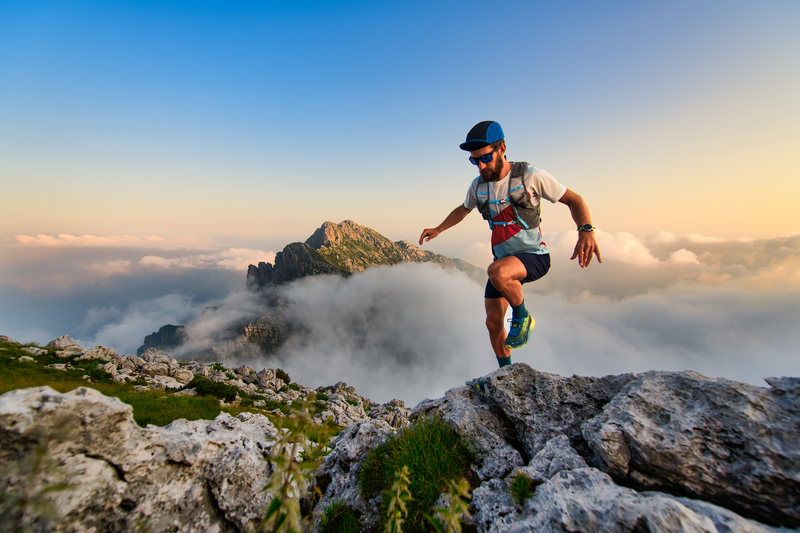
94% of researchers rate our articles as excellent or good
Learn more about the work of our research integrity team to safeguard the quality of each article we publish.
Find out more
PERSPECTIVE article
Front. Chem. Eng. , 16 September 2021
Sec. Computational Methods in Chemical Engineering
Volume 3 - 2021 | https://doi.org/10.3389/fceng.2021.727152
Digitalization in the form of Big Data and Digital Twin inspired applications are hot topics in today's bio-manufacturing organizations. As a result, many organizations are diverting resources (personnel and equipment) to these applications. In this manuscript, a targeted survey was conducted amongst individuals from the Danish biotech industry to understand the current state and perceived future obstacles in implementing digitalization concepts in biotech production processes. The survey consisted of 13 questions related to the current level of application of 1) Big Data analytics and 2) Digital Twins, as well as obstacles to expanding these applications. Overall, 33 individuals responded to the survey, a group spanning from bio-chemical to biopharmaceutical production. Over 73% of the respondents indicated that their organization has an enterprise-wide level plan for digitalization, it can be concluded that the digitalization drive in the Danish biotech industry is well underway. However, only 30% of the respondents reported a well-established business case for the digitalization applications in their organization. This is a strong indication that the value proposition for digitalization applications is somewhat ambiguous. Further, it was reported that digital twin applications (58%) were more widely used than Big Data analytic tools (37%). On top of the lack of a business case, organizational readiness was identified as a critical hurdle that needs to be overcome for both Digital Twin and Big Data applications. Infrastructure was another key hurdle for implementation, with only 6% of the respondents stating that their production processes were 100% covered by advanced process analytical technologies.
The economic value proposition of Industry 4.0 concepts and related technologies versus the traditional engineering approach to production improvements (be it capacity, quality, resource utilization, environmental footprint, or other relevant attributes) is the exploitation of information rather than the implementation of traditional "steel and concrete” solutions to realize such improvements. In bio-manufacturing operations, the tide of digitalization and Industry 4.0 has resulted in two main thrusts of developments, namely Digital Twins and data-driven process monitoring, operation, and optimization. Such concepts promise production improvements without further capital investments in equipment, as illustrated in Figure 1. However, for industry digitalization concepts to have an advantage over traditional equipment-based improvements, the associated infrastructure and the required engineering, operational and maintenance resources need to be managed. For both Digital Twins and data-driven concepts, this delicate balancing act between potential/expected benefits and the difficulty/cost of implementation plays an essential role in becoming a widely implemented set of solutions in the bio-manufacturing industry. Before delving further into this balancing act, the structures and the historical perspectives of these two key developments will be reviewed.
The concept of Digital Twins was first proposed by Greeves in 2002 for Product Lifecycle Management and was then taken up by NASA to describe the development of advanced high fidelity simulations for flight certification and flight testing (Glaessgen and Stargel, 2011). Since this initial work, many other industrial sectors have taken to this naming convention and use this term to describe the development of high-fidelity digital simulations. Examples include aircraft engine and maintenance suppliers (Tuegel et al., 2011) (e.g., GE aviation or Lufthansa Technik), discrete manufacturing suppliers (e.g., Siemens) and the automobile industry, which are now implementing Digital Twins of their assets and systems (Lukowski et al., 2019). In manufacturing operations, the concept of a Digital Twin can take three main forms (Lukowski et al., 2019): 1) A Digital Model, a high-fidelity simulation of a physical object that has been validated using data gathered. In the context of process systems engineering (PSE), this is similar to a fully mechanistic/hybrid process model that has been validated against plant data (Mauricio-Iglesias et al., 2015). A Digital Model allows engineers to conduct offline experiments and test different operational aspects, including testing new control structures. 2) A Digital Shadow, a high-fidelity simulation that can receive information from the physical object (uni-directional communication), exhibits similarities to the concept of state estimation, e.g., to the concepts practised in Kalman filters (Krämer and King, 2016; Krämer and King, 2019). A Digital Shadow allows for dynamic process forecasts to be made in real-time and for making these forecasts available to operators, e.g., through a suitable visualization interface. This flow of information allows the operators to act on the process based on the predictions made, i.e., a kind of 'operator enabled’ Digital Twin is achievable. However, the availability of real-time information-rich data is still somewhat limited in industrial fermentation operations (Lopez et al., 2020; Udugama et al., 2020). 3) Finally, a fully-fledged Digital Twin is discussed, where the Digital Model can both receive information and manipulate/alter the operation of the physical object in real-time. In conceptual terms, this is similar to Model Predictive Control, which has been used extensively for chemical and biochemical process operations (Wegerhoff and Engell, 2016). Hence, it can be argued that the Process Systems Engineering community working on bio-manufacturing operations has employed the fundamental building blocks of Digital Twins for at least a decade. Further, this definition was extended to five levels of Digital Twins (Udugama et al., 2021) that can be achieved in the domain of fermentation-based manufacturing operations (Figure 2). These levels can be described as follows:
• Level 1–3 represents Digital Models that are based on increasingly more complex and thorough process models, with level 3 representing an offline Digital Model that is validated against process data.
• Level 4 represents a Digital Shadow, which acquires and uses data in real-time, allowing the model to forecast future operations of the physical process.
• Level 5 represents a fully-fledged Digital Twin for operations where “real time” process data is used to make future predictions and calculate and execute control actions on the physical production process.
FIGURE 2. five levels of Digital Twins in process operations (Udugama et al., 2021).
