- 1Laboratory of Genetics and Disorders, Key Laboratory of Molecular Medicine and Biotherapy, Aerospace Center Hospital, School of Life Science, Beijing Institute of Technology, Beijing, China
- 2Department of Pathology, Aerospace Center Hospital, Beijing, China
- 3Advanced Technology Research Institute, Beijing Institute of Technology, Jinan, China
Various methods have been developed so far for detecting N6-methyladenosine (m6A). The total m6A level or the m6A status at individual positions on mRNA can be detected and quantified through some sequencing-independent biochemical methods, such as LC/MS, SCARLET, SELECT, and m6A-ELISA. However, the m6A-detection techniques relying on high-throughput sequencing have more effectively advanced the understanding about biological significance of m6A-containing mRNA and m6A pathway at a transcriptomic level over the past decade. Various SGS-based (Second Generation Sequencing-based) methods with different detection principles have been widely employed for this purpose. These principles include m6A-enrichment using antibodies, discrimination of m6A from unmodified A-base by nucleases, a fusion protein strategy relying on RNA-editing enzymes, and marking m6A with chemical/biochemical reactions. Recently, TGS-based (Third Generation Sequencing-based) methods have brought a new trend by direct m6A-detection. This review first gives a brief introduction of current knowledge about m6A biogenesis and function, and then comprehensively describes m6A-profiling strategies including their principles, procedures, and features. This will guide users to pick appropriate methods according to research goals, give insights for developing novel techniques in varying areas, and continue to expand our boundary of knowledge on m6A.
1 Introduction
In the past decade, it was realized that chemical modifications in internal regions of mRNA and long-noncoding RNA (lncRNA) comprise an important layer of gene regulation (Shi et al., 2019), leading to the emergence of the exciting field of epitranscriptomics. Although the epigenetic code in chromatin is widely accepted, it is still unclear whether RNA possesses similar epitranscriptomic code (Fu and He, 2012). As early as the 1970s, m6A modification was found in mRNA and lncRNA of eukaryotes. So far, the top internal base modifications observed in poly(A)-tailed RNAs are m6A, m1A and m5C. Among them, m6A is the most widespread one, accounting for 0.2%–0.6% of all adenosines (Desrosiers et al., 1974; Perry et al., 1975). It was reported that m6A modification regulates mRNA splicing, translation, degradation, and thus takes part in varying physiological processes such as neural development, cell fate transition, immune response, and DNA damage repair (Patil et al., 2016; Xiang et al., 2017; Wang et al., 2018; Winkler et al., 2019).
Extensive exploration has elucidated the major proteins involved in m6A pathway. These factors can be categorized as writers for m6A synthesis, erasers for m6A removal, and readers for m6A recognition. The m6A modification is deposited on mRNA co-transcriptionally in the nucleus (Ke et al., 2017) by a ∼1 MDa m6A writer complex. This complex consists of a hetero-dimer core component, methyltransferase-like 3 (METTL3) (Narayan and Rottman, 1988) and methyltransferase-like 14 (METTL14) (Figure 1A) (Liu et al., 2014). The crystal structure of METTL3-METTL14 complex showed that METTL3 is the catalytic subunit transferring a methyl group from donor SAM (S-adenosyl methionine) to acceptor adenine to form m6A (Wang C. et al., 2016; Wang P. et al., 2016; Sledz and Jinek, 2016). Knocking out METTL3 in Arabidopsis (Zhong et al., 2008), yeasts (Agarwala et al., 2012) or mammalian cells (Geula et al., 2015) results in complete or near-complete m6A depletion in poly(A)-tailed RNAs. Although METTL14 lacks SAM-binding domain and catalytic activity, it is known as an essential partner of METTL3, cooperating with METTL3 on their substrate RNA capture (Wang C. et al., 2016; Wang P. et al., 2016; Sledz and Jinek, 2016). In addition to the core component, alternative accessory proteins were found in the writer complex. These include Wilm’s tumor 1 associated protein (WTAP) (Schwartz et al., 2014b; Liu et al., 2014; Ping et al., 2014), Vir like m6A methyltransferase associated (VIRMA) (Horiuchi et al., 2013; Schwartz et al., 2014b), zinc finger CCCH-type containing 13 (ZC3H13) (Knuckles et al., 2018), HAKAI (Ruzicka et al., 2017; Bawankar et al., 2021; Wang et al., 2021) and RNA binding motif protein 15/15B (RBM15/15B) (Horiuchi et al., 2013; Patil et al., 2016). WTAP was reported to be an essential adaptor of METTL3 and METTL14, guiding their localization to the nuclear speckles, the loci of splicing and transcription (Ping et al., 2014). VIRMA interacts with WTAP, and depletion of VIRMA causes substantial loss of m6A (Haussmann et al., 2016; Lence et al., 2016; Kan et al., 2017). Specially, RBM15 mediates the binding of m6A methyltransferase complex to the U-rich RNA region adjacent to DRACH motif in a WTAP-dependent way (Patil et al., 2016). This model explained how m6A sites are selected from the highly-frequent consensus DRACH (D refers to G, A or U; R refers to G or A; H refers to A, C or U) sequences on mRNAs (Dominissini et al., 2012; Meyer et al., 2012; Fu et al., 2014; Linder et al., 2015) (Figure 1A). Other m6A writers such as METTL16 (Pendleton et al., 2017; Satterwhite and Mansfield, 2022) and a zinc finger protein ZCCHC4 (Ma et al., 2019) were identified to catalyze m6A synthesis on a subset of mRNAs, snRNAs, and rRNAs in the different sequence and structure context.
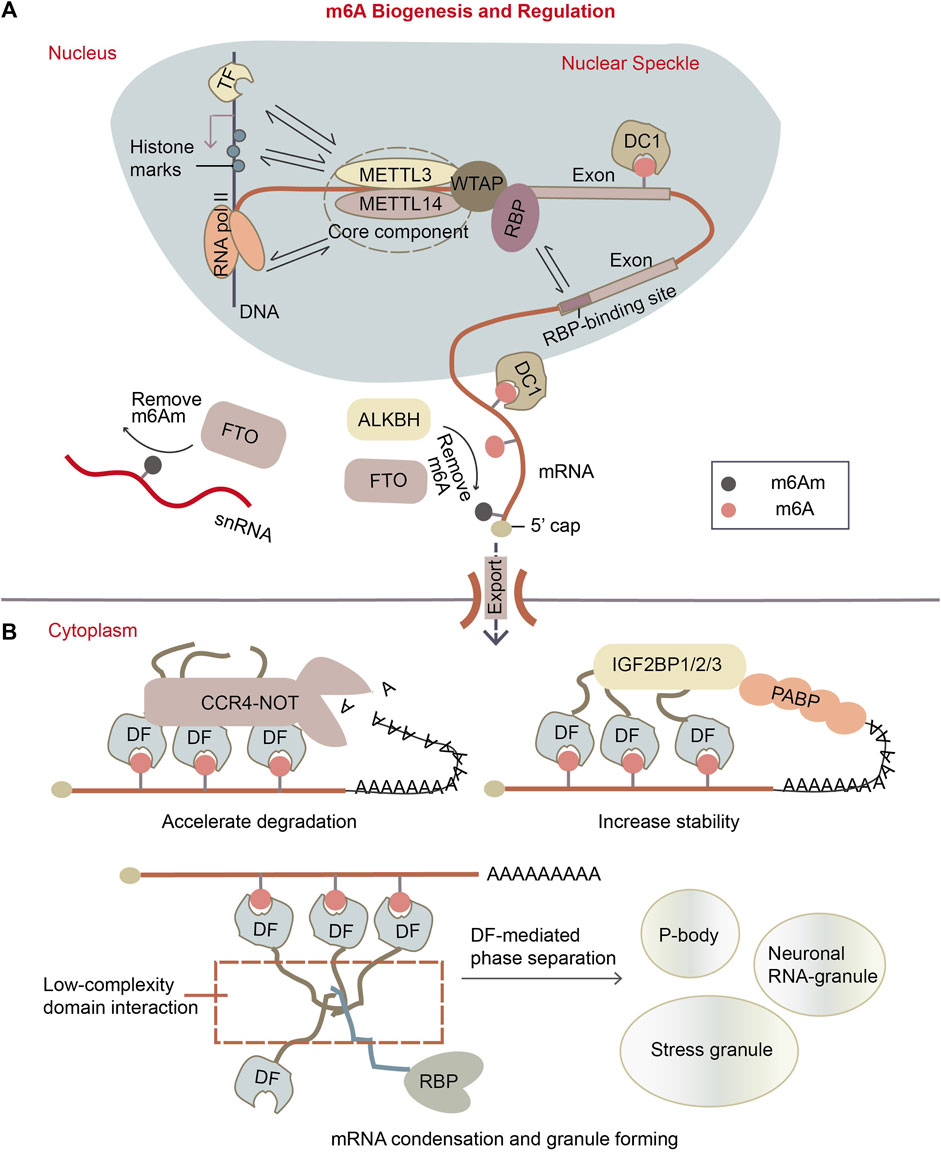
Figure 1. m6A biogenesis and regulation. (A) m6A is installed to mRNAs in the nucleus co-transcriptionally. The m6A writer complex, which comprises the core component of methyltransferase-like protein 3 (METTL3)/14 (METTL14) heterodimer and its accessory proteins. It is located in the nuclear speckle. It targets the potential m6A sites by recruiting RBPs as adapters, it can methylate A site in “DRACH” motif near the RBP-binding sites. Or, writer complex might be recruited to transcription loci by transcription factors (TFs) or histone marks, so methylation can also happen on some specific mRNAs. The m6A erasers are largely localized in the nucleus as well. The main m6A eraser acting on m6A on mRNA is ALKBH5. Fat mass and obesity associated protein (FTO) is found to preferentially target m6Am, especially on small nuclear RNAs (snRNAs). While in the nucleus, m6A can be recognized by specific nuclear reader proteins, mainly YTHDC1 (DC1), which may affect nuclear processes such as transcription, splicing, mRNA exportation. (B) Upon mRNA exports to the cytoplasm, m6A is recognized by specific reader proteins like YTHDF1/2/3 (DF1/2/3) that affect mRNA stability and localization of the mRNA. DFs mediate the degradation of m6A mRNAs by recruiting CCR4-NOT deadenylation complex, while the IGF2BP1/2/3 (insulin-like growth factor 2 mRNA-binding proteins) enhance m6A mRNA stability. Besides, DFs can make mRNA condensates through the low-complexity domain of proteins, forming granules like p-bodies, stress granules and neuronal RNA granules.
Two proteins FTO (fat mass and obesity-associated protein) and ALKBH5 (α-ketoglutarate-dependent dioxygenase alkB homolog 5) were reported to demethylate m6A on mRNA (Figure 1A). FTO protein was determined as demethylase of m6A on mRNA in vitro and in vivo (Jia et al., 2011; Fu et al., 2013). Later, it was revealed that FTO can also demethylate another similar modification m6Am (N6, 2′-O-dimethyladenosine) on snRNA and cap-m6Am on mRNA (Mauer et al., 2017; Mauer et al., 2019). FTO protein is mainly localized in the nucleus, but it was also found in the cytoplasm in particular cell lines (Gulati et al., 2014). ALKBH5 was defined as another m6A eraser on mRNA (Zheng et al., 2013). It is primarily located in the nucleus (Linder et al., 2015) and is most highly expressed in testis. ALKBH5-mediated m6A downregulation influences several cellular pathways such as germ cell development and tumor cell proliferation (Zheng et al., 2013; Zhang et al., 2016a; Zhang et al., 2016b; Zhang et al., 2017).
The molecular function of m6A is majorly mediated by m6A readers with YTH domains, which are capable of recognizing and binding to m6A modification (Luo and Tong, 2014; Theler et al., 2014). These readers include nuclear protein YTHDC1 (DC1) and cytoplasmic proteins YTHDF1/2/3 (DF1/2/3). DC1 regulates mRNA transcription, splicing, and nuclear export through binding to m6A (Figure 1A) (Xiao et al., 2016; Roundtree et al., 2017b), while its paralog DC2 is unlikely involved in m6A pathway due to its low affinity to m6A (Li et al., 2022; Saito et al., 2022). There are still some arguments about what exactly DF proteins do on mRNA. Early research reported that DF paralogs exert different effects on their target mRNAs: DF1 and DF3 promote mRNA translation while DF2 accelerates mRNA degradation (Wang Y. et al., 2014; Wang et al., 2015; Shi et al., 2017). However, recent studies proposed that DF1/2/3 proteins likely have redundant functions without distinguishable preference for particular m6A sites (Lasman et al., 2020; Li et al., 2020; Zaccara and Jaffrey, 2020), and all three DF paralogs can recruit the deadenylation complex CCR4-NOT, thereby decreasing transcript stability (Figure 1B) (Zaccara and Jaffrey, 2020). DF factors can also be condensed through their low-complexity domains (Patil et al., 2018), forming functional phase-separated liquid droplets like p-bodies, stress granules or other RBP granules (Figure 1B) (Ries et al., 2019). Moreover, DF proteins have interaction with various RNA-binding proteins according to proximity labeling experiment (Zaccara and Jaffrey, 2020). This suggests that they possibly regulate mRNA metabolism in different ways through forming diverse reader complexes.
The m6A modification takes part in many biological and pathological processes so a variety of disorders occur once the m6A distribution, stoichiometry or readers changed in cells. It was reported that the m6A pathway regulates the balance between cell pluripotency and differentiation during organismal development (Batista et al., 2014; Chen et al., 2015). Moreover, altered m6A levels on specific gene transcripts are relevant to cancers. The decreased m6A levels on NANOG or FOXM1 mRNAs make the mRNAs stable, leading to the increase in cancer stem cells in breast cancer and glioblatoma (Zhang et al., 2016a; Zhang et al., 2017). On the contrary, the elevation of m6A level on oncogene c-myc mRNA enhances the stability and translation of the transcripts, and thus promotes the self-renewal and proliferation of the leukemia stem cells (Barbieri et al., 2017; Weng et al., 2018; Wang J. et al., 2020; Yankova et al., 2021). The m6A modification also accompanies viral infection (Lichinchi et al., 2016; Wu et al., 2019). HIV infection increases m6A levels on both virus and host mRNAs, and reducing m6A by either downregulation of writers METTL3/METTL14 or upregulation of eraser ALKBH5 suppresses HIV replication. All these observations revealed the functional significance of the m6A pathway.
