- 1Department of Paediatric Surgery, Tianjin Medical University General Hospital, Tianjin, China
- 2College of Life Sciences, Nankai University, Tianjin, China
- 3National Clinical Research Center of Kidney Disease, Jinling Hospital, Nanjing University School of Medicine, Nanjing, China
- 4Department of Clinical Laboratory, Affiliated Maternity Hospital, Nankai University, Tianjin, China
A cavernous hemangioma, well-known as vascular malformation, is present at birth, grows proportionately with the child, and does not undergo regression. Although a cavernous hemangioma has well-defined histopathological characteristics, its origin remains controversial. In the present study, we characterized the cellular heterogeneity of a cavernous hemangioma using single-cell RNA sequencing (scRNA-seq). The main contribution of the present study is that we discovered a large number of embryonic mesenchymal stem cells (MSCs) in a cavernous hemangioma and proposed that cavernous hemangiomas may originate from embryonic MSCs. Further analysis of the embryonic MSCs revealed that: 1) proinflammatory cytokines and related genes TNF, TNFSF13B, TNFRSF12A, TNFAIP6, and C1QTNF6 are significantly involved in the MSC-induced immune responses in cavernous hemangiomas; 2) UCHL1 is up-regulated in the embryonic MSC apoptosis induced by proinflammatory cytokines; 3) the UCHL1-induced apoptosis of MSCs may play an important role in the MSC-induced immune responses in cavernous hemangiomas; and 4) UCHL1 can be used as a marker gene to detect embryonic MSCs at different apoptosis stages. In addition to MSCs, ECs, macrophages, T lymphocytes and NKCs were intensively investigated, revealing the genes and pathways featured in cavernous hemangiomas. The present study revealed the origin of cavernous hemangiomas and reported the marker genes, cell types and molecular mechanisms, which are associated with the origin, formation, progression, diagnosis and therapy of cavernous hemangiomas. The better understanding of the MSC-induced immune responses in benign tumours helps to guide future investigation and treatment of embryonic MSC-caused tumours. Our findings initiated future research for the rediscovery of MSCs, cancers/tumours and the UCHL1-induced apoptosis.
Introduction
Vascular tumours include hemangioma, hemangioendothelioma, angiosarcoma, and their epithelioid variants (Errani et al., 2012). According to the sizes of the affected vessels, hemangiomas are histologically classified as capillary, cavernous, or mixed-type hemangiomas (Fu et al., 2020). A capillary hemangioma (superficial, red, raised), also called strawberry hemangioma, is a tumour of infancy that undergoes a phase of rapid growth and expansion followed by a period of slow but steady regression during childhood. In contrast, a cavernous hemangioma (deep dermal, blue hue), which is now classified as vascular malformation according to the International Society for the Study of Vascular Anomalies (ISSVA) classification (Wang et al., 2019), is present at birth, grows proportionately with the child, and does not undergo regression (Beck and Gosain, 2009). Cavernous hemangiomas have been reported to arise at various sites, including the skin and subcutaneous layers of the head and neck, face, extremities, liver, gastrointestinal tract, and even the thymus (Kim et al., 2018). The tumours are composed of dilated vascular spaces, with thinned smooth muscle walls separated by a variable amount of fibroconnective tissue. Major features used to discriminate cavernous hemangiomas from capillary hemangiomas include the observation of “normal” vascular endothelial cells in cavernous hemangiomas and the over-expression of vascular endothelial growth factor A (VEGFA) and fibroblast growth factor receptor 1 (FGFR1) in capillary hemangiomas during the proliferative stage (Tan et al., 2000).
Three classes of cavernous hemangiomas, hepatic cavernous hemangioma (HCH), retinal cavernous hemangioma (RCH), and cerebral cavernous hemangioma (CCH) that is also known as cerebral cavernous malformation (CCM) are comparatively well studied. As the most common benign tumour of the liver, HCH is present in up to 7% of individuals that participate in autopsy studies. Large HCHs may be associated with thrombosis, scarring, and calcification (Roberts, 2012). Histological examination of the lesions has revealed a network of vascular spaces lined by endothelial cells and separated by a thin fibrous stroma. RCH is composed of clusters of saccular aneurysms filled with dark blood. Microscopic examination of the lesions has revealed multiple thin-walled interconnected vascular spaces lined by flat endothelial cells, with red cell necrosis and partially organised intravascular thrombosis. Furthermore, the vascular spaces in RCHs are bordered by thin, fibrous septa, with occasional nerve fibers and glial cells. CCHs that occur in the central nervous system, most often in the brain, can cause intracranial hemorrhage, seizures, neurological deficits, and even death. CCH has sporadic and familial forms; familial CCHs often display multiple lesions and autosomal dominant inheritance. Ultrastructural studies revealed abnormal or absent blood–brain barrier components, poorly formed tight junctions with gaps between endothelial cells, lack of astrocytic foot processes, and few pericytes in CCHs. Although mutations in the KRIT1 and CCM1 genes have been found in patients with both RCH and CCH, the cause of RCH is still unknown (Wang and Chen, 2017). One possible cause of familial CCHs is the loss-of-function mutations in three genes, KRIT1, CCM2, and PDCD10 (Kim et al., 2016). According to the current theory, hemangiomas originate from neogenesis or revival of dormant embryonic angioblasts and arise through hormonally driven vessel growth (Beck and Gosain, 2009). However, the origins of hemangiomas remain controversial.
Although capillary hemangiomas are mainly treated by surgery, several drugs (e.g., propranolol and glucocorticoids) have been developed to avoid the risks of intraoperative profuse bleeding, postoperative recurrence, long-term scarring, and other complications. The treatment of cavernous hemangiomas is still dependent on excision and venous embolization therapy (Wang et al., 2019). However, inadequate excision causes recurrence (Jang et al., 2011). To develop drugs or other new therapies, more basic research must be conducted to better understand cavernous hemangiomas at the molecular level. In the present study, we characterised the cellular heterogeneity of a cavernous hemangioma using single-cell RNA sequencing (scRNA-seq) (Gao, 2018). Through further analysis of the scRNA-seq data, we aimed to: 1) reveal the comprehensive cellular composition and gene expression profile of a cavernous hemangioma at the single-cell level, and 2) discover the marker genes, cell types, and molecular mechanisms, which are associated with the origin, formation, progression, diagnosis, and therapy of cavernous hemangiomas.
Results
Single-Cell RNA Sequencing and Basic Analyses
The lesion was obtained from a 6-year-old patient diagnosed with a cavernous hemangioma (Supplementary File S1). Using a piece of tissue in the centre of the tumour, scRNA-seq libraries (10x Genomics, United States) were constructed and sequenced to produce ∼163 Gbp of raw data (Materials and Methods). After data cleaning and quality control, a total of 10,784 cells and 22,023 genes were identified to produce a 22,010 × 10,784 matrix and a 13 × 10,784 matrix, representing the expression levels of nuclear and mitochondrial genes, respectively. Using the 22,010 × 10,784 nuclear matrix, 10,784 cells were clustered into 18 clusters with adjusted parameters (Materials and Methods). The identification of each cluster as a specific cell type took two-steps (the rough and exact identification). Firstly, 18 clusters were roughly identified as 18 cell types. Next, three types of endothelial cells were merged into one type. Then, 18 clusters were merged into 16 clusters, which were named according to their cell types (Figure 1A), including fibroblast cell type 1 (fibroblast1), type 2 (fibroblast2), smooth muscle cell (SMC), endothelial cell type 1 (EC1), type 2 (EC2), lymphatic endothelial cell (LEC), T lymphocyte type 1 (TC1), type 2 (TC2), type 3 (TC3), B lymphocyte (BC), mast cell, monocyte derived dendritic cell (mDC), plasmacytoid dendritic cell (pDC), CLEC9A positive dendritic cell (CLEC9A + DC), macrophage type 1 and type 2, respectively. For each of the 16 clusters, differential expression analysis between cells inside and outside the cluster was performed to produce a gene-expression signature (Materials and Methods) including all differentially expressed (DE) genes (Supplementary File S2). By further analysis of gene-expression signatures, 16 clusters were exactly identified as 16 cell types and then renamed as fibroblast, mesenchymal stem cell (MSC), SMC, EC1, EC2, LEC, CD4 positive T cell (CD4+TC), CD8 positive T cell (CD8+TC), natural killer cell (NKC), BC, mast, mDC, pDC, CLEC9A + DC, M1-like macrophage (m1Maph) and M2-like macrophage (m2Maph) clusters, respectively. To confirm the presence of these cell types in the tumour, dilated capillaries, normal capillaries, lymphatic vessels, muscle tissue, and connective tissue were observed by haematoxylin–eosin (HE) staining (Supplementary File S1). As no single gene can be used as a marker to discriminate a cell type from its relatives (e.g., CD8+T from CD4+T), we assigned the top five DE genes as a combination of marker genes (Materials and Methods) to each cell type to help to identify these cell types in future studies (Table 1). The representation of each cell type by the combination of marker genes was also displayed in Venn diagrams (Supplementary Figure S4). We designed a new metric (Materials and Methods), named the union and intersection coverage of a cluster (UICC), to evaluate the representation of a cell type by a combination of marker genes.
