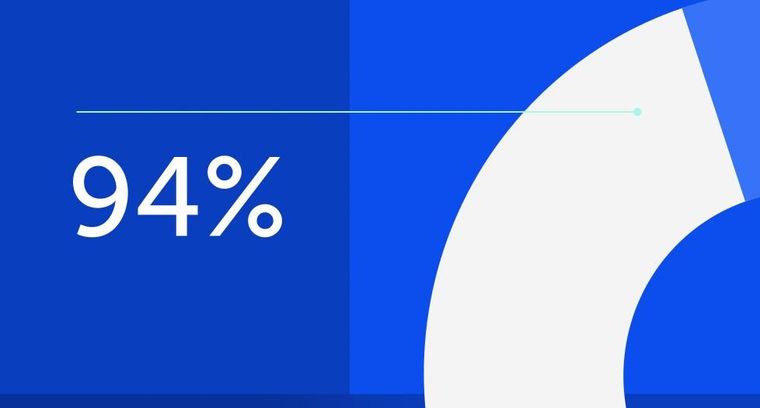
94% of researchers rate our articles as excellent or good
Learn more about the work of our research integrity team to safeguard the quality of each article we publish.
Find out more
ORIGINAL RESEARCH article
Front. Cell Dev. Biol., 13 June 2023
Sec. Cancer Cell Biology
Volume 11 - 2023 | https://doi.org/10.3389/fcell.2023.1193217
This article is part of the Research TopicAdvances and Mechanisms in Bone and Soft Tissue SarcomaView all 3 articles
Background: Bone giant cell tumor (BGCT) is one of the world’s major disease types of locally aggressive bone tumors. In recent years, denosumab treatment has been introduced before curettage surgery. However, the current therapeutic was practical only sometimes, given the local recurrence effects after discontinuation of denosumab. Due to the complex nature of BGCT, this study aims to use bioinformatics to identify potential genes and drugs associated with BGCT.
Methods: The genes that integrate BGCT and fracture healing were determined by text mining. The gene was obtained from the pubmed2ensembl website. We filtered out common genes for the function, and signal pathway enrichment analyses were implemented. The protein–protein interaction (PPI) networks and the hub genes were screened by MCODE built-in Cytoscape software. Lastly, the confirmed genes were queried in the Drug Gene Interaction Database to determine potential genes and drugs.
Results: Our study finally identified 123 common specific genes in bone giant cell tumors and fracture healing text mining concepts. The GO enrichment analysis finally analyzed 115 characteristic genes in BP, CC, and MF. We selected 10 KEGG pathways and identified 68 characteristic genes. We performed protein–protein interaction analysis (PPI) on 68 selected genes and finally identified seven central genes. In this study, these seven genes were substituted into drug–gene interactions, and there were 15 antineoplastic drugs, 1 anti-involving drug, and 1 anti-influenza drug.
Conclusion: The 7 genes (including ANGPT2, COL1A1, COL1A2, CTSK, FGFR1, NTRK2, and PDGFB) and 17 drugs, which have not been used in BGCT, but 6 of them approved by the FDA for other diseases, could be potential genes and drugs, respectively, to improve BGCT treatment. In addition, the correlation study and analysis of potential drugs through genes provide great opportunities to promote the repositioning of drugs and the study of pharmacology in the pharmaceutical industry.
Bone giant cell tumor (BGCT) is a locally aggressive benign bone tumor that is most commonly found in the distal femur and proximal tibia. BGCT accounts for 3%∼5% of all primary bone tumors. The peak onset of BGCT occurs between the ages of 30 and 40. Metastasis of BGCT is rarer than that of other malignancies (Raskin et al., 2013; Montgomery et al., 2019).
Surgery is the primary treatment for bone giant cell tumors. Surgical resection methods include intralesional curettage and motorized reaming or en bloc resection. Autologous and allogeneic cells, and bone cement filled the bone defect (Traub et al., 2016). Local recurrence rates after curettage were about 30% (Balke et al., 2008; Becker et al., 2008; Kivioja et al., 2008; Algawahmed et al., 2010). Surgery-related local adjuncts are currently used to reduce the rate of local recurrence: phenol, hydrogen peroxide, liquid nitrogen, regional chemotherapy, electrocoagulation, and heat generated by acrylic cement; however, no real benefit has been documented (Algawahmed et al., 2010).