There are still some arguments in the field regarding m6A biology, regulation, and function. The classic view believed that m6A is a reversible and dynamic modification, meaning that it can be methylated and demethylated in a regulated manner through its lifecycle (Roundtree et al., 2017a). Recent theory proposed that m6A modification is more likely static, determined by gene architecture, for example the lengths and distribution of exons and introns, and the main role of m6A in the cytoplasm is to mark mRNAs for degradation (Murakami and Jaffrey, 2022). The arguments are mainly focused on whether, how and when m6A positions and levels are regulated, and what are the main effects of m6A modification on mRNA. It is important to examine whether m6A regulation at installing stage is owing to recruitment of writer complex to certain chromatin loci by some transcription factors or epigenetic marks, and whether it is achieved through alterations in the composition or activity of writer complex. Additionally, it is interesting to investigate whether there are more writers, erasers, and readers that function in either the nucleus or the cytoplasm, enabling dynamically reversible m6A modifications. The development of sophisticated m6A profiling techniques, especially those can provide stoichiometry information for each identified m6A site, will help us know more about m6A biology, regulation, and function.
In the past decade, many methods were developed to detect m6A locations and quantify m6A levels on mRNA. They can be categorized into sequencing-independent biochemical methods, SGS-dependent methods, and TGS-dependent methods. The sequencing-independent methods adopted digestion, qPCR, or ELISA to measure m6A (Figure 2). These methods can only quantify total m6A levels on mRNA or measure m6A at individual sites. As the next/second generation sequencing (NGS/SGS) and third generation sequencing (TGS) have undoubtedly become a major force driving the progression of life science (Goodwin et al., 2016), many high throughput-sequencing-based strategies, which aimed at transcriptomic m6A profiling, have been rapidly developed. Based on the ways to capture m6A sites, they are categorized as anti-m6A antibody-dependent and antibody-independent methods. The first-launched method meRIP-seq relied on anti-m6A antibody (Dominissini et al., 2012), which has led a trend in popping up antibody-dependent methods (Supplementary Table S1). Even though these methods have made a valuable contribution, they are limited majorly by the promiscuous nature of the antibody (Schwartz et al., 2013; Schwartz et al., 2014a; Linder et al., 2015; McIntyre et al., 2020) and the absence of high-resolution stoichiometry information. To overcome these problems, antibody-free methods have been developed (Supplementary Table S1). These methods were focused on distinguishing unmodified A-base from m6A, taking advantage of RNA enzymes such as m6A eraser FTO (m6A-SEAL-seq), RNA-editing enzyme APOBEC1 (DART-m6A-seq) or TadA (eTAM-seq), RNA endonuclease MazF (Mazter-seq) (Meyer, 2019; Pandey and Pillai, 2019; Zhang et al., 2019; Wang Y. et al., 2020; Hu et al., 2022; Xiao et al., 2023). In addition, some methods captured m6A sites by in vitro chemical labeling of nitrite-mediated deamination (GLORI) or metabolic labeling of allyl-modified SAM analogs (m6A-label-seq and m6A-SAC-seq) (Shu et al., 2020; Ge et al., 2023; Liu et al., 2023), while others employed algorithms to predict m6A directly with TGS data (Liu et al., 2019; Leger et al., 2021; Pratanwanich et al., 2021).
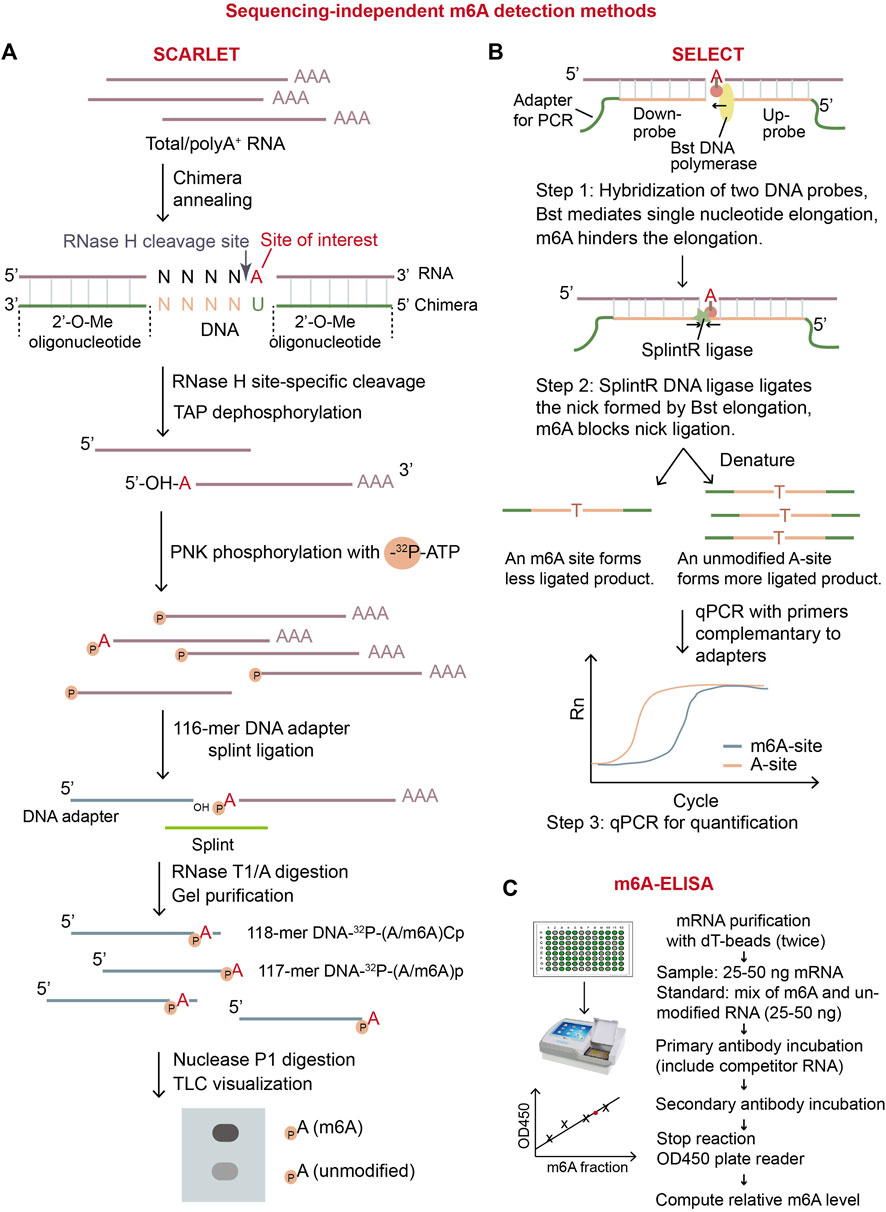
Figure 2. Overview of three sequencing-independent m6A detection methods. (A) Schematic diagram of SCARLET. In this method, specific site of interest is labeled with 32P, and then separate and visualized by TLC. The intensity of the signals of dots representing m6A ribonucleotide and A ribonucleotide will precisely quantify the fraction of m6A on the specific site. (B) Schematic diagram of SELECT (Xiao et al., 2018). This method exploits the principle that m6A modification disturbs DNA elongation and nick ligation at the modified position. Therefore, the quantity of the resulting ligated-DNA oligo represents modification status of A-site on RNA. (C) Illustration of m6A-ELISA. This method can be easily conducted by using commercial m6A-ELISA kit. The major steps are listed in the diagram. TAP: thermosensitive alkaline phosphatase; PNK: polynucleotide kinase.
In this review, we will introduce current m6A detection methods employing sequencing-independent biochemistry or SGS/TGS technology, and discuss their strengths and limits.
2 Sequencing-independent m6A detection methods
Several sequencing-independent biochemical methods can detect and quantify m6A on RNA. These methods include the digestion-based LC/MS (liquid chromatography-tandem mass spectrometry) and SCARLET (Site-specific Cleavage and Radioactive Labeling followed by ligation-assisted Extraction and Thin-layer chromatography), the qPCR-based SELECT (single-base elongation-and ligation-based qPCR amplification method), and ELISA-based m6A-ELISA (Figure 2). Importantly, m6A-ELISA and LC/MS can quantify the m6A levels in total mRNA while SCARLET and SELECT can examine m6A status in individual sites of interest.
The m6A-ELISA method is commercially available. The m6A-ELISA kit provides a standard method to calculate the total m6A levels in RNA samples by using anti-m6A antibody to label m6A-containing RNA (Figure 2C) (Bringmann and Luhrmann, 1987; Ensinck et al., 2023). The advantages of m6A-ELISA are obvious. It is easy to conduct that the whole protocol can be finished in less than a day. It is cost effective and convenient as commercial ELISA kit is available. It has the potential to be adapted for detection of any modifications on RNAs. On the contrary, its disadvantages include limited sensitivity and the absence of location information of m6A. To summarize, m6A-ELISA can be applied to determine relative levels of total m6A in multiple samples.
LC/MS is a commonly-used, total m6A quantification method based on digestion (Thuring et al., 2017; Zaccara et al., 2019; Mathur et al., 2021). In this method, the phosphodiester bonds in purified mRNAs are hydrolyzed with nuclease P1, and the generated nucleoside 5′-monophosphates are further dephosphorylated with alkaline phosphatase for LC-MS analysis. It is an extremely accurate, sensitive, and quantitative method in which m6A nucleoside produces a characteristic mass spectrum (Thuring et al., 2017). The digestion-based LC/MS method can only quantify the total m6A level in mRNA, but not reveal modified gene identities. Also, the contamination from other abundant RNAs such as rRNA will affect the quantification accuracy.
Another widely-used method SCARLET aims to quantify m6A at a given site (Figure 2A) (Liu et al., 2013). In digestion-based method SCARLET, the chemically-modified oligonuleotide (2′-OMe)6–8(2′-H)4(2′-OMe)6–8 first hybridizes to the target m6A region on mRNA. Then, RNase H cleaves the hybrid mRNA site specifically at 5′ of the target adenosine regardless of modified or not. The 5′ end of the adenosine is further labeled with 32P and the radio-labelled mRNA fragment is splint-ligated with a 5′ adaptor, single-stranded 117-mer DNA, using DNA ligase. The RNA part in the ligated fragment is digested with RNase T1/A, the remaining adaptor DNA part with 32P-labeled unmodified-adenosine or m6A at its 3′end (117-mer DNA-32P-(A/m6A)p and 118-mer DNA-32P-(A/m6A)Cp) is gel-purified, digested into mono-nucleotides using nuclease P1. The 32P-labeled adenosine or m6A is visualized and quantified by thin-layer chromatography (TLC) (Liu et al., 2013). Although SCARLET is laborious and requires to use radioactive isotope, it can accurately quantify m6A level in a specific site at single-base resolution. Therefore, it has been used as the “gold standard” in m6A stoichiometry for individual desired sites (Zaccara et al., 2019).
An economic and time-saving qPCR-based method, SELECT, can also determine m6A status at individual sites (Figure 2B) (Xiao et al., 2018). SELECT exploits the ability of m6A to hinder both the single-base elongation by DNA polymerase Bst and the nick-ligation by ligase SplintR (Xiao et al., 2018). In SELECT, two DNA probes, including adapters for qPCR, hybridize to the mRNA region flanking an adenosine site (A-site) of interest. After treatment of Bst and SplintR, if the A-site of interest is unmodified, two DNA probes are ligated efficiently, forming a large number of the full DNA fragments with both up and down adapters; otherwise, if the A-site is modified, two probes are less likely to be ligated, forming very few of the full DNA fragments with both adapters. The full DNA fragments are later amplified by qPCR for quantification. Only the ligated DNA with both up and down adapters can be amplified in qPCR, thus, the value of threshold cycle (CT) of qPCR reflects the initial m6A stoichiometry of the given site. In the experiment, another unmodified A-site near the m6A site of interest on the same mRNA should be used for quantification of the input mRNA with the given m6A site. SELECT is not able to discriminate different modification types on adenosine, meaning that m6A, m1A, and Am will give similar decrease in qPCR signals compared to unmodified adenosine. To validate the modification type, SELECT requires additional RNA samples which have undergone in vitro treatment with m6A eraser or in vivo depletion of m6A biogenesis factors. In general, SELECT can achieve absolute quantitation by introducing standard curves and has potential for wider use in the future because of its flexibility and convenience.
These biochemical methods can quantify the m6A levels in total RNA or at individual sites, however, they cannot give us a comprehensive view on m6A distribution and stoichiometry at a transcriptome level. So, many strategies based on high-throughput sequencing have been emerged.
3 SGS-based m6A detection methods
3.1 Methods relying on anti-m6A antibody
3.1.1 MeRIP-seq
MeRIP-Seq (or m6A-seq) is the first-developed and most-widely-used method for transcriptomic profiling m6A sites on mRNA. In this method, mRNA is randomly fragmented and immunoprecipitated using m6A-specific antibody. Next, RNA-seq libraries are prepared from input and immunoprecipitated RNA according to standard protocol (Figure 3). In theory, the sites closer to m6A will have the higher read coverage. Therefore, when the meRIP sequencing reads are mapped to a reference genome, m6A sites will be centered in the read peaks (Dominissini et al., 2012; Meyer et al., 2012).