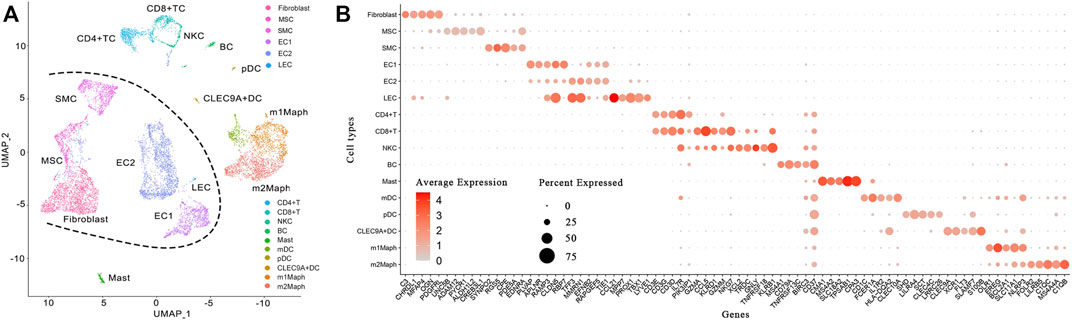
FIGURE 1. Identification of 16 cell types in a cavernous hemangioma. A total of 10,784 cells were finally clustered into 16 clusters and then identified as 16 cell types. The non-immune cell types (fibroblast, MSC, SMC, EC1, EC2 and LEC) were clearly separated from immune cell types (CD4+TC, CD8+TC, NKC, BC, mast, mDC, pDC, CLEC9A + DC, m1Maph and m2Maph) by a borderline (named Nankai borderline in the present study). (A). Uniform Manifold Approximation and Projection (UMAP) method was used to show the clustering results; (B). For each of the 16 clusters, a combination of five marker genes (the symbols of 80 genes can be seen in Table 1) were assigned. MSC: mesenchymal stem cell, SMC: smooth muscle cell, EC1: endothelial cell type 1, EC2: endothelial cell type 2, LEC: lymphatic endothelial cell, CD4+TC: CD4 positive T cell, CD8+TC: CD8 positive T cell, NKC: natural killer cell, BC: B cell, mDC: monocyte derived dendritic cell, pDC: plasmacytoid dendritic cell, CLEC9A + DC: CLEC9A positive dendritic cell, m1Maph: M1-like macrophage and m2Maph: M2-like macrophage.
In a previous study of ascending thoracic aortic aneurysms (ATAAs) (Li et al., 2020), 11 major cell types were identified by known marker genes and they were fibroblast, MSC, SMC1, SMC2, EC, TC, NKC, BC, mast cell, MonoMaphDC (monocyte/macrophage/DC) and plasma cell. Thus, we performed a simple comparison between cell populations in the cavernous hemangioma tissue and those in ATAA tissues and their controls (Li et al., 2020) and found several main differences, particularly, the cavernous hemangioma tissue contained: 1) lower proportion of immune cells (36.32% of the total cells) than ATAA tissues (64.08%), and more than their controls (26.24%); 2) higher proportions of fibroblasts and ECs (24.51 and 30.65%) than ATAA tissues (7.6 and 7.43%) and their controls (13.51 and 14.02%); 3) similar proportion of DCs and macrophages (21.73%) to ATAA tissues (23.71%), much more than the controls of ATAA (7.64%); and 4) much lower proportions of B and plasma cells (1.27% and 0) than ATAA tissues (9.36 and 11.5%) and their controls (4.31 and 2.09%). The above results signified that fibroblasts, ECs, and macrophages in the cavernous hemangioma tissue need to be intensively investigated.
Identification of Fibroblasts and Mesenchymal Stem Cells
The fibroblast1 and fibroblast2 clusters contained 18.85% (2,033/10,784) and 5.66% (610/10,784) of the total cells, respectively. Such proportion of fibroblast1 (18.85%) was markedly higher than that of SMCs (8.17%, 881/10784), which was consistent with the prominent histological features of cavernous hemangiomas where the thinned smooth muscle walls are separated by a variable amount of fibroconnective tissue (Introduction). Fibroblast1 was finally identified as a cluster of fibroblasts, as it highly expressed all the known marker genes, PDGFRA, PDGFRB, MEG3, SCARA5, COL14A1, and OGN (Supplementary Figure S5), which have been reported in a previous study (Kuppe et al., 2021). In contrast, fibroblast2 expressed PDGFRB and MEG3 at high levels (0.5 < LFCio ≤ μ+σ), PDGFRA and COL14A1 at similar levels (|LFCio-μ|≤0.5), OGN at a low level (μ-σ<LFCio<=-0.5), and SCARA5 at a very low level (μ-2σ<LFCio<=-μ-σ), compared with other cells. Although both fibroblast1 and fibroblast2 expressed a number of fibrillin, fibulin, collagen, and elastin genes required in the extracellular matrix (ECM), including FBN1, FBLN1, FBLN2, FBLN5, COL1A1, COL1A2, COL3A1, COL6A1, COL6A2, COL5A2, COL14A1, and ELN (Supplementary Figure S6), fibroblast1 markedly higher expressed most of these genes (e.g., FBLN1, FBLN2, and ELN) than fibroblast2. According to the previous study (Kuppe et al., 2021), pericytes, closely related to fibroblasts, also highly express ECM genes. Then, we examined the expression levels of PDGFRA, NOTCH3, and RGS5 that are three marker genes of pericytes (Li et al., 2020) (Supplementary Figure S5). Pericytes expressed PDGFRA at a low level, while fibroblast2 expressed PDGFRA at a similar level, compared to other cells. Although both fibroblast2 and pericytes expressed NOTCH3 at high levels, fibroblast2 did not originate from pericytes, as pericytes expressed RGS5 at a high level, but fibroblast2 expressed RGS5 at a very low level, compared to other cells. The results in this paragraph indicated that fibroblast2 is a cluster of cells related to, but markedly different from fibroblasts.
For exact identification of fibroblast2, differential expression analysis between cells inside and outside fibroblast2 was performed to generate a gene-expression signature (Supplementary File S2). From the gene-expression signature, 63 coding genes and a long noncoding RNA (lncRNA) gene RP11-14N7.2 (Table 2) were selected for further analysis. Gene ontology (GO) and pathway annotation of the 63 coding genes (Figure 2A) revealed that 57.14% (36/63) of the genes are involved in ECM organisation (GO:0030198), and 15.87% (10/63) of the genes are involved in the response to fibroblast growth (GO:0071774). This finding confirmed that fibroblast2 is a cluster of cells related to fibroblasts. Significantly, many GO annotations were found to be enriched in endodermal cell differentiation and tissue development, including endodermal cell differentiation (GO:0035987), blood vessel development (GO:0001568), skeletal system development (GO:0001501), heart development (GO:0007507), bone development (GO:0060348), muscle organ development (GO:0007517), reproductive structure development (GO:0048608), and lung development (GO:0030324). In particular, TWIST1, SULF1, COL5A1, COL5A2, COL6A1, and FN1 are associated with endodermal cell differentiation (GO:0035987), thus indicating the characteristics of stem or progenitor cells in fibroblast2. Searching literature databases using the 63 coding genes revealed that at least 19 genes had been reported to be over-expressed or up-regulated in stem cells, namely, UNC5B, ADAM12, PYCR1, PHGDH, SLIT2, PFN2, TNFAIP6, TNC, EDIL3, TWIST1, SNAI2, PHLDA2, LOXL2, BMP1, COL5A1, POSTN, ID3, COL6A1, and COL1A1 (Figure 2B). Among the 19 genes, seven (PYCR1, TNFAIP6, EDIL3, TWIST1, LOXL2, BMP1, and COL1A1) have been reported to be expressed in MSCs in the previous studies (Supplementary File S3). Among these seven genes, TWIST1 is a basic helix-loop-helix (bHLH) transcription factor that plays essential and pivotal roles in multiple stages of embryonic development. The over-expression of TWIST1 induces epithelial-mesenchymal transition (EMT), a key process in cancer metastasis (Zhu et al., 2016). Among the 19 genes, UNC5B, ADAM12, and PYCR1 belong to the five marker genes of fibroblast2 (Table 1). Notably, UNC5B has been reported to be a marker gene of epiblast stem cells. According to annotations from the GeneCards database (Marilyn et al., 2002), UNC5B encodes a member of the netrin family of receptors, and the encoded protein mediates the repulsive effect of netrin-1. The protein encoded by UNC5B belongs to a group of proteins called dependence receptors (DpRs), which are involved in embryogenesis and cancer progression. The results in this paragraph indicated that fibroblast2 is a cluster of MSCs.
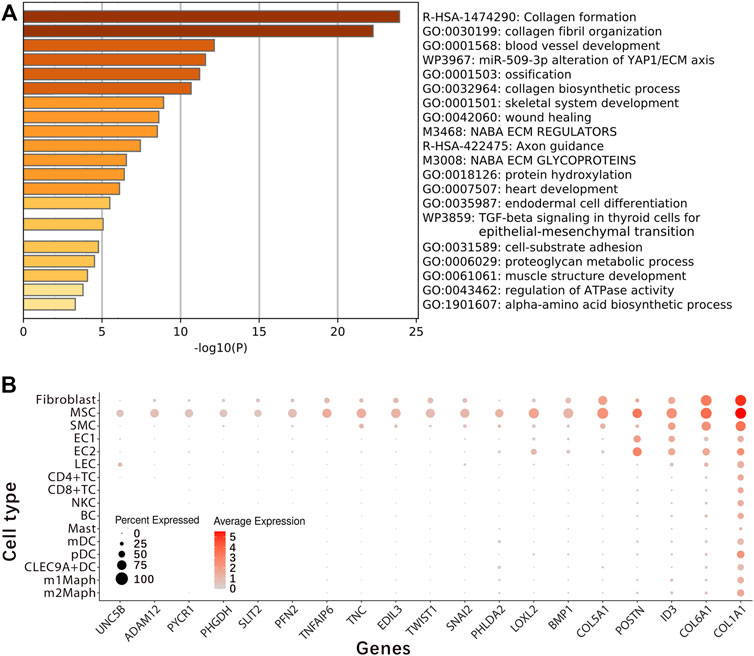
FIGURE 2. Identification of embryonic mesenchymal stem cells. Differential expression analysis between cells inside and outside fibroblast2 was performed to generate a gene-expression signature (Supplementary File S2). From the gene-expression signature, the 63 coding genes and a long noncoding RNA (lncRNA) gene RP11-14N7.2 (Table 2) were selected for the exact identification of fibroblast2. Finally, we concluded that fibroblast2 is a cluster of embryonic mesenchymal stem cells (MSCs). (A). GO and pathway annotation of the 63 coding genes with analysis were performed using the Metascape website; (B). At least 19 genes (UNC5B, ADAM12, PYCR1, PHGDH, SLIT2, PFN2, TNFAIP6, TNC, EDIL3, TWIST1, SNAI2, PHLDA2, LOXL2, BMP1, COL5A1, POSTN, ID3, COL6A1, and COL1A1) have been reported to be over-expressed or up-regulated in stem cells, and among these 19 genes, seven (PYCR1, TNFAIP6, EDIL3, TWIST1, LOXL2, BMP1, and COL1A1) have been reported to be expressed in MSCs in the previous studies (Supplementary File S3).