Denosumab is the most classic systemic treatment drug for BGCT and has been approved by the FDA since 2013 for patients with unresectable tumors or those who have a score of 8 on the skeletal maturity index. BGCT contains two significant cell populations: reactive multinucleated osteoclast-like giant cells expressing nuclear factor KAPpa-B (RANK) receptor activator and neoplastic mononuclear stromal cells expressing the RANK ligand (RANKL). Denosumab is a complete human monoclonal antibody that inhibits the receptor activator of the nuclear factor kappa beta ligand; denosumab prevents osteolysis by inhibiting the recruitment of reactive osteoclast-like giant cells bytumor stromal cells (Thomas et al., 2010). Related meta-analysis showed that denosumab could reduce tumor mass and even decorticate the edge of the lesion to reduce pain and surgical morbidity. However, the preoperative use of denosumab remains controversial (Sano et al., 2020). According to a related systematic review, denosumab treatment may even be associated with an increase in the proportion of patients experiencing local recurrence (Tsukamoto et al., 2020).
It often takes about 10 years for a new drug to go from laboratory development to market. During this period, the investment and workforce required to conduct clinical trials are staggering, and the results of such investments are unpredictable. Therefore, searching for a new range of treatments for existing drugs may be another feasible and effective way to solve the problem of new drug discovery. Currently, increasing attention is being paid to text mining based on the medical and biological literature worldwide. In recent years, the utilization rate of FDA-approved vaccines and drugs has been as high as 30%, which also shows the great potential of text mining. Case in point: propranolol, a form of definitive treatment for coronary heart disease and high blood pressure, has recently been found for osteoporosis and melanoma.
The genes that integrate BGCT and fracture healing were determined by text mining. The gene was obtained on the pubmed2ensembl website. We filtered out common genes for the function and genes implemented in signal pathway enrichment analyses. The protein–protein interaction (PPI) networks and the hub genes were screened by MCODE built-in Cytoscape software. Lastly, the confirmed genes were queried in the Drug Gene Interaction Database to determine potential genes and drugs. Figure 1 shows the workflow of this study.
FIGURE 1. Overall data mining strategy. Text mining was used to identify genes associated with the concepts of BGCT and BF using pubmed2ensemble. Extracted genes were then analyzed for their function and gene ontology using DAVID. Further enrichment was obtained by molecular network analysis using STRING and Cytoscape. The final enriched gene list was then used to determine interactions with known drugs using the Drug Gene Interaction Database.
The web-based service pubmed2ensembl (http://pubmed2ensembl.ls.manchester.ac.uk/) was used to perform text mining. The link extension to the BioMart system links over 2,000,000 articles in PubMed to nearly 150,000 genes in Ensembl from 50 species (Baran et al., 2011). Users allow text-based queries to be performed against PubMed and PubMed Central documents in conjunction with constraints on genomic features. We performed two questions for producing two gene lists in the study, one with the idea of a bone giant cell tumor (BGCT) and the other with the concept of fracture healing (FH). We filtered common genes and then used them to proceed to the next steps.
The study used the DAVID database (http://david.ncifcrf.gov/summary.jsp) for a GO enrichment analysis of the shared genes of the BGCT and FH intersection, including biological process (BP), cellular component (CC), and molecular function (MF). The GO enrichment analysis selected all the top 8 genes with the lowest p-values. DAVID grouping of such identifiers improves the cross-reference capability, particularly across the NCBI and UniProt systems, enabling more than 40 publicly available functional annotation sources to be comprehensively integrated (Dennis et al., 2003).
Later, we centralized 10 genes with the lowest p-values by the DAVID Kyoto Encyclopedia of Genes and Genomes (KEGG) pathways that contained the involved genes. Characteristic genes shared by the GO enrichment and KEGG pathway analyses were selected and included in subsequent analyses (Pathan et al., 2017).
