASRGIG’.
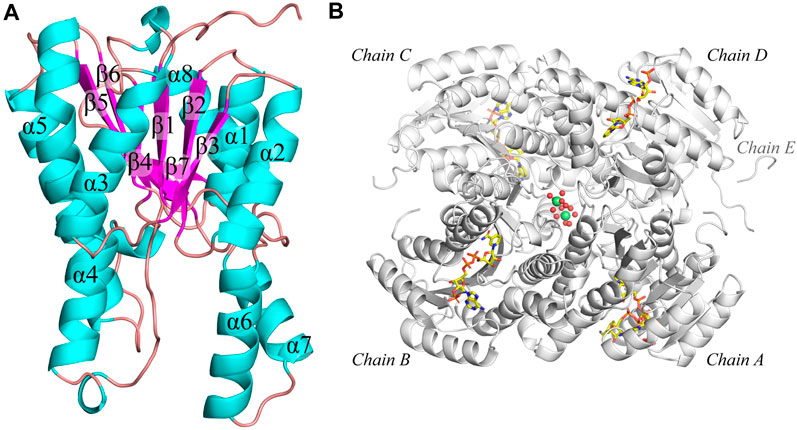
Figure 3. Structure of Tcalid SDR. (A) The secondary structure of Tcalid SDR is highlighted (representative structure of chain C) of NADP-bound Tcalid SDR structure in different colors (helices – cyan, beta-strands – pink, loops - mauve) (B) The tetramer structure (white) of the NADP-bound Tcalid SDR highlighting the bound co-factor (NADP – carbon atoms in yellow) in each sub-unit along with hydrated magnesium ions (lime spheres of magnesium with oxygen atoms of waters shown as smaller red spheres).
The co-factor bound structure of Tcalid SDR was determined by soaking the apoprotein crystal with NADP. Interestingly, in chain A NADP is bound in an alternate conformation, where one of the conformations could possibly represent an encounter complex (Figure 3A). This is in contrast to chains B, C and D, where NADP is fully accommodated in the co-factor binding pocket in the active state conformation comparable to short-chain dehydrogenases NADP-bound structures that were determined previously. One possible reason for the presence of the alternate NADP conformation could be the open orientation of α6 and α7 in chain A, which is typically closed in other chains, e.g., chain C (Figure 4B). Importantly, similar differences were observed between the helix conformation in the apoprotein structure, suggesting that the helix conformation is unlikely to be caused by the entry of NADP during soaking. We suggest that crystal packing forces keep the chain A helices α6 and α7 in place and prevent adoption of the active state (Figure 4). Thus, the observation of the encounter complex is likely a crystallization artifact. It is important to note that chain B also exhibits an open conformation similar to chain A as observed from both structures (Supplementary Figure S3), even after fully accommodating NADP in the co-factor binding pocket of chain B. A helix position like α7 in chains A and B in our Tcalid SDR structure was also observed in Serratia marcescens SDR, where this helix has been described as a lid that covers the co-factor binding domain (Liu et al., 2018). Interestingly, a reductase of the SDR superfamily from the plant Plantago major has a multi-helix “capping domain” covering the of co-factor and substrate binding regions (Fellows et al., 2018), which is possibly analogous to the helices α6 and α7 in the Tcalid SDR structure. NADP as bound in the active state in chain C shows interactions with multiple sidechains along with multiple water molecules in the binding pocket. As expected, the protein environment around the co-factor is very similar to other members of the SDR family, e.g., the Actinorhodin polyketide ketoreductase (PDB ID:1X7G) (Korman et al., 2004) which is the closest sequence homolog to Tcalid SDR with known experimental structure. Structural similarity naturally also applies to the presence of the highly conserved catalytic triad S143, Y156, and K160 in Tcalid SDR (Filling et al., 2002a).