The identified MSCs exhibited characteristics of cancers/tumours. Notably, at least 33 genes in the gene-expression signature (Table 2) are currently being studied in cancers/tumour biology and they are: 1) seven genes (CREB3L1, SLIT2, CHPF, TNFRSF12A, SULF1, UACA and TPM1) which have been reported to be under-expressed or down-regulated (Supplementary Figure S7); 2) 25 genes (UNC5B, ADAM12, PYCR1, PHGDH, GPC1, ENPP1, NCS1, PFN2, TNFAIP6, DCBLD2, EDIL3, P4HA2, TWIST1, SNAI2, KDELR3, CERCAM, CLEC11A, PLOD2, UGDH, LOXL2, GPX8, SERPINH1, PRDX4, POSTN and MYDGF) which have been reported to be over-expressed or up-regulated (Supplementary Figure S8) and 3) UCHL1 which has been reported as a tumour promoter in pancreatic, prostate, and lung cancers but a tumour suppressor in ovarian cancer, hepatocellular cancer, and nasopharyngeal carcinoma (Supplementary File S3). Among these 33 genes, POSTN encodes a secreted extracellular matrix protein that functions in tissue development and regeneration, including wound healing and ventricular remodelling following myocardial infarction. According to a previous study (Malanchi et al., 2012), POSTN is expressed by fibroblasts in normal tissue and the stroma of the primary tumour and plays a role in cancer stem cell maintenance and metastasis. In humans, high expression of POSTN has been detected in various types of cancer, including breast, ovarian, lung, prostate, kidney, intestine, and pancreas (Borecka et al., 2020). In another previous study (Kikuchi et al., 2014), the results have demonstrated the over-expression of POSTN in cancer-associated fibroblasts (CAFs) and suggested that POSTN constitutes the primary tumour niche by supporting cancer cell proliferation through the ERK signalling pathway in gastric cancer. In the present study, we found that POSTN was expressed in MSC, EC2, EC1, and fibroblasts clusters at levels from highest to lowest, which suggested that POSTN is involved in MSC differentiation. The MSCs also exhibited other characteristics of cancers/tumours, e.g., high expression of GAPDH and very high expression of MKI67. MKI67, a tumour proliferation marker, encodes Ki-67, which is associated with cellular proliferation (Figure 4). Accordingly, the expression of MKI67 correlates with tumour grade in many cancers. Then, we proposed that the embryonic MSCs caused the cavernous hemangioma, which need be verified by experiments in the future.
Confirmation and Characterization of the Identified MSCs
In previous studies, MSCs have been discovered from different sources (e.g., placentas, bone marrows and tumours), however, the identification of them is still difficult, as many of the discovered MSCs can not be isolated or cultured. Currently, we only have criteria from International Society for Cellular Therapy (ISCT) to roughly identify MSCs (Dominici et al., 2006), they are: 1) MSCs must be plastic-adherent when maintained in standard culture conditions using tissue culture flasks; 2) most of MSCs (more than 95%) must express NT5E (CD73), THY1 (CD90) and ENG (CD105), and lack expression (only less than 2% positive) of PTPRC (CD45), CD34, CD14 or ITGAM (CD11b), CD79A or CD19 and HLA class II; and 3) MSCs must be able to differentiate to osteoblasts, adipocytes and chondroblasts under standard in vitro differentiating conditions. In general, the identified MSCs in cavernous hemangioma meet the above criteria, as they highly expressed NT5E and THY1, however, lowly or very lowly expressed ENG, PTPRC, CD34, CD14, ITGAM, CD79A, and CD19, compared with other cells. Eventually, the MSCs in the cavernous hemangioma were identified as embryonic MSCs, ruling out other possibilities: 1) they did not originate from pericytes (As described above); 2) they were not CAFs, as VEGFC and HGF were expressed at high levels, TGFB1 and TGFB3 at very high levels, VEGFB at an average level, VEGFA, TGFB2, IL6, and CXCL12 at low levels, and GAS6 at a very low level in the MSCs, whereas these 10 marker genes are highly expressed in CAFs (Sahai et al., 2020); 3) they were not bone marrow-derived MSCs from wound healing process, as the patient carried this tumour at birth without history of injury (Supplementary File S1); 4) they were not generated via EMT (As described above), as the tumour tissue did not contain epithelial cells, although the very high expression of TWIST1 was detected in the MSCs; and 5) they were not transformed from angioblasts, as we only detected the low-level expression of FGF2, which has an essential role in induction of angioblasts with potential to transform into MSCs or hematopoietic cells (hemangioblasts) as reported in a previous study (Slukvin and Vodyanik, 2011). The above results did not rule out the probability that the MSCs in the cavernous hemangioma were generated via endothelial-mesenchymal transition (EndMT), which is crucial during embryonic development and a contributing source of CAFs that participate in tumour growth and metastasis (Mai et al., 2013). Then, we addressed a novel scientific question: do CAFs in certain cancers/tumours originate from embryonic MSCs as CAFs in glioma, breast, gastric and pancreatic cancers originate from bone marrow-derived MSCs? (Liu et al., 2019).
Although MSCs have been reported in human solid tumours (e.g., bone sarcomas, lipomas, or infantile hemangiomas), less is known about them (McLean et al., 2011) and their identities are still uncertain without a gene-expression signature of MSCs as a reference. In the present study, we discovered a large number of embryonic MSCs in a cavernous hemangioma and provided the gene-expression signature of embryonic MSCs using scRNA-seq data. This gene-expression signature can be used as a reference for the exact identification of embryonic MSCs in future studies. Among the 63 coding genes (Table 2) in the gene-expression signature, UCHL1 was identified as the best one for discriminating embryonic MSCs from other cells. Using the antibody of UCHL1, we designed a simple and fast method to identify embryonic MSCs, based on immunohistochemistry (IHC) technique. Then, we detected embryonic MSCs in the tumour tissues of three other patients (Supplementary File S1) and the stained MSCs can be characterized by three features (Figure 3):1) the cytoplasm, not the nuclei (in oval shape), is stained by IHC; 2) the ratio of nuclear size to cell size is slightly smaller than that of an embryonic stem cell; and 3) the stained cells are often located in the connective tissue. As an unexpected and important result, most of these stained MSCs were undergoing cell apoptosis (Figure 3). The above results demonstrated that the embryonic MSCs can be detected by the antibody of UCHL1 using IHC. Although the functions of UCHL1 remain illusive (Described as above). we still characterized it by the following experimental results from previous studies: 1) UCHL1 induces G0/G1 cell cycle arrest and apoptosis through stabilizing p53; 2) UCHL1 concentrations in the blood plasma of boys with cryptorchidism, may reflect the heat-induced apoptosis of germ cells (Dorota et al., 2018); 3) the elevation in UCHL1 concentration is consistent with the severity of neural apoptosis following deep hypothermic circulatory arrest (DHCA) (Zhang et al., 2015) and 4) Inhibition of UCHL1 expression suppresses MSC apoptosis that is induced by proinflammatory cytokines (i.e. IFN-γ and TNF-α) via up-regulation of BCL2 (Gu et al., 2018). These results suggested that the UCHL1-induced apoptosis of MSCs may play an important role in the MSC-induced immune responses in cavernous hemangiomas.
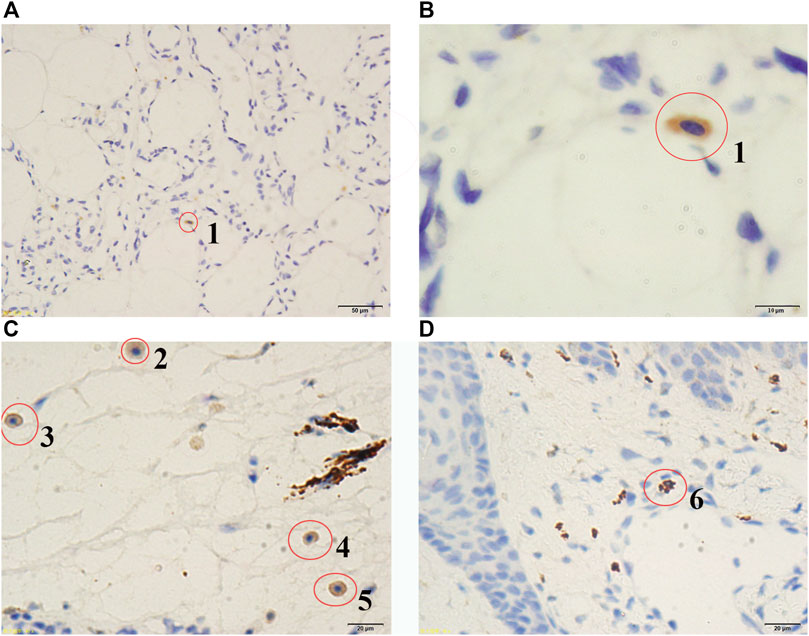
FIGURE 3. Stained embryonic MSCs at different apoptosis stages. Red circles were used to indicate the cells stained by immunohistochemistry (IHC) technique. According to the nuclear sizes, the stained cells were numbered from 1 to 6, which are embryonic mesenchymal stem cells (MSCs) at different apoptosis stages. The larger the stained nuclear size is, the earlier the apoptosis stage is. Microscopes with ordinary lens (A) and oil immersion lens (B) were used to observe tumour tissue of the patient (Admission number: 1097108). Microscopes with ordinary lens (C and D) were used to observe tumour tissues of the patients (Admission numbers: 1089641 and 1082186). The patient information (Admission number: 1097108, 1089641 and 1082186) can be seen in the Supplementary File S1.