The study uses the Search Tool for the Retrieval of Interacting Genes (STRING) database (http://string-db.org), which is an open access database designed to evaluate the protein–protein interaction (PPI) messages of common genes. The STRING (version 10.5) database integrates text mining in PubMed and covers 9.6 million proteins originating from 2031 organisms (Szklarczyk et al., 2015). At first, we uploaded and mapped the list of the GO enrichment analysis and KEGG pathways involved in genes to the STRING website. Then, PPIs of the shared genes with a minimum required interaction score >0.9 (highest confidence) hid the disconnected nodes in the network. After that, Cytoscape software constructed PPI networks. The significant gene modules of the PPI networks were applied to pick out in Cytoscape with the Molecular Complex Detection (MCODE). The parameters were set as follows: the degree cutoff >2, K-scores >2, and node score cutoff >0.2. Finally, we selected the most significant gene modules from the PPI networks for further validation analyses.
To validate the findings of the bioinformatics analysis, lesion tissue from patients was harvested for qRT-PCR validation between the BGCT group (n = 6) and the control group (n = 6). The use of verbal consent was approved by the Southeast Hospital of Xiamen University, and verbal consent was obtained from each participant. Total RNA was extracted from tissue lesions using the TRIzol reagent (Yisheng, Shanghai, China). RNA samples were reverse transcribed to cDNA using the First Strand cDNA Synthesis Kit (Yisheng, China), according to the manufacturer’s instructions, with set conditions of 25°C/5 min, 42°C/30 min, and 85°C/5 min. After completion of reverse transcription, the system was diluted 10-fold because the reaction conditions included pre-denaturation at 95°C for 5 min, and cycling at 95°C/10 s and 60°C/30 s (40 cycles). The primers were validated by NABI blast and then synthesized by Xiamen BoRui Biotechnology Co. The relative expression of mRNA was calculated using the 2−ΔΔCT method. p< 0.05 was considered a statistically significant difference. The primer sequences are shown in Table 1.
QPCR-related genes include ANGPT2, COL1A1, COL1A2, CTSK, FGFR1, NTRK2, and PDGFB.
The final list of genes was used as the potential targets in a search for existing drugs or small organic compounds. The Drug–Gene Interaction Database (DGIdb, www.dgidb.org) is a web resource that consolidates disparate data sources describing drug–gene interactions and gene druggability (Wagner et al., 2016). It provides an intuitive graphical user interface and a documented application programming interface (API) for querying these data. The STITCH database (http://stitch.embl.de/) integrates these disparate data sources for 430,000 chemicals into a single, easy-to-use resource (Szklarczyk et al., 2016). In addition to the increased scope of the database, we have implemented a new network view that gives the user the ability to view binding affinities of chemicals in the interaction network. This enables the user to get a quick overview of the potential effects of the chemical on its interaction partners.
The study is based on the data on the strategy described in Figure 1. We excluded genes with identical names during text mining in pubmed2ensembl in this study. Finally, we determined that BGCT contained 204 genes, bone giant cell tumor 505, and we identified 123 unique genes related to BGCT and FH (Figure 2).
The study is based on the characteristics of the results from the analysis of the identified 123 unique genes by GO enrichment annotations in DAVID. The selection of the enriched biological process annotations resulted in six sets of annotations, which were 1) positive regulation of cell proliferation (p = 1.50E-34), 2) cell proliferation (p = 2.09E-32), 3) response to endogenous stimulus (p = 5.42E-32), 4) regulation of cell proliferation (p = 3.67E-31), 5) response to oxygen-containing compounds (p = 7.10E-31), and 6) response to organic substances (p = 1.89E-30) containing 53, 66, 62, 62, 61, and 77 genes from the query set, respectively (Table 2). Repairing fractures is one of the most complex biological processes that occur during human life. After the surgical removal of the bone giant-cell tumors, multiple biological pathways immediately become activated and are synchronized to respond. Hence, the relatively low p-value makes these biological process annotations relevant. The cellular component annotations revealed that most of the genes are expressed in the extracellular space, extracellular region part, and extracellular region, and other details are seen in Table 3. The analysis of molecular function annotations resulted in the selection of six pathways. Of the six, the most significant molecular function was receptor binding (p = 2.77; E-27), containing 60 genes (Table 4).