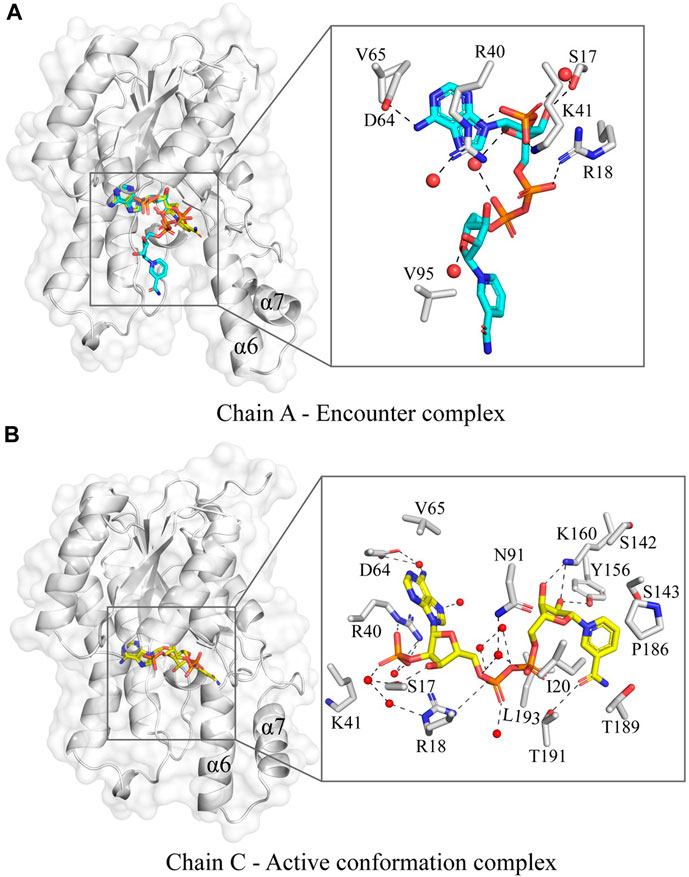
Figure 4. The variation in the NADP conformation in the co-factor bound Tcalid SDR structure. (A) The chain A of Tcalid SDR showing two observed conformations of NADP (conformation A – carbons in cyan and conformation B – carbons in yellow) with a zoomed in view of the encounter complex (NADP in conformation A). (B) The chain C of Tcalid SDR focusing the active conformation complex with the NADP (carbons in yellow) fully accommodated in the binding pocket with a close-up view.
Tcalid SDR characterization
To functionally characterize Tcalid SDR, we first evaluated cofactor preference for our desired reaction using NADH and NADPH and found similar activity with both cofactors regardless of length of reaction time (30 min, 2h, 6h) or enzyme concentration (3.5–6.0 µM) (Supplementary Tables S4, S5). NADH was the preferred cofactor at the manufacturing-relevant time of 6 h, thus, we chose to perform all subsequent experiments with NADH.
Because Tcalid SDR is derived from a thermophilic bacterium, enzyme stability against temperature and pH was expected. Previously, strains of T. caliditerrae have been reported to grow at temperatures ranging from 50 to 70°C (optimum 65°C, no growth below 50°C) and at pH 6.0 to 8.0 (optimum 7.0) (Ming et al., 2014). When we tested the effect of pH on Tcalid SDR, the enzyme remained active at pH 5, 6, 7, and 8 with the best activity at pH 7 and no activity at pH 4 (Table 3A). Conversion at pH 8 decreased by ∼7%, however; ee% remained the same. To test the effect of temperature on enzyme activity, we incubated Tcalid SDR at 25, 30, 50, 70, and 90°C for 30 min and then tested enzyme activity at 25°C (Table 3B). At all temperatures tested, Tcalid SDR has an ee% range between 38 and 53% and a range of 27 and 40% conversion. While a lower ee% was observed at lower percentages of conversion, the ee percentages were close to the calculated theoretical max ee% (Table 3A, 3B). Our results confirm that Tcalid SDR retains activity up to the highest incubation temperature tested (90°C) and can remain active within a broad pH range of 5–8.
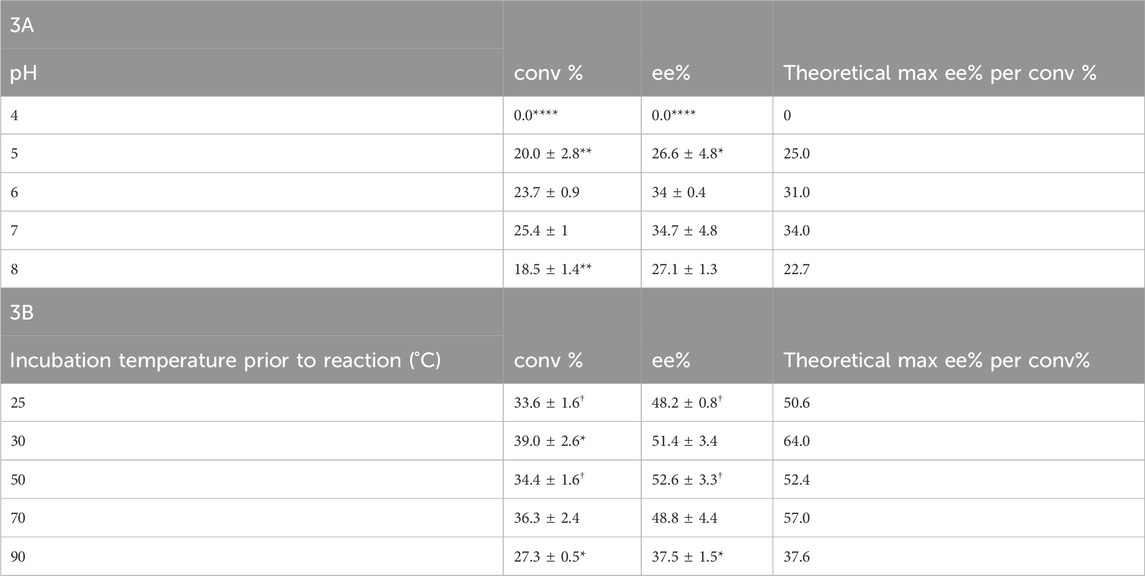
Table 3. Conversion (conv%) and enantioselectivity (ee%) ± SEM (n = 3) pH profile for Tcalid SDR (A) and temperature (n = 3; †, n = 2) profile for Tcalid SDR (B). conv% and ee% are calculated based on chiral chromatography analysis (±2%). Significantly different than WT conversion and enantioselectivity at either pH 7 or incubation temperature 25°C by one-way ANOVA *(p ≤ 0.05), **(p ≤ 0.01), ***(p ≤ 0.001), ****(p ≤ 0.0001).