As proinflammatory cytokines (i.e. IFN-γ and TNF-α) induce the apoptosis of MSCs, we examined the expression levels of the genes encoding INFs (interferons), the receptors of INFs, TNFSFs (tumour necrosis factor superfamily members), TNFRSFs (TNF receptor superfamily members), TNFAIPs (TNF alpha induced proteins) and C1QTNFs (C1q and TNF related proteins) and marker genes of cell apoptosis (CASP1-10, BAX, and BCL2) using our scRNA-seq data. The results (Supplementary File S3) included (Figure 4): 1) the BAX/BCL2 ratio was markedly higher in the embryonic MSCs than other cells (Supplementary Figure S9), indicating the MSC apoptosis; 2) IFNG (encoding IFN-γ) was barely detected in all types of cells (Supplementary Figure S10); 3) TNF (encoding TNF-α) was markedly higher expressed in the M1-like macrophages and mast cells than other cells (Supplementary Figure S11), however, was only detected in 21% of M1-like macrophages and 23.4% of mast cells; 4) TNFSF13B was markedly higher expressed in DCs and macrophages than other cells (Supplementary Figure S11); and 5) TNFRSF12A (Supplementary Figure S12), TNFAIP6 (Supplementary Figure S13), and C1QTNF6 (Supplementary Figure S14) were markedly higher expressed in the embryonic MSCs than other cells. Based on the above results, we concluded that: 1) proinflammatory cytokines and related genes TNF, TNFSF13B, TNFRSF12A, TNFAIP6, and C1QTNF6 are significantly involved in the MSC-induced immune responses in cavernous hemangiomas; 2) UCHL1 is up-regulated in the embryonic MSC apoptosis induced by proinflammatory cytokines (e.g., TNF and TNFSF13B); and 3) UCHL1 can be used as a marker gene to detect embryonic MSCs at different apoptosis stages.
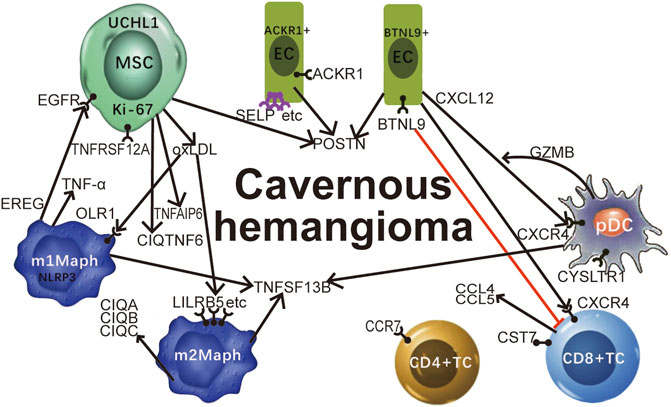
FIGURE 4. Featured genes and pathways in cavernous hemangiomas. Proinflammatory cytokines and related genes TNF (encoding TNF-α), TNFSF13B, TNFRSF12A, TNFAIP6, and C1QTNF6 are significantly involved in the MSC-induced immune responses in cavernous hemangiomas; The MSCs in cavernous hemangioma exhibit characteristics of tumours, e.g., high expression of GAPDH and very high expression of MKI67 (encoding Ki-67). UCHL1 can be used as a marker gene to detect embryonic MSCs at different apoptosis stages. The highly expressed EREG in M1-like macrophages interacted with the highly expressed EGFR in MSCs may cause MSC proliferation and the tumour progression through the binding of EREG to EGFR. MSCs induced the responses of ACKR1 positive endothelial cells (ACKR1+ECs) and BTNL9 positive endothelial cells (BTNL9+ECs). Adhesion molecules including SELP, SELE, and VCAM1 were up-regulated leading to leukocyte transmigration across the endothelium to the site of inflammation. POSTN was expressed at very high levels in MSCs, high levels in the EC2 cluster, and medium levels in the BTNL9+EC and ACKR1+EC. CCR7 has the potential to be a new marker to discriminate the CD4+T cells from the activated CD8+T cells. CST7 was unexpectedly detected to be expressed at high levels in activated CD8+T cells, medium levels in NK cells, and barely detected in non-immune cells. CD8+T cells and NK cells may highly express CCL5 for their infiltration in cavernous hemangiomas, independent on the tumour cell-derived CCL5-IFNG-CXCL9 process. The ligand-receptor interactions between CXCL12 and CXCR4 play an significant role in the immune responses in cavernous hemangioma. The highly expressed BTNL9 in BTNL9+EC may cause checkpoint blockade through the binding of BTNL9 to T-cells. The highly co-expression of CXCR4 and GZMB suggested that pDCs function for anti-tumour as CD8+T cells. We propose that OxLDL induces the OxLDL-OLR1-NLRP3 process in M1-like macrophages via the over-expression of OLR1, whereas OxLDL induces the OxLDL-SRs-C1q process in M2-like macrophages via the over-expression of many SR genes (LILRB5, etc) except OLR1. MSC: mesenchymal stem cell; OxLDL: oxidised low-density lipoprotein; SR: scavenger receptor; TNF: tumour necrosis factor.
Two Clusters of Endothelial Cells
The EC1 and EC2 clusters contained 9.57% (1,032/10,784) and 21.08% (2,273/10,784) of the total cells, respectively. Among the five marker genes of EC1 (Table 1), two (TFF3 and MMRN1) were highly expressed in LECs, whereas the other three (EFNB2, RAPGEF5, and TIE1) were highly expressed in EC2 (Figure 1B). MKI67 (marker of Ki-67 proliferation) was expressed at a markedly higher level in EC2 than EC1. Most of VEGF and FGF genes (HGF, VEGFA, VEGFB, FGF1, FGF9, FGF10, FGF11, FGF13, FGF14, FGF16, FGF18, and FGF22) were expressed at markedly higher levels in EC2 than EC1, while only a few (FGF2, FGF11, and FGF18) were expressed at markedly lower levels in EC2 than EC1 and a few (VEGFC, FGF5, and FGF13) at similar levels (Supplementary Figure S15). The above results suggested that the cells of EC2, not EC1, were undergoing proliferation, since the VEGF proteins mainly induce proliferation of ECs. Such results were consistent with the phenomenon that “normal” vascular ECs can be observed in the lesions of cavernous hemangiomas by microscopic examination (Introduction). However, the cells of EC1 were not really “normal” (As analyzed in the following paragraph). Unexpectedly, VEGFA and VEGFB were detected to be expressed at markedly higher levels in the m1Maph and pDC clusters than other cells, respectively, which merit investigation in future studies.
Although the cells of EC1 were not undergoing proliferation, they were not really “normal” and may belong to immune-responsive ECs, transformed from or by embryonic MSCs, with markedly changed expression of the major histocompatibility complex (MHC) class I and II genes. MHC I genes are expressed by all nucleated cells, while the expression of MHC II genes is limited to antigen-presenting cells (APCs) (Mai et al., 2013). Professional APCs (e.g., BCs, DCs, and macrophages) ubiquitously express MHC II, while cells such as ECs, which are not considered as APCs in the classical view, can induce MHC II expression in response to stimulation. Differential expression analysis (Supplementary File S2) between the EC1 and EC2 clusters revealed that almost all the MHC II genes (particularly, HLA-F, HLA-DMA, HLA-DMB, HLA-DPA1, HLA-DPB1, HLA-DQA1, HLA-DQB1, HLA-DRA, HLA-DRB1, and HLA-DRB5) were expressed at markedly higher levels in EC1 than EC2 (Supplementary Figure S16). Among other MHC II genes, HLA-A, HLA-B, HLA-C, and HLA-E were expressed at very high levels in all types of cells except the mast cells, whereas HLA-DOA, HLA-DOB, HLA-DQA2, and HLA-DQB2 were barely detected (Avg_in ≤ 0.05) in the EC1 and EC2 clusters. Therefore, we concluded that EC1 is a cluster of immune-responsive ECs. Various factors and stimuli, including cytokines, can induce type I activation of ECs, a state of heightened responsiveness (Mai et al., 2013). The type I activation is a rapid response that is mediated by the binding of ligands to the extracellular domains of heterotrimeric G protein-coupled receptors (GPCRs). All the detected GPCRs in the cavernous hemangioma (ADRA1D, ADRA2B, ADRA2C, AVPR2, CNR1, GALR1, GPR20, LPAR4, OXTR, P2RY2, TACR1, CYSLTR1, TAS1R1, TAS2R43, MAS1, GPR156, P2RY8, GPR52, and GPR85) were expressed at markedly higher levels in EC1 than EC2 (Supplementary Figure S17). The above results indicated that the type I activation was induced in EC1. Compared to the type I activation, the type II activation of ECs is a relatively slower response that depends on new gene expression but delivers a more sustained inflammatory response (Mai et al., 2013). The type II activation of ECs in cavernous hemangiomas merits investigation in future studies.