To more intuitively present GO enrichment annotations details, we conducted mapping with R statistical software (version 3.6.1, Figure 3). A total of 115 genes were screened in the GO enrichment analysis of BP, CC, and MF.
FIGURE 3. GO enrichment annotation analysis in the biological process (BP), cellular component (CC), and molecular function (MF) of those gene sets.
We selected 10 KEGG pathways with lower p-values with procedure KEGG pathways enrichment analysis, representing that they were strongly correlated with BGCT and FH (Table 5). The five most enriched biological KEGG pathways were 1) the PI3K-Akt signaling pathway (1.66E-18), 2) pathways in cancer (5.36E-17), 3) MAPK signaling pathway (1.38E-15), 4) proteoglycans in cancer (6.64E-13), and 5) AGE-RAGE signaling pathway in diabetic complications (1.22E-12), containing 32, 36, 27, 21, and 16 genes related to pathway enrichment analysis, respectively, and other highly enriched pathways including the relaxin signaling pathway, focal adhesion, rheumatoid arthritis, Chagas disease, and IL-17 signaling pathway. To bring the details to life, we used R statistics software (version 3.6) for mapping (Figure 4). A total of 68 associated genes were screened out in the 10 KEGG pathways, which will later be used in the protein–protein interaction analysis.
The Protein–protein interaction analysis (PPI) was performed using the STRING database. This study set the following parameters minimum required interaction score: highest confidence (0.900) and hidden nodes in the network. Network stats showed the following results: the number of nodes: 64, the number of edges: 2248, average node degree: 6.94, local clustering coefficient: 0.509, network centralization: 0.358, and PPI enrichment p-value: <1.0e-16 (Figure 5A). After that, Cytoscape software constructed PPI networks. The significant gene modules of the PPI networks were applied to pick out in Cytoscape with the Molecular Complex Detection (MCODE). The parameters were set as follows: the degree cutoff >2, K-scores >2, and node score cutoff >0.2. Finally, based on these criteria, we selected seven central genes, which formed the tightest module network, including ANGPT2, COL1A1, COL1A2, CTSK, FGFR1, NTRK2, and PDGFB (Figure 5B).
FIGURE 5. Protein–protein interaction (PPI) network. (A) Protein–protein interaction (PPI) network of the superposed genes between BGCT and BF. (B) Tightest module from the PPI network.
A qRT-PCR approach was used to detect the expression levels of seven potential genes. The verification result showed that the expression levels of NTRK2, FGFR1, PDGFB, COL1A1, and COL1A2 were significantly increased in gct samples (p < 0.05) (Figure 6), which confirmed the analytical signaling pathway results of bioinformatics were reliable in this study.
Finally, we conducted a drug–gene interaction analysis on the seven genes screened by the Module, and 144 drugs had specific effects on the aforementioned genes and pathways. The potential gene targets were ANGPT2 (4 drugs), COL1A1, COL1A2 (2 drugs each), CTSK (5 drugs), FGFR1 (85 drugs), NTRK2 (42 drugs), and PDGFB (4 drugs). In this study, we selected the drug with PMIDs, and the drug–gene interaction defined that it has been studied to some extent. A list of 17 drugs thought to potentially treat bone giant cell tumors is given in Table 6. There were 15 antineoplastic drugs, 1 antiosteoporosis drug, and 1 anti-influenza drug. The relationship between the genes and pathways corresponding to the 17 drugs is shown in Figure 7.