Biocatalysis scaleup with Tcalid SDR
To further evaluate Tcalid SDR as a starting point for biocatalyst engineering, we test the biocatalytic kinetic resolution of rac-ethynyl-2-methylcyclohexan-1-one with Tcalid SDR in a 100 mL scaleup reaction using an EasyMax reactor. The reaction proceeded to 64% conversion (89% ee) within 3 h and recovered our desired R-ketone with 26% yield (Table 4). Based on 64% conversion, there was a maximum possible yield of 36%. Similar results were obtained in another batch of the same enzyme (56% conv, 80% ee). We show that Tcalid SDR maintains similar enantioselectivity and reactivity towards the S-ketone within a larger reaction volume.

Table 4. Scale-up reaction results with Tcalid SDR. conv% and ee% are calculated based on chiral chromatography analysis (±2%). For experimental details, see Enzymatic Assays and Scaleup methods section.
Tcalid SDR mutational evaluation
Given that little information about Tcalid SDR was available in the public domain, we made select mutations to establish a basic structure-function relationship (Table 5, Supplementary Figure S4). First, catalytic residues were evaluated via alanine scanning of the canonical short-chain dehydrogenase/reductase (SDR) catalytic triad comprised of S143, Y156 and K160 (Filling et al., 2002a; Qiao et al., 2023). Mutation of these residues completely ablate enzyme activity (Figure 5). While the S-Y-K catalytic triad has been reported among SDR enzymes, a secondary catalytic mechanism that extends past the catalytic triad to include an N-S-Y-K catalytic tetrad has also been reported (Filling et al., 2002a; Penning, 2015). A mutagenesis study of a bacterial SDR from C. testosteroni (PDB: 1HXH) reported a residue, N111 plays an essential role in maintaining active site configurations and building up a proton relay system through stabilization of the position of the lysine within the catalytic tetrad (Filling et al., 2002a). When N111 was mutated, C. testosteroni SDR was rendered inactive without affecting oligomerization. We identified N115 as the structural equivalent to N111 in Tcalid SDR. Within our targeted reaction, mutation of N115 to alanine did not substantially alter activity (Figure 5).
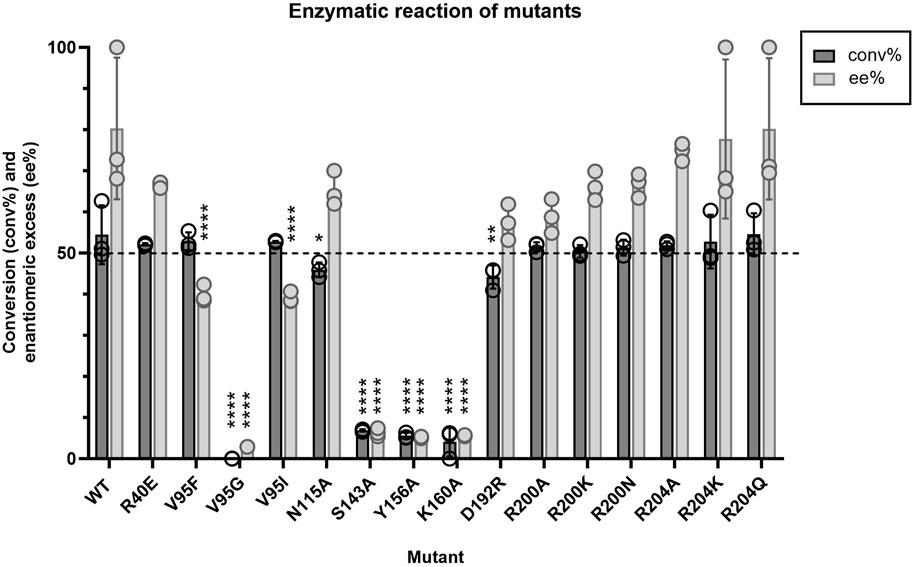
Figure 5. Average conversion (%) and enantiomeric excess (%) results (n = 3) for Tcalid SDR mutants and Tcalid SDR (wild type; WT) within the target kinetic resolution using NADH as the cofactor. Dashed line indicates the targeted conversion (50%) within this reaction. conv% and ee% are calculated based on chiral chromatography analysis (±2%). Symbols represent the values of individual experiments. Bars show mean ± SEM. Significantly different than WT by one-way ANOVA *(p ≤ 0.05), **(p ≤ 0.01), ***(p ≤ 0.001), ****(p ≤ 0.0001).