The literature contains a vast number of implementations in the domain of mechanistic modelling (process models) that fit the description of a Level 1–3 Digital Twin attempt, including work such as (Xu et al., 1999; Rodríguez et al., 2006; Paramita and Kasapis, 2019). From an operational point of view, these tools are mainly used offline by engineering personnel to develop, test and understand alternative process operation strategies. In comparison, Level 4 and Level 5 Digital Twins are focused explicitly on being implemented alongside process operations. They directly influence the process operations through operations guidance via forecasting and visualization or closed-loop control. In comparison to Levels 1–3, are only a limited number of Level 4 Digital twin examples in the domain of bio-manufacturing. Lopez et al. (2020) developed a Level 4 Digital twin, while similar implementations are observed in (Krämer and King, 2016; Kager et al., 2018; Krämer and King, 2019). The development of Level 5 Digital Twins are currently underway in bio-manufacturing. Attempts in (Ehgartner et al., 2017; Mears et al., 2017; Lopez et al., 2020) have shown that even in the bio-manufacturing domain, data can be gathered, future predictions made (using a model), control actions planned and executed in real-time. However, it should be noted that these Level 5 Digital Twin examples use a significantly simplified model, which may be too simplistic to be called a Digital Model.
From an engineering effort point of view, developing a validated Digital Model for a given application already requires the allocation of significant engineering resources. For a Digital Model to accurately depict the behaviour of physical bio-manufacturing processes, key phenomena at multiple scales need to be captured. For example, an accurate representation of a fermentation process might require integrating a cell line model with a mechanistic model and a compartmental model. The cell line model then describes the reactions within a cell that carries out the fermentation. The mechanistic model describes the general time-dependent input and output relationships of the whole fermentation unit operation. Finally, a compartmental model accounts for the spatial dependencies (over time) that may occur in a vessel due to transport phenomena. As such, the creation of a Digital Model is a complex undertaking in practice. However, upgrading a Digital Model into a Digital Shadow only requires the model to operate while taking in real-time data. In contrast, a Digital Twin would require implementing a decision-making algorithm or optimization routine that calculates future movements in manipulated variables enabled by the bi-directional information flow. In practice, a Digital Shadow or Twin could use a surrogate model of a rather complex Digital Model to ensure timely execution, as practised in the implementation of concepts such as Extended Kalman Filters (Cabaneros Lopez et al., 2020).
“Big Data” and “Artificial Intelligence” are topics of great interest in the path towards Industry 4.0. The key driving force for this interest (in the process industries) is the phenomenal success of data-driven concepts in specific areas, as illustrated by AlphaGo of Google’s DeepMind, which can defeat an expert player in the two-player abstract strategy game Go (Silver et al., 2016). Similar examples can also be found in video games where Big Data-based algorithms can excel at complex multi-level games (Arzate Cruz and Ramirez Uresti, 2017).
However, a careful look into these concepts shows that (similar to Digital Twin concepts) the use of data-driven concepts in process operations is hardly new and goes back decades with established ideas such as statistical process control (MacGregor, 1988). In general, these data-driven methodologies and technologies can be divided into:
• Clustering methods can be divided into Hierarchical or Partitional methods (Jain et al., 1999). Methods include K-means (Sabbagh and Ameri, 2020; Singhal and Seborg, 2005), Density-based clustering, mean-shift clustering, BIRCH (balanced iterative reducing and clustering using hierarchies) (Thomas et al., 2018). Clustering, in general, is supported by dimensionality reduction methods.
• Classification based methods include methods based on the concepts of convolutional neural networks (Paoletti et al., 2018). As well as methods such as, support vector machines (Wang et al., 2006; Yin et al., 2014) and random forest methods (Melcher et al., 2015).
• Regression-based methods such as partial least squares (PLS) (Pontius, 2019) or recurrent neural networks such Long-Short Term Memory (LSTM) (Hochreiter and Schmidhuber, 1997) or the Gated Recurrent Unit (GRU) (Cho et al., 2014) and Autoencoders (Bengio, 2011). In addition Generative Adversarial Networks (Paoletti et al., 2018; Wu and Zhao, 2018), reinforcement learning (Aloupis et al., 2015), or other statistical process control and monitoring concepts (Spooner et al., 2018)
Big Data, which is often associated with high volume-, variety-, veracity- and velocity- (4Vs) data, offers to leverage advanced and smarter solutions to revolutionize business products, processes and services. Beyond the popular perception of more insightful data analyses, Big Data is believed to create and exploit new, challenging and more granular data sources, utilizing advanced analytics to create or promote products, processes and services, and respond rapidly to changes in the business (Davenport et al., 2012). The “volume” in 4Vs characterizes the magnitude or amount of data. At the same time, “variety” describes the organization of data such as structured (e.g., transactional data, spreadsheets, relational databases), semi-structured (e.g., log files), and unstructured (e.g., audio, video, images) (Davenport et al., 2012; Chandarana and Vijayalakshmi, 2014; Gandomi and Haider, 2015). Even though semi-structured and unstructured data are hard to analyze, they often carry a lot of information. The rate of data generation, data storage and retrieval (e.g., batch, real-time), as well as the speed of analysis and decision support, is covered by the term “velocity” (Davenport et al., 2012). The “veracity” term puts a spotlight on the possibly inaccurate data, as it could be that the analysis relies on the datasets or data resources with several degrees of precision, accuracy, and trustworthiness (Chandarana and Vijayalakshmi, 2014; Gandomi and Haider, 2015). Over time, the term “value” also appeared. It indicates the anticipated outcomes of processing and analyzing Big Data, i.e., the value added by adopting and integrating various data types and sources.
Prerequisites and methods, and applications to establish and leverage Big Data in the (bio)chemical industries were covered in great detail elsewhere (Udugama et al., 2020).
Compared to other industries, the process industries have decades of experience collecting process data from a vast number of sensors (Venkatasubramanian, 2019; Udugama et al., 2020), which is significantly longer than other manufacturing domains. More specifically, sectors such as fine chemicals, refining, and polymer production enjoyed this “data rich” environment where Real Time Optimization and Model Predictive Control practices have been carried out pervasively (Bauer and Craig, 2008). However, in bio-pharma and bio-based manufacturing sectors, the data gathered lack veracity, variety, and velocity compared to the more data-rich segments. To address this gap, there are research and development efforts underway in developing improved sensor technologies, including the concept of bio-sensors and novel measurement methodologies (Yakovleva et al., 2013; Golabgir and Herwig, 2016; Cabaneros Lopez et al., 2020). Process industries also rely on mathematical models (including industrial process simulations) for process design, optimization and control (Van Der Merwe et al., 2013; Materials, n.d.; Zhang et al., 2018). Chemical engineers are also well versed in combining these concepts to improve their capabilities, be it in using data-driven concepts for parameter estimations of mechanistic models (Sin and Gernaey, 2016). Employing the two concepts side-by-side for improved process predictions (Lopez et al., 2020) or using the underlying process understanding improves the quality of the data-driven process predictions (Gajjar et al., 2018).