The classic treatment of bone giant cell tumors is still local curettage plus postoperative denosumab treatment. However, the current literature shows that the long-term use of denosumab may increase the risk of local recurrence and sometimes lead to side effects such as arthralgias, muscle pain, hypophosphatemia, and hypercalcemia (Luengo-Alonso et al., 2019). The elucidation of the pathophysiology of giant cell tumors of bone, particularly regarding the role of the nuclear factor κ B ligand (RANKL), led to the approval of denosumab by the US Food and Drug Administration (FDA) for the treatment of locally advanced or metastatic GCTB. The treatment paradigm has shifted from local to multidisciplinary treatment, considering denosumab in advanced giant cell tumors where surgical resection alone can lead to severe morbidity (Basu Mallick and Chawla, 2021). A growing number of studies have recently suggested that denosumab may increase the risk of local recurrence in patients undergoing curettage. It may be due to the thickening of the bone margin of the tumor, which intercepts tumor cells during scraping. After denosumab treatment, direct osteogenesis of marginal tumor cells also leads to local recurrence. In vitro studies have shown that denosumab produces a cytostatic response rather than an accurate cytotoxic response to tumor stromal cells (Li et al., 2020). In order to solve this problem, we use bioinformatics tools to identify existing potential fedinomab drugs for the treatment of bone giant cell tumors. Therefore, we identified 4 potential targeting genes and 13 drugs associated with bone giant cell tumors in Table 6.
Histologically, bone giant cell tumors consist of neoplastic spindle-shaped stromal cells, large multinucleated osteoclast-like cells, and their monocytic precursors expressing the corresponding receptor. Cathepsin K and its related transmembrane proton pump V-ATPase are significantly expressed in osteoclast giant cells, which are the main proteolytic enzymes in BGCT and participate in the degradation of bone collagen matrix in the metaphysis of BGCT (Lindeman et al., 2004). In addition, cathepsin K also plays a key role in bone homeostasis; low expression is related to bone resorption damage, and high expression is related to bone loss (Stroup et al., 2001). The mechanism of bone giant cell tumor at the pathological and genetic levels involves many factors. The fibroblast growth factor (FGF) is the key to tumor survival, migration, and tumor angiogenesis. It is overexpressed in a variety of cancers (glioblastoma, lung, gastric cancer, liver, endometrium, and urothelial carcinoma). Mutations of the fibroblast growth factor receptor type 1 (FGFR1) genes are one of the characteristic molecular changes of giant-cell-rich bone tumors (Hartmann et al., 2021). Hasenfratz et al. (2021) reported three cases of denosumab. After treatment, one of the BGCT patients with malignant H3F3A mutation developed to a pleomorphic sarcoma. The results of gene sequencing showed that the H3F3A mutation had been lost in the original bone giant cell tumor, but the FGFR1 mutation still existed. Related findings suggest that the H3F3A mutation analysis is a particular, although less sensitive, diagnostic tool for differentiating GCTB and chondroblastoma-forming tumors from other giant cell tumors (Cleven et al., 2015). In the World Health Organization Classification of Bone and Soft Tissue Tumors, NTRK fusions in sarcomas account for an increasing proportion (Gatalica et al., 2019). Neurotrophic tyrosine kinase 2 (NTRK 2) is a member of the family of receptor tyrosine kinases, which is mainly involved in the development of nerve tissue, differentiation, and metabolism. It has a high affinity for nerve growth factor receptor and can activate MAP kinase and the PIK3CA downstream pathway (Chen et al., 2021). PDGFB-COL1A1 gene fusion is considered to be related to giant-cell fibroblastoma, dermatofibrosarcoma protuberans, and other bone and soft tissue tumors (Li et al., 2018). Zhu and Qiu (1994) carried out a cytogenetic study on 19 cases of bone giant cell tumor and 4 cases of BGCT. It was found that the 22q12 locus was homologous to or adjacent to oncogene PDGF B (sis).
In our study, the drugs responsible for the inhibitor the FGFR1 gene corresponded to the largest number of drug categories (nasty 13). Although these drugs have not been applied for the treatment of BGCT, they have been approved by the FDA for the treatment of other diseases with excellent efficacy. Erdafitinib (JNJ-42756493), an oral pan-FGFR tyrosine kinase inhibitor, has been shown to have targeted regulatory effects on aberrant ligand-dependent FGFR signaling and activated cellular models of non-small cell lung, breast, bladder, endometrial, gastric, and colon cancer, as well as certain hematological malignancies in cytological experiments. In the mouse model of xenotransplantation of FGFR-related stomach, bladder, and squamous NSCLC tumors, erdafitinib also showed strong antitumor activity (Perera et al., 2017). In a multicenter, open-label phase I human trial, erdafitinib administered at 10 mg on a 7-day-on/7-day-off schedule was able to obtain clinical responses and controllable side effects. A phase I human trial in Japan also showed that erdafitinib was well tolerated in patients with a variety of advanced or refractory solid tumors (Nishina et al., 2018).
