- 1Laboratorio de Ciencia de Datos Biomédicos, Escuela de Medicina, Facultad de Ciencias Médicas de la Salud y de la Vida, Universidad Internacional del Ecuador, Quito, Ecuador
- 2Latin American Network for the Implementation and Validation of Clinical Pharmacogenomics Guidelines (RELIVAF-CYTED), Madrid, Spain
- 3Facultad de Ingenierías y Ciencias Aplicadas, Universidad Internacional SEK, Quito, Ecuador
- 4Instituto Nacional de Investigación en Salud Pública, Quito, Ecuador
- 5Medical Oncology Department Hospital Clinic and Translational Genomics and Targeted Therapies in Solid Tumors, IDIBAPS, Barcelona, Spain
- 6Cancer Research Group (CRG), Faculty of Medicine, Universidad de Las Américas, Quito, Ecuador
Colorectal adenocarcinoma (COREAD) is the second most deadly cancer and third most frequently encountered malignancy worldwide. Despite efforts in molecular subtyping and subsequent personalized COREAD treatments, multidisciplinary evidence suggests separating COREAD into colon cancer (COAD) and rectal cancer (READ). This new perspective could improve diagnosis and treatment of both carcinomas. RNA-binding proteins (RBPs), as critical regulators of every hallmark of cancer, could fulfill the need to identify sensitive biomarkers for COAD and READ separately. To detect new RBPs involved in COAD and READ progression, here we used a multidata integration strategy to prioritize tumorigenic RBPs. We analyzed and integrated 1) RBPs genomic and transcriptomic alterations from 488 COAD and 155 READ patients, 2) ∼ 10,000 raw associations between RBPs and cancer genes, 3) ∼ 15,000 immunostainings, and 4) loss-of-function screens performed in 102 COREAD cell lines. Thus, we unraveled new putative roles of NOP56, RBM12, NAT10, FKBP1A, EMG1, and CSE1L in COAD and READ progression. Interestingly, FKBP1A and EMG1 have never been related with any of these carcinomas but presented tumorigenic features in other cancer types. Subsequent survival analyses highlighted the clinical relevance of FKBP1A, NOP56, and NAT10 mRNA expression to predict poor prognosis in COREAD and COAD patients. Further research should be performed to validate their clinical potential and to elucidate their molecular mechanisms underlying these malignancies.
Introduction
Colorectal adenocarcinoma (COREAD) has been ranked as the second most deadly cancer and the third most common malignancy worldwide with an estimated 1.9 million cases and 0.9 million deaths in 2020 (Xi and Xu, 2021). Over the past 10 years, significant advances were achieved in personalized treatments for COREAD patients based on the molecular subtyping (Cohen et al., 2020; López-Cortés et al., 2020; Assis et al., 2022). For example, metastatic COREAD patients, harboring BRAFV600E mutation, have now better treatment options (Mauri et al., 2021). Despite these efforts, molecular subtyping has been insufficient to address the heterogeneity of colon and rectal tumors (Cohen et al., 2020; Liu Z. et al., 2021; Assis et al., 2022). In that context, Paschke et al., after analyzing ∼2000 publications and the results of two large clinical trials, suggested stopping using the term COREAD and started separating into two different tumor identities: colon cancer (COAD) and rectal cancer (READ). Paschke et al., reached this conclusion by describing obvious differences between COAD and READ concerning molecular carcinogenesis, pathology, surgical topography and procedures, and multimodal treatment. This new perspective could improve the identification of new biomarkers and therapeutic targets for both types of cancer (Paschke et al., 2018).
A new emerging understanding of RNA-binding proteins (RBPs) have addressed them as critical modulators of every hallmark of cancer (Abdel-Wahab and Gebauer, 2018; García-Cárdenas et al., 2019; Hanahan, 2022). RBPs can modulate the expression levels of oncogenes and tumor suppressors (Hentze et al., 2018; García-Cárdenas et al., 2019; Kang et al., 2020) by controlling all aspects of their mRNA processing and metabolism, such as capping, polyadenylation, alternative splicing, subcellular localization, nucleocytoplasmic transport, stability, and degradation (Hentze et al., 2018; García-Cárdenas et al., 2019; Kang et al., 2020; Mestre-Farràs et al., 2022). Thus, identification of tumorigenic RBPs could fulfill the need to discover more accurate and sensitive therapeutic targets for COAD and READ (Cohen et al., 2020; Liu Z. et al., 2021; Assis et al., 2022).
In that respect, we previously performed a literature review to identify RBPs implicated in COREAD (García-Cárdenas et al., 2019). As a result, we found 35 RBPs (out of 1,392 described by Hentze et al., 2018) involved in different aspects of COREAD progression, such as angiogenesis, metastasis, or chemotherapy resistance (Hentze et al., 2018). We also showed that these RBPs are implicated in a complex interconnected network where a single RBP can bind to thousands of RNAs. For instance, ELAVL1 targets 21,578 RNAs, whereas KHDRBS1 interacts with 962. These results pointed out the potential of RBPs to regulate cancerous cellular processes and thereby to be used as COAD or READ biomarkers.
In extending the scope of our previous work and discovering new RBPs involved in COAD and READ separately, here we used our previously published multidata integration strategy to prioritized tumorigenic RBPs (García-Cárdenas et al., 2022). Thus, we assembled data from several resources: The Cancer Genome Atlas (Tomczak et al., 2015), The Human Protein Atlas (Pontén et al., 2008), STRING (Szklarczyk et al., 2019), Depmap (Yu et al., 2016) and HumanNet (Kim et al., 2022), and revealed new RBPs associated with both types of cancer. Our results provide a better understanding of COAD and READ biology and potentially unveil new targets for cancer therapy and prognostic biomarkers.
Methods
Gene sets
Hentze et al., compiled all published RNA interactomes and stringently curated a list of 1,393 RBPs (Hentze et al., 2018). After checking for new annotations using Ensembl (http://www.ensembl.org), we found one duplicate (ENSG00000100101 and ENSG00000273899, both corresponded to NOL12), leaving a final list of 1,392 RBPs. The cancer driver genes (n = 2,372) were retrieved from the Network of Cancer Genes 7.0 (NCG7, http://ncg.kcl.ac.uk/) (Dressler et al., 2022) and filtered by COREAD genes (n = 156) (Supplementary Table S1).
Genomic and transcriptomic data exploration
The cBioPortal for Cancer Genomics (https://www.cbioportal.org; accessed on 04 March 2022) was used to analyze and retrieve genomic and transcriptomic alterations of RBPs from datasets that clinically differentiate COAD and READ. Specifically, we used the Colorectal Adenocarcinoma dataset (TCGA, PanCancer Atlas; Hoadley et al., 2018) which has 378 COAD patients and 155 READ patients. We also analyzed the Colon Cancer study (CPTAC-2 Prospective; Vasaikar et al., 2019) which has 110 complete COAD samples. To compare the aforementioned gene sets, genomic and transcriptomic alterations were corrected by the number of patients. As COAD and READ sets have different number of patients, we divided the number of alterations per RBP by number of patients, i.e., the mean of genomic and transcriptomic alterations per RBP. A Mann–Whitney U test was applied when comparing clinical characteristics or genomic and transcriptomic alterations between gene sets (colon vs. rectum) and within each group (COAD and READ stages and subtypes) (Supplementary Tables S2–S7). Additionally, mRNA Z-scores of aberrantly expressed RBPs in COAD and READ were collected and compared using a Mann–Whitney U test (Supplementary Tables S8, S9). A z-score of < −2 or >2 (p-value = <0.05; confidence level 95%) was used as the criteria for RBPs being determined as down/upregulated, respectively.
Gene network construction
Experimental and database interactions between RBPs (n = 1,392) and COREAD proteins (n = 156) (Supplementary Table S10), having an interaction score of 0.9 (highest confidence), were predicted with the STRING database (Szklarczyk et al., 2015; Repana et al., 2019). Then, the network was visualized using the Cytoscape 3.9.1 (Seattle, USA) platform (Shannon et al., 2003).
Protein expression analysis
Protein immunohistochemical levels were extracted from The Human Protein Atlas version 21.1 (https://www.proteinatlas.org; accessed on 15 March 2022) (Uhlén et al., 2015; Thul et al., 2017; Uhlen et al., 2017). We obtained protein expression levels (not detected, low, medium, and high) for 608 RBPs in COAD and 609 RBPs in READ tissues. Protein expression levels of normal tissues were taken from glandular cells, while a consensus level was manually generated for COAD and READ tissues (Supplementary Tables S11, S12) based on the expression levels. Both normal and tumor tissues had antibody validation parameters. Only enhanced and supported parameters were considered for this analysis.
Cancer genetic dependency analysis
RBPs cancer dependency scores from CERES (Meyers et al., 2017) (1,341 available RBPs) and DEMETER2 (Tsherniak et al., 2017; McFarland et al., 2018) (1,255 available RBPs) were obtained from the Dependency Map (DepMap) portal (https://depmap.org/portal) (Yu et al., 2016). A score of 0 = not essential gene for cell survival, whereas a score of −1 corresponds to the median of all common essential genes, i.e., genes whose principal cellular processes are involved in fundamental cell survival pathways. These scores were calculated from gene knock-out (CERES) and knock-down (Demeter) experiments performed in cancer cell lines. CERES reported data from 42 COAD cell lines and three READ cell lines, while DEMETER2 obtained data from 47 COAD cell lines and 10 READ cell lines (Supplementary Tables S13–S16).
Integrative gene network
The prioritized RBPs for COAD and READ were integrated into a disease gene network by using the HumanNet XC (functional gene network extended network by co-citation) latest version (v3 software) (https://www.inetbio.org/humannet) (Kim et al., 2022) and visualized through Cytoscape 3.9.1 (Shannon et al., 2003) (Supplementary Tables S17, S18).
Clinical analysis
The TCGA, PanCancer Atlas (Hoadley et al., 2018) colorectal cancer database was inspected for mRNA expression of prioritized RBPs in colon and rectum patients. Probability of overall survival (OS) and disease-free survival (DFS) were calculated. Curves were obtained by dividing samples in two groups using median z-score as a cutoff in COREAD, COAD, and READ patients. These two groups represent 1) patients with RBP mRNA upregulation (blue lines) and 2) patients with RBP mRNA downregulation (red lines). Differences between groups were calculated using log rank test. Graphical representations and statistical analysis were performed with IBM SPSS, version 22. Only significant comparisons with an N > 20 per group is shown.
Results
Multidata integration strategy to prioritized tumorigenic RNA-binding proteins
We previously published a multidata integration strategy that allowed us to identify PUF60 and SF3A3 as new spliceosome-related breast cancer RBPs (García-Cárdenas et al., 2022). In this work, we used the same strategy to prioritize tumorigenic RBPs that could be used as COAD or READ biomarkers. First, we performed individual analysis of several databases such as The Cancer Genome Atlas (Tomczak et al., 2015), The Human Protein Atlas (Pontén et al., 2008), STRING (Szklarczyk et al., 2019), and Depmap (Yu et al., 2016) to identify RBPs with different cancer-related characteristics: 1) high genomic and transcriptomic alterations, 2) interactions with well-known cancer proteins, 3) aberrant protein expression levels compared with normal tissues, and 4) essential for tumor survival. Next, we performed a rigorous analysis to detect RBPs with all the aforementioned attributes, and thereby prioritizing potential COAD and READ RBPs. Finally, we predicted how these prioritized RBPs are correlated with cancer phenotypes using the HumanNet (Kim et al., 2022) database (Figure 1).
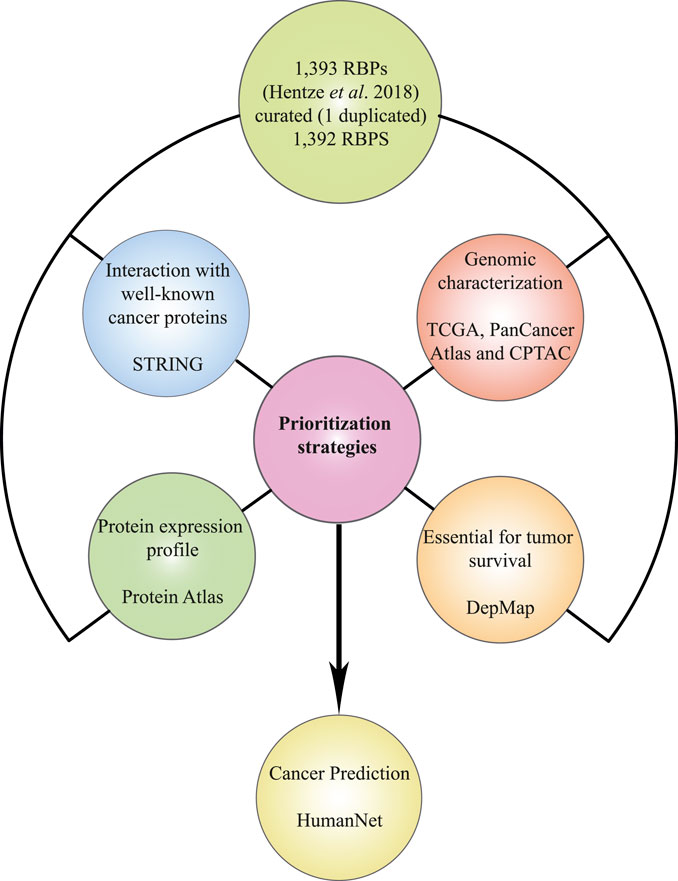
FIGURE 1. Schematic representation of the data mining strategy. All databases interrogated for prioritization: The Cancer Genome Atlas (Tomczak et al., 2015), The Human Protein Atlas (Pontén et al., 2008), STRING (Szklarczyk et al., 2019), and DepMap (Yu et al., 2016), and further cancer association analysis (HumanNet; Kim et al., 2022) are depicted in the multidata integration workflow.