Given that the enzyme is from a thermophilic bacterium, we sought to elucidate structure-function relationships that confer thermostability to Tcalid SDR. A previous study of a related Thermus SDR, T. thermophilus aldose 1-dehydrogenase (TAD), evaluated mutations of D242 and found that this residue is part of a D-G-G sequence motif implicated as a structural factor that confers thermostability through aromatic-aromatic interactions (Asada et al., 2009). This study found that mutation to D242N results in a large decrease in thermostability without dissociation of TAD to a dimer or monomer. Although TAD has only 32% sequence identity to Tcalid SDR, we find A254 is the structural equivalent to D242 and is part of a similar A-G-G sequence motif. We tested two mutations A254D to examine whether mutation to D-G-G in Tcalid SDR retains enzyme thermostability and A254N to evaluate whether this mutation results in a similar loss of thermostability. When enzymes were incubated at 90°C for 30 min, both A254N and A254D resulted in significant decreases for % conversion and ee% compared to WT with A254D displaying the greatest decrease in activity (Figure 6).
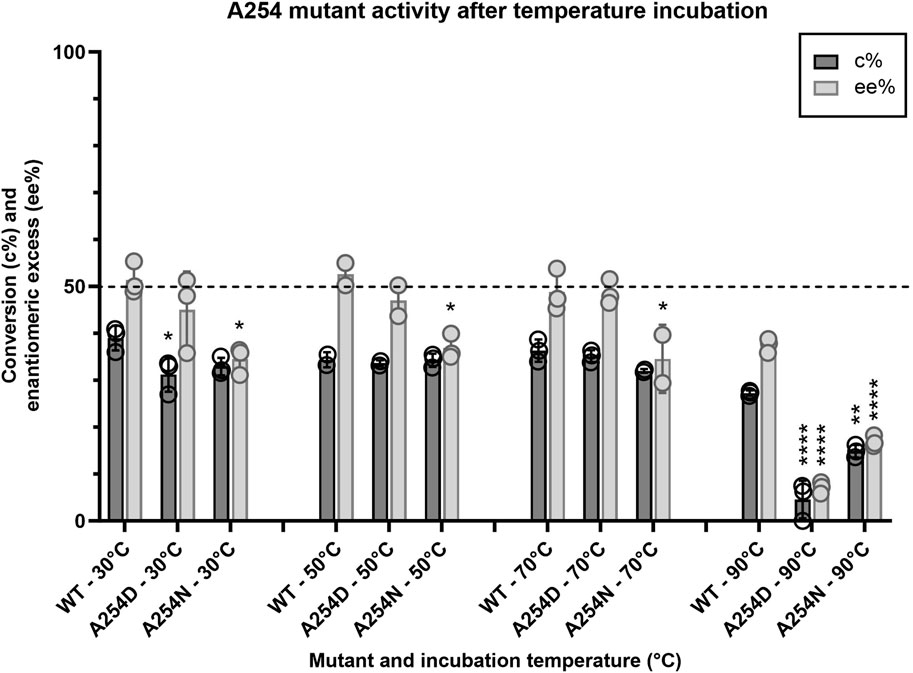
Figure 6. Activity profile of A254 mutants after temperature challenge. Average conversion (%) and enantiomeric excess (%) for two mutants of A254 and WT within the target kinetic resolution after a 30-minute incubation at 30, 50, 70 and 90°C (n = 3; n = 2 for WT-50°C, A254D-50°C and A254N-70°C). After the temperature challenge, all reactions were performed at 25°C with NADH as the cofactor. Dashed line indicates the targeted conversion (50%) within this reaction. conv% and ee% are calculated based on chiral chromatography analysis (±2%). Symbols represent the values of individual experiments. Bars show mean ± SEM. Significantly different than WT (within each respective temperature challenge experiment) by one-way ANOVA *(p ≤ 0.05), **(p ≤ 0.01), ***(p ≤ 0.001), ****(p ≤ 0.0001).
Finally, we investigated a number of single mutants aimed at altering the size of the binding pocket. A well-known example of how binding site structure can influence stereoselectivity is the SDR-family KRED from Lactobacilius kefir in which a 10-site mutant known as Sph is used for the large scale synthesis of a chiral intermediate (Noey et al., 2015). In this work, 3 single point mutants were reported which enhanced stereoselectivity for either the R- or S- enantiomer. Of the single point mutants, A94F was reported to enhance stereoselectivity for the S- enantiomer by ∼10 fold compared to the WT enzyme. Based on this information, we selected several sites to mutate in the entrance tunnel and active site of Tcalid SDR, including mutations at V95, the structural equivalent of A94 in L. kefir (Table 5). We also tested mutants at R200 and R204 which are in the mobile α6 helix that we hypothesized could play a role in the entry or egress of cofactors, substrates, or products. Finally, to investigate the effect of charge near the entrance tunnel, we also investigated mutants R40E and D192R but the results were in-conclusive.