The 7 genes (including ANGPT2, COL1A1, COL1A2, CTSK, FGFR1, NTRK2, and PDGFB) and 17 drugs, which have not been used in BGCT, but 6 drugs approved by the FDA for other diseases, could be potential genes and drugs, respectively, to improve BGCT treatment. In addition, the correlation study and analysis of potential drugs through genes provide great opportunities to promote the repositioning of drugs and the study of pharmacology in the pharmaceutical industry.
The original contributions presented in the study are included in the article/supplementary material; further inquiries can be directed to the corresponding authors.
Conception and design: ZC; administrative support: MS and ZD; provision of study materials or patients: ZC; collection and assembly of data: ZC; data analysis and interpretation: ZC, CZ, HH, and WX. All authors contributed to the article and approved the submitted version.
This project was supported and funded by the Military logistics research program of China (CNJ16C013).
The authors declare that the research was conducted in the absence of any commercial or financial relationships that could be construed as a potential conflict of interest.
All claims expressed in this article are solely those of the authors and do not necessarily represent those of their affiliated organizations, or those of the publisher, the editors, and the reviewers. Any product that may be evaluated in this article, or claim that may be made by its manufacturer, is not guaranteed or endorsed by the publisher.
Algawahmed, H., Turcotte, R., Farrokhyar, F., and Ghert, M. (2010). High-speed burring with and without the use of surgical adjuvants in the intralesional management of giant cell tumor of bone: A systematic review and meta-analysis. Sarcoma 2010, 586090. doi:10.1155/2010/586090
Balke, M., Schremper, L., Gebert, C., Ahrens, H., Streitbuerger, A., Koehler, G., et al. (2008). Giant cell tumor of bone: Treatment and outcome of 214 cases. J. cancer Res. Clin. Oncol. 134, 969–978. doi:10.1007/s00432-008-0370-x
Baran, J., Gerner, M., Haeussler, M., Nenadic, G., and Bergman, C. M. (2011). pubmed2ensembl: a resource for mining the biological literature on genes. PLoS One 6, e24716. doi:10.1371/journal.pone.0024716
Basu Mallick, A., and Chawla, S. P. (2021). Giant cell tumor of bone: An update. Curr. Oncol. Rep. 23, 51. doi:10.1007/s11912-021-01047-5
Becker, W. T., Dohle, J., Bernd, L., Braun, A., and Cserhati, M. (2008). Local recurrence of giant cell tumor of bone after intralesional treatment with and without adjuvant therapy. J. bone Jt. Surg. Am. volume 90, 1060–1067. doi:10.2106/jbjs.d.02771
Chen, T., Wang, Y., Goetz, L., Corey, Z., Dougher, M. C., Smith, J. D., et al. (2021). Novel fusion sarcomas including targetable NTRK and ALK. Ann. diagnostic pathology 54, 151800. doi:10.1016/j.anndiagpath.2021.151800
Cleven, A. H. G., Höcker, S., Briaire-de Bruijn, I., Szuhai, K., Cleton-Jansen, A. M., and Bovée, J. V. M. G. (2015). Mutation analysis of H3F3A and H3F3B as a diagnostic tool for giant cell tumor of bone and chondroblastoma. Am. J. Surg. Pathol. 39, 1576–1583. doi:10.1097/PAS.0000000000000512
Dennis, G., Sherman, B. T., Hosack, D. A., Yang, J., Gao, W., Lane, H. C., et al. (2003). David: Database for annotation, visualization, and integrated discovery. Genome Biol. 4, P3. doi:10.1186/gb-2003-4-5-p3
