- 1Department of Developmental Biology, School of Basic Medical Sciences, Southern Medical University, Guangzhou, China
- 2Department of Obstetrics and Gynecology, Nanfang Hospital, Southern Medical University, Guangzhou, China
The microbiome exerts profound effects on fetal development and health, yet the mechanisms underlying remain elusive. N6-methyladenosine (m6A) plays important roles in developmental regulation. Although it has been shown that the microbiome affects the mRNA m6A modification of the host, it remains unclear whether the maternal microbiome affects m6A epitranscriptome of the fetus so as to impact fetal development. Here, we found that loss of the maternal microbiome altered the expression of m6A writers and erasers, as well as the m6A methylome of the mouse fetal brain and intestine on embryonic day 18. From the m6A profiles, we identified 2,655 and 2,252 m6A modifications regulated by the maternal microbiome in the fetal brain and intestine, respectively, and we demonstrated that these m6A-modified genes were enriched in the neuro/intestinal developmental pathways, such as the Wnt signaling pathway. Finally, we verified that antibiotic treatment mostly recapitulated changes in m6A, and we further showed that the loss of heterozygosity of Mettl3 rescued m6A levels and the expression changes of some developmental genes in the fetal intestine that resulted from antibiotic treatment. Collectively, our data revealed that the maternal microbiome programs the m6A epitranscriptome of the mouse fetal brain and intestine.
Introduction
It is universally recognized that the microbiome exerts profound effects on host physiology and health, including host metabolism, circadian rhythm, intestinal morphology, and the development of the nervous system (Zhang et al., 2020; Brooks et al., 2021; Seki et al., 2021; Willyard, 2021; Wu J. et al., 2021; Wu Q. et al., 2021). Emerging studies have revealed that loss of the maternal microbiome impairs axonogenesis (Vuong et al., 2020), and that maternal exposure to antibiotics contributes to gut dysbiosis, immune dysfunction, and the occurrence of inflammatory bowel disease (IBD) in offspring (Miyoshi et al., 2017). These findings indicated that loss of the maternal microbiome impairs the fetal development and neonatal health in early life. However, the mechanisms underlying the actions of the maternal microbiome on the fetus remain elusive.
N6-methyladenosine (m6A) has been shown to be the most abundant and a highly conserved modification on messenger RNAs (mRNAs) and lncRNAs in mammals (Dominissini et al., 2012; Zhao et al., 2017a; Liu et al., 2020; Shu et al., 2020; Deng et al., 2021). mRNA m6A possesses a consensus motif of RRACH (R denoting G or A, and H reflecting A, C, or U) and it is principally found at stop codons, 3´untranslated regions (3´UTRs), and long exons (Dominissini et al., 2012; Roundtree et al., 2017). m6A is produced by the METTL3–METTL14 core methyltransferase complex (Liu et al., 2014; Liu X. et al., 2021), erased by demethylases FTO and ALKBH5 (Jia et al., 2011; Zheng et al., 2013; Roundtree et al., 2017), and recognized by readers such as the YTH family proteins (Dominissini et al., 2012; Wang X. et al., 2014; Alarcón et al., 2015). m6A regulates various physiological processes, such as RNA stability (Dominissini et al., 2012; Wang X. et al., 2014; Alarcón et al., 2015; Huang et al., 2018), splicing (Xiao et al., 2016; Ke et al., 2017), translation (Meyer et al., 2015; Wang et al., 2015; Zhou et al., 2015; Shi et al., 2017), and signaling pathways (Li H.-B. et al., 2017; Huang H. et al., 2019; Uddin et al., 2021). Also, it occupies important roles in stem cell self-renewal (Li et al., 2018; Liu J. et al., 2021), embryonic development (Batista et al., 2014; Wang Y. et al., 2014; Chen et al., 2015; Geula et al., 2015; Vu et al., 2017; Bertero et al., 2018), tissue development (Zheng et al., 2013; Li H.-B. et al., 2017; Yoon et al., 2017; Zhao et al., 2017b; Wang et al., 2018), tumorigenesis (Ma et al., 2016; Li Z et al., 2017; Su et al., 2018; Huang Y et al., 2019; Su et al., 2020; Chen et al., 2021), and the progression of other human diseases (Fischer et al., 2009; Church et al., 2010; Mathiyalagan et al., 2019). It has been shown that the microbiome affects the mRNA m6A modification on the host tissues, especially the brain, intestine, and liver (Wang et al., 2019; Jabs et al., 2020). However, it remains unclear whether the maternal microbiome affects m6A epitranscriptome of the fetal brain, intestine, and liver so as to impact fetal development.
Herein, we demonstrated that the expression of m6A writers and erasers in the brain and intestine of the mouse fetus is altered by the maternal microbiome. Using MeRIP-seq, we systematically investigated the transcriptome-wide m6A methylome profiles of the mouse fetal brain and intestine, and we discovered that the maternal microbiome programs the fetal m6A methylome, and that m6A-modified genes regulated by the maternal microbiome are enriched in fetal neuro/intestine developmental pathways, such as the Wnt signaling. More importantly, antibiotic treatment recapitulated m6A alterations in the mouse fetal intestine and brain, and loss of heterozygosity of Mettl3 rescued this effect. Our findings collectively indicate that the maternal microbiome programs the m6A epitranscriptome of the mouse fetal brain and intestine, and this may provide a promising basis to explore the mechanisms by which the maternal microbiome influences fetal development and diseases.
Materials and Methods
Fetal Tissues Collection From SPF and GF Mice
Specific pathogen-free (SPF) pregnant mice (n = 3) and germ-free (GF) pregnant mice (n = 3) purchased from GemPharmatech Co., Ltd. were dissected on embryonic day 18 (E18), and the fetal tissues (brain, intestine, and liver) were collected and stored at −80°C for subsequent analyses.
PCR Amplification and qPCR Analysis of 16S rRNA Genes
A total of 40 mg mouse fecal pellets were suspended in 200 μl lysis buffer (5 mM EDTA, 0.2% SDS, 0.2M NaCl, and 0.1M Tris-HCl) supplemented with 4 μl of 20 mg/ml proteinase K. The mixtures were disrupted with a grinding rod and then incubated at 56 °C for 6 h. After centrifugation, the supernatant was used for 16S rRNA gene amplification, and the PCR products were visualized on a 2% agarose gel stained with ethidium bromide under UV light. The supernatant from CONV and ABX mice was used for the 16S rRNA gene qPCR analysis. The 16S rRNA gene was detected using two sets of universal bacterial primers: 27F and 1492R; 8F and 1541R. The primers are listed in Supplementary Table S1.
Tissues Lysate Preparation and Western Blots
Frozen tissues were homogenized and lysed in RIPA buffer (50 mM Tris-HCl pH 7.5, 1% Nonidet P-40, 0.5% sodium deoxycholate, 0.05% SDS, 1 mM EDTA, and 150 mM NaCl) with freshly added phosphorylase inhibitors and protease inhibitors, and then centrifuged for 20 min at 13,000 × g. The supernatant was aspirated and loaded for the Western immunoblotting analysis. The following antibodies are used: METTL3 (A8370, Abclonal, 1:1,000), METTL14 (HPA038002, Sigma-Aldrich, 1:1,000), FTO (27226-1-AP, Proteintech, 1:1,000), ALKBH5 (16837-1-AP, Proteintech, 1:1,000), and β-actin (66009-1-Ig, Proteintech, 1:5,000).
RNA Isolation and mRNA Purification
Fetal mouse tissues were homogenized in 1 ml of TRNzol Universal Reagent (TIANGEN) with glass beads using a LUKYM-I homogenizer, and total RNA was isolated following the manufacturer’s protocol. mRNA was separated from total RNA using a Dynabeads mRNA purification kit (Thermo Fisher Scientific), with two rounds of purification.
