- 1Division of Molecular and Cellular Functions, School of Biological Sciences, Faculty of Biology, Medicine and Health, The University of Manchester, Manchester, United Kingdom
- 2Division of Evolution, School of Biological Sciences, Faculty of Biology, Medicine and Health, The University of Manchester, Manchester, United Kingdom
- 3Section of Protein Science and Biotherapeutics, Department of Bioengineering and Biomedicine, Danish Technical University, Lyngby, Denmark
Functional selectivity refers to the activation of differential signalling and cellular outputs downstream of the same membrane-bound receptor when activated by two or more different ligands. Functional selectivity has been described and extensively studied for G-protein Coupled Receptors (GPCRs), leading to specific therapeutic options for dysregulated GPCRs functions. However, studies regarding the functional selectivity of Receptor Tyrosine Kinases (RTKs) remain sparse. Here, we will summarize recent data about RTK functional selectivity focusing on how the nature and the amount of RTK ligands and the crosstalk of RTKs with other membrane proteins regulate the specificity of RTK signalling. In addition, we will discuss how structural changes in RTKs upon ligand binding affects selective signalling pathways. Much remains to be known about the integration of different signals affecting RTK signalling specificity to orchestrate long-term cellular outcomes. Recent advancements in omics, specifically quantitative phosphoproteomics, and in systems biology methods to study, model and integrate different types of large-scale omics data have increased our ability to compare several signals affecting RTK functional selectivity in a global, system-wide fashion. We will discuss how such methods facilitate the exploration of important signalling hubs and enable data-driven predictions aiming at improving the efficacy of therapeutics for diseases like cancer, where redundant RTK signalling pathways often compromise treatment efficacy.
1 Introduction
Receptor Tyrosine Kinases (RTKs), including Fibroblast and Epidermal Growth Factor Receptors (FGFR and EGFR, respectively), are plasma membrane-bound receptors that play crucial roles during development and in adult tissue homeostasis by regulating several biological processes like cell proliferation, migration, differentiation, and survival (Sigismund et al., 2017; Wintheiser and Silberstein, 2021; Ornitz and Itoh, 2022). Upon ligand binding, RTKs initiate signalling cascades which regulate such cellular responses, and which include both canonical and non-canonical signalling players (Figure 1). For instance, canonical signalling players include the MAPK, STAT3, and the PI3K-AKT signalling pathways, whereas adhesion molecules are considered non-canonical regulators of RTK signalling (Ferguson et al., 2021). Dysregulation of signalling cascades downstream of RTKs is one of the hallmarks of human diseases, including genetic diseases and cancer, and signalling molecules are among the known drug targets (Orrico, 2023). However, how the cells commit to distinct cellular outputs by regulating RTK signalling cascades in response to perturbations is still a mystery. Uncovering how signalling specificity downstream of RTKs is regulated will open new avenues for targeted therapies for patients.
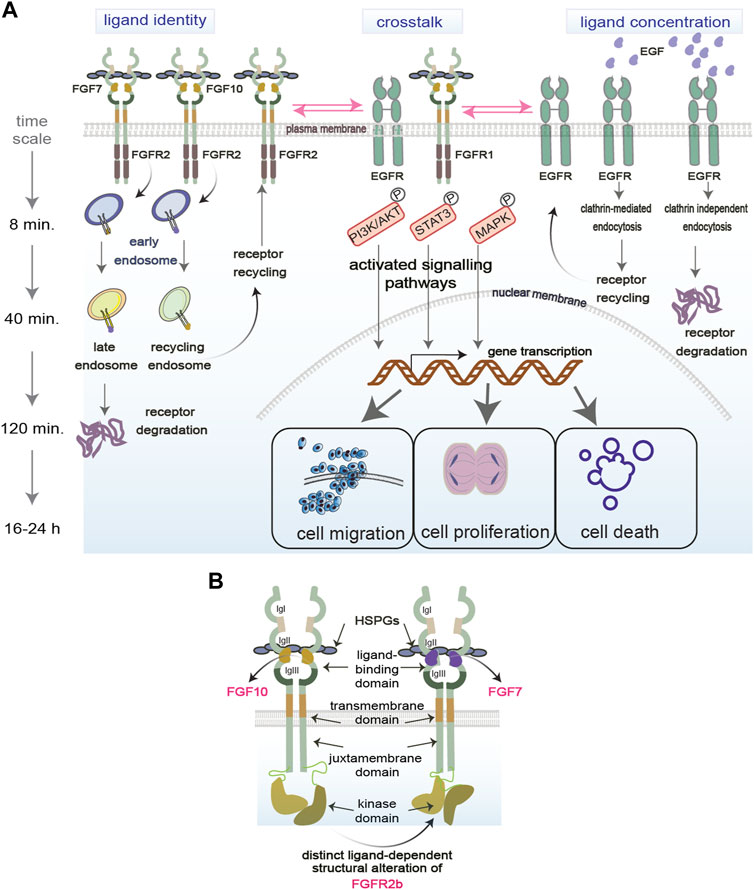
FIGURE 1. Regulation of functional selectivity. (A) RTKs reside on the plasma membrane and are exposed to several factors in the extracellular environment that modulate their functions. Ligand identity and concentration as well as the crosstalk of RTKs with other molecules regulate RTKs signalling (exemplified by PI3K/AKT, STAT3, MAPK) in space (early, late, recycling endosomes) and time (min vs. hours). RTK signalling specificity in turn determines the functional outcomes of the cell (proliferation, migration, death). P, phosphorylation event. (B) Structural regulation of functional selectivity based on the FGFR2b bound to its two known ligands FGF7 or FGF10 and to HSPGs. Different ligands induce different structural alterations that determine the PTMs and downstream signalling. Receptors can dimerise independent of ligands.