Gatalica, Z., Xiu, J., Swensen, J., and Vranic, S. (2019). Molecular characterization of cancers with NTRK gene fusions. Mod. Pathol. 32, 147–153. doi:10.1038/s41379-018-0118-3
Hartmann, W., Harder, D., and Baumhoer, D. (2021). Giant cell-rich tumors of bone. Surg. Pathol. Clin. 14, 695–706. doi:10.1016/j.path.2021.06.010
Hasenfratz, M., Mellert, K., Marienfeld, R., von Baer, A., Schultheiss, M., Roitman, P. D., et al. (2021). Profiling of three H3F3A-mutated and denosumab-treated giant cell tumors of bone points to diverging pathways during progression and malignant transformation. Sci. Rep. 11, 5709. doi:10.1038/s41598-021-85319-x
Kivioja, A. H., Blomqvist, C., Hietaniemi, K., Trovik, C., Walloe, A., Bauer, H. C. F., et al. (2008). Cement is recommended in intralesional surgery of giant cell tumors: A scandinavian sarcoma group study of 294 patients followed for a median time of 5 years. Acta Orthop. 79, 86–93. doi:10.1080/17453670710014815
Li, H., Gao, J., Gao, Y., Lin, N., Zheng, M., and Ye, Z. (2020). Denosumab in giant cell tumor of bone: Current status and pitfalls. Front. Oncol. 10, 580605. doi:10.3389/fonc.2020.580605
Li, N., Zhou, T., Chen, S., Yang, R., Zhu, Q., and Feng, Z. (2018). COL1A1-PDGFB gene fusion in dermatofibrosarcoma protuberans: A useful diagnostic tool and clinicopathological analysis. Int. J. Clin. Exp. pathology 11, 4052–4059.
Lindeman, J. H., Hanemaaijer, R., Mulder, A., Dijkstra, P. D. S., Szuhai, K., Bromme, D., et al. (2004). Cathepsin K is the principal protease in giant cell tumor of bone. Am. J. pathology 165, 593–600. doi:10.1016/s0002-9440(10)63323-8
Luengo-Alonso, G., Mellado-Romero, M., Shemesh, S., Ramos-Pascua, L., and Pretell-Mazzini, J. (2019). Denosumab treatment for giant-cell tumor of bone: A systematic review of the literature. Archives Orthop. trauma Surg. 139, 1339–1349. doi:10.1007/s00402-019-03167-x
Montgomery, C., Couch, C., Emory, C. L., and Nicholas, R. (2019). Giant cell tumor of bone: Review of current literature, evaluation, and treatment options. J. knee Surg. 32, 331–336. doi:10.1055/s-0038-1675815
Nishina, T., Takahashi, S., Iwasawa, R., Noguchi, H., Aoki, M., and Doi, T. (2018). Safety, pharmacokinetic, and pharmacodynamics of erdafitinib, a pan-fibroblast growth factor receptor (FGFR) tyrosine kinase inhibitor, in patients with advanced or refractory solid tumors. Investig. new drugs 36, 424–434. doi:10.1007/s10637-017-0514-4
Pathan, M., Keerthikumar, S., Chisanga, D., Alessandro, R., Ang, C. S., Askenase, P., et al. (2017). A novel community driven software for functional enrichment analysis of extracellular vesicles data. J. Extracell. Vesicles 6, 1321455. doi:10.1080/20013078.2017.1321455
Perera, T. P. S., Jovcheva, E., Mevellec, L., Vialard, J., De Lange, D., Verhulst, T., et al. (2017). Discovery and pharmacological characterization of JNJ-42756493 (erdafitinib), a functionally selective small-molecule FGFR family inhibitor. Mol. cancer Ther. 16, 1010–1020. doi:10.1158/1535-7163.mct-16-0589
Raskin, K. A., Schwab, J. H., Mankin, H. J., and Hornicek, F. J. (2013). Giant cell tumor of bone. J. Am. Acad. Orthop. Surg. 21, 118–126. doi:10.5435/jaaos-21-02-118
Sano, K., Suehara, Y., Okubo, T., Sasa, K., Kurihara, T., Akaike, K., et al. (2020). Preoperative denosumab treatment with curettage may be a risk factor for recurrence of giant cell tumor of bone. J. Orthop. Surg. (Hong Kong) 28, 2309499020929786. doi:10.1177/2309499020929786