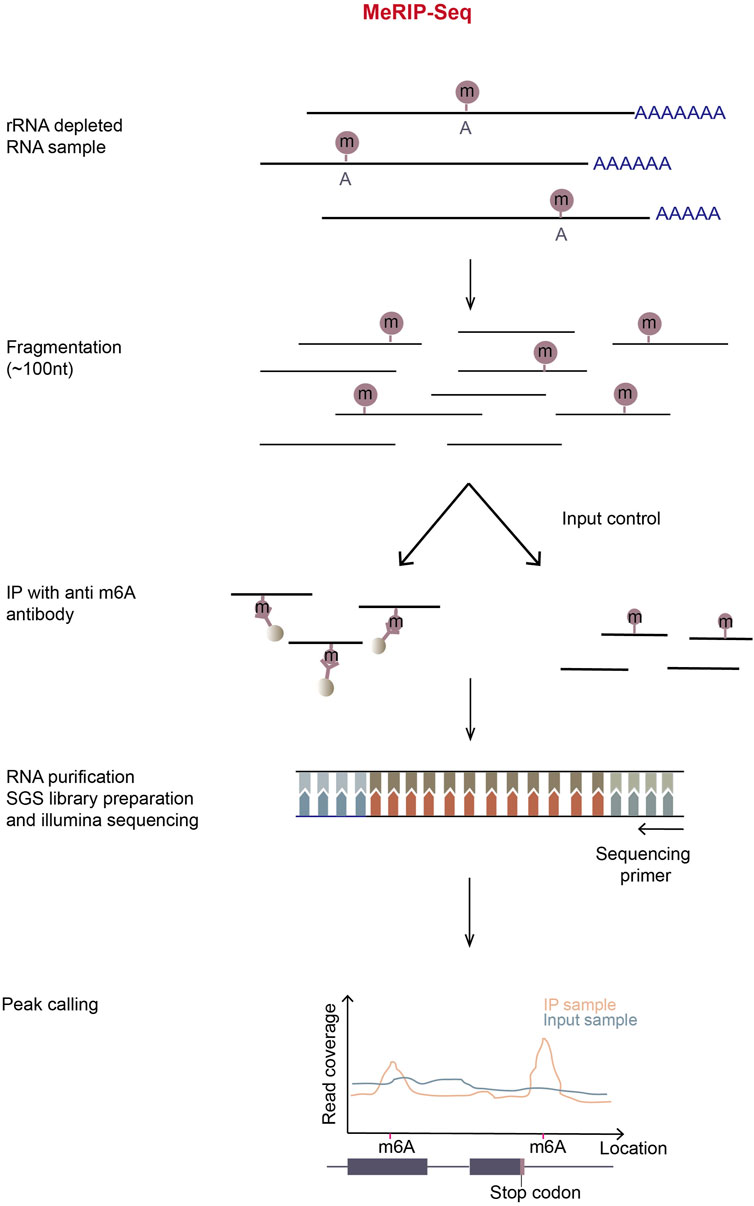
Figure 3. Schematic representation of MeRIP-Seq. In MeRIP-seq, rRNA-depleted or polyA-enriched RNA samples are obtained by treating total RNA with the RiboMinus kit or oligo-dT-conjugated beads and then chemically fragmented with metal ions in appropriate incubation temperature and time. A portion of fragmented RNA is saved for input control, and the residual/remaining RNA is immunoprecipitated using m6A-antibody-coupled Dynabeads. Immunoprecipitation (IP) can be repeated to enhance signal-noise ratio. The input and m6A-enriched RNA fragments are then subjected to the normal RNA-seq library preparation process. The libraries are then sequenced in illumina sequencing platform. The subsequent reads generated are aligned to the reference genome, most reads will pile up at m6A locations in the IP sample. By calculating the read coverage at each location, peaks can be observed around the m6A locations in MeRIP samples.
MeRIP-Seq is relatively stable, convenient, fast, and cost-effective, and can be used in large scale experiments (Zhang et al., 2022). In spite of its usefulness, this method requires a large amount of starting RNA and has high noise background. Furthermore, anti-m6A antibodies possibly mis-recognize other modifications similar to m6A, such as m6Am. Also, only the regions with high methylation levels can be identified with low resolution of m6A sites.
To overcome the low resolution and high background problems, UV-crosslinking-based m6A mapping methods giving nucleotide resolution were subsequently developed (Linder et al., 2015; Koh et al., 2019; Roberts et al., 2021).
3.1.2 miCLIP, meCLIP and m6ACE-seq
miCLIP (m6A individual-nucleotide-resolution cross-linking and immunoprecipitation) has a similar idea to iCLIP method which clarifies the protein-binding sites on RNA (Figure 4A, left) (Konig et al., 2010; Linder et al., 2015). In miCLIP, anti-m6A antibody and fragmented mRNA are incubated together, then the antibody and m6A on mRNA are UV-crosslinked. The RNA-antibody complex is immunoprecipitated and the 5′ end of RNA is radioactively labeled using [γ-32P] ATP through PNK (polynucleotide kinase) reaction. Next, through the denaturing NuPAGE gel-run and the nitrocellulose membrane-transfer, the antibody-RNA complex is separated, visualized and purified. Partial digestion with proteinase K leaves peptide residues on m6A nucleotides, which leads to abortion or introducing C-to-T nucleotide transition at m6A positions during reverse transcription (RT) (Linder et al., 2015). miCLIP can therefore achieve nucleotide resolution in determining m6A sites. However, the radio-isotope labeling will complicate the experiment and low UV-crosslinking efficiency will decrease library complexity. Another CLIP-based technique, MeCLIP (m6A eCLIP) (Roberts et al., 2021) has simplified the process by omitting the steps of radio-labeling and visualizing RNA (Figure 4A, right). In addition, two linear adapters are ligated to RNA/cDNA fragments separately and unique molecular identifier (UMI) is included in the RT primer, which has improved library complexity of MeCLIP.
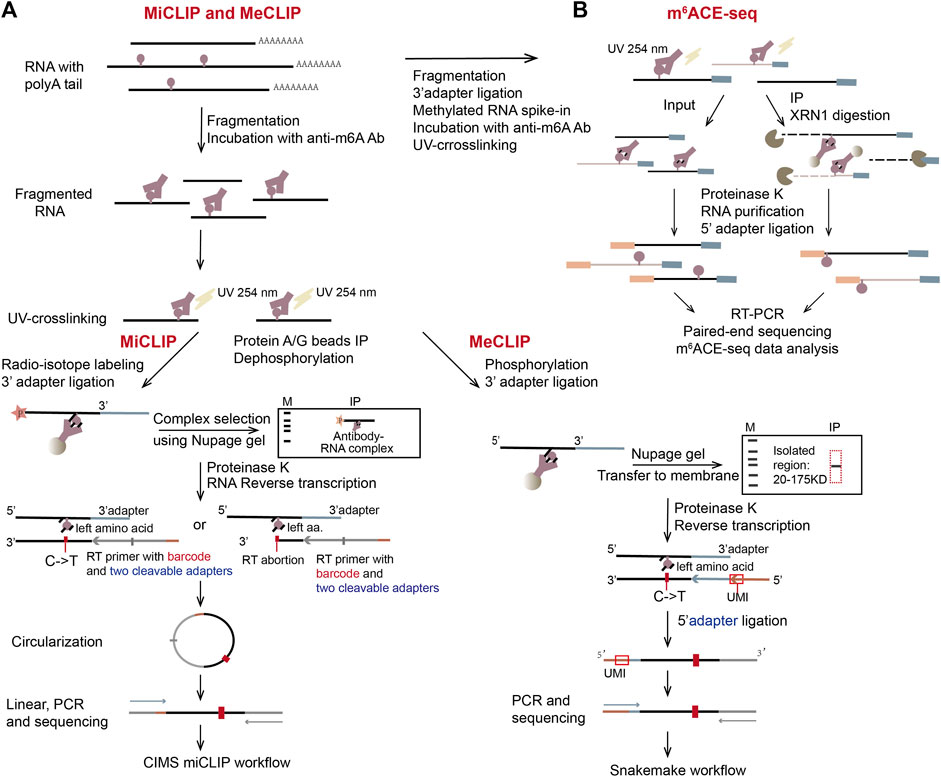
Figure 4. Schematic representation of three CLIP-based methods, miCLIP, meCLIP, and m6ACE-seq. (A) The representation of miCLIP and meCLIP. The two workflows are similar. Three main differences are: fragmented RNAs (100 nt–200 nt) are longer in meCLIP than those in miCLIP; meCLIP omits the radio-labeling step and its procedure includes size-specific isolation of the RNA-antibody complex with the protein ladder in NuPAGE gel; meCLIP ligates 3′ adaptor and 5′ adaptor separately instead of the cDNA circularization. (B) The representation of m6ACE-seq. Instead of gel purification, m6ACE-seq utilizes 5′ to 3′ exonuclease XRN1 to digest the antibody-crosslinked mRNAs from their 5′ ends.
In 2019, another CLIP-based method m6ACE-seq (m6A-cross-linking-exonuclease sequencing) was developed (Koh et al., 2019). In this method, m6A and its antibody are UV-crosslinked and immunoprecipitated, then RNA-antibody complex is digested with a 5′-to-3′ exonuclease XRN1 (Figure 4B). The RNA digestion will stop at crosslinked m6A positions, by which m6A sites are precisely located at single nucleotide resolution (Koh et al., 2019). Compared to the other two CLIP-based methods, m6ACE-seq has abandoned most of the complicated steps. Instead, it uses XRN1 to digest mRNA fragments from the 5′ ends to the first m6A positions, which are protected by crosslinked antibody. Therefore, the following NGS paired-end RNA-seq can produce reads that pile up at locations of m6A, achieving single nucleotide resolution in m6A detection.
3.2 Methods relying on enzymes
3.2.1 DART-m6A-seq
Antibody-free methods have been developed since 2019. The first one is DART-seq (deamination adjacent to RNA modification targets sequencing) (Meyer, 2019; Flamand and Meyer, 2022). The method employs a RNA modification enzyme-based fusion protein system. The fusion protein methodology has been widely applied to detect interactions between RNA-binding proteins (RBPs) and their target RNAs by constructing the fusion protein of a given RBP with an RNA-modification enzyme (Lapointe et al., 2015; McMahon et al., 2016; Jin et al., 2020; Brannan et al., 2021; Piao et al., 2023). It has also been applied for CRISPR-Cas9-based genome editing, where deactivated Cas9 (dCas9) is fused with the nucleic-acid-editing enzyme APOBEC1 to edit desired bases in target genomes under the guidance of guide RNAs (gRNAs) (Komor et al., 2016).
DART-seq takes advantage of the fusion protein of an RNA-editing enzyme APOBEC1 and the YTH domain of m6A reader protein YTHDF2 to mark m6A sites both in cells and in vitro (Figure 5B) (Meyer, 2019). When the fusion protein is expressed in cells, the YTH domain in the fusion protein (Wang X. et al., 2014; Schwartz et al., 2014b) is able to be recruited to the m6A sites. Its APOBEC1 domain could then deaminate adjacent cytosines to uracils in cells. These C-to-U conversions can be identified by conventional mRNA-seq in short-read SGS or long-read TGS platform. Consistent with the known m6A-consensus motif DRm6ACH (Figure 5A), C-to-U transitions are frequently detected at cytosines adjacent to the motif.
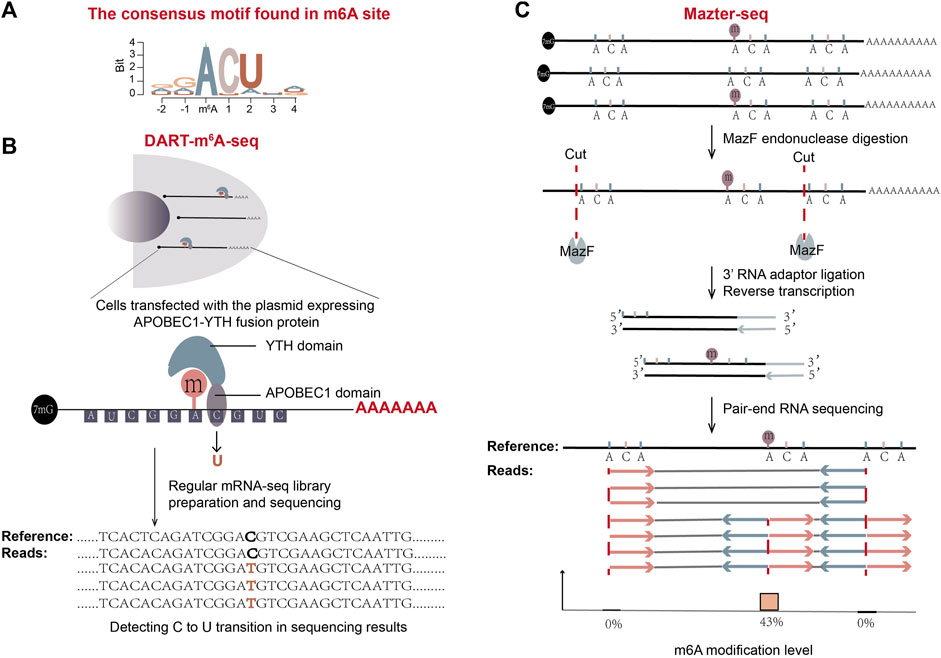
Figure 5. Schematic representation of two antibody-free methods: DART-m6A-Seq and Mazter-Seq. (A) The consensus motif found in m6A sites is shown. (B) The workflow of DART-m6A-seq. (C) The workflow of Mazter-seq (Garcia-Campos et al., 2019). After digestion with MazF, the generated RNA fragments mostly start with 5′ACA. The following standard pair-end RNA-seq gives sequencing reads that are of internal or terminal ACA. The ratio of sequencing reads with internal ACA to reads split at 5′ ends of ACA represents the relative quantity of methylation.
DART-seq requires only a small amount of starting material and has a relatively high recurrence rate. In addition, when DART-seq is combined with a TGS long-read platform, it is able to profile mRNA isoform-specific methylation patterns (Meyer, 2019). Utilizing single-cell sequencing like 10x genomics SGS platform, DART-seq has achieved the single-cell level scDART-seq in 2022, and discovered instinct m6A signatures of individual cells independent from gene expression profiles (Tegowski et al., 2022). At the same time, this method has a short library construction time and simple operation. However, it is hardly employed in some primary cells with low transfection efficiency and is also time-consuming to clone the fusion protein-expressing plasmids. DART-seq accompanies overexpression artifact especially if protein expression time is long, thus an inducible promoter will be a good choice to express the fusion protein.
3.2.2 Mazter-Seq
Another antibody-free m6A detection method, Mazter-Seq or REF-seq (m6A-sensitive RNA-Endoribonuclease-Facilitated sequencing, a similar method developed by another group) (Garcia-Campos et al., 2019; Pandey and Pillai, 2019; Zhang et al., 2019), utilizes a special type of bacteria-derived single-stranded RNA endoribonuclease MazF, whose activity is sensitive to the methylation status of RNA (Imanishi et al., 2017). MazF can recognize and cleave before the ACA motif. But in the case of m6A-CA, in which the first adenosine is methylated, it can’t (Figure 5C). When the extracted mRNA is digested with endoribonuclease MazF, unmethylated ACA sites generate two types of RNA fragments, one with 5′ terminal ACA sequence and the other with a 3′ terminal ending immediately before ACA. On the contrary, methylated m6A-CA sites generate RNA fragments with internal ACA sequence. The terminal features of sliced RNA fragments are then detected by NGS sequencing (Figure 5C). After alignment of reads to a reference genome, the number of reads split immediate-upstream of ACA and the number of reads spanning the ACA positions are counted for each ACA position. The proportion of reads with internal ACA represents the m6A level at the location (Garcia-Campos et al., 2019; Pandey and Pillai, 2019; Zhang et al., 2019).
The Mazter-Seq method has a low false positive rate and is less laborious than the antibody-based methods. Though it is able to provide stoichiometry information for each detected m6A site, there are two major drawbacks of this method. Firstly, the enzyme activity can be influenced by factors other than methylation state. For example, the secondary structure of RNA at an ACA site may decrease the cleaving efficiency of MazF at the site. Therefore, a parallel control experiment from a methyltransferase-knockout mutant is required to neutralize the background, which will add the complexity to the method. Secondly, because MazF enzyme is only able to slice an “ACA” motif among DRACH motifs, Mazter-Seq can determine m6A at ACA positions but not all possible positions. Data showed that its estimated detection rate of m6A sites is only about 20%.
