- 1Chinese Academy of Sciences Key Laboratory of Genomic and Precision Medicine, Beijing Institute of Genomics, Chinese Academy of Sciences and China National Center for Bioinformation, Beijing, China
- 2University of Chinese Academy of Sciences, Beijing, China
Tumor immunotherapy has made great progress in cancer treatment but still faces several challenges, such as a limited number of targetable antigens and varying responses among patients. Alternative splicing (AS) is an essential process for the maturation of nearly all mammalian mRNAs. Recent studies show that AS contributes to expanding cancer-specific antigens and modulating immunogenicity, making it a promising solution to the above challenges. The organoid technology preserves the individual immune microenvironment and reduces the time/economic costs of the experiment model, facilitating the development of splicing-based immunotherapy. Here, we summarize three critical roles of AS in immunotherapy: resources for generating neoantigens, targets for immune-therapeutic modulation, and biomarkers to guide immunotherapy options. Subsequently, we highlight the benefits of adopting organoids to develop AS-based immunotherapies. Finally, we discuss the current challenges in studying AS-based immunotherapy in terms of existing bioinformatics algorithms and biological technologies.
1 Introduction
Immunotherapy harnesses the immune system to eliminate tumor cells through various methods, including immune checkpoint blockade (ICB), monoclonal antibody (mAb), adoptive cell therapy (ACT), and cancer vaccines (Jain, 2021; Zhu et al., 2021). ICB eliminates cancer cells by binding endogenous immunosuppressive molecules via the antibodies, reactivating exhausted CD8+ cytotoxic T lymphocytes (Topalian et al., 2015). Monoclonal antibodies, ACT, and cancer vaccines are primarily designed based on cancer-specific antigens (Scott et al., 2012; Weiner, 2015). The mAb is derived from a single B-cell clone, engineered to recognize tumor cells through antigen-antibody interactions. When utilizing mAbs in oncology, several mechanisms of action exist to destroy the cancer cells (Bayer, 2019), such as blocking cellular processes (Keam, 2020), flagging cells for an immune attack (Czuczman and Gregory, 2010; Goldsmith et al., 2021), or inducing cell death when mAb is paired with a cytotoxic agent (Thomas et al., 2016). ACT, specifically chimeric antigen receptor (CAR) T-cell immunotherapy, is a highly personalized immunotherapy. Patient-derived T cells are modified to carry a CAR that targets a specific tumor antigen. Once modified, these CAR T-cells are reinfused into the patient to target the tumor cells via CAR and eradicate them through a cytotoxic reaction (Zhang et al., 2017). Cancer vaccines contain external immunoreactive components like neoantigen peptides, nucleic acids, or immunomodulatory agents. Instead of targeting and killing tumors, these substances activate anti-tumor immunity through indirect ways, including increasing the production of tumor-specific antigens and introducing cytokines (Morse et al., 2021; Saxena et al., 2021). These immunotherapies have proven to be a success in treating some types of hematological and solid cancers, especially melanoma (Okazaki et al., 2013; Weiss et al., 2019). A recent study showed that immunotherapy improved the median survival of a subset of patients with advanced melanoma from approximately 6 months to nearly 6 years (Knight et al., 2023). However, they still face difficulties, such as a limited number of immunogenic antigens for selection (Kirkwood et al., 2009; Lawson et al., 2015) and a high rate of treatment resistance (Bagchi et al., 2021).
Most current studies focus on somatic mutation-derived neoantigens or treatment resistance, while the potential role of splicing in this process is often overlooked. Alternative splicing is required for the maturation of mammalian mRNAs in that pre-mRNA introns are removed and various exons are selected and joined, generating diverse transcripts and proteins from the same DNA template (Wang et al., 2008; Nilsen and Graveley, 2010). As reported, more than 95% of human genes undergo pre-mRNA splicing (Pan et al., 2008; Ajith et al., 2016; Baralle and Giudice, 2017; Zhang et al., 2020) and splicing dysregulation has been detected in many cancers (Climente-González et al., 2017; Kahles et al., 2018). Trans-regulator disruptions, such as splicing factors mutations (Harbour et al., 2013; Dvinge et al., 2016) and cis-element changes, are frequently occurred in tumors, which contribute to a wide range of splicing dysregulation and influence tumor formation, tumor metastasis and treatment response (Wu et al., 2023).
First, mis-splicing has been shown to play a role in tumor initiation (Gaur et al., 2008; Stavik et al., 2013; Koh et al., 2015; Shirai et al., 2015; Pellagatti et al., 2018; Huan et al., 2020). For instance, c-Myc increases the transcription of splicing regulators PTB, hnRNPA1, and hnRNPA2, leading to an alternative isoform switch from PKM1 to PKM2 that affect the cancer initiation via cell metabolism (David et al., 2010; Yang et al., 2012; Ma et al., 2022). In addition, mis-splicing of tumor suppressor genes, such as BRCA1, PTEN in breast cancer (Okumura et al., 2011), and KRAS in lung cancer (Pio and Montuenga, 2009; Nussinov et al., 2016; Aran et al., 2018), are also reported to promote tumor initiation (Jung et al., 2015; Venkataramany et al., 2022). Second, oncogenes have also been shown to undergo isoforms switching as a mechanism for cancer cells to metastasize (Choi et al., 2019; Inoue et al., 2019; Fish et al., 2021). CD44 produces variant (CD44v) isoforms by alternative splicing of variant exons. These CD44v isoforms are highly expressed in metastatic tumors, and promote epithelial-to-mesenchymal transition and cell invasion (Brown et al., 2011; Chen et al., 2018). Moreover, there are other isoforms have been reported to promote tumor metastasis, including KLF6-SV1 (DiFeo et al., 2009; Hatami et al., 2013), and BRCA1-IRIS (Bogan et al., 2017) in breast cancer. Third, mis-splicing can also contribute to cancer treatment resistance (Mitra et al., 2009; Palladini et al., 2017; Hsu et al., 2020). For example, the HER2D16 splicing variant is highly expressed in a subset of HER2+ breast cancer patients with resistance to trastuzumab, a HER2-targeted therapy (Palladini et al., 2017; Hsu et al., 2020). Similarly, one BRAF(V600E) splicing variant lacks the binding domain of the RAF inhibitor vemurafenib, leading to acquired drug resistance in melanoma patients with BRAF mutations (Poulikakos et al., 2011). Besides the above splicing variants, other cancer-associated splicing events have been summarized in Table 1.
Interestingly, recent studies have highlighted a new function of AS in tumors as an important source to expand the pool of neoantigens (Frankiw et al., 2019; Wang et al., 2021) as well as adjusting tumor immune microenvironments (Li et al., 2019; Zhong et al., 2022), which suggests that AS is also implicated in tumor immunotherapy. Currently, the studies of AS-associated immunotherapy rely on time/cost-consuming animal experiments (Ott et al., 2017; Chen et al., 2019; Arnaud et al., 2020; Cheng et al., 2022). Particularly, immunotherapies targeting AS also need suitable models for assessment before clinical. Thus, organoids, a 3D in vitro culture system derived from autologous tissue stem cells, may facilitate the development of splicing-based immunotherapies (Xu et al., 2018; Yuki et al., 2020). Numerous studies have demonstrated that organoid technology can provide a high-throughput screening and validation platform to reduce experimental costs and improve validation efficiency (Liu L. et al., 2021; Guillen et al., 2022). Moreover, it can also mimic the in vivo microenvironment of the original patient tissue, offering better personalized and rapid models for pre-clinical evaluation (Xu et al., 2018).
In this review, we summarize three critical roles of AS in immunotherapy: neoantigen resources for antigen-based immunotherapy, modulatory targets for adjuvant immunotherapy, and therapeutic biomarkers to guide immunotherapy options. We also highlight the potential advantages of adopting organoids to study splicing-based immunotherapy. Finally, we discuss current challenges in identifying immunotherapy-related AS events and targeting AS in immunotherapy from the perspectives of bioinformatics algorithms and biological technology.
2 Section
2.1 AS serve as neoantigen resources for antigen-based immunotherapy
The effect of immunotherapy varies across tumor types and patient populations (Okazaki et al., 2013; Weiss et al., 2019; Chamoto et al., 2020), highlighting the necessity of developing personalized immunotherapies based on tumor-specific antigens, which are absent in normal tissues (Jhunjhunwala et al., 2021). Studies have shown that patients with more tumor-specific antigens receive increased sensitivity to neoantigen-based immunotherapies, as well as activate more potent anti-tumor immune responses under ICB therapy. Therefore, individuals with a high mutation burden tend to produce more neoantigens, which makes them benefit more from immunotherapy (Ott et al., 2017; Yarchoan et al., 2019; Blass and Ott, 2021).
In addition to the somatic mutation, aberrant splicing is also an essential origination of neoantigens. Mis-splicing in various tumor types leads to the production of tumor-specific peptides (Frankiw et al., 2019). Previous studies have shown that peptides derived from mis-splicing can bind to major histocompatibility complex class I (MHC I) for T-cell recognition (Jayasinghe et al., 2018; Kahles et al., 2018; Smart et al., 2018; Frankiw et al., 2019). Although most aberrant splicing may introduce early stop codons leading to nonsense-mediated mRNA decay (NMD), some of these RNAs can still undergo a pioneer round of translation to produce some peptides to activate the immune system (Apcher et al., 2011). Compared to mutations that typically affect a single amino acid, mis-splicing, especially intron retention, often inserts a non-coding sequence into the transcript to generate more neoepitopes (Smart et al., 2018). Furthermore, many tumors often accompany the dysfunction of splicing factors (SFs), which exhibit widespread mis-splicing across the whole transcriptome (Wang and Aifantis, 2020). This global splicing change can produce more neoantigens than somatic SNV in many tumor types. For instance, in breast and ovarian cancers, mis-splicing produces at least twice as many neoantigens as those generated by nonsynonymous mutations (Jayasinghe et al., 2018; Kahles et al., 2018).
The splicing dysregulation in tumors can accelerate the development of neoantigen-based immunotherapy, as it provides an expanded candidate pool of antigens for positive selection. For example, fibronectin (FN) encoded by FN1 is a valuable AS-derived antigen resource (Villa et al., 2008; Huijbers et al., 2010; Jailkhani et al., 2019; Xie et al., 2019; Wagner et al., 2021). Through alternative splicing, FN1 can generate three distinct adhesive extracellular matrix isoforms, each with unique structural regions: V (IIICS), EIIIA (EDA), and EIIIB (EDB) (Dubin et al., 1995). Studies show that fibronectin containing EDA and EDB segments were significantly upregulated during tumor angiogenesis; while displaying low expression levels in normal adult tissues (Khan et al., 2005; Su et al., 2020). Based on this unique AS pattern, the CAR T-cell, mAbs, and cancer vaccine against EDA or EDB have been developed and shown to reduce tumor growth in several solid tumors, including melanoma and lung adenocarcinoma (Villa et al., 2008; Huijbers et al., 2010; Xie et al., 2019; Wagner et al., 2021). It is worth noting that these two splicing derivatives are accumulated in neovasculature, which is present in most solid tumors. Thus, immunotherapies based on EDA and EDB hold promise for extensive applications across tumors.
CD44 is another important gene whose AS-derived antigens have been targeted by many immunotherapies. For example, the mAb RG7356 targets the CD44s isoform and has shown efficacy in clinical trials for acute myeloid leukemia (Vey et al., 2016; D'Arena et al., 2014). Another mAb selectively targeting CD44v6 has also shown success in treating many cancers, including squamous cell carcinomas and a subset of adenocarcinomas (Heider et al., 2004). In ACT therapies, CD44v6-targeted CAR-T cells coexpressing a suicide gene eradicate autologous leukemia in vivo (Casucci et al., 2013). Besides targeting the FN and CD44 isoform, there also exist many successful immunotherapy designs targeting AS-derived other neoantigens (Table 2), such as a mAb against the splicing structural domain D of tenascin C (Nadal et al., 2020), mAb designed to bind to CLDN18.2 peptide (Sahin et al., 2008), and a cancer vaccination based on D393-CD20 peptide (Vauchy et al., 2015). Although additional function and safety tests are required, these designs hold an excellent prospect for clinical usage.