Detection of highly altered RNA-binding proteins in colon and rectal tumors
To globally determine the genomic and transcriptomic alterations of RBPs in COAD and READ patients, we interrogated two independent datasets, TCGA, PanCancer Atlas (Hoadley et al., 2018) and Colon Cancer study CPTAC-2 Prospective (Vasaikar et al., 2019) including in toto 488 COAD and 155 READ patients. First, we compared RBPs (n = 1,392) genomic and transcriptomic alterations between COAD and READ. Once we corrected by the number of patients (i.e., mean of genomic and transcriptomic alterations per RBP), we found significant differences (p < 0.001) between COAD and READ. In Figure 2A is depicted the percentages of each alteration, where mRNA upregulation accounted for most of the genomic and transcriptomic modifications in COAD and READ. In colon tumors we found that mRNA downregulation and mutations presented equal percentages (15%), whereas mRNA downregulation (20%) occupied the second place followed by mutations (11%) in READ.
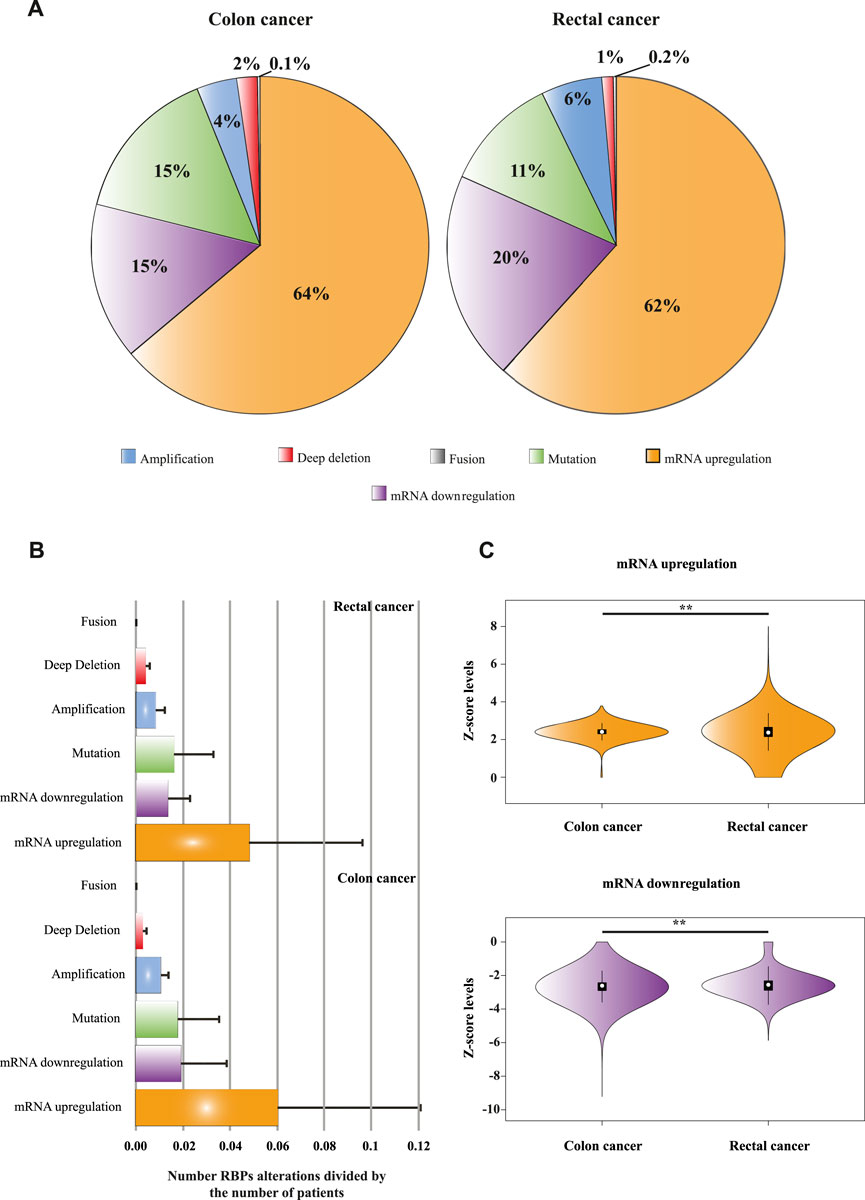
FIGURE 2. Genome and transcriptome alterations of RNA Binding Proteins. (A) A pie chart illustrating different types of RBPs genomic and transcriptomic alterations and their percentages in colon and rectal tumors. Data was obtained from Colorectal Adenocarcinoma study (TCGA, PanCancer Atlas; Hoadley et al., 2018) and Colon Cancer study (CPTAC-2 Prospective; Vasaikar et al., 2019). (B) Number of RBPs alterations (corrected by number of patients, arbitrary units) separated by type. A Mann–Whitney U test was performed to compare alterations between datasets (COAD vs. READ alterations). All possible comparisons between sets presented significant statistically differences (p < 0.001) except mutations and fusions; ns = not significant. (C) Violin plots portraying the differences of mRNA levels (Z-scores) between colon and rectal tumors, a Mann–Whitney U test was performed to compare these data sets. ** = high statistically significant difference.
Although the pattern of RBPs genomic and transcriptomic alterations in COAD and READ are similar (Figure 2A), when they are analyzed separately, significant differences were found (p < 0.001) except for mutations and fusions in COAD vs. READ (Figure 2B; Supplementary Tables S2, S3). Even though RBPs mRNA upregulations account for 64% in COAD vs. 62% in READ, mRNA Z-scores were higher in READ than in COAD (p < 0.001) (Figure 2C; Supplementary Tables S8, S9). The contrary is appreciated when analyzing mRNA downregulation, COAD RBPs z-scores distribution shows a wider mRNA downregulation compared to READ RBPs (p < 0.001) (Figure 2C).
To identify RBPs involved in tumor progression or suppression, RBPs genomic and transcriptomic alterations categories were classified accordingly. In malignant cells, mRNA upregulation and genomic amplifications are related to tumor progressors, while mRNA downregulation and genomic deletions are connected with suppressors (Wurth et al., 2016; Mestre-Farràs et al., 2022). Gene fusion and mutations have been detected in both tumor progressors and suppressors. Based on these principles, we listed the most frequently altered RBPs in COAD and READ (Table 1, Supplementary Tables S2, S3). As expected, most of them have already been related to COAD or READ, and thereby validating our strategy (Table 1). Interestingly, STAU1 was the most altered RBP in both carcinomas, and yet it has never been correlated with COAD or READ; however, it has been associated with prostate cancer (Marcellus et al., 2021). Similarly, some progressors (CHMP4B, CSTF1, and LSM14B) and suppressors RBPs (ATP5F1A, GTF2E2, RTF1, ELAC2, LRRC47, and MRM3) have never been associated with COAD or READ, but present oncogenic properties in other cancer types (Table 1, Supplementary Tables S2, S3). Worthy of note, we also identified RBPs that are unique for each cancer type and others (e.g., CSTF1) that have been never related to cancer (Table 1).
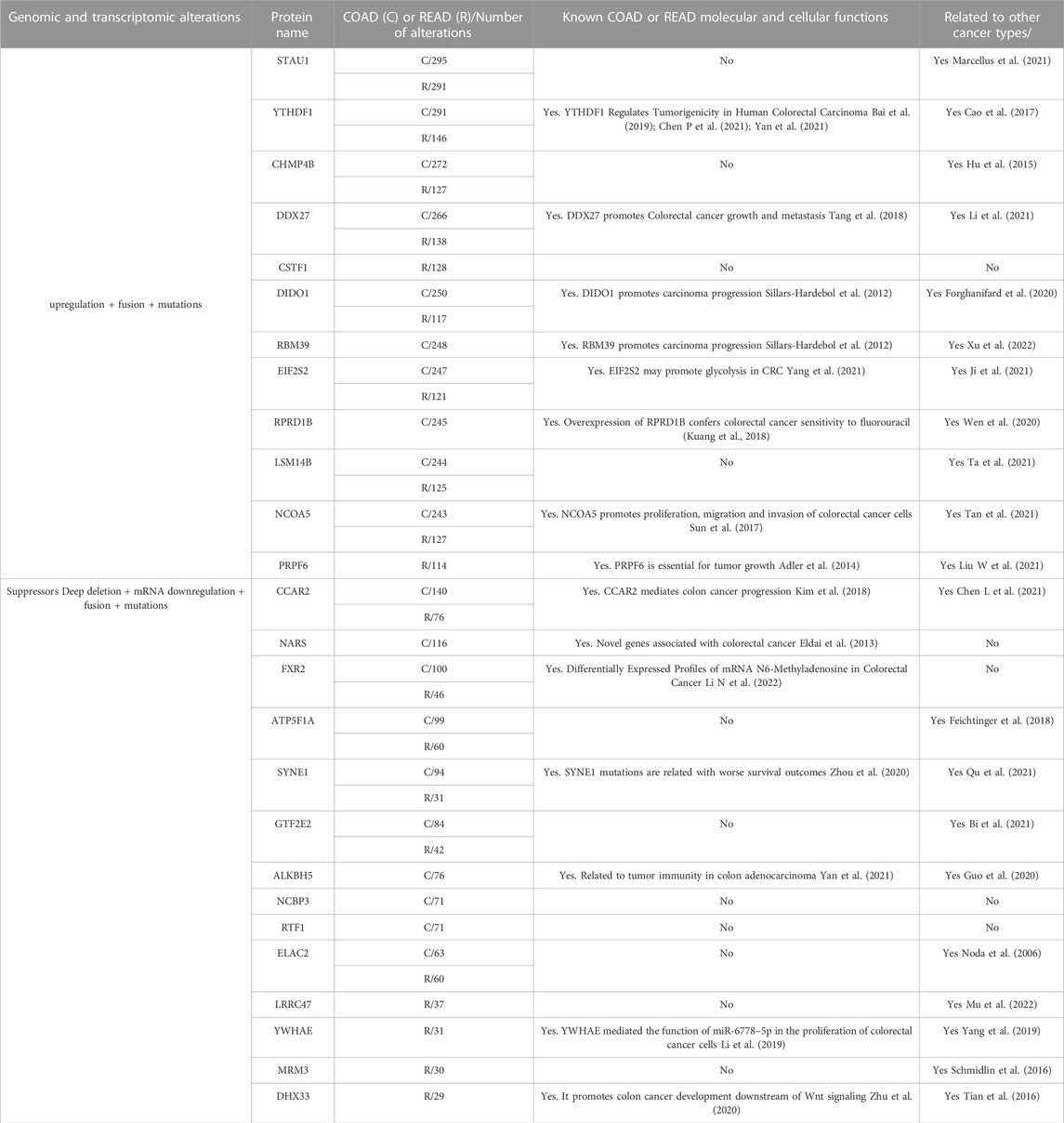
TABLE 1. Most frequently altered RNA-binding proteins in colon and rectal cancer. Data was obtained from Colorectal Adenocarcinoma study (TCGA, PanCancer Atlas; Hoadley et al., 2018) and Colon Cancer study (CPTAC-2 Prospective; Vasaikar et al., 2019).
We, next, determined genomic and transcriptomic alterations of RBPs by subtypes, pole (polymerase ε), microsatellite instability (MSI), genomically stable (GS), and chromosomal instability (CIN) (Figures 3A,B; Table 2; Supplementary Tables S4, S5) and stages (Stage I to IV, Table 2; Figures 3C,D; Supplementary Tables S6, S7). We found statistically significant differences between all subtypes in both carcinomas (Mann–Whitney U, p < 0.001; Figures 3A,B). Pole was the most altered subtype, followed by MSI, CIN, and GS in both malignancies (Figures 3A,B). When comparing subtypes between COAD and READ, we also found statistically significant differences except for COAD GS vs. READ GS subtype (Mann–Whitney U, p < 0.001; Figures 3A,B). In COAD, we also observed equally altered RBPs among subtypes. For example, DST and SYNE1 were highly altered in Pole and MSI, while DDX27, STAU1, and YTHDF1 in GS and CIN. It is important to mention that some of these RBPs were subtype specific such as SYNE2 and UTP20 for Pole, and ANK3 for MSI (Figure 3A). Similarly, we found subtype specific RBPs within READ subtypes that have never related to COREAD. For instance, DYNC2H1, DDX55, CPSF7, CAND1 and LARP4 were found in MSI subtype, TSR1 in Pole, ZCCHC3 in GS, and LSM14B in CIN (Figure 3B).