3.2.3 m6A-SEAL-seq
Compared with Mazter-Seq, m6A-SEAL-Seq (Antibody-free, FTO-assisted chemical labeling method) (Wang Y. et al., 2020) has overcome the sequence context bias in m6A detection. It first uses FTO enzyme to oxidize m6A sites on the fragmented mRNAs in vitro. After m6A is transformed to unstable intermediate hm6A (N6-hydroxylmethyladenosine), the added DTT further converts hm6A to more stable dm6A (N6-dithiolsitolmethyladenosine) (Figure 6A). Next, the RNA fragments are divided into input and dm6A-pulldown groups, and the dm6A-pulldown group undergoes additional treatment. In dm6A-pulldown group, the free sulfhydryl group on dm6A reacts with MTSEA (methanethiosulfonate) on MTSEA-labeled biotin, a commercial thiol-reactive reagent. Later, the biotinylated dm6A is enriched with streptavidin (SA) beads, and the RNA fragments with dm6A are recovered by cleaving the disulfate bonds with DTT. The RNA fragments from input and dm6A-pulldown groups then undergo RNA sequencing respectively. The rough m6A positions will be revealed by the enriched sequencing peaks from the dm6A-pulldown group relative to the input group.
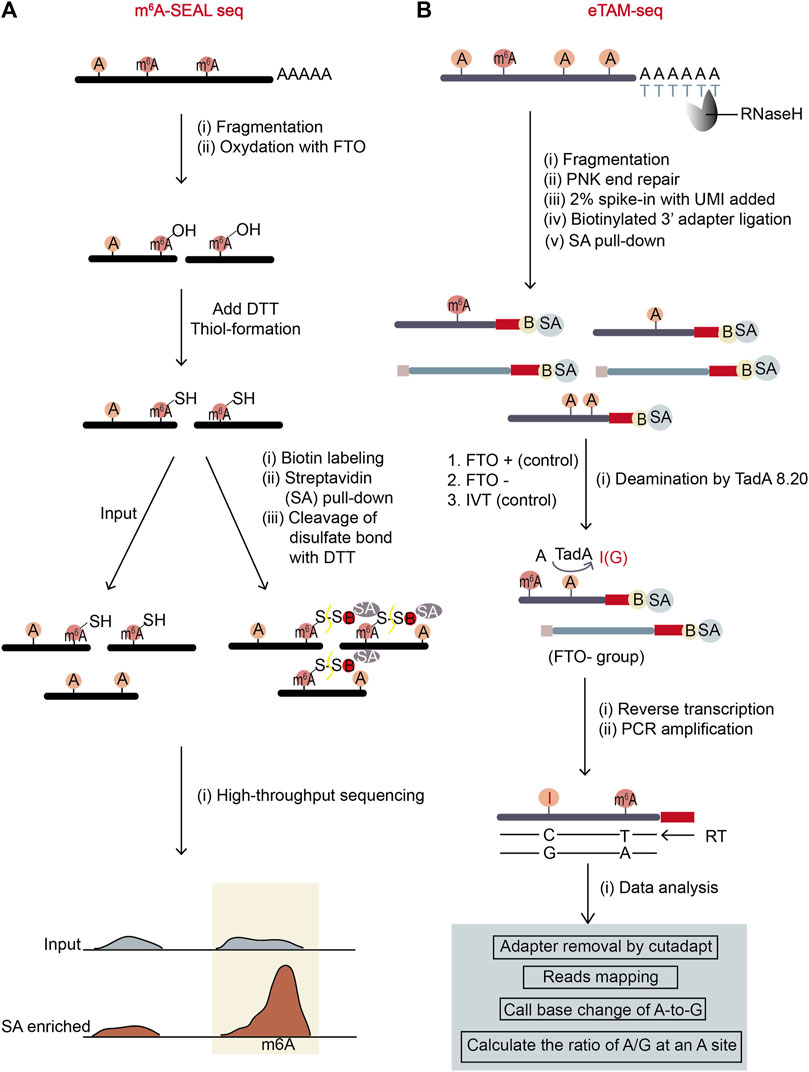
Figure 6. Schematic representation of two antibody-free methods, m6A-SEAL-seq and eTAM-seq. (A) The workflow of m6A-SEAL-seq. The sequential treatment of FTO enzyme and DTT makes m6A sites are biotinylated and enriched by streptavidin (SA)-biotin interaction. The m6A sites are represented by sharp peaks in m6A-pulldown samples. (B) The workflow of eTAM-seq. Unmodified adenosine in fragmented mRNA is turned into inosine by deaminase TadA8.20. Inosine will be recognized as G during NGS sequencing. Therefore, the precise locations and levels of m6A sites can be identified through finding A-sites with low percentages of A-to-G transitions.
This method has complicated experimental procedure as its experimental environment should be controlled properly to retain the intactness of mRNA fragments. It also has low resolution and no stoichiometry information for each m6A site. True m6A sites may be omitted because of the limits on FTO catalytic capability and thiol-labeling efficiency. However, compared to the widely-used meRIP-seq, it is both time saving (the FTO incubation time is as short as 5 min) and cost saving (the usage of biotin-SA for enrichment costs less than the usage of antibody). Further, according to the original publication, the sites found in m6A-SEAL-seq are quite reliable as they overlapped with the sites from other methods to a certain degree.
3.2.4 eTAM-seq
eTAM-seq (evolved TadA-assisted N6-methyladenosine sequencing) is a new method hiring a deaminase to convert unmodified adenosine (A) to inosine (I) in RNA (Xiao et al., 2023). Since inosine reads as guanosine in sequencing, deaminated sites can be identified by A-to-G nucleotide alterations. TadA8.20 was engineered from an E.coli deaminase TadA, possessing robust deamination activity and minimal sequence context dependence (Figure 6B). This hyper-active enzyme can completely deaminate most of unmodified A but not m6A in RNA without breaking the RNA. Therefore, after treating purified RNA with TadA8.20, nearly 99% of A-bases at an unmodified A-site are deanimated and read as G. However, at an m6A site with a certain percentage of m6A modifiaction, unmodified A-bases read as G while m6A-bases read as A. So, the percentage of A-reads at an m6A site represents m6A level at the position. To ensure the accuracy and reduce false positive rates caused by the incomplete deamination of TadA8.20 enzyme, eTAM-seq sets two control groups: one is an RNA sample treated with the m6A eraser FTO, which can remove methyl-group from m6A in vitro (serving as a control to eliminate the bias from other A modifications which are also resistant to TadA8.20); the other is an in vitro transcription (IVT) sample which is an in-vitro-transcribed modification-free cell transcriptome mimic (serving as a control to eliminate the bias from the TadA8.20-not-accessible A-bases in transcriptome) (Figure 6B).
eTAM-seq has lots of merits. It has no sequence bias and provides stoichiometry information for detected m6A sites at single-nucleotide resolution. Importantly, it can quantitatively detect m6A with as few as ten cells in a simple workflow. However, the expression and purification quality of TadA8.20 may affect the sequencing result. To sum up, despite potential limitations, eTAM-seq is an advanced and practical method for m6A site detection and m6A level quantification.
3.3 Methods relying on SAM analogs
3.3.1 m6A-SAC-seq
The method of m6A-SAC-Seq (m6A-selective allyl chemical labeling and sequencing) (Hu et al., 2022; Ge et al., 2023) takes advantage of MjDim1, a dimethyltransferase from M. jannaschii, which can methylate both unmodified adenosine and m6A into a dimethylated form of m6,6A (Figure 7A). The fragmented mRNA was treated with MjDim1 in the presence of a cofactor allylic-SAM instead of SAM. Under this condition, MjDim1 exhibits nearly tenfold preference for m6A over unmodified A-base, and catalyzes m6A into allyl-modified m6A (a6m6A) and unmodified A-base into allyl-modified A (a6A). Subsequent iodine (I2) treatment cyclizes these two substrates respectively, and the cyclized a6m6A (original m6A) generates a tenfold higher mutation rate than cyclized a6A (original unmodified A) in the following reverse transcription with HIV-1 reverse transcriptase (Figure 7A). Thus, the original m6A sites can be identified through their high mutation frequencies in mRNA-sequencing.
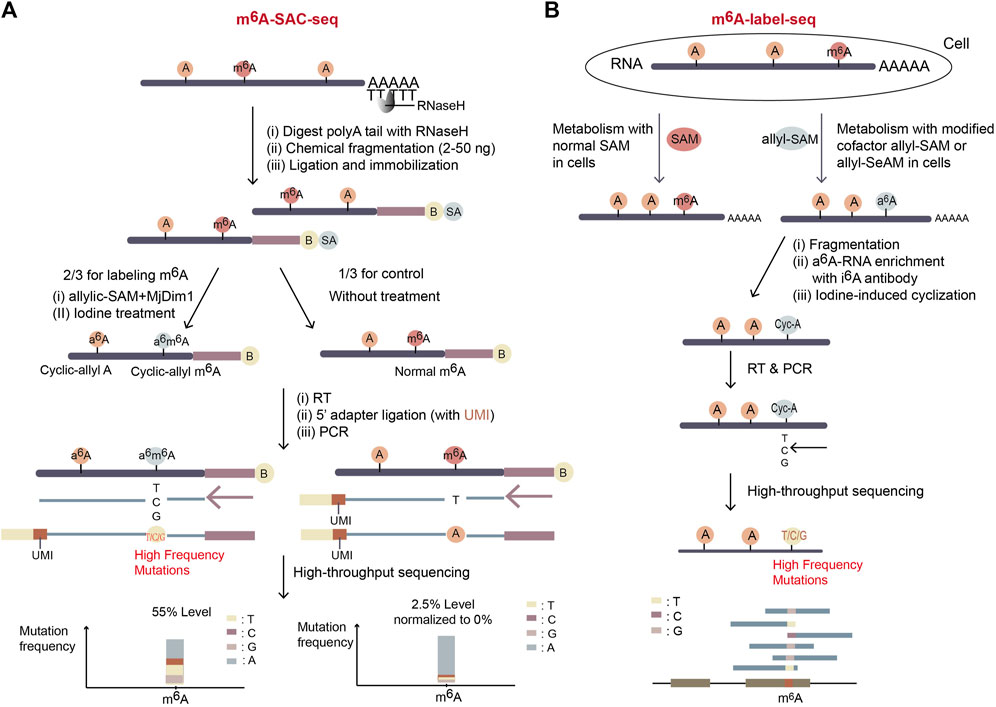
Figure 7. Schematic representation of two SAM analog dependent methods. (A) The workflow of m6A-SAC-seq. It depends on the dimethyltransferase MjDim1 and the cofactor allylic-SAM to turn m6A to a6m6A, which is further cyclized after iodine treatment. And therefore, original m6A sites gain high frequency of mutations during reverse transcription. Further, the mutation frequency at a potential m6A site indicates the m6A level of the site. If a site has mutation frequency more than 5% in the treated group and less than 2.5% in the control untreated group, the site will be considered as a potential m6A site. (B) The workflow of m6A-label-seq. The expected m6A sites on nascent RNA are metabolically labelled as a6A when exposing cells to allyl-SAM or allyl-SeAM. Further iodine-mediated cyclization of a6A sites dramatically increases mutation rates of RT at the sites. The m6A positions and levels are identified by NGS with extraordinary high mutation rates.
So far, since MjDim1 is biased to catalyse mRNA substrates with a more common GAC motif over a less frequent AAC motif, it is uncertain whether all m6A sites could be labeled equally in m6A-SAC-Seq. Further, the complex and time-consuming experimental procedure makes it difficult to use. Nevertheless, this method provides a promising way for m6A profiling since it is free from antibody consumption and rRNA-depleted input can be as low as 30 ng. Also, it reaches single-nucleotide resolution for measuring dynamic change in m6A distribution and stoichiometry of each site. m6A-SAC-Seq has an exciting prospect and can be applicable to many biological studies.
3.3.2 m6A-label-seq
m6A-label-seq (metabolic labeling method detects m6A) (Shu et al., 2020), another method relying on the allyl-modified SAM analogs, was developed in 2020. To distinguish m6A from unmodified A-base, cells are incubated with allyl-SAM or allyl-SeAM so the expected m6A positions are metabolically labeled as N6-allyladenosine (a6A). Fragmented a6A-containing RNA is enriched with the commercial N6-isopentenyladenosine (i6A) antibody and a6A is converted to N1, N6-cyclized adenosine (cyc-A) by iodine treatment. Introduced mutations at cyc-A positions during reverse transcription indicate the m6A sites (Figure 7B).
m6A-label-seq can profile transcriptome-wide m6A positions at base resolution without sequence bias. However, the exposure of living cells to SAM analogs raises some concerns about altered biological pathways, for example, it was reported a weak alteration in gene expression related to endoplasmic reticulum stress and apoptotic signaling (Shu et al., 2020). Also, the step of a6A-RNA enrichment with i6A antibody possibly affects the m6A stoichiometry. Improvement of m6A-label-seq should focus on increasing m6A-labeling yield and meanwhile decreasing artificial effects.
3.4 Methods relying on chemicals—GLORI
GLORI (glyoxal and nitrite-mediated deamination of unmethylated adenosines), a new method developed in 2022, specifically deaminates unmethylated adenosine but not m6A using chemicals, glyoxal (C2H2O2) and nitrite (NaNO2), to locate the original m6A sites in RNA (Liu et al., 2023). It adopted a strategy similar to the bisulfite sequencing method for DNA 5 mC detection (Raiber et al., 2017). In GLORI, glyoxal is first used as a reagent to protect regular G-bases by reacting with their exocyclic amino groups, so the regular G-bases will not be deaminated to X during nitrosation step (Figure 8). Further, glyoxal works as a catalyst to accelerate the deamination of unmodified adenosines in the next step of nitrite existence condition, speeding up the desired A-to-I (G) reaction while concurrently reducing the side reaction of C-to-U deamination. In GLORI, A-to-G transition rate achieved ∼98.0%, with <1.0% G-to-X and ∼3.3% C-to-U transitions. After adenosine deamination, the glyoxal-protected G-bases are recovered to the regular G-bases under alkaline or heated condition. Then, because unmodified A was deaminated to I (G) but m6A was not, the following high-throughput sequencing reveals the m6A positions which didn’t undergo deamination (Figure 8).
