- 1Department of Hematology, Qilu Hospital, Cheeloo College of Medicine, Shandong University, Jinan, China
- 2School of Medicine, Cheeloo College of Medicine, Shandong University, Jinan, China
- 3Hematology and Oncology Unit, Department of Geriatrics, Qilu Hospital, Cheeloo College of Medicine, Shandong University, Jinan, China
- 4Department of Critical Care Medicine, Qilu Hospital of Shandong University, Jinan, China
- 5Shandong Key Laboratory of Immunohematology, Qilu Hospital, Cheeloo College of Medicine, Shandong University, Jinan, China
Acute myeloid leukemia (AML) is one of the most common hematopoietic malignancies in adults. The tumor microenvironment (TME) has a critical effect on AML occurrence, recurrence, and progression. The gene feline leukemia virus subgroup C cellular receptor family member 2 (FLVCR2) belongs to the major facilitator superfamily of transporter protein members, which is primarily involved in transporting small molecules. The potential role of FLVCR2 in the TME in AML has not been investigated. To clarify the expression and role of FLVCR2 in AML, we analyzed the Gene Expression Omnibus and The Cancer Genome Atlas databases and found that FLVCR2 mRNA expression significantly increased among patients with AML. Furthermore, based on an analysis of the Gene Expression Profiling Interactive Analysis database, FLVCR2 upregulation predicted dismal overall survival of patients with AML. Our validation analysis revealed the significant upregulation of FLVCR2 within the bone marrow of AML relative to healthy controls by western blotting and qPCR assays. Gene set enrichment analysis was conducted to explore FLVCR2’s related mechanism in AML. We found that high FLVCR2 expression was related to infiltration degrees of immune cells and immune scores among AML cases, indicating that FLVCR2 possibly had a crucial effect on AML progression through the immune response. Specifically, FLVCR2 upregulation was negatively related to the immune infiltration degrees of activated natural killer cells, activated memory CD4+ T cells, activated dendritic cells, and CD8+ T cells using CIBERSORT analysis. According to the in vitro research, FLVCR2 silencing suppressed AML cell growth and promoted their apoptosis. This study provides insights into FLVCR2’s effect on tumor immunity, indicating that it might serve as an independent prognostic biomarker and was related to immune infiltration within AML.
Introduction
Acute myeloid leukemia (AML) is a frequently observed and highly heterogeneous adult hematologic malignancy, and its features include clonal expansion, impaired differentiation, and apoptosis evasion in normal hematopoietic stem cells (Kumar, 2011; Chen et al., 2019). Therapeutic strategies for AML include traditional chemotherapy, hematopoietic stem cell transplantation, and immunotherapy. Despite the use of new therapeutic targets and drugs, the mortality rate of AML remains high. Long-term overall survival (OS) is less than 40% in patients aged under 60 years and less than 15% in patients aged over 60 years (Dohner et al., 2015). Therefore, defining the mechanisms underlying AML progression and improving the prognosis is necessary.
The causes of AML are typically related to genetic mutations and immune system deficiency. The tumor microenvironment (TME) affects AML pathogenesis and development (Carter et al., 2019; van Galen et al., 2019). TME components, such as changed extracellular matrix, suppressive immunocytes, and soluble factors, have been shown to inhibit tumor immunotherapy and promote AML progression (Ladikou et al., 2020). The immunosuppressive microenvironment mainly contains reduced natural killer (NK) cells and cytotoxic T lymphocytes and the accumulation of myeloid-derived suppressor cells, macrophages, and regulatory T cells (Tregs) (van Galen et al., 2019). Immunocyte-based therapy has gained attention as a possible approach to antitumor therapy. However, immunotherapy has shown less efficacy for AML than for other solid cancers (Austin et al., 2016; Khaldoyanidi et al., 2021). Furthermore, for AML, there is no accurate prognostic model based on the immune microenvironment. Consequently, identifying possible markers for diagnosing and treating AML to improve patient prognosis is urgently necessary.
Feline leukemia virus subgroup C cellular receptor family member 2 (FLVCR2) belongs to the major facilitator superfamily (MFS) of transporter protein members that transport small molecules (Pao et al., 1991). MFS is vital to the movement of various substrates across membranes and for lipid synthesis, which are essential for immune homeostasis (Quistgaard et al., 2016; Zhou et al., 2019; Fu et al., 2021). FLVCR2 is widely expressed in animal tissues, such as the brain, placenta, lung, liver, and kidneys (Duffffy et al., 2010). FLVCR2 transporter controls calcium entering target cells and participates in regulating calcium metabolism and development (Brasier et al., 2004). Moreover, FLVCR2 has been shown to be important for heme transport (Duffffy et al., 2010). In humans, FLVCR2 mutations are related to Fowler syndrome, the cerebral proliferating vascular disease (Meyer et al., 2010). Additionally, FLVCR2 has been demonstrated as a potential biomarker for predicting prostate cancer progression, especially at stage II (Alkhateeb et al., 2019). Evidence on the role of FLVCR2 in AML is limited, and the biological functions of FLVCR2 in AML remain unclear; thus, further research is necessary.
This work comprehensively analyzed the FLVCR2 level within AML by referring to several public databases on public bioinformatics platforms. The results showed that FLVCR2 had high expression within AML, which predicted low survival rates. Pathway analysis showed that FLVCR2 was related to TME and immune-related pathways. Moreover, high FLVCR2 expression was related to infiltration degrees of immune cells and immune scores for AML cases, indicating that FLVCR2 possibly had an important effect on AML progression through immune reaction. FLVCR2 silencing suppressed AML cells proliferation and promoted their apoptosis. Our results provide insights into the AML tumorigenesis mechanism and identify FLVCR2 as the significant independent predictor of AML.
Materials and methods
Data acquisition
This study obtained transcriptome RNA sequencing (RNA-seq) and relevant clinical data for the tumor and normal samples from The Cancer Genome Atlas (TCGA) database (https://portal.gdc.cancer.gov) and then eliminated cases that had incomplete clinical data. This study gained approval from the Ethics Committee of Qilu Hospital (Jinan, China). TCGA database is publicly available under specific guidelines, and the analysis in this study was conducted following the Declaration of Helsinki. This study also obtained the GSE12417 dataset (Metzeler et al., 2008) from the Gene Expression Omnibus (GEO) database (http://www.ncbi.nlm.nih.gov/geo/) to assess the relation between FLVCR2 expression and prognosis.
Detection of differential expression of FLVCR2 in AML
This study used the Gene Expression Profilin Interactive Analysis (GEPIA) online database (http://gepia.cancer-pku.cn/index.html) to evaluate the association of FLVCR2 level with clinicopathological features of AML (Tang et al., 2017). Differential gene expression between patients with AML and normal controls was analyzed using the “Expression DIY” module of GEPIA, matching TCGA-derived Genotype-Tissue Expression Project (GTEx) and normal data, as well as log2 (TPM +1). The “Survival” module in GEPIA was used to evaluate the relation between FLVCR2 level and AML survival.
Gene set enrichment analysis for FLVCR2
This study conducted GSEA based on TCGA-derived RNA-seq data after normalization. GSEA was conducted using the R package “clusterProfiler” (v4.0.3) to identify the potential biological processes and pathways related to FLVCR2 expression in AML progression (Subramanian et al., 2005). This study obtained the HALLMARK gene set from the Molecular Signatures Database. Analyses were repeated 1,000 times. A threshold for significant enrichment was set as a false discovery rate (FDR) < 0.05 and p < 0.05.
Survival analysis of FLVCR2 expression in AML
This study used the online database Kaplan—Meier plotter (www.kmplot.com), including clinical and gene expression profiles (Gyorffy et al., 2013); the TIMER 2.0 online platform (http://timer.comp-genomics.org/) (Li et al., 2020); and PrognoScan (http://dna00.bio.kyutech.ac.jp/PrognoScan/) (Mizuno et al., 2009) to evaluate whether FLVCR2 level predicted AML prognosis. We used an AML dataset to explore the effect of FLVCR2 expression on OS in AML cases. This study also determined hazard ratios and log-rank p values. P < 0.05 was the statistical significance.