Apart from mutations at the catalytic triad positions and V95G, all other mutations tested did not have substantially altered catalytic efficiency or the enantiomeric excess (Figure 5). Even V95F, the structural equivalent of the best point mutant in the aforementioned KRED from L. kefir, did not have a substantial impact on activity.
All mutants and WT were also tested for activity and selectivity with alternative substrates acetophenone and 4-phenylcyclohexanone. No activity was observed towards acetophenone for all mutants and WT (data not shown). When tested for activity towards 4-phenylcyclohexanone, all mutants and WT were selective to obtain cis-4-phenylcyclohexanone with ee% > 100 (data not shown). Of the mutants tested, V95G had significantly decreased % conversion compared to WT (Table 6). Some mutants appeared to increase % conversion (V95I and R200N) while other mutants decreased % conversion (D192R, R204K, and R204A) compared to WT, however; these changes were not statistically significant. Tcalid SDR showed ∼10% less activity towards 4-phenylcyclohexanone compared to our substrate of interest.
Discussion
Enzymatic catalysis has become a promising alternative to traditional synthetic routes, especially for chiral organic compounds that are challenging to synthesize. However, use of biocatalysis in industrial settings is still limited. According to a recent review, the reasons for this are numerous but two of the most commonly cited are poor enzyme activity and tight timelines for implementation (France et al., 2023). Suggested solutions to these problems include evolving the enzyme and screening commercial or WT enzymes, respectively.
Compared to experimental screening of enzymes, in-silico screening can be advantageous from a time and cost perspective. In this work we used an in silico tool, BioMatchMaker® to screen >450K enzymes and identify a SDR KRED from T. caliditerrae capable of catalyzing the desired reaction. The enzyme was characterized via functional screen and solved both the apo and co-factor bound crystal structures were solved to better enable downstream engineering. Although it was not done in the current work, it should be emphasized that the use of an in-silico pipeline, as compared to a commercial kit screen, allows for a priori selection for or against enzyme attributes, for instance, restricting searches to only thermophiles.
The observed preference for the (S) enantiomer is not readily explainable by the homology models used nor by the x-ray crystal structure that was solved subsequently. Using distance between the carbonyl oxygen and the cofactor provided a practical method to discriminate structures during the initial screening, but this alone does not sufficiently describe the mechanism of stereoselectivity. Preliminary attempts to gain a deeper understanding of the mechanism through a co-crystal using both the substrate and racemic mixture were unsuccessful. Notably, the screening methodology employed was successful despite lacking a detailed mechanistic understanding of the enzyme. This is an important screening consideration for biocatalysis as detailed mechanisms aren’t often known a priori.
Our preliminary mutagenesis screen suggests that SDR KRED from T. caliditerrae is relatively resistant to mutations. One possible explanation for the robustness of Tcalid SDR to mutation is the thermophilic nature of Tcalid SDR. While thermophilic enzymes often make an excellent starting point for mutagenesis, the higher stability and mutational robustness of thermophilic enzyme starting point (Bloom et al., 2006; Finch and Kim, 2018) may necessitate a greater number of simultaneous mutations to achieve the desired engineering target. In fact, the authors of the L. kefir mutagenesis note that the most effective designs, including Sph contained multiple mutations that work in conjunction to alter the dynamics of the active site pocket and loops (Noey et al., 2015).
Thermophilic enzymes offer several advantages as biocatalysts due to their unique combination of stability, adaptability, and activity (Atalah et al., 2019). While thermophilic enzymes do present unique engineering challenges, advancements in protein engineering over the last 20 years have led to a number of successful examples of thermophilic enzymes that have been engineered for biocatalysis (Karnaouri et al., 2019; Ramos-Martín et al., 2020; Bell et al., 2021). In our opinion, the solved crystal structures of Tcalid SDR along with promising preliminary scale-up results, suggest that Tcalid SDR represents a promising start point for further protein engineering.
Data availability statement
The data presented in this study are deposited into the RCSB Protein Data Bank, accession numbers 9FE6 and 9FEB.