Typically, developing an immunotherapy strategy targeting splicing-derived neoantigen involves the following steps (Figure 1). First, bioinformatics approaches are used to detect cancer-specific AS events, screen AS-derived peptides, and predict the immunogenicity of peptide candidates. Then, based on the prioritized list of peptide candidates, peptides are synthesized to mimic potential immunogenic epitopes. Next, in vitro validations, such as peptide-MHC stability assay and T-cell function assay, are conducted to evaluate the immunogenicity of candidate peptides. Finally, in vivo validations with animal models are carried out to examine the effect of neoantigen-based immunotherapies. The efficacy of the designed therapy can be evaluated by phenotypes like changes in tumor size and immune cell infiltration (Ott et al., 2017; Chen et al., 2019; Arnaud et al., 2020; Cheng et al., 2022).
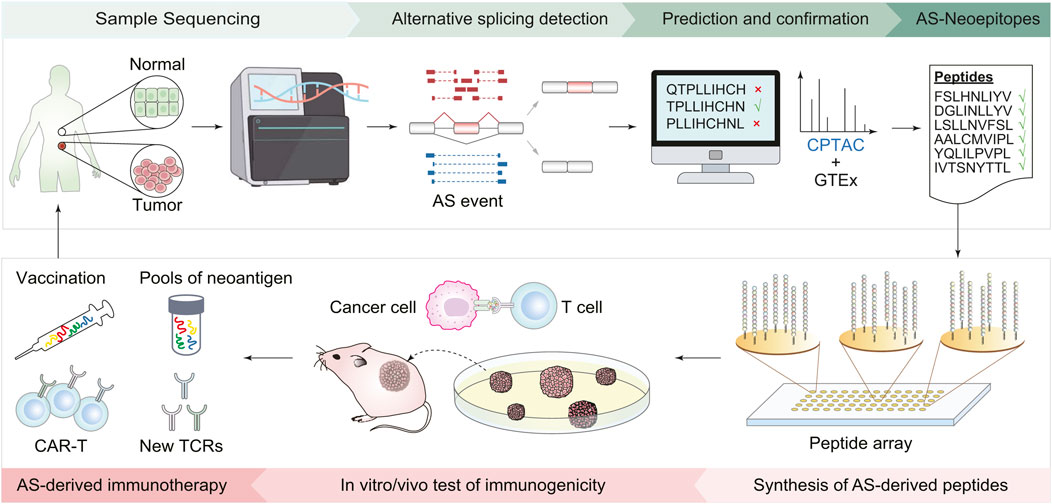
FIGURE 1. Workflow of immunotherapy design based on AS-derived cancer neoantigens. First, collect tumor and normal tissues from cancer patients and perform transcriptomic sequencing. Bioinformatics tools are then employed to identify AS events, screen peptides derived from AS, and predict their immunogenicity. Based on the list of predicted peptide candidates, peptides are synthesized accordingly. The next steps involve validation experiments both in the cells and living organisms, including using cell lines to assess the stability of peptide-MHC, T-cell function to evaluate the immune response of peptide candidates, and mouse models to check the effect of the AS-derived immunotherapies. Finally, new cancer vaccines and CAR-T cells are designed based on immunogenic AS derivates.
Tools have been developed for neoantigen screening by evaluating the binding affinity between splicing-derived peptides and various MHC-I (MHC-II) allotypes (Bayer, 2019). These Peptide-MHC immunogenicity prediction tools generally fall into two categories: scoring-based, for instance, PSSMHCpan (Liu G. et al., 2017) and MixMHCpred (Bassani-Sternberg et al., 2017), and machine learning-based, such as POLYSOLVER (Shukla et al., 2015). Upon extraction of AS-derived antigens that bind strongly to MHC molecules, it is also necessary to use computer tools to assess the immunological activity against the identified antigens. Agent-Based Models (ABMs) are the main computational approaches for such analyses (Butner et al., 2022). In ABM, each cell is represented as a discrete object (agent). These agents interact with their environment following predefined biological rules over discrete time steps (Bonabeau, 2002). One of the most successful ABM methods is IMMSIM (Puzone et al., 2002), which simulates T-cell responses, including T-cell activation, proliferation, differentiation, and antigen recognition. Following this approach (Celada and Seiden, 1992), to date, many ABMs are designed to predict a more comprehensive immune system response, not just the T-cell responses (Celada and Seiden, 1992; Chavali et al., 2008; Pennisi et al., 2013; Madonia et al., 2017; Shou et al., 2022). For example, One ABM model SimB16 was utilized to predict the immune responses of immunotherapy in B16 melanoma (Pappalardo et al., 2011). Another ABM model NetLogo has been successfully adapted to describe the interactions between the immune system and tumor cells (Chiacchio et al., 2014). In addition to ABMs, there are also several alternative approaches, such as Virtual Cell (Resasco et al., 2012) and PySB (Lopez et al., 2013), which use differential equation models to simulate changes in immune cells and cytokines to test the immunological activity. Collectively, these models will greatly speed up the design of immunotherapy targeting splicing-derived neoantigen.
However, the time-consuming development of animal models may not keep pace with the computational identification of antigens for a large-scale candidate screening analysis (Cheng et al., 2022). In future studies, tumor organoids are potential alternative models for the optimization of antigen-based immunotherapy, which will be discussed in subsequent sections.
2.2 AS serve as modulatory targets for adjuvant immunotherapy
As discussed above, the dysregulation of SFs may enhance immunogenicity by inducing widespread splicing defects, suggesting that combining immunotherapy with modulations of splicing factors can improve the therapeutic effect of immunotherapy (Figure 2). In mouse models of several solid tumors, degradation of the splicing factor RBM39 generates numerous AS-derived neoantigens, subsequently stimulating anti-tumor immunity and augmenting the effect of ICB therapy (Lu et al., 2021). In another study of colorectal cancer, the chemical activation of the splicing factor SRSF family achieves a similar effect (Matsushima et al., 2022). Furthermore, splicing manipulation can also boost immunogenicity by generating double-stranded RNA (dsRNAs) (Bowling et al., 2021; Ishak et al., 2021). Recent studies have revealed that spliceosome-targeted therapies can cause widespread cytoplasmic accumulation of mis-spliced mRNAs, a significant portion of which can form dsRNAs. These dsRNAs can be recognized by intracellular immune sensors and induce apoptosis in breast cancer cells (Bowling et al., 2021). Although only confirmed in mouse models, these examples provide compelling evidence that splicing modulation can activate immunogenicity and enhance the response to immunotherapy by potentially turning “cold” tumors into “hot” tumors (Galon and Bruni, 2019).
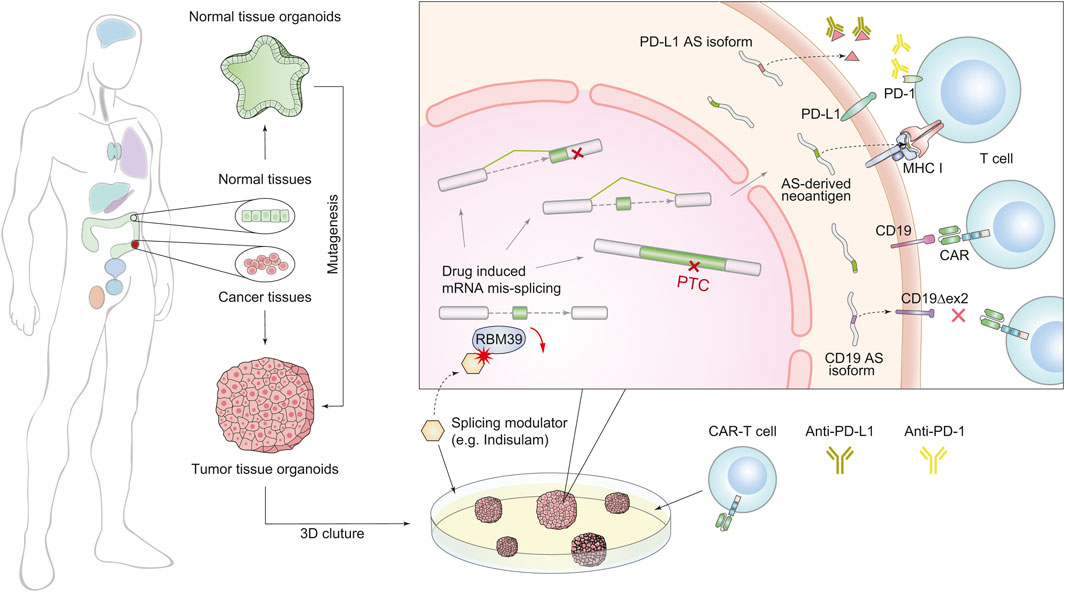
FIGURE 2. Schematic diagram of combined treatment of immunotherapy and splicing modulations using the organoid system. The organoid culture technology (left) accelerates the combination therapy (right). We generated two forms of splicing modulation as a complementary strategy for immunotherapy. The first one is targeting splicing factors to induce global splicing errors for expanding peptide diversity, e.g., RBM39. The second one is targeting AS derivatives that induce treatment resistance, e.g., PD-L1 and CD19 isoforms.
Besides the above contributions of AS-induced immunogenicity, on the other side, many mis-splicing events have also been found to associate with immunotherapy resistance (Gamonet et al., 2015a; Gamonet et al., 2015b; Sotillo et al., 2015; Fischer et al., 2017; Wang and Lee, 2018; Gong et al., 2019; Sciarrillo et al., 2020; Troiani et al., 2020; Deng et al., 2021; Qu et al., 2021; Bourcier and Abdel-Wahab, 2022; Zheng et al., 2022). For example, (Figure 2), in non-small cell lung cancer, secreted PD-L1 splicing isoforms can compete for binding PD-L1 antibodies, resulting in resistance to PD-L1 blockade therapy (Gong et al., 2019). In lung adenocarcinoma, aberrant splicing of the PD-L1 gene can produce a long non-coding RNA (lncRNA), which promotes resistance by enhancing c-Myc activity (Qu et al., 2021). Although CART-19 treatment targeting CD19 achieves a 70% response rate in patients with B cell acute lymphoblastic leukemia, tumors can also evade treatment via generating CD19 isoforms lacking exon 2 (Sotillo et al., 2015). These observations suggest that targeting harmful AS derivates is a complementary strategy for immunotherapy.
It should be noted that AS-driven resistance to immunotherapy works by different mechanisms. Therefore, the approaches to overcome these resistances vary accordingly (Figure 2). The secreted PD-L1 isoforms lead to drug resistance through competition for PD-L1 antibodies. Thus, using PD-1 antibodies or depleting the AS derivatives by antisense oligonucleotides (ASOs) are practical options (Gong et al., 2019). However, CD19 variants alter the antigen structure and escape from recognition by conventional CART-19. To overcome this resistance, depletion of the CD19 splicing variants cannot rescue the lack of CD19 antigen, and a newly engineered CAR T-cell targeting the CD19 AS-derived antigen is a better choice (Sotillo et al., 2015).
2.3 AS serve as therapeutic biomarkers to guide immunotherapy options
Inter-tumor heterogeneity presents a major reason for variable responses to immunotherapy (Saito et al., 2019; Chen et al., 2021). Distinct immune subtypes based on transcriptome successfully predict prognosis and immunotherapy responses in some cancer types (Chen et al., 2021). Alternative splicing is an essential source of transcriptomic and proteomic heterogeneity, which can further help to improve patient stratification. Mis-splicing can remodel the immune microenvironment in tumors. Studies have shown that AS-derived neoantigens can participate in immune reprogramming and directly influence the formation of the tumor microenvironment (TME) (Zhang Y. et al., 2021), suggesting that patients who harbor different splicing substrates may respond differently to the same immunotherapy (Zhang Y. et al., 2021; Su et al., 2022). Another study has validated this hypothesis in lung adenocarcinoma. This study showed that a specific subset of patients with a particular splicing pattern had been found to have higher immunogenicity, leading to better response rates to ICB therapy than other patients (Wu et al., 2022).