Functional annotations
Differentially expressed genes (DEGs) were conducted using Gene Ontology (GO), and Kyoto Encyclopedia of Genes and Genomes (KEGG) analysis with the DAVID database (v6.8; https://david.ncifcrf.gov/summary.jsp) (Huang et al., 2009): p < 0.05 was the significance level. The GO enrichment analysis of FLVCR2 revealed biological process (BP), cellular component (CC), and molecular function (MF) terms. Pathways related to FLVCR2 activity along and its co-expression genes were identified by KEGG analysis. The results of enrichment analysis are shown using corrected p values and normalized enrichment scores.
Protein—protein interaction network analysis of FLVCR2
This study applied the database Search Tool for the Retrieval of Interacting Genes/Proteins (STRING) (https://string-preview.org/), used for retrieving interacting genes and PPI networks, to map the FLVCR2 gene and protein interactions. Significant enrichment was assessed by the thresholds of FDR < 0.05 and p < 0.01.
Immune infiltration analysis
“ESTIMATE” in an R package (Yoshihara et al., 2013) was employed to evaluate the stromal and immune scores of tumor samples. We divided samples into two groups according to the median stromal/immune scores. The difference in the survival probability between the two groups was assessed by Cox regression analysis. Additionally, to evaluate the changes in gene levels in samples, the gene level-based deconvolution algorithm CIBERSORT (http://cibersort.stanford.edu/) was used (Bense et al., 2016; Liu et al., 2017). Proportions of 22 immune cell types within AML were examined through CIBERSORT to assess their correlation with survival probabilities. The relation between FLVCR2 level and immunocyte infiltration degrees was analyzed. Additionally, the Wilcoxon rank-sum test was conducted to compare immunocyte infiltration degrees between low- and high-FLVCR2 expression samples. A p value of <0.05 was the threshold for selecting lymphocytes that might be affected by FLVCR2 level.
Patient specimens
This study acquired bone marrow from 30 subjects with AML and 24 normal subjects, both from Qilu Hospital of Shandong University, China. Next, mononuclear cells were isolated using Ficoll—Paque centrifugation. All subjects provided informed consent as stipulated in the Declaration of Helsinki. Each laboratory experiment using primary specimens gained approval from the Medical Ethics Committee of Qilu Hospital of Shandong University.
Cell culture and transfection
This study obtained human leukemic MOLM-13 and THP-1 cells from the Institute of Hematology and Blood Diseases Hospital, Chinese Academy of Medical Sciences and Peking Union Medical College, Tianjin, China. Next, cells within RPMI-1640 that contained 10% fetal bovine serum were cultivated. FLVCR2 gene sequences were later searched against the National Center for Biotechnology Information’s Gene database. In addition, RNA interference (RNAi) sequences were designed based on the appropriate design principles. GenePharma (Shanghai, China) was responsible for preparing the negative control (NC) and FLVCR2-targeting small interference RNA (siRNA). Lipofectamine RNAiMAX (Invitrogen) was used for transfection within the 6-well plates.
Quantitative reverse-transcription PCR
This study used TRIzol (Invitrogen) to extract total RNA according to specific protocols and measured it using UV spectrophotometry (DeNovix). Subsequently, an RNA sample (1,000 ng) was collected to prepare cDNA through reverse transcription in 10 μl reaction volume using Evo M-MLV RT Premix for qPCR (AG11706). The SYBR Green Premix Pro Taq HS qPCR Kit (AG11701) was applied in RT-qPCR on the Light Cycler 480 Ⅱ (Roche). The results were calculated by the 2^-ΔΔCT method. GAPDH was used as endogenous control.
Western blotting assay
M-PERTM Mammalian Protein Extraction Reagent (Thermo Fisher) was used for cell lysis. After separation through SDS-PAGE, proteins were transferred onto nitrocellulose membranes (Millipore). This study used GAPDH as the reference. Anti-FLVCR2 (proteintech) and anti-GAPDH (ZSGB-BIO China) primary antibodies were used. Next, this assay was incubated with HRP Substrate Luminol Reagent (Millipore), and exposure was performed with ChampChemi (SageCreation).
Cell proliferation test
The proliferation of transfected cells in vitro was analyzed by EdU cell proliferation assay. For EdU assays, treated cells were incubated by the iClick™ EdU Andy Fluor™ 647 Flow Cytometry Assay Kit (GeneCopoeia, A008) and tested by flow cytometry (FCM).
Cell apoptosis analysis
After transfection, supernatants of the cell culture were eliminated before cell collection. After washing with phosphate-buffered saline twice, the cells were added with 1× binding buffer (300 μl). Next, the cells were subject to Annexin V/PI staining (BestBio, Shanghai, China) and FCM (Beckman Coulter) analysis of cell apoptosis within 1 h.
Statistical analysis
This study used R software (version 3.6.3) for statistical analysis. The relation between FLVCR2 level and clinical features was analyzed by logistic regression analysis. OS was defined as the duration between the diagnosis date and the all-cause mortality date. This study conducted Cox proportional hazards regression to identify OS-related clinical characteristics in patients with AML. p < 0.05 (two-sided) denoted statistical significance.
Results
FLVCR2 expression is upregulated in AML
For the exploration of key genes within AML, DEGs were analyzed in the TCGA dataset, and the significance of these genes was tested using immune scores and AML prognosis. FLVCR2 was one of the six overlapping genes among the MFS members (Figure 1A). The other five were MFSD2A, SLC16A2, SLC16A3, SLC17A3, and SLC43A2 (p < 0.05, |log2FoldChange| > 1). We focused on the function of FLVCR2 in the tumorigenesis of AML, and FLVCR2 showed upregulation within AML (Figure 1B). Furthermore, the relation between FLVCR2 and patient clinical features, including race, gender, age, treatment, and neoadjuvant, was investigated (Table 1). The FLVCR2 level showed a strong relation to neoadjuvant and treatment (p < 0.05). Additionally, the proportion of AML cases with low FLVCR2 expression was higher than that with high FLVCR2 expression without treatment or neoadjuvant. However, no other differences were observed between low- and high-FLVCR2 expression groups.
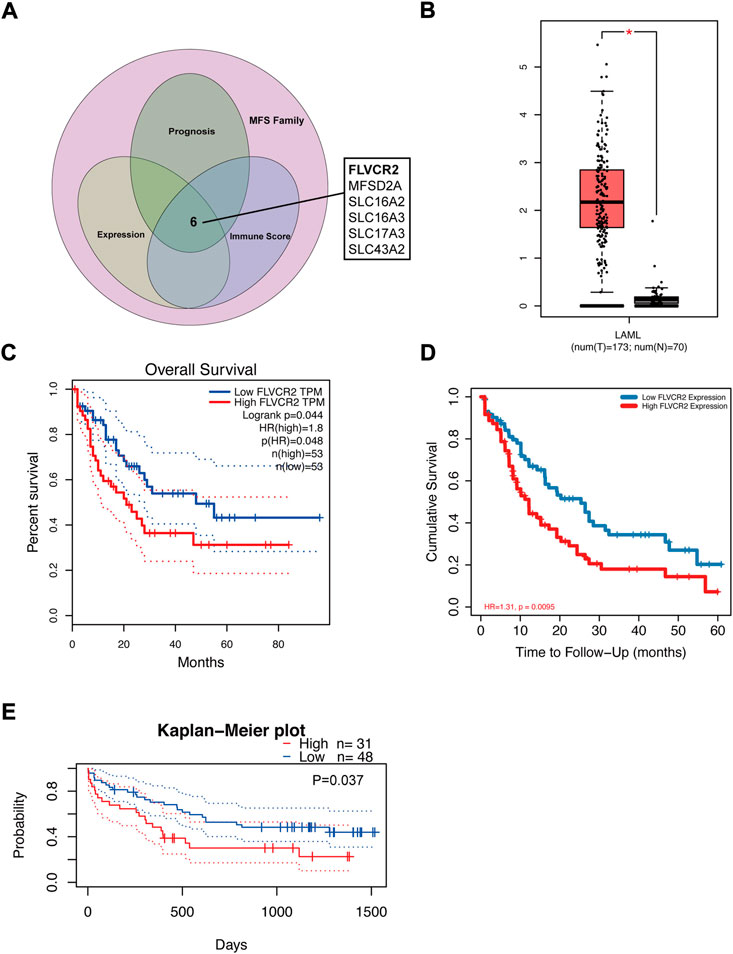
FIGURE 1. FLVCR2 expression is upregulated in AML and FLVCR2 level correlates with prognosis of AML. (A) Schematic graph of screening of MFS Family associated with AML immune score and prognosis. (B) The mRNA expression of FLVCR2 in TCGA AML samples (n = 173) and the GTEx normal samples (n = 70). (C) and (D) The overall survival analyses of FLVCR2 in AML via GEPIA and TCGA online platform. Blue: low FLVCR2 expression; Red: High FLVCR2 expression. (E) Comparing the high and low expression levels of FLVCR2 in AML patients with Kaplan—Meier OS curves by PrognoScan (GSE12417-GPL570) *p < 0.05; **p < 0.01, ***p < 0.001.