2 Functional selectivity
The activation of differential signalling and cellular outputs downstream of the same membrane-bound receptor when activated by two or more different ligands is known as functional selectivity (Karl et al., 2020). Here, we will discuss recent biochemical, cell biological, structural, computational, and system-levels studies of functional selectivity in the context of RTK signalling.
Functional selectivity has been extensively studied for G-protein coupled receptors (GPCRs) to develop more fine-tuned drugs with less side effects (Smith et al., 2018; Onaran and Costa, 2021). However, studies on RTK functional selectivity remain sparse, except for a few advances in the last decade concerning different ligands binding to the same RTK and regulating specific signalling outputs (Karl et al., 2020). Besides distinct ligands priming the receptors for selective signalling pathways, other variables like ligand concentrations in the extracellular environment and crosstalk between RTKs or with other plasma membrane molecules can also mediate differential functional outcomes. The next sections will consider how ligand identity, ligand concentration and other molecules at the plasma membrane drive selective RTK signalling. We will also discuss how functional selectivity is regulated in space and time during receptor trafficking and by RTK structural alterations (Figure 1).
2.1 Ligand identity
Upon binding to the same RTK, distinct ligands initiate specific signalling cascades that lead to differential cellular outcomes (Sato and Nakamura, 2004; Francavilla et al., 2013; Francavilla et al., 2016; Watson et al., 2022) (Figure 1A, on the left). For example, stimulating FGFR2 and EGFR with their cognate ligands regulates distinct cellular outcomes like cell migration, cell proliferation, or cell survival (Francavilla et al., 2013; Francavilla et al., 2016; Watson et al., 2022). This biased signalling due to the binding of distinct ligands to the same receptor was also observed in other RTKs, such as in Insulin receptor family (Bareja et al., 2018). Also different isoforms of ligands can differentially regulate cell signalling, as shown for the two FGF8 isoforms FGF8a and FGF8b, which induces proliferation and differentiation, respectively (Sato and Nakamura, 2004). How ligand identity affects such diversity of signalling outputs is still unknown. Several mechanisms have been suggested, including distinct binding affinity of each ligand to their cognate RTKs; regulation of receptor localisation and post-translational modifications (PTMs); and assembly of specific signalling complexes (Olsen et al., 2006; Zhang et al., 2006; Francavilla et al., 2013; Francavilla et al., 2016) (https://www.biorxiv.org/content/10.1101/2023.09.13.557663v1). For instance, using quantitative phosphoproteomics to analyse global changes of FGFR or EGFR signalling in response to their distinct ligands, it has been shown that distinct PTM profiles on the receptors or on their signalling adaptors drive the assembly of signalling complexes to regulate receptor localization and ultimately cell fate (Francavilla et al., 2013; Francavilla et al., 2016; Smith et al., 2021; Watson et al., 2022). As the dysregulation of the balance between cellular outputs is a hallmark of different diseases, including cancer (Hanahan, 2022), functionally selective RTK signalling holds the promise for the development of improved cancer therapeutics. Therefore, more studies need to be conducted on ligand-receptor relationships through the lens of functional selectivity.
2.2 Ligand concentration
Regulation of cellular fate, particularly during development, depends on the concentration gradient of morphogens, including ligands for different RTKs (Stapornwongkul and Vincent, 2021; Yang et al., 2023). Several studies suggest that concentration-dependent signalling is regulated by a switch-like mechanism, whereby cells respond to extracellular cues only past certain concentration thresholds (Greenfeld et al., 2021; Johnson et al., 2021; Thiemicke and Neuert, 2023). This has been shown for BPM and FGF signalling during early phase of development (Serls et al., 2005; Greenfeld et al., 2021). Consistent with this idea, altering the concentration of the ligands for FGFR or EGFR can “switch” one signalling pathway or cellular outcome to a different outcome (Sato and Nakamura, 2004; Sigismund et al., 2013; Zinkle and Mohammadi, 2018) (https://www.biorxiv.org/content/10.1101/2023.09.13.557663v1) (Figure 1A, on the right). This explains the tight regulation of RTK ligand availability observed in the extracellular matrix under physiological conditions (Thotakura et al., 2019). Indeed, in the case of FGFR ligands binding with low affinity to the Heparan Sulphate Proteoglycans (HSPGs) in the extracellular matrix, an increase in affinity due to changes in the sulphation level of HSPGs, turns a shallow into a steeper one resulting in epithelial cell elongation, but not branching (Makarenkova et al., 2009). Furthermore, a switch-like mechanism provides cells with a degree of buffering, ensuring that stochastic fluctuations in ligand concentration do not initiate unwanted and yet potent mitogenic signalling cascades (Yang et al., 2023). Considering that aberrant RTK ligand concentrations play an important role in cancer (Sharpe et al., 2011), this buffer system is either lost or overloaded, leading to uncontrolled mitogenic signalling. Therefore, more efforts are necessary to uncover the molecular mechanisms integrating the responses to ligand identity and concentration, with the aim of controlling selective cellular outputs in health and diseases.