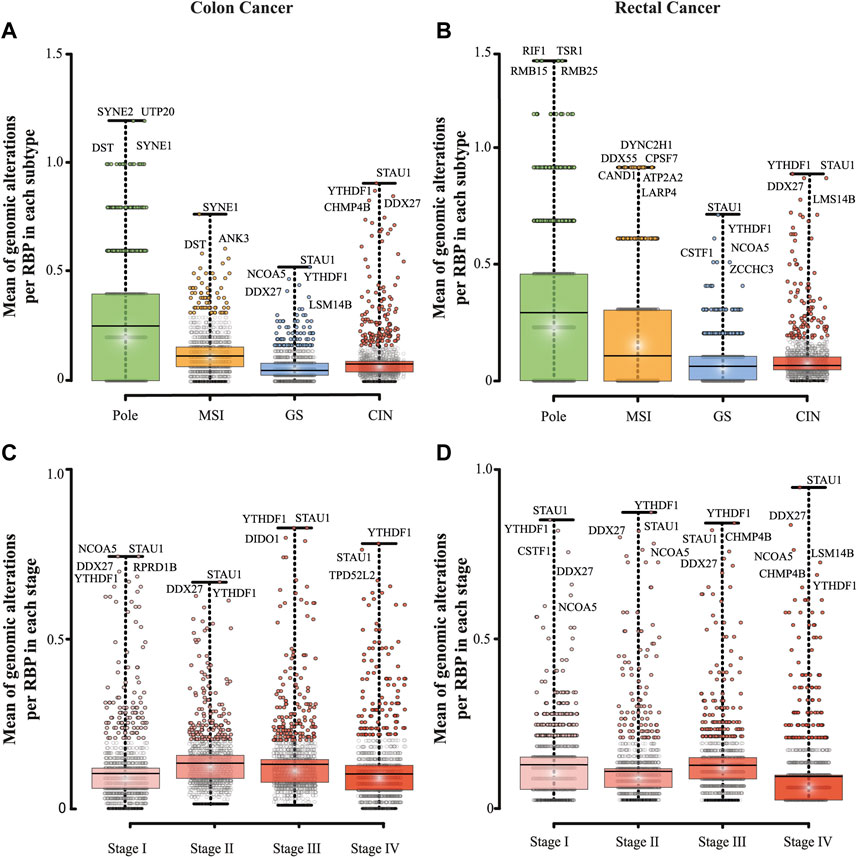
FIGURE 3. Detection of highly altered RNA-binding proteins in colon and rectal carcinomas. RBPs genomic and transcriptomic alterations per subtype (A, B) and stage (C, D) are depicted in boxplots. Data was obtained from Colorectal Adenocarcinoma study (TCGA, PanCancer Atlas; Hoadley et al., 2018) and Colon Cancer study (CPTAC-2 Prospective; Vasaikar et al., 2019). The number of RBPs alterations was divided by the number of patients in each set of data. A Mann–Whitney U test was performed to compare genomic and transcriptomic alterations between sets. All possible comparisons between colon and rectum and within each data set in both carcinomas presented high statistically significant difference (p < 0.001), except for GS subtype when comparing colon vs. rectum, stage 1 vs. stage 4 (in colon cancer), and stage 1 vs. 3 (in rectal cancer); ns = not significant.
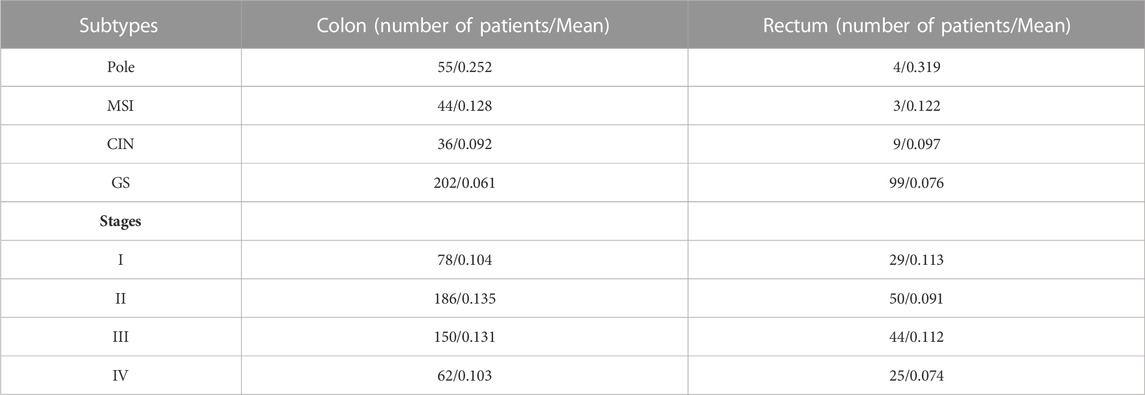
TABLE 2. Mean of genomic and transcriptomic alterations per stage and subtypes and sample number in COAD and READ patients.
We also detected statistically significant differences between all stages in both cancer types (Mann–Whitney U, p < 0.001; Figures 3C,D). In COAD the highest mean of RBPs genomic and transcriptomic alterations were detected in stage II, while in READ they were found in stage I (Figures 3C,D; Table 2). Interestingly, stage IV showed the lowest number of RBPs alterations in both cancers. Despite these differences, STAU1 and YTHDF1 were constantly altered in all stages. This was also observed in GS and CIN subtypes (Figures 3A,B; Table 2). Contrary to STAU1, YTHDF1 has previously been associated with COREAD development (Bai et al., 2019; Chen P. et al., 2021; Yan et al., 2021), showing the potential of STAU1 to be involved in COREAD progression too.
Networking analysis of RNA-Binding proteins vs. colorectal drivers
Protein-protein interaction (PPIs) networks have been proved to be effective in detecting tumorigenic RNA regulons (Wurth et al., 2016; Indacochea et al., 2021). Thus, we next interrogated the STRING database (Szklarczyk et al., 2019) to understand the relationship between RBPs (n = 1,392) and COREAD drivers (n = 139) (Repana et al., 2019) and outline key interactions among them. We identified 37 COREAD proteins interacting with 153 RBPs (Figure 4; Supplementary Table S10). The interactions were obtained from experiments and databases using a highest confidence threshold (interaction score = 0.9). As shown in Figure 4, we detected two main interaction networks around TCERG1 and NAT10. Interestingly, these proteins not only have the ability to bind RNA but also, they were catalogued as COREAD drivers. Interestingly, TCERG1 binds to CDCL5 which in turn binds to five COREAD drivers.
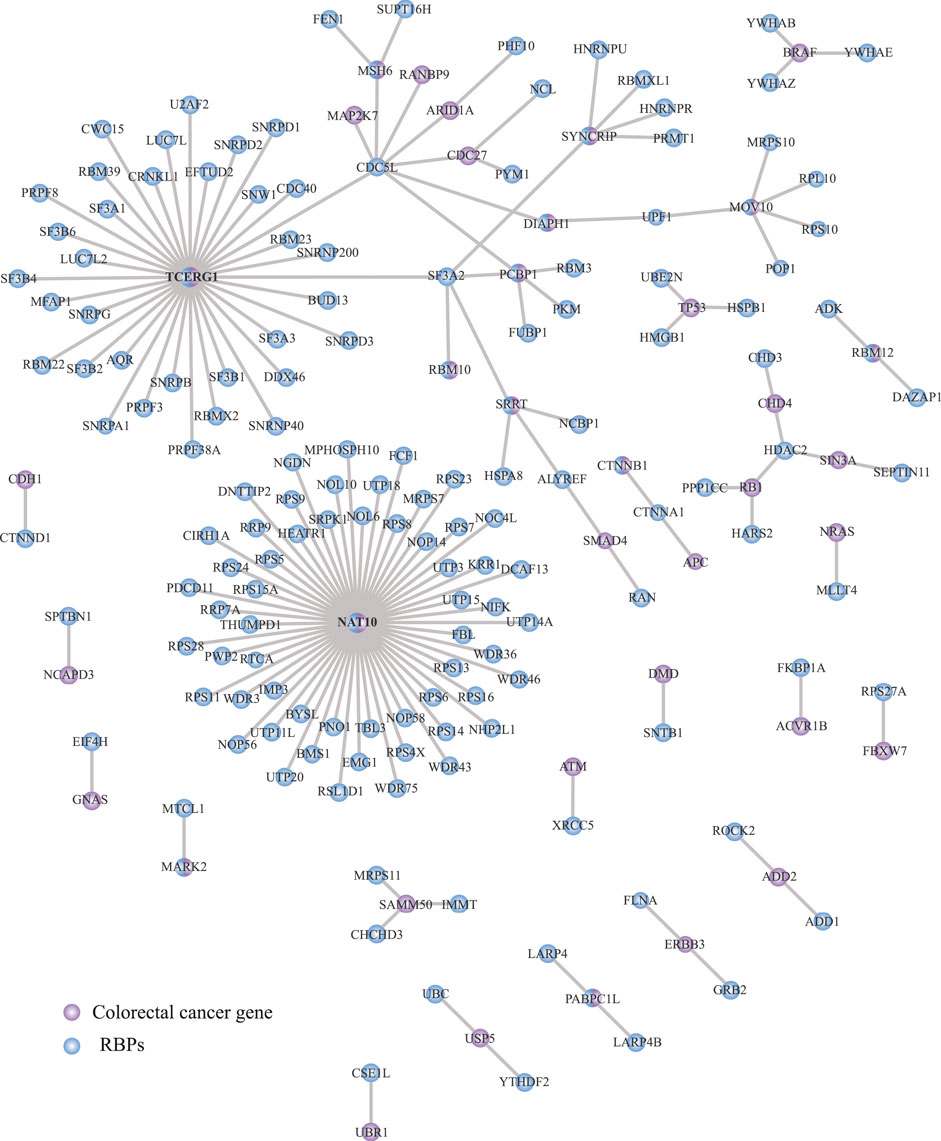
FIGURE 4. Interaction network between RBPs and colorectal cancer driver proteins. STRING portal (Szklarczyk et al., 2019) was used to analyze protein-protein interactions obtained from experiments and databases. A total of 153 RBPs were identified to be associated with 37 COREAD proteins. Blue = RBPs, purple = COREAD proteins.
RNA-binding proteins expression levels in colon and rectal tissues
The Human Protein Atlas (HPA) constitutes a large-scale resource to study antibody-based protein expression patterns in human tissues (Uhlén et al., 2015; Thul et al., 2017; Uhlen et al., 2017). We, therefore, used this tool to identify differentially expressed RBPs between tumoral and normal colon and rectal tissues. Thus, we compared protein immunohistochemical levels (not detected, low, medium, and high) of 608 available RBPs in COAD and 609 RBPs in READ tissues. We detected 211 in colon and 226 in rectum RBPs having at least one variation level (e.g., not detected to low or medium to high) between malignant and healthy colon and rectal tissues, respectively (Figure 5; Supplementary Tables S11, S12).
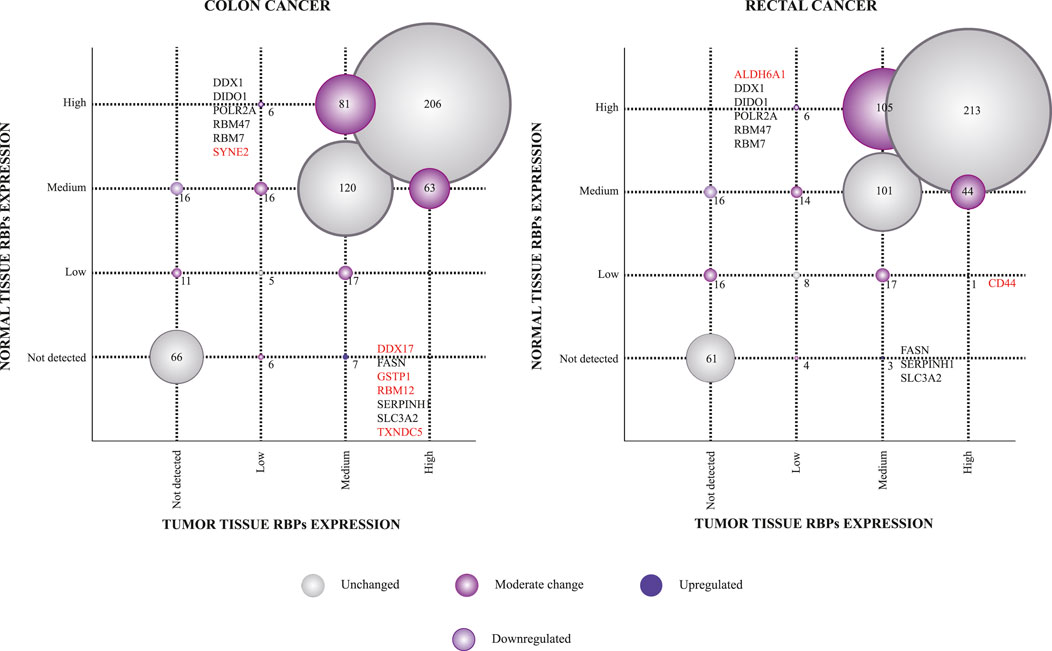
FIGURE 5. Immunohistochemical protein expression profiles of RNA-binding proteins between healthy and tumoral tissues. Scatterplots comparing RBPs immunohistochemical levels between normal and tumoral tissues are presented. The size of the circle is associated with the number of RBPs found in each correlation. RBPs in red are those which are unique for each type of cancer.
