- 1UCIBIO, Departamento de Ciências da Vida, Faculdade de Ciências e Tecnologia, Universidade NOVA de Lisboa, Caparica, Portugal
- 2i4HB, Associate Laboratory – Institute for Health and Bioeconomy, Faculdade de Ciências e Tecnologia, Universidade NOVA de Lisboa, Caparica, Portugal
Despite extensive efforts to unravel tumor behavior and develop anticancer therapies, most treatments fail when advanced to clinical trials. The main challenge in cancer research has been the absence of predictive cancer models, accurately mimicking the tumoral processes and response to treatments. The tumor microenvironment (TME) shows several human-specific physical and chemical properties, which cannot be fully recapitulated by the conventional 2D cell cultures or the in vivo animal models. These limitations have driven the development of novel in vitro cancer models, that get one step closer to the typical features of in vivo systems while showing better species relevance. This review introduces the main considerations required for developing and exploiting tumor spheroids and organoids as cancer models. We also detailed their applications in drug screening and personalized medicine. Further, we show the transition of these models into novel microfluidic platforms, for improved control over physiological parameters and high-throughput screening. 3D culture models have provided key insights into tumor biology, more closely resembling the in vivo TME and tumor characteristics, while enabling the development of more reliable and precise anticancer therapies.
1 Introduction
In the past years, two-dimensional (2D) cell lines and animal models have been the cornerstone of biological research, deepening our understanding of the cellular pathways and molecular mechanisms of diseases, and as preclinical models for drug screening and toxicity testing (Drost and Clevers, 2018). Despite its wide applicability, 2D models are not capable of closely recapitulate all the characteristics found on the in vivo scenario, increasing the challenge of designing new target therapies (e.g., for cancer treatments). Therefore, more complex and advanced models, particularly 3D spheroids and organoids, were developed to answer the need to minimize the gap between the experimental assays and the patient’s tumor responses (Kim et al., 2020; Tang et al., 2022). Generally, 3D models offer improved resemblance to in vivo systems in terms of spatial organization, cellular interactions, gradient dynamics and drug responses (Barbosa et al., 2021). This review highlights the key aspects, advantages, limitations and main applications of each cell model. Further, it also explores the significant advancements that can be achieved by combining novel technologies with more sophisticated models to enhance the success of personalized anticancer therapies (Figure 1).
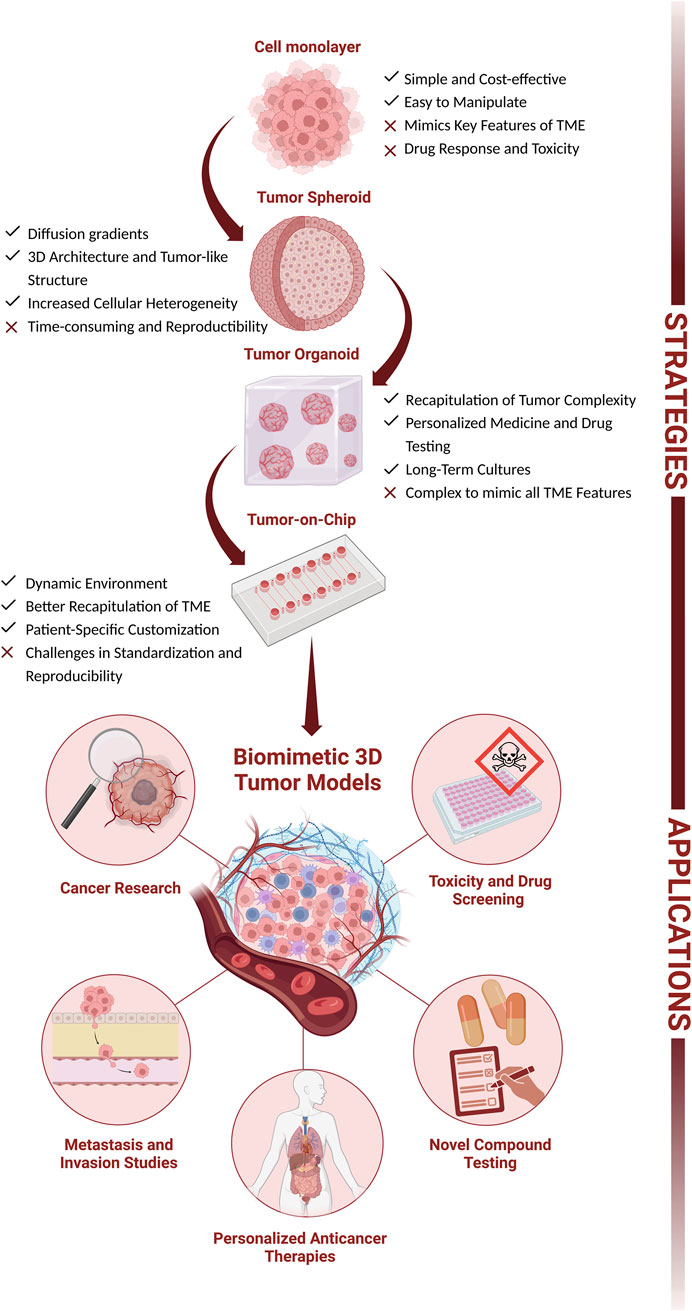
Figure 1. The complexity of cellular culture models: from 2D (cell monolayer) to 3D (tumor spheroids, tumor organoids, and Tumor-on-Chip). Advantages, hurdles, and main applications (cancer research, metastasis and invasion studies, personalized anticancer therapeutics, and drug development and screening). Figure created with BioRender.com.
According to the World Health Organization (WHO), in 2022 there were nearly 20 million new cases of cancer worldwide and, it is expected that this number increase to 35 million in 2050 (Bray et al., 2024; World Health Organization, 2024). These data highlight the urgency in understanding cancer biology and its mechanisms of initiation and progression, to develop more effective biomarkers for early diagnostics and novel therapeutic systems (Bray et al., 2024). Cancer cells are surrounded by different components (cellular and non-cellular), making up the tumor microenvironment (TME) (Figure 2) (Roma-Rodrigues et al., 2019). Regarding the cellular components of TME, these include adipocytes, lymphocytes, endothelial cells from the blood and lymphatic vasculature, pericytes, cancer-associated fibroblasts (CAFs), and tumor-associated macrophages. Within the non-cellular part of TME, the main component is the extracellular matrix (ECM), which is a complex assembly of various macromolecules, like growth factors, fibrous proteins (collagen, elastin, and fibronectin), proteoglycans, glycoproteins and cytokines (Belli et al., 2018; Roma-Rodrigues et al., 2019; Baghy et al., 2023). The main functions of ECM include the support of the 3D structure, maintenance of tissue homeostasis, and modulation of cell-to-cell and cell-matrix interactions, thereby influencing cell differentiation, survival, and proliferation (Belli et al., 2018; Baghy et al., 2023). Besides the ECM, biochemical factors, such as diffusion gradients of molecules, signaling factors, oxygen and nutrients, and biophysical factors, like shear stress and interstitial flow, also play an important role in TME (Li et al., 2023; Manduca et al., 2023).
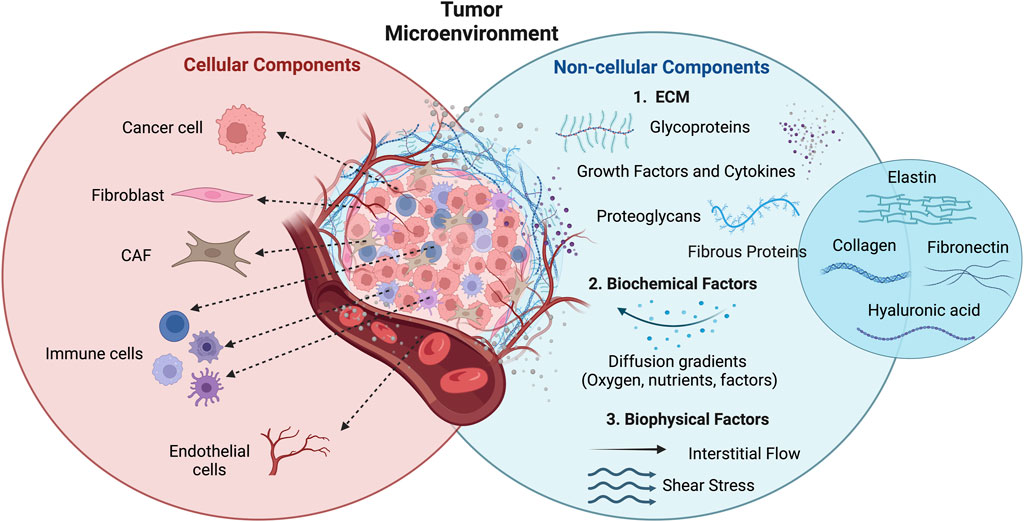
Figure 2. Representation of the TME components and their organization within the tumor mass. Cellular (cancer cells, fibroblasts, immune and endothelial cells, CAFs) and non-cellular (ECM, biochemical factors, and biophysical factors) components. Figure adapted from Benavente et al., 2020 in BioRender.com.
Overall, TME is responsible for the modulation of processes related to cell invasion and metastasis, tumor growth, immune responses, and mechanisms of drug resistance (Baghy et al., 2023). As mentioned, cancer is an intricate process that is dependent on the interaction between cancer cells and TME. Therefore, it is essential to explore new disease models capable of mimicking those interactions to provide more insights into the mechanisms of cancer progression and enhance the effectiveness of novel therapeutic screening (Burgos-Panadero et al., 2019; Babar et al., 2023).
2 Cell models
2.1 2D models
Over the past decades, new anticancer drug candidates have experienced significant attrition rates in clinical trials, largely due to their limited safety or/and lack of efficacy (Li et al., 2023). A contributing factor is the absence of disease models capable of accurately capturing the diversity and complexity of tumors. Preclinical studies, particularly those based on drug screening, heavily rely on 2D cell monolayers due to their ease of implementation, cost-effectiveness, reproducibility, versability, and compatibility with highthroughput screening (Manduca et al., 2023; Nayak et al., 2023; Tosca et al., 2023). However, as explained above and highlighted in Figure 1, these models do not fully recapitulate important features of the TME, including cell-to-cell and cell-matrix interactions, and cellular heterogeneity (Figure 2) (Jiang et al., 2023). Additionally, when cultured as monolayers, tumor cells receive nutrients and oxygen indiscriminately, which does not apply to an in vivo environment, leading to changes in gene and protein expressions, metabolism, and proliferation kinetics (Li et al., 2023; Sharma et al., 2023; Sharma et al., 2024). Conversely, animal models (being mice the most used) are more complex, displaying a complete physiological response. However, this response is murine-specific, and generally, these mice are often immunologically compromised, all of which may impair the predictiveness of the in vivo response in drug testing (Li et al., 2023). To address such concerns, a new generation of mice with human-like features has been recently developed (Li et al., 2021). Still, considerable research should be done to improve their recapitulative potential (Li et al., 2023; Sharma et al., 2023). Moreover, using animals for disease modeling and drug testing raises ethical issues, due to the growing awareness of animal suffering and sacrifice (Kiani et al., 2022). Recently, to circumvent some of the hurdles associated with these humanized mice, different groups (Al-Hamaly et al., 2023; Costa et al., 2024; Fieuws et al., 2024) have been using patient-derived xenograft (PDX) in zebrafish as models (Zebrafish Avatars) for drug screening and predicting individual treatment responses. By using zebrafish embryos, these models have several advantages, such as the speed of data acquisition, as tumor evolution and reaction to therapy can be evaluated within a timeframe that aligns with clinical decision-making and most importantly, revealing their anti-angiogenic and anti-metastatic potentials in vivo (Li et al., 2021; Al-Hamaly et al., 2023; Costa et al., 2024; Fieuws et al., 2024). Despite this enormous potential, standardization of methods and validation in larger clinical trials are needed before translation for personalized cancer medicine. While Zebrafish Avatars are making their way to the clinics, the establishment of other advanced and reliable models for accurate disease modeling, drug development/screening, and personalized medicine are also needed.
2.2 3D models
3D models are acknowledged as promising alternatives to conventional preclinical models. These advanced cell models exhibit increased recapitulative potential of the in vivo environment and are suitable for large-scale screening. These are considerably able to replicate numerous aspects found in native tumors, such as the 3D architecture of tissues, promoting the occurrence of cell-to-cell and cell-matrix interactions, cell polarization, formation of oxygen, nutrient and pH gradients, de novo ECM deposition and growth kinetics (Nayak et al., 2023; Brooks et al., 2024). Recent works reported that 3D models present similar gene expression patterns, drug resistance mechanisms, and signaling pathways activation status to those observed in vivo (Arutyunyan et al., 2023; Miller et al., 2023). These features demonstrate the potential that 3D cultures hold as improved disease models.
These advanced 3D models are generally categorized into spheroids or organoids, according to the native cell material from which the models originate. Spheroids are basic aggregates composed of cells, obtained from different sources, such as primary cells, tissue fragments, or established cell lines (Kim et al., 2023; Wang Y. et al., 2023). These cells aggregate, forming compacted and rounded structures with no specific function. Conversely, organoids derived from stem cells can create complex 3D clusters that self-organize and differentiate into tissue-specific cell types, often resulting in self-renewable tissue-like structures with similar organ functions (Fang et al., 2023). The subsequent sections offer a more in-depth explanation regarding the fundamental characteristics of these models, their main advantages, and challenges.
2.2.1 3D spheroids
Spheroids are the most endorsed 3D models in tumor research due to their simplicity. These are microsized aggregates of closely-packed cells, that can originate from a diverse array of neoplasms (Manduca et al., 2023), namely, from brain (Wanigasekara et al., 2023; Heinrich et al., 2024), breast (Ahvaraki et al., 2024; Ascheid et al., 2024), cervix (Xu et al., 2023; Kumar et al., 2024), colon and rectum (Heydari et al., 2021; Valente et al., 2023), lung (Batista et al., 2024; Li et al., 2024), pancreas (Bano et al., 2024; Struth et al., 2024), and prostate cancer (Rakhmatullina et al., 2024; Song et al., 2024). Spheroids may be composed solely of tumor cells (homotypic spheroids), or also include more types of cells (heterotypic spheroids), such as fibroblasts, immune or endothelial cells (Manduca et al., 2023). Both cells, either from established cell lines or primary tumor tissue, can be used to develop 3D tumor spheroids. Generally, cell line-derived spheroids are easily handled and more adaptable for high-throughput screening. Conversely, primary tissue-derived spheroids are more troublesome to maintain, presenting limited lifespans and variable establishment rates (Tosca et al., 2023), but can be valuable tools to understand how genotypic variations can influence response to treatment, due to inter-patient variability. Additionally, these spheroids can also be applied to personalized medicine.
Spheroids can accurately mimic several in vivo features as already reported above, making them suitable models for more reliable cancer studies (Lee et al., 2023; Li et al., 2023). Strong cell-to-cell interactions and the presence of dense ECM hamper the transport of nutrients, oxygen, and other soluble molecules, leading to differential diffusion and exchange rates, particularly in spheroids larger than 400 μm (Li et al., 2023; Manduca et al., 2023). This results in the formation of different cell layers: a proliferating external region; a transitional sheet of quiescent cells; and a hypoxic, acidic, and necrotic core, as observed in small metastatic lesions and non-vascularized tumors (Valente et al., 2023; Li et al., 2023; Manduca et al., 2023). Such heterogenous multilayered organizations had a pivotal role in endorsing the application of spheroids as preclinical models in drug development and screening. The increased ECM deposition combined with the strong cell-to-cell interactions observed in these models acts as a physical barrier that hampers drug diffusion into spheroids (Tosca et al., 2023). Moreover, senescent cells in the inner zones of spheroids exhibit increased resistance to antiproliferative drugs compared to the proliferative cells at the periphery of the spheroids (Manduca et al., 2023).
3D tumor spheroids can be generated using scaffold-free or scaffold-based approaches (Li et al., 2023). Scaffold-free models enable cells to organize themselves and secrete ECM, mimicking natural interactions. Key scaffold-free techniques comprise agitation, hanging drop, liquid overlay, and magnetic levitation (Tosca et al., 2023). Scaffold-based systems use synthetic [e.g., polyethylene glycol (PEG), polycaprolactone (PLC), poly-L-lactic acid (PLLA) and poly(lactide-co-glycolide) (PLGA)] or natural polymers (e.g., collagen, alginate, Gelatin-Methacrylate (GelMA), laminin-rich ECM, hyaluronic acid and fibrin) (Rodrigues et al., 2018), to support cell growth and mimic the ECM, improving nutrient exchange for cancer cells (Manduca et al., 2023). While scaffold-based methods enable more complex spheroid production, scaffold-free models tend to be simpler and more adaptable to high-throughput screening (Tosca et al., 2023). Additionally, advances in microtechnology and microfabrication resulted in the creation of innovative techniques, such as microfluidics and bioprinting, enabling the production of controlled architectures (e.g., micro-vessel models) that mimic the interactions and the physiological environment of the cells (Rodrigues et al., 2018; Germain et al., 2022). Recently, Liu et al (2024) described the development of a microfluidic-based chip to generate breast cancer spheroids, assess treatment response, and measure cytokine secretion, demonstrating the potential of these new methods for high-throughput generation of spheroids and treatment studies (Liu et al., 2024). Furthermore, new types of biomaterials are also insurging as the next-generation of scaffolds for in vitro cancer modeling (Rodrigues et al., 2018). As an example, methacrylate-gellan gum (Me-GG) scaffolds are being produced through bioprinting technology for 3D models of lung cancer (Villata et al., 2023).
Although tumor spheroids offer a more precise depiction of tumor biology than traditional 2D models, they still face considerable challenges as preclinical tools. One major challenge is the absence of established procedures (like SOPs) for their generation and analysis, which leads to variability in size, shape, and cellular composition across different laboratories (Manduca et al., 2023; Tosca et al., 2023). Thus, impairing the interlaboratory comparison of results and consistent reproduction of the findings. Furthermore, replicating the complex TME, including factors like vascularization and immune cell infiltration, remains an ongoing challenge in spheroid models. Despite all the relevant TME features recapitulated by spheroids, several are still not addressed. These include the 3D architecture of the original tissue, tissue deformation, and interstitial fluid pressure, all of which can greatly impact cancer cell behavior (Manduca et al., 2023).
2.2.2 3D organoids
The organoid formation process involves cell dissociation and re-aggregation in an in vitro 3D environment, forming clusters that can self-organize and differentiate in different cell types. Those clusters capture features of the in vivo microenvironment and are highly similar to actual organs (Corrò et al., 2020; Kim et al., 2020; Tang et al., 2022). Organoids may be derived from various sources, such as primary tissue biopsies, adult stem cells (ASCs, also denominated as primary tissue stem cells), embryonic stem cells (ESCs), and induced pluripotent stem cells (iPSCs). Compared with 2D and models, organoids enable increased patient specificity, being more easily handled and offering improved tumor behavior responses, which makes them advantageous for drug screening, personalized therapy, and diagnosis (Zhao Z. et al., 2022).