2.3 Other molecules
An added layer to the RTK signalling complexity is their interplay with other receptors and molecules within the ECM, such as adhesion molecules, integrins and HSPGs (Ferguson et al., 2021) (Figure 1A, in the middle). The crosstalk between different families of RTKs, for instance between FGFR and EGFR, has been described in several cancer cell models with a role in regulating the balance between cellular outputs like cell proliferation and cell motility (Kunii et al., 2008; Smith et al., 2021). This redundant mechanism regulating mitogenic signalling downstream of different RTKs may be exploited in clinical settings, where targeting multiple RTKs in concert produce better clinical outcomes than single target strategies (Quintanal-Villalonga et al., 2019; Le et al., 2021). RTKs are also known to heterodimerize and interact with other receptor classes (Latko et al., 2019; Chen et al., 2023a). For example, elucidation of GPCR-RTK crosstalk has provided with novel targets for the development of psychotherapeutics (Di Liberto et al., 2019). RTK crosstalk with integrins or adhesion molecules was shown to be essential for the control of EGFR or FGFR localization on the plasma membrane and after internalization, which in turn regulates cell migration (Caswell et al., 2008; Francavilla et al., 2009). More recently, it has been shown that also the crosstalk between FGFR2 and EGFR regulates the balance between cell proliferation and migration when the two receptors interact on the recycling endosomes after internalization (Smith et al., 2021). Together with data showing functional interaction of FGFR with cell-surface molecules like NCAM, galectin, and anosmin 1 (Gonzalez-Martinez et al., 2004; Francavilla et al., 2009; Kucinska et al., 2019), this data points to the crucial role of receptor interaction partners at different cellular compartments, such as the plasma membrane or the endosomes, as facilitators of functional selectivity.
2.4 Spatiotemporal control of functional selectivity
The spatiotemporal control of signalling pathways is essential for cellular functions and RTK endocytosis is among the processes regulating signalling in space and time (Sigismund et al., 2021). After ligand-induced receptor internalization via clathrin- or not clathrin-mediated endocytosis, into early endosomes (Figure 1A, on the right), the ligand-receptor pair is still able to signal. For instance, a low concentration of EGF leads to low levels of the PTM ubiquitination on EGFR, clathrin-mediated endocytosis, the recruitment of the signalling adapter protein Grb2 and sustained signalling activation (Sigismund et al., 2013). Furthermore, EGFR phosphorylates Akt and drive pro-survival signalling cascades from the early endosomes (Wang et al., 2002). Therefore, the endosomes are essential for propagating selected signalling cascades downstream of different RTKs. Depending on ligand identity, RTKs are sorted to either late endosomes for degradation into lysosomes or to the recycling endosomes to go back to the cell surface which affects downstream cellular responses and is regulated by different molecular mechanisms (Figure 1A, on the left). For instance, EGF induces EGFR degradation via the PTMs ubiquitylation and phosphorylation (Francavilla et al., 2016) and the assembly of a specific signalling complex (https://www.biorxiv.org/content/10.1101/2023.09.13.557663v1). On the other hand, FGF10 and TGFα induce phosphorylation-regulated recycling of FGFR2b and EGFR respectively, which results in a pro-migratory phenotype in epithelial cells (Francavilla et al., 2013; Francavilla et al., 2016; Smith et al., 2021). The dichotomy between receptor recycling and degradation regulates not only the fate of the ligand/receptor pair but also signalling duration and specificity. For instance, the availability as well as the retention of receptors on the recycling endosomes controls the phosphorylation of the cell cycle regulator CDK1 (Smith et al., 2021) and of the mTOR/ULK-regulated autophagy pathway (Watson et al., 2022). When the permanence of FGFR2b on recycling endosomes is impaired, the coordination of cell motility and cell cycle is lost (Smith et al., 2021), suggesting that the precise localization of FGFR2b signalling as well as the timing of such localization regulate the balance between different cellular responses in response to distinct ligands.
2.5 Selective signalling via RTK structural alterations
Biased signalling may be also regulated by the structural alterations of RTKs at the plasma membrane which would affect downstream signalling cascades (Figure 1B). Changes in the structural conformation of the same GPCR in response to synthetic agonists are known to affect specific signalling pathways due to unique ligand-receptor interactions (Jóźwiak and Płazińska, 2021). Similarly, altered structural conformations have been observed on RTKs in response to different ligands (Mohammadi et al., 2005; Sarabipour and Hristova, 2016; Karl et al., 2020; Huang et al., 2021). For instance, different structural conformation of the ligand-binding pocket has been reported for EGFR upon binding of its two ligands EGF and TGFα (Huang et al., 2021), thus providing a molecular mechanism underlying functional selectivity of EGFR signalling (Francavilla et al., 2016). Altered conformations of receptors may drive distinct post-translational modifications (PTMs) in their cytoplasmic domain, which subsequently can drive functionally distinct cellular outcomes, as shown for FGFR2b (Francavilla et al., 2013; Sarabipour and Hristova, 2016). Finally, strength of dimerization has also been shown to play a role in biased signalling downstream of both EGFRs and FGFRs (Freed et al., 2017; Huang et al., 2017).
Our understanding of the role of conformational changes and strength of receptor dimerization in functional selectivity has increased due to the growing use of structural biology tools such as cryo-Electron Microscopy (EM), x-ray crystallography and fluorescence-based spectroscopic assays (Freed et al., 2017; Huang et al., 2017; Zinkle and Mohammadi, 2018; Huang et al., 2021). Recent data obtained with cryo-EM indicates that the receptor dimerization process may be asymmetric and monomers of different subfamilies of FGFRs can heterodimerise to drive downstream signalling processes (Chen et al., 2023b). This data challenges our current understanding of how functional selectivity is regulated. We envision that recent advancements in cross-linking mass spectrometry (Klykov et al., 2018; O’Reilly and Rappsilber, 2018) and hydrogen-deuterium exchange mass spectrometry (Narang et al., 2020; Javed et al., 2023), both of which can be used to investigate structural alterations of proteins in a dynamic manner, will open novel avenues for structural functional selectivity.