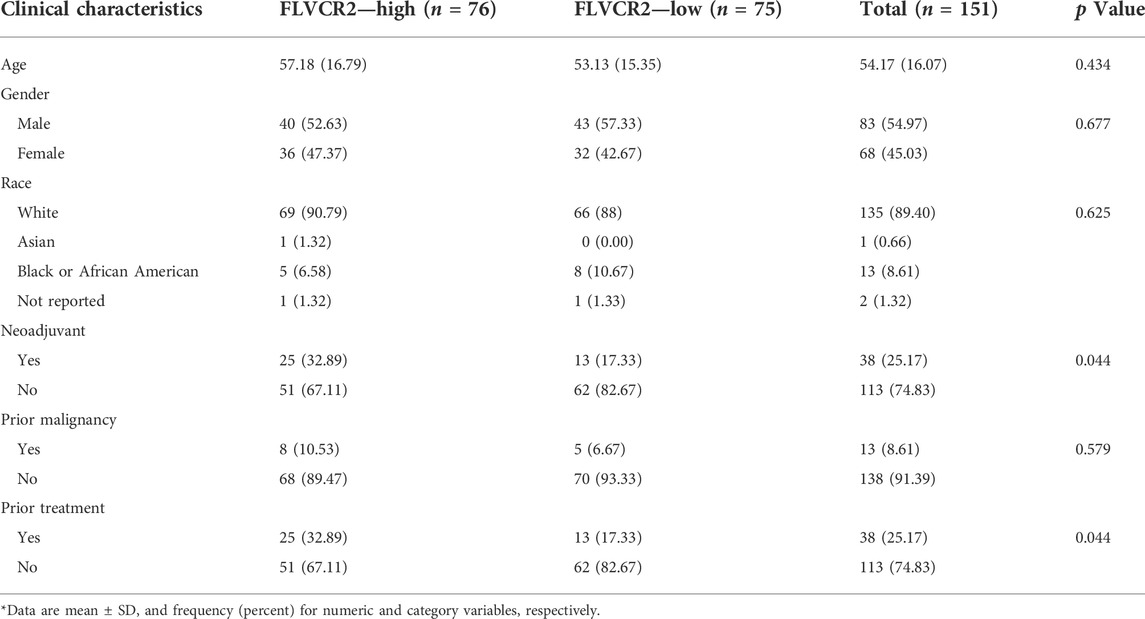
TABLE 1. Description of clinical characteristics for 151 AML sample in both FLVCR2 high/low groups from TCGA.
FLVCR2 level correlates with prognosis of AML
To further understand FLVCR2’s effect on AML, its prognostic role in AML was assessed using the GEPIA database and RNA-seq data. The results showed that high FLVCR2 expression levels predicted poor OS of the patients with AML (Figure 1C). Furthermore, we used TCGA database to assess the correlation with FLVCR2 level and FLVCR2’s prognostic significance for AML. According to the results, FLVCR2 expression in patients with AML was higher than that in normal controls. According to the median expression level of FLVCR2, we divided the AML patients into high FLVCR2 expression group (n = 76) and low FLVCR2 expression group (n = 75). We found that the low FLVCR2 expression was correlated with superior OS in the median cutoff groups in AML (Figure 1D). We also studied the relation between FLVCR2 level and prognostic outcome by using the GSE12417-GPL570 dataset (Figure 1E). Comparing the high and low expression levels of FLVCR2 in AML patients with Kaplan–Meier OS curves by PrognoScan, high FLVCR2 expression levels were also corresponded with poor OS prognosis in AML (Figure 1E). Based on the aforementioned findings, FLVCR2 level was significantly related to AML prognosis and progression.
Protein—protein interaction network and functional annotation of FLVCR2 in AML
Next, we constructed a protein—protein interaction network based on DEGs by using the STRING database to screen for FLVCR2-binding proteins. The results showed that a subset of immune-related proteins interacted with FLVCR2, including HMGCR and CORO1A (Figure 2A). In AML, HMGCR and CORO1A showed a significant correlation with FLVCR2. Studies have showed that HMGCR and CORO1A were involved in regulating T-cell homeostasis, which was important for the tumor microenvironment. These findings made us interested in the role of FLVCR2 in immunity in AML. FLVCR2 might affect the immunomodulation and progression of AML.
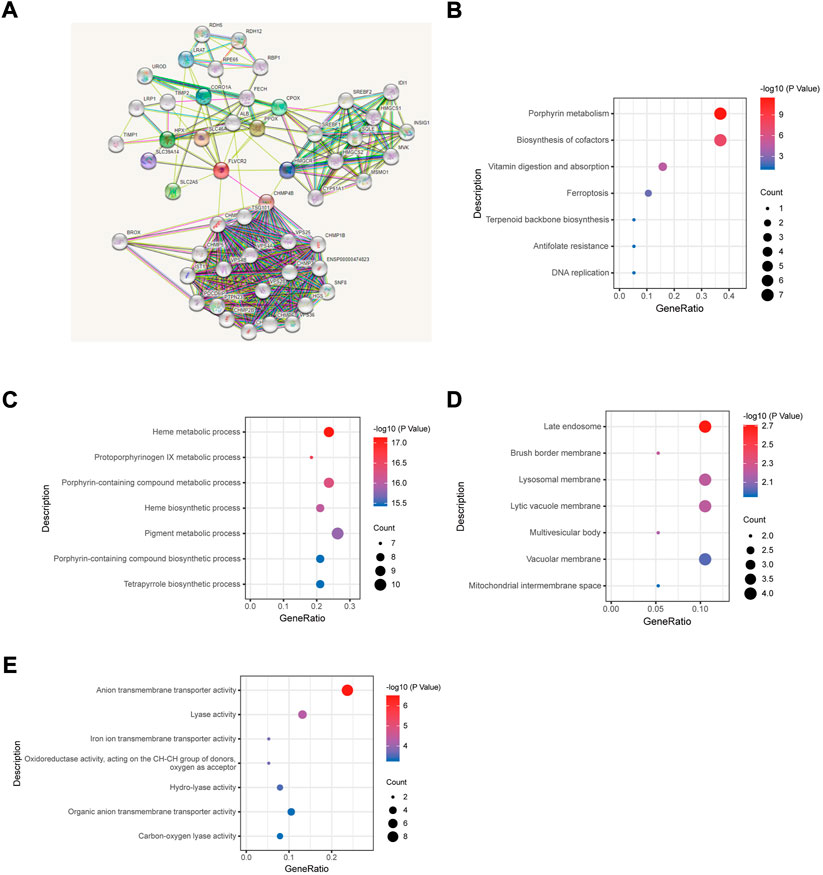
FIGURE 2. Protein—protein interaction network and functional annotation of FLVCR2 in AML. (A) Integrated protein-protein interaction network of FLVCR2 via STRING. (B) Top seven enriched KEGG pathway distribution. (C–E) GO enrichment analysis result of cellular component, molecular function, and biological process.