Furthermore, splicing alterations typically occur in a cancer-specific or stage-specific manner (Bonnal et al., 2020), indicating that the AS can act as biomarkers to optimize the therapeutic strategy (Le et al., 2015; Chandrakesan et al., 2020; Yang et al., 2022). Mis-splicing can serve as biomarkers for immunotherapy response. Similar to the correlation observed between ICB response and tumor mutational burden, patients with a higher mis-splicing disorder in the tumor tissues may present a higher response rate of ICB therapy (Frankiw et al., 2019; Lu et al., 2021; Matsushima et al., 2022). Besides, another study found that specific AS signatures can indicate immune activity and can be used to predict the response to immunotherapy (Chen et al., 2022). Moreover, some splicing variants can lead to resistance to particular immunotherapy. Besides mentioned AS derivates related to resistance, there are also many other AS events related to resistance, highlighting their critical role as therapeutic biomarkers. For example, skipping exons 5 and 6 of CD22 leads to resistance to CD22 CAR T-cells (Zheng et al., 2022), and D393-CD20 can lead to resistance to CD20 mAb therapy (Gamonet et al., 2015b). These observations demonstrate that splicing biomarkers can potentially assist patients in determining immunotherapy choices (Troiani et al., 2020; Qu et al., 2021; Zhao et al., 2021; Weng et al., 2022).
2.4 The benefits of adopting organoids in AS-based immunotherapies
In the above studies, the experimental models play a crucial role in screening AS-derived antigens, evaluating AS-related immunotherapy, and exploring the biological functions of AS events. But the commonly used experimental models in current studies are mainly cancer cell lines and mouse models (Table 2), which have some limitations. First, in vitro culture of cells cannot accurately replicate the interactions with other cell types or the extracellular matrix. Second, differences in the genome and microenvironment between species make it challenging to translate findings from mice to humans. Especially, the nature of poor conservation of intronic sequences and minimal overlap of mis-spliced transcripts between mice and humans (Lieu et al., 2022) suggest that mice may be less useful in modeling patients with AS dysregulation. Last, it is complex and time-consuming to manipulate genes in mice by multigenerational hybridization, which may result in patients missing optimal treatment periods (Shang et al., 2022; Stribbling and Ryan, 2022). Recent studies have shown that organoids are highly effective in disease modeling and are widely utilized for basic research, drug selection and personalized medicine, which may also benefit the splicing-targeted immunotherapies (Drost and Clevers, 2018).
In cancer modeling, tumor organoids can recapitulate the (epi)genetic and phenotypic diversity of distinct tumor cell subclones, as well as their morphological features (LeSavage et al., 2022). Furthermore, tumor organoids also enable the modeling of TME, including the functions of non-neoplastic cells, the signaling of niche-specific soluble factors, and the altered extracellular matrix (Neal et al., 2018; Yuki et al., 2020). To date, many tumor organoids have been developed and reproduced pathological features (Yeung et al., 2010; Boj et al., 2015; Drost et al., 2016; Hubert et al., 2016). For example, tumor organoids with 3D microfluidic devices maintain immune cell composition of the donor tumors and are used to evaluate the response of ICB treatment (Jenkins et al., 2018). Another approach to culture organoids with an air-liquid interface system achieves similar success (Homicsko, 2020). Additionally, tumor organoids can be grown for an extended period, modified to investigate specific genetic alterations and maintain their features across multiple passages, making them wildly used for basic research.
Tumor organoids are a reliable model for the functional study of alternative splicing events (Rossi et al., 2018; Artegiani et al., 2020; Dekkers et al., 2021). For instance, a study uses cortical organoids to demonstrate that the reintroduction of the archaic splicing variant of NOVA1 alters neurodevelopment (Trujillo et al., 2021). Another example of co-culturing organoids and autologous lymphocytes proved that interactions between melanoma tumor cells and T-cells can induce splicing isoform FKBP51s, which is related to the resistance of anti-PD1 blockade therapy (Troiani et al., 2020).
Organoids also provide an ideal model for large-scale drug screening. And specially, drug screening using patient-derived organoids can further guide personalized treatment options. One successful example is ‘eribulin’, selected by cancer xenografts and organoid platforms. Individuals with this treatment achieved complete remission for nearly 5 months (Guillen et al., 2022). More importantly, organoids are valuable for exploring treatment combinations. A previous study has shown that the combination of KRAS inhibitor AMG501 and EGFR inhibitor cetuximab achieves a synergistic effect for treating colorectal cancer organoids with KRASG12C mutation (Amodio et al., 2020). Another tumor organoid from circulating tumor cells of patients proved that GKB202 is a promising adjuvant for 5-FU-based treatment (Li et al., 2021). Furthermore, the organoid is particularly effective in immuno-related drug selection, as the response to immunotherapy is shaped by both cancer cells and the TME (Xu et al., 2018). To date, many organoid platforms have been developed to evaluate the effects of CAR T-cell therapy (Dijkstra et al., 2018; Schnalzger et al., 2019), ICB therapy (Jenkins et al., 2018), or other neoantigen-based immunotherapies (Courau et al., 2019; Gonzalez-Exposito et al., 2019).
Currently, though, there are no organoid platforms for investigating the functional role of alternative splicing, screening AS-derived neoantigens, or directly evaluating splicing-based immunotherapy. The establishment of these platforms in the future will definitely accelerate the application of alternative splicing in immunotherapy (Raue et al., 2023).
3 Discussion
There are questions remain to be addressed in the development of AS-based immunotherapies. Next, we will discuss these challenges in two parts.
3.1 Challenges in the identification of AS events associated with cancer immunotherapy
The priority in identifying immunotherapy-related AS derivates is the comprehensive detection of AS events. Numerous computational tools have been developed to detect AS events, yet selecting the optimal algorithm remains challenging due to the large inconsistencies between the outputs of different software (Wang et al., 2015; Mehmood et al., 2020). Given the different designs and sensitivity of algorithms in detecting different types of AS events, one naive way is to integrate the power of multiple algorithms by manually selecting the results, which will induce artificial bias (Mehmood et al., 2020). There is an urgent need for the customized design of more reliable algorithms, considering the specific characteristics of AS events and research requirements. For example, SF3B1 mutation leads to the cryptical 3`splcing sites (3′ss), many of which are not reported in the latest annotation reference. As there is no method specific to detect these 3′ss events, an effective method is needed to expand the reference by creating a dataset-specific annotation file (DeBoever et al., 2015; Liu and Rabadan, 2021).
In addition to algorithm limitations, sequencing technology also encounters obstacles in identifying AS events. Currently, most studies identify and quantify splicing isoforms starting from bulk short-read RNA-seq data (Cieślik and Chinnaiyan, 2018; Ferragut Cardoso et al., 2022; Toffali et al., 2023). These studies typically map the short-read RNA sequences to a reference genome using software such as MISO (Katz et al., 2010) or rMATS (Shen et al., 2014), or assemble de novo using tools including StringTie (Pertea et al., 2015) or Trinity (Grabherr et al., 2011). These methods enable the identification of splicing junctions and estimation of isoform abundance based on read counts, providing a global picture of alternative splicing events over the bulk tissue level. However, the short sequencing reads are limited to detect complex and full-length novel isoforms (De Paoli-Iseppi et al., 2021). Additionally, Bulk RNA-seq data is unable to depict intratumor heterogeneity or identify AS events specifically and commonly expressed in all cancer clones, which are the important feature of neoantigen. Single-cell long-read RNA-seq is a powerful tool for studying splicing heterogeneity, while it still has limitations such as low throughput and technical noise. Future efforts should focus on developing more efficient single-cell long-read RNA-seq technology (Singh et al., 2019) and customized algorithms. Finally, the best practices may involve coupled analysis using both long reads and short reads sequencing techniques (Au et al., 2013).
After identifying and quantifying AS events, researchers need to pinpoint AS event candidates contributing to cancer immunotherapy. Many in silico tools have been employed to predict immunological activity against new antigens (Rammensee et al., 1993; Lin et al., 2008; Andreatta and Nielsen, 2016; Jurtz et al., 2017) and analyze the function of cancer-related AS events (Kahles et al., 2018; Liu B. et al., 2021; Liu and Rabadan, 2021; Qi et al., 2022). However, experimental validation of the immunogenicity of such computationally predicted neoantigens will need to be seriously assessed. Understanding the function of AS events is often challenging because it is difficult to introduce abnormal AS isoforms into the experimental model. Therefore, many experimental studies are only limited to AS events that lead to NMD, because the loss-of-function consequences are easier to manipulate and interpret (Lareau et al., 2007; Thomas et al., 2020; Bradley and Anczuków, 2023). Excitingly, there are new approaches of DNA/RNA-targeted CAS with CRISPR-based screening to carry out more unbiased analysis of splicing events and their impacts on tumor (Mou et al., 2017). It is worth noting that manipulating DNA sequence may induce off-target effects, such as creating unexpected splice sites and disrupting the chromatin structure.
Moreover, current experiment models in this field are based on cell lines or mouse models, but they harbor limitations as discussed above. Organoids offer an alternative model for investigating AS derivatives and neoantigen screening; however, their application in AS-based immunotherapy is still in its infancy. There is an urgent need to build one-stop organoid platforms for studying splicing-based immunotherapy (Chandrakesan et al., 2020; Yang et al., 2022). It should be noted that all organoids, cell lines, and mouse models possess distinct strengths and limitations. The selection of an appropriate model should be guided by the specific research need.
3.2 Challenges in targeting AS in immunotherapy
AS-derived neoantigen may also display a high tumor heterogeneity with a varying distribution in different tumor clones. Immunotherapies based on one single target could only eliminate a part of tumor cells, which may accelerate tumor evolution and disease relapse. Thus, a better option is using multiple-target immunotherapies (such as polyclonal antibodies) or combining multiple therapy strategies, which may cover all cancer clones. Furthermore, some AS products show dramatically higher expression in tumors, which does not mean these AS events are totally absent in normal cells. For example, the reported AS-derived antigens CD44v isoform, CD20 isoform D393-CD20 (Vauchy et al., 2015) and CLDN18 isoform CLDN18.2 (Sahin et al., 2008), are also detected in normal cells. Targeting these neoantigens would also influence normal cells, which may lead to serious side effects. Therefore, it is necessary to control dosing by individualized assessment before treatments.
Instead of targeting AS-derived neoantigens directly, combining immunotherapy with splicing modulations is also a promising treatment solution. Targeting the splicing regulators can induce global splicing changes to expand peptide diversity, enhance immunogenicity, and increase ICB therapy’s efficacy. However, the increased complexity of tumor transcriptomics may enable the rapid evolution of tumors to develop new carcinogenic characteristics, such as immune evasion and treatment resistance (Jayasinghe et al., 2018; Kahles et al., 2018). Thus, it is crucial to balance the benefits and risks when combining SF modulation with immunotherapy, either by adjusting the dose or the timing of the therapy.
It is difficult to directly target harmful AS derivatives due to the design or delivery of antisense oligonucleotides or small molecule drugs. Only a few compounds that target specific RNA isoforms have shown clinical utility to date (Sheridan, 2021). ASOs are designed to correct splicing errors by binding a reverse complementary sequence in a target pre-mRNA, thereby preventing its interaction with the splicing machinery. However, it is still a challenge to deliver ASOs to tumor lesions. For example, Spinraza, an FDA-approved treatment that corrects the splicing of SMN2 for spinal muscular atrophy, must be administered by direct injection into the spinal column. Besides, there are also small-molecule compounds that can induce targeted RNA degradation to prevent harmful mis-splicings (Umuhire Juru and Hargrove, 2021). The first small-molecule drug is Evrysdi, which also targets and corrects the splicing of SMN2 (Sivaramakrishnan et al., 2017; Sheridan, 2021). It allows oral administration but is relatively more complicated to design than ASOs. Notably, currently these two types of drugs are more commonly used in genetic diseases rather than tumors, which means it still demands extensive efforts to apply these methods in tumor immunotherapy.
In conclusion, we emphasize that alternative splicing presents a promising avenue for immunotherapy. While the current study in this field is still in its early stage, breakthroughs in both bioinformatics algorithms and biological technologies are critical to accelerate the development of AS-based immunotherapies.