To detect highly altered RBPs, we next categorized these proteins as up or downregulated based on a two-variation level difference. As a result, we found seven upregulated RBPs (DDX17, FASN, GSTP1, RBM12, SERPINH1, SLC3A2, and TXNDC5) and six downregulated (DDX1, DIDO1, POLR2A, RBM47, RBM7, and SYNE2) in colon tissues. As anticipated, our strategy identified well-known COREAD proteins, such as DDX17 (Li X. N. et al., 2018), FASN (Yu et al., 2020) or GSTP1 (Sameer et al., 2012), validating our analysis. It is noteworthy to mention that RBM12 and RBM7 have never been implicated in COAD or READ before. Regarding rectal tissues, we identified four upregulated (CD44, FASN, SERPINH1, and SLC3A2) and six downregulated RBPs (ALDH6A1, DDX1, DIDO1, POLR2A, RBM47, and RBM7). Also, several of these proteins have been previously studied in COREAD as ALDH6A1 (Li X. et al., 2022) or DDX1 (Tanaka et al., 2018). Interestingly, CD44, a well-established COREAD protein (Herrlich et al., 1995; Wielenga et al., 2000), was upregulated only in rectal tumors showing its potential to distinguish rectal from colon carcinomas.
Identification of RNA binding proteins involved in COAD and READ cell survival
To identify essential RBPs for COAD and READ cell survival, we interrogated two large-scale CRISPR-Cas9 (CERES; Meyers et al., 2017) and RNAi (DEMETER2; Tsherniak et al., 2017; McFarland et al., 2018) loss-of-function screens using the DepMap portal (https://depmap.org/portal/). (Yu et al., 2016) CERES contains data of 1,341 RBPs in 47 colon and 10 rectal cancer cell lines, while DEMETER2 presents data of 1,255 RBPs in 42 colon and three rectal cancer cell lines. These initiatives calculate a dependency score that represents how vital a gene is to cell survival. A score of 0 indicates non-essentiality, whereas a score of ≤ −1 corresponds to the median of all pan-essential genes. Thus, we identified 352 (87 detected by both screens) and 343 (85 detected by both screens) essential RBPs in colon and rectal tumors, respectively (Figures 6A,B; Supplementary Tables S13–S16). Additionally, Table 3 shows the top five essential RBPs for colon and rectal carcinomas.
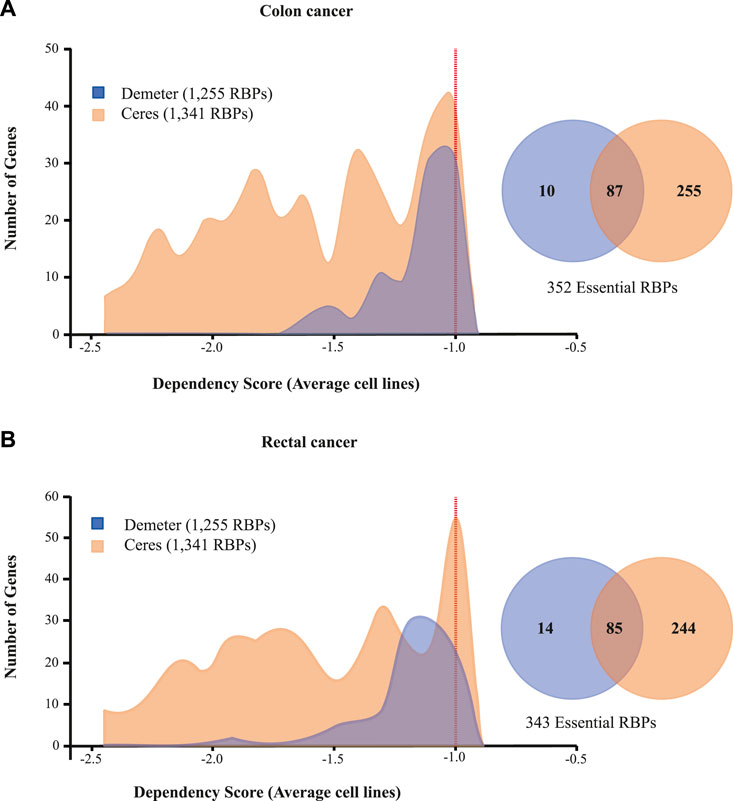
FIGURE 6. RBPs (A) colon and (B) rectal cancer dependencies. Dependency scores of 1,255 (DEMETER2; Tsherniak et al., 2017; McFarland et al., 2018) and 1,341 RBPs (CERES; Meyers et al., 2017) from 89 colon and 13 rectal cancer cell lines are presented.
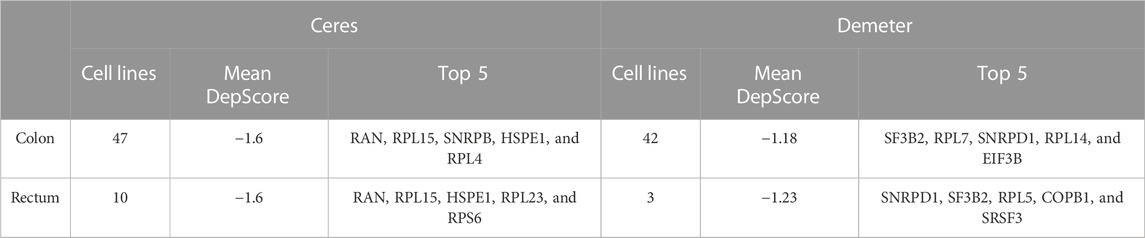
TABLE 3. CRISPR-Cas9 (CERES; Meyers et al., 2017) and RNAi (DEMETER2; Tsherniak et al., 2017; McFarland et al., 2018) cell lines and top five essential RBPs.
Prioritization of RNA-binding proteins in colon and rectal carcinomas
As more and more studies reveal the complex roles of RBPs in cancer progression, new data mining strategies come forward to narrowed down the identification of tumorigenic RBPs (Xing et al., 2021; Chen et al., 2022). To identify potential colon and rectal cancer tumor progressors RBPs, we next used our previously published multidata integration strategy (Figure 1) (García-Cárdenas et al., 2022). Thus, we merged our previous results as follows: 1) first quartile of most genomic and transcriptomic altered RBPs (n = 348) concerning tumor progression-related alterations (mRNA upregulation + genomic amplification + gene mutations + fusions), 2) 153 RBPs presenting PPis with colorectal proteins, 3) 93 (colon) and 69 (rectum) RBPs having moderate or upregulated immunohistochemical variation, 4) 352 (colon) and 343 (rectum) essential RBPs (Figure 7).
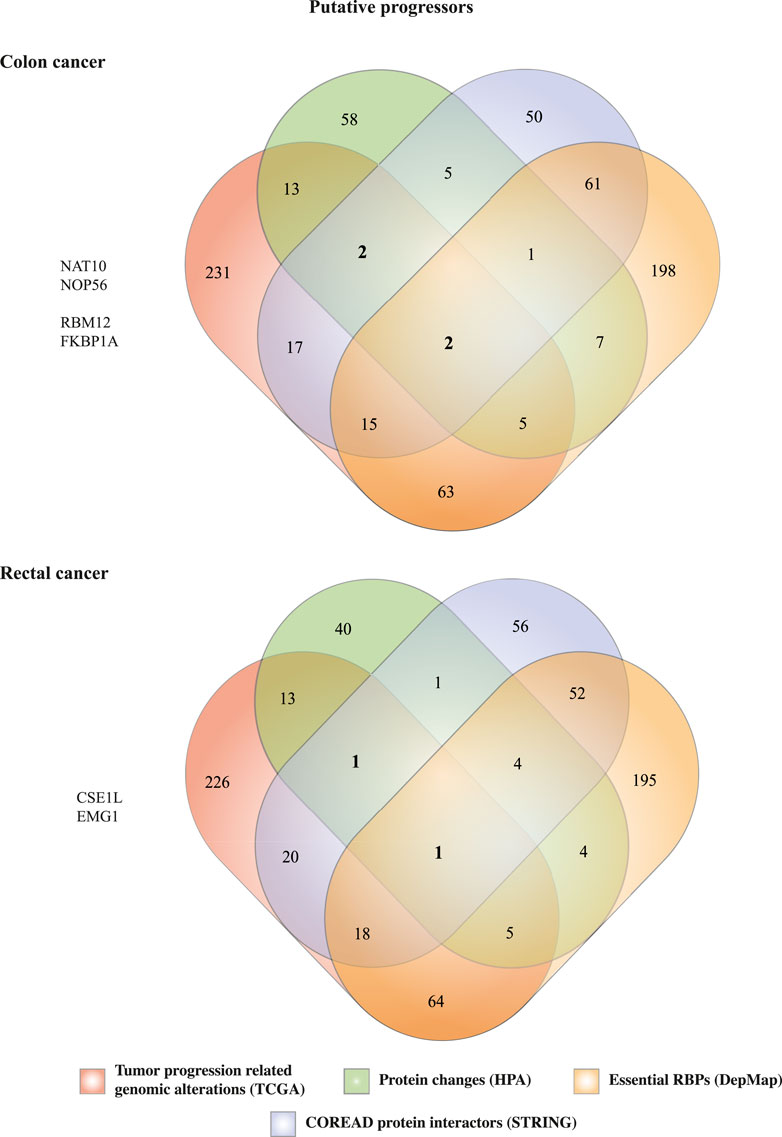
FIGURE 7. Identification of novel RNA-binding proteins involved in colon and rectal carcinomas progression. Prioritization of RBPs in COAD and READ was based on four cancer-related characteristics 1) high genomic and transcriptomic alterations, 2) interactions with well-known cancer proteins, 3) aberrant protein expression levels compared with normal tissues, and/or 4) essential for tumor survival.
In COAD, we identified two RBPs (NAT10 and NOP56) having all four tumor-associated characteristics presented in this study. NAT10 has already been implicated in colorectal cancer in several studies (Zhang et al., 2014; Liu et al., 2016; 2019; Cao et al., 2020), while NOP56 has been found differentially expressed in COREAD by a different data mining approach (Liang et al., 2021). Also, we were able to retrieve two other RBPs (RBM12 and FKBP1A) that were not essential for tumor cell survival, but they could be implicated in any other hallmark of cancer. For instance, FKBP1A overexpression has been correlated with apoptosis inhibition in prostate cancer (Leng et al., 2020); we did not find any reports of its involvement in COREAD. On the other hand, RBM12 has been found to be hypermutated in 619 COREAD tumors (Giannakis et al., 2016), but no further experimentation has been performed.
Similarly, we prioritized two RBPs in rectal cancer, CSE1L which presents all the above-mentioned characteristics, and EMG1 that was not essential for tumor cell survival. CSE1L has been related to colorectal cancer before (Sillars-Hardebol et al., 2012; Tai et al., 2013; Pimiento et al., 2016; Xu et al., 2020), while EMG1 has been poorly studied. Interestingly, NOP56, NAT10, and CSEL1 have been classified as common essential by DepMap survival algorithm. Thus, therapeutic targeting of these RBPs could have a greater impact in cell survival due to their implications in RNA-dependent basic cellular processes.
Finally, we intended to understand how these prioritized RBPs could correlate with cancer in general terms. To that end, we used the HumanNet v3 (Lee et al., 2011; Hwang et al., 2019) to generate networks where only cancer genes and RBPs were considered. We observed intricated connections between these prioritized RBPs with several cancer genes and other RBPs, showing their potential to promote cancer (Figures 8A,B; Supplementary Tables S17, S18).