Author contributions
YS: Writing–review and editing, Writing–original draft, Visualization, Validation, Project administration, Methodology, Investigation, Formal Analysis, Data curation, Conceptualization. BK: Writing–review and editing, Writing–original draft, Methodology, Formal Analysis, Data curation. JH: Writing–review and editing, Investigation. LK: Writing–review and editing, Writing–original draft, Supervision, Project administration, Formal Analysis, Conceptualization. HW: Writing–review and editing, Supervision, Formal Analysis, Conceptualization. SG: Writing–review and editing, Methodology. HN: Writing–review and editing, Writing–original draft, Validation, Supervision, Data curation. SK: Writing–review and editing, Resources. JM: Writing–review and editing, Writing–original draft, Software. Maria Fatima ML: Supervision, Writing–review and editing. CM: Methodology, Data curation, Writing–review and editing. NL: Project administration, Writing–review and editing. FB: Writing–review and editing. NP: Supervision, Writing–review and editing. AN: Writing–review and editing. JS: Writing–review and editing.
Funding
The author(s) declare that financial support was received for the research, authorship, and/or publication of this article. All research described herein was funded by Boehringer Ingelheim. The funder was not involved in the study design, collection, analysis, interpretation of data, the writing of this article, or the decision to submit it for publication.
Acknowledgments
We thank Edneil Antiquena and Xiaole Shao for chiral GC method development. We thank Dr. Linglin Wu and Dr. Jaehee Lee for substrate and enzyme outsourcing. We would also like to thank the Boehringer Ingelheim Post-doctoral committee, especially Curtis Warren and Joe Ashour.
Conflict of interest
YS, BK, JH, LK, HW, SG, HN, SK, FB, NP, AN, and JS were employed by Boehringer Ingelheim. JM and ML were employed by Zymvol. CM and NL were employed by Gecco Biotech. All scientific parties involved in this study are for-profit entities. Boehringer Ingelheim paid both GECCO and Zymvol for their efforts within this collaboration.
Publisher’s note
All claims expressed in this article are solely those of the authors and do not necessarily represent those of their affiliated organizations, or those of the publisher, the editors and the reviewers. Any product that may be evaluated in this article, or claim that may be made by its manufacturer, is not guaranteed or endorsed by the publisher.
Supplementary material
The Supplementary Material for this article can be found online at: https://www.frontiersin.org/articles/10.3389/fchbi.2024.1425501/full#supplementary-material
References
Asada, Y., Endo, S., Inoue, Y., Mamiya, H., Hara, A., Kunishima, N., et al. (2009). Biochemical and structural characterization of a short-chain dehydrogenase/reductase of Thermus thermophilus HB8. Chemico-Biological Interact. 178, 117–126. doi:10.1016/j.cbi.2008.09.018
Atalah, J., Cáceres-Moreno, P., Espina, G., and Blamey, J. M. (2019). Thermophiles and the applications of their enzymes as new biocatalysts. Bioresour. Technol. 280, 478–488. doi:10.1016/j.biortech.2019.02.008
Bateman, A., Martin, M. J., Orchard, S., Magrane, M., Agivetova, R., Ahmad, S., et al. (2021). UniProt: the universal protein knowledgebase in 2021. Nucleic Acids Res. 49, D480–D489. doi:10.1093/nar/gkaa1100
Bell, E. L., Finnigan, W., France, S. P., Green, A. P., Hayes, M. A., Hepworth, L. J., et al. (2021). Biocatalysis. Nat. Rev. Methods Prim. 1, 46. doi:10.1038/s43586-021-00044-z
Bloom, J. D., Labthavikul, S. T., Otey, C. R., and Arnold, F. H. (2006). Protein stability promotes evolvability. Proc. Natl. Acad. Sci. 103, 5869–5874. doi:10.1073/pnas.0510098103
Buller, R., Lutz, S., Kazlauskas, R. J., Snajdrova, R., Moore, J. C., and Bornscheuer, U. T. (2023). From nature to industry: harnessing enzymes for biocatalysis. Science 382, eadh8615. doi:10.1126/science.adh8615
Devine, P. N., Howard, R. M., Kumar, R., Thompson, M. P., Truppo, M. D., and Turner, N. J. (2018). Extending the application of biocatalysis to meet the challenges of drug development. Nat. Rev. Chem. 2, 409–421. doi:10.1038/s41570-018-0055-1