LC-MS/MS Quantification of m6A mRNA Modification
LC-MS/MS was performed essentially as described previously (Li et al., 2020). In brief, purified mRNA was digested to nucleosides by nuclease P1 and CIAP, and then it was diluted to 10 ng/μl using nuclease-free water. The samples were filtered and injected into an Agilent Poroshell 120 column coupled online to an AB SCIEX Triple Quad 5500 LC mass spectrometer (Applied Biosystems) in a positive electrospray ionization mode. Concentrations of m6A and A were determined based on standard curves of the nucleosides, and the m6A/A ratio was calculated.
RT-qPCR
Total RNA (5 μg) from fetal mouse tissues was reverse-transcribed using a GoScript Reverse Transcription System (Promega), and quantitative real-time PCR was executed using a 2 × RealStar Green Power Mixture (GenStar). The fluorescence intensity of the amplification process was monitored using a LightCycler96 system (Roche). The primers are listed in Supplementary Table S2.
Methylated RNA Immunoprecipitation Sequencing
MeRIP experiments were executed as previously reported (Xiao et al., 2019). In brief, approximately 90 µg of total RNA was fragmented into 100- to 300-nucleotide (nt)-long fragments by zinc acetate, followed by the addition of EDTA to terminate the reaction. Then, 5 µg of fragmented RNA was taken as the input control and the remainder was incubated with m6A antibodies (4 μg, Abcam, ab151230) in IP buffer (150 mM NaCl, 0.05% NP-40, and 10 mM Tris-HCl) containing RNase inhibitor (Promega), and the mixture was subsequently bound to wash Dynabeads protein G (Invitrogen). After stringent wash, the m6A-containing fragments were eluted by competition with 1 mg/ml N6-methyladenosine (Selleck Chemicals). Both the immunoprecipitated RNA fragments and the input RNA were ultimately extracted for library construction using a SMARTer Stranded Total RNA-Seq Kit v2 - Pico Input Mammalian (Takara) following the manufacturer’s protocol. We then performed sequencing using an Illumina Nova platform.
MeRIP-Seq Data Processing and Mapping
Prior to mapping, all raw data were filtered to remove adapters, and low-quality reads using Trimmomatic (Bolger et al., 2014). Reads of all samples that mapped to rRNA FASTA sequences from UCSC gene annotation (mm10) using bowtie2 (Langmead and Salzberg, 2012) were discarded, and the remaining reads were aligned to the mouse reference genome (GRCm38) using HISAT2 (Pertea et al., 2016). Then mapped files were filtered to keep unique and high mapping quality reads for further analysis using Picard and SAMtools (Li et al., 2009).
m6A Peak Calling
m6A peaks were identified using MeTPeak. A custom transcriptome annotation file, assembled by StringTie (Pertea et al., 2016) using all sample reads, was created to include intronic and intergenic m6A peaks. All other parameters were set to the default settings. The annotatePeaks.pl script from the Homer software suite (Heinz et al., 2010) was used for m6A peak annotation.
Evaluation of the Similarity of m6A Between Samples
m6A peaks identified in all samples were merged, and featureCounts (Liao et al., 2014) was used to count the fragments that were mapped to the merged peaks. The normalized fragment counts of each peak in MeRIP-seq (MFPKM) were calculated using (methylated fragment counts mapped to the peak × 109)/(length of the peak × total counts of the mapped fragment), and the normalized fragment counts of each peak in input-seq (IFPKM) were calculated using (input fragment counts mapped to the peak × 109)/(length of the peak × total counts of mapped fragments). The methylation level was then calculated for each peak by dividing the MFPKM by the IFPKM. The Pearson correlation coefficient of log2-scaled m6A levels across all samples was calculated using corrplot to represent the similarity of each sample.
Determination of m6A Motif and Distribution Pattern
m6A peaks were used for motif search using the findMotifsGenome.pl script from the Homer software suite, using “-rna” and “-len 5” parameters. The R package Guitar (Cui et al., 2016) was used to analyze and plot the distribution of m6A on mRNA.
Identification of Differentially Methylated Regions
The regions in which the GF group mean m6A level was 1.5 fold higher than the SPF group mean m6A level were defined as GF group up regions. Also, the regions in which the GF group mean m6A level was 1.5 fold lower than the SPF group mean m6A level were defined as GF group down regions.
Gene Ontology Analysis of Differential m6A-Methylated Genes
Differentially methylated regions were assigned to mouse genes using the annotatePeaks.pl script from the Homer software suite. The gene list was used for pathways and GO term enrichment using the clusterProfiler (Wu T. et al., 2021).
MeRIP-qPCR
The input RNA and the immunoprecipitated RNA fragments from mouse fetal tissues were reverse-transcribed using a GoScript Reverse Transcription System (Promega), and then they were analyzed using real-time qPCR. The ratio of immunoprecipitated RNA to the input of each peak was calculated and normalized to GAPDH. The primers are listed in Supplementary Table S3.
Cell Culture and Cell Line Generation
Mouse embryonic stem cell line E14TG2a (mES cells) was cultured with the N2B27 base medium supplemented with 1 mM glutamine (Invitrogen), 1% nonessential amino acids (Invitrogen), 0.15 mM 1-thioglycerol (Sigma), 100 U/ml of penicillin–streptomycin (Invitrogen), 25 μg/ml of BSA (Sigma), 1 μM MEK inhibitor PD0325901 (Selleck Chemicals), 3 μM GSK3β inhibitor CHIR99021 (Selleck Chemicals), 2% KOSR (Thermo Fisher), and 1000 U/ml of ESGRO leukemia inhibitory factor LIF (Millipore) on plates coated with 0.2% gelatin.
Mettl3–/– mES Cell Line Generation
The Mettl3–/– mES cell line was generated using CRISPR-Cas9 as described previously (Shalem et al., 2014) and the sgRNA sequences are shown in Supplementary Table S4. In brief, sgRNAs were designed on http://crispr-era.stanford.edu/and cloned into the pXPR_001 plasmid. Then, pXPR_001 plasmid was transfected into mES cells using Lipofectamine 3000 (Invitrogen, L3000015). After 12 h, 3 μg/ml of puromycin was added and resistant cells were plated for single colony isolation. Colonies with the desired mutation were identified by Sanger sequencing.
RNA Stability Assay
mES cells cultured in 12-well plates at 70–80% confluency were treated with actinomycin D (5 μg/ml final concentration, MCE, HY-17559) for 0, 2, 4, and 8 h before being collected for the extraction of total RNA. RNA was then reverse-transcribed using GoScript Reverse Transcriptase (Promega), and analyzed using real-time qPCR. Expression levels of RNA were calculated and normalized to GAPDH first, and then to the 0 h time point. The mRNA stability of genes was estimated by the half-life of mRNA and calculated using GraphPad Prism 5.0. The primers are listed in Supplementary Table S3.
Animals
All of the mice were group-housed in a temperature-controlled (22 ± 1 °C) room with a 12:12-h light:dark cycle, and they had free access to food and water. Mettl3flox/+ mice were generated by Cyagen by inserting loxP sites with the same direction on both sides of exons 2 and 3 of the Mettl3 gene. Male Mettl3flox/+ mice were crossed with female Mettl3flox/+ mice to obtain Mettl3flox/flox mice. Next, Mettl3flox/flox mice were first crossed with DDX4-Cre mice to generate Mettl3flox/+; DDX4-Cre mice, and the latter were then crossed with wild-type mice to generate Mettl3−/+ heterozygous mice. The genotype of each mouse was determined using the genomic DNA extracted from tail tissue.
Antibiotic Treatment of Mice
To mimic GF status, conventional mice (CONV) were treated with antibiotics (ABX), based on methods previously described (Vuong et al., 2020). In brief, 10- to 12 -weeks-old female mice were provided with a mixture of four antibiotics (vancomycin 0.5 g/L, neomycin 1 g/L, ampicillin 1 g/L, and amphotericin-B 0.1 g/L) in their water for 1 week. Female mice were then paired with male mice and gestational day 0.5 was determined by observation of a copulatory plug. Pregnant mice (n = 3) were maintained on ABX in their drinking water until embryonic day 18 (E18), and then dissected to obtain fetal tissues (brain and intestine).