Moreover, in the area of drug manufacturing, Quality by Design (QbD)—a formal framework that was adopted in the early 2000’s by regulatory agencies. QbD puts forward the ability to monitor and understand the progression (and hence the advanced control) of a process as a key concept in transforming the drug (including biologics) manufacturing processes. QbD identifies a clear need to track and explain the effect of variations on the process and, in turn to correct for these variations in real-time (Narayanan et al., 2020). The similarities between the current Digital Twin trends and the concept of QbD are apparent. From a process control point of view, the regulatory concept of “design space,” a key concept within the QbD framework, can be seen as a drive towards moving away from classical recipe-based validation, which also includes quality testing production. Instead, QbD proposes a “process control-based operational regime” where real-time corrections ensure the on-specification product falls within the “design space” of a process. The linked concept of process analytical technologies (PAT), which refers to the development and implementation of measurements (and further analysis) techniques to track critical quality parameters (Teixeira et al., 2009), is somewhat similar to the concept of data-driven process monitoring. However, in practice, neither all pharmaceutical companies nor all unit operations are capable of supporting PAT.
When analyzing the examples mentioned above, it can be observed that the current Industry 4.0 drive for chemical engineering and chemical engineers is a logical extension of the previous endeavours. To this end, the chemical engineers have a solid “theory” base and understanding of implementing these concepts. At the same time, we are currently at a stage where advances in computing power enable us to “actually” implement these Industry 4.0 concepts in a full-scale production process.
The primary focus of this manuscript will be on understanding and identifying the opportunities in the implementation of Industry 4.0 concepts in industrial bio-manufacturing processes. To this end, a targeted questionnaire was sent to industrial engineers and scientists working in the Danish bio-based manufacturing industry to identify perceived challenges and opportunities. Based on the understanding gained from the responses to the questionnaire, future perspectives building on the current trend in digitalization are provided and followed by conclusions.
Academia has produced a noticeable number of examples of Digital Twins and Big Data-based solutions in lab scale and beyond. Hence, the question can be raised about why these concepts are currently not implemented in the bio-manufacturing industry in their drive towards digitalization and Industry 4.0. To elucidate the industrial perspective on the current state and future plans for Big Data and Digital Twin-based solutions in bio-manufacturing, a survey was developed and distributed (digitally) by e-mail to known industrial practitioners in Denmark. This “closed” distribution means that the responses received are from individuals who are working in the bio-manufacturing industry day in and day out. Choosing to focus on Denmark and particularly the Region of Zealand is justified by the presence of a cluster of large world-class bio-manufacturing organizations in this area. In fact, the Danish municipality of Kalundborg, which is part of this region, is home to the largest biomanufacturing cluster in Scandinavia.
The survey consisted of the following 13 questions. In this survey, the terms digitalization and Industry 4.0 are used interchangeably as different organizations use either or both these words to describe similar programs that primarily rely on Digital Twins or Big Data-based tools.
Survey for the industrial perspectives on big data and digital twins
(Answers to this survey will be anonymous).
1–What is your current role? *
• Engineer/manager Process design
• Engineer/manager Operations
• Engineer/manager product development
• Scientist–R&D
• Other:
2–Which industry do you work in? *
• Energy/Chemical
• Food/Dairy
• Pharma
• Other:
3–In which country do you work? *
4–When did you graduate (from your highest degree)? *
• < 1970
• 1971–1980
• 1981–1990
• 1991–2000
• 2001–2010
• 2011–Now
5–Does your organization have a plan for digitalization and/or implementing Industry 4.0 concepts in the future? *
• No
• Yes
5. a If answered “Yes” in question 5–What resources both human and infrastructure have been allocated to drive this?
5. b. If answered “Yes” in question 5–Has your organization clearly identified the benefits of digitalization and/or implementing Industry 4.0 concepts for your company?
• Yes, we have a business case or cases (know exactly)
• Somewhat, we have a general idea (including company-wide directives towards digitalization)
• No
• other:
6–Do you use any advanced representations of your production line (Digital Twin, 3D model, a simulation model) that support flexible configuration and redesign, performance benchmarking or maintenance work? *
• No
• Yes (If yes, can you give us a generic example? please fill below)
• Other:
7–Do you use any advanced data-driven technique such as deep neural networks or model-based controls/operator guidance in your production line? *
• No
• Yes (If yes, can you give us a generic example? please fill below)
• Other:
8–Do you use advanced data-driven or model-based methodologies during the early-stage design, feed, detailed design, plant upgrading, and/or testing of your production processes? *
• No
• Yes (If yes, can you give us a generic example? please fill below)
• Other:
9–In your production, do you have the ability to monitor key specific variables related to the product quality (e.g., product/impurity concentration, etc.) beyond the standard process variables (temperature, pressure, flow, level, pH measurements, etc.)? *
• No
• Yes (If yes, how many variables? please list a few examples. Please fill below)
• Other:
10–In terms of implementing a digitalization solution (such as model-based forecasting or control) in a production setting, what is the most critical hurdle out of the following: *
• Lack of a business case (costs outweigh the benefits of the implementation)
• Engineering knowhow (i.e., developing the models)
• Infrastructure limitations (including lack of hardware, lack of sensors or installation difficulty)
• Organizational readiness (including lack of resources or willingness)
• Regulatory restrictions
• Employee time (i.e., manpower)
• Software limitations (including a lack of data management tools, e.g., process historians)
• other:
11–In terms of implementing a data-driven solution (such as data-based forecasting or control) in a production setting, what is the most critical hurdle out of the following: *
• Lack of a business case (costs outweigh the benefits of the implementation)
• Data know-how (i.e., training and implementing the correct model)
• Infrastructure limitations (including lack of hardware and lack of sensors or installation difficulty)
• Lack of good quality data (4V’s, veracity, velocity, variety and volume)
• Organizational readiness (including lack of resources or willingness)
• Regulatory restrictions
• Employee time (i.e., manpower)
• Software limitations (including a lack of data management tools, e.g., process historians)
• other:
12–Approximately how many units have sensors/PAT tools related to the product quality (critical quality attributes) installed? *
• 100%
• 75%
• 50%
• <25%
• Don’t know
• Other:
13–Anything we have missed concerning digitalization/AI? Any thoughts or ideas welcome to share with us.
In this section of the manuscript, we will analyze the responses received for the above 13 questions. Overall, 33 responses were received from individuals working in the Danish biotech industry. During the correspondence with the questionnaire recipients, it was communicated that only a single individual from a given sub-group would answer the questions. Therefore, these 33 responses reflect the view of 33 different sub-organizations (e.g., departments within a factory) within the Danish biotech industry. Questions 1, 3 and 4 were included in the survey to identify the respondents’ demographics and ensure no bias exists between different groups. Question 2 was a “check” question that allowed us to ensure the respondents were from the Danish biotech industry. Figure 4 illustrates the responses received for Questions 1, 3 and 4.