To understand FLVCR2’s functional implications within AML between two groups, we performed GO and KEGG pathway enrichment analyses. By means of GO enrichment analysis, we filtered the top seven cellular components (CC), biological process (BP), and molecular function (MF) categories based on the following criteria: p value <0.01 and FDR <0.05 (Figures 2C–E). The GO-BP terms involved in the metabolic process were discovered. Additionally, GO-CC terms were related to transporter activity. Moreover, KEGG pathway analysis (Figure 2B) indicated the enrichment of metabolism, ferroptosis, and DNA replication pathways, which were key pathways closely related to tumorigenesis. This may explain the correlation between the FLVCR2 higher expression level and poor prognosis in AML.
FLVCR2 regulates immune response in AML
To understand FLVCR2’s functional implications within AML between two groups, we conducted GSEA for FLVCR2, based on LinkedOmics database, to investigate the possible BPs and pathways. Based on the normalized enrichment scores, the most significantly enriched signaling pathway in terms of FLVCR2 gene expression was selected. As shown in Figures 3A,B, high levels of FLVCR2 were enriched in immunoactivity-related pathways, including the immune response signaling pathway, intercellular adhesion of leukocytes, leukocyte activation regulation, positive cytokine production regulation, and neutrophil-mediated immunity. The analysis also revealed categories negatively correlated with high FLVCR2 levels: methylation, RNA catabolic processing, mRNA processing, tRNA metabolic process, protein-DNA complex subunit organization, and ribonucleoprotein complex biogenesis. These results prompted us to conduct a subsequent study on the effect of FLVCR2 on immune infiltration in AML.
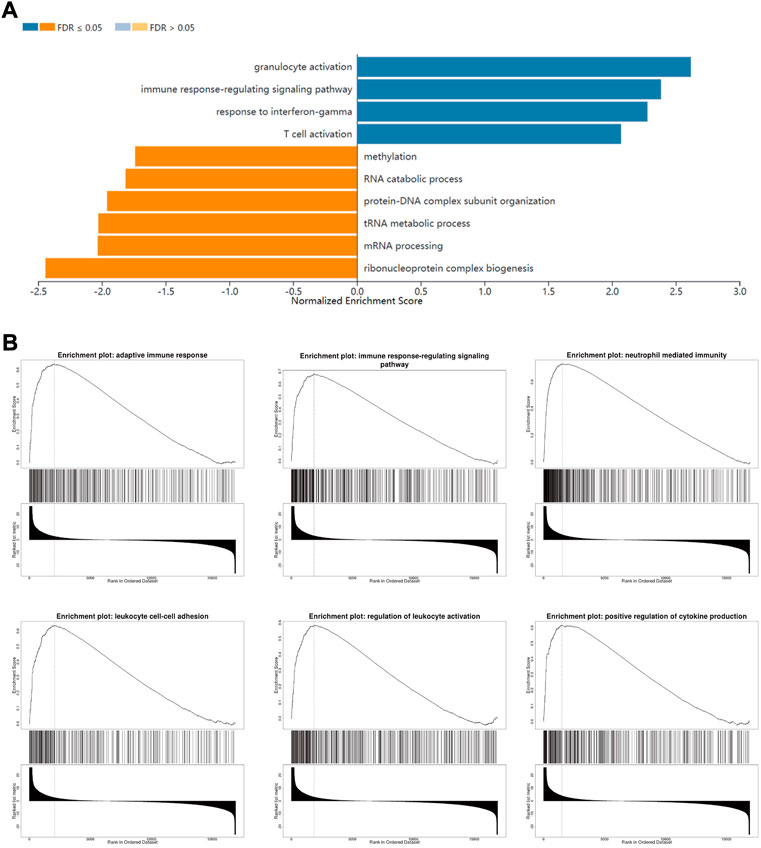
FIGURE 3. FLVCR2 regulates immune response in AML. (A) Significantly enriched biological processes of GSEA annotations of FLVCR2 in AML (LinkedOmics). (B) GSEA enrichment plots of specified biological processes.
Relation between FLVCR2 expression and TME
FLVCR2 level has been suggested to be associated with immune pathways in AML. Because immune and stromal cells represent the main TME components, this study analyzed the effect of FLVCR2 on TME. First, the stromal and immune scores of AML cases were determined. Next, the effects on the prognostic outcome were assessed. The results showed that low immune scores predicted improved prognosis, but stromal scores were not significantly related to OS (Figures 4A,B). Further correlation analysis showed that FLVCR2 expression showed a positive relation to immune score, with significant difference detected through dichotomization (Figures 4C,D). This result suggests the role of FLVCR2 in affecting TME activity and immunocyte infiltration.
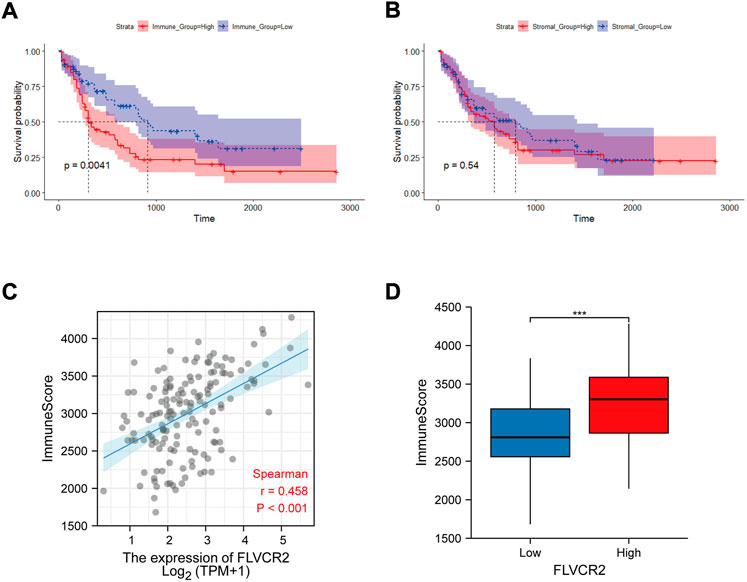
FIGURE 4. Relation between FLVCR2 expression and tumor immune environment. (A,B) Correlation analysis between OS and immune score (left) or stromal score (right). (C,D) Correlation between FLVCR2 and immune score by linear graph (left) or dichotomy graph (right). *p < 0.05; **p < 0.01, ***p < 0.001.
FLVCR2 level relates to immunocyte infiltration in AML
Next, we examined the association between FLVCR2 mRNA level and immunocyte infiltration. According to correlation analysis, FLVCR2 expression showed a positive relation to the infiltration degrees of monocytes and macrophages M2 (Figure 5A). Furthermore, this study used the CIBERSORT algorithm to explore gene levels in the samples to calculate scores for 22 immunocyte subtypes between low and high FLVCR2 expression groups (Figure 5B). As shown in Figure 5B, plasma cells, naive B cells, CD8+ T cells, resting NK cells, CD4+ memory T cells, monocytes, mast cells, and M2 macrophages represented the main immune cells affected by FLVCR2 level. For AML cases, FLVCR2 upregulation was significantly related to the high proportion of monocytes. However, FLVCR2 upregulation was negatively associated with some immunocyte types, such as plasma cells, activated CD4 T cells memory, activated NK cells, CD8+ T cells, M1 macrophages, and activated dendritic cells (Figure 5C). The results showed that FLVCR2 expression was significantly related to the immune infiltration level, which might contribute to an immune inhibitory phenotype of TME in AML. These findings led a heavy hint that FLVCR2 affects patient survival via interacting with immune infiltration in AML.