Statistical Analysis
We expressed our measurement data as mean ± SEM. T tests were used for comparisons between two groups. Significant differences were represented by asterisks as follows: *p < 0.05, **p < 0.01, and ***p < 0.001, and ns, not significant.
Results
Loss of the Maternal Microbiome Alters the Expression of m6A Writers and Erasers in the Fetal Brain and Intestine
We initially collected fecal pellets from germ-free (GF, n = 3) and specific pathogen-free (SPF, n = 3) pregnant mice, and the absence of intestinal microbiota in the GF mice was confirmed by 16S rRNA gene amplification (Supplementary Figure S1A). We, then, examined the levels of m6A regulators in the mouse fetal brain, intestine, and liver, including writers (METTL3 and METTL14), erasers (FTO and ALKBH5), and readers (YTH-domain family proteins). Using RT-qPCR, we determined that mRNA levels of m6A writers and erasers are highly expressed in the fetal brain and intestine from GF pregnant mice (hereafter designated GFB and GFI, respectively) compared to the corresponding tissues from SPF pregnant mice (hereafter designated SPFB and SPFI, respectively). However, the differences in m6A reader expression levels are much less marked (Figures 1A,B). Nevertheless, the expression of these proteins is similar in the fetal livers of these two types of mice (Supplementary Figure S1B). A similar tendency in the alteration of protein expression is also uncovered using the Western blotting analysis (Figures 1C,D). Taken together, these results indicated that loss of the maternal microbiome alters the expression of m6A writers and erasers in the fetal brain and intestine.
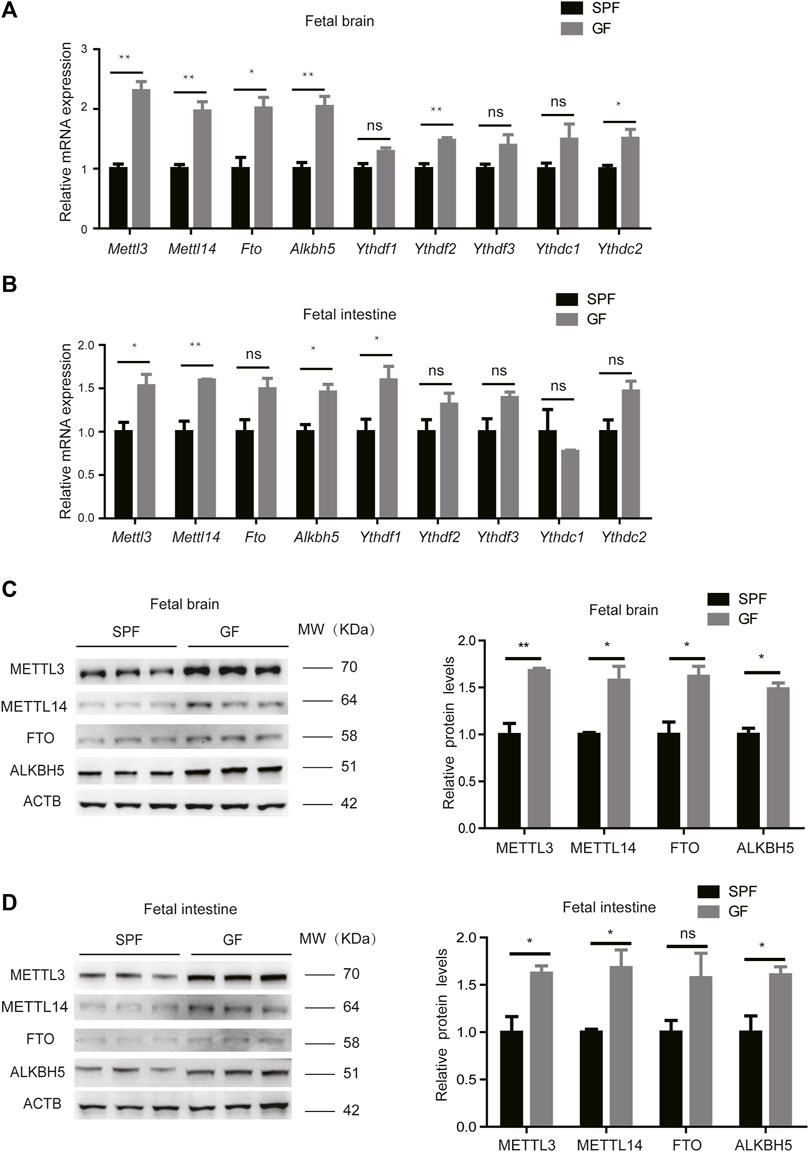
Figure 1. Loss of the maternal microbiome alters the expression of m6A writers and erasers in fetal mice. (A,B) Relative mRNA expression levels of m6A writers, erasers, and readers in fetal brains (A) and intestines (B) of SPF and GF mice. (C,D) Western blots showing the protein expression of m6A writers and erasers in fetal brains (C) and intestines (D) of SPF and GF mice, and relative protein expression levels were calculated based on the band density in Western blotting results.
m6A Modification Profiles in the Fetal Brain and Intestine
To further investigate whether the maternal microbiome participates in modulating the m6A epitranscriptome of offspring, we first detected total m6A levels of mouse fetal tissues. We did not observe an apparent change in the global mRNA m6A levels between SPF and GF mice as revealed by LC-MS/MS (Supplementary Figure S2A). We, thus, characterized m6A methylomes of both mouse fetal brain and intestine (SPFB and GFB and SPFI and GFI—using two independent biological replicates for both) by an m6A-immuno-coprecipitation sequencing (MeRIP-seq) analysis. The samples of the same tissue type were clustered well (Figure 2A) and the classic GGAC motif was observed in the fetal brain and intestine (Figure 2B). In agreement with previous studies (Dominissini et al., 2012; Roundtree et al., 2017), the distribution of m6A signals around mRNA in the two types of fetal tissue samples was mostly presented in the CDS and 3’UTR, and to a lesser extent in the 5’UTR (Figure 2C). We identified the numbers of m6A peaks from these fetal tissues (17,526 in SPFB, 16,885 in GFB, 14,436 in SPFI, and 13,781 in GFI), and we ascertained that approximately three-fourths of the m6A peaks overlapped in both fetal brain and intestine (Figure 2D). Compared with SPFB, GFB showed some changes in patterns of m6A peaks, with a relative elevation in exonic (SPF 26.81% vs. GFB 28%) and intronic regions (SPF 27.86% vs. GFB 29.38%), and a relative diminution in the 3’untranslated region (3’UTR) from 18 to 16.96% (Figure 2E). Compared with SPFI, the GFI also showed some alterations in patterns of m6A peaks with a relative augmentation in exonic regions (SPFI 30.68% vs. GFI 32.5%), and a relative reduction in intronic regions from 30.12 to 29.18% and intergenic regions of 7.7–6.41% (Figure 2F).