Author contributions
NH and ZL conceived the idea. NH and ZL drafted the manuscript and prepared the artworks. ZL supervised the whole work. All authors contributed to the article and approved the submitted version.
Funding
This work was supported by the National Natural Science Foundation of China (grant 32170565), National Key R&D Program of China (grant 2022YFC2704202), the Beijing Natural Science Foundation (grant Z200023) and the CAS Hundred Talents Program.
Conflict of interest
The authors declare that the research was conducted in the absence of any commercial or financial relationships that could be construed as a potential conflict of interest.
Publisher’s note
All claims expressed in this article are solely those of the authors and do not necessarily represent those of their affiliated organizations, or those of the publisher, the editors and the reviewers. Any product that may be evaluated in this article, or claim that may be made by its manufacturer, is not guaranteed or endorsed by the publisher.
References
Ajith, S., Gazzara, M. R., Cole, B. S., Shankarling, G., Martinez, N. M., Mallory, M. J., et al. (2016). Position-dependent activity of CELF2 in the regulation of splicing and implications for signal-responsive regulation in T cells. RNA Biol. 13 (6), 569–581. doi:10.1080/15476286.2016.1176663
Amodio, V., Yaeger, R., Arcella, P., Cancelliere, C., Lamba, S., Lorenzato, A., et al. (2020). EGFR blockade reverts resistance to KRAS(G12C) inhibition in colorectal cancer. Cancer Discov. 10 (8), 1129–1139. doi:10.1158/2159-8290.CD-20-0187
Andreatta, M., and Nielsen, M. (2016). Gapped sequence alignment using artificial neural networks: Application to the MHC class I system. Bioinformatics 32 (4), 511–517. doi:10.1093/bioinformatics/btv639
Apcher, S., Daskalogianni, C., Lejeune, F., Manoury, B., Imhoos, G., Heslop, L., et al. (2011). Major source of antigenic peptides for the MHC class I pathway is produced during the pioneer round of mRNA translation. Proc. Natl. Acad. Sci. U. S. A. 108 (28), 11572–11577. doi:10.1073/pnas.1104104108
Aran, V., Masson Domingues, P., Carvalho de Macedo, F., Moreira de Sousa, C. A., Caldas Montella, T., de Souza Accioly, M. T., et al. (2018). A cross-sectional study examining the expression of splice variants K-RAS4A and K-RAS4B in advanced non-small-cell lung cancer patients. Lung Cancer 116, 7–14. doi:10.1016/j.lungcan.2017.12.005
Arnaud, M., Duchamp, M., Bobisse, S., Renaud, P., Coukos, G., and Harari, A. (2020). Biotechnologies to tackle the challenge of neoantigen identification. Curr. Opin. Biotechnol. 65, 52–59. doi:10.1016/j.copbio.2019.12.014
Artegiani, B., Hendriks, D., Beumer, J., Kok, R., Zheng, X., Joore, I., et al. (2020). Fast and efficient generation of knock-in human organoids using homology-independent CRISPR-Cas9 precision genome editing. Nat. Cell Biol. 22 (3), 321–331. doi:10.1038/s41556-020-0472-5
Au, K. F., Sebastiano, V., Afshar, P. T., Durruthy, J. D., Lee, L., Williams, B. A., et al. (2013). Characterization of the human ESC transcriptome by hybrid sequencing. Proc. Natl. Acad. Sci. U. S. A. 110 (50), E4821–E4830. doi:10.1073/pnas.1320101110
Bagchi, S., Yuan, R., and Engleman, E. G. (2021). Immune checkpoint inhibitors for the treatment of cancer: Clinical impact and mechanisms of response and resistance. Annu. Rev. Pathology Mech. Dis. 16 (1), 223–249. doi:10.1146/annurev-pathol-042020-042741
Baralle, F. E., and Giudice, J. (2017). Alternative splicing as a regulator of development and tissue identity. Nat. Rev. Mol. Cell Biol. 18 (7), 437–451. doi:10.1038/nrm.2017.27
Bassani-Sternberg, M., Chong, C., Guillaume, P., Solleder, M., Pak, H., Gannon, P. O., et al. (2017). Deciphering HLA-I motifs across HLA peptidomes improves neo-antigen predictions and identifies allostery regulating HLA specificity. PLoS Comput. Biol. 13 (8), 5725. doi:10.1371/journal.pcbi.1005725
Bayer, V. (2019). An overview of monoclonal antibodies. Seminars Oncol. Nurs. 35 (5), 150927. doi:10.1016/j.soncn.2019.08.006
Blass, E., and Ott, P. A. (2021). Advances in the development of personalized neoantigen-based therapeutic cancer vaccines. Nat. Rev. Clin. Oncol. 18 (4), 215–229. doi:10.1038/s41571-020-00460-2
Bogan, D., Meile, L., El Bastawisy, A., Yousef, H. F., Zekri, A. R. N., Bahnassy, A. A., et al. (2017). The role of BRCA1-IRIS in the development and progression of triple negative breast cancers in Egypt: Possible link to disease early lesion. BMC Cancer 17 (1), 329. doi:10.1186/s12885-017-3283-8
Boise, L. H., González-García, M., Postema, C. E., Ding, L., Lindsten, T., Turka, L. A., et al. (1993). bcl-x, a bcl-2-related gene that functions as a dominant regulator of apoptotic cell death. Cell 74 (4), 597–608. doi:10.1016/0092-8674(93)90508-n
Boj, S. F., Hwang, C. I., Baker, L. A., Chio, I. I. C., Engle, D. D., Corbo, V., et al. (2015). Organoid models of human and mouse ductal pancreatic cancer. Cell 160 (1-2), 324–338. doi:10.1016/j.cell.2014.12.021
Bonabeau, E. (2002). Agent-based modeling: Methods and techniques for simulating human systems. Proc. Natl. Acad. Sci. 99 (3), 7280–7287. doi:10.1073/pnas.082080899
Bonnal, S. C., López-Oreja, I., and Valcárcel, J. (2020). Roles and mechanisms of alternative splicing in cancer - implications for care. Nat. Rev. Clin. Oncol. 17 (8), 457–474. doi:10.1038/s41571-020-0350-x
Bourcier, J., and Abdel-Wahab, O. (2022). Splicing-mediated antigen escape from immunotherapy for B-cell malignancies. Blood Cancer Discov. 3 (2), 87–89. doi:10.1158/2643-3230.BCD-21-0200
Bowling, E. A., Wang, J. H., Gong, F., Wu, W., Neill, N. J., Kim, I. S., et al. (2021). Spliceosome-targeted therapies trigger an antiviral immune response in triple-negative breast cancer. Cell 184 (2), 384–403.e21. doi:10.1016/j.cell.2020.12.031
Bradley, R. K., and Anczuków, O. (2023). RNA splicing dysregulation and the hallmarks of cancer. Nat. Rev. Cancer 23 (3), 135–155. doi:10.1038/s41568-022-00541-7
Brown, R. L., Reinke, L. M., Damerow, M. S., Perez, D., Chodosh, L. A., Yang, J., et al. (2011). CD44 splice isoform switching in human and mouse epithelium is essential for epithelial-mesenchymal transition and breast cancer progression. J. Clin. Invest. 121 (3), 1064–1074. doi:10.1172/JCI44540
Butner, J. D., Dogra, P., Chung, C., Pasqualini, R., Arap, W., Lowengrub, J., et al. (2022). Mathematical modeling of cancer immunotherapy for personalized clinical translation. Nat. Comput. Sci. 2 (12), 785–796. doi:10.1038/s43588-022-00377-z
Casucci, M., Nicolis di Robilant, B., Falcone, L., Camisa, B., Norelli, M., Genovese, P., et al. (2013). CD44v6-targeted T cells mediate potent antitumor effects against acute myeloid leukemia and multiple myeloma. Blood 122 (20), 3461–3472. doi:10.1182/blood-2013-04-493361
Celada, F., and Seiden, P. E. (1992). A computer model of cellular interactions in the immune system. Immunol. Today 13 (2), 56–62. doi:10.1016/0167-5699(92)90135-T
Chamoto, K., Hatae, R., and Honjo, T. (2020). Current issues and perspectives in PD-1 blockade cancer immunotherapy. Int. J. Clin. Oncol. 25 (5), 790–800. doi:10.1007/s10147-019-01588-7
Chan, L. K. Y., Lau, T. S., Chung, K. Y., Tam, C., Cheung, T. H., Yim, S. F., et al. (2021). Short-form thymic stromal lymphopoietin (sfTSLP) is the predominant isoform expressed by gynaecologic cancers and promotes tumour growth. Cancers (Basel) 13 (5), 980. doi:10.3390/cancers13050980
Chandrakesan, P., Panneerselvam, J., May, R., Weygant, N., Qu, D., Berry, W. R., et al. (2020). DCLK1-Isoform2 alternative splice variant promotes pancreatic tumor immunosuppressive M2-macrophage polarization. Mol. Cancer Ther. 19 (7), 1539–1549. doi:10.1158/1535-7163.MCT-19-0776
Chavali, A. K., Gianchandani, E. P., Tung, K. S., Lawrence, M. B., Peirce, S. M., and Papin, J. A. (2008). Characterizing emergent properties of immunological systems with multi-cellular rule-based computational modeling. Trends Immunol. 29 (12), 589–599. doi:10.1016/j.it.2008.08.006
Chen, C., Zhao, S., Karnad, A., and Freeman, J. W. (2018). The biology and role of CD44 in cancer progression: Therapeutic implications. J. Hematol. Oncol. 11 (1), 64. doi:10.1186/s13045-018-0605-5
Chen, F., Zou, Z., Du, J., Su, S., Shao, J., Meng, F., et al. (2019). Neoantigen identification strategies enable personalized immunotherapy in refractory solid tumors. J. Clin. Invest. 129 (5), 2056–2070. doi:10.1172/JCI99538
Chen, X. X., Zhang, B. H., Lu, Y. C., Li, Z. Q., Chen, C. Y., Yang, Y. C., et al. (2022). A novel 16-gene alternative mRNA splicing signature predicts tumor relapse and indicates immune activity in stage I-III hepatocellular carcinoma. Front. Pharmacol. 13, 939912. doi:10.3389/fphar.2022.939912
Chen, Y. P., Lv, J. W., Mao, Y. P., Li, X. M., Li, J. Y., Wang, Y. Q., et al. (2021). Unraveling tumour microenvironment heterogeneity in nasopharyngeal carcinoma identifies biologically distinct immune subtypes predicting prognosis and immunotherapy responses. Mol. Cancer 20 (1), 14. doi:10.1186/s12943-020-01292-5
Cheng, R., Xu, Z., Luo, M., Wang, P., Cao, H., Jin, X., et al. (2022). Identification of alternative splicing-derived cancer neoantigens for mRNA vaccine development. Briefings Bioinforma. 23 (2), bbab553. doi:10.1093/bib/bbab553
Chiacchio, F., Pennisi, M., Russo, G., Motta, S., and Pappalardo, F. (2014). Agent-based modeling of the immune system: NetLogo, a promising framework. Biomed. Res. Int. 2014, 907171. doi:10.1155/2014/907171
Choi, S., Wang, D., Chen, X., Tang, L. H., Verma, A., Chen, Z., et al. (2019). Function and clinical relevance of RHAMM isoforms in pancreatic tumor progression. Mol. Cancer 18 (1), 92. doi:10.1186/s12943-019-1018-y
Cieślik, M., and Chinnaiyan, A. M. (2018). Cancer transcriptome profiling at the juncture of clinical translation. Nat. Rev. Genet. 19 (2), 93–109. doi:10.1038/nrg.2017.96