2.2.2.1 Formation of organoids
Even though the origin of the organoids may be different, the formation process is similar for all of them. Overall, it consists of an initial tissue dissociation into single cells or clusters, followed by their embedment into a 3D matrix (like Matrigel®) and cultured in rich media supplemented with specific growth and differentiation factors (such as epidermal growth factor, fibroblast growth factors, R-spondin, Noggin and retinoic acid). Still, the specific formulation of cell media needs to be tailored to suit the unique requirements of each cell and organoid type being modelled (Drost and Clevers, 2018; Corrò et al., 2020; Kim et al., 2020; Tang et al., 2022). Tissue dissociation methods are categorized as enzymatic or mechanical methods (Jiang et al., 2023). The enzymatic methods are the most frequently used and require an enzymatic digestion to dissolve the ECM. The enzymatic cocktail composition may differ according to the tissue type, being enzymes like collagenase, elastase, and dispase are the most commonly applied. Although mechanical digestion is faster and more cost-effective, it often resultsin higher cell death and lower cellular recovery (Richter et al., 2021). Therefore, combining both methods can yield better results (Jiang et al., 2023).
To avoid direct contact with the dish and, consequently, the adherence of the cells to their surface organoids are formed in a suspension culture. To this end, various types of techniques can be employed, which are divided into scaffold-free and scaffold-based techniques, for spheroid formation (Luo et al., 2021; Górnicki et al., 2024). Scaffold-free methods involve creating droplets of a specific culture medium that contain cells, which hang from a plate due to gravity and surface tension. On the other hand, scaffolds are biological or synthetic hydrogels that can mimic ECM. For example, Matrigel® is constituted by a jelly-like mixture of proteins and growth factors recovered from Engelbreth-Holm-Swarm (EHS) mouse sarcoma cells (Luo et al., 2021; Zhao Z. et al., 2022; Abuwatfa et al., 2024; Górnicki et al., 2024). Matrigel® provides structure and enables cell communication, recapitulating the native features of tumors (Luo et al., 2021).
Cell culture medium is also an important factor for organoid development since its previous adaptation helps the cells to perform certain functions that 2D models are not able to recapitulate (Sato et al., 2009; Corrò et al., 2020). A study developed by Li et al. (1987) reported that breast epithelial organoids could form channels and cavities when grown on specific extract and were able to synthesize and secrete the milk protein (Li et al., 1987). This work has triggered interest and paved the way for many other works in organoids from different sources.
2.2.2.2 Sources
When forming organoids, the selection of the appropriate biological material is essential to guarantee the structure and functionality of the models. Therefore, the selection is dependent on the intended application (like disease modeling, developmental biology, drug screening, or personalized medicine). The different sources for organoids generation can be classified as conventional [e.g., pluripotent stem cells (PSCs), ASCs and primary tissue biopsies] and non-conventional (liquid biopsies) (Figure 3) (Yang et al., 2019).
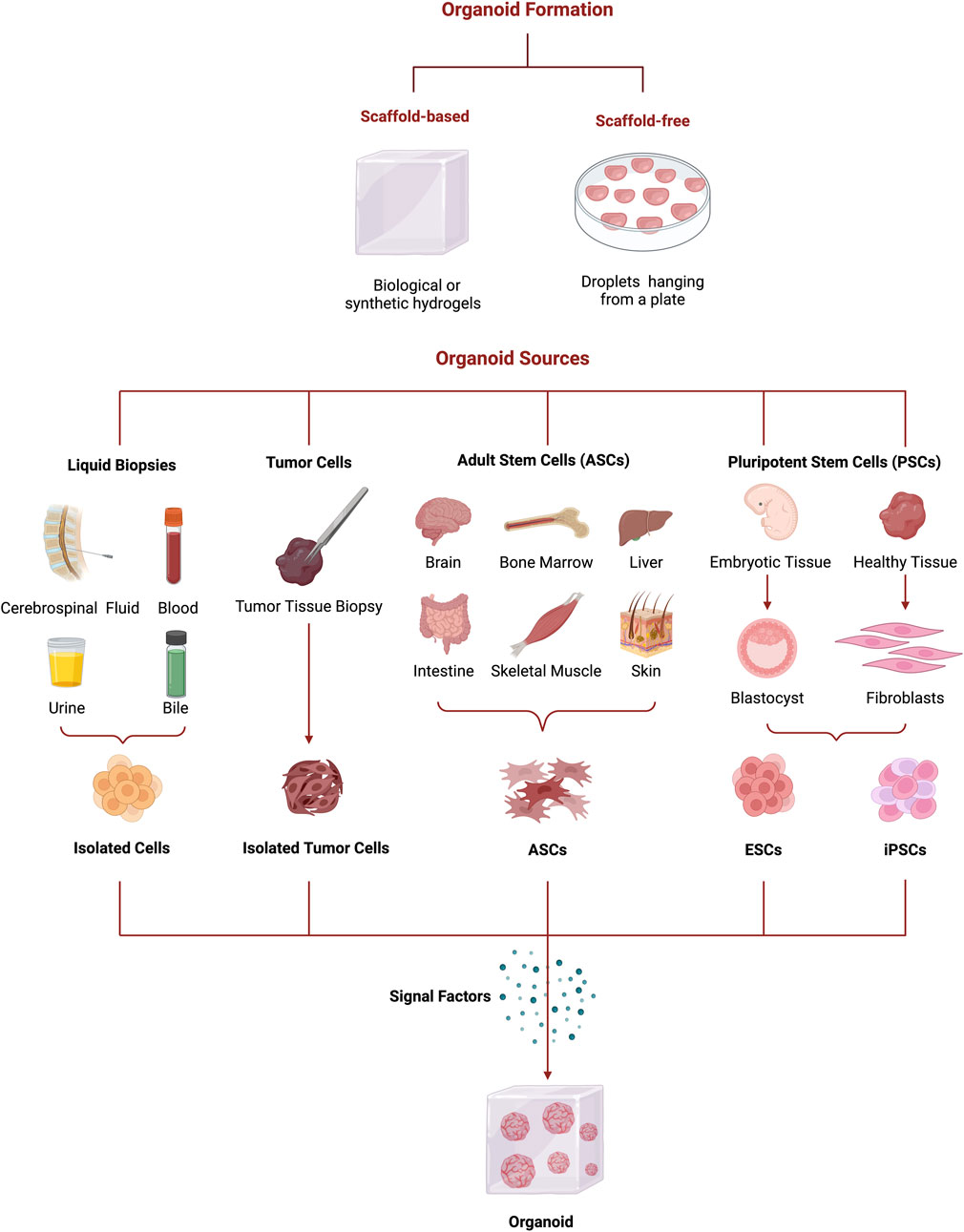
Figure 3. Schematic representation of organoids formation from several organoids’ sources (liquid biopsies, tumor biopsies, ASCs, and PSCs) to the different culture methods (scaffold-based and scaffold-free) and signal factors. Figure created with BioRender.com.
Among all sources, PSCs are the most used. Examples include ESCs, which are retrieved from the inner cell mass of blastocysts, and iPSCs, obtained by reprogramming differentiated cells back into a pluripotent state (Narsinh et al., 2011; Lei and Schaffer, 2013; Takahashi and Yamanaka, 2013). The main feature of PSCs, that makes them highly valuable for organoid formation, is their pluripotency, allowing the creation of different organoid models, from brain to liver to intestinal organoids, each accurately reflecting the complexity and cellular diversity of the respective tissues (Paşca et al., 2015; Kim et al., 2020; Shankaran et al., 2021; Ouchi and Koike, 2023). Considering this ability, organoids derived from PSCs are extensively used to model diseases, since they accurately recapitulate the pathophysiology of conditions, such as neurodegenerative diseases, liver disorders, and gastrointestinal diseases in a controlled 3D environment (Jensen et al., 2009; Spence et al., 2011; Desbordes and Studer, 2013; Schwartz et al., 2016; Amin and Paşca, 2018; Silva and Haggarty, 2020). Still, applying PSCs to organoid generation comes with several challenges, the reprograming process being the major one. Achieving specific cell types with high efficiency and reproducibility is an onerous procedure, requiring precise and tight control over the differentiation environment since it can introduce mutations and alter gene expression patterns (Takahashi and Yamanaka, 2016; Shao et al., 2017). The risk of tumorigenicity, particularly with the use of oncogenic factors like c-MYC, also poses a significant hurdle (Okita et al., 2007; Yamanaka, 2020). Furthermore, the use of ESCs raises significant ethical concerns, and iPSCs provide a more ethically acceptable alternative (Moradi et al., 2019; Rodriguez-Polo and Behr, 2022).
While PSCs are commonly used for organoid generation due to their high abundance and ability to differentiate into any cell type, ASCs are multipotent, meaning they can only differentiate into a restricted variety of cell types associated with their tissue of origin (Young and Black Jr, 2004; Sobhani et al., 2017). Despite that, they require less cellular transformation processes, allowing to generate organoids that better capture the configuration, function and cellular composition of the native tissue (Clevers, 2016; Brassard and Lutolf, 2019; Wu et al., 2023). ASCs-derived organoids have a great impact in several applications such as disease modeling, particularly in tissue-specific diseases, drug testing, and regenerative medicine, considering that they provide a high degree of tissue specificity. In addition, the use of ASCs also reduces ethical concerns associated with embryonic sources and can be patient-specific, allowing personalized medicine approaches (Jin et al., 2024).
Nevertheless, primary tissues are the best source for organoids formation with higher resemblance to the native tissue, since they allow cells to preserve their original genetic, epigenetic, and phenotypic characteristics (Huang et al., 2015; Mo and Izpisua Belmonte, 2019). The process of obtaining primary tissues typically involves invasive procedures such as needle and endoscopic biopsies or surgical resections, which afterward are dissociated and cultured as previously explained (Gao et al., 2018; Nuciforo et al., 2018; Seidlitz et al., 2019). Organoids derived from primary tissue can be composed of a mixture of epithelial, immune, stromal, and other specialized cells, depending on the tissues’ origin, leading to intrinsic heterogeneity. This feature has become relevant since it allows the development of patient-specific models to evaluate individual disease progression and develop tailored treatments accounting for the genetic and phenotypic landscape of each patient (Ooft et al., 2019; Hao et al., 2022; Zu et al., 2023; Smabers et al., 2024). Nevertheless, the implementation of organoids as pre-clinical models has been hampered by the finite proliferative capacity of primary cells, preventing expansion and long-term culture; the necessity for invasive procedures to obtain tissue samples; limited representation of rare cell types; poor functional differentiation; low phenotypic stability; and poor uniformity and consistency across organoid replicates (Jiang et al., 2017; Hu et al., 2018; Kasagi et al., 2018; Calà et al., 2023).
To surpass the hurdles associated with the obtention processes of cell samples, novel minimally invasive sources of biological material have been explored, particularly liquid biopsies (Junqueira-Neto et al., 2019; Alix-Panabieres, 2020). Liquid biopsies can be acquired through blood, with the isolation of circulating tumor cells (CTCs), or other body fluids (e.g., bile, cerebrospinal fluid, and urine) (De Mattos-Arruda et al., 2015; Reckamp et al., 2016; Alix-Panabières and Pantel, 2021; Dell’Olio et al., 2021). CTCs isolation requires highly specialized and sensitive techniques, due to the scare amount of target cells in these fluids (Cristofanilli et al., 2005; Chinen et al., 2013; Harb et al., 2013; Harouaka et al., 2013; Abdulla et al., 2018; Neves et al., 2019). Bile, endoscopic or percutaneous procedures can collect samples containing epithelial cells from the biliary tract (Saxena et al., 2015; Zhao X. et al., 2015). Cerebrospinal fluid is obtained via lumbar puncture, and urine samples are collected non-invasively (Pearce, 1994; Dörrenhaus et al., 2000; Kaeffer, 2011; Engelborghs et al., 2017). Organoids originating from those sources are extremely valuable as pre-clinical models (Drost et al., 2015; Broutier et al., 2017; Zhao et al., 2017). For instance, CTCs from blood are used as a model to the study of metastatic cancers (Bardelli and Pantel, 2017; Yang et al., 2019), bile cells allow the study of cholangiocarcinoma and cholangiopathies (Soroka et al., 2019b; Soroka et al., 2019a; Kinoshita et al., 2023), urine cells offer insights into kidney diseases and bladder cancer (Walz et al., 2023), and cerebrospinal fluid cells can be used to model brain barrier permeability and neuroepithelial barrier and secretory functions (Pellegrini et al., 2020). Despite the potential highlighted by these organoid models, the lack of standardized protocols for using liquid biopsies in organoid formation, as variations in collection, processing, and storage of samples can lead to inconsistent results, creating difficulties in reproducibility and comparison between studies (Vasseur et al., 2021; Lawrence et al., 2023; Sidaway, 2023).
In the future, novel techniques and improvements of the already established methods may expand applications of organoids as alternative cancer models. These may include advances in tissue engineering and biomaterials, progress in the microfluidic devices (Qiao et al., 2024), improvement of the methods to acquire the biological materials (especially in the cases of ASCs, primary tissues, and liquid biopsies) (Martins et al., 2021; Bex and Mathon, 2022; Shegekar et al., 2023), and standardization of procedures for organoid integration in drug screening platforms and CRISPR-based gene editing (Matano et al., 2015; Sun et al., 2019; Takeda et al., 2019; Truong et al., 2019).
2.2.2.3 Types of organoids
The types of organoids can be differentiated by their tissue origin, which can include various organs, including the gastrointestinal (GI) tract, stomach, pancreas, intestine, liver, brain, retina, kidney, and others (Figure 3) (Ashok et al., 2020). It is crucial to comprehend the microenvironment in which the initial cellular population is placed to produce viable organoids (Zhao Z. et al., 2022). GI tract organoids have additional complexity since they require a deeper understanding of homeostasis and tube’ segment development, which are formed during human development, thus involving different molecular mechanisms and active signaling pathways (Günther et al., 2022).
Intestine organoids derived from ASCs have an established long-term culture protocol, in which WNT and EGF play a key role in their maintenance and BMP promotes villi differentiation. In contrast, PSCs-derived organoids require a new protocol to support their growth and maturation (Merker et al., 2016; Zhang et al., 2021). Studies involving the grafting of gut organoids into mice demonstrated sustained integration, underscoring their tissue-repairing capabilities (Drost and Clevers, 2018). Given the molecular and physiological similarities of both stomach and intestinal, the protocols for their formation are almost identical (Merker et al., 2016). For both liver and pancreatic organoids, it has been shown that they can be generated through cycling LGR5+ cells in Matrigel® while supplemented with the appropriate cell medium (Huch et al., 2013; Hindley et al., 2016).
Brain organoids are the most difficult to produce due to the complex system composed mainly of neurons and glial cells (Tang et al., 2022). Consequently, these models are still not well established, and, for this reason, most brain studies still rely on 2D cultures or simple aggregates (Corrò et al., 2020). Recently, Lancaster et al. (2013) were able to originate “mini-brains” in Matrigel®, by supplementing the medium with Hedgehog, FGF, BMP, and WNT growth factors (Lancaster et al., 2013).
2.2.2.4 Advantages and limitations
Traditional models of human development and diseases typically involve 2D cell and tissue transplantation into in vivo models, including genetically engineered mice and PDXs. However, those alternative models do not completely recapitulate the human conditions (like tissue architecture and complexity), and some are too expensive and take too long to be produced. PDOs emerge as a viable alternative to those models (Huang et al., 2021; Taelman et al., 2022). Once the culture conditions have been optimized taking into consideration the origin of the tissue, PDOs can be easily generated by collecting samples through needle biopsies, urine, or bronchial wash material (Kaushik et al., 2018; Corrò et al., 2020; Jensen and Little, 2023). Compared to the 2D cultures, organoids can recapitulate the 3D structure, maintaining the heterogeneity and cell function, and do not induce significant genetic changes. Organoids are also less expensive and less time-consuming than PDXs and genetically engineered mouse models, therefore being more suitable for high-throughput screening (Figure 4) (Heydari et al., 2021; Huang et al., 2021).
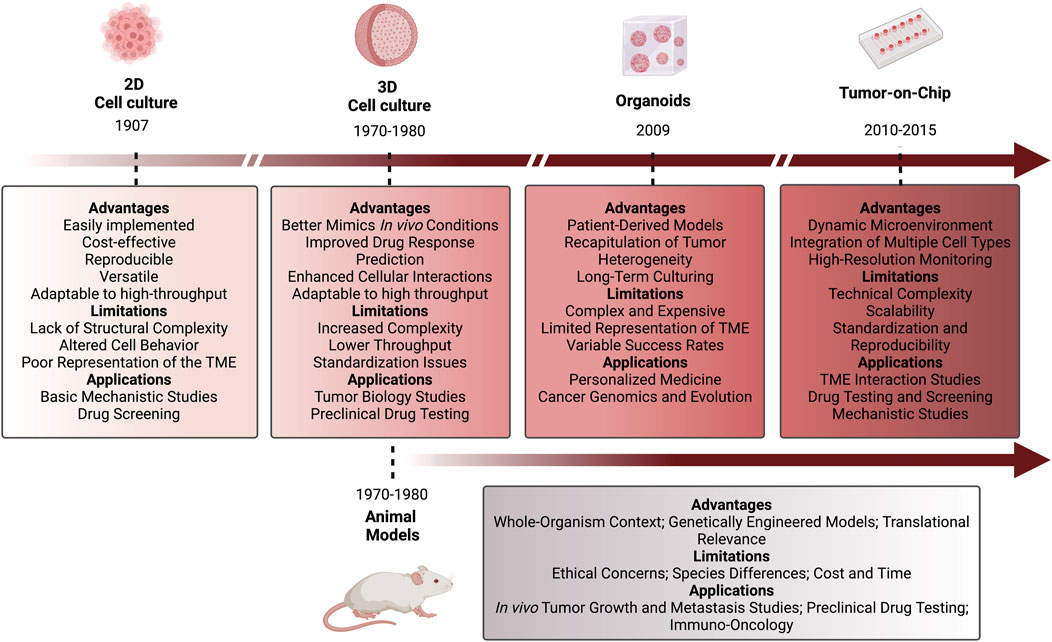
Figure 4. Chronological representation of the evolution of 2D and 3D cellular models and animal models. Description of the advantages, limitations, and applications of each represented model. 2D cell culture models were the first to be implemented in research (1907), followed by 3D cell cultures and animal models (1970–1980), then organoids (2009), and lastly Tumor-on-Chip models (2010–2015). Figure created with BioRender.com.
Regarding their limitations, organoids still fail to completely mimic all TME components and interactions, which compromises the observed outcome when, for instance, evaluating the response of a tumor to immunotherapy (Table 1; Figure 4). The different proliferation rate of each cell type and the requirement for specific growth factors and oxygen also prevents the development of more sophisticated models (Kaushik et al., 2018; Huang et al., 2021). Also, the lack of vascularization is another limitation of organoids, confining the maximum size attained (Ashok et al., 2020). Nevertheless, recent studies opened a window to overcome this organoid’s limitation. For example, Neal et al. (2018) used a gas-fluid-surface method to culture organoids above a layer of fibroblasts, prolonging the survival time of fibroblasts and immune cells (Neal et al., 2018). An alternative study focused on co-cultures of organoids and peripheral blood lymphocytes to measure the T cell-mediated killing proficiency of matched tumor organoids (Dijkstra et al., 2018).