Emsley, P., Lohkamp, B., Scott, W. G., and Cowtan, K. (2010). Features and development of Coot. Acta Crystallogr. Sect. D. Biol. Crystallogr. 66, 486–501. doi:10.1107/s0907444910007493
Fellows, R., Russo, C. M., Silva, C. S., Lee, S. G., Jez, J. M., Chisholm, J. D., et al. (2018). A multisubstrate reductase from Plantago major: structure-function in the short chain reductase superfamily. Sci. Rep. 8, 14796. doi:10.1038/s41598-018-32967-1
Filling, C., Berndt, K. D., Benach, J., Knapp, S., Prozorovski, T., Nordling, E., et al. (2002a). Critical residues for structure and catalysis in short-chain dehydrogenases/reductases. J. Biol. Chem. 277, 25677–25684. doi:10.1074/jbc.m202160200
Finch, A. J., and Kim, J. R. (2018). Thermophilic proteins as versatile scaffolds for protein engineering. Microorganisms 6, 97. doi:10.3390/microorganisms6040097
France, S. P., Lewis, R. D., and Martinez, C. A. (2023). The evolving nature of biocatalysis in pharmaceutical research and development. JACS Au 3, 715–735. doi:10.1021/jacsau.2c00712
Hekkelman, M. L., de Vries, I., Joosten, R. P., and Perrakis, A. (2023). AlphaFill: enriching AlphaFold models with ligands and cofactors. Nat. Methods 20, 205–213. doi:10.1038/s41592-022-01685-y
Ji, S., Pan, Y., Zhu, L., Tan, J., Tang, S., Yang, Q., et al. (2021). A novel 7α-hydroxysteroid dehydrogenase: magnesium ion significantly enhances its activity and thermostability. Int. J. Biol. Macromol. 177, 111–118. doi:10.1016/j.ijbiomac.2021.02.082
Jumper, J., Evans, R., Pritzel, A., Green, T., Figurnov, M., Ronneberger, O., et al. (2021). Highly accurate protein structure prediction with AlphaFold. Nature 596, 583–589. doi:10.1038/s41586-021-03819-2
Kallberg, Y., Oppermann, U., Jörnvall, H., and Persson, B. (2022). Short-chain dehydrogenases/reductases (SDRs). Eur. J. Biochem. 269, 4409–4417. doi:10.1046/j.1432-1033.2002.03130.x
Karnaouri, A., Antonopoulou, I., Zerva, A., Dimarogona, M., Topakas, E., Rova, U., et al. (2019). Thermophilic enzyme systems for efficient conversion of lignocellulose to valuable products: structural insights and future perspectives for esterases and oxidative catalysts. Bioresour. Technol. 279, 362–372. doi:10.1016/j.biortech.2019.01.062
Kim, S., Ga, S., Bae, H., Sluyter, R., Konstantinov, K., Shrestha, L. K., et al. (2024). Multidisciplinary approaches for enzyme biocatalysis in pharmaceuticals: protein engineering, computational biology, and nanoarchitectonics. EES Catal. 2, 14–48. doi:10.1039/d3ey00239j
Korman, T. P., Hill, J. A., and Vu, T. N. (2004). Actinorhodin Polyketide Ketoreductase, act KR, with NADP bound. doi:10.2210/pdb1x7g/pdb
Liu, J.-S., Kuan, Y.-C., Tsou, Y., Lin, T.-Y., Hsu, W.-H., Yang, M.-T., et al. (2018). Structure-guided design of Serratia marcescens short-chain dehydrogenase/reductase for stereoselective synthesis of (R)-phenylephrine. Sci. Rep. 8, 2316. doi:10.1038/s41598-018-19235-y
McCoy, A. J., Grosse-Kunstleve, R. W., Adams, P. D., Winn, M. D., Storoni, L. C., and Read, R. J. (2007). Phaser crystallographic software. J. Appl. Crystallogr. 40, 658–674. doi:10.1107/s0021889807021206
Ming, H., Yin, Y.-R., Li, S., Nie, G.-X., Yu, T.-T., Zhou, E.-M., et al. (2014). Thermus caliditerrae sp. nov., a novel thermophilic species isolated from a geothermal area. Int. J. Syst. Evol. Microbiol. 64, 650–656. doi:10.1099/ijs.0.056838-0
Mohammadi, S., Narimani, Z., Ashouri, M., Firouzi, R., and Karimi-Jafari, M. H. (2022). Ensemble learning from ensemble docking: revisiting the optimum ensemble size problem. Sci. Rep. 12, 410. doi:10.1038/s41598-021-04448-5
Monza, E., Acebes, S., Lucas, M. F., and Guallar, V. (2017). “Directed enzyme evolution: advances and applications,” in Directed enzyme evolution: advances and applications, 257–284. doi:10.1007/978-3-319-50413-1_10
Niefind, K., Müller, J., Riebel, B., Hummel, W., and Schomburg, D. (2003). The crystal structure of R-specific alcohol dehydrogenase from Lactobacillus brevis suggests the structural basis of its metal dependency. J. Mol. Biol. 327, 317–328. doi:10.1016/s0022-2836(03)00081-0
Noey, E. L., Tibrewal, N., Jiménez-Osés, G., Osuna, S., Park, J., Bond, C. M., et al. (2015). Origins of stereoselectivity in evolved ketoreductases. Proc. Natl. Acad. Sci. U. S. A. 112, E7065–E7072. doi:10.1073/pnas.1507910112