EC1 was further clustered into two subtypes: ACKR1 positive endothelial cell (ACKR1+EC) and BTNL9 positive endothelial cell (BTNL9+EC), including 664 and 368 cells, respectively. ACKR1, SELP, SELE, VCAM1, and CADM3 were expressed at markedly higher levels in ACKR1+EC than BTNL9+EC (Supplementary Figure S18). Among the five genes, ACKR1 encodes a glycosylated membrane protein as a non-specific receptor for several chemokines and may regulate chemokine bioavailability and consequently, leukocyte recruitment through two distinct mechanisms; CADM3 encodes a calcium-independent cell-cell adhesion protein that can form homodimers or heterodimers with other nectin proteins; the three other genes (SELP, SELE, and VCAM1) encode adhesion molecules that induce the adhesion of immune cells, such as leukocytes, to the endothelium and facilitate transmigration to underlying tissues (Mai et al., 2013). However, another adhesion molecule ICAM1 was not detected in all types of cells. The expression of genes encoding adhesion molecules can be induced by oxidised low-density lipoprotein (OxLDL) (Mai et al., 2013). OxLDL induces cellular processes through scavenger receptors (SRs) (Alquraini and El Khoury, 2020), which are a large family of structurally diverse receptors recognizing a range of ligands including modified LDLs, selected polyanionic ligands, and microbial structures. Then, we found 10 known SR genes (OLR1, MRC1, STAB1, CD163, MSR1, CD36, CD68, CXCL16, CLEC7A, and LILRB5) by searching top 100 DE genes from the gene-expression signatures of EC1, m1Maph, and m2Maph. However, almost all of them were expressed at very low levels in both EC1 and EC2 clusters except STAB1 and CD36 (Supplementary Figure S19). Among the 10 SR genes, only CD36 was expressed at a very high level in EC1, but exhibited a lower average expression level in ACKR1+EC than in BTNL9+EC. The above results suggested that the up-regulated expression of adhesion molecules in ACKR1+EC may be induced by OxLDL through CD36, but more likely through other SRs. However, it is not clear why OxLDL did not induce expression of the genes encoding adhesion molecules SELP, SELE, VCAM1, and ICAM1 in BTNL9+EC.
BTNL9 and CXCL12 can be used to characterize BTNL9+EC, as they were expressed at markedly higher levels in BTNL9+EC than ACKR1+EC (Supplementary Figure S18). As a member of the BTN/MOG Ig-superfamily, BTNL9 is expressed in a variety of tissues in humans and mice, and functions as a negative regulator of immune cell activation. Recombinant BTNL9–Fc has been demonstrated to bind to many immune cells, including macrophages, T, B, and dendritic cells. In particular, BTNL9 has been reported to inhibit CD8+ T-cell proliferation (Arnett and Viney, 2014), however, its receptors are still unknown. According to previous studies, CXCL12 encodes a stromal cell-derived alpha chemokine member of the intercrine family and stimulates the migration of monocytes and T-lymphocytes through its receptors encoded by CXCR4 and ACKR3 (Supplementary Figure S18). In the present study, CXCL12 was detected to be significant lower expressed in the immune cells than the non-immune cells, particularly fibroblast and EC1, while CXCR4 was detected to be significant lower expressed in the non-immune cells than the immune cells, particularly pDC and CD8+TC. Unexpectedly, ACKR3 was barely detected (Avg_in ≤ 0.05) to be expressed in the immune cells except m1Maph, and very lowly expressed in the non-immune cells except fibroblast. The above results suggested that (Figure 4): 1) the ligand-receptor interactions between CXCL12 and CXCR4 play a significant role in the immune responses in cavernous hemangiomas; and 2) the high expression of BTNL9 may cause checkpoint blockade in BTNL9+EC through the binding of BTNL9 to T-cells (Figure 4).
Two Subsets of Macrophages
Two subsets of macrophages were intensively investigated. A number of previous studies have revealed that a considerable degree of monocyte–macrophage heterogeneity exists when various marker genes are used to identify macrophage subsets (Mihail and Christian, 2011). An over-simplified generalisation of this concept recognises M1 and M2 macrophages. M1 macrophages have inflammatory and anti-tumour properties, while M2 macrophages have anti-inflammatory and tumour-promoting abilities (Han et al., 2019). M1 macrophages mainly secrete proteins encoded by interleukin 12A (IL12A), interleukin 12 B (IL12B), and tumour necrosis factor (TNF), whereas M2 macrophages typically produce proteins encoded by interleukin 10 (IL10), interleukin 1 receptor antagonist (IL1RN), and interleukin 1 receptor type 2 (IL1R2) (Frumento et al., 2006). The published marker genes for M1 macrophages include IL1B and NFKB1, while those for M2 macrophages include MERTK, MRC1, STAB1, and CD163. Among the above 12 marker genes (Supplementary Figure S19), no single one can be used to clearly discriminate M1 from M2 macrophages. For instance, 65.84% (607/922) of the macrophage type-1 cells and 88.18% (1,134/1,286) of the macrophage type-2 cells expressed CD163 at similar average levels. Another instance is MERTK that was expressed at very high levels in both macrophage type 1 and type 2 cells. Then, we identified the macrophage type-1 and type-2 clusters as M1-like and M2-like macrophage (m1Maph and m2Maph) clusters, using the five marker genes (Table 1) together, respectively.
In m1Maph, 66.3, 83.4, 64.5, 82.5, and 71.3% of the cells expressed the five marker genes OLR1, EREG, BCL2A1, SLC11A1, and NLRP3, respectively (Figure 1B). According to annotations from the GeneCards database (Marilyn et al., 2002), OLR1 (also named LOX-1), as a notable SR gene, is up-regulated in responses to stimulation by OxLDL, proinflammatory cytokines, and proatherogenic factors such as angiotensin II in ECs. According to a previous study (Wen et al., 2020), OxLDL-induced NLRP3 inflammasome activation in macrophages plays a vital role in atherogenesis. Thus, the detection of OLR1 and NLRP3 at very high expression levels revealed that OxLDL induced NLRP3 inflammasome activation in m1Maph through OLR1 (named as the OxLDL-OLR1-NLRP3 process). Although the nine other SR genes (MRC1, STAB1, CD163, MSR1, CD36, CD68, CXCL16, CLEC7A, and LILRB5) were also expressed at very high levels in m1Maph, they may not be involved in or contribute slightly to the OxLDL-NLRP3 process, as they were very highly expressed with markedly lower co-expression of OLR1 and NLRP3 in m2Maph. Among all types of cells in the cavernous hemangioma, only m1Maph highly expressed EREG, which provided a deeper understanding of the origin and functions of epiregulin in the immune responses. According to annotations from the GeneCards database (Marilyn et al., 2002), the protein, epiregulin, encoded by EREG is a ligand of EGFR and structurally related erb-b2 receptor tyrosine kinase 4 (ERBB4). According to previous studies, EREG may be involved in a wide range of biological processes, including inflammation, wound healing, oocyte maturation, and cell proliferation. In particular, EREG promotes cancer progression in various human tissues. By single-cell transcriptome analysis, a previous study (Ma et al., 2021) has revealed that EREG is predominantly expressed in macrophages in the TME and induces EGFR-tyrosine kinase inhibitor (TKI) resistance in the treatment of non-small cell lung cancer (NSCLC) by preventing apoptosis through the EGFR/ErbB2 heterodimer. In the present study, EGFR was expressed at high levels in fibroblasts, SMCs and MSCs, while ERBB4 was barely detected in all types of cells. The above results suggested that 1) m1Maph may promote the tumour progression through the binding of EREG to EGFR, challenging the current theory that M1 macrophages have inflammatory and anti-tumour properties. 2) EGFR inhibitors can be used to treat cavernous hemangiomas.
In m2Maph, 66.3, 72.6, 83.3, 78, and 85% of the cells expressed the five marker genes FOLR2, LILRB5, C1QC, MS4A4A, and C1QB, respectively (Figure 1B). According to previous studies, the serum complement subcomponent, C1q, is composed of 18 polypeptide chains which include six A-chains, six B-chains, and six C-chains, encoded by complement C1q A chain (C1QA), complement C1q B chain (C1QB), and complement C1q C chain (C1QC) genes. The C1q protein enhances the survival and efferocytosis of macrophage foam cells, which is thought to be induced by LDL, including OxLDL or minimally modified LDL (mmLDL) (Shashkin et al., 2005). In addition, understanding the molecular mechanisms underlying OxLDL- and mmLDL-induced macrophage foam cell formation is of fundamental importance for atherosclerosis and cardiovascular disease. Among all types of cells in the cavernous hemangioma, only m2Maph highly expressed C1QA, C1QB, and C1QC, which provided a deeper understanding of the origin and functions of C1q in the immune responses. However, whether m2Maph included macrophage foam cells is unknown. Another previous study (Chen et al., 2021) has reported that the expression levels of C1QA, C1QB, and C1QC are positively related to M1, M2 macrophages and CD8+ cells, and negatively correlated with M0 macrophages, whereas our results demonstrated that C1QA, C1QB, and C1QC were expressed at very high levels in m2Maph, very low levels in m1Maph and barely detected in activated CD8+T cells. The detection of highly expressed LILRB5, C1QA, C1QB, and C1QC suggested that OxLDL induced the inflammatory activation of C1q (named as the OxLDL-LILRB5-C1q process) in m2Maph through LILRB5. Although the nine other SR genes (OLR1, MRC1, STAB1, CD163, MSR1, CD36, CD68, CXCL16, and CLEC7A) were also expressed at very high levels in m2Maph, they may not be involved in or contribute minimally to the OxLDL-LILRB5-C1q process, as they were very highly expressed with markedly lower co-expression of LILRB5, C1QA, C1QB, and C1QC in m1Maph. Based on the above results, we proposed that OxLDL induces the OxLDL-OLR1-NLRP3 process in m1Maph and the OxLDL-LILRB5-C1q process in m2Maph (Figure 4).