Stroup, G. B., Lark, M. W., Veber, D. F., Bhattacharyya, A., Blake, S., Dare, L. C., et al. (2001). Potent and selective inhibition of human cathepsin K leads to inhibition of bone resorption in vivo in a nonhuman primate. J. bone mineral Res. 16, 1739–1746. doi:10.1359/jbmr.2001.16.10.1739
Szklarczyk, D., Franceschini, A., Wyder, S., Forslund, K., Heller, D., Huerta-Cepas, J., et al. (2015). STRING v10: Protein-protein interaction networks, integrated over the tree of life. Nucleic Acids Res. 43, D447–D452. doi:10.1093/nar/gku1003
Szklarczyk, D., Santos, A., von Mering, C., Jensen, L. J., Bork, P., and Kuhn, M. (2016). Stitch 5: Augmenting protein-chemical interaction networks with tissue and affinity data. Nucleic Acids Res. 44, D380–D384. doi:10.1093/nar/gkv1277
Thomas, D., Henshaw, R., Skubitz, K., Chawla, S., Staddon, A., Blay, J. Y., et al. (2010). Denosumab in patients with giant-cell tumour of bone: An open-label, phase 2 study. Lancet. Oncol. 11, 275–280. doi:10.1016/s1470-2045(10)70010-3
Traub, F., Singh, J., Dickson, B. C., Leung, S., Mohankumar, R., Blackstein, M. E., et al. (2016). Efficacy of denosumab in joint preservation for patients with giant cell tumour of the bone. Eur. J. cancer 59, 1–12. doi:10.1016/j.ejca.2016.01.006
Tsukamoto, S., Tanaka, Y., Mavrogenis, A. F., Kido, A., Kawaguchi, M., and Errani, C. (2020). Is treatment with denosumab associated with local recurrence in patients with giant cell tumor of bone treated with curettage? A systematic review. Clin. Orthop. Relat. Res. 478, 1076–1085. doi:10.1097/corr.0000000000001074
Wagner, A. H., Coffman, A. C., Ainscough, B. J., Spies, N. C., Skidmore, Z. L., Campbell, K. M., et al. (2016). DGIdb 2.0: Mining clinically relevant drug-gene interactions. Nucleic Acids Res. 44, D1036–D1044. doi:10.1093/nar/gkv1165
Keywords: bone giant cell tumor, fracture healing, potential genes, potential drugs, drug treatment
Citation: Chen Z, Zhang C, Hong H, Xu W, Sha M and Ding Z (2023) Potential alternative drug treatment for bone giant cell tumor. Front. Cell Dev. Biol. 11:1193217. doi: 10.3389/fcell.2023.1193217
Received: 24 March 2023; Accepted: 30 May 2023;
Published: 13 June 2023.
Edited by:
Zhichang Zhang, Shanghai No.6 People’s Hospital, ChinaReviewed by:
Kai Zheng, 960th Hospital of the PLA, ChinaCopyright © 2023 Chen, Zhang, Hong, Xu, Sha and Ding. This is an open-access article distributed under the terms of the Creative Commons Attribution License (CC BY). The use, distribution or reproduction in other forums is permitted, provided the original author(s) and the copyright owner(s) are credited and that the original publication in this journal is cited, in accordance with accepted academic practice. No use, distribution or reproduction is permitted which does not comply with these terms.
*Correspondence: Mo Sha, c2hhbW81QDE2My5jb20=; Zhenqi Ding, ZHpxaUB4bXUuZWR1LmNu
†These authors have contributed equally to this work and share first authorship
Disclaimer: All claims expressed in this article are solely those of the authors and do not necessarily represent those of their affiliated organizations, or those of the publisher, the editors and the reviewers. Any product that may be evaluated in this article or claim that may be made by its manufacturer is not guaranteed or endorsed by the publisher.
Research integrity at Frontiers
Learn more about the work of our research integrity team to safeguard the quality of each article we publish.