3 Systems biology of functional selectivity
Traditional biochemical and structural methods have been studying functional selectivity by focusing on a small number of targets, failing to account for the complexity and the interconnected nature of the cellular signalling architecture. Systems biology, a field that deals with the emergent properties driven by the complex interactions among biological entities, provides the conceptual and computational frameworks to overcome this barrier (Yue and Dutta, 2022) (Table 1). The last decade has seen a rapid rise in the availability of omics’ data for functional selectivity due to technological advances (Dai and Shen, 2022), and the tandem rise in computational and algorithmic tools (Chen et al., 2023a; Procopio et al., 2023). For instance, phosphoproteomics provided the community with a rich source of large-scale, unbiased data on RTK signalling (Franciosa et al., 2023). A systems level approach would now focus on the global phosphorylation landscape of signalling and their roles when integrated in a system (e.g., a cell) instead of focusing only on selected kinases, PTMs, or one pathway.
One way in which systems biology enables us to study functional selectivity is by building complex networks that can model a particular system (Barabási and Oltvai, 2004; Ma’ayan, 2011). In fact, network science has proved to be a valuable tool in describing and predicting protein-protein interaction networks (Chen et al., 2008; Kovács et al., 2019), gene regulatory and metabolic networks (Lacroix et al., 2008; Emmert-Streib et al., 2014), and in inferring the role of PTMs (Watson et al., 2021; Leutert et al., 2023), among others. By identifying network substructures that are preferentially activated by a particular ligand, network analysis can play a crucial role in studying functional selectivity. For example, building protein interaction networks can uncover novel interaction partners under functionally selective conditions, including cell perturbation with different ligands (Kovács et al., 2019).
Systems biology also provides tools to build mathematical models from large-scale omics’ data. For example, protein co-expression patterns can be used to infer regulatory relationships and subsequent network structure (Mayer et al., 2016). Boolean models can then be used to simulate and study dynamic effects in these networks (Hemedan et al., 2022). Since these models only allows binary states, which is often not the case in biological systems, multistate logic-based models exist that allow the modelling of multiple states (Morris et al., 2010). Other modelling methods include, but are not limited to, ordinary/partial differential equations (ODE/PDE), stochastic models or constraint-based models (see references in Table 1). However, to study functional selectivity via computational modelling of proteomics data, network/logic-based approaches or machine learning algorithms may be more appropriate, since ODE/PDE require dynamic information of signalling entities, which are not always captured by proteomics experiments, and constraint-based models are mostly suited to metabolic networks. Integrating data from different omics’ technologies provides a more comprehensive view of cellular systems, which can elucidate functional selectivity at multiple levels of a protein’s lifecycle (Chen et al., 2023b). More recently, single-cell omics’ analysis has emerged to reveal new mechanisms of functional selectivity based on cell heterogeneity, thus offering insights into how the same signal can lead to different outcomes in different cellular contexts (Ahmad and Budnik, 2023).
4 Conclusion and perspectives
While pharmacological investigations into GPCR ligand bias has made significant strides in recent years, research into RTK ligand bias is lagging behind. More work needs to be done to investigate the role of RTK functional selectivity in health and disease. Furthermore, the availability of large-scale data and state of the art computational methodologies, when utilised in the framework of systems biology, holds the potential to model living systems and being able to predict biological outcomes. If applied to functional selectivity, system biology will allow for rapid evaluation of different functionally selective conditions in a high-throughput manner in silico. This information will then be used to design targeted experiments to validate hypothesis as well as better therapeutics that can preferentially bias RTKs towards a particular cellular outcome (e.g., apoptosis in tumour cells). Research and applications of RTK functional selectivity will take inspiration from the field of GPCRs, where machine learning algorithms have been used to predict if particular chemical scaffolds are more likely to show G-protein or β-arrestin bias (Sanchez et al., 2021). As more data become available on RTK signalling and functional bias with various synthetic and natural ligands, the accumulation of data could facilitate the development of predictive tools like those developed for GPCR ligand bias. Ultimately, this heralds a new era of precision medicine, where the therapeutics are more effective with minimal to no side effects.
Author contributions
SS: Visualization, Writing–original draft. J-MS: Funding acquisition, Writing–review and editing. CF: Conceptualization, Funding acquisition, Supervision, Visualization, Writing–review and editing.
Funding
The author(s) declare financial support was received for the research, authorship, and/or publication of this article. SS: BBSRC Doctoral Training Programme (BB/T008725/1). J-MS: Medical Research Council (MR/T016043/1), Engineering and Physical Sciences Research Council (EP/V038095/1). CF: Wellcome Trust (107636/Z/15/Z and 107636/Z/15/A), the Biotechnology and Biological Sciences Research Council (BB/X001970/1), the Medical Research Council (MR/T016043/1), the NNF Young Investigator Award (call 2022, NNF22OC0070845).
Acknowledgments
We thank all the members of the CF and J-MS team for reading the manuscript and providing suggestions.
Conflict of interest
The authors declare that the research was conducted in the absence of any commercial or financial relationships that could be construed as a potential conflict of interest.
Publisher’s note
All claims expressed in this article are solely those of the authors and do not necessarily represent those of their affiliated organizations, or those of the publisher, the editors and the reviewers. Any product that may be evaluated in this article, or claim that may be made by its manufacturer, is not guaranteed or endorsed by the publisher.