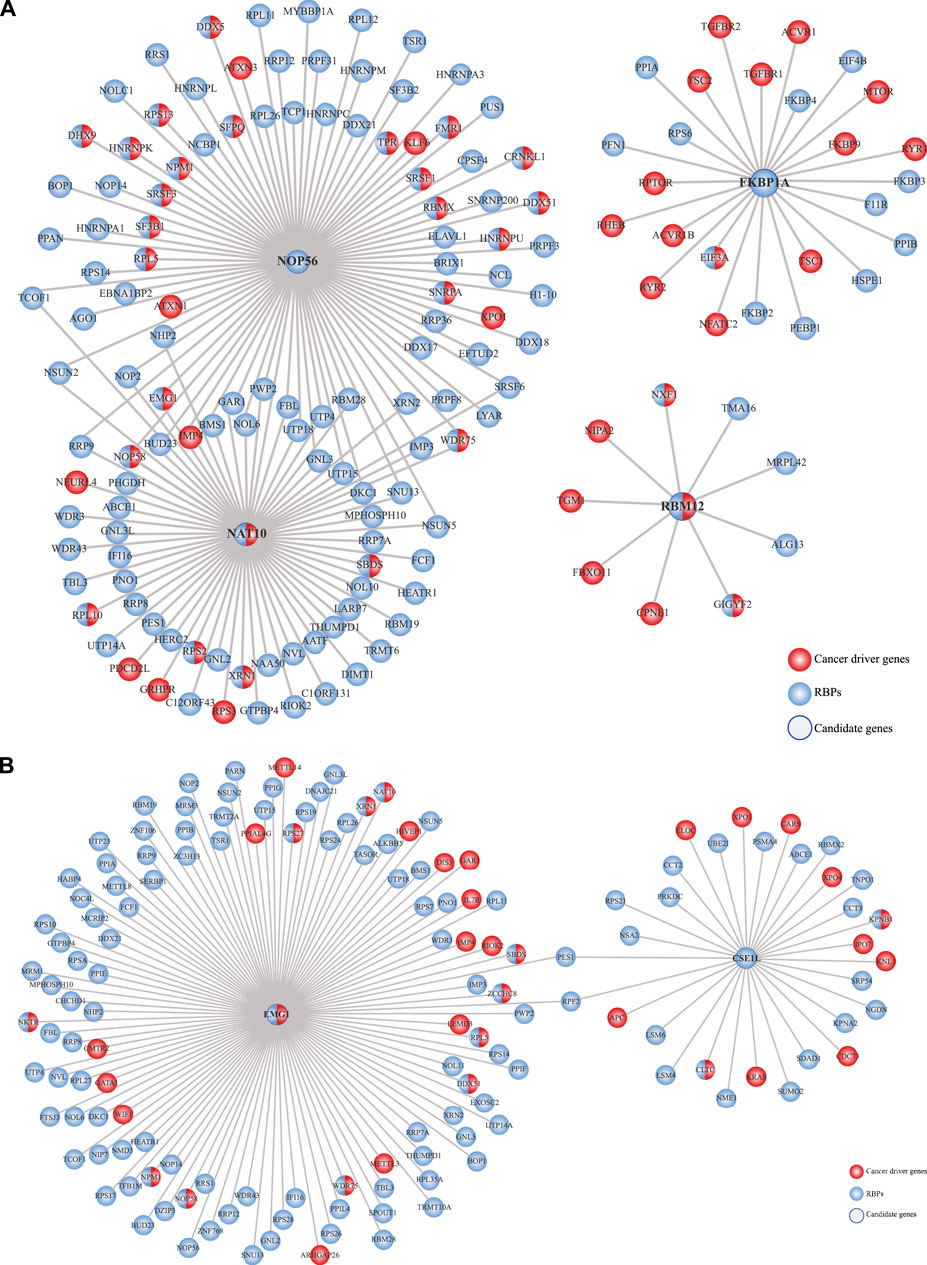
FIGURE 8. Prioritized RBPs correlate with cancer genes. Previously prioritized RBPs in (A) colon cancer (NAT10, NOP56, RBM12, and FKBP1A) and (B) rectal cancer (CSE1L and EMG1) were correlated with cancer genes by networking analysis using the HumanNet v3 database (Kim et al., 2022).
RBPs mRNA expression levels as determinants of clinical outcomes
To explore the clinical relevance of RBPs mRNA expression (upregulation vs. downregulation) in COREAD, COAD, and READ patients, we have interrogated TCGA, PanCancer Atlas (Hoadley et al., 2018) database for mRNA expression of prioritized RBPs (NAT10, NOP56, RBM12, FKBP1A, CSE1L, and EMG1) in those populations and calculated several clinical aspects (OS and DFS). In COREAD population, we only found FKBP1A expression is related with an adverse outcome in overall survival (OS; p < 0.05) (Figure 9A).
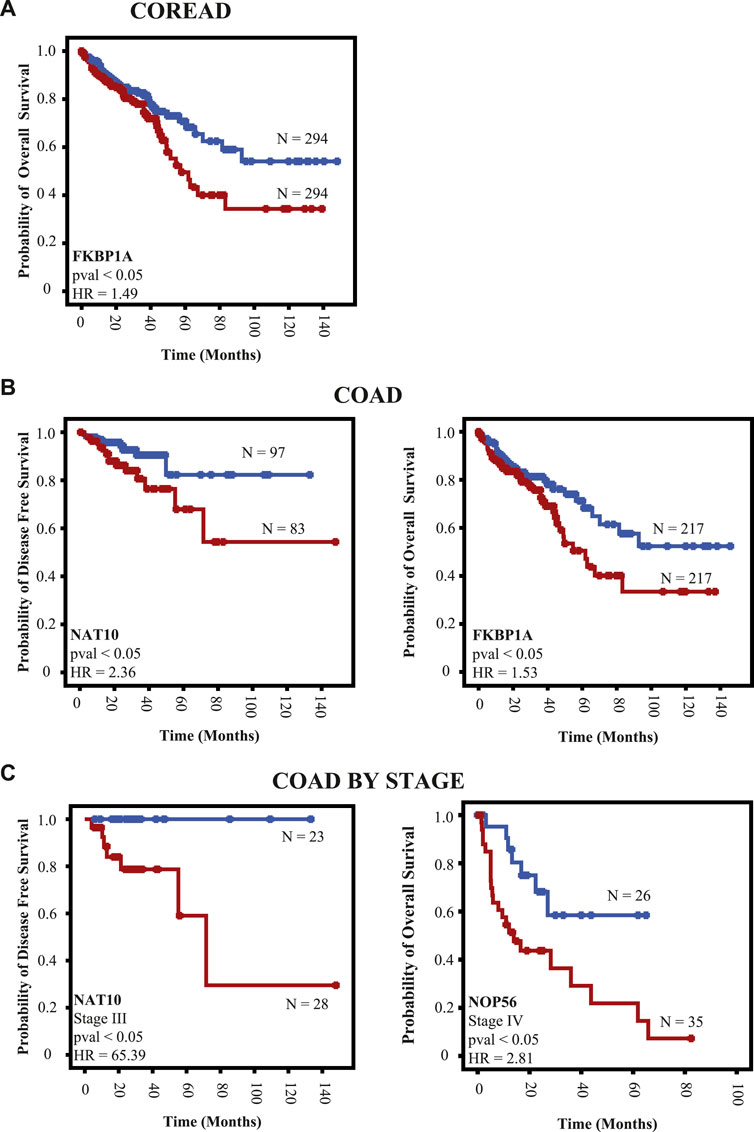
FIGURE 9. RBPs expression as a determinant of clinical outcome. We interrogated TCGA data using as a cutoff point the median of mRNA expression. (A) Prognosis in COREAD population (B) prognosis in colon cancer patients regardless the stage, and (C) colon cancer patients by stage. Red and blue lines indicate downregulation and upregulation mRNA, respectively.
Due to the fact that colon and rectum cancer are different clinical entities (Paschke et al., 2018), we have explored whether these relevant RBPs could predict clinical outcomes. In COAD patients, NAT10 and FKBP1A are related with adverse outcome in DFS and OS, respectively (p < 0.05; Figure 9B). We also found that NAT10 is related with poor outcome in DFS of stage III COAD patients (p < 0.05; Figure 9C). Moreover, high expression of NOP56 in primary tumors are related with bad outcome in OS of stage IV COAD patients (p < 0.05; Figure 9C). No other clinical outcomes were predicted by RBPs mRNA expression in COREAD, COAD, and READ patients.
Discussion
The development and implementation of multi-omics approaches along with modern bioinformatic technologies have provided new insights in COREAD biology (Chierici et al., 2020; Yin et al., 2020; Heo et al., 2021). In that respect, several studies have attempted to molecularly characterize COREAD tumors (Schlicker et al., 2012; Budinska et al., 2013; De Sousa E Melo et al., 2013; Marisa et al., 2013; Sadanandam et al., 2013; Roepman et al., 2014). Guinney et al., by integrating several subtyping algorithms, proposed the Consensus Molecular Subtypes of colorectal cancer to establish a baseline for clinical decision making (Guinney et al., 2015). Nevertheless, according to Paschke et al., COREAD has been treated as one entity when several clinical and molecular aspects (e.g., epidemiology, carcinogenic risk, molecular carcinogenesis, etc.) indicate the contrary (Paschke et al., 2018). Consequently, Paschke et al., suggested separating COREAD into COAD and READ, implementing a new perspective to discover novel biomarkers for both cancer types, which holds the potential to improve subtype-based clinical interventions (Paschke et al., 2018). In this regard, RBPs as emerging regulators of cancerous processes (Wurth and Gebauer, 2015; Corrado et al., 2016; Hentze et al., 2018; Kang et al., 2020; Zhang et al., 2020; Chen et al., 2022), could fulfill this need.
In this work, we used our previously published multidata integration strategy to prioritized tumorigenic RBPs in COAD and READ separately (García-Cárdenas et al., 2022). First, we determined genomic and transcriptomic alteration profiles of RBPs in COAD and READ patients. As is shown in Figure 2A, most of the alterations were found in mRNA levels: mRNA upregulation (64% in colon and 62% in rectum) followed by mRNA downregulation (15% in colon and 20% in rectum). Even though genomic and transcriptomic alteration profiles of COAD and READ were similar, we found significant differences among alteration types (Figure 2B) and the level of up and downregulation (Figure 2C), supporting Paschke et al., findings at least regarding RNA metabolism (Paschke et al., 2018). These results also agree with a comprehensive transcriptomic analysis performed by Zhang et al., in which RBPs are predominantly upregulated across cancer types (Zhang et al., 2020). These alterations will probably influence key post-transcriptional processes involved in COAD and READ development.
Despite the genomic and transcriptomic alteration similarities among the subtypes of both carcinomas, most altered RBPs in each subtype differed (Figures 3A,B). At least in READ, most of the highly altered RBPs are unique for this type of cancer, e.g., TSR1, TSR1, DYNC2H1, DDX55, CPSF7, CAND1, LARP4, ZCCHC3, and LSM14B and they have never been associated with COREAD. These findings support Paschke et al., suggestion (Paschke et al., 2018), several RBPs and maybe not only RBPs could have been ignored when we studied as COREAD but, when they are separated in COAD and READ, new putative biomarkers are discovered.
Unlike subtypes, we observed dissimilar patterns of RBPs genomic and transcriptomic alterations among stages in colon and rectal tumors. Stage II was the most altered in COAD, whereas in READ the most altered one was stage I (Figures 3C,D; Table 2). Despite these differences, we found proteins that were constantly altered in all stages. For example, STAU1 and YTHDF1 were highly altered across stages in both carcinomas. Contrary to STAU1, YTHDF1 has previously been associated with COREAD development (Bai et al., 2019; Chen P. et al., 2021; Yan et al., 2021). Interestingly, STAU1 has been related with pancreatic cancer (Marcellus et al., 2021) and its misregulation impacts cell cycle regulation (Bonnet-Magnaval and DesGroseillers, 2021), showing the potential of this protein to also be involved in COREAD progression.
Networking analysis has been shown to be a powerful tool to identify tumorigenic proteins in cancer (Wurth et al., 2016; Indacochea et al., 2021; García-Cárdenas et al., 2022). Similarly, PPIs between RBPs and COREAD drivers allowed us to determine two functional modules, such as NAT10 and TCERG1, which were central elements (Figure 4). These RBPs have also been catalogued as COREAD drivers. In fact, NAT10 suppresses tumor proliferation by activating p53; in COREAD, NAT10 activity is decreased resulting in p53 malfunction and, therefore, uncontrollable cell division (Liu et al., 2016). Concerning TCERG1 subnetwork, we observed intricated connections between RBPs and COREAD drivers. For example, TCERG1 interacts with CDC5L which in turn connects with six COREAD drivers (MAP2K7, RANBP9, ARID1A, CDC27, MSH6, and DIAPH1). Interestingly, CDC5L has been related to other cancer types, such as prostate cancer (Li X. et al., 2018) and osteosarcoma (Lu et al., 2008).
We next examined protein immunohistochemical levels (the Human Protein Atlas database) to identify differentially expressed RBPs in colon and rectal tumor tissues (Pontén et al., 2008). In fact, immunohistochemistry (IHC) is a widely used approach in histopathology for cancer diagnosis. Thus, we found DDX17, GSTP1, RBM12, and TXNDC5 to be overexpressed only in COAD, while CD44 is exclusively upregulated in READ. Similarly, we found SYNE2 and ALDH6A1 to be exclusively downregulated in COAD and READ, respectively. Further IHC studies should be performed to address their potential as diagnostic biomarkers of colon and rectal tumors, separately. As anticipated, our strategy also identified well-known COREAD proteins (Figure 5), such as DDX17 (Li X. N. et al., 2018), ALDH6A1 (Li X. et al., 2022), DDX1 (Tanaka et al., 2018), FASN (Yu et al., 2020), and GSTP1 (Sameer et al., 2012), validating our analysis. It is noticeable to mention that RBM12 and RBM7 have never been implicated in COAD or READ before.