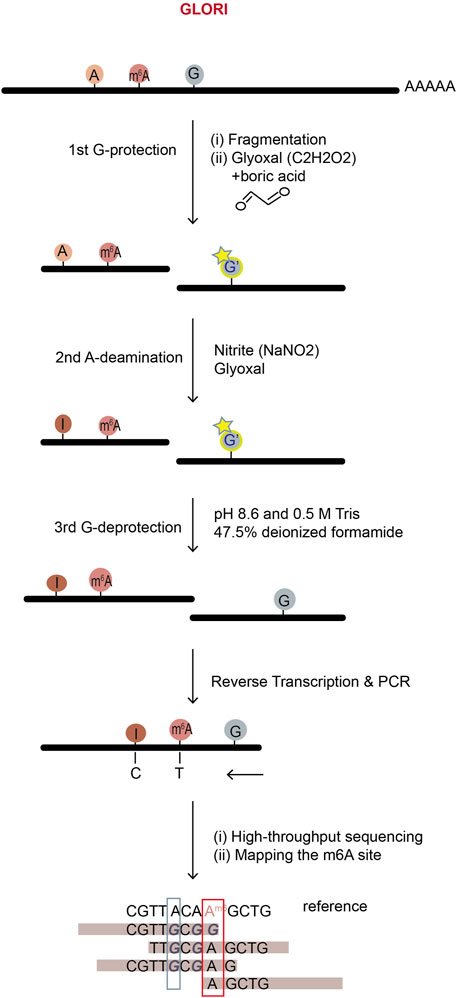
Figure 8. The workflow of GLORI m6A sequencing. GLORI uses nitrite to deaminate unmodified A into I (G), while m6A and G, C nucleotides are protected. Thus, nearly complete A-to-G transition represents an unmodified A-site; incomplete A-to-G transition represents an m6A site and the m6A level can be calculated by the ratio of A to (A + G) at the site in mRNA-sequencing.
GLORI fully depends on chemical reactions, so compared to the methods relying on enzymes, it works more stably with better repeatability and lower cost. It provides quite precise estimation of both m6A positions and stoichiometry using only 100 ng of mRNA. However, the extracted RNA has to undergo a series of complex chemical processing in vitro, which challenges the RNA integrity and might raise concern about mRNA degradation. Nevertheless, a simple and fast protocol at low cost makes GLORI have the potential to be widely used in the field.
4 TGS-based m6A detection methods
The advantages of TGS include simple sequencing library preparation and extremely long sequencing reads, making them suitable for RNA isoform measurement and long genome assembly. Even though TGS has relatively high error rates, development of TGS has pushed the advancement of m6A detection strategies (Saletore et al., 2012). Direct RNA sequencing in Oxford Nanopore Technology (ONT) platform and single-molecule, real-time (SMRT) reverse transcription in PacBio platform have been employed in m6A detection (Schadt et al., 2010; Vilfan et al., 2013; van Dijk et al., 2018; Abebe et al., 2022).
4.1 m6A detection using ONT direct RNA sequencing
Since the modified and unmodified ribonucleotides display differentiated signal patterns in Oxford direct RNA sequencing (DRS), through decoding these signals, DRS has provided a fascinating system for detection of RNA modifications. Thus, DRS combined with computational analysis is able to identify modified sites at single-nucleotide resolution and has been widely applied in profiling various RNA modifications, including those in RNA viruses (Liu et al., 2019; Kim et al., 2020; Leger et al., 2021; Pratanwanich et al., 2021; Abebe et al., 2022; Hong et al., 2022). In Oxford DRS, each consecutive 5-mer nucleotide inside the nanopore determines a blockage effect on the ionic current, so the patterns of current intensity change can be used to identify the transiting nucleotides regardless of whether modifications are present. Although extracting RNA modification information from DRS reads is still challenging, the issue is highly in need to solve (Keller et al., 2018; Zhang R. et al., 2021). New approaches have adopted the analysis of base call error rates or raw signals “squiggles” or both (Loose et al., 2016; Abebe et al., 2022).
4.1.1 The algorithms based on base call error rates
In nanopore DRS, the raw current intensities are recorded in real time from an RNA molecule, forming a squiggle graph. Algorithms such as guppy are able to carry out base calls from raw signals, generating RNA sequences and also assigning a probability score for each nucleotide to signify the accuracy of the call (Soneson et al., 2019; Wick et al., 2019). Because m6A-modified nucleotides affect the ionic current differently from their unmodified counterparts, error rates are significantly high near the modified nucleotides. Therefore, algorithms, such as EpiNano, DRUMMER, Eligos2, JACUSA2, DiffErr, are able to identify m6A sites based on error rates of base calls (Parker et al., 2020; Price et al., 2020; Jenjaroenpun et al., 2021; Liu et al., 2021; Piechotta et al., 2022).
Epinano (Liu et al., 2021), a supervised learning algorithm, aims at using base call error features to train the support vector machine (SVM)-based classifier and predicting the m6A (Figure 9A). The training data were from two sets of in vitro-transcribed RNAs, which comprised all possible 5-mer sequences with m6A-modified or unmodified adenosines. Three features, base quality, deletion frequency and mismatch frequency from m6A-modified and unmodified data sets were used to train the SVM model (Figure 9A). The trained model could then be used for prediction of m6A at the positions of RRACH motifs (Liu et al., 2019; Smith et al., 2020; Liu et al., 2021).
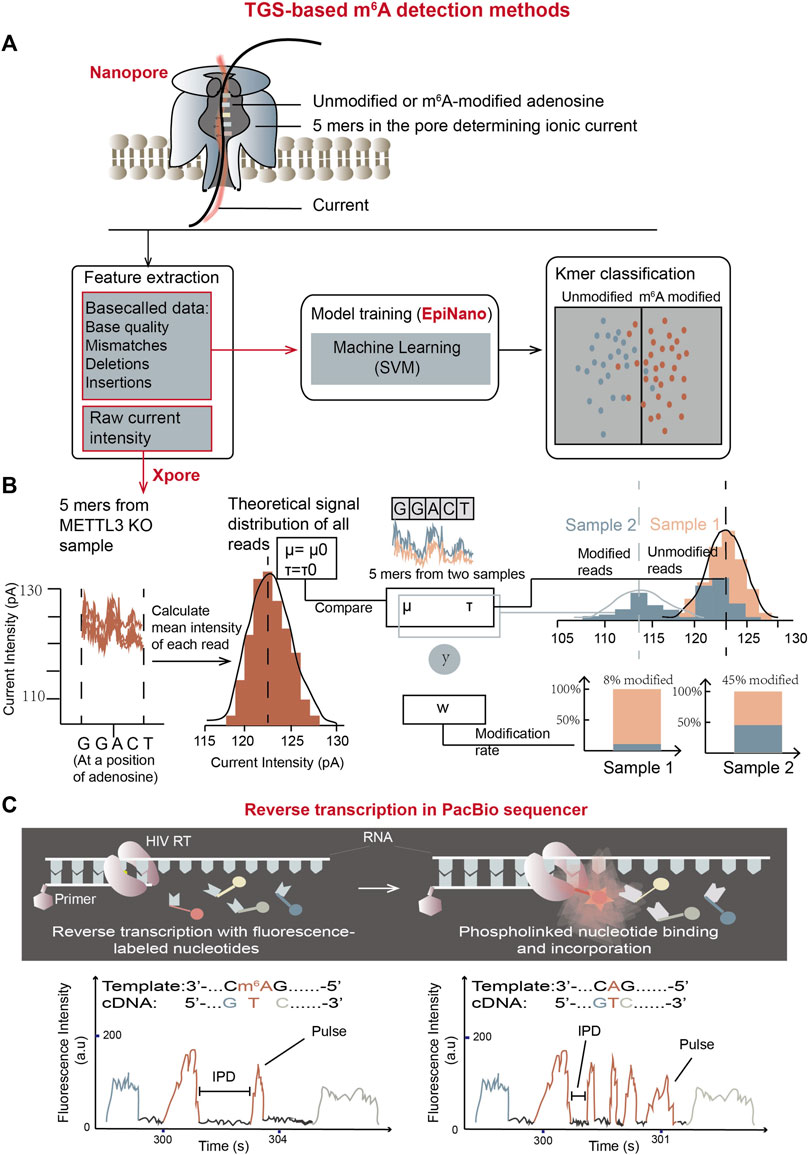
Figure 9. m6A detection methods using TGS platforms. (A) The schematic overview of m6A detection using Oxford direct RNA sequencing (DRS) combined with “base call error rate”-based EpiNano algorithm. The trained model is able to classify bases in an undetermined RNA sample into m6A-modified group and unmodified group. (B) The schematic workflow of m6A quantification using Oxford DRS combined with xPore algorithm which is based on raw current signals (Pratanwanich et al., 2021). In the graph, y represents input (5-mer reads), and w represents the weight of the modified reads at said location. In detail, 92% of reads in sample-1 (orange sample) belong to the theoretical Gaussian distribution, meaning the modification rate of sample-1 at the location is 8%; while the modification rate of sample-2 (blue sample) at the location is 45%. (C) Schematic representation of m6A detection using PacBio SMRT reverse transcription (Vilfan et al., 2013). Two movies showed below represent the trace of reverse transcription from a m6A-containing template (left) and an unmodified template (right). Compared with unmodified residues, the pulse frequency is reduced while IPD is elevated in m6A-modified residues, which can work as features to determine m6A modifications in unknown samples.
DRUMMER, Eligos2, JACUSA2, and DiffErr algorithms mainly rely on significantly different error profiles between two sets of RNAs with m6A or without m6A (Parker et al., 2020; Price et al., 2020; Jenjaroenpun et al., 2021; Piechotta et al., 2022). Two comparative sets of RNAs could come from either in vitro transcription or in vivo impairment of the relevant gene functions. The m6A locations are determined through multiple statistical computation of insertions, deletions and substitutions between two conditions in these algorithms.
4.1.2 Algorithms based on raw ionic current signals
Nanocompore, xPore, Tombo, m6Anet, MINES, nanom6A, Yanocomp, and DENA are algorithms developed to detect m6A by analyzing the raw current signals (Lorenz et al., 2020; Gao et al., 2021; Leger et al., 2021; Pratanwanich et al., 2021; Abebe et al., 2022; Qin et al., 2022). The raw signals are dissected into “events” and assigned to their corresponding nucleotides. Further ionic flow features such as current intensity and dwell time in the raw signals are exacted and employed to train a model or implement comparative analyses based on statistical tools in these algorithms.
In 2021, xPore (Pratanwanich et al., 2021), an algorithm to measure m6A quantitatively in multiple samples using raw current signal intensity as a feature was developed (Figure 9B). The assumption in xPore is that when modeling m6A modification states at a single genomic locus, raw current signal intensity should have two Gaussian distributions corresponding to unmodified and modified RNA species. These two distributions could be shared across different samples and allow individual reads from the genomic locus to fit both distributions with different degrees. With this assumption, xPore models current intensity from multiple samples by two Gaussian Mixture Model (GMM), which is a form of supervised learning (Pratanwanich et al., 2021). The algorithm requires prior information about the theoretical signal distribution of unmodified RNA species to guide model development. For each particular location of 5-mer nucleotides with an adenosine in the middle, intensity-level mean of each read is calculated first. Then, the distributions and standard deviations of the mean intensity from all reads covering the particular position are computed for different samples by GMM to separate mean intensity from all reads into two gaussian distributions corresponding to modified and unmodified adenosine-containing sites. m6A rates at each location for different samples could then be calculated by read numbers in the two distributions. xPore can accurately quantify RNA modifications at the positions with high modification rates (higher than 25%).
Nanocompore was developed in which both current intensity and dwell time features are used to cluster signals from m6A modified and unmodified RNA species with a univariate pairwise test or a bivariate classification method based on two-component GMM clustering (Leger et al., 2021). The following logistic regression test determines whether the distributions of two clusters are significantly different. Using these grouping methods, Nanocompore is able to conduct m6A calls by comparing an undetermined RNA sample with an unmodified control sample.
One great advantage of these nanopore DRS methods is their straight forward procedure. These methods don’t need complex experimental signal transformation processes or special treatment of mRNA molecules. Nanopore DRS raw data can maintain the original RNA modification information. Besides, they analyze entire mRNA molecules, which allows to observe m6A at specifically-spliced isoforms and correlate m6A status with other transcript features. However, these methods require extensive iterative sequencing signal measurement to calculate the error frequency in calling RNA sequences, which makes them inaccurate in detecting m6A sites with low modification rates. Proper control groups, improved algorithms, new nanopore proteins specially engineered for m6A detection, and large simulations are required to improve the accuracy and sensitivity of the currently-used methods.
4.2 The m6A detection using PacBio SMRT reverse transcription
PacBio platform employs sequencing by synthesis (SBS) using fluorescence-labelled dNTP, and invents a new method called zero mode waveguides (ZMWs) to detect fluorescent signals emitted only from the area of single molecular DNA synthesis. The ZMW provides a highly confined optical observation volume, enabling single-molecule-resolved biophysical studies in the existence of other fluorescent molecules (Eid et al., 2009).
PacBio Single molecule Real Time (SMRT) DNA sequencing has been optimized to RNA sequencing by utilizing an HIV reverse transcriptase (HIV RT) to determine both RNA sequences and modifications simultaneously in PacBio sequencer (Vilfan et al., 2013). Similar to SMRT DNA sequencing (Eid et al., 2009), RT activity is visualized by phospho-linked deoxyribonucleotides, in which the terminal phosphates carry fluorophores. The fluorescent label is released during nucleotide incorporation so the cDNA synthesis can be detected in real time (Figure 9C). Furthermore, at RNA m6A sites, the complementary nucleotide incorporation displays the different kinetics compared with unmodified counterparts. Fluorescent pulse frequency at m6A locations is reduced compared with their unmodified RNA control locations, indicating that the binding of phospho-linked nucleotide is affected by m6A on template RNA. In addition, the interpulse durations (IPDs) at m6A-modified positions are increased than those at unmodified positions, meaning that the binding rates of complementary TTP at m6A sites are decreased by approximately 5-fold. Based on this principle, comparing the distributions of IPDs between two RNA species can reveal the precise locations of m6A (Vilfan et al., 2013).
Although this method has not reached the transcriptomic level, it has however, opened up the possibility for genome-wide m6A detection using PacBio SMRT reverse transcription.
5 Discussion
This comprehensive review presented major sequencing-independent m6A detection methods as well as SGS-based and TGS-based transcriptome-wide m6A detection methods. Since these methods carry distinct features, proper methods should be chosen considering the criteria such as the required starting materials, sensitivity, stoichiometry, site resolution, bias in detection, convenience of reagent and software, procedure simplicity, result reproducibility, and cost (Supplementary Table S1).