References
Ahmad, R., and Budnik, B. (2023). A review of the current state of single-cell proteomics and future perspective. Anal. Bioanal. Chem. 415 (28), 6889–6899. doi:10.1007/s00216-023-04759-8
Barabási, A.-L., and Oltvai, Z. N. (2004). Network biology: understanding the cell's functional organization. Nat. Rev. Genet. 5 (2), 101–113. doi:10.1038/nrg1272
Bareja, A., Patel, S., Hodgkinson, C. P., Payne, A., and Dzau, V. J. (2018). Understanding the mechanism of bias signaling of the insulin-like growth factor 1 receptor: effects of LL37 and HASF. Cell Signal 46, 113–119. doi:10.1016/j.cellsig.2018.02.013
Caswell, P. T., Chan, M., Lindsay, A. J., McCaffrey, M. W., Boettiger, D., and Norman, J. C. (2008). Rab-coupling protein coordinates recycling of alpha5beta1 integrin and EGFR1 to promote cell migration in 3D microenvironments. J. Cell Biol. 183 (1), 143–155. doi:10.1083/jcb.200804140
Chen, C., Wang, J., Pan, D., Wang, X., Xu, Y., Yan, J., et al. (2023a). Applications of multi-omics analysis in human diseases. MedComm (2020) 4 (4), e315. doi:10.1002/mco2.315
Chen, L., Fu, L., Sun, J., Huang, Z., Fang, M., Zinkle, A., et al. (2023b). Structural basis for FGF hormone signalling. Nature 618 (7966), 862–870. doi:10.1038/s41586-023-06155-9
Chen, P.-Y., Deane, C. M., and Reinert, G. (2008). Predicting and validating protein interactions using network structure. PLoS Comput. Biol. 4 (7), e1000118. doi:10.1371/journal.pcbi.1000118
Dai, X., and Shen, L. (2022). Advances and trends in omics technology development. Front. Med. 9, 911861. doi:10.3389/fmed.2022.911861
Di Liberto, V., Mudo, G., and Belluardo, N. (2019). Crosstalk between receptor tyrosine kinases (RTKs) and G protein-coupled receptors (GPCR) in the brain: focus on heteroreceptor complexes and related functional neurotrophic effects. Neuropharmacology 152, 67–77. doi:10.1016/j.neuropharm.2018.11.018
Emmert-Streib, F., Dehmer, M., and Haibe-Kains, B. (2014). Gene regulatory networks and their applications: understanding biological and medical problems in terms of networks. Front. Cell Dev. Biol. 2, 38. doi:10.3389/fcell.2014.00038
Ferguson, H. R., Smith, M. P., and Francavilla, C. (2021). Fibroblast growth factor receptors (FGFRs) and noncanonical partners in cancer signaling. Cells 10 (5), 1201. doi:10.3390/cells10051201
Francavilla, C., Cattaneo, P., Berezin, V., Bock, E., Ami, D., de Marco, A., et al. (2009). The binding of NCAM to FGFR1 induces a specific cellular response mediated by receptor trafficking. J. Cell Biol. 187 (7), 1101–1116. doi:10.1083/jcb.200903030
Francavilla, C., Papetti, M., Rigbolt, K. T. G., Pedersen, A.-K., Sigurdsson, J. O., Cazzamali, G., et al. (2016). Multilayered proteomics reveals molecular switches dictating ligand-dependent EGFR trafficking. Nat. Struct. Mol. Biol. 23 (6), 608–618. doi:10.1038/nsmb.3218
Francavilla, C., Rigbolt, K. T. G., Emdal, K. B., Carraro, G., Vernet, E., Bekker-Jensen, D. B., et al. (2013). Functional proteomics defines the molecular switch underlying FGF receptor trafficking and cellular outputs. Mol. Cell 51 (6), 707–722. doi:10.1016/j.molcel.2013.08.002
Franciosa, G., Locard-Paulet, M., Jensen, L. J., and Olsen, J. V. (2023). Recent advances in kinase signaling network profiling by mass spectrometry. Curr. Opin. Chem. Biol. 73, 102260. doi:10.1016/j.cbpa.2022.102260
Freed, D. M., Bessman, N. J., Kiyatkin, A., Salazar-Cavazos, E., Byrne, P. O., Moore, J. O., et al. (2017). EGFR ligands differentially stabilize receptor dimers to specify signaling kinetics. Cell 171 (3), 683–695. doi:10.1016/j.cell.2017.09.017
Gjerga, E., Trairatphisan, P., Gabor, A., Koch, H., Chevalier, C., Ceccarelli, F., et al. (2020). Converting networks to predictive logic models from perturbation signalling data with CellNOpt. Bioinformatics 36 (16), 4523–4524. doi:10.1093/bioinformatics/btaa561
Gonzalez-Martinez, D., Kim, S. H., Hu, Y., Guimond, S., Schofield, J., Winyard, P., et al. (2004). Anosmin-1 modulates fibroblast growth factor receptor 1 signaling in human gonadotropin-releasing hormone olfactory neuroblasts through a heparan sulfate-dependent mechanism. J. Neurosci. 24 (46), 10384–10392. doi:10.1523/JNEUROSCI.3400-04.2004
Greenfeld, H., Lin, J., and Mullins, M. C. (2021). The BMP signaling gradient is interpreted through concentration thresholds in dorsal-ventral axial patterning. PLoS Biol. 19 (1), e3001059. doi:10.1371/journal.pbio.3001059
Hanahan, D. (2022). Hallmarks of cancer: new dimensions. Cancer Discov. 12 (1), 31–46. doi:10.1158/2159-8290.CD-21-1059