Climente-González, H., Porta-Pardo, E., Godzik, A., and Eyras, E. (2017). The functional impact of alternative splicing in cancer. Cell Rep. 20 (9), 2215–2226. doi:10.1016/j.celrep.2017.08.012
Courau, T., Bonnereau, J., Chicoteau, J., Bottois, H., Remark, R., Assante Miranda, L., et al. (2019). Cocultures of human colorectal tumor spheroids with immune cells reveal the therapeutic potential of MICA/B and NKG2A targeting for cancer treatment. J. Immunother. Cancer 7 (1), 74. doi:10.1186/s40425-019-0553-9
Czuczman, M. S., and Gregory, S. A. (2010). The future of CD20 monoclonal antibody therapy in B-cell malignancies. Leuk. Lymphoma 51 (6), 983–994. doi:10.3109/10428191003717746
D'Arena, G., Calapai, G., and Deaglio, S. (2014). Anti-CD44 mAb for the treatment of B-cell chronic lymphocytic leukemia and other hematological malignancies: Evaluation of WO2013063498. Expert Opin. Ther. Pat. 24 (7), 821–828. doi:10.1517/13543776.2014.915942
David, C. J., Chen, M., Assanah, M., Canoll, P., and Manley, J. L. (2010). HnRNP proteins controlled by c-Myc deregulate pyruvate kinase mRNA splicing in cancer. Nature 463 (7279), 364–368. doi:10.1038/nature08697
De Paoli-Iseppi, R., Gleeson, J., and Clark, M. B. (2021). Isoform age - splice isoform profiling using long-read technologies. Front. Mol. Biosci. 8, 711733. doi:10.3389/fmolb.2021.711733
DeBoever, C., Ghia, E. M., Shepard, P. J., Rassenti, L., Barrett, C. L., Jepsen, K., et al. (2015). Transcriptome sequencing reveals potential mechanism of cryptic 3' splice site selection in SF3B1-mutated cancers. PLoS Comput. Biol. 11 (3), e1004105. doi:10.1371/journal.pcbi.1004105
Dekkers, J. F., van Vliet, E. J., Sachs, N., Rosenbluth, J. M., Kopper, O., Rebel, H. G., et al. (2021). Long-term culture, genetic manipulation and xenotransplantation of human normal and breast cancer organoids. Nat. Protoc. 16 (4), 1936–1965. doi:10.1038/s41596-020-00474-1
Deng, K., Yao, J., Huang, J., Ding, Y., and Zuo, J. (2021). Abnormal alternative splicing promotes tumor resistance in targeted therapy and immunotherapy. Transl. Oncol. 14 (6), 101077. doi:10.1016/j.tranon.2021.101077
DiFeo, A., Martignetti, J. A., and Narla, G. (2009). The role of KLF6 and its splice variants in cancer therapy. Drug Resist Updat 12 (1-2), 1–7. doi:10.1016/j.drup.2008.11.001
Dijkstra, K. K., Cattaneo, C. M., Weeber, F., Chalabi, M., van de Haar, J., Fanchi, L. F., et al. (2018). Generation of tumor-reactive T cells by Co-culture of peripheral blood lymphocytes and tumor organoids. Cell 174 (6), 1586–1598. doi:10.1016/j.cell.2018.07.009
Drost, J., and Clevers, H. (2018). Organoids in cancer research. Nat. Rev. Cancer 18 (7), 407–418. doi:10.1038/s41568-018-0007-6
Drost, J., Karthaus, W. R., Gao, D., Driehuis, E., Sawyers, C. L., Chen, Y., et al. (2016). Organoid culture systems for prostate epithelial and cancer tissue. Nat. Protoc. 11 (2), 347–358. doi:10.1038/nprot.2016.006
Dubin, D., Peters, J. H., Brown, L. F., Logan, B., Kent, K. C., Berse, B., et al. (1995). Balloon catheterization induced arterial expression of embryonic fibronectins. Arterioscler. Thromb. Vasc. Biol. 15 (11), 1958–1967. doi:10.1161/01.atv.15.11.1958
Dvinge, H., Kim, E., Abdel-Wahab, O., and Bradley, R. K. (2016). RNA splicing factors as oncoproteins and tumour suppressors. Nat. Rev. Cancer 16 (7), 413–430. doi:10.1038/nrc.2016.51
Escobar-Hoyos, L. F., Penson, A., Kannan, R., Cho, H., Singh, R. K., et al. (2020). Altered RNA splicing by mutant p53 activates oncogenic RAS signaling in pancreatic cancer. Cancer Cell 38 (2), 198–211. doi:10.1016/j.ccell.2020.05.010
Ferragut Cardoso, A. P., Banerjee, M., Al-Eryani, L., Sayed, M., Wilkey, D. W., Merchant, M. L., et al. (2022). Temporal modulation of differential alternative splicing in HaCaT human keratinocyte cell line chronically exposed to arsenic for up to 28 wk. Environ. Health Perspect. 130 (1), 17011. doi:10.1289/EHP9676
Fischer, J., Paret, C., El Malki, K., Alt, F., Wingerter, A., Neu, M. A., et al. (2017). CD19 isoforms enabling resistance to CART-19 immunotherapy are expressed in B-ALL patients at initial diagnosis. J. Immunother. Hagerst. Md. 1997) 40 (5), 187–195. doi:10.1097/CJI.0000000000000169
Fish, L., Khoroshkin, M., Navickas, A., Garcia, K., Culbertson, B., Hänisch, B., et al. (2021). A prometastatic splicing program regulated by SNRPA1 interactions with structured RNA elements. Science 372 (6543), 7531. doi:10.1126/science.abc7531
Frankiw, L., Baltimore, D., and Li, G. (2019). Alternative mRNA splicing in cancer immunotherapy. Nat. Rev. Immunol. 19 (11), 675–687. doi:10.1038/s41577-019-0195-7
Galon, J., and Bruni, D. (2019). Approaches to treat immune hot, altered and cold tumours with combination immunotherapies. Nat. Rev. Drug Discov. 18 (3), 197–218. doi:10.1038/s41573-018-0007-y
Gamonet, C., Bole-Richard, E., Delherme, A., Aubin, F., Toussirot, E., Garnache-Ottou, F., et al. (2015a). New CD20 alternative splice variants: Molecular identification and differential expression within hematological B cell malignancies. Exp. Hematol. Oncol. 5 (1), 7–15. doi:10.1186/s40164-016-0036-3
Gamonet, C., Bole-Richard, E., Delherme, A., Aubin, F., Toussirot, E., Garnache-Ottou, F., et al. (2015b). New CD20 alternative splice variants: Molecular identification and differential expression within hematological B cell malignancies. Exp. Hematol. Oncol. 5, 7. doi:10.1186/s40164-016-0036-3
Gaur, S., Shively, J. E., Yen, Y., and Gaur, R. K. (2008). Altered splicing of CEACAM1 in breast cancer: Identification of regulatory sequences that control splicing of CEACAM1 into long or short cytoplasmic domain isoforms. Mol. Cancer 7, 46. doi:10.1186/1476-4598-7-46
Georgilis, A., Klotz, S., Hanley, C. J., Herranz, N., Weirich, B., Morancho, B., et al. (2018). PTBP1-Mediated alternative splicing regulates the inflammatory secretome and the pro-tumorigenic effects of senescent cells. Cancer Cell 34 (1), 85–102. doi:10.1016/j.ccell.2018.06.007
Goldsmith, S. R., Foley, N., and Schroeder, M. A. (2021). Daratumumab for the treatment of multiple myeloma. Drugs Today (Barc) 57 (10), 591–605. doi:10.1358/dot.2021.57.10.3313853
Gong, B., Kiyotani, K., Sakata, S., Nagano, S., Kumehara, S., Baba, S., et al. (2019). Secreted PD-L1 variants mediate resistance to PD-L1 blockade therapy in non-small cell lung cancer. J. Exp. Med. 216 (4), 982–1000. doi:10.1084/jem.20180870
Gonzalez-Exposito, R., Semiannikova, M., Griffiths, B., Khan, K., Barber, L. J., Woolston, A., et al. (2019). CEA expression heterogeneity and plasticity confer resistance to the CEA-targeting bispecific immunotherapy antibody cibisatamab (CEA-TCB) in patient-derived colorectal cancer organoids. J. Immunother. Cancer 7 (1), 101. doi:10.1186/s40425-019-0575-3
Grabherr, M. G., Haas, B. J., Yassour, M., Levin, J. Z., Thompson, D. A., Amit, I., et al. (2011). Full-length transcriptome assembly from RNA-Seq data without a reference genome. Nat. Biotechnol. 29 (7), 644–652. doi:10.1038/nbt.1883
Guillen, K. P., Fujita, M., Butterfield, A. J., Scherer, S. D., Bailey, M. H., Chu, Z., et al. (2022). A human breast cancer-derived xenograft and organoid platform for drug discovery and precision oncology. Nat. Cancer 3 (2), 232–250. doi:10.1038/s43018-022-00337-6
Harbour, J. W., Roberson, E. D. O., Anbunathan, H., Onken, M. D., Worley, L. A., and Bowcock, A. M. (2013). Recurrent mutations at codon 625 of the splicing factor SF3B1 in uveal melanoma. Nat. Genet. 45 (2), 133–135. doi:10.1038/ng.2523
Hatami, R., Sieuwerts, A. M., Izadmehr, S., Yao, Z., Qiao, R. F., Papa, L., et al. (2013). KLF6-SV1 drives breast cancer metastasis and is associated with poor survival. Sci. Transl. Med. 5 (169), 169ra12. doi:10.1126/scitranslmed.3004688
Heider, K. H., Kuthan, H., Stehle, G., and Munzert, G. (2004). CD44v6: A target for antibody-based cancer therapy. Cancer Immunol. Immunother. 53 (7), 567–579. doi:10.1007/s00262-003-0494-4
Homicsko, K. (2020). Organoid technology and applications in cancer immunotherapy and precision medicine. Curr. Opin. Biotechnol. 65, 242–247. doi:10.1016/j.copbio.2020.05.002
Hsu, C. C., Liao, B. C., Liao, W. Y., Markovets, A., Stetson, D., Thress, K., et al. (2020). Exon 16-skipping HER2 as a novel mechanism of osimertinib resistance in EGFR l858r/t790m-positive non-small cell lung cancer. J. Thorac. Oncol. 15 (1), 50–61. doi:10.1016/j.jtho.2019.09.006
Huan, L., Guo, T., Wu, Y., Xu, L., Huang, S., Xu, Y., et al. (2020). Hypoxia induced LUCAT1/PTBP1 axis modulates cancer cell viability and chemotherapy response. Mol. Cancer 19 (1), 11. doi:10.1186/s12943-019-1122-z
Hubert, C. G., Rivera, M., Spangler, L. C., Wu, Q., Mack, S. C., Prager, B. C., et al. (2016). A three-dimensional organoid culture system derived from human glioblastomas recapitulates the hypoxic gradients and cancer stem cell heterogeneity of tumors found in vivo. Cancer Res. 76 (8), 2465–2477. doi:10.1158/0008-5472.CAN-15-2402
Huijbers, E. J., Ringvall, M., Femel, J., Kalamajski, S., Lukinius, A., Abrink, M., et al. (2010). Vaccination against the extra domain-B of fibronectin as a novel tumor therapy. Faseb J. 24 (11), 4535–4544. doi:10.1096/fj.10-163022
Inoue, D., Chew, G. L., Liu, B., Michel, B. C., Pangallo, J., D'Avino, A. R., et al. (2019). Spliceosomal disruption of the non-canonical BAF complex in cancer. Nature 574 (7778), 432–436. doi:10.1038/s41586-019-1646-9
Ishak, C. A., Loo Yau, H., and De Carvalho, D. D. (2021). Spliceosome-targeted therapies induce dsRNA responses. Immunity 54 (1), 11–13. doi:10.1016/j.immuni.2020.12.012
Jailkhani, N., Ingram, J. R., Rashidian, M., Rickelt, S., Tian, C., Mak, H., et al. (2019). Noninvasive imaging of tumor progression, metastasis, and fibrosis using a nanobody targeting the extracellular matrix. Proc. Natl. Acad. Sci. U. S. A. 116 (28), 14181–14190. doi:10.1073/pnas.1817442116