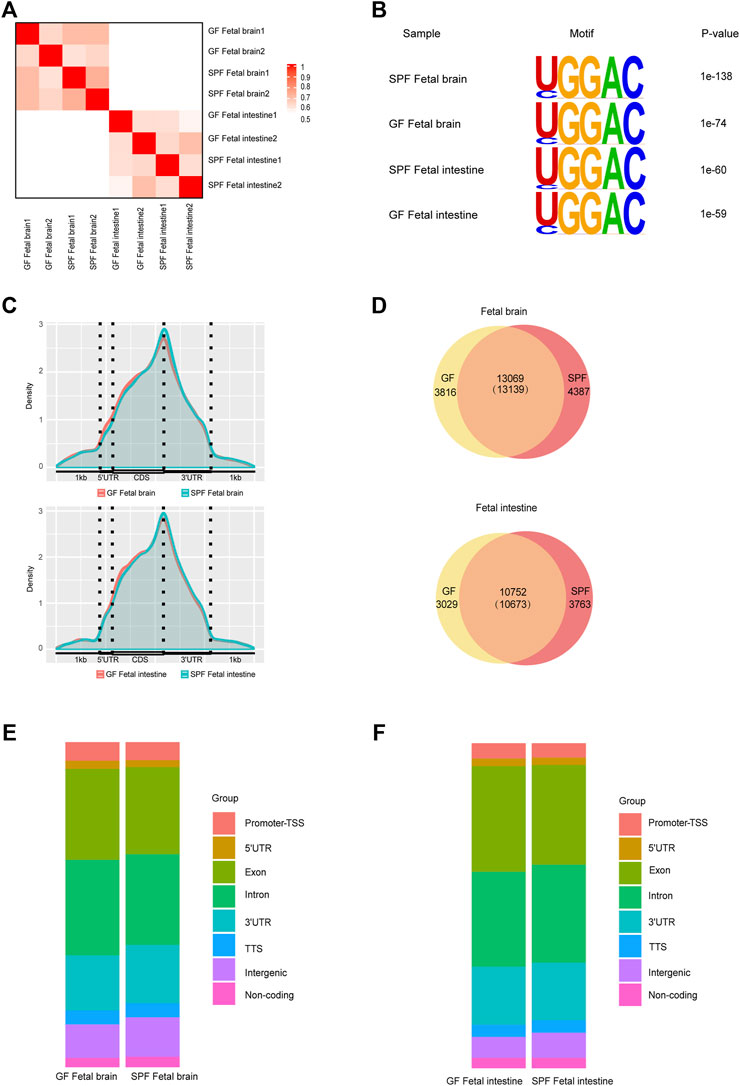
Figure 2. Modification profiles of m6A in the fetal brain and intestine. (A) Similarity (using Pearson’s correlation) of m6A peaks between each pair of samples. (B) Motif analysis of m6A peaks in fetal brains and intestines of SPF and GF mice. (C) Pattern distribution of m6A across the mRNA regions in the fetal brain and intestine. m6A peaks were mapped back to the corresponding genes, and assigned as originating from the 5′-UTR, coding sequence (CDS), or 3′-UTR. (D) Venn diagram showing the overlap of m6A peaks between fetal brains and intestines of SPF and GF mice. (E,F) Bar charts showing the distribution of m6A peaks in the fetal brain (E) and intestine (F).
The Maternal Microbiome Regulates the m6A of Neurodevelopment Genes in the Mouse Fetal Brain
To investigate the dynamic characteristics of m6A methylation, we further analyzed the differential m6A peaks in mouse fetal tissues. As shown in Figure 3A, GFB manifested 2072 upregulated m6A peaks and 583 downregulated m6A peaks (with the criterion of fold-change ≥1.5). In further examination of the genomic distribution in all three mRNA regions of differential m6A peaks, we demonstrated that a majority of the differential m6A peaks were in CDS and 3’UTR (Supplementary Figure S3A). Mapping these reads of differential m6A peaks to the genome, we identified 1147 genes with upregulated m6A peaks and 496 genes with downregulated m6A peaks (Supplementary Figure S3B). To further study the biological significance of dysregulated m6A modifications in the fetal brain, we conducted GO analyses of differentially m6A-methylated genes (Figure 3B and Supplementary Figure S3C). We concentrated on the function of m6A-hypermethylated genes and showed that these genes were significantly enriched in pathways related to neurodevelopment, such as synapse formation and axonogenesis. The read coverage plot of a representative gene Cabp1 associated with neurodevelopment was depicted in Figure 3C, and the m6A levels of genes (Sema4c, Cobl, Cabp1, Insr, Ntng2, Gabrg2, and Plxna3) were increased in GFB as revealed by using the MeRIP-qPCR analysis (Figure 3D). In addition, the transcript levels of these genes were confirmed by using the RT-qPCR analysis (Supplementary Figure S3D). Collectively, these data suggest that the maternal microbiome regulates the m6A of neurodevelopment genes in the mouse fetal brain.
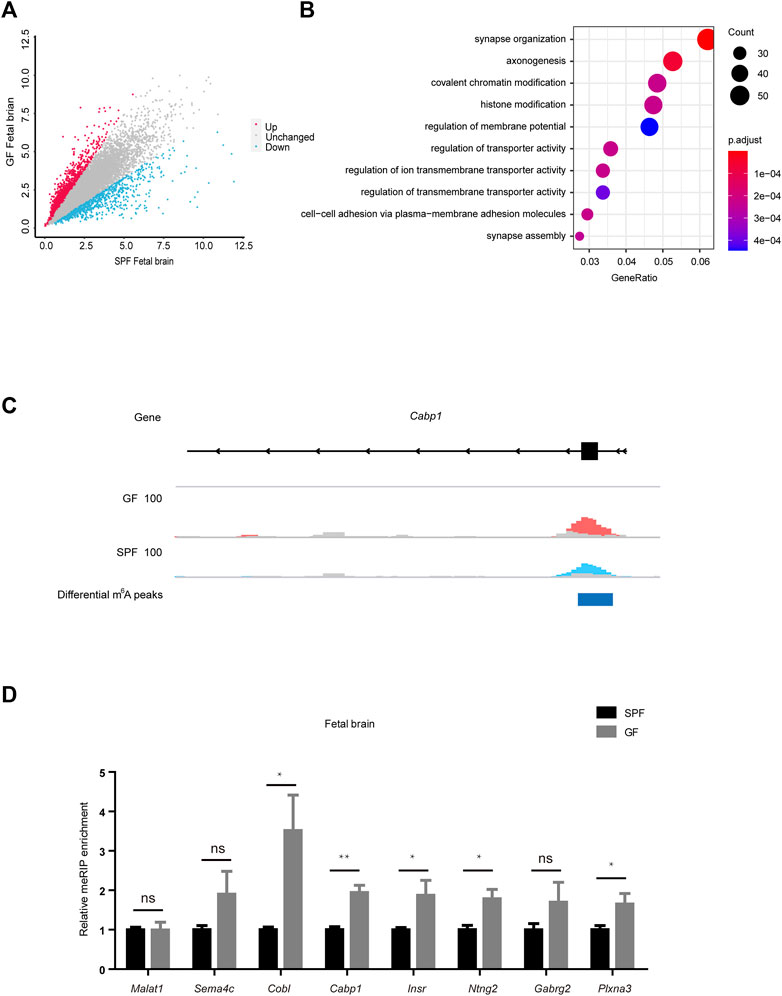
Figure 3. Maternal microbiome regulates the m6A of neurodevelopment genes in the mouse fetal brain. (A) Scatter diagram shows the number of differential m6A peaks in the GF fetal brain. (B) Gene ontology-enrichment analysis of genes containing upregulated m6A peaks in the GF fetal brain. (C) Integrated genome viewer (IGV) shows the distribution of representative differential m6A peaks in Cabp1. GF IP, SPF IP, and input are shown in red, blue, and gray, respectively. (D) Validation of the relative m6A enrichments of Sema4c, Cobl, Cabp1, Insr, Ntng2, Gabrg2, and Plxna3 in SPF and GF fetal brains by m6A-immunoprecipitation (IP)-qPCR.