Disadvantages in organoid systems, such as the use of Matrigel®, mice-specificity of some tumors, batch-to-batch variability, high cost, and safety issues, may complicate the development of standardized protocols, as the ones established for the 2D cultures (Kozlowski et al., 2021), which have been hampering broader application of organoids in drug development and regenerative medicine (Kim et al., 2022). Additionally, the culture medium requires a cocktail of different growth factors, which may need several optimizations, to prevent the disruption of the natural morphogen gradients of the tissues (Ashok et al., 2020; Huang et al., 2021). Therefore, the use of alternative scaffolds with well-defined composition has been investigated, such as natural matrices that would not have the batch-to-batch variability that Matrigel® has or the utilization of synthetic hydrogels, since these enable the manipulation of biochemical and biophysical matrix properties (Kozlowski et al., 2021 and for review see Ashok et al., 2020). Finally, the necessity to fine-tune several parameters contributes to the elevated cost of organoid development as well as the complexity of their scale-up (Ashok et al., 2020).
Overall, even though organoid technology is still in its early stages of maturation when compared to other models, its potential in pharmaceutical drug testing and molecular medicine is very promising.
3 Main applications of each model in cancer research
The various models described can be applied in several applications. Depending on the purpose of the study, different models may be required. The tendency is the use of more complete and complex models (e.g., PDOs and Tumor-on-Chip) to achieve the most accurate response possible. The following sections will focus on the main applications of each model in drug development and screening and personalized medicine, as well as the principal advantages and challenges that have been found over the years.
3.1 Drug development and screening
As already stated, one of the major challenges preventing the development of efficient anticancer drugs is the poor translatability of preclinical results, typically obtained from 2D cell cultures, xenografts, and animal models. Consequently, advanced 3D cell models, such as tumor spheroids and organoids, have been endorsed as alternative models to develop preclinical studies (Hirschhaeuser et al., 2010; Hamilton and Rath, 2019; Driehuis et al., 2020a; Schueler et al., 2022).
Despite their advantages in biomedical applications, the use of spheroids and organoids is still barely reported (Schueler et al., 2022). Challenges remain since these 3D models have yet to be routinely included in the drug screening procedures, and the animal models were not entirely replaced by them (Heinrich et al., 2021). The ability of tumor spheroids and PDOs to recognize promising drug candidates that failed in traditional 2D cell assays, before in vivo experiments has been widely highlighted over the last years (Hirschhaeuser et al., 2010; Pauli et al., 2017; Praharaj et al., 2018; Driehuis et al., 2019; Jacob et al., 2020; Qu et al., 2021; Qu et al., 2024; Chen et al., 2022; LeSavage et al., 2022; Cabeza-Segura et al., 2023; Wang H.-M. et al., 2023).
Lately, the most promising application of 3D organoids in cancer therapy involves drug screening of novel agents and personalized medicine. Established organoid repositories can be leveraged for screening assays, hastening the discovery of potentially effective agents from new compounds or finding alternative applications for existing drugs. Moreover, organoids improve the study of combinatory drugs and new methods to overcome drug resistance (Tosca et al., 2023).
Currently, the standard models for anticancer drug development and screening (e.g., small molecule drugs and chemotherapy agents) are 2D cancer cell lines and tumor tissue animal transplantation (e.g., xenografts) models (Kitaeva et al., 2020; Langhans, 2021; Zhang et al., 2023; Qu et al., 2024). Xenograft models are useful in drug screening since they retain tumor heterogeneity and simulate the TME. Still, they present major disadvantages like long experimental periods, high costs, and unsuitability for high-throughput screening (Gao et al., 2015; Langhans, 2021; Zhao H. et al., 2022). Traditional toxicological screening methods using 2D cultures and in vivo models often fail to predict human adverse reactions precisely. Being organ toxicity a major reason for drug failure and withdrawal, even post-approval (Raghavan et al., 2021). Hence, organoids have become invaluable in drug research and development, improving drug toxicity detection, high-throughput screening, and pharmacokinetic studies (Clevers, 2016; Boretto et al., 2019; Saito et al., 2019; Driehuis et al., 2020b; Brooks et al., 2021; Ren et al., 2022; Yuan et al., 2022; Yang and Yu, 2023).
Advances in in vitro tumor models, including 3D spheroids and organoids, have significantly hastened the drug discovery and development process (Qu et al., 2024). Some studies have shown that spheroids better recapitulate drug responses in xenograft mice compared to 2D cell cultures. However, these studies only conducted qualitative comparisons with a limited number of cell lines and drugs. A more comprehensive analysis incorporating a wider variety of cancer cell lines and anticancer agents is necessary (Broutier et al., 2017; Qu et al., 2024).
Regarding organoid models, several studies have comparatively assessed drug responses obtained in both PDOs and PDXs, with the original patients’ responses obtained in the clinical scenario, showing good consistency across models. Still, the small sample sizes, different experimental designs (e.g., drug concentrations and exposure time), variation in the protocols for PDO culture, and varying efficacy thresholds used in PDOs, PDXs, and in patients may attenuate these findings. Thus, more wide-ranging studies with quantitative data and previously established criteria are needed to accurately assess the value of PDOs as pre-clinical models (Kita et al., 2019; Qu et al., 2024).
Nevertheless, both spheroids and organoids have the potential to become standard cancer models, providing clinical data of increased relevance, and enhancing the translational effectiveness of preclinical studies. Ultimately, widening treatment options for cancer patients, while reducing the exploit of animal models (Tosca et al., 2023).
3.2 Personalized medicine
As already mentioned, personalized medicine is another promising application of 3D cultures. In the past years, significant effort has been made to develop accurate cancer models. Such was achieved by transitioning simplistic monolayer cultures to sophisticated 3D systems, mostly relying on primary tumor sources, like PDOs. Hopefully, continued improvements in tumor modeling could lead to the development of reliable 3D “patient avatars,” that might help in guiding therapeutic selection for cancer patients (Fong et al., 2017). In this regard, Pauli et al. (2017) established a precision cancer care platform, by combining whole-exome sequencing with a living biobank for high-throughput drug screenings using PDOs. Conversely, Ho et al. (2018) highlighted advancements in 3D organoid technology, showing its use in cancer and other disorders, like modeling complex hereditary diseases.
Organoids also play a significant role in the discovery of novel tumor biomarkers (Huang et al., 2020; Ukai et al., 2020; Low et al., 2021). A meta-analysis conducted by G. E Wensink in 2018, analyzed 17 studies of PDOs for testing personalized tumor response (Beutel et al., 2021). Results from those studies revealed that PDOs hold tremendous clinical value as a predictive tool for cancer patients’ personalized treatment response (Qu et al., 2024). As such, integrating these models with individual genomics data and drug development pipeline could expedite personalized medicine findings, rendering for tailored treatment regimens, with improved efficacy and reduced side effects, ultimately enhancing patient survival and quality of life (Qu et al., 2024). Supplementary Table S1 includes examples of the main applications of 3D models for cancer therapy (Tosca et al., 2023).
Although it is improbable that these 3D cancer models will fully replace animal models at this time, they are anticipated to become a crucial intermediary between 2D in vitro and in vivo models. Such has been endorsed in the latest FDA Modernization Act 2.0, which admits the use of alternative models like spheroids and organoids in place of certain animal studies, could increase their popularity and standardization [Booij et al., 2022; S.5002—117th Congress (2021–2022): FDA Modernization Act 2.0., 2022]. Altogether, using 3D models to eliminate ineffective treatments earlier could reduce the costs associated with cancer research, animal use, and ethical concerns (Qu et al., 2024).
3.3 Gene modulation and editing
PDOs with monogenic diseases can be edited to correct the disease-causing mutations. Such was proved by Schwank, G., et al (2013) while using CRISPR/Cas9 to correct a faulty cystic fibrosis transmembrane conductance regulator gene in intestinal organoids from two cystic fibrosis patients (Schwank et al., 2013; El Harane et al., 2023). Live-cell microscopy showed that the corrected organoids expanded rapidly, unlike the uncorrected controls (Schwank et al., 2013).
CRISPR/Cas9 has proven nifty for activating or deactivating tumor suppressor genes, inactivating oncogenes, and correcting disease-causing mutations. The use of this technique has significantly improved the outcomes of creating new organoid and cell line models. However, despite its promise, phenotypic rescues using CRISPR/Cas9 in human organoids remain limited. Developing transplantation techniques for genome-edited PDOs is essential for clinical applications (El Harane et al., 2023).
3.4 Immunotherapy
Dysregulations in the TME contribute to frequent relapse when conventional cancer treatments are applied. Forecasting when patients will not respond to certain therapies is essential for enhancing cancer therapy (El Harane et al., 2023). In the last years, immunotherapy has demonstrated encouraging outcomes in various cancers, such as melanoma and lung cancer. However, its effectiveness varies among patient groups due to the complex nature of the TME, which can lead to resistance to therapeutic agents (Kirkwood et al., 2012).
Utilizing 3D models for evaluating immunotherapies offers a promising alternative strategy, since CAR-T therapy has shown encouraging results in certain liquid tumors but remains less effective in solid tumors (Ma et al., 2019). As so, reliable preclinical models are needed to replicate human cell surface markers and evaluate CAR-T efficacy on these new targets (Ning et al., 2024). The co-culture model of tumor organoids and CAR-T cells offers advantages over traditional preclinical models and has been used as a supplementary approach in research. Still, the current literature indicates that this model has limitations. Recently, Mei et. al (2024) listed clinical trials involving the application of PDOs for immunotherapy registered in EudraCT and in Clinicaltrials.gov (Mei et al., 2024). For example, a system named BEHAV3D was created to investigate the interactions between immune cells and PDOs through imaging and transcriptomics (Dekkers et al., 2023; El Harane et al., 2023). BEHAV3D showed the ability to trace over 150,000 engineered T cells with PDOs and researchers used it to examine cancer metabolome-sensing TEG cells (αβ T cells engineered with a γδ TCR). The results have shown significant variation in TEG cell killing effectiveness across PDO cultures from biobanks. They also found that the molecular mechanisms and response of cellular immunotherapy varied among different PDO cultures and even among individual organoids within the same culture. This highlights the platform’s ability to capture both inter- and intra-patient heterogeneity, a major challenge in treating solid tumors. Additionally, they showed that type I INF can prime resistant organoids for TEG-mediated killing (El Harane et al., 2023).
Despite the limitations described above, organoids have been used in several completed and ongoing clinical trials. These trials aimed at establishing or improving protocols for organoid generation, developing biobanks, extending the understanding of disease-specific patterns, seeking new potential therapies, and pursuing novel personalized treatments (Supplementary Table S2) (Foo et al., 2022). All these clinical trials highlight the value of organoids as a new “golden standard” for cancer modeling. As research continues, the integration of organoids into clinical trials is likely to expand, potentially leading to more effective and tailored therapies.
3.5 Tumor-on-chip (ToC)
The leveraging of microfluidics and tissue engineering allowed us to achieve an important milestone in cancer modeling, with the development of Tumor-on-Chip (ToC) technology (Giannitelli et al., 2024). ToC is a promising candidate to replace conventional experimental models, providing a more comprehensive and realistic model by integrating physical, chemical, and biological cues of tumor development and metastasis (Dsouza et al., 2022; Liu et al., 2022). The implementation of ToC can bridge the gap between traditional cell cultures and in vivo animal models, offering unprecedented potential in the realms of drug discovery, cancer research, and personalized medicine (Lovitt et al., 2014; Riedl et al., 2017; Chaicharoenaudomrung et al., 2019; Schuster et al., 2020; Vulto and Joore, 2021).
The milestones leading to the development of ToC devices comprise advancements in microfabrication and microfluidics. ToC integrates microfluidics, biomaterials, and living cells to create micro-engineered environments that closely mimic the in vivo TME (Table 2) (Liu et al., 2021). These devices can simulate various physiological conditions, such as nutrient and oxygen gradients (Chen et al., 2011; Brennan et al., 2014), mechanical forces (Lanz et al., 2017), and cellular interactions (Menon et al., 2014; Zou et al., 2015; Manoharan et al., 2024), providing a more accurate platform to enhance our understanding of cancer biology, reduce the time and cost associated with drug development, and improve clinical outcomes by enabling more effective and personalized treatment strategies (Lovitt et al., 2014; Vulto and Joore, 2021).
The first microfluidic chip was developed in the 1980s, and the subsequent integration of living cells into these devices in the early 2000s (Whitesides, 2006). More recently, multi-organ devices are being developed by incorporating patient-derived cells and tissues, enhancing the relevance of these models for targeted medicine (Xu et al., 2016; Giannitelli et al., 2024). This combination of technologies endorsed the development of intricate platforms that replicate the dynamic environment of tissues and tumors, improving the reliability of preclinical studies (Ronaldson-Bouchard and Vunjak-Novakovic, 2018; Turetta et al., 2018).
Typically, ToC devices are fabricated using advanced microfabrication techniques, including photolithography (Xu et al., 2016), soft lithography (Xia and Whitesides, 1998; Silverio and Cardoso de Freitas, 2018), 3D printing (Lee and Cho, 2016; Yang et al., 2017; Steinberg et al., 2023) and laser ablation (Hsieh et al., 2017; Mansour et al., 2023). Each fabrication method offers unique advantages and drawbacks, making them suitable for different aspects of ToC device creation (Table 3). The choice of the technique depends on the specific requirements of the study, including precision, material compatibility, cost, and scalability. Integrating multiple techniques can often provide a balanced approach, leveraging the strengths of each to overcome individual limitations.
Materials commonly used include PDMS (Ng et al., 2002), hydrogels (Hoch et al., 2013), and other biocompatible polymers (Ren et al., 2013; Terrell et al., 2020). In the same manner as the fabrication methods, each material has its advantages and limitations, affecting their suitability for different applications. Generally, PDMS is the most popular material for its flexibility and ease of fabrication, but its chemical nature leads to the absorption of small hydrophobic molecules, narrowing its application in drug studies (McDonald and Whitesides, 2002; Auner et al., 2019). Conversely, PMMA and PC provide better mechanical strength but lack gas permeability, limiting the oxygen supply to the cells (Altmann et al., 2011; Palacio-Castañeda et al., 2020; Rodriguez et al., 2020; Bērziņa et al., 2021). Hydrogels offer a realistic 3D environment but have stability and reproducibility issues (Song et al., 2014; Chen et al., 2020; Terrell et al., 2020). Glass provides excellent optical clarity but is brittle (Aralekallu et al., 2023), while paper-based materials are low-cost and easy to handle but have poor optical properties for imaging, are less durable and offer less control over fluid flow (Ren et al., 2013; Ren et al., 2014). More recently, PS has also been explored due to its superior properties (Berthier et al., 2012). Among them, the ease of fabrication with more consistent and predictable surface properties than PDMS and PMMA, while allowing effortless molding and surface-treatment to enhance cell adhesion or create specific patterns for cell growth. The excellent optical transparency, similar to glass, makes it suitable for high-resolution imaging and microscopy. Additionally, PS also shows high chemical resistance, comparable to PMMA and PC, outperforming PDMS, which can absorb small hydrophobic molecules. Overall, the choice of material often depends on the specific requirements of the experiment and the desired balance between ease of use, biocompatibility, and mechanical properties (Ren et al., 2013).
These materials and techniques can be incorporated into several designs to replicate the architecture and functionality of tumors (Ronaldson-Bouchard and Vunjak-Novakovic, 2018). The design and functionality of the devices vary with the desired application and tumor models in study but generally include features such as microchannels, wells, and chambers to culture cells and tissues (Whitesides, 2006). Additionally, devices with increased complexity can be developed by integrating sensors, controllers, and imaging modules (Table 4) (Ronaldson-Bouchard and Vunjak-Novakovic, 2018). Altogether, this allows for precise control over the architecture and functionality of the chips.
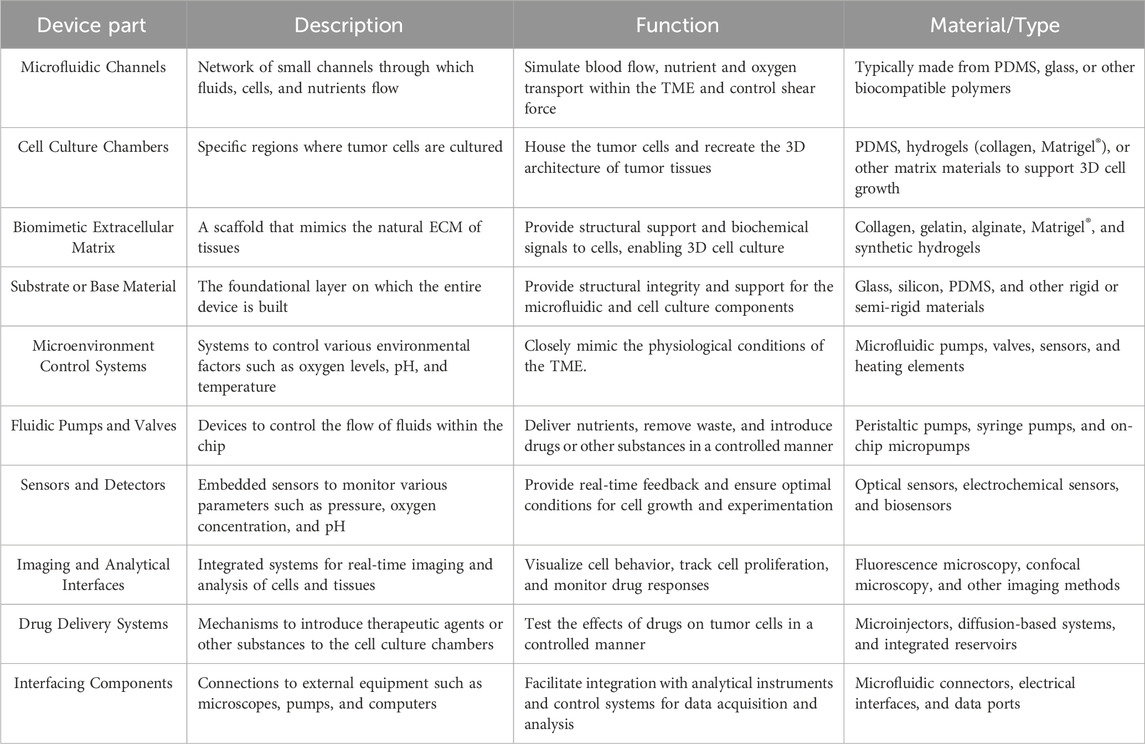
Table 4. Description, function, and used materials of components of ToC devices that can be incorporated into a whole system.
Microfluidics in ToC devices allows for precise fluid control, enabling the recreation of TME conditions, such as perfusion rates for shear stress, nutrient gradients, differential diffusion rates of stimuli and cellular factors, and oxygen levels (Antoine et al., 2015; NDong et al., 2015).
3.5.1 Applications in cancer modeling and drug screening
The ability of ToC to replicate the key features of TME (Mastrangeli et al., 2019; Mittal et al., 2019; Park et al., 2019; Thompson et al., 2020; Vulto and Joore, 2021; Dsouza et al., 2022; Li et al., 2023), holds great potential in disease modeling, drug screening, and personalized medicine. These devices enable researchers to conduct experiments under physiologically relevant conditions, accelerating the drug discovery process and enhancing the predictive power of preclinical studies (Table 5) (Hachey and Hughes, 2018).