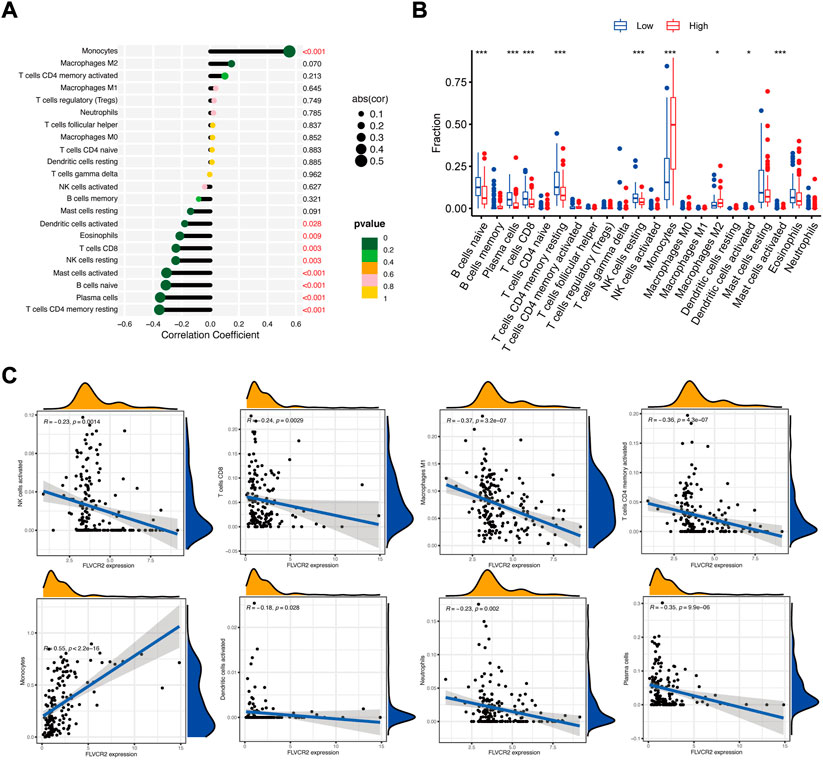
FIGURE 5. FLVCR2 level relates to immunocyte infiltration in AML. (A) FLVCR2 is correlated with immune infiltration in AML. (B,C) Correlations between FLVCR2 expression and the infiltration abundances of selected immune cells in AML through CIBERSORT algorithm. *p < 0.05; **p < 0.01, ***p < 0.001.
FLVCR2 differential analysis among several cancers
For exploring FLVCR2 expression between cancer and healthy samples from several cancers, this study used web server TCGA and GTEx. FLVCR2 expression increased in tumors, such as renal papillary cell carcinoma, renal clear cell carcinoma, and low-grade glioma (LGG), compared to that in matched healthy samples (Figure 6A), and FLVCR2 downregulation predicted the dismal OS for 518 LGG cases (Figure 6B). However, the expression level of FLVCR2 in skin cutaneous melanoma (SKCM) decreased relative to that in healthy samples, and low FLVCR2 expression predicted poor SKCM patient survival (Figure 6C).
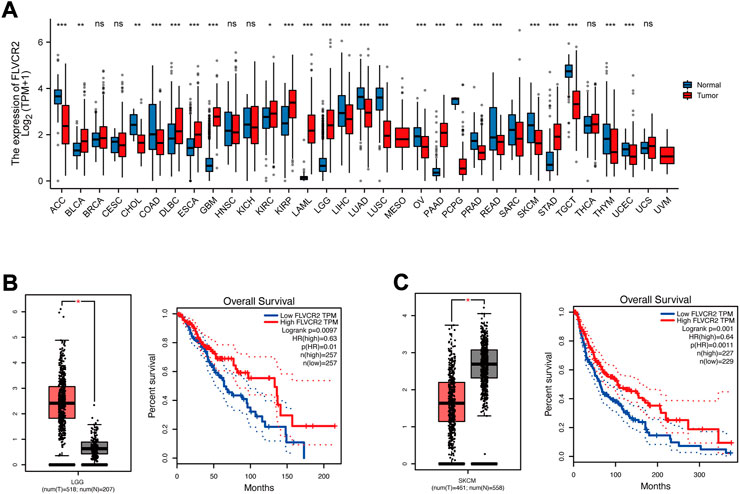
FIGURE 6. FLVCR2 differential analysis among several cancers. (A) The mRNA expression of the FLVCR2 expression profile across multiple tumor samples (red) and paired normal tissues (blue). (B) The mRNA expression of FLVCR2 in LGG samples (tumor samples in red from TCGA, and normal samples in gray from GTEx). The overall survival analyses of FLVCR2 in LGG. Blue: low FLVCR2 expression; Red: High FLVCR2 expression. (C) The mRNA expression of FLVCR2 in SKCM samples (tumor samples in red from TCGA, and normal samples in gray from GTEx). The overall survival analyses of FLVCR2 in SKCM. Blue: low FLVCR2 expression; Red: High FLVCR2 expression. *p < 0.05; **p < 0.01, ***p < 0.001.
FLVCR2 shows high expression in primary AML specimens
This study measured FLVCR2 mRNA expression in bone marrow specimens collected from AML cases (n = 30) and healthy subjects (n = 24). Table 2 displays clinical features. The FLVCR2 level significantly elevated in AML bone marrow in comparison with that of controls (p < 0.01, Figure 7A). The expression of FLVCR2 depended on the efficacy of the therapy. The FLVCR2 levels were significantly reduced during therapy when patients achieved complete remission but remained constant in those with no remission. FLVCR2 expression was higher in relapsed or refractory AML cases (n = 15, p < 0.01) than in those achieving complete response (n = 15, Figure 7B).
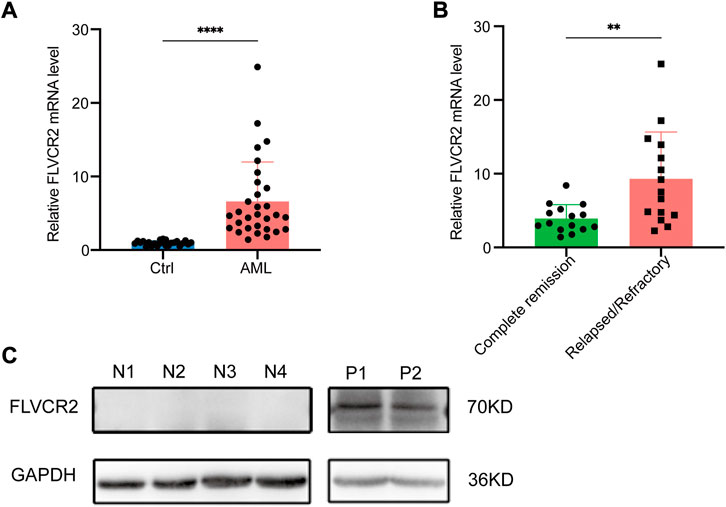
FIGURE 7. FLVCR2 shows high expression within primary AML specimens. (A) Quantitative mRNA expression of FLVCR2 by RT-qPCR in AML patient samples (n = 30) and controls (Ctrl, n = 24). (B) Quantitative mRNA expression of FLVCR2 in patients with relapsed or refractory AML (n = 15) and patients with complete remission (n = 15). *p < 0.05; **p < 0.01, ***p < 0.001. (C) Representative western blotting analysis of FLVCR2 protein level in patients with newly-diagnosed AML (P1 and P2, n = 2) relative to control (N1-N4, n = 4). In A and B, p values were determined by two-sided unpaired t-test.
Furthermore, we detected the FLVCR2 protein expression levels, and confirmed that the protein level of FLVCR2 was increased in AML patients compared with that in healthy donors (Figure 7C). Based on the aforementioned results, FLVCR2 level was associated with treatment effect.
FLVCR2 inhibition suppresses AML cells proliferation and promotes their apoptosis
To improve the exploration of FLVCR2’s function during AML progression, we prepared siRNAs targeting FLVCR2 (si-FLVCR2) and NC siRNAs (si-NC). The FLVCR2 level markedly elevated within THP-1and MOLM-13 cells in comparison with that of heathy controls (p < 0.01, Figure 8A). Next, we examined siRNA knockdown efficiency. The results showed that transfection with si-FLVCR2 significantly decreased the FLVCR2 level within THP-1 and MOLM-13 cells relative to si-NC (Figure 8B). The proliferation of THP-1 and MOLM-13 cells was examined using the EdU proliferation assay. The results showed that si-FLVCR2 transfection markedly suppressed the proliferation of THP-1 and MOLM-13 cells (p < 0.05, Figures 8C,D). FCM was conducted to analyze THP-1 and MOLM-13 cell apoptosis after FLVCR2 interference. The results showed that FLVCR2 interference promoted THP-1 and MOLM-13 cell apoptosis (Figures 8E,F). In conclusion, FLVCR2 has a critical effect in regulating cell apoptosis and maintaining cell proliferation in AML.