The Maternal Microbiome Regulates Fetal Intestinal m6A-Modified Genes in the Wnt Signaling Pathway
As shown in Figure 4A, GFI reflected 2068 upregulated m6A peaks and 184 downregulated m6A peaks (with a fold-change ≥1.5). Further examination of the genomic distribution in all three mRNA regions of the differential m6A peaks revealed that most of the differential m6A peaks were in CDS and 3′UTR (Supplementary Figure S4A). When we mapped these reads of differential m6A peaks to the genome, we identified 1590 genes with upregulated m6A peaks and 166 genes with downregulated m6A peaks (Supplementary Figure S4B). To further assess the biological significance of dysregulated m6A modification in the fetal intestine, we executed GO analysis of differentially m6A-methylated genes (Figure 4B and Supplementary Figure S4C). When we concentrated on the functions of m6A-hypermethylated genes, we found that they were significantly enriched in the Wnt signaling pathway. The read coverage plot of a representative gene Wnt4 is shown in Figure 4C. The differential m6A levels of representative genes (Wnt4, Fzd5, Fzd8, Sulf1, Sox13, Axin2, and Abl2) were confirmed by the MeRIP-qPCR analysis (Figure 4D), and their transcript levels were all attenuated in GFI compared to SPFI as revealed by the RT-qPCR analysis (Figure 4E). This indicates that differential m6A modifications in these two types of fetal intestines are correlated with the expression of genes enriched in the Wnt signaling pathways. Next, we knocked out Mettl3 (Mettl3-/-) in the mES cell line using CRISPR/Cas9, and we consistently found that Mettl3 knockout significantly decreased m6A levels of representative genes while increasing mRNA expression levels (Figures 4F,G). We further investigated whether the changes in m6A methylation would affect mRNA levels of representative genes in mESC. We observed that in the presence of actinomycin D (an inhibitor of mRNA transcription), Mettl3 knockout retards the degradation of representative genes mRNAs (Figure 4H). Collectively, these data suggest that the maternal microbiome regulates fetal intestinal m6A-modified genes in the Wnt signaling pathway.
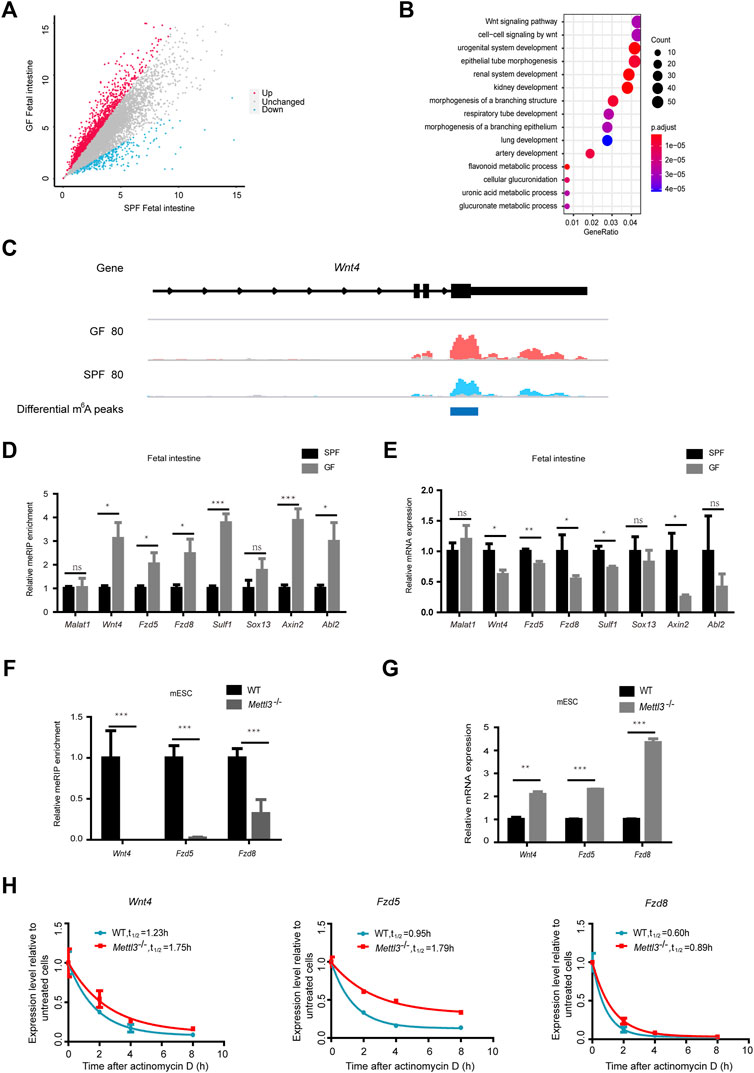
Figure 4. Maternal microbiome regulates fetal intestinal m6A-modified genes in the Wnt signaling pathway. (A) Scatter diagram shows the number of differential m6A peaks in the GF fetal intestine. (B) Gene ontology-enrichment analysis of genes containing upregulated m6A peaks in the GF fetal intestine. (C) IGV shows the distribution of representative differential m6A peaks in Wnt4. GF IP, SPF IP, and input are shown in red, blue, and gray, respectively. (D) Validation of the relative m6A enrichments of Wnt4, Fzd5, Fzd8, Sulf1, Sox13, Axin2, and Abl2 in SPF and GF fetal intestines by m6A-immunoprecipitation (IP)-qPCR. (E) Validation of the relative mRNA expression levels of Wnt4, Fzd5, Fzd8, Sulf1, Sox13, Axin2, and Abl2 in SPF and GF fetal intestines. (F) Validation of the relative m6A enrichments of Wnt4, Fzd5, and Fzd8 in the WT and Mettl3-/- mouse embryonic stem cell line E14TG2a by m6A-immunoprecipitation (IP)-qPCR. (G) Validation of the relative mRNA expression levels of Wnt4, Fzd5, and Fzd8 in the WT and Mettl3-/- mouse embryonic stem cell line E14TG2a. (H) Half-life (t1/2) of Wnt4, Fzd5, and Fzd8 mRNA in the WT and Mettl3-/- mouse embryonic stem cell line E14TG2a after actinomycin D treatment.
Antibiotic Treatment Mostly Recapitulates m6A Change in the Mouse Fetal Intestine and Brain
To confirm the aforementioned results, we treated CONV pregnant mice with a mixture of four antibiotics (vancomycin, neomycin, ampicillin, and amphotericin-B) to mimic germ-free status (ABX mice) and validated that intestinal microbiota were almost exhausted by the 16S rRNA gene qPCR analysis (Supplementary Figure S5A). Similar to our previous experimental results, the mRNA expression levels of Mettl3 and Fto in the ABX fetal brain were slightly higher than those in the CONV fetal brain (Figure 5A), while the mRNA expression levels of both m6A writers and erasers in the ABX fetal intestine were significantly increased compared to the CONV fetal intestine (Figure 5B). In addition, the expression of these proteins remained unchanged in fetal livers from both CONV and ABX (Supplementary Figure S5B). As for the protein expression levels of m6A writers and erasers, we noted a universal tendency for them to increase in the ABX fetal brain and intestine (Figures 5C,D). We then determined the m6A levels and the expression of representative genes regulated by the maternal microbiome in the ABX and CONV fetal brain and intestine, and we found that the m6A levels of these genes in the ABX brain and intestine were also increased relative to CONV (Figures 5E,F), and their transcript levels were confirmed by the RT-qPCR analysis (Figures 5G,H). Collectively, these data show that antibiotic treatment mostly recapitulates m6A alterations in the mouse fetal intestine and brain.