From analyzing Figure 3A, it can be seen that most of the participants were from the pharma and the food and BioSolutions sectors, while four participants were from the biochemical industry and a single respondent from the area of wastewater treatment. These responses are in line with the large industrial organizations found in Denmark. Figure 3B shows that most of the respondents worked in R&D, trailed by process operations and process development. In other words, most of the respondents to this survey worked in development roles instead of actual operations. According to Figure 3C, the respondents had differing experience levels, ranging from 40+ years to less than 10 years, more or less evenly distributed. Despite the respondents representing a wide range of demographics, the was no significant difference in the answers provided to the subsequent technical questions between groups.
FIGURE 3. Demographics of the respondents; (A) illustrates the industry, (B) illustrates the current role (in the industry) (C) illustrates the year of graduation (level of experience) (D) illustrates the plans for digitalization within the organization.
Figure 3D identified a clear divide between respondents where 24 out of the 33 respondents confirmed that their organization had a plan for digitalization while 9 said no such plan exists. This result shows that despite the critical importance most organizations and academia within bio-manufacturing have placed on digitalization, close to 30% of the respondents indicate that their organizations do not have a plan to implement these technologies.
For respondents who indicated that their organization had a digitalization/Industry 4.0 plan, two follow-up questions (5a and 5b) were given. These questions intended to understand the current commitment (in terms of infrastructure and human expertise) the organizations were making towards this transformation and the perceived benefits (whether or not a clear business case could be established) the organization saw in these investments. Generally, the responses to Question 5a belonged to one of the four following groups:
1. The organization had a centralized approach of forming a standalone department/projects. Response example: “Digitalization and big data departments/groups are established throughout the company to support Industry 4.0”
2. The organization took distributed approach where employees and resources were spread out across the organization. Response example: “Internal and external human resources, upgrades to DCS and Datalake technology. Investments into additional sensor technology”
3. The organization built-up infrastructure within the organization while no dedicated employees were assigned/hired. Response example: “We have infrastructure being build up and hopefully the manpower will go along”
4. The organization provided general “lip service” where the top management had indicated the “need” for transformation but hadn’t committed any resources yet. Response example: “upper management has set the direction that it is important”
Figure 4A illustrates to which category each of the responses belonged. In contrast, Figure 4B shows the state of the business case for implementation of digitalization/Industry 4.0 in the bio-manufacturing industry.
FIGURE 4. Perceived cost vs benefit of the digital transformation; (A) illustrates how resources are allocated; (B) illustrates the business case (economic benefit) of digitalization.
From analyzing Figure 4A, it can be seen that the largest subgroup of organizations deployed resources in a distributed manner where digitalization experts and infrastructure were embedded into different departments and groups across the organization. In the centralized resource approach, dedicated departments and business units were established to handle the digital transformation. These are two starkly different management approaches to tackle the challenge of raising the digitalization level of industrial processes. Which approach would prove to be the most beneficial is yet to be seen/understood. Furthermore, 2 respondents indicated that their organization was building up infrastructure, though they did not explicitly assign personnel to introduce digitalization measures. Based on further comments made by these two respondents, a conclusion can be drawn that personnel assignment would begin once infrastructure was close to completion. Meanwhile, 3 respondents said their management was talking about the importance of digitalization but had not allocated any resources. Overall, only 21 out of 33 respondents (or ∼63%) reported that engineering work in infrastructure or human capacity related to the digital transformation was being carried out within their organization.
The answers to Question 5b show that there were only 10 well-defined business cases for implementing digitalization/Industry 4.0 concepts within the respective organization. From a business point of view, this means that about 30% of the respondents indicated an application that provided a clear economic need (cost vs. benefit) for applying digitalization technologies. The other 14 respondents mainly were aware of a strategic need to invest in digitalization/Industry 4.0 concepts, e.g., “Riding the same wave as everybody else.”
From a purely economic point of view, these results show that most organizations could not see an immediate and well-defined economic benefit from investing in digitalization/Industry 4.0. Nevertheless, some organizations decided to invest into digital transformation to account for a strategic need. Hence, if this “momentum” into digitalization is to be maintained in the long term, economic benefits must become more apparent. In parallel to technical developments, this endeavour also requires techno-economic analysis concepts such as the methodology outlined in (Udugama et al., 2018) for advanced process control. The technical benefits can be converted into economic values and onwards to a business case. In addition to this, it also seems clear that there is a communication and leadership task for management in clearly conveying the expected benefits from investing in digitalization, short and long term, for the organization. For management to succeed, it must adequately analyze and identify these expected operational benefits.
Questions 6, 7, 8, and 9 were devised in the survey to identify the industrial applications of digitalization and Industry 4.0 concepts in use. These four questions were formulated to identify the following key aspects:
1. Does the organization use Digital Twins (in some form) to support plant operations?
2. Does the organization use Big Data or model-based control (in some form) to support process control or operator guidance?
3. Does the organization use Big Data or Digital Twins (including other computer-aided concepts such as CFD) in plant design and capacity improvement projects?
4. Does the organization have used advanced sensors to monitor production?
Figure 5 illustrates the answers of all 33 respondents to these mandatory questions in the survey.
FIGURE 5. The current state of digitalization technologies used in industry; (A) illustrates the use of Digital Twins during plant operations, (B) illustrates the use of Big Data analytics during plant operations, (C) illustrates the use of Digital Twins during process development, (D) illustrates the use of advanced sensors during plant operations.
From analyzing Figure 5A, it can be seen that most respondents noted that they had some form of Digital Twin in use to aid plant operations, which includes the use of a process simulation model and even a capacity model. Three respondents stated that their organization was developing this type of model. However, 11 out of 33 (33%) respondents indicated that they had no Digital Twin of any sort that could aid in process operations. The following selected answers also shed some light on the type of models that were in use:
“Many different types, 3D simulations, advanced 0D, 1D tools” “We use BIOWIN for simulation of WWTP’s, we have a project together with DTU regarding N2O emissions, we also have project together with KWB on joint control system with an industrial wastewater treatment plant” “Mechanistic modelling of selected unit operations, Flow sheet modelling to evaluate alternatives” “capacity models 3D simulations”
According to Figure 5B, 21 out of 33 respondents (∼64%) stated that they did not use any type of Big Data analytic tool or model-based control concept for direct process control or operator guidance. This means the vast majority of these organizations are not leveraging these digitalization concepts in achieving operational improvements. The following selected answers show where digitalization tools were used directly in process control or operator guidance.