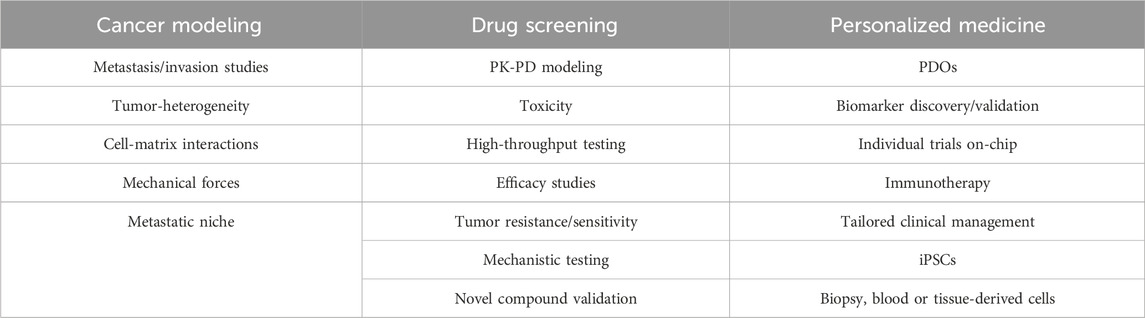
Table 5. Main applications of ToC in the fields of cancer modeling, drug screening, and personalized medicine.
Regarding ToC devices applied for cancer modeling, most studies are focused on assessing the impact of TME in metastasis (Portillo-Lara and Annabi, 2016; Hachey and Hughes, 2018), invasion processes (Wong et al., 2017), tumor heterogeneity (Acosta et al., 2014) and cell-matrix interactions (Zervantonakis et al., 2012; Zhao Y. et al., 2015). Such studies are performed by controlling different physiological parameters such as hypoxic gradients, ECM composition, and shear force. As models, co-cultures of cancer cells with inflammatory cells (e.g., macrophages), CAFs, and endothelial cells are typically performed in ToC applied to cancer modeling given its pivotal role in the onset of tumor progression (Sung et al., 2011; Zervantonakis et al., 2012; Sleeboom et al., 2018).
Besides cancer modeling, ToC devices can also be designed to offer a high-throughput platform for drug screening, allowing researchers to test multiple drugs and dosages, simultaneously. This is particularly useful for identifying potential drug candidates in a quick and automated manner. In fact, ToC has been described as a more reliable alternative for drug screening (Sun et al., 2017; Zhang et al., 2018; Dhiman et al., 2019), new drug validation (Gao et al., 2021), resistance (Rosa et al., 2014; Patel et al., 2015) and toxicity studies (Chen et al., 2015; Jeong et al., 2016). Several studies in the fields of drug screening, biomarker discovery, and immunotherapy testing have been conducted using ToC (Table 6). The results showed improved predictability, ability to high-throughput, and better insights regarding the mechanisms of drug activity and tumor responses (Mazzocchi et al., 2018; Chi et al., 2020; Skardal et al., 2020). Overall, this highlights the potential of ToC platforms for drug screening and improving the success rate of new compounds when advanced to clinical trials (Kim et al., 2012; Santo et al., 2017; Zhang et al., 2018; Dhiman et al., 2019).
3.5.2 Applications in personalized therapy and precision medicine
ToC technology enables the customization of devices to reflect the unique characteristics of individual patient tumors, rendering it extremely advantageous for personalized medicine (Hachey and Hughes, 2018). The former aims to identify the most effective and least toxic therapy for each patient, unlike the “one-size-fits-all” approach used on standard oncology drugs, which often results in low efficacy and significant side effects (Ashley, 2016). This low efficacy hampers FDA approval of new drugs, and even approved drugs typically offer only marginal survival benefits (DiMasi et al., 2016).
Personalized medicine emphasizes the need for molecular testing on patient-derived samples to identify specific genomic aberrations and categorize cancer subtypes based on population-level genetics (Izumchenko et al., 2017). These tests allow informed treatment decisions, treating patients based on the most prominent characteristics of their tumor cohort (Chen et al., 2016; Izumchenko et al., 2017; Hachey and Hughes, 2018). However, accurately modeling the key characteristics of actual tumors has been challenging due to the limitations of established cancer cell lines (Ben-David et al., 2017).
Effective targeted therapies require models with high clinical predictive value, which is where ToC technology excels. By using patient-derived cells and tissues, ToC devices create personalized models for testing therapeutic responses and tailoring treatment strategies (Mathur et al., 2015; Huebsch et al., 2016; Takebe et al., 2017). Chip-based tumor models incorporating tissue samples from resistant sites, like primary and metastatic lesions are invaluable for studying tumor evolution and developing treatments that address tumor heterogeneity (Lancaster and Knoblich, 2014; van de Wetering et al., 2015; Bruna et al., 2016; Rios and Clevers, 2018).
Several cell sources can be exploited in chip-based personalized medicine, including primary cells from surgical resections, biopsies, aspirates, and blood samples (Papapetrou, 2016). From stem cell advances, organoid technology was developed, allowing it to retain genetic heterogeneity and mimic in vivo treatment responses of tumors. Still, organoids lack key anatomical features of tumors, like tissue-tissue interfaces, vascular compartments, dynamic fluid flow, and mechanical forces. Integrating organoids into chip-based models may better mimic organ-specific structures and gene expression (Lancaster and Knoblich, 2014; Rios and Clevers, 2018; Vlachogiannis et al., 2018).
Alternatively, cancer stem cells can be selected and differentiated on-chip, allowing to recreate native cell populations. This approach allows testing drugs on both healthy and tumor tissues from the same patient, which is ideal for accurate cancer modeling, drug testing and detecting treatment response variants (Burridge et al., 2016; Fong et al., 2016; Ronaldson-Bouchard and Vunjak-Novakovic, 2018). Nevertheless, generating healthy organoids for drug toxicity screening is impractical, requiring the use of patient-specific iPSCs (Hachey and Hughes, 2018).
4 Chips for the future
To overcome the current barriers in personalized medicine, ToC systems could be applied to cultivate patient’s cells (Haque et al., 2022; Zeng et al., 2023; Maulana et al., 2024). This approach allows high-throughput drug testing directly on an “avatar-on-a-chip,” predicting the patient’s response to various therapies. The results from these models can guide oncologists to the most effective treatments, a concept called two-way personalized medicine. This method aims to identify the best therapeutic regimen for a patient’s specific cancer before any treatment begins, offering a personalized drug screening tool for clinical use (Supplementary Table S2) (Kang et al., 2008; Caballero et al., 2017).
Individualized tumor chips can also facilitate “micro”-clinical trials, establishing multiple patient-specific tumor chips using cells and materials derived from each patient. These in vitro trials enable the testing of various drug doses and schedules, guiding treatment timing, and combination therapies, and discovering new agents (Supplementary Table S2). Drugs approved for specific cancers or those not traditionally used for cancer can be tested and potentially repurposed based on the results obtained on-chip, increasing treatment options and enhancing our understanding of cancer resistance mechanisms (Esch et al., 2015; Caballero et al., 2017).
ToC technology has the potential to transform oncology drug development and clinical management by enabling the sorting of patients based on individual characteristics without large-scale clinical trials, reducing human risk. It also permits therapeutic screening for patient groups unsuitable for standard trials, such as those with comorbidities, rare cancers, recurrent diseases, or pediatric patients. High-throughput experiments, combined with molecular testing, can elucidate gene-drug interactions, stratify tumor subtypes, and inform clinical trials (Supplementary Table S2), allowing for more precise patient categorization based on TME characteristics (Peterson and Houghton, 2004; Izumchenko et al., 2016; Izumchenko et al., 2017; Hachey and Hughes, 2018).
4.1 Challenges and considerations
ToC technology is extremely valuable for personalized medicine, requiring only a few patient cells and providing rapid and automated results within a clinically relevant timeframe. Even so, there are some challenges requiring thoughtful attention, such as the difficulty in generating primary cultures, the limited availability of viable patient-derived cells post-analysis, and the need for homogeneous replicates to represent tumor heterogeneity for comparative studies (Bhatia and Ingber, 2014; Dhiman et al., 2019). Additionally, regulatory and logistical hurdles associated with the use of patient-derived cells raise ethical considerations, including issues related to informed consent and privacy. Several other technical challenges exist, such as the complexity of device fabrication; the need for standardized protocols to ensure the scalability and reproducibility of the devices; the lack of laboratory frameworks for integrating multiple cell types and components; and the intellectual property aspects associated with the fabrication and commercialization of the devices (Huh et al., 2013; Edington et al., 2018).
4.2 Future directions and innovations
Before ToC technology can be widely adopted in precision medicine, it must overcome those challenges and deliver consistent results. Given its interdisciplinary nature, researchers, physicians, and regulatory bodies need to make a joint effort to address the remaining challenges that have been preventing ToC models from becoming the future of cancer research and personalized medicine.
To do so, studies using ToC devices must be performed to validate the clinical relevance of these chip-based models as translational tools (Bhatia and Ingber, 2014; Dhiman et al., 2019). For that, co-clinical trials should be conducted, comparing drug responses in ToC models with patient outcomes. Drug manufacturers and research centers can integrate in vitro studies using patient-derived biopsy tissue with in vivo trials, demonstrating the potential of ToC to improve drug development and patient treatment success. Implement accurate phenotypic and genotypic profiling to ensure ToC models closely resemble the original patient tumors. Additionally, establishing biobanks of human primary tissues and fostering cooperation among investigators can enhance the use of chip-based models (Supplementary Table S2).
Given the fast pace of engineering, emerging technologies such as advanced microfabrication techniques, nanotechnology, and organ-on-chip platforms are poised to enhance the capabilities of ToC devices. Also, the integration of artificial intelligence (AI) and machine learning (ML) with ToC technology offers significant potential for data analysis and interpretation. AI and ML can help identify patterns, predict outcomes, and optimize experimental designs, thereby accelerating research and improving therapeutic strategies.
5 Conclusion
Developing effective anticancer therapies remains challenging due to the limitations of traditional models in accurately mimicking human tumor biology and treatment responses. Conventional 2D cell cultures and animal models fall short in replicating the complex TME, essential for understanding tumor behavior and therapeutic outcomes. This has led to the rise of advanced in vitro models like tumor spheroids and organoids, which better represent the 3D structure and biological complexity of in vivo tumors. These 3D models, by closely mimicking the TME, have been providing key insights into tumor biology and improving drug response predictability, thus facilitating the development of more reliable anticancer therapies. The integration of these models into microfluidic platforms further enhances their utility by allowing better control over physiological conditions and enabling high-throughput screening.
Effective targeted therapies require models with high clinical predictive value, which is where ToC technology excels. ToC devices use patient-derived cells and tissues to create personalized models for testing therapeutic responses and tailoring treatment strategies. These models, incorporating tissue samples from primary and metastatic lesions, are invaluable for studying tumor evolution and developing treatments that address tumor heterogeneity. ToC systems can be applied to cultivate patient cells, allowing high-throughput drug testing on an “avatar-on-a-chip” model, predicting patient responses to therapies. This two-way personalized medicine approach aims to identify the best therapeutic regimen for a patient’s specific cancer before treatment begins. Individualized tumor chips can also facilitate “micro”-clinical trials, testing various drug doses and schedules, and guiding treatment timing and combination therapies. However, the successful implementation of these models faces several challenges, including the need for standardized protocols, overcoming technical limitations, and addressing ethical and regulatory concerns.
Continuous advancements in microfabrication, tissue engineering, and the integration of artificial intelligence and machine learning are expected to enhance the capabilities of these models. These innovations will improve the accuracy, functionality, and scalability of 3D cancer models, accelerating their adoption in preclinical research and personalized medicine.
In conclusion, the development and exploitation of tumor spheroids, organoids, and ToC technology represent significant advancements in cancer modeling. These systems grant a more precise depiction of human-derived tumors, providing a powerful platform for drug development and personalized therapeutic strategies. As technology evolves, these models are poised to play a crucial role in the future of cancer research, leading to more effective treatments and improved patient outcomes.
Author contributions
SC: Formal Analysis, Investigation, Methodology, Validation, Visualization, Writing–original draft, Writing–review and editing. BO: Formal Analysis, Investigation, Methodology, Validation, Visualization, Writing–original draft, Writing–review and editing. RV: Formal Analysis, Investigation, Methodology, Validation, Visualization, Writing–original draft, Writing–review and editing. DF: Formal Analysis, Investigation, Methodology, Validation, Visualization, Writing–original draft, Writing–review and editing. AL: Formal Analysis, Investigation, Methodology, Validation, Visualization, Writing–original draft, Writing–review and editing. PB: Conceptualization, Funding acquisition, Project administration, Supervision, Validation, Writing–review and editing. AF: Conceptualization, Funding acquisition, Investigation, Project administration, Supervision, Validation, Writing–review and editing.
Funding
The author(s) declare that financial support was received for the research, authorship, and/or publication of this article. This work was co-financed by national funds from FCT - Fundação para a Ciência e a Tecnologia, I.P., in the scope of the project UIDP/04378/2020 (10.54499/UIDP/04378/2020) and UIDB/04378/2020 (10.54499/UIDB/04378/2020) of the Research Unit on Applied Molecular Biosciences - UCIBIO and the project LA/P/0140/2020 of the Associate Laboratory Institute for Health and Bioeconomy - i4HB, project NANOHEAT (DOI 10.54499/2022.04315.PTDC) and doctoral grants 2021.08629.BD (SC), 2020.07660.BD (BO), 2022.09845.BD (RV), 2020.06599.BD (DF) and 2022.12161.BD (AL).
Acknowledgments
Figures were created with BioRender.com.
Conflict of interest
The authors declare that the research was conducted in the absence of any commercial or financial relationships that could be construed as a potential conflict of interest.
The author(s) declared that they were an editorial board member of Frontiers, at the time of submission. This had no impact on the peer review process and the final decision.
Generative AI statement
The author(s) declare that no Generative AI was used in the creation of this manuscript.
Publisher’s note
All claims expressed in this article are solely those of the authors and do not necessarily represent those of their affiliated organizations, or those of the publisher, the editors and the reviewers. Any product that may be evaluated in this article, or claim that may be made by its manufacturer, is not guaranteed or endorsed by the publisher.