Then, we explored RBPs-based COAD and READ cell dependencies by interrogating two large-scale loss-of-function screens CERES (Meyers et al., 2017) and DEMETER2 (Tsherniak et al., 2017; McFarland et al., 2018). In colon we found 352 essentials RBPs, while in READ we identified 343 RBPs (both CRISPR-Cas9 and RNAi methods included) (Figures 6A,B). In other words, 25% of all known RBPs are essentials for oncogenic cells survival, unsurprisingly given the crucial role of RBPs in RNA metabolism. In Table 3, we listed the top five essentials RBPs in both types of cancer based on the DepScore. The same scenario of the previous analyses is repeated, we obtained RBPs that have been related to COREAD (e.g., SF3B2, RPL7, and SNRPD1), and others that have not (e.g., COPB1) (Supplementary Tables S13–S16) (Boleij et al., 2010; Fijneman et al., 2012; Xu et al., 2021).
Compelling studies have shown the potential of RBPs to promote cancer development. RBPs are widely altered in cancer cells, control hundreds to thousands RNAs, and interact with cancer driver proteins (Wurth and Gebauer, 2015; Hentze et al., 2018; García-Cárdenas et al., 2019). With that in mind, we reasoned that the integration of our previous analyses could narrow down the identification of potential COAD and READ RBPs. Our data mining strategy (Figure 1) allowed us to identify four proteins in COAD (NAT10, NOP56, RBM12, and FKBP1A) and two in READ (CSE1L and EMG1) (Figure 7). NAT10 and CSE1L have already been involved in COREAD (Sillars-Hardebol et al., 2012; Tai et al., 2013; Zhang et al., 2014; Liu et al., 2016; 2019; Pimiento et al., 2016; Cao et al., 2020; Xu et al., 2020). NOP56 and RBM12 were already identified in COREAD by different data mining approaches (Liang et al., 2021), validating our results. Additionally, to the best of our knowledge, no prior studies have associated FKBP1A and EMG1 with cancer before. To highlight their relevance in cancer, we interrogated the HumanNet v3 and found that NOP56, RBM12, FKBP1A and EMG1 are highly interconnected with cancer genes and other RBPs, showing their potential to form tumorigenic RNA-regulons (Figures 8A,B).
Finally, we explored the clinical implications of these prioritized RBPs. Upregulation of FKBP1A, NAT10, and NOP56 mRNA expression could predict clinical outcomes in COREAD and COAD patients. Similarly, mRNA upregulation of NAT10 and NOP56 are related with poor outcomes depending on COAD staging. This is clinically relevant since COAD therapy is defined by stage (Argilés et al., 2020; Cervantes et al., 2022). Stage II COAD patients are not always candidate of adjuvant therapy. However, adjuvant chemotherapy is a relevant treatment for stage III COAD patients because it decreases the risk of relapse (Argilés et al., 2020). In daily clinical practice there are no specific biomarker to predict a relapse. According to our results, NAT10 mRNA upregulation is related with adverse outcome in DFS of stage III COAD patients. Similarly, NOP56 overexpression in primary tumors is associated with poor prognosis in DFS and OS of stage IV COAD patients. These results highlight the clinical relevance of FKBP1A, NAT10, and NOP56. Despite these promising findings, a high number of patients are needed to validate these results in specifical clinical scenarios.
In summary, we analyzed and integrated data from 488 COAD and 155 READ patients, 102 cancer cell lines, more than 15,000 immunostainings, and ∼10,000 raw associations between RBPs and cancer genes to unravel new RBPs involved in COAD (NOP56, NAT10, RBM12, and FKBP1A) and READ (EMG1 and CSE1L). Further analyses allowed us to identify potential clinical applications of FKBP1A, NAT10, and NOP56 as biomarkers of specific outcomes.
Data availability statement
The datasets presented in this study can be found in online repositories. The names of the repository/repositories and accession number(s) can be found in the article/Supplementary Material.
Author contributions
Conceptualization, SG, AI, and JG-C; methodology, SG, AI, and JG-C; investigation, SG, AI, and JG-C; data curation, SG and AL-C. writing original draft preparation, SG and JG-C; writing editing, NG-C, IA-C, AL-C, AI, and DP-C; visualization, SG, NG-C, IA-C, and DP-C; supervision, SG and AI All authors have read and agreed to the published version of the manuscript.
Conflict of interest
The authors declare that the research was conducted in the absence of any commercial or financial relationships that could be construed as a potential conflict of interest.
Publisher’s note
All claims expressed in this article are solely those of the authors and do not necessarily represent those of their affiliated organizations, or those of the publisher, the editors and the reviewers. Any product that may be evaluated in this article, or claim that may be made by its manufacturer, is not guaranteed or endorsed by the publisher.
Supplementary material
The Supplementary Material for this article can be found online at: https://www.frontiersin.org/articles/10.3389/fcell.2023.1088057/full#supplementary-material
References
Abdel-Wahab, O., and Gebauer, F. (2018). Editorial overview: Cancer genomics: RNA metabolism and translation in cancer pathogenesis and therapy. Curr. Opin. Genet. Dev. 48, iv. vi. doi:10.1016/j.gde.2018.01.007
Adler, A. S., McCleland, M. L., Yee, S., Yaylaoglu, M., Hussain, S., Cosino, E., et al. (2014). An integrative analysis of colon cancer identifies an essential function for PRPF6 in tumor growth. Genes Dev. 28, 1068–1084. doi:10.1101/gad.237206.113
Argilés, G., Tabernero, J., Labianca, R., Hochhauser, D., Salazar, R., Iveson, T., et al. (2020). Localised colon cancer: ESMO clinical practice guidelines for diagnosis, treatment and follow-up. Ann. Oncol. Off. J. Eur. Soc. Med. Oncol. 31, 1291–1305. doi:10.1016/J.ANNONC.2020.06.022
Assis, J. V. de, Coutinho, L. A., Oyeyemi, I. T., Oyeyemi, O. T., and Grenfell, R. F. e. Q. (2022). Diagnostic and therapeutic biomarkers in colorectal cancer: A review. Am. J. Cancer Res. 12, 661.
Bai, Y., Yang, C., Wu, R., Huang, L., Song, S., Li, W., et al. (2019). YTHDF1 regulates tumorigenicity and cancer stem cell-like activity in human colorectal carcinoma. Front. Oncol. 9, 332. doi:10.3389/FONC.2019.00332
Bi, G., Zhu, D., Bian, Y., Huang, Y., Zhan, C., Yang, Y., et al. (2021). Knockdown of GTF2E2 inhibits the growth and progression of lung adenocarcinoma via RPS4X in vitro and in vivo. Cancer Cell Int. 21, 181. doi:10.1186/S12935-021-01878-Z
Boleij, A., Roelofs, R., Schaeps, R. M. J., Schülin, T., Glaser, P., Swinkels, D. W., et al. (2010). Increased exposure to bacterial antigen RpL7/L12 in early stage colorectal cancer patients. Cancer 116, 4014–4022. doi:10.1002/CNCR.25212
Bonnet-Magnaval, F., and DesGroseillers, L. (2021). The Staufen1-dependent cell cycle regulon or how a misregulated RNA-binding protein leads to cancer. Biol. Rev. Camb. Philos. Soc. 96, 2192–2208. doi:10.1111/BRV.12749
Budinska, E., Popovici, V., Tejpar, S., D’Ario, G., Lapique, N., Sikora, K. O., et al. (2013). Gene expression patterns unveil a new level of molecular heterogeneity in colorectal cancer. J. Pathol. 231, 63–76. doi:10.1002/PATH.4212
Cao, J., Hou, P., Chen, J., Wang, P., Wang, W., Liu, W., et al. (2017). The overexpression and prognostic role of DCAF13 in hepatocellular carcinoma. Tumor Biol. 39, 1010428317705753. doi:10.1177/1010428317705753
Cao, Y., Yao, M., Wu, Y., Ma, N., Liu, H., and Zhang, B. (2020). N-acetyltransferase 10 promotes micronuclei formation to activate the senescence-associated secretory phenotype machinery in colorectal cancer cells. Transl. Oncol. 13, 100783. doi:10.1016/J.TRANON.2020.100783
Cervantes, A., Adam, R., Roselló, S., Arnold, D., Normanno, N., Taïeb, J., et al. (2022). Metastatic colorectal cancer: ESMO clinical practice guideline for diagnosis, treatment and follow-up. Ann. Oncol. 34, 10–32. doi:10.1016/J.ANNONC.2022.10.003/ATTACHMENT/5A57CC70-CCE4-401B-A657-C12E03275943/MMC1.PDF
Chen, L., Zhou, S. J., Xu, Y., Liao, Q. M., Zou, Y. S., and Pei, H. (2021). CCAR2 promotes a malignant phenotype of osteosarcoma through Wnt/β-catenin-dependent transcriptional activation of SPARC. Biochem. Biophys. Res. Commun. 580, 67–73. doi:10.1016/J.BBRC.2021.09.070
Chen, P., Liu, X. Q., Lin, X., Gao, L. Y., Zhang, S., and Huang, X. (2021). Targeting YTHDF1 effectively re-sensitizes cisplatin-resistant colon cancer cells by modulating GLS-mediated glutamine metabolism. Mol. Ther. Oncolytics 20, 228–239. doi:10.1016/J.OMTO.2021.01.001
Chen, Y., Qin, H., and Zheng, L. (2022). Research progress on RNA−binding proteins in breast cancer. Front. Oncol. 12, 4303. doi:10.3389/fonc.2022.974523
Chierici, M., Bussola, N., Marcolini, A., Francescatto, M., Zandonà, A., Trastulla, L., et al. (2020). Integrative network fusion: A multi-omics approach in molecular profiling. Front. Oncol. 10, 1065. doi:10.3389/fonc.2020.01065
Cohen, R., Pudlarz, T., Delattre, J. F., Colle, R., and André, T. (2020). Molecular targets for the treatment of metastatic colorectal cancer. Cancers (Basel) 12, 2350–2418. doi:10.3390/CANCERS12092350
Corrado, G., Tebaldi, T., Costa, F., Frasconi, P., and Passerini, A. (2016). RNAcommender: Genome-wide recommendation of RNA-protein interactions. Bioinformatics 32, 3627–3634. doi:10.1093/bioinformatics/btw517
De Sousa E Melo, F., Wang, X., Jansen, M., Fessler, E., Trinh, A., De Rooij, L. P. M. H., et al. (2013). Poor-prognosis colon cancer is defined by a molecularly distinct subtype and develops from serrated precursor lesions. Nat. Med. 195 19, 614–618. doi:10.1038/nm.3174
Dressler, L., Bortolomeazzi, M., Keddar, M. R., Misetic, H., Sartini, G., Acha-Sagredo, A., et al. (2022). Comparative assessment of genes driving cancer and somatic evolution in non-cancer tissues: An update of the network of cancer genes (NCG) resource. Genome Biol. 231 23, 35–22. doi:10.1186/S13059-022-02607-Z
Eldai, H., Periyasamy, S., Al Qarni, S., Al Rodayyan, M., Muhammed Mustafa, S., Deeb, A., et al. (2013). Novel genes associated with colorectal cancer are revealed by high resolution cytogenetic analysis in a patient specific manner. PLoS One 8, e76251. doi:10.1371/JOURNAL.PONE.0076251
Feichtinger, R. G., Schäfer, G., Seifarth, C., Mayr, J. A., Kofler, B., and Klocker, H. (2018). Reduced levels of ATP synthase subunit ATP5F1A correlate with earlier-onset prostate cancer. Oxid. Med. Cell. Longev. 2018, 1347174. doi:10.1155/2018/1347174
Fijneman, R. J. A., De Wit, M., Pourghiasian, M., Piersma, S. R., Pham, T. V., Warmoes, M. O., et al. (2012). Proximal fluid proteome profiling of mouse colon tumors reveals biomarkers for early diagnosis of human colorectal cancer. Clin. Cancer Res.PROTEOME-PROFILING-OF-MOUSE-COLON 18, 2613–2624. doi:10.1158/1078-0432.CCR-11-1937