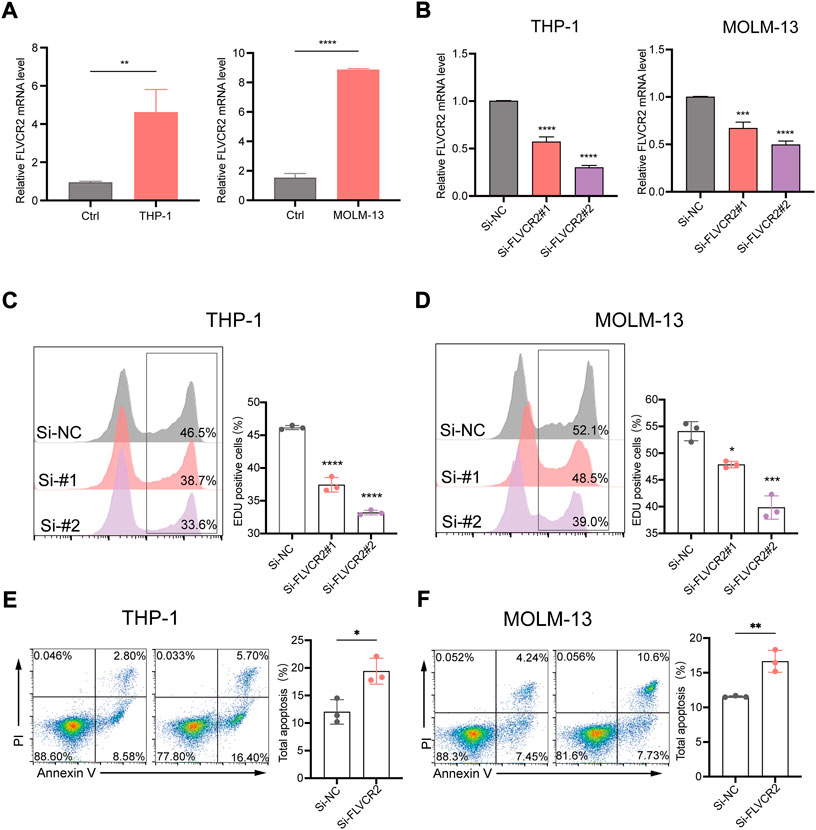
FIGURE 8. FLVCR2 inhibition suppresses AML cell proliferation and promotes their apoptosis. (A) Quantitative mRNA expression of FLVCR2 by RT-qPCR in THP-1, MOLM-13 cells and controls. (B) THP-1 and MOLM-13 cells were transfected with si-NC, si-FLVCR2#1, or si-FLVCR2#2. Knockdown efficiency of FLVCR2 in cells was measured by RT-qPCR. (C,D) Cell proliferation was evaluated by EdU assay, and si-FLVCR2 inhibited THP-1 and MOLM-13 cell proliferative activities compared with the si-NC group. (E,F) After 48 h of transfection, cell apoptosis was measured by flow cytometry. The cell apoptotic rates between si-NC and si-FLVCR2 groups were analyzed in THP-1 and MOLM-13 cells. N = 3.*p < 0.05; **p < 0.01, ***p < 0.001. In B-D, p values were determined by one-way ANOVA; In Aand E-F, p values were determined by two-sided unpaired t-test.
Discussion
AML is a highly heterogeneous, malignant tumor with varied prognoses. The pathogenic mechanisms underlying AML have not been fully elucidated. Therefore, novel biomarkers are necessary to understand mechanisms related to AML. FLVCR2 was reported to be associated with Fowler syndrome (Meyer et al., 2010). Subsequently, FLVCR2 was found to be a potential biomarker for predicting prostate cancer (Alkhateeb et al., 2019). Nonetheless, its effect on AML remains unclear. In this study, FLVCR2 level was considered an essential predictor of clinical outcomes of AML.
The study examined FLVCR2’s level, prognostic significance, and potential mechanism in AML by using TCGA datasets. The results showed that FLVCR2 mRNA levels increased more in AML samples than in normal samples according to the results obtained through GEPIA and GEO database. FLVCR2 level elevated in relapsed/refractory AML cases relative to complete remission AML cases, suggesting that FLVCR2 level might be related to treatment efficacy and prognostic value. We then performed prognostic analysis and found that FLVCR2 upregulation was associated with poor prognosis, suggesting that FLVCR2 functions as an oncogene. DEGs associated with increased FLVCR2 levels were enriched in immunity-associated pathways. Our findings indicate that FLVCR2 may be an independent prognostic factor that influences tumorigenesis and tumor immunology in AML progression. However, its biological involvement in AML remains to be explored.
Furthermore, we investigated the biological functions and mechanisms of action of FLVCR2 in AML. The literature (Pao et al., 1998) showed that FLVCR2 was an MFS transporting small substances across the membranes upon chemiosmotic gradient stimulation, which helped to maintain homeostasis in cells. In our study, the functional annotation of FLVCR2 revealed that cell–cell adhesion, cellular defense response, translational initiation, and ribonucleoprotein complex biogenesis were closely associated with FLVCR2 expression. Studies have shown that adhesion molecules are closely related to tumorigenesis and development (Sisto et al., 2021; Ito et al., 2022), which is consistent with our results. The cell–cell adhesion events enriched in the high FLVCR2 expression group show that FLVCR2 plays a critical role in AML tumorigenesis. Therefore, although the precise mechanisms of tumor progression have not been elucidated, the aforementioned signaling pathways were confirmed to be related to AML.
Moreover, PPI network analysis revealed that CORO1A and HMGCR had a low but significant correlation with FLVCR2. CORO1A, responsible for encoding the Trp-Asp repeat protein, is related to different cell events. CORO1A depletion possibly results in compromised T-cell activity and homeostasis due to the defect in the T-cell receptor pathway (Mueller et al., 2008). Additionally, HMGCR primarily regulates the activation, survival, progression, and resistance of T lymphocytes. The changed HMGCR level regulates immune function, and the metabolism status of T cells. HMGCR synthetase represents the enzyme involved in purine biosynthesis pathway, which is related to increased cancer phenotypes such as tumor cell invasion and growth. These findings further highlight that FLVCR2 has an important effect on the genesis and immunomodulation of AML.
Immune-based therapies have become important in cancer treatment (Abril-Rodriguez and Ribas, 2017). However, patients receiving immunotherapy finally experience drug resistance because of immune escape. TME has gained attention in mediating immune reactions in cancer, and targeting TME components is expected to be a novel treatment strategy for AML. Studies have verified that the interactions between TME and AML are mainly mediated by various immune cells, which have critical effects on cancer immune escape (Li et al., 2020). TME is mainly composed of myeloid-derived suppressor cells, macrophages, and tumor-infiltrating lymphocytes (TILs) (Mitteldorf et al., 2017). TILs represent a key part of the TME and are classified into two types: tumor-antagonizing and tumor-promoting immune cells (Lei et al., 2020). Studies have found that TME contributes to immunological changes during AML progression (Tettamanti et al., 2022). Therefore, this study analyzed the relation between FLVCR2 and immune infiltration within AML. According to our review of the literature, this study is the first to support the link between FLVCR2 and tumor immunity in AML. We found that high FLVCR2 expression showed a negative relation to NK cells, CD4+ T cells, and CD8+ T cells, whereas a positive relation was observed with Tregs and M2 macrophages in AML. Tumor eradication by CD8+ T cells involves tumor antigens, antigen presentation, T-cell activation, and tumor cell killing (Steven et al., 2016). The low NK cell and CD8+ T cell proportions within tumors predict a dismal AML prognosis (Zhou et al., 2011; Truxova et al., 2020). By contrast, some articles have suggested that a high tumor-infiltrating Tregs proportion predicts a poor outcome of AML (Shenghui et al., 2011). Therefore, upregulated FLVCR2 probably suppresses tumor immunity, which contributes to the escape of tumor cells and promotes carcinogenesis, indicating a possible mechanism by which FLVCR2 affects the OS of patients with AML. The in vitro results demonstrated that FLVCR2 interference inhibited cell proliferation activities and increased the incidence of apoptosis, verifying that FLVCR2 was important for regulating cell apoptosis and maintaining cell proliferation within AML.