Supplementary material
The Supplementary Material for this article can be found online at: https://www.frontiersin.org/articles/10.3389/fcell.2024.1507388/full#supplementary-material
References
Abdulla, A., Liu, W., Gholamipour-Shirazi, A., Sun, J., and Ding, X. (2018). High-throughput isolation of circulating tumor cells using cascaded inertial focusing microfluidic channel. Anal. Chem. 90, 4397–4405. doi:10.1021/acs.analchem.7b04210
Abuwatfa, W. H., Pitt, W. G., and Husseini, G. A. (2024). Scaffold-based 3D cell culture models in cancer research. J. Biomed. Sci. 31, 7. doi:10.1186/s12929-024-00994-y
Acosta, M. A., Jiang, X., Huang, P.-K., Cutler, K. B., Grant, C. S., Walker, G. M., et al. (2014). A microfluidic device to study cancer metastasis under chronic and intermittent hypoxia. Biomicrofluidics 8, 054117. doi:10.1063/1.4898788
Ahvaraki, A., Gheytanchi, E., Behroodi, E., Latifi, H., Vakhshiteh, F., Bagheri, Z., et al. (2024). Advanced co-culture 3D breast cancer model to study cell death and nanodrug sensitivity of tumor spheroids. Biochem. Eng. J. 209, 109400. doi:10.1016/j.bej.2024.109400
Al-Hamaly, M. A., Turner, L. T., Rivera-Martinez, A., Rodriguez, A., and Blackburn, J. S. (2023). Zebrafish cancer avatars: a translational platform for analyzing tumor heterogeneity and predicting patient outcomes. Int. J. Mol. Sci. 24, 2288. doi:10.3390/ijms24032288
Alix-Panabieres, C. (2020). Perspective: the future of liquid biopsy. Nature 579, S9. doi:10.1038/d41586-020-00844-5
Alix-Panabières, C., and Pantel, K. (2021). Liquid biopsy: from discovery to clinical application. Cancer Discov. 11, 858–873. doi:10.1158/2159-8290.CD-20-1311
Altmann, B., Steinberg, T., Giselbrecht, S., Gottwald, E., Tomakidi, P., Bächle-Haas, M., et al. (2011). Promotion of osteoblast differentiation in 3D biomaterial micro-chip arrays comprising fibronectin-coated poly(methyl methacrylate) polycarbonate. Biomaterials 32, 8947–8956. doi:10.1016/j.biomaterials.2011.08.023
Amin, N. D., and Paşca, S. P. (2018). Building models of brain disorders with three-dimensional organoids. Neuron 100, 389–405. doi:10.1016/j.neuron.2018.10.007
Antoine, E. E., Vlachos, P. P., and Rylander, M. N. (2015). Tunable collagen I hydrogels for engineered physiological tissue micro-environments. PLoS One 10, e0122500. doi:10.1371/journal.pone.0122500
Aralekallu, S., Boddula, R., and Singh, V. (2023). Development of glass-based microfluidic devices: a review on its fabrication and biologic applications. Mater Des. 225, 111517. doi:10.1016/j.matdes.2022.111517
Arutyunyan, I. V., Soboleva, A. G., Kovtunov, E. A., Kosyreva, A. M., Kudelkina, V. V., Alekseeva, A. I., et al. (2023). Gene expression profile of 3D spheroids in comparison with 2D cell cultures and tissue strains of diffuse high-grade gliomas. Bull. Exp. Biol. Med. 175, 576–584. doi:10.1007/s10517-023-05906-y
Ascheid, D., Baumann, M., Pinnecker, J., Friedrich, M., Szi-Marton, D., Medved, C., et al. (2024). A vascularized breast cancer spheroid platform for the ranked evaluation of tumor microenvironment-targeted drugs by light sheet fluorescence microscopy. Nat. Commun. 15, 3599. doi:10.1038/s41467-024-48010-z
Ashley, E. A. (2016). Towards precision medicine. Nat. Rev. Genet. 17, 507–522. doi:10.1038/nrg.2016.86
Ashok, A., Choudhury, D., Fang, Y., and Hunziker, W. (2020). Towards manufacturing of human organoids. Biotechnol. Adv. 39, 107460. doi:10.1016/j.biotechadv.2019.107460
Auner, A. W., Tasneem, K. M., Markov, D. A., McCawley, L. J., and Hutson, M. S. (2019). Chemical-PDMS binding kinetics and implications for bioavailability in microfluidic devices. Lab. Chip 19, 864–874. doi:10.1039/C8LC00796A
Babar, Q., Saeed, A., Tabish, T. A., Sarwar, M., and Thorat, N. D. (2023). Targeting the tumor microenvironment: potential strategy for cancer therapeutics. Biochimica Biophysica Acta (BBA) - Mol. Basis Dis. 1869, 166746. doi:10.1016/j.bbadis.2023.166746
Baghy, K., Ladányi, A., Reszegi, A., and Kovalszky, I. (2023). Insights into the tumor microenvironment—components, functions and therapeutics. Int. J. Mol. Sci. 24, 17536. doi:10.3390/ijms242417536
Bano, S., Alburquerque, J. Q., Roberts, H. J., Pang, S., Huang, H.-C., and Hasan, T. (2024). Minocycline and photodynamic priming significantly improve chemotherapy efficacy in heterotypic spheroids of pancreatic ductal adenocarcinoma. J. Photochem Photobiol. B 255, 112910. doi:10.1016/j.jphotobiol.2024.112910
Barbosa, M. A. G., Xavier, C. P. R., Pereira, R. F., Petrikaitė, V., and Vasconcelos, M. H. (2021). 3D cell culture models as recapitulators of the tumor microenvironment for the screening of anti-cancer drugs. Cancers (Basel) 14, 190. doi:10.3390/cancers14010190
Bardelli, A., and Pantel, K. (2017). Liquid biopsies, what we do not know (yet). Cancer Cell 31, 172–179. doi:10.1016/j.ccell.2017.01.002
Batista, J. E., dos, S., Rodrigues, M. B., Bristot, I. J., Silva, V., Bernardy, S., et al. (2024). Systematic screening of synthetic organochalcogen compounds with anticancer activity using human lung adenocarcinoma spheroids. Chem. Biol. Interact. 396, 111047. doi:10.1016/j.cbi.2024.111047
Belli, C., Trapani, D., Viale, G., D’Amico, P., Duso, B. A., Della Vigna, P., et al. (2018). Targeting the microenvironment in solid tumors. Cancer Treat. Rev. 65, 22–32. doi:10.1016/j.ctrv.2018.02.004
Benavente, S., Sánchez-García, A., Naches, S., Lleonart, M. E., and Lorente, J. (2020). Therapy-induced modulation of the tumor microenvironment: new opportunities for cancer therapies. Front. Oncol. 10, 582884. doi:10.3389/fonc.2020.582884
Ben-David, U., Ha, G., Tseng, Y.-Y., Greenwald, N. F., Oh, C., Shih, J., et al. (2017). Patient-derived xenografts undergo mouse-specific tumor evolution. Nat. Genet. 49, 1567–1575. doi:10.1038/ng.3967
Berthier, E., Young, E. W. K., and Beebe, D. (2012). Engineers are from PDMS-land, biologists are from polystyrenia. Lab. Chip 12, 1224–1237. doi:10.1039/C2LC20982A
Bērziņa, S., Harrison, A., Taly, V., and Xiao, W. (2021). Technological advances in tumor-on-chip technology: from bench to bedside. Cancers (Basel) 13, 4192. doi:10.3390/cancers13164192
Beutel, A. K., Schütte, L., Scheible, J., Roger, E., Müller, M., Perkhofer, L., et al. (2021). A prospective feasibility trial to challenge patient–derived pancreatic cancer organoids in predicting treatment response. Cancers (Basel) 13, 2539. doi:10.3390/cancers13112539
Bex, A., and Mathon, B. (2022). Advances, technological innovations, and future prospects in stereotactic brain biopsies. Neurosurg. Rev. 46, 5. doi:10.1007/s10143-022-01918-w
Bhatia, S. N., and Ingber, D. E. (2014). Microfluidic organs-on-chips. Nat. Biotechnol. 32, 760–772. doi:10.1038/nbt.2989
Booij, T. H., Cattaneo, C. M., and Hirt, C. K. (2022). Tumor organoids as a research tool: how to exploit them. Cells 11, 3440. doi:10.3390/cells11213440
Boretto, M., Maenhoudt, N., Luo, X., Hennes, A., Boeckx, B., Bui, B., et al. (2019). Patient-derived organoids from endometrial disease capture clinical heterogeneity and are amenable to drug screening. Nat. Cell Biol. 21, 1041–1051. doi:10.1038/s41556-019-0360-z
Brassard, J. A., and Lutolf, M. P. (2019). Engineering stem cell self-organization to build better organoids. Cell Stem Cell 24, 860–876. doi:10.1016/j.stem.2019.05.005
Bray, F., Laversanne, M., Sung, H., Ferlay, J., Siegel, R. L., Soerjomataram, I., et al. (2024). Global cancer statistics 2022: GLOBOCAN estimates of incidence and mortality worldwide for 36 cancers in 185 countries. CA Cancer J. Clin. 74, 229–263. doi:10.3322/caac.21834
Brennan, M. D., Rexius-Hall, M. L., Elgass, L. J., and Eddington, D. T. (2014). Oxygen control with microfluidics. Lab. Chip 14, 4305–4318. doi:10.1039/C4LC00853G
Brooks, A., Liang, X., Zhang, Y., Zhao, C.-X., Roberts, M. S., Wang, H., et al. (2021). Liver organoid as a 3D in vitro model for drug validation and toxicity assessment. Pharmacol. Res. 169, 105608. doi:10.1016/j.phrs.2021.105608
Brooks, A., Zhang, Y., Chen, J., and Zhao, C.-X. (2024). Cancer metastasis-on-a-chip for modeling metastatic cascade and drug screening. Adv. Healthc. Mater 13, 2302436. doi:10.1002/adhm.202302436
Broutier, L., Mastrogiovanni, G., Verstegen, M. M. A., Francies, H. E., Gavarró, L. M., Bradshaw, C. R., et al. (2017). Human primary liver cancer–derived organoid cultures for disease modeling and drug screening. Nat. Med. 23, 1424–1435. doi:10.1038/nm.4438
Bruna, A., Rueda, O. M., Greenwood, W., Batra, A. S., Callari, M., Batra, R. N., et al. (2016). A biobank of breast cancer explants with preserved intra-tumor heterogeneity to screen anticancer compounds. Cell 167, 260–274. doi:10.1016/j.cell.2016.08.041
Burgos-Panadero, R., Lucantoni, F., Gamero-Sandemetrio, E., Cruz-Merino, L. de la, Álvaro, T., and Noguera, R. (2019). The tumour microenvironment as an integrated framework to understand cancer biology. Cancer Lett. 461, 112–122. doi:10.1016/j.canlet.2019.07.010
Burridge, P. W., Li, Y. F., Matsa, E., Wu, H., Ong, S.-G., Sharma, A., et al. (2016). Human induced pluripotent stem cell–derived cardiomyocytes recapitulate the predilection of breast cancer patients to doxorubicin-induced cardiotoxicity. Nat. Med. 22, 547–556. doi:10.1038/nm.4087
Caballero, D., Kaushik, S., Correlo, V. M., Oliveira, J. M., Reis, R. L., and Kundu, S. C. (2017). Organ-on-chip models of cancer metastasis for future personalized medicine: from chip to the patient. Biomaterials 149, 98–115. doi:10.1016/j.biomaterials.2017.10.005
Cabeza-Segura, M., Garcia-Micò, B., La Noce, M., Nicoletti, G. F., Conti, V., Filippelli, A., et al. (2023). How organoids can improve personalized treatment in patients with gastro-esophageal tumors. Curr. Opin. Pharmacol. 69, 102348. doi:10.1016/j.coph.2023.102348
Calà, G., Sina, B., De Coppi, P., Giobbe, G. G., and Gerli, M. F. M. (2023). Primary human organoids models: current progress and key milestones. Front. Bioeng. Biotechnol. 11, 1058970. doi:10.3389/fbioe.2023.1058970
Chaicharoenaudomrung, N., Kunhorm, P., and Noisa, P. (2019). Three-dimensional cell culture systems as an in vitro platform for cancer and stem cell modeling. World J. Stem Cells 11, 1065–1083. doi:10.4252/wjsc.v11.i12.1065
Chen, C.-C., Li, H.-W., Wang, Y.-L., Lee, C.-C., Shen, Y.-C., Hsieh, C.-Y., et al. (2022). Patient-derived tumor organoids as a platform of precision treatment for malignant brain tumors. Sci. Rep. 12, 16399. doi:10.1038/s41598-022-20487-y
Chen, Y., Gao, D., Liu, H., Lin, S., and Jiang, Y. (2015). Drug cytotoxicity and signaling pathway analysis with three-dimensional tumor spheroids in a microwell-based microfluidic chip for drug screening. Anal. Chim. Acta 898, 85–92. doi:10.1016/j.aca.2015.10.006
Chen, Y.-A., King, A. D., Shih, H.-C., Peng, C.-C., Wu, C.-Y., Liao, W.-H., et al. (2011). Generation of oxygen gradients in microfluidic devices for cell culture using spatially confined chemical reactions. Lab. Chip 11, 3626–3633. doi:10.1039/c1lc20325h
Chen, Z., Li, W., Zhang, Y., Yu, M., Shan, L., Yuan, D., et al. (2016). Establishment of a gastric cancer subline with high metastatic potential using a novel microfluidic system. Sci. Rep. 6, 38376. doi:10.1038/srep38376
Chen, Z., Wang, F., Zhang, J., Sun, X., Yan, Y., Wang, Y., et al. (2020). Study on development of composite hydrogels with tunable structures and properties for tumor-on-a-chip research. Front. Bioeng. Biotechnol. 8, 611796. doi:10.3389/fbioe.2020.611796
Chi, C.-W., Lao, Y.-H., Ahmed, A. H. R., Benoy, E. C., Li, C., Dereli-Korkut, Z., et al. (2020). High-throughput tumor-on-a-chip platform to study tumor–stroma interactions and drug pharmacokinetics. Adv. Healthc. Mater 9, 2000880. doi:10.1002/adhm.202000880
Chinen, L. T. D., De Carvalho, F. M., Rocha, B. M. M., Aguiar, C. M., Abdallah, E. A., Campanha, D., et al. (2013). Cytokeratin-based CTC counting unrelated to clinical follow up. J. Thorac. Dis. 5, 593–599. doi:10.3978/j.issn.2072-1439.2013.09.18
Clevers, H. (2016). Modeling development and disease with organoids. Cell 165, 1586–1597. doi:10.1016/j.cell.2016.05.082
Corrò, C., Novellasdemunt, L., and Li, V. S. W. (2020). A brief history of organoids. Am. J. Physiology-Cell Physiology 319, C151-C165–C165. doi:10.1152/ajpcell.00120.2020
Costa, B., Estrada, M. F., Gomes, A., Fernandez, L. M., Azevedo, J. M., Póvoa, V., et al. (2024). Zebrafish Avatar-test forecasts clinical response to chemotherapy in patients with colorectal cancer. Nat. Commun. 15, 4771. doi:10.1038/s41467-024-49051-0
Cristofanilli, M., Hayes, D. F., Budd, G. T., Ellis, M. J., Stopeck, A., Reuben, J. M., et al. (2005). Circulating tumor cells: a novel prognostic factor for newly diagnosed metastatic breast cancer. J. Clin. Oncol. 23, 1420–1430. doi:10.1200/JCO.2005.08.140
Dekkers, J. F., Alieva, M., Cleven, A., Keramati, F., Wezenaar, A. K. L., van Vliet, E. J., et al. (2023). Uncovering the mode of action of engineered T cells in patient cancer organoids. Nat. Biotechnol. 41, 60–69. doi:10.1038/s41587-022-01397-w
Dell’Olio, F., Su, J., Huser, T., Sottile, V., Cortés-Hernández, L. E., and Alix-Panabières, C. (2021). Photonic technologies for liquid biopsies: recent advances and open research challenges. Laser Phot. Rev. 15, 2000255. doi:10.1002/lpor.202000255
De Mattos-Arruda, L., Mayor, R., Ng, C. K. Y., Weigelt, B., Martínez-Ricarte, F., Torrejon, D., et al. (2015). Cerebrospinal fluid-derived circulating tumour DNA better represents the genomic alterations of brain tumours than plasma. Nat. Commun. 6, 8839. doi:10.1038/ncomms9839
Desbordes, S. C., and Studer, L. (2013). Adapting human pluripotent stem cells to high-throughput and high-content screening. Nat. Protoc. 8, 111–130. doi:10.1038/nprot.2012.139
Dhiman, N., Kingshott, P., Sumer, H., Sharma, C. S., and Rath, S. N. (2019). On-chip anticancer drug screening – recent progress in microfluidic platforms to address challenges in chemotherapy. Biosens. Bioelectron. 137, 236–254. doi:10.1016/j.bios.2019.02.070
Dijkstra, K. K., Cattaneo, C. M., Weeber, F., Chalabi, M., van de Haar, J., Fanchi, L. F., et al. (2018). Generation of tumor-reactive T cells by Co-culture of peripheral blood lymphocytes and tumor organoids. Cell 174, 1586–1598. doi:10.1016/j.cell.2018.07.009
DiMasi, J. A., Grabowski, H. G., and Hansen, R. W. (2016). Innovation in the pharmaceutical industry: new estimates of R&D costs. J. Health Econ. 47, 20–33. doi:10.1016/j.jhealeco.2016.01.012
Dörrenhaus, A., Müller, J. I. F., Golka, K., Jedrusik, P., Schulze, H., and Föllmann, W. (2000). Cultures of exfoliated epithelial cells from different locations of the human urinary tract and the renal tubular system. Arch. Toxicol. 74, 618–626. doi:10.1007/s002040000173
Driehuis, E., Gracanin, A., Vries, R. G. J., Clevers, H., and Boj, S. F. (2020a). Establishment of pancreatic organoids from normal tissue and tumors. STAR Protoc. 1, 100192. doi:10.1016/j.xpro.2020.100192
Driehuis, E., Kretzschmar, K., and Clevers, H. (2020b). Establishment of patient-derived cancer organoids for drug-screening applications. Nat. Protoc. 15, 3380–3409. doi:10.1038/s41596-020-0379-4
Driehuis, E., van Hoeck, A., Moore, K., Kolders, S., Francies, H. E., Gulersonmez, M. C., et al. (2019). Pancreatic cancer organoids recapitulate disease and allow personalized drug screening. Proc. Natl. Acad. Sci. 116, 26580–26590. doi:10.1073/pnas.1911273116
Drost, J., and Clevers, H. (2018). Organoids in cancer research. Nat. Rev. Cancer 18, 407–418. doi:10.1038/s41568-018-0007-6
Drost, J., van Jaarsveld, R. H., Ponsioen, B., Zimberlin, C., van Boxtel, R., Buijs, A., et al. (2015). Sequential cancer mutations in cultured human intestinal stem cells. Nature 521, 43–47. doi:10.1038/nature14415
Dsouza, V. L., Kuthethur, R., Kabekkodu, S. P., and Chakrabarty, S. (2022). Organ-on-Chip platforms to study tumor evolution and chemosensitivity. Biochimica Biophysica Acta (BBA) - Rev. Cancer 1877, 188717. doi:10.1016/j.bbcan.2022.188717
Edington, C. D., Chen, W. L. K., Geishecker, E., Kassis, T., Soenksen, L. R., Bhushan, B. M., et al. (2018). Interconnected microphysiological systems for quantitative biology and pharmacology studies. Sci. Rep. 8, 4530. doi:10.1038/s41598-018-22749-0
El Harane, S., Zidi, B., El Harane, N., Krause, K.-H., Matthes, T., and Preynat-Seauve, O. (2023). Cancer spheroids and organoids as novel tools for research and therapy: state of the art and challenges to guide precision medicine. Cells 12, 1001. doi:10.3390/cells12071001
Engelborghs, S., Niemantsverdriet, E., Struyfs, H., Blennow, K., Brouns, R., Comabella, M., et al. (2017). Consensus guidelines for lumbar puncture in patients with neurological diseases. Alzheimer’s and Dementia Diagnosis, Assess. and Dis. Monit. 8, 111–126. doi:10.1016/j.dadm.2017.04.007
Esch, E. W., Bahinski, A., and Huh, D. (2015). Organs-on-chips at the frontiers of drug discovery. Nat. Rev. Drug Discov. 14, 248–260. doi:10.1038/nrd4539
Eslami Amirabadi, H., SahebAli, S., Frimat, J. P., Luttge, R., and den Toonder, J. M. J. (2017). A novel method to understand tumor cell invasion: integrating extracellular matrix mimicking layers in microfluidic chips by “selective curing.”. Biomed. Microdevices 19, 92. doi:10.1007/s10544-017-0234-8
Fang, G., Chen, Y.-C., Lu, H., and Jin, D. (2023). Advances in spheroids and organoids on a chip. Adv. Funct. Mater 33, 2215043. doi:10.1002/adfm.202215043
FDA Modernization Act 2.0 (2022). S.5002—117th congress (2021–2022): FDA modernization act 2.0. Available at: https://www.congress.gov/bill/117th-congress/senate-bill/5002 (Accessed August 14, 2024).