Jain, K. K. (2021). Personalized immuno-oncology. Med. Princ. Pract. 30 (1), 1–16. doi:10.1159/000511107
Jayasinghe, R. G., Cao, S., Gao, Q., Wendl, M. C., Vo, N. S., Reynolds, S. M., et al. (2018). Systematic analysis of splice-site-creating mutations in cancer. Cell Rep. 23 (1), 270–281.e3. doi:10.1016/j.celrep.2018.03.052
Jenkins, R. W., Aref, A. R., Lizotte, P. H., Ivanova, E., Stinson, S., Zhou, C. W., et al. (2018). Ex vivo profiling of PD-1 blockade using organotypic tumor spheroids. Cancer Discov. 8 (2), 196–215. doi:10.1158/2159-8290.CD-17-0833
Jhunjhunwala, S., Hammer, C., and Delamarre, L. (2021). Antigen presentation in cancer: Insights into tumour immunogenicity and immune evasion. Nat. Rev. Cancer 21 (5), 298–312. doi:10.1038/s41568-021-00339-z
Jung, H., Lee, D., Lee, J., Park, D., Kim, Y. J., Park, W. Y., et al. (2015). Intron retention is a widespread mechanism of tumor-suppressor inactivation. Nat. Genet. 47 (11), 1242–1248. doi:10.1038/ng.3414
Jurtz, V., Paul, S., Andreatta, M., Marcatili, P., Peters, B., and Nielsen, M. (2017). NetMHCpan-4.0: Improved peptide–MHC class I interaction predictions integrating eluted ligand and peptide binding affinity data. J. Immunol. 199 (9), 3360–3368. doi:10.4049/jimmunol.1700893
Kahles, A., Lehmann, K. V., Toussaint, N. C., Hüser, M., Stark, S. G., Sachsenberg, T., et al. (2018). Comprehensive analysis of alternative splicing across tumors from 8,705 patients. Cancer Cell 34 (2), 211–224.e6. doi:10.1016/j.ccell.2018.07.001
Katz, Y., Wang, E. T., Airoldi, E. M., and Burge, C. B. (2010). Analysis and design of RNA sequencing experiments for identifying isoform regulation. Nat. Methods 7 (12), 1009–1015. doi:10.1038/nmeth.1528
Keam, S. J. (2020). Trastuzumab deruxtecan: First approval. Drugs 80 (5), 501–508. doi:10.1007/s40265-020-01281-4
Khan, Z. A., Chan, B. M., Uniyal, S., Barbin, Y. P., Farhangkhoee, H., Chen, S., et al. (2005). EDB fibronectin and angiogenesis -- a novel mechanistic pathway. Angiogenesis 8 (3), 183–196. doi:10.1007/s10456-005-9017-6
Kim, E., Ilagan, J. O., Liang, Y., Daubner, G. M., Lee, S. C. W., Ramakrishnan, A., et al. (2015). SRSF2 mutations contribute to myelodysplasia by mutant-specific effects on exon recognition. Cancer Cell 27 (5), 617–630. doi:10.1016/j.ccell.2015.04.006
Kirkwood, J. M., Lee, S., Moschos, S. J., Albertini, M. R., Michalak, J. C., Sander, C., et al. (2009). Immunogenicity and antitumor effects of vaccination with peptide vaccine+/-granulocyte-monocyte colony-stimulating factor and/or IFN-alpha2b in advanced metastatic melanoma: Eastern Cooperative Oncology Group Phase II Trial E1696. Clin. Cancer Res. 15 (4), 1443–1451. doi:10.1158/1078-0432.CCR-08-1231
Knight, A., Karapetyan, L., and Kirkwood, J. M. (2023). Immunotherapy in melanoma: Recent advances and future directions. Cancers (Basel) 15 (4), 1106. doi:10.3390/cancers15041106
Koh, C. M., Bezzi, M., Low, D. H. P., Ang, W. X., Teo, S. X., Gay, F. P. H., et al. (2015). MYC regulates the core pre-mRNA splicing machinery as an essential step in lymphomagenesis. Nature 523 (7558), 96–100. doi:10.1038/nature14351
Lamba, J. K., Chauhan, L., Shin, M., Loken, M. R., Pollard, J. A., Wang, Y. C., et al. (2017). CD33 splicing polymorphism determines gemtuzumab ozogamicin response in de novo acute myeloid leukemia: Report from randomized phase III children's oncology group trial AAML0531. J. Clin. Oncol. 35 (23), 2674–2682. doi:10.1200/JCO.2016.71.2513
Lareau, L. F., Inada, M., Green, R. E., Wengrod, J. C., and Brenner, S. E. (2007). Unproductive splicing of SR genes associated with highly conserved and ultraconserved DNA elements. Nature 446 (7138), 926–929. doi:10.1038/nature05676
Lawson, D. H., Lee, S., Zhao, F., Tarhini, A. A., Margolin, K. A., Ernstoff, M. S., et al. (2015). Randomized, placebo-controlled, phase III trial of yeast-derived granulocyte-macrophage colony-stimulating factor (GM-CSF) versus peptide vaccination versus GM-CSF plus peptide vaccination versus placebo in patients with No evidence of disease after complete surgical resection of locally advanced and/or stage IV melanoma: A trial of the eastern cooperative oncology group-American college of radiology imaging network cancer research group (E4697). J. Clin. Oncol. 33 (34), 4066–4076. doi:10.1200/JCO.2015.62.0500
Le, K. Q., Prabhakar, B. S., Hong, W. j., and Li, L. c. (2015). Alternative splicing as a biomarker and potential target for drug discovery. Acta Pharmacol. Sin. 36 (10), 1212–1218. doi:10.1038/aps.2015.43
Lee, S. C., North, K., Kim, E., Jang, E., Obeng, E., Lu, S. X., et al. (2018). Synthetic lethal and convergent biological effects of cancer-associated spliceosomal gene mutations. Cancer Cell 34 (2), 225–241. doi:10.1016/j.ccell.2018.07.003
LeSavage, B. L., Suhar, R. A., Broguiere, N., Lutolf, M. P., and Heilshorn, S. C. (2022). Next-generation cancer organoids. Nat. Mater. 21 (2), 143–159. doi:10.1038/s41563-021-01057-5
Li, T. J., Lin, T. W., Wu, S. P., Chu, H. T., Kuo, Y. H., Chiou, J. F., et al. (2021). Patient-derived tumor chemosensitization of GKB202, an antrodia cinnamomea mycelium-derived bioactive compound. Molecules 26 (19), 6018. doi:10.3390/molecules26196018
Li, Z. X., Zheng, Z. Q., Wei, Z. H., Zhang, L. L., Li, F., Lin, L., et al. (2019). Comprehensive characterization of the alternative splicing landscape in head and neck squamous cell carcinoma reveals novel events associated with tumorigenesis and the immune microenvironment. Theranostics 9 (25), 7648–7665. doi:10.7150/thno.36585
Lieu, Y. K., Liu, Z., Ali, A. M., Wei, X., Penson, A., Zhang, J., et al. (2022). SF3B1 mutant-induced missplicing of MAP3K7 causes anemia in myelodysplastic syndromes. Proc. Natl. Acad. Sci. U. S. A. 119 (1), e2111703119. doi:10.1073/pnas.2111703119
Lin, H. H., Zhang, G. L., Tongchusak, S., Reinherz, E. L., and Brusic, V. (2008). Evaluation of MHC-II peptide binding prediction servers: Applications for vaccine research. BMC Bioinforma. 9 (12), S22. doi:10.1186/1471-2105-9-S12-S22
Liu, B., Liu, Z., Chen, S., Ki, M., Erickson, C., Reis-Filho, J. S., et al. (2021b). Mutant SF3B1 promotes AKT- and NF-κB-driven mammary tumorigenesis. J. Clin. Invest. 131 (1), e138315. doi:10.1172/JCI138315
Liu, G., Li, D., Li, Z., Qiu, S., Li, W., Chao, C. C., et al. (2017a). PSSMHCpan: A novel PSSM-based software for predicting class I peptide-HLA binding affinity. Gigascience 6 (5), 1–11. doi:10.1093/gigascience/gix017
Liu, L., Yu, L., Li, Z., Li, W., and Huang, W. (2021a). Patient-derived organoid (PDO) platforms to facilitate clinical decision making. J. Transl. Med. 19 (1), 40. doi:10.1186/s12967-020-02677-2
Liu, X., Si, W., He, L., Ren, J., Yang, Z., et al. (2017b). JMJD6 promotes melanoma carcinogenesis through regulation of the alternative splicing of PAK1, a key MAPK signaling component. Mol. Cancer 16 (1), 175. doi:10.1186/s12943-017-0744-2
Liu, Z., and Rabadan, R. (2021). Computing the role of alternative splicing in cancer. Trends Cancer 7 (4), 347–358. doi:10.1016/j.trecan.2020.12.015
Lopez, C. F., Muhlich, J. L., Bachman, J. A., and Sorger, P. K. (2013). Programming biological models in Python using PySB. Mol. Syst. Biol. 9, 646. doi:10.1038/msb.2013.1
Lu, S. X., De Neef, E., Thomas, J. D., Sabio, E., Rousseau, B., Gigoux, M., et al. (2021). Pharmacologic modulation of RNA splicing enhances anti-tumor immunity. Cell 184 (15), 4032–4047.e31. doi:10.1016/j.cell.2021.05.038
Ma, W. K., Voss, D. M., Scharner, J., Costa, A. S. H., Lin, K. T., Jeon, H. Y., et al. (2022). ASO-based PKM splice-switching therapy inhibits hepatocellular carcinoma growth. Cancer Res. 82 (5), 900–915. doi:10.1158/0008-5472.CAN-20-0948
Madonia, A., Melchiorri, C., Bonamano, S., Marcelli, M., Bulfon, C., Castiglione, F., et al. (2017). Computational modeling of immune system of the fish for a more effective vaccination in aquaculture. Bioinformatics 33 (19), 3065–3071. doi:10.1093/bioinformatics/btx341
Matsushima, S., Ajiro, M., Iida, K., Chamoto, K., Honjo, T., and Hagiwara, M. (2022). Chemical induction of splice-neoantigens attenuates tumor growth in a preclinical model of colorectal cancer. Sci. Transl. Med. 14 (673), 6056. doi:10.1126/scitranslmed.abn6056
Mehmood, A., Laiho, A., Venäläinen, M. S., McGlinchey, A. J., Wang, N., and Elo, L. L. (2020). Systematic evaluation of differential splicing tools for RNA-seq studies. Brief. Bioinform 21 (6), 2052–2065. doi:10.1093/bib/bbz126
Mitra, D., Brumlik, M. J., Okamgba, S. U., Zhu, Y., Duplessis, T. T., Parvani, J. G., et al. (2009). An oncogenic isoform of HER2 associated with locally disseminated breast cancer and trastuzumab resistance. Mol. Cancer Ther. 8 (8), 2152–2162. doi:10.1158/1535-7163.MCT-09-0295
Morse, M. A., Gwin, W. R., and Mitchell, D. A. (2021). Vaccine therapies for cancer: Then and now. Target Oncol. 16 (2), 121–152. doi:10.1007/s11523-020-00788-w
Mou, H., Smith, J. L., Peng, L., Yin, H., Moore, J., Zhang, X. O., et al. (2017). CRISPR/Cas9-mediated genome editing induces exon skipping by alternative splicing or exon deletion. Genome Biol. 18 (1), 108. doi:10.1186/s13059-017-1237-8
Nadal, L., Corbellari, R., Villa, A., Weiss, T., Weller, M., Neri, D., et al. (2020). Novel human monoclonal antibodies specific to the alternatively spliced domain D of Tenascin C efficiently target tumors in vivo. MAbs 12 (1), 1836713. doi:10.1080/19420862.2020.1836713
Neal, J. T., Li, X., Zhu, J., Giangarra, V., Grzeskowiak, C. L., Ju, J., et al. (2018). Organoid modeling of the tumor immune microenvironment. Cell 175 (7), 1972–1988. doi:10.1016/j.cell.2018.11.021
Nilsen, T. W., and Graveley, B. R. (2010). Expansion of the eukaryotic proteome by alternative splicing. Nature 463 (7280), 457–463. doi:10.1038/nature08909