Previous studies have confirmed the importance of identifying a biomarker for tumorigenesis and development of particular cancer by combining bioinformatics analysis and experimental demonstration. Gao et al. (2021) found that SLC2A3 was correlated with EMT and immune signature in colorectal cancer. Fan et al. (2022) verified that SLC25A38 was a novel biomarker for metastasis and clinical outcome in uveal melanoma. Our study focused on identifying FLVCR2 as a novel biomarker for AML, which may be an independent prognostic factor that influenced tumorigenesis and tumor immunology in AML progression. Despite our findings, this study has limitations. Hence, further investigations should analyze mechanisms related to FLVCR2-medicated tumor immunity and biological functions in vivo.
In conclusion, this study first identifies the prognostic value of FLVCR2 in patients with AML. FLVCR2 expression is associated with immunocyte infiltration, such as NK cells, T cells, or macrophages in AML. Our results suggest that FLVCR2 may predict treatment outcomes and prognosis in patients with AML and play a critical role in regulating cell apoptosis and maintaining cell proliferation within AML. With an improved understanding of its function, FLVCR2 may be developed as a component of targeted therapies for AML treatment.
Data availability statement
The datasets presented in this study can be found in online repositories. The names of the repository/repositories and accession number(s) can be found in the article/Supplementary Material.
Ethics statement
The studies involving human participants were reviewed and approved by the Medical Ethical Committee of Qilu Hospital, Shandong University. The patients/participants provided their written informed consent to participate in this study.
Author contributions
CJ and FL conceived and designed the study. XS and GM performed most of the analyses and experiments and wrote the manuscript. XB, JZ, and ML performed some experiment. FZ, TS, and DM edited the manuscript.
Funding
This work was supported by grants from the Distinguished Taishan Scholars in Climbing Plan (tspd20210321), the Natural Science Foundation of Shandong Province (ZR2021QH138), the National Natural Science Foundation of China (Nos. 81770159, 81873425, 82070160, and 82170182).
Conflict of interest
The authors declare that the research was conducted in the absence of any commercial or financial relationships that could be construed as a potential conflict of interest.
Publisher’s note
All claims expressed in this article are solely those of the authors and do not necessarily represent those of their affiliated organizations, or those of the publisher, the editors and the reviewers. Any product that may be evaluated in this article, or claim that may be made by its manufacturer, is not guaranteed or endorsed by the publisher.
Supplementary material
The Supplementary Material for this article can be found online at: https://www.frontiersin.org/articles/10.3389/fcell.2022.978786/full#supplementary-material
Abbreviations
AML, Acute myeloid leukemia; BP, Biological process; CC, Cellular component; DEGs, Differentially expressed genes; FCM, Flow cytometry; GEO, Gene Expression Omnibus; GEPIA, Gene Expression Profiling Interactive Analysis; GO, Gene Ontology; GSEA, Gene set enrichment analysis; KEGG, Kyoto encyclopedia of genes and genomes; LGG, Low-grade glioma; MF, Molecular function; MFS, Major facilitator superfamily; NC, Negative control; NK, Natural killer; OS, Overall survival; PPI, Protein–protein interaction; SKCM, Skin cutaneous melanoma; TCGA, The Cancer Genome Atlas; TILs, Tumor-infiltrating lymphocytes; TME, Tumor microenvironment; Tregs, regulatory T cells.
References
Abril-Rodriguez, G., and Ribas, A. (2017). Snapshot: Immune checkpoint inhibitors. Cancer Cell 31, 848–848.e1. doi:10.1016/j.ccell.2017.05.010
Alkhateeb, A., Rezaeian, I., Singireddy, S., Cavallo-Medved, D., Porter, L. A., and Rueda, L. (2019). Transcriptomics signature from next-generation sequencing data reveals new transcriptomic biomarkers related to prostate cancer. Cancer Inf. 18, 1176935119835522. doi:10.1177/1176935119835522
Austin, R., Smyth, M. J., and Lane, S. W. (2016). Harnessing the immune system in acute myeloid leukaemia. Crit. Rev. Oncol. Hematol. 103, 62–77. doi:10.1016/j.critrevonc.2016.04.020
Bense, R. D., Sotiriou, C., Piccartgebhart, M. J., Haanen, J. B. A. G., van Vugt, M. A. T. M., de Vries, E. G. E., et al. (2016). Relevance of tumor-infiltrating immune cell composition and functionality for disease outcome in breast cancer. J. Natl. Cancer Inst. 109 (1), djw192. doi:10.1093/jnci/djw192
Brasier, G., Tikellis, C., Xuereb, L., Craigie, J., Casley, D., Kovacs, C. S., et al. (2004). Novel hexad repeats conserved in a putative transporter with restricted expression in cell types associated with growth, calcium exchange and homeostasis. Exp. Cell Res. 293, 31–42. doi:10.1016/j.yexcr.2003.10.002
Carter, B. Z., Mak, P. Y., Wang, X., Tao, W., Ruvolo, V., Mak, D., et al. (2019). An ARC-regulated il1β/cox-2/PGE2/β-catenin/ARC circuit controls leukemia-microenvironment interactions and confers drug resistance in AML. Cancer Res. 79, 1165–1177. doi:10.1158/0008-5472.CAN-18-0921
Chen, X., Pan, J., Wang, S., Hong, S., Hong, S., and He, S. (2019). The epidemiological trend of acute myeloid leukemia in childhood: A population-based analysis. J. Cancer 10, 4824–4835. doi:10.7150/jca.32326
Dohner, H., Weisdorf, D. J., and Bloomfield, C. D. (2015). Acute myeloid leukemia. N. Engl. J. Med. 373, 1136–1152. doi:10.1056/NEJMra1406184
Duffffy, S. P., Shing, J., Saraon, P., Berger, L. C., Eiden, M. V., Wilde, A., et al. (2010). The Fowler syndrome-associated protein FLVCR2 is an importer of heme. Mol. Cell. Biol. 30, 5318–5324. doi:10.1128/MCB.00690-10
Fan, Z., Duan, J., Luo, P., Shao, L., Chen, Q., Tan, X., et al. (2022). SLC25A38 as a novel biomarker for metastasis and clinical outcome in uveal melanoma. Cell Death Dis. 11 (134), 330. doi:10.1038/s41419-022-04718-8
Fu, G., Guy, C. S., Chapman, N. M., Palacios, G., Wei, J., Zhou, P., et al. (2021). Metabolic control of TFH cells and humoral immunity by phosphatidylethanolamine. Nature 595, 724–729. doi:10.1038/s41586-021-03692-z
Gao, H., Liang, J., Duan, J., Chen, L., Li, H., Zhen, T., et al. (2021). A prognosis marker SLC2A3 correlates with EMT and immune signature in colorectal cancer. Front. Oncol. 15 (11), 638099. doi:10.3389/fonc.2021.638099
Gyorffy, B., Surowiak, P., Budczies, J., and Lanczky, A. (2013). Online survival analysis software to assess the prognostic value of biomarkers using transcriptomic data in non-small-cell lung cancer. PLoS One 8, e82241. doi:10.1371/journal.pone.0082241
Huang, da W., Sherman, B. T., and Lempicki, R. A. (2009). Systematic and integrative analysis of large gene lists using DAVID bioinformatics resources. Nat. Protoc. 4, 44–57. doi:10.1038/nprot.2008.211