The sequencing-independent biochemical methods can achieve two goals: m6A-ELISA and LC/MS could quantify the m6A level in total mRNA; SCARLET and SELECT could investigate m6A status at individual sites (Figure 2; Supplementary Table S1). Among them, m6A-ELISA, LC/MS, and SCARLET have been extensively used. For rough quantification of total m6A levels, m6A-ELISA is a good choice because it is a simple, cheap, and commercially-available method (Figure 2C). This method is very suitable for comparison of total m6A levels in different samples using ∼5 μg of total RNA. Comparing with m6A-ELISA, LC/MS can more accurately quantify total m6A levels in samples with great sensitivity to the m6A level change using ∼50 μg of total RNA. Also, LC/MS can discriminate different nucleosides with/without varying modifications, so it will be good to quantify the differentiation in nucleoside compositions of total mRNAs from multiple samples. If individual candidate m6A sites are expected to have important function, SCARLET can precisely validate the modification status of the sites and quantify m6A and unmodified adenosine levels at the sites (Figure 2A). Anyway, SCARLET needs to use ∼1 μg of mRNA (>20 μg of total RNA) and 32P-radioisotope. If only a small amount of RNA is available, a radioisotope-free, qPCR-based method SELECT can be employed to quantify individual candidate m6A sites using as low as 0.2 ng of mRNA (Figure 2B).
To meet the increasing requirements for epitranscriptomic study, many high-throughput sequencing-based m6A profiling methods have been developed. So far, there are three main categories for these methods: 1) SGS-based anti-m6A antibody-dependent methods; 2) SGS-based anti-m6A antibody-independent methods, which rely on enzymes, SAM analogs, or chemicals; 3) TGS and machine learning-based methods.
In the first category, MeRIP and CLIP-based miCLIP, meCLIP and m6ACE-seq, all rely on m6A antibody (Supplementary Table S1). MeRIP and miCLIP are the most widely used methods so far and have revealed transcriptomic m6A modification for the first time. Also, CLIP-based miCLIP, meCLIP and m6ACE-seq have achieved the single-nucleotide resolution in m6A-site detection. However, the requirement in a large amount of mRNA (10–20 μg of mRNA for CLIP-based methods and 400 μg of mRNA for MeRIP) and the massive dependence on anti-m6A antibody cause major limitations (McIntyre et al., 2020). Due to low specificity of antibody, these methods usually exhibit high noise. Detection accuracy in these methods can be improved after calibration m6A signals by a negative control—a modification-free, endogenous transcriptome-resembling, synthetic RNA library (Zhang Z. et al., 2021). This provides a way to evaluate and calibrate some noise in the antibody-dependent m6A detection methods. Nevertheless, some anti-m6A antibodies may react with m6Am dimethyl adenosine as well, which will make these methods hardly distinguish the two modifications (Schwartz et al., 2013; Schwartz et al., 2014a). More importantly, the antibody-based methods are not ideal for accurate m6A quantification, which is fundamental in addressing critical questions about m6A cellular function and its response to environmental stimuli (Meyer and Jaffrey, 2014; Schwartz, 2016; Grozhik and Jaffrey, 2018). Therefore, new methods have been emerged.
The methods in the second category are based on enzymes, SAM analogs, or chemicals. DART-seq and Mazter-seq/REF-seq can map m6A sites at single-base resolution, while others such as m6A-SAC-seq, eTAM-seq, and GLORI can achieve stoichiometric measurement at each m6A site in addition to single-base resolution. Specially, scDART-seq can achieve single-cell m6A profiling, which is necessary for highly-heterogeneous tissues and is critical for identification of cell-specific m6A patterns and roles. Furthermore, long-read DART-seq using PacBio platform can determine m6A sites transcript-isoform specifically. Considering simple procedure and low cost, the recently-developed GLORI and eTAM-seq have good potential, especially if commercial TadA8.20 enzyme is available in eTAM-seq (Supplementary Table S1). However, among these new methods, Mazter-seq/REF-seq can only detect m6A sites with an ACA motif, accounting for 16%–25% of total m6A sites. Also, DART-seq relies on the transfection efficiency of cells and accompanies an over-expression problem. In addition, m6A-SAC-seq exhibits a bias of m6A detection towards the GAC sites, and its quantification relies on a standard curve from spike-in RNA. In summary, these new methods give great opportunity to illustrate new biological phenomena in the field.
The methods in the third category are based on TGS and machine learning. This developing area provides new opportunity. Nanopore DRS-based methods are able to collect signals directly from original RNA molecules, retaining untransformed modification information (Supplementary Table S1). Further, the simple library construction makes TGS-based methods time saving and convenient to use. Also, they have achieved the single-nucleotide resolution, and Nonocompore even provides mRNA isoform-specific detection. However, they have limitations in high cost, low accuracy, and high requirements for RNA quality. For example, the quantitation results of xPore are not in accordance with other methods like REF-seq (Zhang et al., 2019), which may be raised from the variance of the same 5 mers’ current intensity. Besides, the machine learning algorithms used for model training may not be really suitable for m6A prediction. Thus, TGS-based methods solicit further improvement both in computational algorithms and experimental techniques such as RT enzyme, motor/nanopore protein and library construction.
In summary, current technology has facilitated significant advancement in m6A research, and it will be useful to combine the transcriptomic methods with the site-specific quantification methods like SCARLET or SELECT. Since most SGS-based methods, including the latest eTAM and GLORI, need in vitro fragmentation and antibody, enzymatic or chemical treatments before sequencing library construction, isoform-specific m6A information is lost. Therefore, it is necessary to put effort into developing mRNA isoform-specific m6A profiling methods and three-dimensional m6A pattern profiling methods. Anyway, the methods introduced here can meet varying requirements of m6A research, and will provide important insights for developing new strategies in different fields.
Author contributions
YY: Conceptualization, Data curation, Investigation, Visualization, Writing–original draft, Writing–review and editing. YL: Validation, Writing–review and editing. YW: Data curation, Validation, Writing–review and editing. XW: Validation, Writing–review and editing. CQ: Supervision, Validation, Writing–review and editing. WP: Supervision, Validation, Writing–review and editing. HJ: Conceptualization, Funding acquisition, Supervision, Validation, Writing–original draft, Writing–review and editing.
Funding
The author(s) declare that financial support was received for the research, authorship, and/or publication of this article. The work was supported by National Natural Science Foundation of China (31970622) and by the Fundamental Research Funds for the Central Universities.
Conflict of interest
The authors declare that the research was conducted in the absence of any commercial or financial relationships that could be construed as a potential conflict of interest.
Publisher’s note
All claims expressed in this article are solely those of the authors and do not necessarily represent those of their affiliated organizations, or those of the publisher, the editors and the reviewers. Any product that may be evaluated in this article, or claim that may be made by its manufacturer, is not guaranteed or endorsed by the publisher.
Supplementary material
The Supplementary Material for this article can be found online at: https://www.frontiersin.org/articles/10.3389/fcell.2024.1392159/full#supplementary-material
References
Abebe, J. S., Verstraten, R., and Depledge, D. P. (2022). Nanopore-based detection of viral RNA modifications. mBio 13, e0370221. doi:10.1128/mbio.03702-21
Agarwala, S. D., Blitzblau, H. G., Hochwagen, A., and Fink, G. R. (2012). RNA methylation by the MIS complex regulates a cell fate decision in yeast. PLoS Genet. 8, e1002732. doi:10.1371/journal.pgen.1002732
Barbieri, I., Tzelepis, K., Pandolfini, L., Shi, J., Millan-Zambrano, G., Robson, S. C., et al. (2017). Promoter-bound METTL3 maintains myeloid leukaemia by m(6)A-dependent translation control. Nature 552, 126–131. doi:10.1038/nature24678
Batista, P. J., Molinie, B., Wang, J., Qu, K., Zhang, J., Li, L., et al. (2014). m(6)A RNA modification controls cell fate transition in mammalian embryonic stem cells. Cell Stem Cell 15, 707–719. doi:10.1016/j.stem.2014.09.019
Bawankar, P., Lence, T., Paolantoni, C., Haussmann, I. U., Kazlauskiene, M., Jacob, D., et al. (2021). Hakai is required for stabilization of core components of the m(6)A mRNA methylation machinery. Nat. Commun. 12, 3778. doi:10.1038/s41467-021-23892-5
Brannan, K. W., Chaim, I. A., Marina, R. J., Yee, B. A., Kofman, E. R., Lorenz, D. A., et al. (2021). Robust single-cell discovery of RNA targets of RNA-binding proteins and ribosomes. Nat. Methods 18, 507–519. doi:10.1038/s41592-021-01128-0
Bringmann, P., and Luhrmann, R. (1987). Antibodies specific for N6-methyladenosine react with intact snRNPs U2 and U4/U6. FEBS Lett. 213, 309–315. doi:10.1016/0014-5793(87)81512-0
Chen, T., Hao, Y. J., Zhang, Y., Li, M. M., Wang, M., Han, W., et al. (2015). m(6)A RNA methylation is regulated by microRNAs and promotes reprogramming to pluripotency. Cell Stem Cell 16, 289–301. doi:10.1016/j.stem.2015.01.016
Desrosiers, R., Friderici, K., and Rottman, F. (1974). Identification of methylated nucleosides in messenger RNA from Novikoff hepatoma cells. Proc. Natl. Acad. Sci. U. S. A. 71, 3971–3975. doi:10.1073/pnas.71.10.3971
Dominissini, D., Moshitch-Moshkovitz, S., Schwartz, S., Salmon-Divon, M., Ungar, L., Osenberg, S., et al. (2012). Topology of the human and mouse m6A RNA methylomes revealed by m6A-seq. Nature 485, 201–206. doi:10.1038/nature11112
Eid, J., Fehr, A., Gray, J., Luong, K., Lyle, J., Otto, G., et al. (2009). Real-time DNA sequencing from single polymerase molecules. Science 323, 133–138. doi:10.1126/science.1162986
Ensinck, I., Sideri, T., Modic, M., Capitanchik, C., Vivori, C., Toolan-Kerr, P., et al. (2023). m6A-ELISA, a simple method for quantifying N6-methyladenosine from mRNA populations. RNA 29, 705–712. doi:10.1261/rna.079554.122
Flamand, M. N., and Meyer, K. D. (2022). m6A and YTHDF proteins contribute to the localization of select neuronal mRNAs. Nucleic Acids Res. 50, 4464–4483. doi:10.1093/nar/gkac251
Fu, Y., Dominissini, D., Rechavi, G., and He, C. (2014). Gene expression regulation mediated through reversible m⁶A RNA methylation. Nat. Rev. Genet. 15, 293–306. doi:10.1038/nrg3724
Fu, Y., and He, C. (2012). Nucleic acid modifications with epigenetic significance. Curr. Opin. Chem. Biol. 16, 516–524. doi:10.1016/j.cbpa.2012.10.002
Fu, Y., Jia, G., Pang, X., Wang, R. N., Wang, X., Li, C. J., et al. (2013). FTO-mediated formation of N6-hydroxymethyladenosine and N6-formyladenosine in mammalian RNA. Nat. Commun. 4, 1798. doi:10.1038/ncomms2822
Gao, Y., Liu, X., Wu, B., Wang, H., Xi, F., Kohnen, M. V., et al. (2021). Quantitative profiling of N(6)-methyladenosine at single-base resolution in stem-differentiating xylem of Populus trichocarpa using Nanopore direct RNA sequencing. Genome Biol. 22, 22. doi:10.1186/s13059-020-02241-7
Garcia-Campos, M. A., Edelheit, S., Toth, U., Safra, M., Shachar, R., Viukov, S., et al. (2019). Deciphering the "m(6)A code" via antibody-independent quantitative profiling. Cell 178, 731–747. doi:10.1016/j.cell.2019.06.013
Ge, R., Ye, C., Peng, Y., Dai, Q., Zhao, Y., Liu, S., et al. (2023). m(6)A-SAC-seq for quantitative whole transcriptome m(6)A profiling. Nat. Protoc. 18, 626–657. doi:10.1038/s41596-022-00765-9
Geula, S., Moshitch-Moshkovitz, S., Dominissini, D., Mansour, A. A., Kol, N., Salmon-Divon, M., et al. (2015). Stem cells. m6A mRNA methylation facilitates resolution of naive pluripotency toward differentiation. Science 347, 1002–1006. doi:10.1126/science.1261417
Goodwin, S., Mcpherson, J. D., and Mccombie, W. R. (2016). Coming of age: ten years of next-generation sequencing technologies. Nat. Rev. Genet. 17, 333–351. doi:10.1038/nrg.2016.49