Hemedan, A. A., Niarakis, A., Schneider, R., and Ostaszewski, M. (2022). Boolean modelling as a logic-based dynamic approach in systems medicine. Comput. Struct. Biotechnol. J. 20, 3161–3172. doi:10.1016/j.csbj.2022.06.035
Hoops, S., Sahle, S., Gauges, R., Lee, C., Pahle, J., Simus, N., et al. (2006). COPASI--a COmplex PAthway SImulator. Bioinformatics 22 (24), 3067–3074. doi:10.1093/bioinformatics/btl485
Huang, Y., Ognjenovic, J., Karandur, D., Miller, K., Merk, A., Subramaniam, S., et al. (2021). A molecular mechanism for the generation of ligand-dependent differential outputs by the epidermal growth factor receptor. Elife 10, e73218. doi:10.7554/eLife.73218
Huang, Z., Tan, Y., Gu, J., Liu, Y., Song, L., Niu, J., et al. (2017). Uncoupling the mitogenic and metabolic functions of FGF1 by tuning FGF1-FGF receptor dimer stability. Cell Rep. 20 (7), 1717–1728. doi:10.1016/j.celrep.2017.06.063
Irurzun-Arana, I., Pastor, J. M., Troconiz, I. F., and Gomez-Mantilla, J. D. (2017). Advanced Boolean modeling of biological networks applied to systems pharmacology. Bioinformatics 33 (7), 1040–1048. doi:10.1093/bioinformatics/btw747
Javed, W., Griffiths, D., and Politis, A. (2023). Hydrogen/deuterium exchange-mass spectrometry of integral membrane proteins in native-like environments: current scenario and the way forward. Essays Biochem. 67 (2), 187–200. doi:10.1042/EBC20220173
Johnson, A. N., Li, G., Jashnsaz, H., Thiemicke, A., Kesler, B. K., Rogers, D. C., et al. (2021). A rate threshold mechanism regulates MAPK stress signaling and survival. Proc. Natl. Acad. Sci. U. S. A. 118 (2), e2004998118. doi:10.1073/pnas.2004998118
Jóźwiak, K., and Płazińska, A. (2021). Structural insights into ligand—receptor interactions involved in biased agonism of G-protein coupled receptors. Molecules 26 (4), 851. doi:10.3390/molecules26040851
Karl, K., Paul, M. D., Pasquale, E. B., and Hristova, K. (2020). Ligand bias in receptor tyrosine kinase signaling. J. Biol. Chem. 295 (52), 18494–18507. doi:10.1074/jbc.REV120.015190
Klamt, S., Saez-Rodriguez, J., and Gilles, E. D. (2007). Structural and functional analysis of cellular networks with CellNetAnalyzer. BMC Syst. Biol. 1, 2. doi:10.1186/1752-0509-1-2
Klarner, H., Streck, A., and Siebert, H. (2017). PyBoolNet: a python package for the generation, analysis and visualization of boolean networks. Bioinformatics 33 (5), 770–772. doi:10.1093/bioinformatics/btw682
Klykov, O., Steigenberger, B., Pektaş, S., Fasci, D., Heck, A. J. R., and Scheltema, R. A. (2018). Efficient and robust proteome-wide approaches for cross-linking mass spectrometry. Nat. Protoc. 13 (12), 2964–2990. doi:10.1038/s41596-018-0074-x
Kolpakov, F., Akberdin, I., Kashapov, T., Kiselev, L., Kolmykov, S., Kondrakhin, Y., et al. (2019). BioUML: an integrated environment for systems biology and collaborative analysis of biomedical data. Nucleic Acids Res. 47 (1), W225–W233. doi:10.1093/nar/gkz440
Kovács, I. A., Luck, K., Spirohn, K., Wang, Y., Pollis, C., Schlabach, S., et al. (2019). Network-based prediction of protein interactions. Nat. Commun. 10 (1), 1240. doi:10.1038/s41467-019-09177-y
Kucinska, M., Porebska, N., Lampart, A., Latko, M., Knapik, A., Zakrzewska, M., et al. (2019). Differential regulation of fibroblast growth factor receptor 1 trafficking and function by extracellular galectins. Cell Commun. Signal 17 (1), 65. doi:10.1186/s12964-019-0371-1
Kunii, K., Davis, L., Gorenstein, J., Hatch, H., Yashiro, M., Di Bacco, A., et al. (2008). FGFR2-amplified gastric cancer cell lines require FGFR2 and Erbb3 signaling for growth and survival. Cancer Res. 68 (7), 2340–2348. doi:10.1158/0008-5472.CAN-07-5229
Lacroix, V., Cottret, L., Thébault, P., and Sagot, M.-F. (2008). An introduction to metabolic networks and their structural analysis. IEEE/ACM Trans. Comput. Biol. Bioinforma. 5 (4), 594–617. doi:10.1109/TCBB.2008.79
Latko, M., Czyrek, A., Porebska, N., Kucinska, M., Otlewski, J., Zakrzewska, M., et al. (2019). Cross-talk between fibroblast growth factor receptors and other cell surface proteins. Cells 8 (5), 455. doi:10.3390/cells8050455
Le, X., Nilsson, M., Goldman, J., Reck, M., Nakagawa, K., Kato, T., et al. (2021). Dual EGFR-VEGF pathway inhibition: a promising strategy for patients with EGFR-mutant NSCLC. J. Thorac. Oncol. 16 (2), 205–215. doi:10.1016/j.jtho.2020.10.006
Leutert, M., Barente, A. S., Fukuda, N. K., Rodriguez-Mias, R. A., and Villen, J. (2023). The regulatory landscape of the yeast phosphoproteome. Nat. Struct. Mol. Biol. 30, 1761–1773. doi:10.1038/s41594-023-01115-3
Maarleveld, T. R., Olivier, B. G., and Bruggeman, F. J. (2013). StochPy: a comprehensive, user-friendly tool for simulating stochastic biological processes. PLoS One 8 (11), e79345. doi:10.1371/journal.pone.0079345