Nittka, S., Böhm, C., Zentgraf, H., and Neumaier, M. (2008). The CEACAM1-mediated apoptosis pathway is activated by CEA and triggers dual cleavage of CEACAM1. Oncogene 27 (26), 3721–3728. doi:10.1038/sj.onc.1211033
Nussinov, R., Tsai, C. J., Chakrabarti, M., and Jang, H. (2016). A new view of ras isoforms in cancers. Cancer Res. 76 (1), 18–23. doi:10.1158/0008-5472.CAN-15-1536
Okazaki, T., Chikuma, S., Iwai, Y., Fagarasan, S., and Honjo, T. (2013). A rheostat for immune responses: The unique properties of PD-1 and their advantages for clinical application. Nat. Immunol. 14 (12), 1212–1218. doi:10.1038/ni.2762
Okumura, N., Yoshida, H., Kitagishi, Y., Nishimura, Y., and Matsuda, S. (2011). Alternative splicings on p53, BRCA1 and PTEN genes involved in breast cancer. Biochem. Biophys. Res. Commun. 413 (3), 395–399. doi:10.1016/j.bbrc.2011.08.098
Ott, P. A., Hu, Z., Keskin, D. B., Shukla, S. A., Sun, J., Bozym, D. J., et al. (2017). An immunogenic personal neoantigen vaccine for patients with melanoma. Nature 547 (7662), 217–221. doi:10.1038/nature22991
Palladini, A., Nicoletti, G., Lamolinara, A., Dall'Ora, M., Balboni, T., Ianzano, M. L., et al. (2017). HER2 isoforms co-expression differently tunes mammary tumor phenotypes affecting onset, vasculature and therapeutic response. Oncotarget 8 (33), 54444–54458. doi:10.18632/oncotarget.17088
Pan, Q., Shai, O., Lee, L. J., Frey, B. J., and Blencowe, B. J. (2008). Deep surveying of alternative splicing complexity in the human transcriptome by high-throughput sequencing. Nat. Genet. 40 (12), 1413–1415. doi:10.1038/ng.259
Pappalardo, F., Martinez Forero, I., Pennisi, M., Palazon, A., Melero, I., and Motta, S. (2011). SimB16: Modeling induced immune system response against B16-melanoma. PLoS One 6 (10), e26523. doi:10.1371/journal.pone.0026523
Pellagatti, A., Armstrong, R. N., Steeples, V., Sharma, E., Repapi, E., Singh, S., et al. (2018). Impact of spliceosome mutations on RNA splicing in myelodysplasia: Dysregulated genes/pathways and clinical associations. Blood 132 (12), 1225–1240. doi:10.1182/blood-2018-04-843771
Pennisi, M., Rajput, A. M., Toldo, L., and Pappalardo, F. (2013). Agent based modeling of Treg-Teff cross regulation in relapsing-remitting multiple sclerosis. BMC Bioinforma. 14 (16), S9. doi:10.1186/1471-2105-14-S16-S9
Pertea, M., Pertea, G. M., Antonescu, C. M., Chang, T. C., Mendell, J. T., and Salzberg, S. L. (2015). StringTie enables improved reconstruction of a transcriptome from RNA-seq reads. Nat. Biotechnol. 33 (3), 290–295. doi:10.1038/nbt.3122
Pio, R., and Montuenga, L. M. (2009). Alternative splicing in lung cancer. J. Thorac. Oncol. 4 (6), 674–678. doi:10.1097/JTO.0b013e3181a520dc
Poulikakos, P. I., Persaud, Y., Janakiraman, M., Kong, X., Ng, C., Moriceau, G., et al. (2011). RAF inhibitor resistance is mediated by dimerization of aberrantly spliced BRAF(V600E). Nature 480 (7377), 387–390. doi:10.1038/nature10662
Puzone, R., Kohler, B., Seiden, P., and Celada, F. (2002). IMMSIM, a flexible model for in machina experiments on immune system responses. Future Gener. Comput. Syst. 18 (7), 961–972. doi:10.1016/s0167-739x(02)00075-4
Qi, T., Wu, Y., Fang, H., Zhang, F., Liu, S., Zeng, J., et al. (2022). Genetic control of RNA splicing and its distinct role in complex trait variation. Nat. Genet. 54 (9), 1355–1363. doi:10.1038/s41588-022-01154-4
Qu, S., Jiao, Z., Lu, G., Yao, B., Wang, T., Rong, W., et al. (2021). PD-L1 lncRNA splice isoform promotes lung adenocarcinoma progression via enhancing c-Myc activity. Genome Biol. 22 (1), 104. doi:10.1186/s13059-021-02331-0
Rammensee, H. G., Falk, K., and Rötzschke, O. (1993). Peptides naturally presented by MHC class I molecules. Annu. Rev. Immunol. 11 (1), 213–244. doi:10.1146/annurev.iy.11.040193.001241
Raue, K. D., Duffy, J. T., Babak, M. V., and Balyasnikova, I. V. (2023). Modeling glioblastoma complexity with organoids for personalized treatments. Trends Mol. Med. 29 (4), 282–296. doi:10.1016/j.molmed.2023.01.002
Resasco, D. C., Gao, F., Morgan, F., Novak, I. L., Schaff, J. C., and Slepchenko, B. M. (2012). Virtual cell: Computational tools for modeling in cell biology. Wiley Interdiscip. Rev. Syst. Biol. Med. 4 (2), 129–140. doi:10.1002/wsbm.165
Rossi, G., Manfrin, A., and Lutolf, M. P. (2018). Progress and potential in organoid research. Nat. Rev. Genet. 19 (11), 671–687. doi:10.1038/s41576-018-0051-9
Sahin, U., Koslowski, M., Dhaene, K., Usener, D., Brandenburg, G., Seitz, G., et al. (2008). Claudin-18 splice variant 2 is a pan-cancer target suitable for therapeutic antibody development. Clin. Cancer Res. 14 (23), 7624–7634. doi:10.1158/1078-0432.CCR-08-1547
Saito, Y., Horiuchi, S., Morooka, H., Ibi, T., Takahashi, N., Ikeya, T., et al. (2019). Inter-tumor heterogeneity of PD-L1 expression in non-small cell lung cancer. J. Thorac. Dis. 11 (12), 4982–4991. doi:10.21037/jtd.2019.12.24
Saxena, M., van der Burg, S. H., Melief, C. J. M., and Bhardwaj, N. (2021). Therapeutic cancer vaccines. Nat. Rev. Cancer 21 (6), 360–378. doi:10.1038/s41568-021-00346-0
Schnalzger, T. E., de Groot, M. H., Zhang, C., Mosa, M. H., Michels, B. E., Röder, J., et al. (2019). 3D model for CAR-mediated cytotoxicity using patient-derived colorectal cancer organoids. Embo J. 38 (12), e100928. doi:10.15252/embj.2018100928
Sciarrillo, R., Wojtuszkiewicz, A., Assaraf, Y. G., Jansen, G., Kaspers, G. J. L., Giovannetti, E., et al. (2020). The role of alternative splicing in cancer: From oncogenesis to drug resistance. Drug Resist Updat 53, 100728. doi:10.1016/j.drup.2020.100728
Scott, A. M., Wolchok, J. D., and Old, L. J. (2012). Antibody therapy of cancer. Nat. Rev. Cancer 12 (4), 278–287. doi:10.1038/nrc3236
Shang, P., Yu, L., Cao, S., Guo, C., and Zhang, W. (2022). An improved cell line-derived xenograft humanized mouse model for evaluation of PD-1/PD-L1 blocker BMS202-induced immune responses in colorectal cancer. Acta Biochim. Biophys. Sin. (Shanghai) 54 (10), 1497–1506. doi:10.3724/abbs.2022145
Shen, S., Park, J. W., Lu, Z. x., Lin, L., Henry, M. D., Wu, Y. N., et al. (2014). rMATS: robust and flexible detection of differential alternative splicing from replicate RNA-Seq data. Proc. Natl. Acad. Sci. U. S. A. 111 (51), E5593–E5601. doi:10.1073/pnas.1419161111
Sheridan, C. (2021). First small-molecule drug targeting RNA gains momentum. Nat. Biotechnol. 39 (1), 6–8. doi:10.1038/s41587-020-00788-1
Shirai, C. L., Ley, J. N., White, B. S., Kim, S., Tibbitts, J., Shao, J., et al. (2015). Mutant U2AF1 expression alters hematopoiesis and pre-mRNA splicing in vivo. Cancer Cell 27 (5), 631–643. doi:10.1016/j.ccell.2015.04.008
Shou, Y., Johnson, S. C., Quek, Y. J., Li, X., and Tay, A. (2022). Integrative lymph node-mimicking models created with biomaterials and computational tools to study the immune system. Mater Today Bio 14, 100269. doi:10.1016/j.mtbio.2022.100269
Shukla, S. A., Rooney, M. S., Rajasagi, M., Tiao, G., Dixon, P. M., Lawrence, M. S., et al. (2015). Comprehensive analysis of cancer-associated somatic mutations in class I HLA genes. Nat. Biotechnol. 33 (11), 1152–1158. doi:10.1038/nbt.3344
Singh, M., Al-Eryani, G., Carswell, S., Ferguson, J. M., Blackburn, J., Barton, K., et al. (2019). High-throughput targeted long-read single cell sequencing reveals the clonal and transcriptional landscape of lymphocytes. Nat. Commun. 10 (1), 3120. doi:10.1038/s41467-019-11049-4
Sivaramakrishnan, M., McCarthy, K. D., Campagne, S., Huber, S., Meier, S., Augustin, A., et al. (2017). Binding to SMN2 pre-mRNA-protein complex elicits specificity for small molecule splicing modifiers. Nat. Commun. 8 (1), 1476. doi:10.1038/s41467-017-01559-4
Smart, A. C., Margolis, C. A., Pimentel, H., He, M. X., Miao, D., Adeegbe, D., et al. (2018). Intron retention is a source of neoepitopes in cancer. Nat. Biotechnol. 36 (11), 1056–1058. doi:10.1038/nbt.4239
Smith, M. A., Choudhary, G. S., Pellagatti, A., Choi, K., Bolanos, L. C., Bhagat, T. D., et al. (2019). U2AF1 mutations induce oncogenic IRAK4 isoforms and activate innate immune pathways in myeloid malignancies. Nat. Cell Biol. 21 (5), 640–650. doi:10.1038/s41556-019-0314-5
Sotillo, E., Barrett, D. M., Black, K. L., Bagashev, A., Oldridge, D., Wu, G., et al. (2015). Convergence of acquired mutations and alternative splicing of CD19 enables resistance to CART-19 immunotherapy. Cancer Discov. 5 (12), 1282–1295. doi:10.1158/2159-8290.CD-15-1020
Stavik, B., Tinholt, M., Sletten, M., Skretting, G., Sandset, P. M., and Iversen, N. (2013). TFPIα and TFPIβ are expressed at the surface of breast cancer cells and inhibit TF-FVIIa activity. J. Hematol. Oncol. 6, 5. doi:10.1186/1756-8722-6-5
Stribbling, S. M., and Ryan, A. J. (2022). The cell-line-derived subcutaneous tumor model in preclinical cancer research. Nat. Protoc. 17 (9), 2108–2128. doi:10.1038/s41596-022-00709-3
Su, H. X., Yang, G., Su, F., Hu, C. X., Zhang, T., Ran, J. T., et al. (2022). Landscape of alternative splicing events related to prognosis and immune infiltration in glioma: A data analysis and basic verification. J. Immunol. Res. 2022, 2671891. doi:10.1155/2022/2671891
Su, X., Ma, X., Xie, X., Wu, H., Wang, L., Feng, Y., et al. (2020). FN-EDA mediates angiogenesis of hepatic fibrosis via integrin-VEGFR2 in a CD63 synergetic manner. Cell Death Discov. 6 (1), 140. doi:10.1038/s41420-020-00378-9
Thomas, A., Teicher, B. A., and Hassan, R. (2016). Antibody-drug conjugates for cancer therapy. Lancet Oncol. 17 (6), e254–e262. doi:10.1016/S1470-2045(16)30030-4
Thomas, J. D., Polaski, J. T., Feng, Q., De Neef, E. J., Hoppe, E. R., McSharry, M. V., et al. (2020). RNA isoform screens uncover the essentiality and tumor-suppressor activity of ultraconserved poison exons. Nat. Genet. 52 (1), 84–94. doi:10.1038/s41588-019-0555-z