“MPC and RTO for selected processes. PID tuning tool.” “real time model based optimizers/predictive maintenance.” “Predictive maintenance for example (predicting health conditions)”
On the other hand, Figure 5C shows that a majority of the organizations used Big Data analytics and/or Digital Twin concepts in plant design and revamps. However, 10 out of 33 respondents (∼30%) claimed no such tools were used in their organizations. The following selected answer illustrates where these digitalization tools were used:
“Digital twins of new equipment and processes” “We are in the very early stage of that” “Mechanistic modelling of selected unit operations, Flow sheet modelling to evaluate alternatives” “statistical modeling”
From Figure 5D, it can be seen that most respondents worked for an organization that used advanced sensors in production processes. However, 9 out of the 33 (∼30%) said their organization had no such advanced measurement capabilities, while 2 respondents stated that their organization was working on it. The following selected answers shed light on the type of advanced sensors being used:
“At line HPLC, Raman spectroscopy” “HPLC, ELISA, qPCR” “Product concentration, Impurities, Oxygen level” “concentrations, impurities but only after QC analysis has been done”
A clear trend was spotted by analyzing the raw data behind these four figures, where 9 out of 33 respondents provided negative answers to the questions related to all four subfigures. That is likely because if an organization was lagging in using Digital Twins in operations, they were also likely to lag in applying Big Data analytics and model-based approaches for process control/operator guidance. These organizations are also expected to be lagging in plant design and advanced sensor implementation. Moreover, the raw data also suggest that mechanistic modelling/Digital Twin solutions dominated Big Data analytic concepts in the industry. This is likely because process engineers are familiar with these concepts. Further, from the answers to questions 6 a and 6 b, a majority of organizations used digitalization concepts as an aid for engineers. Still, these tools were yet to be used in “real time” on the operation floor to support the production operations closely. This would require these tools to be made available for plant operators or integrated into the process control system.
Danish biomanufacturing industry includes both organizations leading the digital transformation in terms of resources, implementations, and business cases and organizations that do not even have a digitalization plan. This section of the manuscript focuses on formulating an opinion on the future developments that must take place to make digitalization and Industry 4.0 concepts standard in the bio-manufacturing industry.
Questions 10, 11 and 12 of the survey were formulated to facilitate this discussion by identifying the aim to identify.
1. the critical hurdles that must be overcome to implement Digital Twins in bio-manufacturing operations,
2. the critical hurdles to implementing Big Data analytics in bio-manufacturing, and
3. the actual state of advanced process monitoring solutions in the production line.
Figure 6 illustrates the responses to these questions. In questions 7a and 7b, the respondents were able to make multiple choices.
FIGURE 6. Critical hurdles in implementing digitalization concepts; (A) illustrates the hurdles identified for implementing Digital Twins in operations, (B) illustrates the hurdles identified for implementing Big Data analytics in operations, (C) illustrates the current state of advances process monitoring carried out during operations.
Figure 6A shows the multiple hurdles that must be overcome to implement Digital Twin concepts in operations. Organizational readiness was identified as the single biggest hurdle, followed by infrastructure, Manpower, Business case, Technical limitations, and regulations. Regarding technical limitations, a key hurdle is integrating these state of the art solutions to legacy control systems from the decades past, while a lack of a straightforward methodology to pinpoint the economic benefit of digitalization is a crucial hurdle to establishing a business case. Failure from management in showing a clear path for navigating through the regulatory requirements is a potential showstopper for biopharmaceutical manufacturing where regulations are extensive. The need to attract and retain expert-level employees to develop and maintain digitalization solutions is also a challenge that must be overcome.
Figure 6B, shows a very similar set of critical hurdles that must be overcome to implement Big Data analytics. In comparison to Figure 6A, infrastructure was identified as a key hurdle that must be overcome in applying big data analytics. This is likely due to big data analytics solutions need for upgraded sensors, data storage and handling capabilities as a prerequisite for implementation.
In Figures 6A,B, organizational readiness is identified as a significant hurdle in implementing Big Data analytics and Digital Twins, hence, in the overall digital transformation. From an organizational point of view, implementing either solution in an operations support/guidance role requires coordinating multiple departments and stakeholders within an organization, including plant operators who will be the end customer. From the perspective of the technical department (and in many cases top management), these technologies can seem like potential “game changers”, which promise to improve plant productivity (hence the operating margins) without the need for large infrastructure investment. However, for a plant operations team focused on achieving on specification operations, any new development requires an adjustment of their routines, which at first increases the chance of off-specification operations. Moreover, in many situations where productivity improvements are achieved through operational changes, production is more susceptible to process variations (Udugama et al., 2018). In addition, any new technology that is introduced leads to a learning period for the plant operators, which makes their job more difficult. Similar requirements may arise in other departments as well. Consequently, there is a strong need to explicitly take these considerations into account.
The number of respondents who saw infrastructure as a key hurdle in Digital Twins and Big Data analytics is also significant. For Digital Twins, this is likely because a Digital Twin solution, particularly for close operational support, requires integration to the operator consoles or the process control systems. This means there is a hardware requirement to enable the “real time” data acquisition, process prediction and control action calculation. Considering the technical requirements such as redundancies, this requires dedicated infrastructure commitment and qualified manpower, which can be hard to find.
In comparison, the number of respondents who saw infrastructure as a key hurdle in implementing Big Data analytics is significantly higher. This is likely due to the implementation requirement for further advanced sensors, big data repositories and computational power to carry out analytics at scale, which requires dedicated hardware. Although implementing a Digital Twin concept for operations is not trivial, the implementation of Big Data analytic tools would require significantly more hardware both in terms of data gathering and computation. This was also conveyed by a respondent as follows “Lack of good quality data (4V’s, veracity, velocity, variety and volume).”
It can also be seen that both manpower and technical limitations were listed as hurdles (Figure 6A,B). For manpower, this is due to the need for dedicated Big Data experts and process modelling experts. In Big Data, experts who have traditionally not been a part of the operational organization need to be hired. In terms of cost, infrastructure developments are likely more costly than the technical work that needs to develop these concepts. It should be noted that these are likely projects that require a high level of technical education (for example, a Master level degree or even a PhD) to perform. For a location such as Kalundborg, it is somewhat challenging to recruit and retain highly educated talents because of the relative remoteness from Copenhagen.
In terms of technical limitations, both Big Data and Digital Twin technologies require the integration of multiple databases and systems, including legacy control systems designed as standalone systems with limited “real time” data transfer capabilities.
Overall, there is a need to build up technical infrastructure further, high throughput/automated data collection (online/PAT) and human expertise in these areas. Moreover, while the industry can take care of the infrastructure build up, universities need to further improve the course offerings in the field of digitalization and focus on providing further input into industry. A respondent also remarked this as follows. “Seminar from you on Teams to make us up to date.” Collaboration between universities and industry, in developing a technical yet practice-oriented curriculum that directly addresses the needs of current industry employees with respect to digitalization and Industry 4.0 technology, is thus likely to play an important role.