Ma’ayan, A. (2011). Introduction to network analysis in systems biology. Sci. Signal. 4 (190), tr5. doi:10.1126/scisignal.2001965
Makarenkova, H. P., Hoffman, M. P., Beenken, A., Eliseenkova, A. V., Meech, R., Tsau, C., et al. (2009). Differential interactions of FGFs with heparan sulfate control gradient formation and branching morphogenesis. Sci. Signal 2 (88), ra55. doi:10.1126/scisignal.2000304
Matsuoka, Y., Funahashi, A., Ghosh, S., and Kitano, H. (2014). Modeling and simulation using CellDesigner. Methods Mol. Biol. 1164, 121–145. doi:10.1007/978-1-4939-0805-9_11
Mayer, G., Marcus, K., Eisenacher, M., and Kohl, M. M. (2016). Boolean modeling techniques for protein co-expression networks in systems medicine. Expert Rev. Proteomics 13 (6), 555–569. doi:10.1080/14789450.2016.1181546
Mohammadi, M., Olsen, S. K., and Ibrahimi, O. A. (2005). Structural basis for fibroblast growth factor receptor activation. Cytokine Growth Factor Rev. 16 (2), 107–137. doi:10.1016/j.cytogfr.2005.01.008
Morris, M. K., Saez-Rodriguez, J., Sorger, P. K., and Lauffenburger, D. A. (2010). Logic-based models for the analysis of cell signaling networks. Biochemistry 49 (15), 3216–3224. doi:10.1021/bi902202q
Mullen, K. M., and van Stokkum, I. H. M. (2007). TIMP: an R package for modeling multi-way spectroscopic measurements. J. Stat. Softw. 18 (3), 1–46. doi:10.18637/jss.v018.i03
Mussel, C., Hopfensitz, M., and Kestler, H. A. (2010). BoolNet--an R package for generation, reconstruction and analysis of Boolean networks. Bioinformatics 26 (10), 1378–1380. doi:10.1093/bioinformatics/btq124
Naldi, A., Berenguier, D., Faure, A., Lopez, F., Thieffry, D., and Chaouiya, C. (2009). Logical modelling of regulatory networks with GINsim 2.3. Biosystems 97 (2), 134–139. doi:10.1016/j.biosystems.2009.04.008
Narang, D., Lento, C., and J Wilson, D. (2020). HDX-MS: an analytical tool to capture protein motion in action. Biomedicines 8 (7), 224. doi:10.3390/biomedicines8070224
Olsen, S. K., Li, J. Y., Bromleigh, C., Eliseenkova, A. V., Ibrahimi, O. A., Lao, Z., et al. (2006). Structural basis by which alternative splicing modulates the organizer activity of FGF8 in the brain. Genes Dev. 20 (2), 185–198. doi:10.1101/gad.1365406
Onaran, H. O., and Costa, T. (2021). Conceptual and experimental issues in biased agonism. Cell Signal 82, 109955. doi:10.1016/j.cellsig.2021.109955
O’Reilly, F. J., and Rappsilber, J. (2018). Cross-linking mass spectrometry: methods and applications in structural, molecular and systems biology. Nat. Struct. Mol. Biol. 25 (11), 1000–1008. doi:10.1038/s41594-018-0147-0
Ornitz, D. M., and Itoh, N. (2022). New developments in the biology of fibroblast growth factors. WIREs Mech. Dis. 14 (4). doi:10.1002/wsbm.1549
Orrico, K. B. (2023). Basic concepts of cancer genetics and receptor tyrosine kinase inhibition for pharmacists. A narrative review. J. Oncol. Pharm. Pract. 29 (5), 1187–1195. doi:10.1177/10781552231153814
Procopio, A., Cesarelli, G., Donisi, L., Merola, A., Amato, F., and Cosentino, C. (2023). Combined mechanistic modeling and machine-learning approaches in systems biology – a systematic literature review. Comput. Methods Programs Biomed. 240, 107681. doi:10.1016/j.cmpb.2023.107681
Quintanal-Villalonga, A., Molina-Pinelo, S., Cirauqui, C., Ojeda-Márquez, L., Marrugal, Á., Suarez, R., et al. (2019). FGFR1 cooperates with EGFR in lung cancer oncogenesis, and their combined inhibition shows improved efficacy. J. Thorac. Oncol. 14 (4), 641–655. doi:10.1016/j.jtho.2018.12.021
Sanchez, J. E., Kc, G. B., Franco, J., Allen, W. J., Garcia, J. D., and Sirimulla, S. (2021). BiasNet: a model to predict ligand bias toward GPCR signaling. J. Chem. Inf. Model 61 (9), 4190–4199. doi:10.1021/acs.jcim.1c00317
Sarabipour, S., and Hristova, K. (2016). Mechanism of FGF receptor dimerization and activation. Nat. Commun. 7, 10262. doi:10.1038/ncomms10262
Sato, T., and Nakamura, H. (2004). The Fgf8 signal causes cerebellar differentiation by activating the Ras-ERK signaling pathway. Development 131 (17), 4275–4285. doi:10.1242/dev.01281
Serls, A. E., Doherty, S., Parvatiyar, P., Wells, J. M., and Deutsch, G. H. (2005). Different thresholds of fibroblast growth factors pattern the ventral foregut into liver and lung. Development 132 (1), 35–47. doi:10.1242/dev.01570
Sharpe, R., Pearson, A., Herrera-Abreu, M. T., Johnson, D., Mackay, A., Welti, J. C., et al. (2011). FGFR signaling promotes the growth of triple-negative and basal-like breast cancer cell lines both in vitro and in vivo. Clin. Cancer Res. 17 (16), 5275–5286. doi:10.1158/1078-0432.CCR-10-2727