Fieuws, C., Bek, J. W., Parton, B., De Neef, E., De Wever, O., Hoorne, M., et al. (2024). Zebrafish avatars: toward functional precision medicine in low-grade serous ovarian cancer. Cancers (Basel) 16, 1812. doi:10.3390/cancers16101812
Fong, A. H., Romero-López, M., Heylman, C. M., Keating, M., Tran, D., Sobrino, A., et al. (2016). Three-dimensional adult cardiac extracellular matrix promotes maturation of human induced pluripotent stem cell-derived cardiomyocytes. Tissue Eng. Part A 22, 1016–1025. doi:10.1089/ten.tea.2016.0027
Fong, E. L. S., Toh, T. B., Yu, H., and Chow, E. K.-H. (2017). 3D culture as a clinically relevant model for personalized medicine. SLAS Technol. 22, 245–253. doi:10.1177/2472630317697251
Foo, M. A., You, M., Chan, S. L., Sethi, G., Bonney, G. K., Yong, W.-P., et al. (2022). Clinical translation of patient-derived tumour organoids-bottlenecks and strategies. Biomark. Res. 10, 10. doi:10.1186/s40364-022-00356-6
Gao, H., Korn, J. M., Ferretti, S., Monahan, J. E., Wang, Y., Singh, M., et al. (2015). High-throughput screening using patient-derived tumor xenografts to predict clinical trial drug response. Nat. Med. 21, 1318–1325. doi:10.1038/nm.3954
Gao, M., Lin, M., Rao, M., Thompson, H., Hirai, K., Choi, M., et al. (2018). Development of patient-derived gastric cancer organoids from endoscopic biopsies and surgical tissues. Ann. Surg. Oncol. 25, 2767–2775. doi:10.1245/s10434-018-6662-8
Gao, Y., Peng, H., Li, L., Wang, F., Meng, J., Huang, H., et al. (2021). Screening of high-efficiency and low-toxicity antitumor active components in Macleaya cordata seeds based on the competitive effect of drugs on double targets by a new laminar flow chip. Analyst 146, 4934–4944. doi:10.1039/D1AN00754H
Germain, N., Dhayer, M., Dekiouk, S., and Marchetti, P. (2022). Current advances in 3D bioprinting for cancer modeling and personalized medicine. Int. J. Mol. Sci. 23, 3432. doi:10.3390/ijms23073432
Giannitelli, S. M., Peluzzi, V., Raniolo, S., Roscilli, G., Trombetta, M., Mozetic, P., et al. (2024). On-chip recapitulation of the tumor microenvironment: a decade of progress. Biomaterials 306, 122482. doi:10.1016/j.biomaterials.2024.122482
Górnicki, T., Lambrinow, J., Golkar-Narenji, A., Data, K., Domagała, D., Niebora, J., et al. (2024). Biomimetic scaffolds - a novel approach to three dimensional cell culture techniques for potential implementation in tissue engineering. Nanomaterials 14, 531. doi:10.3390/nano14060531
Günther, C., Winner, B., Neurath, M. F., and Stappenbeck, T. S. (2022). Organoids in gastrointestinal diseases: from experimental models to clinical translation. Gut 71, 1892–1908. doi:10.1136/gutjnl-2021-326560
Hachey, S. J., and Hughes, C. C. W. (2018). Applications of tumor chip technology. Lab. Chip 18, 2893–2912. doi:10.1039/C8LC00330K
Hamilton, G., and Rath, B. (2019). Applicability of tumor spheroids for in vitro chemosensitivity assays. Expert Opin. Drug Metab. Toxicol. 15, 15–23. doi:10.1080/17425255.2019.1554055
Hao, M., Cao, Z., Wang, Z., Xin, J., Kong, B., Xu, J., et al. (2022). Patient-derived organoid model in the prediction of chemotherapeutic drug response in colorectal cancer. ACS Biomater. Sci. Eng. 8, 3515–3525. doi:10.1021/acsbiomaterials.2c00354
Haque, M. R., Wessel, C. R., Leary, D. D., Wang, C., Bhushan, A., and Bishehsari, F. (2022). Patient-derived pancreatic cancer-on-a-chip recapitulates the tumor microenvironment. Microsyst. Nanoeng. 8, 36. doi:10.1038/s41378-022-00370-6
Harb, W., Fan, A., Tran, T., Danila, D. C., Keys, D., Schwartz, M., et al. (2013). Mutational analysis of circulating tumor cells using a novel microfluidic collection device and qPCR assay. Transl. Oncol. 6, 528–538. doi:10.1593/tlo.13367
Harouaka, R. A., Nisic, M., and Zheng, S.-Y. (2013). Circulating tumor cell enrichment based on physical properties. SLAS Technol. 18, 455–468. doi:10.1177/2211068213494391
Heinrich, M. A., Huynh, N.-T., Heinrich, L., and Prakash, J. (2024). Understanding glioblastoma stromal barriers against NK cell attack using tri-culture 3D spheroid model. Heliyon 10, e24808. doi:10.1016/j.heliyon.2024.e24808
Heinrich, M. A., Mostafa, A. M. R. H., Morton, J. P., Hawinkels, L. J. A. C., and Prakash, J. (2021). Translating complexity and heterogeneity of pancreatic tumor: 3D in vitro to in vivo models. Adv. Drug Deliv. Rev. 174, 265–293. doi:10.1016/j.addr.2021.04.018
Heydari, Z., Moeinvaziri, F., Agarwal, T., Pooyan, P., Shpichka, A., Maiti, T. K., et al. (2021). Organoids: a novel modality in disease modeling. Biodes Manuf. 4, 689–716. doi:10.1007/s42242-021-00150-7
Hindley, C. J., Cordero-Espinoza, L., and Huch, M. (2016). Organoids from adult liver and pancreas: stem cell biology and biomedical utility. Dev. Biol. 420, 251–261. doi:10.1016/j.ydbio.2016.06.039
Hirschhaeuser, F., Menne, H., Dittfeld, C., West, J., Mueller-Klieser, W., and Kunz-Schughart, L. A. (2010). Multicellular tumor spheroids: an underestimated tool is catching up again. J. Biotechnol. 148, 3–15. doi:10.1016/j.jbiotec.2010.01.012
Ho, B. X., Pek, N. M. Q., and Soh, B.-S. (2018). Disease modeling using 3D organoids derived from human induced pluripotent stem cells. Int. J. Mol. Sci. 19, 936. doi:10.3390/ijms19040936
Hoch, E., Hirth, T., Tovar, G. E. M., and Borchers, K. (2013). Chemical tailoring of gelatin to adjust its chemical and physical properties for functional bioprinting. J. Mater Chem. B 1, 5675–5685. doi:10.1039/c3tb20745e
Hsieh, Y.-K., Chen, S.-C., Huang, W.-L., Hsu, K.-P., Gorday, K., Wang, T., et al. (2017). Direct micromachining of microfluidic channels on biodegradable materials using laser ablation. Polym. (Basel) 9, 242. doi:10.3390/polym9070242
Hu, H., Gehart, H., Artegiani, B., Löpez-Iglesias, C., Dekkers, F., Basak, O., et al. (2018). Long-term expansion of functional mouse and human hepatocytes as 3D organoids. Cell 175, 1591–1606. doi:10.1016/j.cell.2018.11.013
Huang, J.-W., Pan, H.-J., Yao, W.-Y., Tsao, Y.-W., Liao, W.-Y., Wu, C.-W., et al. (2013). Interaction between lung cancer cell and myofibroblast influenced by cyclic tensile strain. Lab. Chip 13, 1114–1120. doi:10.1039/C2LC41050H
Huang, L., Bockorny, B., Paul, I., Akshinthala, D., Frappart, P.-O., Gandarilla, O., et al. (2020). PDX-derived organoids model in vivo drug response and secrete biomarkers. JCI Insight 5, e135544. doi:10.1172/jci.insight.135544
Huang, L., Holtzinger, A., Jagan, I., BeGora, M., Lohse, I., Ngai, N., et al. (2015). Ductal pancreatic cancer modeling and drug screening using human pluripotent stem cell– and patient-derived tumor organoids. Nat. Med. 21, 1364–1371. doi:10.1038/nm.3973
Huang, Y., Huang, Z., Tang, Z., Chen, Y., Huang, M., Liu, H., et al. (2021). Research progress, challenges, and breakthroughs of organoids as disease models. Front. Cell Dev. Biol. 9, 740574. doi:10.3389/fcell.2021.740574
Huch, M., Dorrell, C., Boj, S. F., van Es, J. H., Li, V. S. W., van de Wetering, M., et al. (2013). In vitro expansion of single Lgr5+ liver stem cells induced by Wnt-driven regeneration. Nature 494, 247–250. doi:10.1038/nature11826
Huebsch, N., Loskill, P., Deveshwar, N., Spencer, C. I., Judge, L. M., Mandegar, M. A., et al. (2016). Miniaturized iPS-cell-derived cardiac muscles for physiologically relevant drug response analyses. Sci. Rep. 6, 24726. doi:10.1038/srep24726
Huh, D., Kim, H. J., Fraser, J. P., Shea, D. E., Khan, M., Bahinski, A., et al. (2013). Microfabrication of human organs-on-chips. Nat. Protoc. 8, 2135–2157. doi:10.1038/nprot.2013.137
Izumchenko, E., Meir, J., Bedi, A., Wysocki, P., Hoque, M., and Sidransky, D. (2016). Patient-derived xenografts as tools in pharmaceutical development. Clin. Pharmacol. Ther. 99, 612–621. doi:10.1002/cpt.354
Izumchenko, E., Paz, K., Ciznadija, D., Sloma, I., Katz, A., Vasquez-Dunddel, D., et al. (2017). Patient-derived xenografts effectively capture responses to oncology therapy in a heterogeneous cohort of patients with solid tumors. Ann. Oncol. 28, 2595–2605. doi:10.1093/annonc/mdx416
Jacob, F., Salinas, R. D., Zhang, D. Y., Nguyen, P. T. T., Schnoll, J. G., Wong, S. Z. H., et al. (2020). A patient-derived glioblastoma organoid model and biobank recapitulates inter- and intra-tumoral heterogeneity. Cell 180, 188–204. doi:10.1016/j.cell.2019.11.036
Jeibouei, S., Monfared, A. K., Hojat, A., Aref, A. R., Shams, F., Dolati, M., et al. (2024). Human-derived Tumor-On-Chip model to study the heterogeneity of breast cancer tissue. Biomater. Adv. 162, 213915. doi:10.1016/j.bioadv.2024.213915
Jensen, J., Hyllner, J., and Björquist, P. (2009). Human embryonic stem cell technologies and drug discovery. J. Cell Physiol. 219, 513–519. doi:10.1002/jcp.21732
Jensen, K. B., and Little, M. H. (2023). Organoids are not organs: sources of variation and misinformation in organoid biology. Stem Cell Rep. 18, 1255–1270. doi:10.1016/j.stemcr.2023.05.009
Jeong, S.-Y., Lee, J.-H., Shin, Y., Chung, S., and Kuh, H.-J. (2016). Co-culture of tumor spheroids and fibroblasts in a collagen matrix-incorporated microfluidic chip mimics reciprocal activation in solid tumor microenvironment. PLoS One 11, e0159013. doi:10.1371/journal.pone.0159013
Jiang, M., Li, H., Zhang, Y., Yang, Y., Lu, R., Liu, K., et al. (2017). Transitional basal cells at the squamous–columnar junction generate Barrett’s oesophagus. Nature 550, 529–533. doi:10.1038/nature24269
Jiang, X., Oyang, L., Peng, Q., Liu, Q., Xu, X., Wu, N., et al. (2023). Organoids: opportunities and challenges of cancer therapy. Front. Cell Dev. Biol. 11, 1232528. doi:10.3389/fcell.2023.1232528
Jin, H., Xue, Z., Liu, J., Ma, B., Yang, J., and Lei, L. (2024). Advancing organoid engineering for tissue regeneration and biofunctional reconstruction. Biomater. Res. 28, 0016. doi:10.34133/bmr.0016
Junqueira-Neto, S., Batista, I. A., Costa, J. L., and Melo, S. A. (2019). Liquid biopsy beyond circulating tumor cells and cell-free DNA. Acta Cytol. 63, 479–488. doi:10.1159/000493969
Kaeffer, B. (2011). Survival of exfoliated epithelial cells: a delicate balance between anoikis and apoptosis. Biomed. Res. Int. 2011, 534139. doi:10.1155/2011/534139
Kang, L., Chung, B. G., Langer, R., and Khademhosseini, A. (2008). Microfluidics for drug discovery and development: from target selection to product lifecycle management. Drug Discov. Today 13, 1–13. doi:10.1016/j.drudis.2007.10.003
Kasagi, Y., Chandramouleeswaran, P. M., Whelan, K. A., Tanaka, K., Giroux, V., Sharma, M., et al. (2018). The esophageal organoid system reveals functional interplay between notch and cytokines in reactive epithelial changes. Cell Mol. Gastroenterol. Hepatol. 5, 333–352. doi:10.1016/j.jcmgh.2017.12.013
Kaushik, G., Ponnusamy, M. P., and Batra, S. K. (2018). Concise review: current status of three-dimensional organoids as preclinical models. Stem Cells 36, 1329–1340. doi:10.1002/stem.2852
Kiani, A. K., Pheby, D., Henehan, G., Brown, R., Sieving, P., Sykora, P., et al. (2022). Ethical considerations regarding animal experimentation. J. Prev. Med. Hyg. 63, E255–E266. doi:10.15167/2421-4248/jpmh2022.63.2S3.2768
Kim, C., Bang, J. H., Kim, Y. E., Lee, S. H., and Kang, J. Y. (2012). On-chip anticancer drug test of regular tumor spheroids formed in microwells by a distributive microchannel network. Lab. Chip 12, 4135–4142. doi:10.1039/C2LC40570A
Kim, J., Koo, B.-K., and Knoblich, J. A. (2020). Human organoids: model systems for human biology and medicine. Nat. Rev. Mol. Cell Biol. 21, 571–584. doi:10.1038/s41580-020-0259-3
Kim, S., Min, S., Choi, Y. S., Jo, S.-H., Jung, J. H., Han, K., et al. (2022). Tissue extracellular matrix hydrogels as alternatives to Matrigel for culturing gastrointestinal organoids. Nat. Commun. 13, 1692. doi:10.1038/s41467-022-29279-4
Kim, W., Gwon, Y., Park, S., Kim, H., and Kim, J. (2023). Therapeutic strategies of three-dimensional stem cell spheroids and organoids for tissue repair and regeneration. Bioact. Mater 19, 50–74. doi:10.1016/j.bioactmat.2022.03.039
Kinoshita, K., Tsukamoto, Y., Hirashita, Y., Fuchino, T., Kurogi, S., Uchida, T., et al. (2023). Efficient establishment of bile-derived organoids from biliary cancer patients, Lab. Invest. 103, 100105. doi:10.1016/j.labinv.2023.100105
Kirkwood, J. M., Butterfield, L. H., Tarhini, A. A., Zarour, H., Kalinski, P., and Ferrone, S. (2012). Immunotherapy of cancer in 2012. CA Cancer J. Clin. 62, 309–335. doi:10.3322/caac.20132
Kita, Y., Hamada, A., Saito, R., Teramoto, Y., Tanaka, R., Takano, K., et al. (2019). Systematic chemical screening identifies disulfiram as a repurposed drug that enhances sensitivity to cisplatin in bladder cancer: a summary of preclinical studies. Br. J. Cancer 121, 1027–1038. doi:10.1038/s41416-019-0609-0
Kitaeva, K. V., Rutland, C. S., Rizvanov, A. A., and Solovyeva, V. V. (2020). Cell culture based in vitro test systems for anticancer drug screening. Front. Bioeng. Biotechnol. 8, 322. doi:10.3389/fbioe.2020.00322
Kozlowski, M. T., Crook, C. J., and Ku, H. T. (2021). Towards organoid culture without Matrigel. Commun. Biol. 4, 1387. doi:10.1038/s42003-021-02910-8
Kumar, R., Iden, M., Tsaih, S.-W., Schmidt, R., Ojesina, A. I., and Rader, J. S. (2024). Deciphering the divergent transcriptomic landscapes of cervical cancer cells grown in 3D and 2D cell culture systems. Front. Cell Dev. Biol. 12, 1413882. doi:10.3389/fcell.2024.1413882
Lancaster, M. A., and Knoblich, J. A. (2014). Organogenesis in a dish: modeling development and disease using organoid technologies. Science 1979, 1247125. doi:10.1126/science.1247125
Lancaster, M. A., Renner, M., Martin, C.-A., Wenzel, D., Bicknell, L. S., Hurles, M. E., et al. (2013). Cerebral organoids model human brain development and microcephaly. Nature 501, 373–379. doi:10.1038/nature12517
Langhans, S. A. (2021). Using 3D in vitro cell culture models in anti-cancer drug discovery. Expert Opin. Drug Discov. 16, 841–850. doi:10.1080/17460441.2021.1912731
Lanz, H. L., Saleh, A., Kramer, B., Cairns, J., Ng, C. P., Yu, J., et al. (2017). Therapy response testing of breast cancer in a 3D high-throughput perfused microfluidic platform. BMC Cancer 17, 709. doi:10.1186/s12885-017-3709-3
Lawrence, R., Watters, M., Davies, C. R., Pantel, K., and Lu, Y.-J. (2023). Circulating tumour cells for early detection of clinically relevant cancer. Nat. Rev. Clin. Oncol. 20, 487–500. doi:10.1038/s41571-023-00781-y
Lee, H., and Cho, D.-W. (2016). One-step fabrication of an organ-on-a-chip with spatial heterogeneity using a 3D bioprinting technology. Lab. Chip 16, 2618–2625. doi:10.1039/C6LC00450D
Lee, S.-Y., Koo, I.-S., Hwang, H. J., and Lee, D. W. (2023). In vitro three-dimensional (3D) cell culture tools for spheroid and organoid models. SLAS Discov. 28, 119–137. doi:10.1016/j.slasd.2023.03.006
Lei, Y., and Schaffer, D. V. (2013). A fully defined and scalable 3D culture system for human pluripotent stem cell expansion and differentiation. Proc. Natl. Acad. Sci. 110, E5039–E5048. doi:10.1073/pnas.1309408110
LeSavage, B. L., Suhar, R. A., Broguiere, N., Lutolf, M. P., and Heilshorn, S. C. (2022). Next-generation cancer organoids. Nat. Mater 21, 143–159. doi:10.1038/s41563-021-01057-5
Li, M. L., Aggeler, J., Farson, D. A., Hatier, C., Hassell, J., and Bissell, M. J. (1987). Influence of a reconstituted basement membrane and its components on casein gene expression and secretion in mouse mammary epithelial cells. Proc. Natl. Acad. Sci. 84, 136–140. doi:10.1073/pnas.84.1.136
Li, W., Zhou, Z., Zhou, X., Khoo, B. L., Gunawan, R., Chin, Y. R., et al. (2023). 3D biomimetic models to reconstitute tumor microenvironment in vitro: spheroids, organoids, and tumor-on-a-chip. Adv. Healthc. Mater 12, e2202609. doi:10.1002/adhm.202202609
Li, X., Zhou, Y., Luo, L., Zheng, S., Deng, J., and Luan, T. (2024). Chlorinated anthracenes induced pulmonary immunotoxicity in 3D coculture spheroids simulating the lung microenvironment. Environ. Sci. Technol. 58, 11923–11934. doi:10.1021/acs.est.4c02957
Li, Z., Zheng, W., Wang, H., Cheng, Y., Fang, Y., Wu, F., et al. (2021). Application of animal models in cancer research: recent progress and future prospects. Cancer Manag. Res. 13, 2455–2475. doi:10.2147/CMAR.S302565
Liu, M., Wang, Y., Wang, C., Li, P., Qiu, J., Yang, N., et al. (2024). A microfluidic 3D-tumor-spheroid model for the evaluation of targeted therapies from angiogenesis-related cytokines at the single spheroid level. Adv. Healthc. Mater n/a, 2402321. doi:10.1002/adhm.202402321
Liu, X., Fang, J., Huang, S., Wu, X., Xie, X., Wang, J., et al. (2021). Tumor-on-a-chip: from bioinspired design to biomedical application. Microsyst. Nanoeng. 7, 50. doi:10.1038/s41378-021-00277-8
Liu, X., Su, Q., Zhang, X., Yang, W., Ning, J., Jia, K., et al. (2022). Recent advances of organ-on-a-chip in cancer modeling research. Biosens. (Basel) 12, 1045. doi:10.3390/bios12111045
Lovitt, C., Shelper, T., and Avery, V. (2014). Advanced cell culture techniques for cancer drug discovery. Biol. (Basel) 3, 345–367. doi:10.3390/biology3020345
Low, R. R. J., Lim, W. W., Nguyen, P. M., Lee, B., Christie, M., Burgess, A. W., et al. (2021). The diverse applications of pancreatic ductal adenocarcinoma organoids. Cancers (Basel) 13, 4979. doi:10.3390/cancers13194979
Luo, Z., Zhou, X., Mandal, K., He, N., Wennerberg, W., Qu, M., et al. (2021). Reconstructing the tumor architecture into organoids. Adv. Drug Deliv. Rev. 176, 113839. doi:10.1016/j.addr.2021.113839
Ma, S., Li, X., Wang, X., Cheng, L., Li, Z., Zhang, C., et al. (2019). Current progress in CAR-T cell therapy for solid tumors. Int. J. Biol. Sci. 15, 2548–2560. doi:10.7150/ijbs.34213