In both Big Data analytics and Digital Twin solutions, the operators will have to follow a set of suggestions generated by a “computer”. This can be somewhat troublesome as the processes are often validated under a standardized operating procedure that must be followed to ensure GMP compatibility. In these situations, implementing these solutions is incompatible with following a standard operating procedure. While the concept of QbD and its updates attempt to create an avenue for this type of operation, this is not so straightforward, particularly not for the “black box” methods used in Big Data analytics. Even if these methods can be deemed acceptable, to introduce some of these applications in direct operations, a set of complex re-validation steps might be needed, which would require engagement and discussions between experts from industry, academics and regulatory agencies.
From analyzing Figure 6C, quite a straightforward conclusion can be drawn. That is, only 2 out of 33 (∼6%) respondents had 100% coverage of advanced sensors and measurements in their production line. From a practical point, the first requirement for implementing either of these concepts is the need for advanced sensors. These sensors can then be used to predict the current state of the process in “real-time”, which, in turn, enables informed operator guidance and control steps can be used taken to improve production. To this end, if ubiquitous digitalization is to be achieved in the bio-manufacturing industry, advanced sensors must cover the overall production process.
The survey results illustrate clearly that many organizations desire to implement digitalization concepts. However, the business case for the digitalization solution is often unclear. Especially the data availability (quality and quantity, among others) can be regarded as a catch 22 situation. On the one hand, one needs to elicit the value to the overall manufacturing organization before significant resources are invested in obtaining Big Data. Indeed, unlike social media, where Big Data is available virtually for free (mostly pending corresponding user consent and agreement about their data), Big Data in manufacturing has a significant cost element. As such, it requires expensive automated data collection systems (PAT sensors, collection, storage, maintenance, etc.). On the other hand, if there is no Big Data available from manufacturing, the whole concept of Big Data analytics (hence an essential pillar of digitalization) becomes irrelevant.
Hence:
• Further tools and methods must be developed to establish the business case, including the triple bottom line (Economics, society, and environment).
• Appropriate strategies to address the cost of Big Data are needed. In this regard, the increasing use of first-principles models to complement data needs for Big Data analytics could be considered and hybrid modelling concepts. In addition, flagship projects focusing on critical processing steps in manufacturing could be used to demonstrate the value before scaling up the implementation across entire manufacturing systems.
• Management must seek to develop a more comprehensive plan or roadmap for implementing digitalization in the manufacturing organization. I.e. management must formulate a fairly comprehensive strategic plan, prioritize initial targets for digitalization that provide the best cost/benefit case, and ensure that the comprehensive digitalization strategy is rolled out across multiple years, building on the initial prioritized cases towards secondary and tertiary priorities. The strategic digitalization road map itself must be flexible enough to allow for adjustments, as the organizational use-cases of digitalization, big data and data analytics become increasingly developed
Even if a business case is developed, the following represents an additional set of hurdles that must be overcome. Hence, there is a need for:
• Development of tools and methods to capture and incorporate stakeholder requirements and, in particular, develop operator-friendly digitalization solutions that will make the onboarding of this critical stakeholder easier.
• Development of the infrastructure “backbone” needed for implementing digitalization solutions.
• Educating skilled technical personnel who can support the development of digitalization solutions.
• Clearly identifying the regulator requirements such that digitalization solutions can be developed to circumvent regulatory re-validation.
• Finding the “right”-size of the developments at each stage across the multiple-year timeframe where Digitalisation can be rolled out. So, for example, building enough infrastructure to enable the initial phases of the strategic roadmap to be implemented, but not building vastly more backbone than there is a reasonable need for in the initial stages. This is particularly important given the rapid pace of hardware and software/application developments in this area.
There is still a long journey ahead to completely digitalize bio-manufacturing operations despite the initial enthusiasm and investments. Most Danish Biotec organizations have plans for digitalization, while some have no concrete digitalization plans. Even when an organization has a plan, the business case for implementing a digitalization solution seems to be often missing. Consequently, developing methodologies to identify the economic value proposition of digitalization is an immediate and burning need. Addressing organizational readiness issues as opposed to technical issues was a more direct concern. Hence, there is also a need for developing and demonstrating methodologies and concepts that can efficiently address this requirement. Finally, even for industrial organizations with a clear economic business case, there is a need to continuously invest in appropriate instrumentation such as sensors and analytical technologies to ensure all production processes can be operated with Digitalization aids.
The original contributions presented in the study are included in the article/Supplementary Material, further inquiries can be directed to the corresponding authors.
IAU, MO, PL, and CBa wrote the initial draft while CBe, JH, KG, and GS reviewed and improved the document.
CBe is the Project Director for Knowledge Hub Zealand. The Knowledge Hub Zealand partnership has partners from government and from the educational sector, as well as two commercial partners, Novo Nordisk A/S and Novozymes A/S.
The remaining authors declare that the research was conducted in the absence of any commercial or financial relationships that could be construed as a potential conflict of interest.
All claims expressed in this article are solely those of the authors and do not necessarily represent those of their affiliated organizations, or those of the publisher, the editors and the reviewers. Any product that may be evaluated in this article, or claim that may be made by its manufacturer, is not guaranteed or endorsed by the publisher.
The authors wish to acknowledge the financial support provided Novo Nordisk Foundation (AIMBio) project (Grant number NNF19SA0035474). The authors also wish to acknowledge the individuals in the Danish Biotech industry who took the time and effort to answer the survey.
Aloupis, G., Demaine, E. D., Guo, A., and Viglietta, G. (2015). Classic Nintendo Games Are (Computationally) Hard. Theor. Comput. Sci. 586, 135–160. doi:10.1016/j.tcs.2015.02.037
Arzate Cruz, C., and Ramirez Uresti, J. A. (2017). Player-centered Game AI from a Flow Perspective: Towards a Better Understanding of Past Trends and Future Directions. Entertainment Comput. 20, 11–24. doi:10.1016/j.entcom.2017.02.003
Bauer, M., and Craig, I. K. (2008). Economic Assessment of Advanced Process Control - A Survey and Framework. J. Process Control. 18, 2–18. doi:10.1016/j.jprocont.2007.05.007
Bengio, Y. (2011). “Deep Learning of Representations for Unsupervised and Transfer Learning,” in JMLR Work. Conf. Proc.