Forghanifard, M. M., Naeimi Khorasanizadeh, P., Abbaszadegan, M. R., Javdani Mallak, A., and Moghbeli, M. (2020). Role of DIDO1 in progression of esophageal squamous cell carcinoma. J. Gastrointest. Cancer 51, 83–87. doi:10.1007/S12029-019-00212-1
García-Cárdenas, J. M., Armendáriz-Castillo, I., Pérez-Villa, A., Indacochea, A., Jácome-Alvarado, A., López-Cortés, A., et al. (2022). Integrated in silico analyses identify PUF60 and SF3A3 as new spliceosome-related breast cancer RNA-binding proteins. Biol 11, 481. Page 481 11. doi:10.3390/BIOLOGY11040481
García-Cárdenas, J. M., Guerrero, S., López-Cortés, A., Armendáriz-Castillo, I., Guevara-Ramírez, P., Pérez-Villa, A., et al. (2019). Post-transcriptional regulation of colorectal cancer: A focus on RNA-binding proteins. Front. Mol. Biosci. 6, 65. doi:10.3389/fmolb.2019.00065
Giannakis, M., Mu, X. J., Shukla, S. A., Qian, Z. R., Cohen, O., Nishihara, R., et al. (2016). Genomic correlates of immune-cell infiltrates in colorectal carcinoma. Cell Rep. 15, 857–865. doi:10.1016/J.CELREP.2016.03.075
Guinney, J., Dienstmann, R., Wang, X., de Reyniès, A., Schlicker, A., Soneson, C., et al. (2015). The consensus molecular subtypes of colorectal cancer. Nat. Med. 21, 1350–1356. doi:10.1038/nm.3967
Guo, X., Li, K., Jiang, W., Hu, Y., Xiao, W., Huang, Y., et al. (2020). RNA demethylase ALKBH5 prevents pancreatic cancer progression by posttranscriptional activation of PER1 in an m6A-YTHDF2-dependent manner. Mol. Cancer 19, 91. doi:10.1186/S12943-020-01158-W
Hanahan, D. (2022). Hallmarks of cancer: New dimensions. Cancer Discov. 12, 31–46. doi:10.1158/2159-8290.CD-21-1059
Hentze, M. W., Castello, A., Schwarzl, T., and Preiss, T. (2018). A brave new world of RNA-binding proteins. Nat. Rev. Mol. Cell Biol. 19, 327–341. doi:10.1038/nrm.2017.130
Heo, Y. J., Hwa, C., Lee, G.-H., Park, J.-M., and An, J.-Y. (2021). Integrative multi-omics approaches in cancer research: From biological networks to clinical subtypes. Mol. Cells 44, 433–443. doi:10.14348/molcells.2021.0042
Herrlich, P., Pals, S., and Ponta, H. (1995). CD44 in colon cancer. Eur. J. Cancer 31A, 1110–1112. doi:10.1016/0959-8049(95)00252-E
Hoadley, K. A., Yau, C., Hinoue, T., Wolf, D. M., Lazar, A. J., Drill, E., et al. (2018). Cell-of-Origin patterns dominate the molecular classification of 10,000 tumors from 33 types of cancer. Cell 173, 291–304.e6. doi:10.1016/j.cell.2018.03.022
Hu, B., Jiang, D., Chen, Y., Wei, L., Zhang, S., Zhao, F., et al. (2015). High CHMP4B expression is associated with accelerated cell proliferation and resistance to doxorubicin in hepatocellular carcinoma. Tumour Biol. 36, 2569–2581. doi:10.1007/S13277-014-2873-1
Hwang, S., Kim, C. Y., Yang, S., Kim, E., Hart, T., Marcotte, E. M., et al. (2019). HumanNet v2: Human gene networks for disease research. Nucleic Acids Res. 47, D573–D580. doi:10.1093/nar/gky1126
Indacochea, A., Santiago, G., Ureña, M., Araujo, F., Coll, O., Lleonart, M. E., et al. (2021). Cold-inducible RNA binding protein promotes breast cancer cell malignancy by regulating Cystatin C levels. RNA 27, 190–201. doi:10.1261/RNA.076422.120
Ji, P., Wang, H., Cheng, Y., and Liang, S. (2021). Prognostic prediction and gene regulation network of EIF2S2 in hepatocellular carcinoma based on data mining. J. Gastrointest. Oncol. 12, 3061–3078. doi:10.21037/JGO-21-748
Kang, D., Lee, Y., and Lee, J. S. (2020). RNA-binding proteins in cancer: Functional and therapeutic perspectives. Cancers (Basel) 12, 2699–2733. doi:10.3390/CANCERS12092699
Kim, C. Y., Baek, S., Cha, J., Yang, S., Kim, E., Marcotte, E. M., et al. (2022). HumanNet v3: An improved database of human gene networks for disease research. Nucleic Acids Res. 50, D632–D639. doi:10.1093/NAR/GKAB1048
Kim, H. J., Moon, S. J., Kim, S. H., Heo, K., and Kim, J. H. (2018). DBC1 regulates Wnt/β-catenin-mediated expression of MACC1, a key regulator of cancer progression, in colon cancer. Cell Death Dis. 9, 831. doi:10.1038/S41419-018-0899-9
Kuang, Y. S., Wang, Y., Ding, L. D., Yang, L., Wang, Y., Liu, S. H., et al. (2018). Overexpression of CREPT confers colorectal cancer sensitivity to fluorouracil. World J. Gastroenterol. 24, 475–483. doi:10.3748/WJG.V24.I4.475
Lee, I., Blom, U. M., Wang, P. I., Shim, J. E., and Marcotte, E. M. (2011). Prioritizing candidate disease genes by network-based boosting of genome-wide association data. Genome Res. 21, 1109–1121. doi:10.1101/gr.118992.110
Leng, W., Liu, Q., Zhang, S., Sun, D., and Guo, Y. (2020). LncRNA AFAP1-AS1 modulates the sensitivity of paclitaxel-resistant prostate cancer cells to paclitaxel via miR-195-5p/FKBP1A axis. Cancer Biol. Ther. 21, 1072–1080. doi:10.1080/15384047.2020.1829266
Li, H., Jin, X., Liu, B., Zhang, P., Chen, W., and Li, Q. (2019). CircRNA CBL.11 suppresses cell proliferation by sponging miR-6778-5p in colorectal cancer. BMC Cancer 19, 826. doi:10.1186/S12885-019-6017-2
Li N, N., Guo, Q., Zhang, Q., Chen, B. J., Li, X. A., and Zhou, Y. (2022). Comprehensive analysis of differentially expressed profiles of mRNA N6-methyladenosine in colorectal cancer. Front. Cell Dev. Biol. 9, 760912. doi:10.3389/FCELL.2021.760912
Li, S., Ma, J., Zheng, A., Song, X., Chen, S., and Jin, F. (2021). DEAD-box helicase 27 enhances stem cell-like properties with poor prognosis in breast cancer. J. Transl. Med. 19, 334. doi:10.1186/S12967-021-03011-0
Li, X. N., Wang, Z. J., Ye, C. X., Zhao, B. C., Li, Z. L., and Yang, Y. (2018). RNA sequencing reveals the expression profiles of circRNA and indicates that circDDX17 acts as a tumor suppressor in colorectal cancer. J. Exp. Clin. Cancer Res. 37, 325. doi:10.1186/S13046-018-1006-X
Li, X., Wang, N., Wu, Y., Liu, Y., and Wang, R. (2022). ALDH6A1 weakens the progression of colon cancer via modulating the RAS/RAF/MEK/ERK pathway in cancer cell lines. Gene 842, 146757. doi:10.1016/J.GENE.2022.146757
Li, X., Wang, X., Song, W., Xu, H., Huang, R., Wang, Y., et al. (2018). Oncogenic properties of NEAT1 in prostate cancer cells depend on the cdc5l–AGRN transcriptional regulation circuit. Cancer Res. 78, 4138–4149. doi:10.1158/0008-5472.CAN-18-0688
Liang, Q., Du, X., Mao, L., and Wang, G. (2021). Molecular characterization of colorectal cancer: A five-gene prognostic signature based on RNA-binding proteins. Saudi J. Gastroenterol. 27, 223–233. doi:10.4103/SJG.SJG_530_20
Liu, W., Wang, C., Wang, S., Zeng, K., Wei, S., Sun, N., et al. (2021). PRPF6 promotes androgen receptor/androgen receptor-variant 7 actions in castration-resistant prostate cancer cells. Int. J. Biol. Sci. 17, 188–203. doi:10.7150/IJBS.50810
Liu, X., Tan, Y., Zhang, C., Zhang, Y., Zhang, L., Ren, P., et al. (2016). NAT10 regulates p53 activation through acetylating p53 at K120 and ubiquitinating Mdm2. EMBO Rep. 17, 349–366. doi:10.15252/EMBR.201540505
Liu, Z., Liu, X., Li, Y., Ren, P., Zhang, C., Wang, L., et al. (2019). miR-6716-5p promotes metastasis of colorectal cancer through downregulating NAT10 expression. Cancer Manag. Res. 11, 5317–5332. doi:10.2147/CMAR.S197733
Liu, Z., Zhang, Y., Dang, Q., Wu, K., Jiao, D., Li, Z., et al. (2021). Genomic alteration characterization in colorectal cancer identifies a prognostic and metastasis biomarker: FAM83A|Ido1. Front. Oncol. 11, 607. doi:10.3389/fonc.2021.632430
López-Cortés, A., Paz-y-Miño, C., Guerrero, S., Jaramillo-Koupermann, G., León Cáceres, Á., Intriago-Baldeón, D. P., et al. (2020). Pharmacogenomics, biomarker network, and allele frequencies in colorectal cancer. Pharmacogenomics J. 20, 136–158. doi:10.1038/s41397-019-0102-4
Lu, X.-Y., Lu, Y., Zhao, Y.-J., Jaeweon, K., Kang, J., Xiao-Nan, L., et al. (2008). Cell cycle regulator gene CDC5L, a potential target for 6p12-p21 amplicon in osteosarcoma. Mol. Cancer Res. 6, 937–946. doi:10.1158/1541-7786.MCR-07-2115
Marcellus, K. A., Crawford Parks, T. E., Almasi, S., and Jasmin, B. J. (2021). Distinct roles for the RNA-binding protein Staufen1 in prostate cancer. BMC Cancer 21, 120. doi:10.1186/S12885-021-07844-2
Marisa, L., de Reyniès, A., Duval, A., Selves, J., Gaub, M. P., Vescovo, L., et al. (2013). Gene expression classification of colon cancer into molecular subtypes: Characterization, validation, and prognostic value. PLOS Med. 10, e1001453. doi:10.1371/JOURNAL.PMED.1001453
Mauri, G., Bonazzina, E., Amatu, A., Tosi, F., Bencardino, K., Gori, V., et al. (2021). The evolutionary landscape of treatment for BRAFV600E mutant metastatic colorectal cancer. Cancers (Basel) 13, 137–215. doi:10.3390/CANCERS13010137
McFarland, J. M., Ho, Z. V., Kugener, G., Dempster, J. M., Montgomery, P. G., Bryan, J. G., et al. (2018). Improved estimation of cancer dependencies from large-scale RNAi screens using model-based normalization and data integration. Nat. Commun. 9, 4610. doi:10.1038/s41467-018-06916-5
Mestre-Farràs, N., Guerrero, S., Bley, N., Rivero, E., Coll, O., Borràs, E., et al. (2022). Melanoma RBPome identification reveals PDIA6 as an unconventional RNA-binding protein involved in metastasis. Nucleic Acids Res. 50, 8207–8225. doi:10.1093/NAR/GKAC605
Meyers, R. M., Bryan, J. G., McFarland, J. M., Weir, B. A., Sizemore, A. E., Xu, H., et al. (2017). Computational correction of copy number effect improves specificity of CRISPR–Cas9 essentiality screens in cancer cells. Nat. Genet. 49, 1779–1784. doi:10.1038/ng.3984
Mu, W., Xie, Y., Li, J., Yan, R., Zhang, J., Liu, Y., et al. (2022). High expression of PDZ-binding kinase is correlated with poor prognosis and immune infiltrates in hepatocellular carcinoma. World J. Surg. Oncol. 20, 22. doi:10.1186/S12957-021-02479-W
Noda, D., Itoh, S., Watanabe, Y., Inamitsu, M., Dennler, S., Itoh, F., et al. (2006). ELAC2, a putative prostate cancer susceptibility gene product, potentiates TGF-beta/Smad-induced growth arrest of prostate cells. Oncogene 25, 5591–5600. doi:10.1038/SJ.ONC.1209571
Paschke, S., Jafarov, S., Staib, L., Kreuser, E. D., Maulbecker-Armstrong, C., Roitman, M., et al. (2018). Are colon and rectal cancer two different tumor entities? A proposal to abandon the term colorectal cancer. Int. J. Mol. Sci. 19, 2577. doi:10.3390/IJMS19092577
Pimiento, J. M., Neill, K. G., Henderson-Jackson, E., Eschrich, S. A., Chen, D. T., Husain, K., et al. (2016). Knockdown of CSE1L gene in colorectal cancer reduces tumorigenesis in vitro. Am. J. Pathol. 186, 2761–2768. doi:10.1016/J.AJPATH.2016.06.016
Pontén, F., Jirström, K., and Uhlen, M. (2008). The human protein atlas—A tool for pathology. J. Pathol. 216, 387–393. doi:10.1002/path.2440
Qu, Y., Gao, N., and Wu, T. (2021). Expression and clinical significance of SYNE1 and MAGI2 gene promoter methylation in gastric cancer. Med. Baltim. 100, e23788. doi:10.1097/MD.0000000000023788