Toffali, L., D'Ulivo, B., Giagulli, C., Montresor, A., Zenaro, E., Delledonne, M., et al. (2023). An isoform of the giant protein titin is a master regulator of human T lymphocyte trafficking. Cell Rep. 42 (5), 112516. doi:10.1016/j.celrep.2023.112516
Topalian, S. L., Drake, C. G., and Pardoll, D. M. (2015). Immune checkpoint blockade: A common denominator approach to cancer therapy. Cancer Cell 27 (4), 450–461. doi:10.1016/j.ccell.2015.03.001
Troiani, T., Giunta, E. F., Tufano, M., Vigorito, V., Arrigo, P. D., Argenziano, G., et al. (2020). Alternative macrophage polarisation associated with resistance to anti-PD1 blockade is possibly supported by the splicing of FKBP51 immunophilin in melanoma patients. Br. J. Cancer 122 (12), 1782–1790. doi:10.1038/s41416-020-0840-8
Trujillo, C. A., Rice, E. S., Schaefer, N. K., Chaim, I. A., Wheeler, E. C., Madrigal, A. A., et al. (2021). Reintroduction of the archaic variant of NOVA1 in cortical organoids alters neurodevelopment. Science 371 (6530), eaax2537. doi:10.1126/science.aax2537
Ullmark, T., Järvstråt, L., Sandén, C., Montano, G., Jernmark-Nilsson, H., Lilljebjörn, H., et al. (2017). Distinct global binding patterns of the Wilms tumor gene 1 (WT1) -KTS and +KTS isoforms in leukemic cells. Haematologica 102 (2), 336–345. doi:10.3324/haematol.2016.149815
Umuhire Juru, A., and Hargrove, A. E. (2021). Frameworks for targeting RNA with small molecules. J. Biol. Chem. 296, 100191. doi:10.1074/jbc.REV120.015203
Vauchy, C., Gamonet, C., Ferrand, C., Daguindau, E., Galaine, J., Beziaud, L., et al. (2015). CD20 alternative splicing isoform generates immunogenic CD4 helper T epitopes. Int. J. Cancer 137 (1), 116–126. doi:10.1002/ijc.29366
Venkataramany, A. S., Schieffer, K. M., Lee, K., Cottrell, C. E., Wang, P. Y., Mardis, E. R., et al. (2022). Alternative RNA splicing defects in pediatric cancers: New insights in tumorigenesis and potential therapeutic vulnerabilities. Ann. Oncol. 33 (6), 578–592. doi:10.1016/j.annonc.2022.03.011
Vey, N., Delaunay, J., Martinelli, G., Fiedler, W., Raffoux, E., Prebet, T., et al. (2016). Phase I clinical study of RG7356, an anti-CD44 humanized antibody, in patients with acute myeloid leukemia. Oncotarget 7 (22), 32532–32542. doi:10.18632/oncotarget.8687
Villa, A., Trachsel, E., Kaspar, M., Schliemann, C., Sommavilla, R., Rybak, J. N., et al. (2008). A high-affinity human monoclonal antibody specific to the alternatively spliced EDA domain of fibronectin efficiently targets tumor neo-vasculature in vivo. Int. J. Cancer 122 (11), 2405–2413. doi:10.1002/ijc.23408
Wagner, J., Wickman, E., Shaw, T. I., Anido, A. A., Langfitt, D., Zhang, J., et al. (2021). Antitumor effects of CAR T cells redirected to the EDB splice variant of fibronectin. Cancer Immunol. Res. 9 (3), 279–290. doi:10.1158/2326-6066.CIR-20-0280
Wang, B. D., and Lee, N. H. (2018). Aberrant RNA splicing in cancer and drug resistance. Cancers (Basel) 10 (11), 458. doi:10.3390/cancers10110458
Wang, E., and Aifantis, I. (2020). RNA splicing and cancer. Trends Cancer 6 (8), 631–644. doi:10.1016/j.trecan.2020.04.011
Wang, E. T., Sandberg, R., Luo, S., Khrebtukova, I., Zhang, L., Mayr, C., et al. (2008). Alternative isoform regulation in human tissue transcriptomes. Nature 456 (7221), 470–476. doi:10.1038/nature07509
Wang, J., Ye, Z., Huang, T. H. M., Shi, H., and Jin, V. (2015). A survey of computational methods in transcriptome-wide alternative splicing analysis. Biomol. Concepts 6 (1), 59–66. doi:10.1515/bmc-2014-0040
Wang, T. Y., Liu, Q., Ren, Y., Alam, S. K., Wang, L., Zhu, Z., et al. (2021). A pan-cancer transcriptome analysis of exitron splicing identifies novel cancer driver genes and neoepitopes. Mol. Cell 81 (10), 2246–2260.e12. doi:10.1016/j.molcel.2021.03.028
Weiner, G. J. (2015). Building better monoclonal antibody-based therapeutics. Nat. Rev. Cancer 15 (6), 361–370. doi:10.1038/nrc3930
Weiss, S. A., Wolchok, J. D., and Sznol, M. (2019). Immunotherapy of melanoma: Facts and hopes. Clin. Cancer Res. 25 (17), 5191–5201. doi:10.1158/1078-0432.CCR-18-1550
Weng, Y., Qian, H., Hong, L., Zhao, S., Deng, X., and Shen, B. (2022). Identification of EMT-related alternative splicing event of TMC7 to promote invasion and migration of pancreatic cancer. Front. Immunol. 13, 1089008. doi:10.3389/fimmu.2022.1089008
Wheeler, E. C., Vora, S., Mayer, D., Kotini, A. G., Olszewska, M., Park, S. S., et al. (2022). Integrative RNA-omics discovers GNAS alternative splicing as a phenotypic driver of splicing factor-mutant neoplasms. Cancer Discov. 12 (3), 836–855. doi:10.1158/2159-8290.CD-21-0508
Wu, Q., Feng, L., Wang, Y., Mao, Y., Di, X., Zhang, K., et al. (2022). Multi-omics analysis reveals RNA splicing alterations and their biological and clinical implications in lung adenocarcinoma. Signal Transduct. Target Ther. 7 (1), 270. doi:10.1038/s41392-022-01098-5
Wu, S., Huang, Y., Zhang, M., Gong, Z., Wang, G., Zheng, X., et al. (2023). ASCancer atlas: A comprehensive knowledgebase of alternative splicing in human cancers. Nucleic Acids Res. 51 (D1), D1196–d1204. doi:10.1093/nar/gkac955
Xie, Y. J., Dougan, M., Jailkhani, N., Ingram, J., Fang, T., Kummer, L., et al. (2019). Nanobody-based CAR T cells that target the tumor microenvironment inhibit the growth of solid tumors in immunocompetent mice. Proc. Natl. Acad. Sci. U. S. A. 116 (16), 7624–7631. doi:10.1073/pnas.1817147116
Xu, H., Lyu, X., Yi, M., Zhao, W., Song, Y., and Wu, K. (2018). Organoid technology and applications in cancer research. J. Hematol. Oncol. 11 (1), 116. doi:10.1186/s13045-018-0662-9
Yang, W., Zheng, Y., Xia, Y., Ji, H., Chen, X., Guo, F., et al. (2012). ERK1/2-dependent phosphorylation and nuclear translocation of PKM2 promotes the Warburg effect. Nat. Cell Biol. 14 (12), 1295–1304. doi:10.1038/ncb2629
Yang, Y. S., Jin, X., Li, Q., Chen, Y. Y., Chen, F., Zhang, H., et al. (2022). Superenhancer drives a tumor-specific splicing variant of MARCO to promote triple-negative breast cancer progression. Proc. Natl. Acad. Sci. U. S. A. 119 (46), e2207201119. doi:10.1073/pnas.2207201119
Yarchoan, M., Albacker, L. A., Hopkins, A. C., Montesion, M., Murugesan, K., Vithayathil, T. T., et al. (2019). PD-L1 expression and tumor mutational burden are independent biomarkers in most cancers. JCI Insight 4 (6), e126908. doi:10.1172/jci.insight.126908
Yeung, T. M., Gandhi, S. C., Wilding, J. L., Muschel, R., and Bodmer, W. F. (2010). Cancer stem cells from colorectal cancer-derived cell lines. Proc. Natl. Acad. Sci. U. S. A. 107 (8), 3722–3727. doi:10.1073/pnas.0915135107
Yoshimi, A., Lin, K. T., Wiseman, D. H., Rahman, M. A., Pastore, A., Wang, B., et al. (2019). Coordinated alterations in RNA splicing and epigenetic regulation drive leukaemogenesis. Nature 574 (7777), 273–277. doi:10.1038/s41586-019-1618-0
Yuan, J. H., Liu, X. N., Wang, T. T., Pan, W., Tao, Q. F., Zhou, W. P., et al. (2017). The MBNL3 splicing factor promotes hepatocellular carcinoma by increasing PXN expression through the alternative splicing of lncRNA-PXN-AS1. Nat. Cell Biol. 19 (7), 820–832. doi:10.1038/ncb3538
Yuki, K., Cheng, N., Nakano, M., and Kuo, C. J. (2020). Organoid models of tumor immunology. Trends Immunol. 41 (8), 652–664. doi:10.1016/j.it.2020.06.010
Zhang, C., Liu, J., Zhong, J. F., and Zhang, X. (2017). Engineering CAR-T cells. Biomark. Res. 5, 22. doi:10.1186/s40364-017-0102-y
Zhang, F., Wang, H., Yu, J., Yao, X., Yang, S., Li, W., et al. (2021b). LncRNA CRNDE attenuates chemoresistance in gastric cancer via SRSF6-regulated alternative splicing of PICALM. Mol. Cancer 20 (1), 6. doi:10.1186/s12943-020-01299-y
Zhang, M., Han, Y., Liu, J., Liu, L., Zheng, L., Chen, Y., et al. (2020). Rbm24 modulates adult skeletal muscle regeneration via regulation of alternative splicing. Theranostics 10 (24), 11159–11177. doi:10.7150/thno.44389
Zhang, Y., Wu, X., Li, J., Sun, K., Li, H., Yan, L., et al. (2021a). Comprehensive characterization of alternative splicing in renal cell carcinoma. Brief. Bioinform 22 (5), bbab084. doi:10.1093/bib/bbab084
Zhao, Y., Aldoss, I., Qu, C., Crawford, J. C., Gu, Z., Allen, E. K., et al. (2021). Tumor-intrinsic and -extrinsic determinants of response to blinatumomab in adults with B-ALL. Blood 137 (4), 471–484. doi:10.1182/blood.2020006287
Zheng, S., Gillespie, E., Naqvi, A. S., Hayer, K. E., Ang, Z., Torres-Diz, M., et al. (2022). Modulation of CD22 protein expression in childhood leukemia by pervasive splicing aberrations: Implications for CD22-directed immunotherapies. Blood Cancer Discov. 3 (2), 103–115. doi:10.1158/2643-3230.BCD-21-0087
Zhong, W., Wu, Y., Zhu, M., Zhong, H., Huang, C., Lin, Y., et al. (2022). Alternative splicing and alternative polyadenylation define tumor immune microenvironment and pharmacogenomic landscape in clear cell renal carcinoma. Mol. Ther. Nucleic Acids 27, 927–946. doi:10.1016/j.omtn.2022.01.014
Keywords: alternative splicing, immunotherapy, cancer, organoid, precision medicine
Citation: Han N and Liu Z (2023) Targeting alternative splicing in cancer immunotherapy. Front. Cell Dev. Biol. 11:1232146. doi: 10.3389/fcell.2023.1232146
Received: 31 May 2023; Accepted: 01 August 2023;
Published: 10 August 2023.
Edited by:
Zoltán Wiener, Semmelweis University, HungaryReviewed by:
Arianna Palladini, University of Pavia, ItalyCopyright © 2023 Han and Liu. This is an open-access article distributed under the terms of the Creative Commons Attribution License (CC BY). The use, distribution or reproduction in other forums is permitted, provided the original author(s) and the copyright owner(s) are credited and that the original publication in this journal is cited, in accordance with accepted academic practice. No use, distribution or reproduction is permitted which does not comply with these terms.
*Correspondence: Zhaoqi Liu, liuzq@big.ac.cn