Manduca, N., Maccafeo, E., De Maria, R., Sistigu, A., and Musella, M. (2023). 3D cancer models: one step closer to in vitro human studies. Front. Immunol. 14, 1175503. doi:10.3389/fimmu.2023.1175503
Manoharan, T. J. M., Ravi, K., Suresh, A. P., Acharya, A. P., and Nikkhah, M. (2024). Engineered tumor–immune microenvironment on A chip to study T cell–macrophage interaction in breast cancer progression. Adv. Healthc. Mater 13, e2303658. doi:10.1002/adhm.202303658
Mansour, H., Soliman, E. A., El-Bab, A. M. F., Matsushita, Y., and Abdel-Mawgood, A. L. (2023). Fabrication and characterization of microfluidic devices based on boron-modified epoxy resin using CO2 laser ablation for bio-analytical applications. Sci. Rep. 13, 12623. doi:10.1038/s41598-023-39054-0
Martins, I., Ribeiro, I. P., Jorge, J., Gonçalves, A. C., Sarmento-Ribeiro, A. B., Melo, J. B., et al. (2021). Liquid biopsies: applications for cancer diagnosis and monitoring. Genes (Basel) 12, 349. doi:10.3390/genes12030349
Mastrangeli, M., Millet, S., and van den Eijnden-van Raaij, J. (2019). Organ-on-chip in development: towards a roadmap for organs-on-chip. ALTEX 36, 650–668. doi:10.14573/altex.1908271
Matano, M., Date, S., Shimokawa, M., Takano, A., Fujii, M., Ohta, Y., et al. (2015). Modeling colorectal cancer using CRISPR-Cas9–mediated engineering of human intestinal organoids. Nat. Med. 21, 256–262. doi:10.1038/nm.3802
Mathur, A., Loskill, P., Shao, K., Huebsch, N., Hong, S., Marcus, S. G., et al. (2015). Human iPSC-based cardiac microphysiological system for drug screening applications. Sci. Rep. 5, 8883. doi:10.1038/srep08883
Maulana, T. I., Teufel, C., Cipriano, M., Roosz, J., Lazarevski, L., van den Hil, F. E., et al. (2024). Breast cancer-on-chip for patient-specific efficacy and safety testing of CAR-T cells. Cell Stem Cell 31, 989–1002.e9. doi:10.1016/j.stem.2024.04.018
Mazzocchi, A. R., Rajan, S. A. P., Votanopoulos, K. I., Hall, A. R., and Skardal, A. (2018). In vitro patient-derived 3D mesothelioma tumor organoids facilitate patient-centric therapeutic screening. Sci. Rep. 8, 2886. doi:10.1038/s41598-018-21200-8
McDonald, J. C., and Whitesides, G. M. (2002). Poly(dimethylsiloxane) as a material for fabricating microfluidic devices. Acc. Chem. Res. 35, 491–499. doi:10.1021/ar010110q
Mei, J., Liu, X., Tian, H., Chen, Y., Cao, Y., Zeng, J., et al. (2024). Tumour organoids and assembloids: patient-derived cancer avatars for immunotherapy. Clin. Transl. Med. 14, e1656. doi:10.1002/ctm2.1656
Menon, N. V., Chuah, Y. J., Cao, B., Lim, M., and Kang, Y. (2014). A microfluidic co-culture system to monitor tumor-stromal interactions on a chip. Biomicrofluidics 8, 064118. doi:10.1063/1.4903762
Merker, S. R., Weitz, J., and Stange, D. E. (2016). Gastrointestinal organoids: how they gut it out. Dev. Biol. 420, 239–250. doi:10.1016/j.ydbio.2016.08.010
Miller, C. P., Fung, M., Jaeger-Ruckstuhl, C. A., Xu, Y., Warren, E. H., Akilesh, S., et al. (2023). Therapeutic targeting of tumor spheroids in a 3D microphysiological renal cell carcinoma-on-a-chip system. Neoplasia 46, 100948. doi:10.1016/j.neo.2023.100948
Mittal, R., Woo, F. W., Castro, C. S., Cohen, M. A., Karanxha, J., Mittal, J., et al. (2019). Organ-on-chip models: implications in drug discovery and clinical applications. J. Cell Physiol. 234, 8352–8380. doi:10.1002/jcp.27729
Mo, L., and Izpisua Belmonte, J. C. (2019). Organoids — preclinical models of human disease. N. Engl. J. Med. 380, 569–579. doi:10.1056/NEJMra1806175
Moghimi, N., Hosseini, S. A., Dalan, A. B., Mohammadrezaei, D., Goldman, A., and Kohandel, M. (2023). Controlled tumor heterogeneity in a co-culture system by 3D bio-printed tumor-on-chip model. Sci. Rep. 13, 13648. doi:10.1038/s41598-023-40680-x
Moradi, S., Mahdizadeh, H., Šarić, T., Kim, J., Harati, J., Shahsavarani, H., et al. (2019). Research and therapy with induced pluripotent stem cells (iPSCs): social, legal, and ethical considerations. Stem Cell Res. Ther. 10, 341. doi:10.1186/s13287-019-1455-y
Narsinh, K. H., Plews, J., and Wu, J. C. (2011). Comparison of human induced pluripotent and embryonic stem cells: fraternal or identical twins? Mol. Ther. 19, 635–638. doi:10.1038/mt.2011.41
Nayak, P., Bentivoglio, V., Varani, M., and Signore, A. (2023). Three-dimensional in vitro tumor spheroid models for evaluation of anticancer therapy: recent updates. Cancers (Basel) 15, 4846. doi:10.3390/cancers15194846
Ndong, C., Tate, J. A., Kett, W. C., Batra, J., Demidenko, E., Lewis, L. D., et al. (2015). Tumor cell targeting by iron oxide nanoparticles is dominated by different factors in vitro versus in vivo. PLoS One 10, e0115636. doi:10.1371/journal.pone.0115636
Neal, J. T., Li, X., Zhu, J., Giangarra, V., Grzeskowiak, C. L., Ju, J., et al. (2018). Organoid modeling of the tumor immune microenvironment. Cell 175, 1972–1988. doi:10.1016/j.cell.2018.11.021
Neves, M., Azevedo, R., Lima, L., Oliveira, M. I., Peixoto, A., Ferreira, D., et al. (2019). Exploring sialyl-Tn expression in microfluidic-isolated circulating tumour cells: a novel biomarker and an analytical tool for precision oncology applications. N. Biotechnol. 49, 77–87. doi:10.1016/j.nbt.2018.09.004
Ng, J. M. K., Gitlin, I., Stroock, A. D., and Whitesides, G. M. (2002). Components for integrated poly(dimethylsiloxane) microfluidic systems. Electrophoresis 23, 3461–3473. doi:10.1002/1522-2683(200210)23:20<3461::AID-ELPS3461>3.0.CO;2-8
Ning, R.-X., Liu, C.-Y., Wang, S.-Q., Li, W.-K., Kong, X., and He, Z.-W. (2024). Application status and optimization suggestions of tumor organoids and CAR-T cell co-culture models. Cancer Cell Int. 24, 98. doi:10.1186/s12935-024-03272-x
Nuciforo, S., Fofana, I., Matter, M. S., Blumer, T., Calabrese, D., Boldanova, T., et al. (2018). Organoid models of human liver cancers derived from tumor needle biopsies. Cell Rep. 24, 1363–1376. doi:10.1016/j.celrep.2018.07.001
Okita, K., Ichisaka, T., and Yamanaka, S. (2007). Generation of germline-competent induced pluripotent stem cells. Nature 448, 313–317. doi:10.1038/nature05934
Ooft, S. N., Weeber, F., Dijkstra, K. K., McLean, C. M., Kaing, S., van Werkhoven, E., et al. (2019). Patient-derived organoids can predict response to chemotherapy in metastatic colorectal cancer patients. Sci. Transl. Med. 11, eaay2574. doi:10.1126/scitranslmed.aay2574
Ouchi, R., and Koike, H. (2023). Modeling human liver organ development and diseases with pluripotent stem cell-derived organoids. Front. Cell Dev. Biol. 11, 1133534. doi:10.3389/fcell.2023.1133534
Palacio-Castañeda, V., Kooijman, L., Venzac, B., Verdurmen, W., and Le Gac, S. (2020). Metabolic switching of tumor cells under hypoxic conditions in a tumor-on-a-chip model. Micromachines (Basel) 11, 382. doi:10.3390/mi11040382
Papapetrou, E. P. (2016). Patient-derived induced pluripotent stem cells in cancer research and precision oncology. Nat. Med. 22, 1392–1401. doi:10.1038/nm.4238
Park, S. E., Georgescu, A., and Huh, D. (2019). Organoids-on-a-chip. Science 364, 960–965. doi:10.1126/science.aaw7894
Paşca, A. M., Sloan, S. A., Clarke, L. E., Tian, Y., Makinson, C. D., Huber, N., et al. (2015). Functional cortical neurons and astrocytes from human pluripotent stem cells in 3D culture. Nat. Methods 12, 671–678. doi:10.1038/nmeth.3415
Patel, D., Gao, Y., Son, K., Siltanen, C., Neve, R. M., Ferrara, K., et al. (2015). Microfluidic co-cultures with hydrogel-based ligand trap to study paracrine signals giving rise to cancer drug resistance. Lab. Chip 15, 4614–4624. doi:10.1039/C5LC00948K
Pauli, C., Hopkins, B. D., Prandi, D., Shaw, R., Fedrizzi, T., Sboner, A., et al. (2017). Personalized in vitro and in vivo cancer models to guide precision medicine. Cancer Discov. 7, 462–477. doi:10.1158/2159-8290.CD-16-1154
Pearce, J. M. (1994). Walter essex wynter, quincke, and lumbar puncture. J. Neurol. Neurosurg. Psychiatry 57, 179. doi:10.1136/jnnp.57.2.179
Pellegrini, L., Bonfio, C., Chadwick, J., Begum, F., Skehel, M., and Lancaster, M. A. (2020). Human CNS barrier-forming organoids with cerebrospinal fluid production. Science 369, eaaz5626. doi:10.1126/science.aaz5626
Peterson, J. K., and Houghton, P. J. (2004). Integrating pharmacology and in vivo cancer models in preclinical and clinical drug development. Eur. J. Cancer 40, 837–844. doi:10.1016/j.ejca.2004.01.003
Portillo-Lara, R., and Annabi, N. (2016). Microengineered cancer-on-a-chip platforms to study the metastatic microenvironment. Lab. Chip 16, 4063–4081. doi:10.1039/C6LC00718J
Praharaj, P. P., Bhutia, S. K., Nagrath, S., Bitting, R. L., and Deep, G. (2018). Circulating tumor cell-derived organoids: current challenges and promises in medical research and precision medicine. Biochimica Biophysica Acta (BBA) - Rev. Cancer 1869, 117–127. doi:10.1016/j.bbcan.2017.12.005
Prince, E., Kheiri, S., Wang, Y., Xu, F., Cruickshank, J., Topolskaia, V., et al. (2022). Microfluidic arrays of breast tumor spheroids for drug screening and personalized cancer therapies. Adv. Healthc. Mater 11, e2101085. doi:10.1002/adhm.202101085
Qiao, Z., Teng, X., Liu, A., and Yang, W. (2024). Novel isolating approaches to circulating tumor cell enrichment based on microfluidics: a review. Micromachines (Basel) 15, 706. doi:10.3390/mi15060706
Qu, J., Kalyani, F. S., Liu, L., Cheng, T., and Chen, L. (2021). Tumor organoids: synergistic applications, current challenges, and future prospects in cancer therapy. Cancer Commun. 41, 1331–1353. doi:10.1002/cac2.12224
Qu, S., Xu, R., Yi, G., Li, Z., Zhang, H., Qi, S., et al. (2024). Patient-derived organoids in human cancer: a platform for fundamental research and precision medicine. Mol. Biomed. 5, 6. doi:10.1186/s43556-023-00165-9
Raghavan, S., Winter, P. S., Navia, A. W., Williams, H. L., DenAdel, A., Lowder, K. E., et al. (2021). Microenvironment drives cell state, plasticity, and drug response in pancreatic cancer. Cell 184, 6119–6137.e26. doi:10.1016/j.cell.2021.11.017
Rakhmatullina, A. R., Zolotykh, M. A., Filina, Y. V., Mingaleeva, R. N., Sagdeeva, A. R., Boulygina, E. A., et al. (2024). Development of a novel prostate Cancer-Stroma Sphere (CSS) model for in vitro tumor microenvironment studies. Transl. Oncol. 44, 101930. doi:10.1016/j.tranon.2024.101930
Reckamp, K. L., Melnikova, V. O., Karlovich, C., Sequist, L. V., Camidge, D. R., Wakelee, H., et al. (2016). A highly sensitive and quantitative test platform for detection of NSCLC EGFR mutations in urine and plasma. J. Thorac. Oncol. 11, 1690–1700. doi:10.1016/j.jtho.2016.05.035
Ren, K., Chen, Y., and Wu, H. (2014). New materials for microfluidics in biology. Curr. Opin. Biotechnol. 25, 78–85. doi:10.1016/j.copbio.2013.09.004
Ren, K., Zhou, J., and Wu, H. (2013). Materials for microfluidic chip fabrication. Acc. Chem. Res. 46, 2396–2406. doi:10.1021/ar300314s
Ren, X., Chen, W., Yang, Q., Li, X., and Xu, L. (2022). Patient-derived cancer organoids for drug screening: basic technology and clinical application. J. Gastroenterol. Hepatol. 37, 1446–1454. doi:10.1111/jgh.15930
Richter, M., Piwocka, O., Musielak, M., Piotrowski, I., Suchorska, W. M., and Trzeciak, T. (2021). From donor to the lab: a fascinating journey of primary cell lines. Front. Cell Dev. Biol. 9, 711381. doi:10.3389/fcell.2021.711381
Riedl, A., Schlederer, M., Pudelko, K., Stadler, M., Walter, S., Unterleuthner, D., et al. (2017). Comparison of cancer cells in 2D vs 3D culture reveals differences in AKT–mTOR–S6K signaling and drug responses. J. Cell Sci. 130, 203–218. doi:10.1242/jcs.188102
Rios, A. C., and Clevers, H. (2018). Imaging organoids: a bright future ahead. Nat. Methods 15, 24–26. doi:10.1038/nmeth.4537
Rodrigues, T., Kundu, B., Silva-Correia, J., Kundu, S. C., Oliveira, J. M., Reis, R. L., et al. (2018). Emerging tumor spheroids technologies for 3D in vitro cancer modeling. Pharmacol. Ther. 184, 201–211. doi:10.1016/j.pharmthera.2017.10.018
Rodriguez, A. D., Horowitz, L. F., Castro, K., Kenerson, H., Bhattacharjee, N., Gandhe, G., et al. (2020). A microfluidic platform for functional testing of cancer drugs on intact tumor slices. Lab. Chip 20, 1658–1675. doi:10.1039/C9LC00811J
Rodriguez-Polo, I., and Behr, R. (2022). Non-human primate pluripotent stem cells for the preclinical testing of regenerative therapies. Neural Regen. Res. 17, 1867–1874. doi:10.4103/1673-5374.335689
Roma-Rodrigues, C., Mendes, R., Baptista, P. V., and Fernandes, A. R. (2019). Targeting tumor microenvironment for cancer therapy. Int. J. Mol. Sci. 20, 840. doi:10.3390/ijms20040840
Ronaldson-Bouchard, K., and Vunjak-Novakovic, G. (2018). Organs-on-a-Chip: a fast track for engineered human tissues in drug development. Cell Stem Cell 22, 310–324. doi:10.1016/j.stem.2018.02.011
Rosa, R., Monteleone, F., Zambrano, N., and Bianco, R. (2014). In vitro and in vivo models for analysis of resistance to anticancer molecular therapies. Curr. Med. Chem. 21, 1595–1606. doi:10.2174/09298673113209990226
Saito, Y., Muramatsu, T., Kanai, Y., Ojima, H., Sukeda, A., Hiraoka, N., et al. (2019). Establishment of patient-derived organoids and drug screening for biliary tract carcinoma. Cell Rep. 27, 1265–1276. doi:10.1016/j.celrep.2019.03.088
Santo, V. E., Rebelo, S. P., Estrada, M. F., Alves, P. M., Boghaert, E., and Brito, C. (2017). Drug screening in 3D in vitro tumor models: overcoming current pitfalls of efficacy read-outs. Biotechnol. J. 12. doi:10.1002/biot.201600505
Sato, T., Vries, R. G., Snippert, H. J., van de Wetering, M., Barker, N., Stange, D. E., et al. (2009). Single Lgr5 stem cells build crypt-villus structures in vitro without a mesenchymal niche. Nature 459, 262–265. doi:10.1038/nature07935
Saxena, P., Kumbhari, V., Zein, M. E. L., and Khashab, M. A. (2015). Preoperative biliary drainage. Dig. Endosc. 27, 265–277. doi:10.1111/den.12394
Schueler, J., Borenstein, J., Buti, L., Dong, M., Masmoudi, F., Hribar, K., et al. (2022). How to build a tumor: an industry perspective. Drug Discov. Today 27, 103329. doi:10.1016/j.drudis.2022.07.014
Schuster, B., Junkin, M., Kashaf, S. S., Romero-Calvo, I., Kirby, K., Matthews, J., et al. (2020). Automated microfluidic platform for dynamic and combinatorial drug screening of tumor organoids. Nat. Commun. 11, 5271. doi:10.1038/s41467-020-19058-4
Schwank, G., Koo, B.-K., Sasselli, V., Dekkers, J. F., Heo, I., Demircan, T., et al. (2013). Functional repair of CFTR by CRISPR/Cas9 in intestinal stem cell organoids of cystic fibrosis patients. Cell Stem Cell 13, 653–658. doi:10.1016/j.stem.2013.11.002
Schwartz, R. E., Bram, Y., and Frankel, A. (2016). Pluripotent stem cell-derived hepatocyte-like cells: a tool to study infectious disease. Curr. Pathobiol. Rep. 4, 147–156. doi:10.1007/s40139-016-0113-7
Seidlitz, T., Merker, S. R., Rothe, A., Zakrzewski, F., von Neubeck, C., Grützmann, K., et al. (2019). Human gastric cancer modelling using organoids. Gut 68, 207–217. doi:10.1136/gutjnl-2017-314549
Shankaran, A., Prasad, K., Chaudhari, S., Brand, A., and Satyamoorthy, K. (2021). Advances in development and application of human organoids. 3 Biotech. 11, 257. doi:10.1007/s13205-021-02815-7
Shao, Y., Taniguchi, K., Gurdziel, K., Townshend, R. F., Xue, X., Yong, K. M. A., et al. (2017). Self-organized amniogenesis by human pluripotent stem cells in a biomimetic implantation-like niche. Nat. Mater 16, 419–425. doi:10.1038/nmat4829
Sharma, K., Dey, S., Karmakar, R., and Rengan, A. K. (2024). A comprehensive review of 3D cancer models for drug screening and translational research. Cancer Innov. 3, e102. doi:10.1002/cai2.102
Sharma, R., Restan Perez, M., da Silva, V. A., Thomsen, J., Bhardwaj, L., Andrade, T. A. M., et al. (2023). 3D bioprinting complex models of cancer. Biomater. Sci. 11, 3414–3430. doi:10.1039/D2BM02060B
Shegekar, T., Vodithala, S., and Juganavar, A. (2023). The emerging role of liquid biopsies in revolutionising cancer diagnosis and therapy. Cureus 15, e43650. doi:10.7759/cureus.43650
Sidaway, P. (2023). New data confirm clinical utility of ctDNA. Nat. Rev. Clin. Oncol. 20, 63. doi:10.1038/s41571-022-00716-z
Silva, M. C., and Haggarty, S. J. (2020). Human pluripotent stem cell–derived models and drug screening in CNS precision medicine. Ann. N. Y. Acad. Sci. 1471, 18–56. doi:10.1111/nyas.14012
Silverio, V., and Cardoso de Freitas, S. (2018). “Microfabrication techniques for microfluidic devices,” in Complex fluid-flows in microfluidics (Cham: Springer International Publishing), 25–51. doi:10.1007/978-3-319-59593-1_2
Skardal, A., Aleman, J., Forsythe, S., Rajan, S., Murphy, S., Devarasetty, M., et al. (2020). Drug compound screening in single and integrated multi-organoid body-on-a-chip systems. Biofabrication 12, 025017. doi:10.1088/1758-5090/ab6d36
Sleeboom, J. J. F., Eslami Amirabadi, H., Nair, P., Sahlgren, C. M., and den Toonder, J. M. J. (2018). Metastasis in context: modeling the tumor microenvironment with cancer-on-a-chip approaches. Dis. Model Mech. 11, dmm033100. doi:10.1242/dmm.033100
Smabers, L. P., Wensink, E., Verissimo, C. S., Koedoot, E., Pitsa, K.-C., Huismans, M. A., et al. (2024). Organoids as a biomarker for personalized treatment in metastatic colorectal cancer: drug screen optimization and correlation with patient response. J. Exp. and Clin. Cancer Res. 43, 61. doi:10.1186/s13046-024-02980-6
Sobhani, A., Khanlarkhani, N., Baazm, M., Mohammadzadeh, F., Najafi, A., Mehdinejadiani, S., et al. (2017). Multipotent stem cell and current application. Acta Med. Iran. 55, 6–23.