Cabaneros Lopez, P., Udugama, I. A., Thomsen, S. T., Roslander, C., Junicke, H., Iglesias, M. M., et al. (2020). Transforming Data to Information: A Parallel Hybrid Model for Real‐time State Estimation in Lignocellulosic Ethanol Fermentation. Biotechnol. Bioeng. 118, 579–591. doi:10.1002/bit.27586
Chandarana, P., and Vijayalakshmi, M. (2014). “Big Data Analytics Frameworks,” in 2014 Int. Conf. Circuits, Syst. Commun. Inf. Technol. Appl. Piscataway: IEEE, 430–434. doi:10.1109/CSCITA.2014.6839299
Cho, K., Van Merriënboer, B., Gulcehre, C., Bahdanau, D., Bougares, F., Schwenk, H., and Bengio, Y. (2014). “Learning Phrase Representations Using RNN Encoder-Decoder for Statistical Machine Translation,” in EMNLP 2014 - 2014 Conf. Empir. Methods Nat. Lang. Process. Proc. Conf. doi:10.3115/v1/d14-1179
Ehgartner, D., Hartmann, T., Heinzl, S., Frank, M., Veiter, L., Kager, J., et al. (2017). Controlling the Specific Growth Rate via Biomass Trend Regulation in Filamentous Fungi Bioprocesses. Chem. Eng. Sci. 172, 32–41. doi:10.1016/j.ces.2017.06.020
Gajjar, S., Kulahci, M., and Palazoglu, A. (2018). Real-time Fault Detection and Diagnosis Using Sparse Principal Component Analysis. J. Process Control. 67, 112–128. doi:10.1016/j.jprocont.2017.03.005
Gandomi, A., and Haider, M. (2015). Beyond the Hype: Big Data Concepts, Methods, and Analytics. Int. J. Inf. Manage. 35, 137–144. doi:10.1016/j.ijinfomgt.2014.10.007
Glaessgen, E. H., and Stargel, D. S. (2011). “The Digital Twin Paradigm for Future NASA and U.S. Air Force Vehicles,” in 53rd Struct. Struct. Dyn. Mater. Conf. Spec. Sess. Digit. Twin.
Golabgir, A., and Herwig, C. (2016). Combining Mechanistic Modeling and Raman Spectroscopy for Real-Time Monitoring of Fed-Batch Penicillin Production. Chem. Ingenieur Technik 88, 764–776. doi:10.1002/cite.201500101
Hochreiter, S., and Schmidhuber, J. (1997). Long Short-Term Memory. Neural Comput. 9, 1735–1780. doi:10.1162/neco.1997.9.8.1735
Jain, A. K., Murty, M. N., and Flynn, P. J. (1999). Data Clustering. ACM Comput. Surv. 31, 264–323. doi:10.1145/331499.331504
Kager, J., Herwig, C., and Stelzer, I. V. (2018). State Estimation for a Penicillin Fed-Batch Process Combining Particle Filtering Methods with Online and Time Delayed Offline Measurements. Chem. Eng. Sci. 177, 234–244. doi:10.1016/j.ces.2017.11.049
Krämer, D., and King, R. (2019). A Hybrid Approach for Bioprocess State Estimation Using NIR Spectroscopy and a Sigma-point Kalman Filter. J. Process Control. 82, 91–104. doi:10.1016/j.jprocont.2017.11.008
Krämer, D., and King, R. (2016). On-line Monitoring of Substrates and Biomass Using Near-Infrared Spectroscopy and Model-Based State Estimation for Enzyme Production by S. cerevisiae. IFAC-PapersOnLine. 49, 609–614. doi:10.1016/j.ifacol.2016.07.235
Lopez, P. C., Abeykoon Udugama, I., Thomsen, S. T., Bayer, C., Junicke, H., and Gernaey, K. V. (2020). Promoting the Co-utilisation of Glucose and Xylose in Lignocellulosic Ethanol Fermentations Using a Data-Driven Feed-Back Controller. Biotechnol. Biofuels 13. doi:10.1186/s13068-020-01829-2
Lopez, P. C., Udugama, I. A., Thomsen, S. T., Roslander, C., Junicke, H., Mauricio‐Iglesias, M., et al. (2020). Towards a Digital Twin: a Hybrid Data‐driven and Mechanistic Digital Shadow to Forecast the Evolution of Lignocellulosic Fermentation. Biofuels, Bioprod. Bioref. 14, 1046–1060. doi:10.1002/bbb.2108
Lopez, P. C., Udugama, I. A., Thomsen, S. T., Roslander, C., Junicke, H., Mauricio‐Iglesias, M., et al. (2020). Towards a Digital Twin: a Hybrid Data‐driven and Mechanistic Digital Shadow to Forecast the Evolution of Lignocellulosic Fermentation. Biofuels, Bioprod. Bioref. 14, 1046–1060. doi:10.1002/bbb.2108
Lukowski, G., Rauch, A., and Rosendahl, T. (2019). “The Virtual Representation of the World Is Emerging,” in Future Telco (Cham: Springer), 165–173. doi:10.1007/978-3-319-77724-5_14
Materials, V. (n.d.). VMGSim. Available at: https://virtualmaterials.com/VMGSim.
Mauricio-Iglesias, M., Gernaey, K. V., and Huusom, J. K. (2015). “State Estimation in Fermentation of Lignocellulosic Ethanol. Focus on the Use of Ph Measurements,” in 12th Int. Symp. Process Syst. Eng. 25th Eur. Symp. Comput. Aided Process Eng. Copenhagen (Copenhagen, Denmark: Denmark.). doi:10.1016/b978-0-444-63577-8.50140-6
Mears, L., Stocks, S. M., Albaek, M. O., Cassells, B., Sin, G., and Gernaey, K. V. (2017). A Novel Model-Based Control Strategy for Aerobic Filamentous Fungal Fed-Batch Fermentation Processes. Biotechnol. Bioeng. 114, 1459–1468. doi:10.1002/bit.26274
Melcher, M., Scharl, T., Spangl, B., Luchner, M., Cserjan, M., Bayer, K., et al. (2015). The Potential of Random forest and Neural Networks for Biomass and Recombinant Protein Modeling in Escherichia coli Fed‐batch Fermentations. Biotechnol. J. 10, 1770–1782. doi:10.1002/biot.201400790
Narayanan, H., Luna, M. F., Stosch, M., Cruz Bournazou, M. N., Polotti, G., Morbidelli, M., et al. (2020). Bioprocessing in the Digital Age: The Role of Process Models. Biotechnol. J. 15, 1900172. doi:10.1002/biot.201900172
Paoletti, M. E., Haut, J. M., Plaza, J., and Plaza, A. (2018). A New Deep Convolutional Neural Network for Fast Hyperspectral Image Classification. ISPRS J. Photogrammetry Remote Sensing 145, 120–147. doi:10.1016/j.isprsjprs.2017.11.021
Paramita, V. D., and Kasapis, S. (2019). Molecular Dynamics of the Diffusion of Natural Bioactive Compounds from High-Solid Biopolymer Matrices for the Design of Functional Foods. Food Hydrocolloids 88, 301–319. doi:10.1016/j.foodhyd.2018.09.007
Pontius, K. (2019). Monitoring of Bioprocesses. Opportunities and Challenges: Opportunities and Challenges. PhD thesis. Technical University of Denmark.