Grozhik, A. V., and Jaffrey, S. R. (2018). Distinguishing RNA modifications from noise in epitranscriptome maps. Nat. Chem. Biol. 14, 215–225. doi:10.1038/nchembio.2546
Gulati, P., Avezov, E., Ma, M., Antrobus, R., Lehner, P., O'rahilly, S., et al. (2014). Fat mass and obesity-related (FTO) shuttles between the nucleus and cytoplasm. Biosci. Rep. 34, e00144. doi:10.1042/BSR20140111
Haussmann, I. U., Bodi, Z., Sanchez-Moran, E., Mongan, N. P., Archer, N., Fray, R. G., et al. (2016). m(6)A potentiates Sxl alternative pre-mRNA splicing for robust Drosophila sex determination. Nature 540, 301–304. doi:10.1038/nature20577
Hong, A., Kim, D., Kim, V. N., and Chang, H. (2022). Analyzing viral epitranscriptomes using nanopore direct RNA sequencing. J. Microbiol. 60, 867–876. doi:10.1007/s12275-022-2324-4
Horiuchi, K., Kawamura, T., Iwanari, H., Ohashi, R., Naito, M., Kodama, T., et al. (2013). Identification of Wilms' tumor 1-associating protein complex and its role in alternative splicing and the cell cycle. J. Biol. Chem. 288, 33292–33302. doi:10.1074/jbc.M113.500397
Hu, L., Liu, S., Peng, Y., Ge, R., Su, R., Senevirathne, C., et al. (2022). m(6)A RNA modifications are measured at single-base resolution across the mammalian transcriptome. Nat. Biotechnol. 40, 1210–1219. doi:10.1038/s41587-022-01243-z
Imanishi, M., Tsuji, S., Suda, A., and Futaki, S. (2017). Detection of N(6)-methyladenosine based on the methyl-sensitivity of MazF RNA endonuclease. Chem. Commun. (Camb) 53, 12930–12933. doi:10.1039/c7cc07699a
Jenjaroenpun, P., Wongsurawat, T., Wadley, T. D., Wassenaar, T. M., Liu, J., Dai, Q., et al. (2021). Decoding the epitranscriptional landscape from native RNA sequences. Nucleic Acids Res. 49, e7. doi:10.1093/nar/gkaa620
Jia, G., Fu, Y., Zhao, X., Dai, Q., Zheng, G., Yang, Y., et al. (2011). N6-methyladenosine in nuclear RNA is a major substrate of the obesity-associated FTO. Nat. Chem. Biol. 7, 885–887. doi:10.1038/nchembio.687
Jin, H., Xu, W., Rahman, R., Na, D., Fieldsend, A., Song, W., et al. (2020). TRIBE editing reveals specific mRNA targets of eIF4E-BP in Drosophila and in mammals. Sci. Adv. 6, eabb8771. doi:10.1126/sciadv.abb8771
Kan, L., Grozhik, A. V., Vedanayagam, J., Patil, D. P., Pang, N., Lim, K. S., et al. (2017). The m(6)A pathway facilitates sex determination in Drosophila. Nat. Commun. 8, 15737. doi:10.1038/ncomms15737
Ke, S., Pandya-Jones, A., Saito, Y., Fak, J. J., Vagbo, C. B., Geula, S., et al. (2017). m(6)A mRNA modifications are deposited in nascent pre-mRNA and are not required for splicing but do specify cytoplasmic turnover. Genes Dev. 31, 990–1006. doi:10.1101/gad.301036.117
Keller, M. W., Rambo-Martin, B. L., Wilson, M. M., Ridenour, C. A., Shepard, S. S., Stark, T. J., et al. (2018). Direct RNA sequencing of the coding complete influenza A virus genome. Sci. Rep. 8, 14408. doi:10.1038/s41598-018-32615-8
Kim, D., Lee, J. Y., Yang, J. S., Kim, J. W., Kim, V. N., and Chang, H. (2020). The architecture of SARS-CoV-2 transcriptome. Cell 181, 914–921. doi:10.1016/j.cell.2020.04.011
Knuckles, P., Lence, T., Haussmann, I. U., Jacob, D., Kreim, N., Carl, S. H., et al. (2018). Zc3h13/Flacc is required for adenosine methylation by bridging the mRNA-binding factor Rbm15/Spenito to the m(6)A machinery component Wtap/Fl(2)d. Genes Dev. 32, 415–429. doi:10.1101/gad.309146.117
Koh, C. W. Q., Goh, Y. T., and Goh, W. S. S. (2019). Atlas of quantitative single-base-resolution N(6)-methyl-adenine methylomes. Nat. Commun. 10, 5636. doi:10.1038/s41467-019-13561-z
Komor, A. C., Kim, Y. B., Packer, M. S., Zuris, J. A., and Liu, D. R. (2016). Programmable editing of a target base in genomic DNA without double-stranded DNA cleavage. Nature 533, 420–424. doi:10.1038/nature17946
Konig, J., Zarnack, K., Rot, G., Curk, T., Kayikci, M., Zupan, B., et al. (2010). iCLIP reveals the function of hnRNP particles in splicing at individual nucleotide resolution. Nat. Struct. Mol. Biol. 17, 909–915. doi:10.1038/nsmb.1838
Lapointe, C. P., Wilinski, D., Saunders, H. A., and Wickens, M. (2015). Protein-RNA networks revealed through covalent RNA marks. Nat. Methods 12, 1163–1170. doi:10.1038/nmeth.3651
Lasman, L., Krupalnik, V., Viukov, S., Mor, N., Aguilera-Castrejon, A., Schneir, D., et al. (2020). Context-dependent functional compensation between Ythdf m(6)A reader proteins. Genes Dev. 34, 1373–1391. doi:10.1101/gad.340695.120
Leger, A., Amaral, P. P., Pandolfini, L., Capitanchik, C., Capraro, F., Miano, V., et al. (2021). RNA modifications detection by comparative Nanopore direct RNA sequencing. Nat. Commun. 12, 7198. doi:10.1038/s41467-021-27393-3
Lence, T., Akhtar, J., Bayer, M., Schmid, K., Spindler, L., Ho, C. H., et al. (2016). m(6)A modulates neuronal functions and sex determination in Drosophila. Nature 540, 242–247. doi:10.1038/nature20568
Li, L., Krasnykov, K., Homolka, D., Gos, P., Mendel, M., Fish, R. J., et al. (2022). The XRN1-regulated RNA helicase activity of YTHDC2 ensures mouse fertility independently of m(6)A recognition. Mol. Cell 82, 1678–1690.e12. doi:10.1016/j.molcel.2022.02.034
Li, Y., Bedi, R. K., Moroz-Omori, E. V., and Caflisch, A. (2020). Structural and dynamic insights into redundant function of YTHDF proteins. J. Chem. Inf. Model 60, 5932–5935. doi:10.1021/acs.jcim.0c01029
Lichinchi, G., Gao, S., Saletore, Y., Gonzalez, G. M., Bansal, V., Wang, Y., et al. (2016). Dynamics of the human and viral m(6)A RNA methylomes during HIV-1 infection of T cells. Nat. Microbiol. 1, 16011. doi:10.1038/nmicrobiol.2016.11
Linder, B., Grozhik, A. V., Olarerin-George, A. O., Meydan, C., Mason, C. E., and Jaffrey, S. R. (2015). Single-nucleotide-resolution mapping of m6A and m6Am throughout the transcriptome. Nat. Methods 12, 767–772. doi:10.1038/nmeth.3453
Liu, C., Sun, H., Yi, Y., Shen, W., Li, K., Xiao, Y., et al. (2023). Absolute quantification of single-base m(6)A methylation in the mammalian transcriptome using GLORI. Nat. Biotechnol. 41, 355–366. doi:10.1038/s41587-022-01487-9
Liu, H., Begik, O., Lucas, M. C., Ramirez, J. M., Mason, C. E., Wiener, D., et al. (2019). Accurate detection of m(6)A RNA modifications in native RNA sequences. Nat. Commun. 10, 4079. doi:10.1038/s41467-019-11713-9
Liu, H., Begik, O., and Novoa, E. M. (2021). EpiNano: detection of m(6)A RNA modifications using Oxford nanopore direct RNA sequencing. Methods Mol. Biol. 2298, 31–52. doi:10.1007/978-1-0716-1374-0_3
Liu, J., Yue, Y., Han, D., Wang, X., Fu, Y., Zhang, L., et al. (2014). A METTL3-METTL14 complex mediates mammalian nuclear RNA N6-adenosine methylation. Nat. Chem. Biol. 10, 93–95. doi:10.1038/nchembio.1432
Liu, N., Parisien, M., Dai, Q., Zheng, G., He, C., and Pan, T. (2013). Probing N6-methyladenosine RNA modification status at single nucleotide resolution in mRNA and long noncoding RNA. RNA 19, 1848–1856. doi:10.1261/rna.041178.113
Loose, M., Malla, S., and Stout, M. (2016). Real-time selective sequencing using nanopore technology. Nat. Methods 13, 751–754. doi:10.1038/nmeth.3930
Lorenz, D. A., Sathe, S., Einstein, J. M., and Yeo, G. W. (2020). Direct RNA sequencing enables m(6)A detection in endogenous transcript isoforms at base-specific resolution. RNA 26, 19–28. doi:10.1261/rna.072785.119
Luo, S., and Tong, L. (2014). Molecular basis for the recognition of methylated adenines in RNA by the eukaryotic YTH domain. Proc. Natl. Acad. Sci. U. S. A. 111, 13834–13839. doi:10.1073/pnas.1412742111
Ma, H. H., Wang, X. Y., Cai, J. B., Dai, Q., Natchiar, S. K., Lv, R. T., et al. (2019). N6-Methyladenosine methyltransferase ZCCHC4 mediates ribosomal RNA methylation. Nat. Chem. Biol. 15, 549. doi:10.1038/s41589-019-0233-6
Mathur, L., Jung, S., Jang, C., and Lee, G. (2021). Quantitative analysis of m(6)A RNA modification by LC-MS. Star. Protoc. 2, 100724. doi:10.1016/j.xpro.2021.100724
Mauer, J., Luo, X., Blanjoie, A., Jiao, X., Grozhik, A. V., Patil, D. P., et al. (2017). Reversible methylation of m(6)A(m) in the 5' cap controls mRNA stability. Nature 541, 371–375. doi:10.1038/nature21022
Mauer, J., Sindelar, M., Despic, V., Guez, T., Hawley, B. R., Vasseur, J. J., et al. (2019). FTO controls reversible m(6)Am RNA methylation during snRNA biogenesis. Nat. Chem. Biol. 15, 340–347. doi:10.1038/s41589-019-0231-8
Mcintyre, A. B. R., Gokhale, N. S., Cerchietti, L., Jaffrey, S. R., Horner, S. M., and Mason, C. E. (2020). Limits in the detection of m(6)A changes using MeRIP/m(6)A-seq. Sci. Rep. 10, 6590. doi:10.1038/s41598-020-63355-3
Mcmahon, A. C., Rahman, R., Jin, H., Shen, J. L., Fieldsend, A., Luo, W., et al. (2016). TRIBE: hijacking an RNA-editing enzyme to identify cell-specific targets of RNA-binding proteins. Cell 165, 742–753. doi:10.1016/j.cell.2016.03.007
Meyer, K. D. (2019). DART-seq: an antibody-free method for global m(6)A detection. Nat. Methods 16, 1275–1280. doi:10.1038/s41592-019-0570-0
Meyer, K. D., and Jaffrey, S. R. (2014). The dynamic epitranscriptome: N6-methyladenosine and gene expression control. Nat. Rev. Mol. Cell Biol. 15, 313–326. doi:10.1038/nrm3785
Meyer, K. D., Saletore, Y., Zumbo, P., Elemento, O., Mason, C. E., and Jaffrey, S. R. (2012). Comprehensive analysis of mRNA methylation reveals enrichment in 3' UTRs and near stop codons. Cell 149, 1635–1646. doi:10.1016/j.cell.2012.05.003
Murakami, S., and Jaffrey, S. R. (2022). Hidden codes in mRNA: control of gene expression by m(6)A. Mol. Cell 82, 2236–2251. doi:10.1016/j.molcel.2022.05.029
Narayan, P., and Rottman, F. M. (1988). An in vitro system for accurate methylation of internal adenosine residues in messenger RNA. Science 242, 1159–1162. doi:10.1126/science.3187541
Pandey, R. R., and Pillai, R. S. (2019). Counting the cuts: MAZTER-seq quantifies m(6)A levels using a methylation-sensitive ribonuclease. Cell 178, 515–517. doi:10.1016/j.cell.2019.07.006
Parker, M. T., Knop, K., Sherwood, A. V., Schurch, N. J., Mackinnon, K., Gould, P. D., et al. (2020). Nanopore direct RNA sequencing maps the complexity of Arabidopsis mRNA processing and m(6)A modification. Elife 9, e49658. doi:10.7554/eLife.49658
Patil, D. P., Chen, C. K., Pickering, B. F., Chow, A., Jackson, C., Guttman, M., et al. (2016). m(6)A RNA methylation promotes XIST-mediated transcriptional repression. Nature 537, 369–373. doi:10.1038/nature19342
Patil, D. P., Pickering, B. F., and Jaffrey, S. R. (2018). Reading m(6)A in the transcriptome: m(6)a-binding proteins. Trends Cell Biol. 28, 113–127. doi:10.1016/j.tcb.2017.10.001
Pendleton, K. E., Chen, B., Liu, K., Hunter, O. V., Xie, Y., Tu, B. P., et al. (2017). The U6 snRNA m(6)A methyltransferase METTL16 regulates SAM synthetase intron retention. Cell 169, 824–835. doi:10.1016/j.cell.2017.05.003
Perry, R. P., Kelley, D. E., Friderici, K., and Rottman, F. (1975). The methylated constituents of L cell messenger RNA: evidence for an unusual cluster at the 5' terminus. Cell 4, 387–394. doi:10.1016/0092-8674(75)90159-2
Piao, W. L., Li, C., Sun, P. K., Yang, M. M., Ding, Y. S., Song, W., et al. (2023). Identification of RNA-binding protein targets with HyperTRIBE in Saccharomyces cerevisiae. Int. J. Mol. Sci. 24, 9033. doi:10.3390/ijms24109033
Piechotta, M., Naarmann-De Vries, I. S., Wang, Q., Altmuller, J., and Dieterich, C. (2022). RNA modification mapping with JACUSA2. Genome Biol. 23, 115. doi:10.1186/s13059-022-02676-0
Ping, X. L., Sun, B. F., Wang, L., Xiao, W., Yang, X., Wang, W. J., et al. (2014). Mammalian WTAP is a regulatory subunit of the RNA N6-methyladenosine methyltransferase. Cell Res. 24, 177–189. doi:10.1038/cr.2014.3
Pratanwanich, P. N., Yao, F., Chen, Y., Koh, C. W. Q., Wan, Y. K., Hendra, C., et al. (2021). Identification of differential RNA modifications from nanopore direct RNA sequencing with xPore. Nat. Biotechnol. 39, 1394–1402. doi:10.1038/s41587-021-00949-w
Price, A. M., Hayer, K. E., Mcintyre, A. B. R., Gokhale, N. S., Abebe, J. S., Della Fera, A. N., et al. (2020). Direct RNA sequencing reveals m(6)A modifications on adenovirus RNA are necessary for efficient splicing. Nat. Commun. 11, 6016. doi:10.1038/s41467-020-19787-6
Qin, H., Ou, L., Gao, J., Chen, L., Wang, J. W., Hao, P., et al. (2022). DENA: training an authentic neural network model using Nanopore sequencing data of Arabidopsis transcripts for detection and quantification of N(6)-methyladenosine on RNA. Genome Biol. 23, 25. doi:10.1186/s13059-021-02598-3
Raiber, E. A., Beraldi, D., Martinez Cuesta, S., Mcinroy, G. R., Kingsbury, Z., Becq, J., et al. (2017). Base resolution maps reveal the importance of 5-hydroxymethylcytosine in a human glioblastoma. NPJ Genom Med. 2, 6. doi:10.1038/s41525-017-0007-6