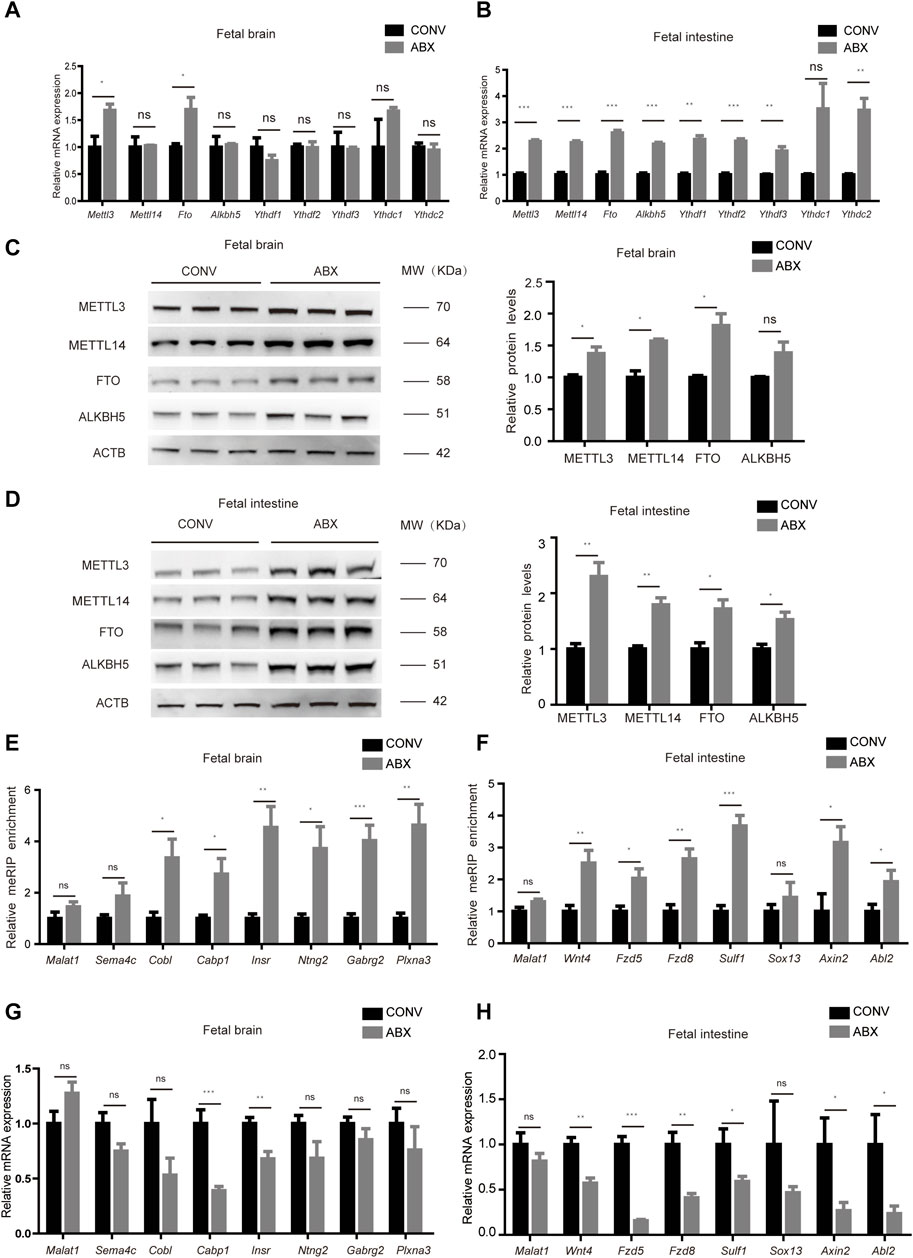
Figure 5. Antibiotic treatment mostly recapitulates m6A alterations in the mouse fetal intestine and brain. (A,B) Relative mRNA expression levels of m6A writers and erasers in CONV and ABX fetal brains (A) and intestines (B). (C,D) Western blot shows the protein expression levels of m6A writers and erasers in fetal brains (C) and intestines (D) of CONV and ABX mice, and relative protein expression levels were calculated based on the band density in Western blotting results. (E,G) Validation of the relative m6A enrichment of Sema4c, Cobl, Cabp1, Insr, Ntng2, Gabrg2, and Plxna3 in CONV and ABX fetal brains (E) and of Wnt4, Fzd5, Fzd8, Sulf1, Sox13, Axin2, and Abl2 in CONV and ABX fetal intestines (G) by m6A-immunoprecipitation (IP)-qPCR. (F,H) Validation of the relative mRNA expression levels of Sema4c, Cobl, Cabp1, Insr, Ntng2, Gabrg2, and Plxna3 in CONV and ABX fetal brains (F) and of Wnt4, Fzd5, Fzd8, Sulf1, Sox13, Axin2, and Abl2 in CONV and ABX fetal intestines (H).
Loss of Heterozygosity of Mettl3 Inhibits the Susceptibility of the Mouse Fetal Intestine to the Maternal Microbiome
To further confirm that the expression of developmental genes was regulated by m6A as programed by the maternal microbiome, we generated Mettl3−/+ heterozygous mice (Supplementary Figures S6A,B). Because the homozygous knockout of Mettl3 was embryonically lethal, we crossed Mettl3 heterozygous knockout male mice (Mettl3−/+) with wild-type (WT) female mice. The latter were provided with water (i.e., the offspring of CONV and Mettl3−/+ mice) or ABX (i.e., the offspring of ABX and ABX + Mettl3−/+mice). As expected, there were no significant differences in m6A levels of representative genes between CONV and Mettl3−/+ fetal intestines, however, m6A levels of representative genes increased in the ABX fetal intestine but not in the ABX + Mettl3−/+ fetal intestine, compared with the CONV fetal intestine (Figure 6A). Correspondingly, mRNA expression levels of representative genes showed no differences between CONV and Mettl3−/+ fetal intestines, while they were significantly reduced in the ABX fetal intestine but not in the ABX + Mettl3−/+ fetal intestine, compared with the CONV fetal intestine (Figure 6B). Our collective results, therefore, indicate that the maternal microbiome affects the developmental gene expression via m6A modifications.
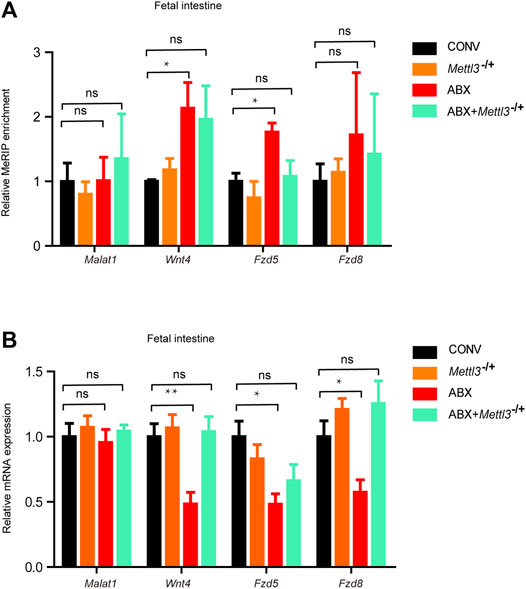
Figure 6. Loss of heterozygosity of Mettl3 inhibits the susceptibility of the mouse fetal intestine to the maternal microbiome. (A) Validation of the relative m6A enrichments of Wnt4, Fzd5, and Fzd8 in the intestines of CONV, Mettl3−/+, ABX, and ABX + Mettl3−/+ fetal mice by m6A-immunoprecipitation (IP)-qPCR. (B) Validation of the relative mRNA expression levels of Wnt4, Fzd5, and Fzd8 in the intestines of CONV, Mettl3−/+, ABX, and ABX + Mettl3−/+ fetal mice.
Discussion
The microbiome and m6A modifications are closely related to human health and disease, and previous studies have shown that host m6A is strongly affected by the mouse microbiome (Wang et al., 2019; Jabs et al., 2020). However, the impact of the maternal microbiome on the m6A epitranscriptome of the mouse fetus has not yet been elucidated. In this study, we profiled the transcriptome-wide m6A methylome of the mouse fetal brain and intestine, demonstrated the alterations in m6A methylation caused by the maternal microbiome, identified differential m6A peaks, and showed that genes with dysregulated m6A peaks were potentially active in fetal development.
In a recent study, Wang et al. (2019)ascertained that both m6A writers and erasers were highly overexpressed in the GF brain compared to the SPF brain regardless of RNA and protein levels. Intriguingly, our results also revealed that the maternal microbiome also altered the expression of m6A writers and erasers in the mouse fetal brain and intestine, and that expression was recapitulated by antibiotic treatment.