T Lymphocytes and NKCs
The TC1, TC2, and TC3 clusters containing 5.53% (596/10,784), 2.85% (307/10,784), and 1.86% (201/10,784) of the total cells, respectively, were further identified as CD4+TC, CD8+TC, and NKC clusters (Described as above). The cells in the three clusters were entangled, as CD4+TC, CD8+TC, and NKC expressed some common genes without significant differences. For instance, both CD8+TC and NKC expressed NKG7 (a marker gene of NK cells) at very high levels. Therefore, we compared the expression levels of more relevant genes to confirm the cell types of the three clusters. The NK cells were confirmed based on the following evidences (Supplementary Figure S20): 1) the average expression levels of CD3D, CD3E, CD3G, CD4, CD8A, and CD8B (marker genes of T cells) in NKC were lower than 5% of those in CD4+TC and CD8+TC, respectively; 2) the average expression level of NKG7 in NKC was approximately 92-fold higher than that in CD4+TC; and 3) the average expression levels of GZMA, GZMB, GZMH, GZMK, GZMM, CRTAM, and GNLY in NKC were approximately 12.3, 51, 4.56, 102.2, 1, 11.2, and 151-fold higher than those in CD4+TC, respectively. Although both CD4+TC and CD8+TC expressed CD8B (a marker gene of CD8+T cells) at a similar level, the cells in CD4+TC could still be clearly discriminated from the cells in CD8+TC based on the following evidences: 1) the average expression level of CD4 in CD4+TC was higher than 5-fold of that in CD8+TC; 2) the average expression level of CD8A in CD8+TC was higher than 4-fold of that in CD4+TC; 3) the average expression levels of NKG7, GZMA, GZMB, GZMH, GZMK, GZMM, CRTAM, and GNLY in CD4+TC were markedly lower than those in NKC and CD8+TC; and 4) the average expression levels of GZMA, GZMH, GZMK, and GZMM in CD8+TC were higher than 2-fold of those in CD4+TC, while the average expression levels of GZMB, CRTAM, and GNLY in CD8+TC were only approximately 53.1, 35.7, and 15% of those in NKC, respectively. By further analysis of gene-expression signatures of CD4+TC, CD8+TC, and NKC, we obtained two new results (Figure 4): 1) CCR7 was expressed at a markedly higher level than CD4, CD8A, and CD8B, and the average expression level of CCR7 in CD4+TC was higher than 38-fold of that in CD8+TC and 20-fold of that in NKC; and 2) CST7 was detected to be significant lower expressed in the non-immune cells than the immune cells, particularly CD8+TC and NKC. However, the expression of CST7 has been detected in various human cancer cell lines established from malignant tumours. According to annotations from the GeneCards database (Marilyn et al., 2002), CST7 encodes a glycosylated cysteine protease inhibitor with a putative role in immune regulation through the inhibition of a unique target in the hematopoietic system. Based on the above results, we concluded that CCR7 has the potential to be a new marker to discriminate CD4+T cells from activated CD8+T cells. The specific functions of highly expressed CST7 in CD8+T and NK cells merit investigation in future studies.
Among the top 20 highly expressed genes ranked by their average (the arithmetic mean) expression levels, both CCL4 and CCL5 encode chemokine ligands that have chemokinetic and inflammatory functions by binding to their receptors encoded by CCR5. According to previous studies of CCL4 (Mukaida et al., 2020) and CCL5 (Dangaj et al., 2019), the over-expression of CCL5 is associated with CD8+T cell infiltration in solid tumours, while CCL4 can promote tumour development and progression by recruiting regulatory T cells and pro-tumorigenic macrophages, and acting on other resident cells (e.g., fibroblasts and endothelial cells) present in the tumour microenvironment (TME) to facilitate their pro-tumorigenic capacities. In some situations, CCL4 can enhance tumour immunity by recruiting cytolytic lymphocytes and macrophages with phagocytic ability. Furthermore, the previous study (Dangaj et al., 2019) has reported that the T cell infiltration requires tumour cell-derived CCL5, and this process is amplified by IFN-γ-inducible, myeloid cell-secreted CXCL9. As IFN-γ is encoded by IFNG, we named this amplification process as tumour cell-derived CCL5-IFNG-CXCL9 process. In the previous study (Dangaj et al., 2019), the co-expression of CCL5 and CXCL9 has been detected to reveal immunoreactive tumours with prolonged survival and response to PD-1 inhibition. By examining the expression levels of CCL4, CCL5, CCR5, IFNG, CXCL9, and PDCD1 (well-known as PD-1), we found that (Supplementary Figure S21): 1) CCL4 was detected to be significant lower expressed in the non-immune cells than the immune cells, particularly CD8+TC, NKC and m2Maph; 2) CCL5 was expressed at the highest level in CD8+TC and the second highest level in NKC; 3) CCR5 was very lowly expressed in CD8+TC and m1Maph and barely detected in other cells and; 4) IFNG and CXCL9 were barely detected in all types of cells; and 5) PDCD1 (well-known as PD-1) was only barely detected in CD4+TC and CD8+TC. Based on the above results, we concluded that the tumour cell-derived CCL5-IFNG-CXCL9 process was not induced in cavernous hemangiomas. The specific functions of highly expressed CCL4 and CCL5 in CD8+T and NK cells merit investigation in future studies (Figure 4).
Other Cells
For the other five clusters, LECs, BCs, mDCs, pDCs, and CLEC9A + DCs contained 0.35% (38/10784), 1.1% (119/10784), 2.47% (266/10784), 0.33% (36/10784), and 0.44% (47/10784) of the total cells, respectively. Differential expression analysis (Materials and Methods) between cells inside and outside each cluster was performed to produce a gene-expression signature including all DE genes (Supplementary File S2). The top five DE genes were selected as a combination of marker genes for each cell type (Table 1). Of note, CXCR4, GZMB, and CYSLTR1 were detected to be expressed in the pDC cluster at very high levels (Figure 4). The high co-expression of CXCR4 and GZMB suggested that pDCs function for anti-tumour as CD8+T cells in cavernous hemangiomas. Although the proportion of pDCs was markedly lower than that of CD8+T cells, their contribution to anti-tumour activity may complement the loss caused by checkpoint blockade in CD8+T cells. CYSLTR1 encodes a protein that is a second receptor for cysteinyl leukotrienes and is thought to be the main receptor mediating cysteinyl leukotriene receptor smooth-muscle contraction and inflammatory cell cytokine production in asthma. However, the specific functions of highly expressed CYSLTR1 in pDCs remain unknown.
Conclusion and Discussion
In the present study, we identified 16 cell types in a cavernous hemangioma using scRNA-seq and provided a gene-expression signature (Supplementary File S2) and a combination of five marker genes (Table 1) to each cell type. These gene-expression signatures can be used as references for the exact identification of these cell types in future studies. The main contribution of the present study is that we discovered a large number (5.66% of the total cells) of embryonic MSCs in the cavernous hemangioma. According to the current theory, hemangiomas originate from neogenesis or revival of dormant embryonic angioblasts and arise through hormonally driven vessel growth (Introduction). However, we ruled out the possibility that the MSCs were transformed from angioblasts. We also ruled out the possibilities that the MSCs were pericyte derivatives, CAFs, bone marrow-derived MSCs or generated via EMT. Therefore, we proposed that cavernous hemangiomas may originate embryonic MSCs. Different types of hemangiomas probable originate from embryonic MSCs in different tissues or under different conditions. Further analysis of the embryonic MSCs revealed that:1) proinflammatory cytokines and related genes TNF, TNFSF13B, TNFRSF12A, TNFAIP6, and C1QTNF6 are significantly involved in the MSC-induced immune responses in cavernous hemangiomas; 2) UCHL1 is up-regulated in the embryonic MSC apoptosis induced by proinflammatory cytokines (e.g., TNF and TNFSF13B); 3) the UCHL1-induced apoptosis of MSCs may play an important role in the MSC-induced immune responses in cavernous hemangiomas; and 4) UCHL1 can be used as a marker gene to detect embryonic MSCs at different apoptosis stages. In addition to MSCs, ECs, macrophages, T lymphocytes and NKCs were intensively investigated, revealing the genes and pathways featured in cavernous hemangiomas (Figure 4).
Our on coming research work will be conducted, following two clues: identification of additional embryonic MSCs-caused cancers/tumours and investigation of UCHL1-induced apoptosis in other types of cancers/tumours. To achieve the first goal, it requires the use of the gene expression signature from the present study as a reference to identify the embryonic MSCs in more other types of cancers/tumours with the combination of different machine learning methods on public scRNA-seq data. The discovery of UCHL1-induced apoptosis of MSCs in cavernous hemangiomas preliminarily explained the benign nature of cavernous hemangiomas. However, the molecular mechanism underlying the UCHL1-induced apoptosis of MSCs is still unknown. We proposed that proinflammatory cytokines (e.g., TNF and TNFSF13B) triggered the UCHL1-induced apoptosis through their receptors (e.g., TNFRSF12A) and this process can be regulated by other proteins (e.g., TNFAIP6 and C1QTNF6). The better understanding of the MSC-induced immune responses in benign tumours helps to guide future investigation and treatment of embryonic MSC-caused tumours. For example, POSTN and UCHL1 can be used to control the differentiation, development or apoptosis of transplanted MSCs. Proinflammatory cytokines (e.g., IFN-γ and TNF-α) can be used to treat hemangiomas or other embryonic MSC-caused tumours via the UCHL1-induced apoptosis of MSCs. As TNFAIP6 can be induced by both IFN-γ and TNF-α, it can be used as a marker to evaluate the treatment.
Materials and Methods
10x Genomics Library Preparation and Sequencing
A piece of tissue in the centre of the tumour (Results) was digested for 0.5 h at 37°C in the enzyme solution (Enzyme H, R and A) using a gentleMACS Dissociator, following the manufacturer’s instruction. The single-cell suspension was filtered with a 40-μm-diameter cell strainer (FALCON, United States), then washed twice with RPMI1640 wash buffer at 4°C. Cell viability was determined by trypan blue staining with TC20 automated cell counter (BioRad, United States). The ratio of viable cells in single-cell suspension was more than 85%. The single-cell suspension was adjusted to have a concentration of 700–1,200 cells/μl, and then processed to generate 10x libraries with the Chromium Single Cell 3’ Reagent Kits v3 CG000183 (10x Genomics, United States) following the manufacturer’s instruction. These libraries were sequenced by an Illumina NovaSeq 6,000 sequencer, producing 542,088,397 pairs of 150-bp reads (∼163 Gbp raw scRNA-seq data).