Novoa, E. M., Pouplana, L. R., Barril, X., and Orozco, M. (2010). Ensemble docking from homology models. J. Chem. Theory Comput. 6, 2547–2557. doi:10.1021/ct100246y
Pallara, C., Cabot, D., Rivas, J., Brun, S., Seco, J., Abuasaker, B., et al. (2022). Peptidomimetics designed to bind to RAS effector domain are promising cancer therapeutic compounds. Sci. Rep. 12, 15810. doi:10.1038/s41598-022-19703-6
Penning, T. M. (2015). The aldo-keto reductases (AKRs): overview. Chemico-Biological Interact. 234, 236–246. doi:10.1016/j.cbi.2014.09.024
Qiao, L., Luo, Z., Chen, H., Zhang, P., Wang, A., and Sheldon, R. A. (2023). Engineering ketoreductases for the enantioselective synthesis of chiral alcohols. Chem. Commun. 59, 7518–7533. doi:10.1039/d3cc01474f
Ramírez, D., and Caballero, J. (2016). Is it reliable to use common molecular docking methods for comparing the binding affinities of enantiomer pairs for their protein target? Int. J. Mol. Sci. 17, 525. doi:10.3390/ijms17040525
Ramos-Martín, J., Khiari, O., Alcántara, A. R., and Sánchez-Montero, J. M. (2020). Biocatalysis at extreme temperatures: enantioselective synthesis of both enantiomers of mandelic acid by transesterification catalyzed by a thermophilic lipase in ionic liquids at 120 °C. Catalysts 10, 1055. doi:10.3390/catal10091055
Rao, S. T., and Rossmann, M. G. (1973). Comparison of super-secondary structures in proteins. J. Mol. Biol. 76, 241–256. doi:10.1016/0022-2836(73)90388-4
Sadiq, S. K., and Coveney, P. V. (2015). Computing the role of near Attack conformations in an enzyme-catalyzed nucleophilic bimolecular reaction. J. Chem. Theory Comput. 11, 316–324. doi:10.1021/ct5008845
Seco, J., Luque, F. J., and Barril, X. (2009). Binding site detection and druggability index from first principles. J. Med. Chem. 52, 2363–2371. doi:10.1021/jm801385d
Velasquez-López, Y., Tejera, E., and Perez-Castillo, Y. (2022). Can docking scoring functions guarantee success in virtual screening? Virtual Screen. Drug Docking, 1–41. doi:10.1016/bs.armc.2022.08.008
Wamser, N., Wu, H., Buono, F., Brundage, A., Ricci, F., Lorenz, J. C., et al. (2022). Discovery and process development of a scalable biocatalytic kinetic resolution toward synthesis of a sterically hindered chiral ketone. Org. Process Res. Dev. 26, 1820–1830. doi:10.1021/acs.oprd.2c00067
Wiederstein, M., and Sippl, M. J. (2007). ProSA-web: interactive web service for the recognition of errors in three-dimensional structures of proteins. Nucleic Acids Res. 35, W407–W410. doi:10.1093/nar/gkm290
Woodley, J. M. (2019). Accelerating the implementation of biocatalysis in industry. Appl. Microbiol. Biotechnol. 103, 4733–4739. doi:10.1007/s00253-019-09796-x
Keywords: ketoreductase, kinetic resolution, in silico screening, asymmetric synthesis, BioMatchMaker, biocatalysis, Thermus caliditerrae
Citation: Sosa Y, Kapur B, Hurtak J, Kingsley LJ, Wu H, Gruber S, Nar H, Khattabi S, Moral JS, Lucas MF, Martin C, Lončar N, Buono F, Pefaur N, Nixon AE and Song JJ (2024) In silico enzyme screening identifies an SDR ketoreductase from Thermus caliditerrae as an attractive biocatalyst and promising candidate for protein engineering. Front. Chem. Biol 3:1425501. doi: 10.3389/fchbi.2024.1425501
Received: 29 April 2024; Accepted: 12 June 2024;
Published: 23 July 2024.
Edited by:
Adam Offenbacher, East Carolina University, United StatesReviewed by:
Athanasios Papakyriakou, National Centre of Scientific Research Demokritos, GreecePiia Bartos, University of Eastern Finland, Finland
Copyright © 2024 Sosa, Kapur, Hurtak, Kingsley, Wu, Gruber, Nar, Khattabi, Moral, Lucas, Martin, Lončar, Buono, Pefaur, Nixon and Song. This is an open-access article distributed under the terms of the Creative Commons Attribution License (CC BY). The use, distribution or reproduction in other forums is permitted, provided the original author(s) and the copyright owner(s) are credited and that the original publication in this journal is cited, in accordance with accepted academic practice. No use, distribution or reproduction is permitted which does not comply with these terms.
*Correspondence: Laura J. Kingsley, bGF1cmEua2luZ3NsZXlAYm9laHJpbmdlci1pbmdlbGhlaW0uY29t; Hao Wu, aGFvLnd1QGJvZWhyaW5nZXItaW5nZWxoZWltLmNvbQ==
†ORCID: Yvett Sosa, orcid.org/0009-0005-9238-3348; Laura J. Kingsley, orcid.org/0000-0002-5566-3974; Hao Wu, orcid.org/0000-0003-4593-1629