Sigismund, S., Algisi, V., Nappo, G., Conte, A., Pascolutti, R., Cuomo, A., et al. (2013). Threshold-controlled ubiquitination of the EGFR directs receptor fate. EMBO J. 32 (15), 2140–2157. doi:10.1038/emboj.2013.149
Sigismund, S., Avanzato, D., and Lanzetti, L. (2017). Emerging functions of the EGFR in cancer. Mol. Oncol. 12 (1), 3–20. doi:10.1002/1878-0261.12155
Sigismund, S., Lanzetti, L., Scita, G., and Di Fiore, P. P. (2021). Endocytosis in the context-dependent regulation of individual and collective cell properties. Nat. Rev. Mol. Cell Biol. 22 (9), 625–643. doi:10.1038/s41580-021-00375-5
Smith, J. S., Lefkowitz, R. J., and Rajagopal, S. (2018). Biased signalling: from simple switches to allosteric microprocessors. Nat. Rev. Drug Discov. 17 (4), 243–260. doi:10.1038/nrd.2017.229
Smith, M. P., Ferguson, H. R., Ferguson, J., Zindy, E., Kowalczyk, K. M., Kedward, T., et al. (2021). Reciprocal priming between receptor tyrosine kinases at recycling endosomes orchestrates cellular signalling outputs. EMBO J. 40 (14), e107182. doi:10.15252/embj.2020107182
Stapornwongkul, K. S., and Vincent, J. P. (2021). Generation of extracellular morphogen gradients: the case for diffusion. Nat. Rev. Genet. 22 (6), 393–411. doi:10.1038/s41576-021-00342-y
Stoll, G., Caron, B., Viara, E., Dugourd, A., Zinovyev, A., Naldi, A., et al. (2017). MaBoSS 2.0: an environment for stochastic Boolean modeling. Bioinformatics 33 (14), 2226–2228. doi:10.1093/bioinformatics/btx123
Thiemicke, A., and Neuert, G. (2023). Rate thresholds in cell signaling have functional and phenotypic consequences in non-linear time-dependent environments. Front. Cell Dev. Biol. 11, 1124874. doi:10.3389/fcell.2023.1124874
Thotakura, S., Basova, L., and Makarenkova, H. P. (2019). FGF gradient controls boundary position between proliferating and differentiating cells and regulates lacrimal gland growth dynamics. Front. Genet. 10, 362. doi:10.3389/fgene.2019.00362
Wang, Y., Pennock, S., Chen, X., and Wang, Z. (2002). Endosomal signaling of epidermal growth factor receptor stimulates signal transduction pathways leading to cell survival. Mol. Cell Biol. 22 (20), 7279–7290. doi:10.1128/MCB.22.20.7279-7290.2002
Watson, J., Ferguson, H. R., Brady, R. M., Ferguson, J., Fullwood, P., Mo, H., et al. (2022). Spatially resolved phosphoproteomics reveals fibroblast growth factor receptor recycling-driven regulation of autophagy and survival. Nat. Commun. 13 (1), 6589. doi:10.1038/s41467-022-34298-2
Watson, J., Schwartz, J. M., and Francavilla, C. (2021). Using multilayer heterogeneous networks to infer functions of phosphorylated sites. J. Proteome Res. 20 (7), 3532–3548. doi:10.1021/acs.jproteome.1c00150
Wintheiser, G. A., and Silberstein, P. (2021). Physiology, tyrosine kinase receptors. Treasure Island (FL): StatPearls Publishing.
Yang, Y., Li, S., and Luo, L. (2023). Responses of organ precursors to correct and incorrect inductive signals. Trends Cell Biol. S0962-8924 (23), 00191–00195. doi:10.1016/j.tcb.2023.08.008
Yue, R., and Dutta, A. (2022). Computational systems biology in disease modeling and control, review and perspectives. Syst. Biol. Appl. 8 (1), 37. doi:10.1038/s41540-022-00247-4
Zhang, X., Ibrahimi, O. A., Olsen, S. K., Umemori, H., Mohammadi, M., and Ornitz, D. M. (2006). Receptor specificity of the fibroblast growth factor family: the complete mammalian fgf family. J. Biol. Chem. 281 (23), 15694–15700. doi:10.1074/jbc.M601252200
Keywords: functional selectivity, ligand bias, ligand concentration, modelling, receptor tyrosine kinase (RTK), cell signalling, FGFR, EGFR
Citation: Samad SS, Schwartz J-M and Francavilla C (2024) Functional selectivity of Receptor Tyrosine Kinases regulates distinct cellular outputs. Front. Cell Dev. Biol. 11:1348056. doi: 10.3389/fcell.2023.1348056
Received: 01 December 2023; Accepted: 19 December 2023;
Published: 08 January 2024.
Edited by:
Jiri Novotny, Charles University, CzechiaReviewed by:
Francesca Sacco, University of Rome Tor Vergata, ItalyCopyright © 2024 Samad, Schwartz and Francavilla. This is an open-access article distributed under the terms of the Creative Commons Attribution License (CC BY). The use, distribution or reproduction in other forums is permitted, provided the original author(s) and the copyright owner(s) are credited and that the original publication in this journal is cited, in accordance with accepted academic practice. No use, distribution or reproduction is permitted which does not comply with these terms.
*Correspondence: Jean-Marc Schwartz, Jean-Marc.Schwartz@manchester.ac.uk; Chiara Francavilla, chiafra@dtu.dk