Rodríguez, J., Kleerebezem, R., Lema, J. M., and Van Loosdrecht, M. C. M. (2006). Modeling Product Formation in Anaerobic Mixed Culture Fermentations. Biotechnol. Bioeng. 93, 592–606. doi:10.1002/bit.20765
Sabbagh, R., and Ameri, F. (2020). A Framework Based on K-Means Clustering and Topic Modeling for Analyzing Unstructured Manufacturing Capability Data. J. Comput. Inf. Sci. Eng. 20, 011005. doi:10.1115/1.4044506
Silver, D., Huang, A., Maddison, C. J., Guez, A., Sifre, L., van den Driessche, G., et al. (2016). Mastering the Game of Go with Deep Neural Networks and Tree Search. Nature 529, 484–489. doi:10.1038/nature16961
Singhal, A., and Seborg, D. E. (2005). Clustering Multivariate Time-Series Data. J. Chemometrics 19, 427–438. doi:10.1002/cem.945
Spooner, M., Kold, D., and Kulahci, M. (2018). Harvest Time Prediction for Batch Processes. Comput. Chem. Eng. 117, 32–41. doi:10.1016/j.compchemeng.2018.05.019
Teixeira, A. P., Oliveira, R., Alves, P. M., and Carrondo, M. J. T. (2009). Advances in On-Line Monitoring and Control of Mammalian Cell Cultures: Supporting the PAT Initiative. Biotechnol. Adv. 27, 726–732. doi:10.1016/j.biotechadv.2009.05.003
Thomas, M. C., Zhu, W., and Romagnoli, J. A. (2018). Data Mining and Clustering in Chemical Process Databases for Monitoring and Knowledge Discovery. J. Process Control. 67, 160–175. doi:10.1016/j.jprocont.2017.02.006
Tuegel, E. J., Ingraffea, A. R., Eason, T. G., and Spottswood, S. M. (2011). Reengineering Aircraft Structural Life Prediction Using a Digital Twin. Int. J. Aerospace Eng. 2011, 1–14. doi:10.1155/2011/154798
Udugama, I. A., Gargalo, C. L., Yamashita, Y., Taube, M. A., Palazoglu, A., Young, B. R., et al. (2020). The Role of Big Data in Industrial (Bio)Chemical Process Operations. Ind. Eng. Chem. Res. 59, 15297–15283. doi:10.1021/acs.iecr.0c01872
Udugama, I. A., Lopez, P. C., Gargalo, C. L., Li, X., Bayer, C., and Gernaey, K. V. (2021). Digital Twin in Biomanufacturing: Challenges and Opportunities towards its Implementation. Syst. Microbiol. Biomanuf 1, 257–274. doi:10.1007/s43393-021-00024-0
Udugama, I. A., Taube, M. A., Mansouri, S. S., Kirkpatrick, R., Gernaey, K. V., Yu, W., et al. (2018). A Systematic Methodology for Comprehensive Economic Assessment of Process Control Structures. Ind. Eng. Chem. Res. 57, 13116–13130. doi:10.1021/acs.iecr.8b01883
Van Der Merwe, A. B., Cheng, H., Görgens, J. F., and Knoetze, J. H. (2013). Comparison of Energy Efficiency and Economics of Process Designs for Biobutanol Production from Sugarcane Molasses. Fuel 105, 451–458. doi:10.1016/j.fuel.2012.06.058
Venkatasubramanian, V. (2019). The Promise of Artificial Intelligence in Chemical Engineering: Is it Here, Finally? Aiche J. 65, 466–478. doi:10.1002/aic.16489
Wang, J., Yu, T., and Jin, C. (2006). On-line Estimation of Biomass in Fermentation Process Using Support Vector Machine. Chin. J. Chem. Eng. 14, 383–388. doi:10.1016/S1004-9541(06)60087-6
Wegerhoff, S., and Engell, S. (2016). Control of the Production of Saccharomyces cerevisiae on the Basis of a Reduced Metabolic Model * *This Research Is Funded by the German Ministry of Research an Technology (BMBF) in the Context of the YeastSent Project (031A301A): "Volatile Metabolites as Proxy for Metabolic Network Operation of Saccharomyces cerevisiae". IFAC-PapersOnLine. 49, 201–206. doi:10.1016/j.ifacol.2016.12.126
Wu, H., and Zhao, J. (2018). Deep Convolutional Neural Network Model Based Chemical Process Fault Diagnosis. Comput. Chem. Eng. 115, 185–197. doi:10.1016/j.compchemeng.2018.04.009
Xu, B., Jahic, M., and Enfors, S.-O. (1999). Modeling of Overflow Metabolism in Batch and Fed-Batch Cultures of Escherichia coli. Biotechnol. Prog. 15, 81–90. doi:10.1021/bp9801087
Yakovleva, M., Bhand, S., and Danielsson, B. (2013). The Enzyme Thermistor-A Realistic Biosensor Concept. A Critical Review. Analytica Chim. Acta 766, 1–12. doi:10.1016/j.aca.2012.12.004
Yin, S., Gao, X., Karimi, H. R., and Zhu, X. (2014). Study on Support Vector Machine-Based Fault Detection in Tennessee Eastman Process. Abstract Appl. Anal. 2014, 1–8. doi:10.1155/2014/836895
Keywords: digitalization, digital twin, big data, industry perspectives, applicability, roadmap
Citation: A. Udugama I, Öner M, Lopez PC, Beenfeldt C, Bayer C, Huusom JK, Gernaey KV and Sin G (2021) Towards Digitalization in Bio-Manufacturing Operations: A Survey on Application of Big Data and Digital Twin Concepts in Denmark. Front. Chem. Eng. 3:727152. doi: 10.3389/fceng.2021.727152
Received: 18 June 2021; Accepted: 30 August 2021;
Published: 16 September 2021.
Edited by:
Micaela Demichela, Politecnico di Torino, ItalyReviewed by:
Rajagopalan Srinivasan, Indian Institute of Technology Madras, IndiaCopyright © 2021 A. Udugama, Öner, Lopez, Beenfeldt, Bayer, Huusom, Gernaey and Sin. This is an open-access article distributed under the terms of the Creative Commons Attribution License (CC BY). The use, distribution or reproduction in other forums is permitted, provided the original author(s) and the copyright owner(s) are credited and that the original publication in this journal is cited, in accordance with accepted academic practice. No use, distribution or reproduction is permitted which does not comply with these terms.
*Correspondence: Krist V. Gernaey, a3ZnQGt0LmR0dS5kaw==; Gürkan Sin, Z3NpQGt0LmR0dS5kaw==
Disclaimer: All claims expressed in this article are solely those of the authors and do not necessarily represent those of their affiliated organizations, or those of the publisher, the editors and the reviewers. Any product that may be evaluated in this article or claim that may be made by its manufacturer is not guaranteed or endorsed by the publisher.
Research integrity at Frontiers
Learn more about the work of our research integrity team to safeguard the quality of each article we publish.