Ries, R. J., Zaccara, S., Klein, P., Olarerin-George, A., Namkoong, S., Pickering, B. F., et al. (2019). m(6)A enhances the phase separation potential of mRNA. Nature 571, 424–428. doi:10.1038/s41586-019-1374-1
Roberts, J. T., Porman, A. M., and Johnson, A. M. (2021). Identification of m(6)A residues at single-nucleotide resolution using eCLIP and an accessible custom analysis pipeline. RNA 27, 527–541. doi:10.1261/rna.078543.120
Roundtree, I. A., Evans, M. E., Pan, T., and He, C. (2017a). Dynamic RNA modifications in gene expression regulation. Cell 169, 1187–1200. doi:10.1016/j.cell.2017.05.045
Roundtree, I. A., Luo, G. Z., Zhang, Z., Wang, X., Zhou, T., Cui, Y., et al. (2017b). YTHDC1 mediates nuclear export of N(6)-methyladenosine methylated mRNAs. Elife 6, e31311. doi:10.7554/eLife.31311
Ruzicka, K., Zhang, M., Campilho, A., Bodi, Z., Kashif, M., Saleh, M., et al. (2017). Identification of factors required for m(6) A mRNA methylation in Arabidopsis reveals a role for the conserved E3 ubiquitin ligase HAKAI. New Phytol. 215, 157–172. doi:10.1111/nph.14586
Saito, Y., Hawley, B. R., Puno, M. R., Sarathy, S. N., Lima, C. D., Jaffrey, S. R., et al. (2022). YTHDC2 control of gametogenesis requires helicase activity but not m(6)A binding. Genes Dev. 36, 180–194. doi:10.1101/gad.349190.121
Saletore, Y., Meyer, K., Korlach, J., Vilfan, I. D., Jaffrey, S., and Mason, C. E. (2012). The birth of the Epitranscriptome: deciphering the function of RNA modifications. Genome Biol. 13, 175. doi:10.1186/gb-2012-13-10-175
Satterwhite, E. R., and Mansfield, K. D. (2022). RNA methyltransferase METTL16: targets and function. Wiley Interdiscip. Reviews-Rna 13, e1681. doi:10.1002/wrna.1681
Schadt, E. E., Turner, S., and Kasarskis, A. (2010). A window into third-generation sequencing. Hum. Mol. Genet. 19, R227–R240. doi:10.1093/hmg/ddq416
Schwartz, S., Agarwala, S. D., Mumbach, M. R., Jovanovic, M., Mertins, P., Shishkin, A., et al. (2013). High-resolution mapping reveals a conserved, widespread, dynamic mRNA methylation program in yeast meiosis. Cell 155, 1409–1421. doi:10.1016/j.cell.2013.10.047
Schwartz, S., Bernstein, D. A., Mumbach, M. R., Jovanovic, M., Herbst, R. H., Leon-Ricardo, B. X., et al. (2014a). Transcriptome-wide mapping reveals widespread dynamic-regulated pseudouridylation of ncRNA and mRNA. Cell 159, 148–162. doi:10.1016/j.cell.2014.08.028
Schwartz, S., Mumbach, M. R., Jovanovic, M., Wang, T., Maciag, K., Bushkin, G. G., et al. (2014b). Perturbation of m6A writers reveals two distinct classes of mRNA methylation at internal and 5' sites. Cell Rep. 8, 284–296. doi:10.1016/j.celrep.2014.05.048
Shi, H., Wang, X., Lu, Z., Zhao, B. S., Ma, H., Hsu, P. J., et al. (2017). YTHDF3 facilitates translation and decay of N(6)-methyladenosine-modified RNA. Cell Res. 27, 315–328. doi:10.1038/cr.2017.15
Shi, H., Wei, J., and He, C. (2019). Where, when, and how: context-dependent functions of RNA methylation writers, readers, and erasers. Mol. Cell 74, 640–650. doi:10.1016/j.molcel.2019.04.025
Shu, X., Cao, J., Cheng, M., Xiang, S., Gao, M., Li, T., et al. (2020). A metabolic labeling method detects m(6)A transcriptome-wide at single base resolution. Nat. Chem. Biol. 16, 887–895. doi:10.1038/s41589-020-0526-9
Sledz, P., and Jinek, M. (2016). Structural insights into the molecular mechanism of the m(6)A writer complex. Elife 5, e18434. doi:10.7554/eLife.18434
Smith, M. A., Ersavas, T., Ferguson, J. M., Liu, H., Lucas, M. C., Begik, O., et al. (2020). Molecular barcoding of native RNAs using nanopore sequencing and deep learning. Genome Res. 30, 1345–1353. doi:10.1101/gr.260836.120
Soneson, C., Yao, Y., Bratus-Neuenschwander, A., Patrignani, A., Robinson, M. D., and Hussain, S. (2019). A comprehensive examination of Nanopore native RNA sequencing for characterization of complex transcriptomes. Nat. Commun. 10, 3359. doi:10.1038/s41467-019-11272-z
Tegowski, M., Flamand, M. N., and Meyer, Kd. (2022). scDART-seq reveals distinct m6A signatures and mRNA methylation heterogeneity in single cells. Mol. Cell 82 (4), 868–878.e10. doi:10.1016/j.molcel.2021.12.038
Theler, D., Dominguez, C., Blatter, M., Boudet, J., and Allain, F. H. (2014). Solution structure of the YTH domain in complex with N6-methyladenosine RNA: a reader of methylated RNA. Nucleic Acids Res. 42, 13911–13919. doi:10.1093/nar/gku1116
Thuring, K., Schmid, K., Keller, P., and Helm, M. (2017). LC-MS analysis of methylated RNA. Methods Mol. Biol. 1562, 3–18. doi:10.1007/978-1-4939-6807-7_1
Van Dijk, E. L., Jaszczyszyn, Y., Naquin, D., and Thermes, C. (2018). The third revolution in sequencing technology. Trends Genet. 34, 666–681. doi:10.1016/j.tig.2018.05.008
Vilfan, I. D., Tsai, Y. C., Clark, T. A., Wegener, J., Dai, Q., Yi, C., et al. (2013). Analysis of RNA base modification and structural rearrangement by single-molecule real-time detection of reverse transcription. J. Nanobiotechnology 11, 8. doi:10.1186/1477-3155-11-8
Wang, C., Zhu, Y., Bao, H., Jiang, Y., Xu, C., Wu, J., et al. (2016a). A novel RNA-binding mode of the YTH domain reveals the mechanism for recognition of determinant of selective removal by Mmi1. Nucleic Acids Res. 44, 969–982. doi:10.1093/nar/gkv1382
Wang, J., Wang, P., Han, G., Zhang, T., Chang, J., Yin, R., et al. (2020a). Leukemogenic chromatin alterations promote AML leukemia stem cells via a KDM4C-ALKBH5-AXL signaling Axis. Cell Stem Cell 27 (1), 81–97. doi:10.1016/j.stem.2020.04.001
Wang, P., Doxtader, K. A., and Nam, Y. (2016b). Structural basis for cooperative function of Mettl3 and Mettl14 methyltransferases. Mol. Cell 63, 306–317. doi:10.1016/j.molcel.2016.05.041
Wang, X., Lu, Z., Gomez, A., Hon, G. C., Yue, Y., Han, D., et al. (2014a). N6-methyladenosine-dependent regulation of messenger RNA stability. Nature 505, 117–120. doi:10.1038/nature12730
Wang, X., Zhao, B. S., Roundtree, I. A., Lu, Z., Han, D., Ma, H., et al. (2015). N(6)-methyladenosine modulates messenger RNA translation efficiency. Cell 161, 1388–1399. doi:10.1016/j.cell.2015.05.014
Wang, Y., Li, Y., Toth, J. I., Petroski, M. D., Zhang, Z., and Zhao, J. C. (2014b). N6-methyladenosine modification destabilizes developmental regulators in embryonic stem cells. Nat. Cell Biol. 16, 191–198. doi:10.1038/ncb2902
Wang, Y., Li, Y., Yue, M., Wang, J., Kumar, S., Wechsler-Reya, R. J., et al. (2018). N(6)-methyladenosine RNA modification regulates embryonic neural stem cell self-renewal through histone modifications. Nat. Neurosci. 21, 195–206. doi:10.1038/s41593-017-0057-1
Wang, Y., Xiao, Y., Dong, S., Yu, Q., and Jia, G. (2020b). Antibody-free enzyme-assisted chemical approach for detection of N(6)-methyladenosine. Nat. Chem. Biol. 16, 896–903. doi:10.1038/s41589-020-0525-x
Wang, Y., Zhang, L., Ren, H., Ma, L., Guo, J., Mao, D., et al. (2021). Role of Hakai in m(6)A modification pathway in Drosophila. Nat. Commun. 12, 2159. doi:10.1038/s41467-021-22424-5
Weng, H., Huang, H., Wu, H., Qin, X., Zhao, B. S., Dong, L., et al. (2018). METTL14 inhibits hematopoietic stem/progenitor differentiation and promotes leukemogenesis via mRNA m(6)A modification. Cell Stem Cell 22, 191–205. doi:10.1016/j.stem.2017.11.016
Wick, R. R., Judd, L. M., and Holt, K. E. (2019). Performance of neural network basecalling tools for Oxford Nanopore sequencing. Genome Biol. 20, 129. doi:10.1186/s13059-019-1727-y
Winkler, R., Gillis, E., Lasman, L., Safra, M., Geula, S., Soyris, C., et al. (2019). m(6)A modification controls the innate immune response to infection by targeting type I interferons. Nat. Immunol. 20, 173–182. doi:10.1038/s41590-018-0275-z
Wu, F., Cheng, W., Zhao, F., Tang, M., Diao, Y., and Xu, R. (2019). Association of N6-methyladenosine with viruses and related diseases. Virol. J. 16, 133. doi:10.1186/s12985-019-1236-3
Xiang, Y., Laurent, B., Hsu, C. H., Nachtergaele, S., Lu, Z., Sheng, W., et al. (2017). RNA m(6)A methylation regulates the ultraviolet-induced DNA damage response. Nature 543, 573–576. doi:10.1038/nature21671
Xiao, W., Adhikari, S., Dahal, U., Chen, Y. S., Hao, Y. J., Sun, B. F., et al. (2016). Nuclear m(6)A reader YTHDC1 regulates mRNA splicing. Mol. Cell 61, 507–519. doi:10.1016/j.molcel.2016.01.012
Xiao, Y., Wang, Y., Tang, Q., Wei, L., Zhang, X., and Jia, G. (2018). An elongation- and ligation-based qPCR amplification method for the radiolabeling-free detection of locus-specific N(6) -methyladenosine modification. Angew. Chem. Int. Ed. Engl. 57, 15995–16000. doi:10.1002/anie.201807942
Xiao, Y. L., Liu, S., Ge, R., Wu, Y., He, C., Chen, M., et al. (2023). Transcriptome-wide profiling and quantification of N(6)-methyladenosine by enzyme-assisted adenosine deamination. Nat. Biotechnol. 41, 993–1003. doi:10.1038/s41587-022-01587-6
Yankova, E., Albertella, M., Rak, J., De Braekeleer, E., Tsagkogeorga, G., Pilka, ES, et al. (2021). Small-molecule inhibition of METTL3 as a strategy against myeloid leukaemia. Nature 593 (7860), 597–601. doi:10.1038/s41586-021-03536-w
Zaccara, S., and Jaffrey, S. R. (2020). A unified model for the function of YTHDF proteins in regulating m(6)a-modified mRNA. Cell 181, 1582–1595. doi:10.1016/j.cell.2020.05.012
Zaccara, S., Ries, R. J., and Jaffrey, S. R. (2019). Reading, writing and erasing mRNA methylation. Nat. Rev. Mol. Cell Biol. 20, 608–624. doi:10.1038/s41580-019-0168-5
Zhang, C., Samanta, D., Lu, H., Bullen, J. W., Zhang, H., Chen, I., et al. (2016a). Hypoxia induces the breast cancer stem cell phenotype by HIF-dependent and ALKBH5-mediated m⁶A-demethylation of NANOG mRNA. Proc. Natl. Acad. Sci. U. S. A. 113, E2047–E2056. doi:10.1073/pnas.1602883113
Zhang, C., Zhi, W. I., Lu, H., Samanta, D., Chen, I., Gabrielson, E., et al. (2016b). Hypoxia-inducible factors regulate pluripotency factor expression by ZNF217- and ALKBH5-mediated modulation of RNA methylation in breast cancer cells. Oncotarget 7, 64527–64542. doi:10.18632/oncotarget.11743
Zhang, L., Cheng, Y., Xue, Z., Li, J., Wu, N., Yan, J., et al. (2022). Sevoflurane impairs m6A-mediated mRNA translation and leads to fine motor and cognitive deficits. Cell Biol. Toxicol. 38, 347–369. doi:10.1007/s10565-021-09601-4
Zhang, R., Wang, P., Ma, X., Wu, Y., Luo, C., Qiu, L., et al. (2021a). Nanopore-based direct RNA-sequencing reveals a high-resolution transcriptional landscape of porcine reproductive and respiratory syndrome virus. Viruses 13, 2531. doi:10.3390/v13122531
Zhang, S., Zhao, B. S., Zhou, A., Lin, K., Zheng, S., Lu, Z., et al. (2017). m(6)A demethylase ALKBH5 maintains tumorigenicity of glioblastoma stem-like cells by sustaining FOXM1 expression and cell proliferation program. Cancer Cell 31, 591–606. doi:10.1016/j.ccell.2017.02.013
Zhang, Z., Chen, L. Q., Zhao, Y. L., Yang, C. G., Roundtree, I. A., Zhang, Z., et al. (2019). Single-base mapping of m(6)A by an antibody-independent method. Sci. Adv. 5, eaax0250. doi:10.1126/sciadv.aax0250
Zhang, Z., Chen, T., Chen, H. X., Xie, Y. Y., Chen, L. Q., Zhao, Y. L., et al. (2021b). Systematic calibration of epitranscriptomic maps using a synthetic modification-free RNA library. Nat. Methods 18, 1213–1222. doi:10.1038/s41592-021-01280-7
Zheng, G., Dahl, J. A., Niu, Y., Fedorcsak, P., Huang, C. M., Li, C. J., et al. (2013). ALKBH5 is a mammalian RNA demethylase that impacts RNA metabolism and mouse fertility. Mol. Cell 49, 18–29. doi:10.1016/j.molcel.2012.10.015
Keywords: N6-methyladenosine, m6A detection, epitranscriptomics, RNA modifications, gene regulation
Citation: Yang Y, Lu Y, Wang Y, Wen X, Qi C, Piao W and Jin H (2024) Current progress in strategies to profile transcriptomic m6A modifications. Front. Cell Dev. Biol. 12:1392159. doi: 10.3389/fcell.2024.1392159
Received: 27 February 2024; Accepted: 18 June 2024;
Published: 11 July 2024.
Edited by:
Giovanni Nassa, University of Salerno, ItalyReviewed by:
Xiguang Xu, Virginia Tech, United StatesPayel Sen, National Institute on Aging (NIH), United States
Copyright © 2024 Yang, Lu, Wang, Wen, Qi, Piao and Jin. This is an open-access article distributed under the terms of the Creative Commons Attribution License (CC BY). The use, distribution or reproduction in other forums is permitted, provided the original author(s) and the copyright owner(s) are credited and that the original publication in this journal is cited, in accordance with accepted academic practice. No use, distribution or reproduction is permitted which does not comply with these terms.
*Correspondence: Hua Jin, huajin@bit.edu.cn; Weilan Piao, weilanpiao@bit.edu.cn; Changhai Qi, pathology721@126.com
†Leading contact