ScRNA-Seq Data Processing
Using the software Cell Ranger v4.0.0, we aligned 542,088,397 read2 sequences (∼) to the human genome GRCh38, generating an UMI-count matrix (33,694 genes × 6,794,880 cell barcodes). The cell-calling algorithm in Cell Ranger was used to identify 12,018 cells from the 6,794,880 cell barcodes. Then, a total of 10,784 cells and 22,023 genes were retained with a median of 2,084 genes per cell after quality control (QC) filtering using the following parameters: 1) genes detected in <3 cells were excluded; 2) cells with <200 genes were excluded; 3) cells with >30% mitochondrial RNA UMI counts or >5% hemoglobin RNA UMI counts were excluded; 4) 982 doublet artifacts were removed with DoubletFinder. Finally, a 22,010 × 10,784 matrix and a 13 × 10,784 matrix were produced to represent expression levels of nuclear and mitochondrial genes, respectively. The data including two matrices is available at the NCBI GEO database under the accession number GSE188515.
The R package Seurat v4.1.0 and other R packages (e.g., ggplot2) were used for scRNA-seq data analysis on R v4.1.3 (Gao et al., 2014). Each column of the nuclear UMI-count matrix (22,010 × 10,784) was normalized using the NormalizeData function by dividing the sum of each column, then multiplying by a scale factor of 10,000 and taking its natural-log transformed value. We selected 2000 highly variable genes on the basis of the average expression and dispersion per gene using the FindVariableFeatures function. After data scaling, principal component analysis (PCA) was performed on the 2000 highly variable genes (not the total 22,010 genes) using the RunPCA function. The top 50 principal components were chosen from 100 calculated principal components for cell clustering using the FindNeighbors and FindClusters functions with parameters (algorithm = 4, resolution = 0.4). The Uniform Manifold Approximation and Projection (UMAP) method was used on the PCA&clustering result to show all the clusters.
Identification of Cell Types and Selection of Marker Genes
For each cluster, differential expression analysis between cells inside and outside the cluster was performed to produce a gene-expression signature using the R package DESeq2. We used the function (scran:computeSumFactors) for scRNA-seq data analysis in DESeq2 for the normalization of the nuclear UMI-count matrix (22,010 × 10,784), which is different from the default normalization method in DESeq2 or that in Seurat. Then, we run the main function DESeq with the following parameters (test = “LRT”, fitType = “glmGamPoi”, minReplicatesForReplace = Inf, useT = TRUE, minmu = 1e-6). Genes with expression levels below 10% in both of the two groups of cells, were filtered out. For each cluster, a gene-expression signature includes all differentially expressed (DE) genes meeting two criteria (adjusted p-value ≤ 0.01 and LFCio ≥ 2). LFCio is the 2-based log-transformed fold change (i.e.,“log2FoldChange” calculated by DESeq2) between the average (arithmetic mean) expression levels of a gene inside and that outside a cluster, and Avg_in is the average expression level of a gene in the cluster. The identification of each cluster as a specific cell type took two-steps (the rough and exact identification). Firstly, each cluster was roughly identified by comparing several selected DE genes to known marker genes. As for the known marker genes, 1) most of them were used according to records in an online database (http://biocc.hrbmu.edu.cn/CellMarker/) by Harbin Medical University; and 2) a few were used according to records in published papers, e.g., LINC00926 (Zhang et al., 2018).
Then, each cluster was exactly identified by further analysis of its gene-expression signature. GO and pathway annotation with analysis were performed using the Metascape website (https://metascape.org/gp) (Zhou et al., 2019). The top five DE genes was selected as a combination of marker genes from the gene-expression signature for each cluster (Table 1), meeting the following criteria: 1) the percentage of cells that expressed the gene inside the cluster (PCTin) > 60%; and 2) the ratio between the percentage of cells that expressed the gene inside (PCTin) and outside the cluster (PCTout) is ranked in the top five. As LFCio or the ratio between PCTin and PCTout can only be used to evaluate the identification of a cell type by a single marker gene, we designed another metric UICC to evaluate the representation of a cell type by a combination of marker genes. The cardinal number of the union set of cells expressed marker genes is divided by the number of all cells in a cluster to calculate the union coverage of a cluster (UCC). The cardinal number of the intersection set of cells expressed marker genes is divided by the number of all cells in a cluster to calculate the intersection coverage of a cluster (ICC). To balance UCC and ICC, we designed UICC, which is calculated by multiplying UCC by ICC.
Data Availability Statement
The original contributions presented in the study are included in the article/Supplementary Material, further inquiries can be directed to the corresponding authors. Finally, a 22,010 × 10,784 matrix and a 13 × 10,784 matrix were produced to represent expression levels of nuclear and mitochondrial genes, respectively. The data including two matrices is available at the NCBI GEO database under the accession number GSE188515.
Ethics Statement
The studies involving human participants were reviewed and approved by Authorization of The Ethical Committee of Tianjin Medical University General Hospital. Written informed consent to participate in this study was provided by the participants’ legal guardian/next of kin. Written informed consent was obtained from the minor(s)’ legal guardian/next of kin for the publication of any potentially identifiable images or data included in this article.
Author Contributions
SG conceived the project. SG and DS supervised the present study. FJ performed the surgery. YL collected the sample and performed the histological examination. JS performed programming. CL performed statistics and plotting. SG and JS analyzed the data. SF, HW and BR collected specimens and clinical data. SG drafted the main manuscript text. SG and DM revised the manuscript.
Funding
This work was supported by the National Natural Science Foundation of China (81770537 and 82070554) to DS. The funding bodies played no role in the study design, data collection, analysis, interpretation, or manuscript writing.
Conflict of Interest
The authors declare that the research was conducted in the absence of any commercial or financial relationships that could be construed as a potential conflict of interest.
Publisher’s Note
All claims expressed in this article are solely those of the authors and do not necessarily represent those of their affiliated organizations, or those of the publisher, the editors and the reviewers. Any product that may be evaluated in this article, or claim that may be made by its manufacturer, is not guaranteed or endorsed by the publisher.
Acknowledgments
We are grateful for the help from the following doctors at Tianjin Medical University General Hospital: Fang Liu, Wenjing Song and Xiaohui Liang from the pathology department; Junping Wang from the medical imaging department. Special thanks need to be given to Tung On Yau from School of Science and Technology, Nottingham Trent University, United Kingdom, Jishou Ruan from School of Mathematical Sciences, and Chang Liu and Jianlin Cui from School of Medicine, Nankai University. We would like to thank Editage (www.editage.cn) for polishing part of this manuscript in English language. This manuscript was online as a preprint on 2 September 2021 at https://biorxiv.org/cgi/content/short/2021.09.02.458742v1.
Supplementary Material
The Supplementary Material for this article can be found online at: https://www.frontiersin.org/articles/10.3389/fcell.2022.916045/full#supplementary-material
References
Alquraini, A., and El Khoury, J. (2020). Scavenger Receptors. Curr. Biol. 30 (14), R790–R795. doi:10.1016/j.cub.2020.05.051
Arnett, H. A., and Viney, J. L. (2014). Immune Modulation by Butyrophilins. Nat. Rev. Immunol. 14 (8), 559–569. doi:10.1038/nri3715
Beck, D. O., and Gosain, A. K. (2009). The Presentation and Management of Hemangiomas. Plastic Reconstr. Surg. 123 (6), 181e–191e. doi:10.1097/prs.0b013e3181a65c59
Borecka, P., Ratajczak-Wielgomas, K., Ciaputa, R., Kandefer-Gola, M., Janus, I., Piotrowska, A., et al. (2020). Expression of Periostin in Cancer-Associated Fibroblasts in Mammary Cancer in Female Dogs. Vivo 34 (3), 1017–1026. doi:10.21873/invivo.11870
Chen, L. H., Liu, J. F., Lu, Y.-., He, X. Y., and Zhou, H. H. (2021). Complement C1q (C1qA, C1qB, and C1qC) May Be a Potential Prognostic Factor and an Index of Tumor Microenvironment Remodeling in Osteosarcoma. Front. Oncol., 11. doi:10.3389/fonc.2021.642144
Dangaj, D., Bruand, M., Grimm, A. J., Ronet, C., Barras, D., Duttagupta, P. A., et al. (2019). Cooperation between Constitutive and Inducible Chemokines Enables T Cell Engraftment and Immune Attack in Solid Tumors. Cancer Cell. 35 (6), 885–900. doi:10.1016/j.ccell.2019.05.004
Dominici, M., Le Blanc, K., Mueller, I., Slaper-Cortenbach, I., Marini, F. C., Krause, D. S., et al. (2006). Minimal Criteria for Defining Multipotent Mesenchymal Stromal Cells. The International Society for Cellular Therapy Position Statement. Cytotherapy 8 (4), 315–317. doi:10.1080/14653240600855905
Dorota, T. B., Ewa, M., Marzena, T., Beata, S., Marta, K., Ewa, G., et al. (2018). Overexpression of Ubiquitin Carboxyl-Terminal Hydrolase 1 (UCHL1) in Boys with Cryptorchidism. Plos One 13 (2), e0191806.