Ito, Y., Takasawa, A., Takasawa, K., Murakami, T., Akimoto, T., Kyuno, D., et al. (2022). Aberrant expression of claudin-6 contributes to malignant potentials and drug resistance of cervical adenocarcinoma. Cancer Sci. 113, 1519–1530. doi:10.1111/cas.15284
Khaldoyanidi, S., Nagorsen, D., Stein, A., Ossenkoppele, G., and Subklewe, M. (2021). Immune Biology of acute myeloid leukemia: Implications for immunotherapy. J. Clin. Oncol. 39, 419–432. doi:10.1200/JCO.20.00475
Kumar, C. C. (2011). Genetic abnormalities and challenges in the treatment of acute myeloid leukemia. Genes Cancer 2, 95–107. doi:10.1177/1947601911408076
Ladikou, E. E., Sivaloganathan, H., Pepper, A., and Chevassut, T. (2020). Acute myeloid leukaemia in its niche: The bone marrow microenvironment in acute myeloid leukaemia. Curr. Oncol. Rep. 22 (3), 27. doi:10.1007/s11912-020-0885-0
Lei, X., Lei, Y., Li, J. K., Du, W. X., Li, R. G., Yang, J., et al. (2020). Immune cells within the tumor microenvironment: Biological functions and roles in cancer immunotherapy. Cancer Lett. 470, 126–133. doi:10.1016/j.canlet.2019.11.009
Li, T., Fu, J., Zeng, Z., Cohen, D., Li, J., Chen, Q., et al. (2020). TIMER2.0 for analysis of tumor-infiltrating immune cells. Nucleic Acids Res. 48, W509-W514. doi:10.1093/NAR/GKAA407
Liu, X., Wu, S., Yang, Y., Zhao, M., Zhu, G., and Hou, Z. (2017). The prognostic landscape of tumor-infiltrating immune cell and immunomodulators in lung cancer. Pharmacother 95, 55–61. doi:10.1016/j.biopha.2017.08.003
Metzeler, K. H., Hummel, M., Bloomfield, C. D., Spiekermann, K., Braess, J., Sauerland, M. C., et al. (2008). An 86-probe-set gene-expression signature predicts survival in cytogenetically normal acute myeloid leukemia. Blood 112 (10), 4193–4201. doi:10.1182/blood-2008-02-134411
Meyer, E., Ricketts, C., Morgan, N. V., Morris, M. R., Pasha, S., Tee, L. J., et al. (2010). Mutations in FLVCR2 are associated with proliferative vasculopathy and hydranencephaly-hydrocephaly syndrome (Fowler syndrome). Am. J. Hum. Genet. 86, 471–478. doi:10.1016/j.ajhg.2010.02.004
Mitteldorf, C., Berisha, A., Pfaltz, M. C., Broekaert, S. M. C., Schon, M. P., Kerl, K., et al. (2017). Tumor microenvironment and checkpoint molecules in primary cutaneous diffuse large B-cell lymphoma-new therapeutic targets. Am. J. Surg. Pathol. 41 (7), 998–1004. doi:10.1097/PAS.0000000000000851
Mizuno, H., Kitada, K., Nakai, K., and Sarai, A. (2009). PrognoScan: A new database for meta-analysis of the prognostic value of genes. BMC Med. Genomics 2, 18. doi:10.1186/1755-8794-2-18
Mueller, P., Massner, J., Jayachandran, R., Combaluzier, B., Albrecht, I., Gatfield, J., et al. (2008). Regulation of T cell survival through coronin-1-mediated generation of inositol-1, 4, 5-trisphosphate and calcium mobilization after T cell receptor triggering. Nat. Immunol. 9 (4), 424–431. doi:10.1038/ni1570
Pao, G. M., Wu, L. F., Johnson, K. D., Hofte, H., Chrispeels, M. J., Sweet, G., et al. (1991). Evolution of the MIP family of integral membrane transport proteins. Mol. Microbiol. 5, 33–37. doi:10.1111/j.1365-2958.1991.tb01823.x
Pao, S. S., Paulsen, I. T., and Saier, M. H. (1998). Major facilitator superfamily. Microbiol. Mol. Biol. Rev. 62, 1–34. doi:10.1128/MMBR.62.1.1-34.1998
Quistgaard, E. M., Löw, C., Guettou, F., and Nordlund, P. (2016). Understanding transport by the major facilitator superfamily (MFS): Structures pave the way. Nat. Rev. Mol. Cell Biol. 17, 123–132. doi:10.1038/nrm.2015.25
Shenghui, Z., Yixiang, H., Jianbo, W., Kang, Y., Laixi, B., Yan, Z., et al. (2011). Elevated frequencies of CD4⁺ CD25⁺ CD127lo regulatory T cells is associated to poor prognosis in patients with acute myeloid leukemia. Int. J. Cancer 129, 1373–1381. doi:10.1002/ijc.25791
Sisto, M., Ribatti, D., and Lisi, S. (2021). Cadherin signaling in cancer and autoimmune diseases. Int. J. Mol. Sci. 22 (24), 13358. doi:10.3390/ijms222413358
Steven, A., Fisher, S. A., and Robinson, B. W. (2016). Immunotherapy for lung cancer. Respirology 21, 821–833. doi:10.1111/resp.12789
Subramanian, A., Tamayo, P., Mootha, V. K., Mukherjee, S., Ebert, B. L., Gillette, M. A., et al. (2005). Gene set enrichment analysis: A knowledge-based approach for interpreting genome-wide expression profiles. Proc. Natl. Acad. Sci. U. S. A. 102 (43), 15545–15550. doi:10.1073/pnas.0506580102
Tang, Z., Li, C., Kang, B., Gao, G., Li, C., and Zhang, Z. (2017). GEPIA: A web server for cancer and normal gene expression profiling and interactive analyses. Nucleic Acids Res. 45, W98-W102–W102. doi:10.1093/nar/gkx247
Tettamanti, S., Pievani, A., Biondi, A., Dotti, G., and Serafini, M. (2022). Catch me if you can: How AML and its niche escape immunotherapy. Leukemia 36 (1), 13–22. doi:10.1038/s41375-021-01350-x
Truxova, I., Kasikova, L., Salek, C., Hensler, M., Lysak, D., Holicek, P., et al. (2020). Calreticulin exposure on malignant blasts correlates with improved natural killer cell-mediated cytotoxicity in acute myeloid leukemia patients. Haematologica 105 (7), 1868–1878. doi:10.3324/haematol.2019.223933
van Galen, P., Hovestadt, V., Wadsworth Ii, M. H., Hughes, T. K., Griffin, G. K., Battaglia, S., et al. (2019). Single-cell RNA-seq reveals AML hierarchies relevant to disease progression and immunity. Cell 176, 1265–1281. doi:10.1016/j.cell.2019.01.031
Yoshihara, K., Shahmoradgoli, M., Martínez, E., Vegesna, R., Kim, H., TorresGarcia, W., et al. (2013). Inferring tumour purity and stromal and immune cell admixture from expression data. Nat. Commun. 4, 2612. doi:10.1038/ncomms3612
Zhou, J., Chi, X., Cheng, M., Huang, X., Liu, X., Fan, J., et al. (2019). Zika virus degrades the ω-3 fatty acid transporter Mfsd2a in brain microvascular endothelial cells and impairs lipid homeostasis. Sci. Adv. 5, eaax7142. doi:10.1126/sciadv.aax7142
Keywords: acute myeloid leukemia, FLVCR2, tumor microenvironment, prognosis, immune cell infiltration
Citation: Su X, Ma G, Bai X, Zhang J, Li M, Zhang F, Sun T, Ma D, Lu F and Ji C (2022) The prognostic marker FLVCR2 associated with tumor progression and immune infiltration for acute myeloid leukemia. Front. Cell Dev. Biol. 10:978786. doi: 10.3389/fcell.2022.978786
Received: 26 June 2022; Accepted: 29 September 2022;
Published: 12 October 2022.
Edited by:
Birija Sankar Patro, Bhabha Atomic Research Centre (BARC), IndiaReviewed by:
Sanjiban Chakrabarty, Manipal Academy of Higher Education, IndiaSavita Kulkarni, Bhabha Atomic Research Centre (BARC), India
Copyright © 2022 Su, Ma, Bai, Zhang, Li, Zhang, Sun, Ma, Lu and Ji. This is an open-access article distributed under the terms of the Creative Commons Attribution License (CC BY). The use, distribution or reproduction in other forums is permitted, provided the original author(s) and the copyright owner(s) are credited and that the original publication in this journal is cited, in accordance with accepted academic practice. No use, distribution or reproduction is permitted which does not comply with these terms.
*Correspondence: Fei Lu, bHVmZWlzZHUyQDE2My5jb20=; Chunyan Ji, amljaHVueWFuQHNkdS5lZHUuY24=
†These authors have contributed equally to this work