Previous studies have depicted depletion of the maternal microbiome as impairing fetal thalamocortical axonogenesis (Vuong et al., 2020). Our results suggested that loss of the maternal microbiome contributed to m6A-hypermethylated genes in GFB, and that these genes were significantly enriched in pathways related to neurodevelopment, including synapse formation and axonogenesis. Moreover, the mRNA expression levels of these m6A-hypermethylated genes were commensurately altered, implying that m6A plays a key role in effects engendered by the maternal microbiome on fetal neurodevelopment. It is worth noting that increasing evidence reveals a functional link between gut bacteria and neurodegenerative diseases such as Alzheimer’s disease and Parkinson’s diseases (Sampson et al., 2016; Bhattarai et al., 2021; Willyard, 2021), and the abnormality in m6A is involved in neurological dysfunction and behavioral defects (Mitropoulos et al., 2017; Chen et al., 2019; Han et al., 2020; Huang et al., 2020). However, although it remains elusive whether the regulation of fetal neurodevelopment by the maternal gestational microbiome increases the risk of neurologic diseases in adulthood, we expect that the elucidation of such a mechanism will provide a foundation for future novel treatments of nerve diseases.
The Wnt signaling pathway is highly conserved from nematodes to mammals (Kohn and Moon, 2005; Clevers and Nusse, 2012) and is involved in many aspects of embryonic development (Clevers, 2006; MacDonald et al., 2009). Current evidence indicates that the Wnt signaling pathway regulates the self-renewal or differentiation of intestinal stem cells (Reya and Clevers, 2005; Böttcher et al., 2021). Our results showed that upregulated m6A genes in GFI were enriched in the Wnt signaling pathway but that mRNA expression levels of these genes were downregulated compared with SPFI, the mechanism of which is that m6A accelerates the degradation of these genes mRNA. These results reveal that the maternal microbiome regulates the gene expression in Wnt signaling by m6A in the fetal intestine, and this may constitute a mechanism whereby loss of the maternal microbiome impairs fetal intestinal development. In addition, maternal exposure to antibiotics promotes gut dysbiosis and increases the risk of inflammatory bowel diseases in offspring (Miyoshi et al., 2017). Although such data suggest that the maternal gestational microbiome exerts a critical effect on the onset and progression of intestinal diseases in offspring, the precise role of m6A in this action requires further clarification.
For further verification, we treated conventional mice (CONV) with antibiotics (ABX) to mimic GF status. Although antibiotic treatment mostly recapitulates m6A change in mouse fetus, some differences in m6A epitranscriptome between GF and ABX mouse still exist. For example, the mRNA expression levels of Mettl14 and Alkbh5 were increased in GFB but not in the ABX mouse fetal brain, which may be caused by some individual differences among different mice due to age, nutritional status, or other factors (Jabs et al., 2020). In addition, ABX treatments are unable to completely eradicate the microbiome so there still exists a small amount (about 5%) of the microbiome in ABX mice compared with GF mice, and the acute or subacute aseptic state simulated by antibiotic treatment is not exactly the same as the chronic rearing under an aseptic environment for a long time (Vuong et al., 2020), which may also be some important reasons why the m6A epitranscriptome of GF mouse has some difference from the ABX mouse.
Collectively, our data reveal programing of the maternal microbiome on m6A modifications in the mouse fetus and should assist in unveiling the underlying mechanisms by which gut dysbiosis precipitates human disease. With progressively maturing analyses and technical developments, we expect that m6A will evolve into a potential therapeutic target of microbiota-directed disease.
Data Availability Statement
The datasets presented in this study can be found in online repositories. The names of the repository and accession number can be found as follows: http://bigd.big.ac.cn/gsa, CRA006146.
Ethics Statement
The animal study was reviewed and approved by the Southern Medical University Experimental Animal Ethics Committee. Guangzhou. China.
Author Contributions
YL, ZhW, and LX designed and supervised the project. ZX, SL, ZL, JC, HW, ZiW, and QR performed experiments. ZL and JC conducted the bioinformatic analysis. YL and ZX wrote the manuscript with input from all authors.
Funding
This work was supported by the National Key R&D Program of China (grant nos. 2019YFA0802303 and 2021YFA0805400), the Guangdong Basic and Applied Basic Research Foundation (grant no. 2022A1515011821), and the National Demonstration Center for Experimental Education of Basic Medical Sciences (Southern Medical University).
Conflict of Interest
The authors declare that the research was conducted in the absence of any commercial or financial relationships that could be construed as a potential conflict of interest.
Publisher’s Note
All claims expressed in this article are solely those of the authors and do not necessarily represent those of their affiliated organizations, or those of the publisher, the editors, and the reviewers. Any product that may be evaluated in this article, or claim that may be made by its manufacturer, is not guaranteed or endorsed by the publisher.
Acknowledgments
We thank S. Xiao for discussion and critical reading of the manuscript.
Supplementary Material
The Supplementary Material for this article can be found online at: https://www.frontiersin.org/articles/10.3389/fcell.2022.882994/full#supplementary-material
References
Alarcón, C. R., Goodarzi, H., Lee, H., Liu, X., Tavazoie, S., and Tavazoie, S. F. (2015). HNRNPA2B1 Is a Mediator of m6A-dependent Nuclear RNA Processing Events. Cell 162 (6), 1299–1308. doi:10.1016/j.cell.2015.08.011
Batista, P. J., Molinie, B., Wang, J., Qu, K., Zhang, J., Li, L., et al. (2014). m6A RNA Modification Controls Cell Fate Transition in Mammalian Embryonic Stem Cells. Cell Stem Cell 15 (6), 707–719. doi:10.1016/j.stem.2014.09.019
Bertero, A., Brown, S., Madrigal, P., Osnato, A., Ortmann, D., Yiangou, L., et al. (2018). The SMAD2/3 Interactome Reveals that TGFβ Controls m6A mRNA Methylation in Pluripotency. Nature 555 (7695), 256–259. doi:10.1038/nature25784
Bhattarai, Y., Si, J., Pu, M., Ross, O. A., McLean, P. J., Till, L., et al. (2021). Role of Gut Microbiota in Regulating Gastrointestinal Dysfunction and Motor Symptoms in a Mouse Model of Parkinson's Disease. Gut Microbes 13 (1), 1866974. doi:10.1080/19490976.2020.1866974
Bolger, A. M., Lohse, M., and Usadel, B. (2014). Trimmomatic: A Flexible Trimmer for Illumina Sequence Data. Bioinformatics 30 (15), 2114–2120. doi:10.1093/bioinformatics/btu170