Errani, C., Zhang, L., Panicek, D. M., Healey, J. H., and Antonescu, C. R. (2012). Epithelioid Hemangioma of Bone and Soft Tissue: a Reappraisal of a Controversial Entity. Clin. Orthop. Relat. Res. 470 (5), 1498–1506. doi:10.1007/s11999-011-2070-0
Frumento, G., Piazza, T., Di Carlo, E., and Ferrini, S. (2006). Targeting Tumor-Related Immunosuppression for Cancer Immunotherapy. Endocr. Metab. Immune Disord. Drug Targets 6 (3), 233–237. doi:10.2174/187153006778250019
Fu, J. X., Zou, Y. N., Han, Z. H., Yu, H. X-J., and Wang, X. J. (2020). Small Bowel Racemose Hemangioma Complicated with Obstruction and Chronic Anemia: A Case Report and Review of Literature. World J. Gastroenterol. 26 (14), 1674–1682. doi:10.3748/wjg.v26.i14.1674
Gao, S. (2018). Data Analysis in Single-Cell Transcriptome Sequencing. Methods Mol. Biol. 1754, 311–326. doi:10.1007/978-1-4939-7717-8_18
Gao, S., Ou, J., and Xiao, K. (2014). R Language and Bioconductor in Bioinformatics Applications(Chinese Edition). Tianjin: Tianjin Science and Technology Translation Publishing Ltd.
Gu, Y., Ding, X., Huang, J., Xue, M., Zhang, J., Wang, Q., et al. (2018). The Deubiquitinating Enzyme UCHL1 Negatively Regulates the Immunosuppressive Capacity and Survival of Multipotent Mesenchymal Stromal Cells. Cell. Death Dis. 9 (5), 459. doi:10.1038/s41419-018-0532-y
Han, D., Fang, Y., Guo, Y., Hong, W., Tu, J., and Wei, W. (2019). The Emerging Role of Long Non-coding RNAs in Tumor-Associated Macrophages. J. Cancer 10 (26), 6738–6746. doi:10.7150/jca.35770
Jang, C. H., Choi, H. S., Hong, Y. S., and Cho, Y. B. (2011). Cavernous Hemangioma of the Tympanic Membrane. Clin. Exp. Otorhinolaryngol. 4 (2), 109–111. doi:10.3342/ceo.2011.4.2.109
Kikuchi, Y., Kunita, A., Iwata, C., Komura, D., Nishiyama, T., Shimazu, K., et al. (2014). The Niche Component Periostin Is Produced by Cancer-Associated Fibroblasts, Supporting Growth of Gastric Cancer through ERK Activation. Am. J. Pathology 184 (3), 859–870. doi:10.1016/j.ajpath.2013.11.012
Kim, H., Pawlikowska, L., Hua, S., and Young, W. L. (2016). Genetics and Vascular Biology of Angiogenesis and Vascular Malformations Stroke (Sixth Edition). Amsterdam: Elsevier.
Kim, J. H., Choi, J. G., and Son, B. C. (2018). Venous Malformation (Cavernous Hemangioma) of the Supraorbital Nerve. Asian J. Neurosurg. 13 (2), 499–502. doi:10.4103/ajns.AJNS_166_16
Kuppe, C., Ibrahim, M. M., Kranz, J., Zhang, X., Ziegler, S., Perales-Patón, J., et al. (2021). Decoding Myofibroblast Origins in Human Kidney Fibrosis. Nature 589 (7841), 281–286. doi:10.1038/s41586-020-2941-1
Li, Y., Ren, P., Dawson, A., Vasquez, H. G., Ageedi, W., Zhang, C., et al. (2020). Single-Cell Transcriptome Analysis Reveals Dynamic Cell Populations and Differential Gene Expression Patterns in Control and Aneurysmal Human Aortic Tissue. Circulation 142 (14), 1374–1388. doi:10.1161/circulationaha.120.046528
Liu, T., Han, C., Wang, S., Fang, P., Ma, Z., Xu, L., et al. (2019). Cancer-associated Fibroblasts: An Emerging Target of Anti-cancer Immunotherapy. J. Hematol. Oncol. 12 (1), 86. doi:10.1186/s13045-019-0770-1
Ma, S., Zhang, L., Ren, Y., Dai, W., Chen, T., Luo, L., et al. (2021). Epiregulin Confers EGFR-TKI Resistance via EGFR/ErbB2 Heterodimer in Non-small Cell Lung Cancer. Oncogene 40 (14), 2596–2609. doi:10.1038/s41388-021-01734-4
Mai, J., Virtue, A., Shen, J., Wang, H., and Yang, X.-F. (2013). An Evolving New Paradigm: Endothelial Cells - Conditional Innate Immune Cells. J. Hematol. Oncol. 6 (1), 61. doi:10.1186/1756-8722-6-61
Malanchi, I., Santamaria-Martínez, A., Susanto, E., Peng, H., Lehr, H.-A., Delaloye, J.-F., et al. (2012). Interactions between Cancer Stem Cells and Their Niche Govern Metastatic Colonization. Nature 481 (7379), 85–89. doi:10.1038/nature10694
Marilyn, S., Irina, S., Orit, S., Michal, L., Shai, S. O., Avital, A., et al. (2002). GeneCards 2002: towards a Complete, Object-Oriented, Human Gene Compendium. Bioinformatics 18 (11), 1542–1543.
McLean, K., Gong, Y., Choi, Y., Deng, N., Yang, K., Bai, S., et al. (2011). Human Ovarian Carcinoma–Associated Mesenchymal Stem Cells Regulate Cancer Stem Cells and Tumorigenesis via Altered BMP Production. J. Clin. Investig. 121 (8), 3206–3219. doi:10.1172/JCI45273
Mihail, H., and Christian, W. (2011). Differential Role of Monocyte Subsets in Atherosclerosis. Thromb. Haemost. 105 (11), 757–762.
Mukaida, N., Sasaki, S.-i., and Baba, T. (2020). CCL4 Signaling in the Tumor Microenvironment. Adv. Exp. Med. Biol. 1231, 23–32. doi:10.1007/978-3-030-36667-4_3
Roberts, L. R. (2012). Liver and Biliary Tract Tumors. Goldman's Cecil Medicine (the 24th Edition). Amsterdam: Elsevier, 1297–1303. doi:10.1016/b978-1-4377-1604-7.00202-5
Sahai, E., Astsaturov, I., Cukierman, E., Denardo, D. G., Egeblad, M., Evans, R. M., et al. (2020). A Framework for Advancing Our Understanding of Cancer-Associated Fibroblasts. Nat. Rev. Cancer 20 (3), 174–186. doi:10.1038/s41568-019-0238-1
Shashkin, P., Dragulev, B., and Ley, K. (2005). Macrophage Differentiation to Foam Cells. Cpd 11 (23), 3061–3072. doi:10.2174/1381612054865064
Slukvin, I. I., and Vodyanik, M. (2011). Endothelial Origin of Mesenchymal Stem Cells. Cell. Cycle 10 (9), 1370–1373. doi:10.4161/cc.10.9.15345
Tan, S. T., Velickovic, M., Ruger, B. M., and Davis, P. F. (2000). Cellular and Extracellular Markers of Hemangioma. Plastic Reconstr. Surg. 106 (3), 529–538. doi:10.1097/00006534-200009010-00001
Wang, W.-y., Wang, L.-h., Huang, G., Lin, Z.-y., and Lin, H. (2019). Intralesional Injection of Triamcinolone Acetonide for Cavernous Hemangiomas. Med. Baltim. 98 (37), e16986. doi:10.1097/md.0000000000016986
Wang, W., and Chen, L. (2017). Cavernous Hemangioma of the Retina. Retina 37 (4), 611–621. doi:10.1097/iae.0000000000001374
Wen, J., Chang, Y., Huo, S., Li, W., Huang, H., Gao, Y., et al. (2020). Tanshinone IIA Attenuates Atherosclerosis via Inhibiting NLRP3 Inflammasome Activation. Aging (Albany NY) 12 (1). doi:10.18632/aging.202202
Zhang, M. J., Ntranos, V., and Tse, D. (2018). One Read Per Cell Per Gene Is Optimal for Single-Cell RNA-Seq.
Zhang, Y.-P., Zhu, Y.-B., Duan, D. D., Fan, X.-M., He, Y., Su, J.-W., et al. (2015). Serum UCH-L1 as a Novel Biomarker to Predict Neuronal Apoptosis Following Deep Hypothermic Circulatory Arrest. Int. J. Med. Sci. 12 (7), 576–582. doi:10.7150/ijms.12111
Zhou, Y., Zhou, B., Pache, L., Chang, M., Khodabakhshi, A. H., Tanaseichuk, O., et al. (2019). Metascape Provides a Biologist-Oriented Resource for the Analysis of Systems-Level Datasets. Nat. Commun. 10 (1), 1523. doi:10.1038/s41467-019-09234-6
Keywords: MSC, UCHL1, vascular tumour, stem cell, scRNA-seq
Citation: Ji F, Liu Y, Shi J, Liu C, Fu S, Wang H, Ren B, Mi D, Gao S and Sun D (2022) Single-Cell Transcriptome Analysis Reveals Mesenchymal Stem Cells in Cavernous Hemangioma. Front. Cell Dev. Biol. 10:916045. doi: 10.3389/fcell.2022.916045
Received: 08 April 2022; Accepted: 13 June 2022;
Published: 05 July 2022.
Edited by:
Yanquan Zhang, University of Kentucky, United StatesReviewed by:
Seiji Yamamoto, University of Toyama, JapanChaohao Li, University of Kentucky, United States
Copyright © 2022 Ji, Liu, Shi, Liu, Fu, Wang, Ren, Mi, Gao and Sun. This is an open-access article distributed under the terms of the Creative Commons Attribution License (CC BY). The use, distribution or reproduction in other forums is permitted, provided the original author(s) and the copyright owner(s) are credited and that the original publication in this journal is cited, in accordance with accepted academic practice. No use, distribution or reproduction is permitted which does not comply with these terms.
*Correspondence: Shan Gao, Z2FvX3NoYW5AbWFpbC5uYW5rYWkuZWR1LmNu; Daqing Sun, c2RxY2hyaXMyMDE5QHRtdS5lZHUuY24=
†These authors have contributed equally to this work