Song, H.-H. G., Park, K. M., and Gerecht, S. (2014). Hydrogels to model 3D in vitro microenvironment of tumor vascularization. Adv. Drug Deliv. Rev. 79–80, 19–29. doi:10.1016/j.addr.2014.06.002
Song, W. H., Lim, Y. S., Kim, J.-E., Kang, H. Y., Lee, C., Rajbongshi, L., et al. (2024). A marine collagen-based 3D scaffold for in vitro modeling of human prostate cancer niche and anti-cancer therapeutic discovery. Mar. Drugs 22, 295. doi:10.3390/md22070295
Soroka, C. J., Assis, D. N., Alrabadi, L. S., Roberts, S., Cusack, L., Jaffe, A. B., et al. (2019a). Bile-derived organoids from patients with primary sclerosing cholangitis recapitulate their inflammatory immune profile. Hepatology 70, 871–882. doi:10.1002/hep.30470
Soroka, C. J., Assis, D. N., and Boyer, J. L. (2019b). “Patient-derived organoids from human bile: an in vitro method to study cholangiopathies,” in Experimental cholestasis research. Editor M. Vinken (New York, NY: Springer New York), 363–372. doi:10.1007/978-1-4939-9420-5_24
Spence, J. R., Mayhew, C. N., Rankin, S. A., Kuhar, M. F., Vallance, J. E., Tolle, K., et al. (2011). Directed differentiation of human pluripotent stem cells into intestinal tissue in vitro. Nature 470, 105–109. doi:10.1038/nature09691
Steinberg, E., Friedman, R., Goldstein, Y., Friedman, N., Beharier, O., Demma, J. A., et al. (2023). A fully 3D-printed versatile tumor-on-a-chip allows multi-drug screening and correlation with clinical outcomes for personalized medicine. Commun. Biol. 6, 1157. doi:10.1038/s42003-023-05531-5
Struth, E., Labaf, M., Karimnia, V., Liu, Y., Cramer, G., Dahl, J. B., et al. (2024). Drug resistant pancreatic cancer cells exhibit altered biophysical interactions with stromal fibroblasts in imaging studies of 3D co-culture models. Sci. Rep. 14, 20698. doi:10.1038/s41598-024-71372-9
Sun, J., Carlson-Stevermer, J., Das, U., Shen, M., Delenclos, M., Snead, A. M., et al. (2019). CRISPR/Cas9 editing of APP C-terminus attenuates β-cleavage and promotes α-cleavage. Nat. Commun. 10, 53. doi:10.1038/s41467-018-07971-8
Sun, J., Liu, W., Li, Y., Gholamipour-Shirazi, A., Abdulla, A., and Ding, X. (2017). An on-chip cell culturing and combinatorial drug screening system. Microfluid Nanofluidics 21, 125. doi:10.1007/s10404-017-1959-7
Sung, K. E., Yang, N., Pehlke, C., Keely, P. J., Eliceiri, K. W., Friedl, A., et al. (2011). Transition to invasion in breast cancer: a microfluidic in vitro model enables examination of spatial and temporal effects. Integr. Biol. 3, 439–450. doi:10.1039/c0ib00063a
Taelman, J., Diaz, M., and Guiu, J. (2022). Human intestinal organoids: promise and challenge. Front. Cell Dev. Biol. 10, 854740. doi:10.3389/fcell.2022.854740
Takahashi, K., and Yamanaka, S. (2013). Induced pluripotent stem cells in medicine and biology. Development 140, 2457–2461. doi:10.1242/dev.092551
Takahashi, K., and Yamanaka, S. (2016). A decade of transcription factor-mediated reprogramming to pluripotency. Nat. Rev. Mol. Cell Biol. 17, 183–193. doi:10.1038/nrm.2016.8
Takebe, T., Zhang, B., and Radisic, M. (2017). Synergistic engineering: organoids meet organs-on-a-chip. Cell Stem Cell 21, 297–300. doi:10.1016/j.stem.2017.08.016
Takeda, H., Kataoka, S., Nakayama, M., Ali, M. A. E., Oshima, H., Yamamoto, D., et al. (2019). CRISPR-Cas9–mediated gene knockout in intestinal tumor organoids provides functional validation for colorectal cancer driver genes. Proc. Natl. Acad. Sci. 116, 15635–15644. doi:10.1073/pnas.1904714116
Tang, X.-Y., Wu, S., Wang, D., Chu, C., Hong, Y., Tao, M., et al. (2022). Human organoids in basic research and clinical applications. Signal Transduct. Target Ther. 7, 168. doi:10.1038/s41392-022-01024-9
Terrell, J. A., Jones, C. G., Kabandana, G. K. M., and Chen, C. (2020). From cells-on-a-chip to organs-on-a-chip: scaffolding materials for 3D cell culture in microfluidics. J. Mater Chem. B 8, 6667–6685. doi:10.1039/D0TB00718H
Thompson, C. L., Fu, S., Heywood, H. K., Knight, M. M., and Thorpe, S. D. (2020). Mechanical stimulation: a crucial element of organ-on-chip models. Front. Bioeng. Biotechnol. 8, 602646. doi:10.3389/fbioe.2020.602646
Tosca, E. M., Ronchi, D., Facciolo, D., and Magni, P. (2023). Replacement, reduction, and refinement of animal experiments in anticancer drug development: the contribution of 3D in vitro cancer models in the drug efficacy assessment. Biomedicines 11, 1058. doi:10.3390/biomedicines11041058
Truong, D., Puleo, J., Llave, A., Mouneimne, G., Kamm, R. D., and Nikkhah, M. (2016). Breast cancer cell invasion into a three dimensional tumor-stroma microenvironment. Sci. Rep. 6, 34094. doi:10.1038/srep34094
Truong, V. A., Hsu, M.-N., Kieu Nguyen, N. T., Lin, M.-W., Shen, C.-C., Lin, C.-Y., et al. (2019). CRISPRai for simultaneous gene activation and inhibition to promote stem cell chondrogenesis and calvarial bone regeneration. Nucleic Acids Res. 47, e74. doi:10.1093/nar/gkz267
Turetta, M., Ben, F. D., Brisotto, G., Biscontin, E., Bulfoni, M., Cesselli, D., et al. (2018). Emerging technologies for cancer research: towards personalized medicine with microfluidic platforms and 3D tumor models. Curr. Med. Chem. 25, 4616–4637. doi:10.2174/0929867325666180605122633
Ukai, S., Honma, R., Sakamoto, N., Yamamoto, Y., Pham, Q. T., Harada, K., et al. (2020). Molecular biological analysis of 5-FU-resistant gastric cancer organoids; KHDRBS3 contributes to the attainment of features of cancer stem cell. Oncogene 39, 7265–7278. doi:10.1038/s41388-020-01492-9
Valente, R., Cordeiro, S., Luz, A., Melo, M. C., Rodrigues, C. R., Baptista, P. V., et al. (2023). Doxorubicin-sensitive and -resistant colorectal cancer spheroid models: assessing tumor microenvironment features for therapeutic modulation. Front. Cell Dev. Biol. 11, 1310397. doi:10.3389/fcell.2023.1310397
van de Wetering, M., Francies, H. E., Francis, J. M., Bounova, G., Iorio, F., Pronk, A., et al. (2015). Prospective derivation of a living organoid biobank of colorectal cancer patients. Cell 161, 933–945. doi:10.1016/j.cell.2015.03.053
Vasseur, A., Kiavue, N., Bidard, F.-C., Pierga, J.-Y., and Cabel, L. (2021). Clinical utility of circulating tumor cells: an update. Mol. Oncol. 15, 1647–1666. doi:10.1002/1878-0261.12869
Villata, S., Canta, M., Baruffaldi, D., Roppolo, I., Pirri, C. F., and Frascella, F. (2023). 3D bioprinted GelMA platform for the production of lung tumor spheroids. Bioprinting 36, e00310. doi:10.1016/j.bprint.2023.e00310
Vlachogiannis, G., Hedayat, S., Vatsiou, A., Jamin, Y., Fernández-Mateos, J., Khan, K., et al. (2018). Patient-derived organoids model treatment response of metastatic gastrointestinal cancers. Science 1979 (359), 920–926. doi:10.1126/science.aao2774
Vulto, P., and Joore, J. (2021). Adoption of organ-on-chip platforms by the pharmaceutical industry. Nat. Rev. Drug Discov. 20, 961–962. doi:10.1038/s41573-021-00323-0
Walz, S., Pollehne, P., Geng, R., Schneider, J., Maas, M., Aicher, W. K., et al. (2023). A protocol for organoids from the urine of bladder cancer patients. Cells 12, 2188. doi:10.3390/cells12172188
Wang, H.-M., Zhang, C.-Y., Peng, K.-C., Chen, Z.-X., Su, J.-W., Li, Y.-F., et al. (2023). Using patient-derived organoids to predict locally advanced or metastatic lung cancer tumor response: a real-world study. Cell Rep. Med. 4, 100911. doi:10.1016/j.xcrm.2022.100911
Wang, Y., Liu, M., Zhang, Y., Liu, H., and Han, L. (2023). Recent methods of droplet microfluidics and their applications in spheroids and organoids. Lab. Chip 23, 1080–1096. doi:10.1039/D2LC00493C
Wanigasekara, J., Cullen, P. J., Bourke, P., Tiwari, B., and Curtin, J. F. (2023). Advances in 3D culture systems for therapeutic discovery and development in brain cancer. Drug Discov. Today 28, 103426. doi:10.1016/j.drudis.2022.103426
Whitesides, G. M. (2006). The origins and the future of microfluidics. Nature 442, 368–373. doi:10.1038/nature05058
Wong, A. H.-H., Li, H., Jia, Y., Mak, P.-I., Martins, R. P. da S., Liu, Y., et al. (2017). Drug screening of cancer cell lines and human primary tumors using droplet microfluidics. Sci. Rep. 7, 9109. doi:10.1038/s41598-017-08831-z
World Health Organization (2024). The top 10 causes of death. Available at: https://www.who.int/news-room/fact-sheets/detail/the-top-10-causes-of-death (Accessed November 1, 2024).
Wu, W., Zhou, W., Jiang, J., Wang, M., Zhang, J., Yang, J., et al. (2023). Mechanical stimuli-induced CCL2 restores adult mouse cells to regenerate hair follicles. Mol. Ther. Nucleic Acids 32, 94–110. doi:10.1016/j.omtn.2023.03.002
Xia, Y., and Whitesides, G. M. (1998). Soft lithography. Angew. Chem. Int. Ed. 37, 550–575. doi:10.1002/(SICI)1521-3773(19980316)37:5<550::AID-ANIE550>3.0.CO;2-G
Xu, X., Shen, L., Li, W., Liu, X., Yang, P., and Cai, J. (2023). ITGA5 promotes tumor angiogenesis in cervical cancer. Cancer Med. 12, 11983–11999. doi:10.1002/cam4.5873
Xu, Z., Li, E., Guo, Z., Yu, R., Hao, H., Xu, Y., et al. (2016). Design and construction of a multi-organ microfluidic chip mimicking the in vivo microenvironment of lung cancer metastasis. ACS Appl. Mater Interfaces 8, 25840–25847. doi:10.1021/acsami.6b08746
Yamanaka, S. (2020). Pluripotent stem cell-based cell therapy - promise and challenges. Cell Stem Cell 27, 523–531. doi:10.1016/j.stem.2020.09.014
Yang, C., Xia, B.-R., Jin, W.-L., and Lou, G. (2019). Circulating tumor cells in precision oncology: clinical applications in liquid biopsy and 3D organoid model. Cancer Cell Int. 19, 341. doi:10.1186/s12935-019-1067-8
Yang, Q., Lian, Q., and Xu, F. (2017). Perspective: fabrication of integrated organ-on-a-chip via bioprinting. Biomicrofluidics 11, 031301. doi:10.1063/1.4982945
Yang, R., and Yu, Y. (2023). Patient-derived organoids in translational oncology and drug screening. Cancer Lett. 562, 216180. doi:10.1016/j.canlet.2023.216180
Young, H. E., and Black, A. C. (2004). Adult stem cells. Anat. Rec. A Discov. Mol. Cell Evol. Biol. 276A, 75–102. doi:10.1002/ar.a.10134
Yuan, B., Zhao, X., Wang, X., Liu, E., Liu, C., Zong, Y., et al. (2022). Patient-derived organoids for personalized gallbladder cancer modelling and drug screening. Clin. Transl. Med. 12, e678. doi:10.1002/ctm2.678
Zeng, X., Ma, Q., Li, X.-K., You, L.-T., Li, J., Fu, X., et al. (2023). Patient-derived organoids of lung cancer based on organoids-on-a-chip: enhancing clinical and translational applications. Front. Bioeng. Biotechnol. 11, 1205157. doi:10.3389/fbioe.2023.1205157
Zervantonakis, I. K., Hughes-Alford, S. K., Charest, J. L., Condeelis, J. S., Gertler, F. B., and Kamm, R. D. (2012). Three-dimensional microfluidic model for tumor cell intravasation and endothelial barrier function. Proc. Natl. Acad. Sci. 109, 13515–13520. doi:10.1073/pnas.1210182109
Zhang, H., Xiao, L., Li, Q., Qi, X., and Zhou, A. (2018). Microfluidic chip for non-invasive analysis of tumor cells interaction with anti-cancer drug doxorubicin by AFM and Raman spectroscopy. Biomicrofluidics 12, 024119. doi:10.1063/1.5024359
Zhang, M.-M., Yang, K.-L., Cui, Y.-C., Zhou, Y.-S., Zhang, H.-R., Wang, Q., et al. (2021). Current trends and research topics regarding intestinal organoids: an overview based on bibliometrics. Front. Cell Dev. Biol. 9, 609452. doi:10.3389/fcell.2021.609452
Zhang, S., Shen, J., Wang, X., Sun, X., Wu, Y., Zhang, M.-R., et al. (2023). Integration of organoids in peptide drug discovery: rise of the high-throughput screening. VIEW 4, 20230010. doi:10.1002/VIW.20230010
Zhao H., H., Cheng, Y., Li, J., Zhou, J., Yang, H., Yu, F., et al. (2022). Droplet-engineered organoids recapitulate parental tissue transcriptome with inter-organoid homogeneity and inter-tumor cell heterogeneity. Fundam. Res. doi:10.1016/j.fmre.2022.05.018
Zhao, J., Zeng, Z., Sun, J., Zhang, Y., Li, D., Zhang, X., et al. (2017). A novel model of P-glycoprotein inhibitor screening using human small intestinal organoids. Basic Clin. Pharmacol. Toxicol. 120, 250–255. doi:10.1111/bcpt.12680
Zhao X., X., Dong, J., Jiang, K., Huang, X., and Zhang, W. (2015). Comparison of percutaneous transhepatic biliary drainage and endoscopic biliary drainage in the management of malignant biliary tract obstruction: a meta-analysis. Dig. Endosc. 27, 137–145. doi:10.1111/den.12320
Zhao Y., Y., Wang, D., Xu, T., Liu, P., Cao, Y., Wang, Y., et al. (2015). Bladder cancer cells re-educate TAMs through lactate shuttling in the microfluidic cancer microenvironment. Oncotarget 6, 39196–39210. doi:10.18632/oncotarget.5538
Zhao, Z., Chen, X., Dowbaj, A. M., Sljukic, A., Bratlie, K., Lin, L., et al. (2022). Organoids. Nat. Rev. Methods Prim. 2, 94. doi:10.1038/s43586-022-00174-y
Zou, H., Yue, W., Yu, W.-K., Liu, D., Fong, C.-C., Zhao, J., et al. (2015). Microfluidic platform for studying chemotaxis of adhesive cells revealed a gradient-dependent migration and acceleration of cancer stem cells. Anal. Chem. 87, 7098–7108. doi:10.1021/acs.analchem.5b00873
Keywords: 3D models, spheroids, patient-derived organoids (PDOs), chips, drug screening, personalized medicine
Citation: Cordeiro S, Oliveira BB, Valente R, Ferreira D, Luz A, Baptista PV and Fernandes AR (2024) Breaking the mold: 3D cell cultures reshaping the future of cancer research. Front. Cell Dev. Biol. 12:1507388. doi: 10.3389/fcell.2024.1507388
Received: 07 October 2024; Accepted: 13 November 2024;
Published: 26 November 2024.
Edited by:
Daniela Gabbia, University of Padova, ItalyReviewed by:
Roshan Kumar, Medical College of Wisconsin, United StatesEric Ramirez-Salazar, Wistar Institute, United States
Copyright © 2024 Cordeiro, Oliveira, Valente, Ferreira, Luz, Baptista and Fernandes. This is an open-access article distributed under the terms of the Creative Commons Attribution License (CC BY). The use, distribution or reproduction in other forums is permitted, provided the original author(s) and the copyright owner(s) are credited and that the original publication in this journal is cited, in accordance with accepted academic practice. No use, distribution or reproduction is permitted which does not comply with these terms.
*Correspondence: Pedro V. Baptista, cG12YkBmY3QudW5sLnB0; Alexandra R. Fernandes, bWEuZmVybmFuZGVzQGZjdC51bmwucHQ=
†These authors have contributed equally to this work