Repana, D., Nulsen, J., Dressler, L., Bortolomeazzi, M., Kuppili Venkata, S., Tourna, A., et al. (2019). The network of cancer genes (NCG): A comprehensive catalogue of known and candidate cancer genes from cancer sequencing screens. Genome Biol. 20, 1–12. doi:10.1186/s13059-018-1612-0
Roepman, P., Schlicker, A., Tabernero, J., Majewski, I., Tian, S., Moreno, V., et al. (2014). Colorectal cancer intrinsic subtypes predict chemotherapy benefit, deficient mismatch repair and epithelial-to-mesenchymal transition. Int. J. Cancer 134, 552–562. doi:10.1002/IJC.28387
Sadanandam, A., Lyssiotis, C. A., Homicsko, K., Collisson, E. A., Gibb, W. J., Wullschleger, S., et al. (2013). A colorectal cancer classification system that associates cellular phenotype and responses to therapy. Nat. Med. 195 19, 619–625. doi:10.1038/nm.3175
Sameer, A. S., Qadri, Q., and Siddiqi, M. A. (2012). GSTP1 I105V polymorphism and susceptibility to colorectal cancer in Kashmiri population. DNA Cell Biol. 31, 74–79. doi:10.1089/DNA.2011.1297
Schlicker, A., Beran, G., Chresta, C. M., McWalter, G., Pritchard, A., Weston, S., et al. (2012). Subtypes of primary colorectal tumors correlate with response to targeted treatment in colorectal cell lines. BMC Med. Genomics 5, 66. doi:10.1186/1755-8794-5-66
Schmidlin, T., Garrigues, L., Lane, C. S., Mulder, T. C., van Doorn, S., Post, H., et al. (2016). Assessment of SRM, MRM(3), and DIA for the targeted analysis of phosphorylation dynamics in non-small cell lung cancer. Proteomics 16, 2193–2205. doi:10.1002/PMIC.201500453
Shannon, P., Markiel, A., Ozier, O., Baliga, N. S., Wang, J. T., Ramage, D., et al. (2003). Cytoscape: A software environment for integrated models of biomolecular interaction networks. Genome Res. 13, 2498–2504. doi:10.1101/gr.1239303
Sillars-Hardebol, A. H., Carvalho, B., Belién, J. A. M., De Wit, M., Delis-Van Diemen, P. M., Tijssen, M., et al. (2012). CSE1L, DIDO1 and RBM39 in colorectal adenoma to carcinoma progression. Cell. Oncol. (Dordr). 35, 293–300. doi:10.1007/S13402-012-0088-2
Sun, K., Wang, S., He, J., Xie, Y., He, Y., Wang, Z., et al. (2017). NCOA5 promotes proliferation, migration and invasion of colorectal cancer cells via activation of PI3K/AKT pathway. Oncotarget 8, 107932–107946. doi:10.18632/ONCOTARGET.22429
Szklarczyk, D., Franceschini, A., Wyder, S., Forslund, K., Heller, D., Huerta-Cepas, J., et al. (2015). STRING v10: Protein-protein interaction networks, integrated over the tree of life. Nucleic Acids Res. 43, D447–D452. doi:10.1093/nar/gku1003
Szklarczyk, D., Gable, A. L., Lyon, D., Junge, A., Wyder, S., Huerta-Cepas, J., et al. (2019). STRING v11: Protein-protein association networks with increased coverage, supporting functional discovery in genome-wide experimental datasets. Nucleic Acids Res. 47, D607–D613. doi:10.1093/nar/gky1131
Ta, H. D. K., Wang, W. J., Phan, N. N., Ton, N. T. A., Anuraga, G., Ku, S. C., et al. (2021). Potential therapeutic and prognostic values of LSM family genes in breast cancer. Cancers (Basel) 13, 4902. doi:10.3390/CANCERS13194902
Tai, C. J., Su, T. C., Jiang, M. C., Chen, H. C., Shen, S. C., Lee, W. R., et al. (2013). Correlations between cytoplasmic CSE1L in neoplastic colorectal glands and depth of tumor penetration and cancer stage. J. Transl. Med. 11, 29. doi:10.1186/1479-5876-11-29
Tan, Y., Liu, F., and Xu, P. (2021). Knockdown of NCOA5 suppresses viability, migration and epithelial-mesenchymal transition, and induces adhesion of breast cancer cells. Oncol. Lett. 22, 694. doi:10.3892/OL.2021.12955
Tanaka, K., Ikeda, N., Miyashita, K., Nuriya, H., and Hara, T. (2018). DEAD box protein DDX1 promotes colorectal tumorigenesis through transcriptional activation of the LGR5 gene. Cancer Sci. 109, 2479–2489. doi:10.1111/CAS.13661
Tang, J., Chen, H., Wong, C. C., Liu, D., Li, T., Wang, X., et al. (2018). DEAD-box helicase 27 promotes colorectal cancer growth and metastasis and predicts poor survival in CRC patients. Oncogene 37, 3006–3021. doi:10.1038/S41388-018-0196-1
Thul, P. J., Åkesson, L., Wiking, M., Mahdessian, D., Geladaki, A., Ait Blal, H., et al. (2017). A subcellular map of the human proteome. Science 356, eaal3321. doi:10.1126/science.aal3321
Tian, Q. H., Zhang, M. F., Luo, R. G., Fu, J., He, C., Hu, G., et al. (2016). DHX33 expression is increased in hepatocellular carcinoma and indicates poor prognosis. Biochem. Biophys. Res. Commun. 473, 1163–1169. doi:10.1016/J.BBRC.2016.04.033
Tomczak, K., Czerwińska, P., and Wiznerowicz, M. (2015). The cancer genome atlas (TCGA): An immeasurable source of knowledge. Contemp. Oncol. Pozn. 19, 68–77. doi:10.5114/wo.2014.47136
Tsherniak, A., Vazquez, F., Montgomery, P. G., Weir, B. A., Kryukov, G., Cowley, G. S., et al. (2017). Defining a cancer dependency map. Cell 170, 564–576.e16. doi:10.1016/j.cell.2017.06.010
Uhlén, M., Fagerberg, L., Hallström, B. M., Lindskog, C., Oksvold, P., Mardinoglu, A., et al. (2015). Proteomics. Tissue-based map of the human proteome. Science 347, 1260419. doi:10.1126/science.1260419
Uhlen, M., Zhang, C., Lee, S., Sjöstedt, E., Fagerberg, L., Bidkhori, G., et al. (2017). A pathology atlas of the human cancer transcriptome. Science 357, eaan2507. doi:10.1126/science.aan2507
Vasaikar, S., Huang, C., Wang, X., Petyuk, V. A., Savage, S. R., Wen, B., et al. (2019). Proteogenomic analysis of human colon cancer reveals new therapeutic opportunities. Cell 177, 1035–1049.e19. doi:10.1016/J.CELL.2019.03.030
Wen, N., Bian, L., Gong, J., and Meng, Y. (2020). Overexpression of cell-cycle related and expression-elevated protein in tumor (CREPT) in malignant cervical cancer. J. Int. Med. Res. 48, 300060519895089. doi:10.1177/0300060519895089
Wielenga, V. J. M., Van der Neut, R., Offerhaus, G. J. A., and Pals, S. T. (2000). CD44 glycoproteins in colorectal cancer: Expression, function, and prognostic value. Adv. Cancer Res. 77, 169–187. doi:10.1016/S0065-230X(08)60787-3
Wurth, L., and Gebauer, F. (2015). RNA-binding proteins, multifaceted translational regulators in cancer. Biochim. Biophys. Acta - Gene Regul. Mech. 1849, 881–886. doi:10.1016/j.bbagrm.2014.10.001
Wurth, L., Papasaikas, P., Olmeda, D., Bley, N., Calvo, G. T., Guerrero, S., et al. (2016). UNR/CSDE1 drives a post-transcriptional program to promote melanoma invasion and metastasis. Cancer Cell 30, 694–707. doi:10.1016/j.ccell.2016.10.004
Xi, Y., and Xu, P. (2021). Global colorectal cancer burden in 2020 and projections to 2040. Transl. Oncol. 14, 101174. doi:10.1016/J.TRANON.2021.101174
Xing, Q., Liu, S., Luan, J., Wang, Y., and Ma, L. (2021). A novel 13 RNA binding proteins (RBPs) signature could predict prostate cancer biochemical recurrence. Pathol. - Res. Pract. 225, 153587. doi:10.1016/J.PRP.2021.153587
Xu, B., Yang, N., Liu, Y., Kong, P., Han, M., and Li, B. (2020). Circ_cse1l inhibits colorectal cancer proliferation by binding to eIF4A3. Med. Sci. Monit. 26, e923876. doi:10.12659/MSM.923876
Xu, H., Wong, C. C., Li, W., Zhou, Y., Li, Y., Wang, L., et al. (2021). RING-finger protein 6 promotes colorectal tumorigenesis by transcriptionally activating SF3B2. Oncogene 40, 6513–6526. doi:10.1038/S41388-021-01872-9
Xu, Y., Nijhuis, A., and Keun, H. C. (2022). RNA-binding motif protein 39 (RBM39): An emerging cancer target. Br. J. Pharmacol. 179, 2795–2812. doi:10.1111/BPH.15331
Yan, G., An, Y., Xu, B., Wang, N., Sun, X., and Sun, M. (2021). Potential impact of ALKBH5 and YTHDF1 on tumor immunity in colon adenocarcinoma. Front. Oncol. 11, 1. doi:10.3389/fonc.2021.670490
Yang, J. W., Yuan, L. L., Gao, Y., Liu, X. S., Wang, Y. J., Zhou, L. M., et al. (2021). 18 F-FDG PET/CT metabolic parameters correlate with EIF2S2 expression status in colorectal cancer. J. Cancer 12, 5838–5847. doi:10.7150/JCA.57926
Yang, Y. F., Lee, Y. C., Wang, Y. Y., Wang, C. H., Hou, M. F., and Yuan, S. S. F. (2019). YWHAE promotes proliferation, metastasis, and chemoresistance in breast cancer cells. Kaohsiung J. Med. Sci. 35, 408–416. doi:10.1002/KJM2.12075
Yin, Z., Yan, X., Wang, Q., Deng, Z., Tang, K., Cao, Z., et al. (2020). Detecting prognosis risk biomarkers for colon cancer through multi-omics-based prognostic analysis and target regulation simulation modeling. Front. Genet. 11, 524. doi:10.3389/fgene.2020.00524
Yu, C., Mannan, A. M., Yvone, G. M., Ross, K. N., Zhang, Y.-L., Marton, M. A., et al. (2016). High-throughput identification of genotype-specific cancer vulnerabilities in mixtures of barcoded tumor cell lines. Nat. Biotechnol. 34, 419–423. doi:10.1038/nbt.3460
Yu, W., Ling, J., Yu, H., Du, J., and Liu, T. (2020). AZGP1 suppresses the process of colorectal cancer after upregulating FASN expression via mTOR signal pathway. Gen. Physiol. Biophys. 39, 239–248. doi:10.4149/GPB_2019061
Zhang, B., Babu, K. R., Lim, C. Y., Kwok, Z. H., Li, J., Zhou, S., et al. (2020). A comprehensive expression landscape of RNA-binding proteins (RBPs) across 16 human cancer types. RNA Biol. 17, 211–226. doi:10.1080/15476286.2019.1673657
Zhang, H., Hou, W., Wang, H. L., Liu, H. J., Jia, X. Y., Zheng, X. Z., et al. (2014). GSK-3β-regulated N-acetyltransferase 10 is involved in colorectal cancer invasion. Clin. Cancer Res. 20, 4717–4729. doi:10.1158/1078-0432.CCR-13-3477
Zhou, Y., Cheng, X., Zhang, F., Chen, Q., Chen, X., Shen, Y., et al. (2020). Integrated multi-omics data analyses for exploring the co-occurring and mutually exclusive gene alteration events in colorectal cancer. Hum. Mutat. 41, 1588–1599. doi:10.1002/HUMU.24059
Keywords: RNA-binding proteins (RBPs), colorectal adenocarcinoma (COREAD), rectum, colon, biomarkers, cancer, data mining, multi-omics
Citation: García-Cárdenas JM, Armendáriz-Castillo I, García-Cárdenas N, Pesantez-Coronel D, López-Cortés A, Indacochea A and Guerrero S (2023) Data mining identifies novel RNA-binding proteins involved in colon and rectal carcinomas. Front. Cell Dev. Biol. 11:1088057. doi: 10.3389/fcell.2023.1088057
Received: 03 November 2022; Accepted: 13 February 2023;
Published: 13 June 2023.
Edited by:
Nadine Bley, University of Halle, GermanyReviewed by:
Markus Glaß, Martin Luther University of Halle-Wittenberg, GermanyTony Gutschner, Martin Luther University of Halle-Wittenberg, Germany
Copyright © 2023 García-Cárdenas, Armendáriz-Castillo, García-Cárdenas, Pesantez-Coronel, López-Cortés, Indacochea and Guerrero. This is an open-access article distributed under the terms of the Creative Commons Attribution License (CC BY). The use, distribution or reproduction in other forums is permitted, provided the original author(s) and the copyright owner(s) are credited and that the original publication in this journal is cited, in accordance with accepted academic practice. No use, distribution or reproduction is permitted which does not comply with these terms.
*Correspondence: Alberto Indacochea, indacochea@recerca.clinic.cat; Santiago Guerrero, sxguerrero@gmail.com