Böttcher, A., Büttner, M., Tritschler, S., Sterr, M., Aliluev, A., Oppenländer, L., et al. (2021). Non-canonical Wnt/PCP Signalling Regulates Intestinal Stem Cell Lineage Priming towards Enteroendocrine and Paneth Cell Fates. Nat. Cell Biol. 23 (1), 23–31. doi:10.1038/s41556-020-00617-2
Brooks, J. F., Behrendt, C. L., Ruhn, K. A., Lee, S., Raj, P., Takahashi, J. S., et al. (2021). The Microbiota Coordinates Diurnal Rhythms in Innate Immunity with the Circadian Clock. Cell 184 (16), 4154–4167. doi:10.1016/j.cell.2021.07.001
Chen, H., Gao, S., Liu, W., Wong, C.-C., Wu, J., Wu, J., et al. (2021). RNA N6-Methyladenosine Methyltransferase METTL3 Facilitates Colorectal Cancer by Activating the m6A-GLUT1-mTORC1 axis and Is a Therapeutic Target. Gastroenterology 160 (4), 1284–1300. doi:10.1053/j.gastro.2020.11.013
Chen, T., Hao, Y.-J., Zhang, Y., Li, M.-M., Wang, M., Han, W., et al. (2015). m6A RNA Methylation Is Regulated by MicroRNAs and Promotes Reprogramming to Pluripotency. Cell Stem Cell 16 (3), 289–301. doi:10.1016/j.stem.2015.01.016
Chen, X., Yu, C., Guo, M., Zheng, X., Ali, S., Huang, H., et al. (2019). Down-Regulation of m6A mRNA Methylation Is Involved in Dopaminergic Neuronal Death. ACS Chem. Neurosci. 10 (5), 2355–2363. doi:10.1021/acschemneuro.8b00657
Church, C., Moir, L., McMurray, F., Girard, C., Banks, G. T., Teboul, L., et al. (2010). Overexpression of Fto Leads to Increased Food Intake and Results in Obesity. Nat. Genet. 42 (12), 1086–1092. doi:10.1038/ng.713
Clevers, H., and Nusse, R. (2012). Wnt/β-Catenin Signaling and Disease. Cell 149 (6), 1192–1205. doi:10.1016/j.cell.2012.05.012
Clevers, H. (2006). Wnt/β-Catenin Signaling in Development and Disease. Cell 127 (3), 469–480. doi:10.1016/j.cell.2006.10.018
Cui, X., Wei, Z., Zhang, L., Liu, H., Sun, L., Zhang, S.-W., et al. (2016). Guitar: An R/Bioconductor Package for Gene Annotation Guided Transcriptomic Analysis of RNA-Related Genomic Features. BioMed Res. Int. 2016, 1–8. doi:10.1155/2016/8367534
Deng, S., Zhang, H., Zhu, K., Li, X., Ye, Y., Li, R., et al. (2021). M6A2Target: A Comprehensive Database for Targets of m6A Writers, Erasers and Readers. Brief. Bioinform. 22 (3), bbaa055. doi:10.1093/bib/bbaa055
Dominissini, D., Moshitch-Moshkovitz, S., Schwartz, S., Salmon-Divon, M., Ungar, L., Osenberg, S., et al. (2012). Topology of the Human and Mouse m6A RNA Methylomes Revealed by m6A-Seq. Nature 485 (7397), 201–206. doi:10.1038/nature11112
Fischer, J., Koch, L., Emmerling, C., Vierkotten, J., Peters, T., Brüning, J. C., et al. (2009). Inactivation of the Fto Gene Protects from Obesity. Nature 458 (7240), 894–898. doi:10.1038/nature07848
Geula, S., Moshitch-Moshkovitz, S., Dominissini, D., Mansour, A. A., Kol, N., Salmon-Divon, M., et al. (2015). m6A mRNA Methylation Facilitates Resolution of Naïve Pluripotency toward Differentiation. Science 347 (6225), 1002–1006. doi:10.1126/science.1261417
Han, M., Liu, Z., Xu, Y., Liu, X., Wang, D., Li, F., et al. (2020). Abnormality of m6A mRNA Methylation Is Involved in Alzheimer's Disease. Front. Neurosci. 14, 98. doi:10.3389/fnins.2020.00098
Heinz, S., Benner, C., Spann, N., Bertolino, E., Lin, Y. C., Laslo, P., et al. (2010). Simple Combinations of Lineage-Determining Transcription Factors Prime Cis-Regulatory Elements Required for Macrophage and B Cell Identities. Mol. Cell 38 (4), 576–589. doi:10.1016/j.molcel.2010.05.004
Huang, H., Camats-Perna, J., Medeiros, R., Anggono, V., and Widagdo, J. (2020). Altered Expression of the m6A Methyltransferase METTL3 in Alzheimer's Disease. eNeuro 7 (5), 0125. doi:10.1523/ENEURO.0125-20.2020
Huang, H., Weng, H., Sun, W., Qin, X., Shi, H., Wu, H., et al. (2018). Recognition of RNA N6-Methyladenosine by IGF2BP Proteins Enhances mRNA Stability and Translation. Nat. Cell Biol. 20 (3), 285–295. doi:10.1038/s41556-018-0045-z
Huang H, H., Weng, H., Zhou, K., Wu, T., Zhao, B. S., Sun, M., et al. (2019). Histone H3 Trimethylation at Lysine 36 Guides m6A RNA Modification Co-transcriptionally. Nature 567 (7748), 414–419. doi:10.1038/s41586-019-1016-7
Huang Y, Y., Su, R., Sheng, Y., Dong, L., Dong, Z., Xu, H., et al. (2019). Small-Molecule Targeting of Oncogenic FTO Demethylase in Acute Myeloid Leukemia. Cancer Cell 35 (4), 677–691. doi:10.1016/j.ccell.2019.03.006
Jabs, S., Biton, A., Bécavin, C., Nahori, M.-A., Ghozlane, A., Pagliuso, A., et al. (2020). Impact of the Gut Microbiota on the m6A Epitranscriptome of Mouse Cecum and Liver. Nat. Commun. 11 (1), 1344. doi:10.1038/s41467-020-15126-x
Jia, G., Fu, Y., Zhao, X., Dai, Q., Zheng, G., Yang, Y., et al. (2011). N6-Methyladenosine in Nuclear RNA Is a Major Substrate of the Obesity-Associated FTO. Nat. Chem. Biol. 7 (12), 885–887. doi:10.1038/nchembio.687
Ke, S., Pandya-Jones, A., Saito, Y., Fak, J. J., Vågbø, C. B., Geula, S., et al. (2017). m6A mRNA Modifications Are Deposited in Nascent Pre-mRNA and Are Not Required for Splicing but Do Specify Cytoplasmic Turnover. Genes Dev. 31 (10), 990–1006. doi:10.1101/gad.301036.117
Kohn, A. D., and Moon, R. T. (2005). Wnt and Calcium Signaling: B-Catenin-independent Pathways. Cell Calcium 38 (3-4), 439–446. doi:10.1016/j.ceca.2005.06.022
Langmead, B., and Salzberg, S. L. (2012). Fast Gapped-Read Alignment with Bowtie 2. Nat. Methods. 9 (4), 357–359. doi:10.1038/nmeth.1923
Li H. B, H.-B., Tong, J., Zhu, S., Batista, P. J., Duffy, E. E., Zhao, J., et al. (2017). m6A mRNA Methylation Controls T Cell Homeostasis by Targeting the IL-7/STAT5/SOCS Pathways. Nature 548 (7667), 338–342. doi:10.1038/nature23450
Li, H., Handsaker, B., Wysoker, A., Fennell, T., Ruan, J., Homer, N., et al. (2009). The Sequence Alignment/Map Format and SAMtools. Bioinformatics 25 (16), 2078–2079. doi:10.1093/bioinformatics/btp352
Li, Y., Xia, L., Tan, K., Ye, X., Zuo, Z., Li, M., et al. (2020). N6-Methyladenosine Co-transcriptionally Directs the Demethylation of Histone H3K9me2. Nat. Genet. 52 (9), 870–877. doi:10.1038/s41588-020-0677-3
Li, Z., Qian, P., Shao, W., Shi, H., He, X. C., Gogol, M., et al. (2018). Suppression of m6A Reader Ythdf2 Promotes Hematopoietic Stem Cell Expansion. Cell Res. 28 (9), 904–917. doi:10.1038/s41422-018-0072-0
Li Z, Z., Weng, H., Su, R., Weng, X., Zuo, Z., Li, C., et al. (2017). FTO Plays an Oncogenic Role in Acute Myeloid Leukemia as a N 6 -Methyladenosine RNA Demethylase. Cancer Cell 31 (1), 127–141. doi:10.1016/j.ccell.2016.11.017
Liao, Y., Smyth, G. K., and Shi, W. (2014). FeatureCounts: An Efficient General Purpose Program for Assigning Sequence Reads to Genomic Features. Bioinformatics 30 (7), 923–930. doi:10.1093/bioinformatics/btt656
Liu, J. E., Li, K., Cai, J., Zhang, M., Zhang, X., Xiong, X., et al. (2020). Landscape and Regulation of m6A and m6Am Methylome across Human and Mouse Tissues. Mol. Cell 77 (2), 426–440. doi:10.1016/j.molcel.2019.09.032
Liu J, J., Gao, M., He, J., Wu, K., Lin, S., Jin, L., et al. (2021). The RNA m6A Reader YTHDC1 Silences Retrotransposons and Guards ES Cell Identity. Nature 591 (7849), 322–326. doi:10.1038/s41586-021-03313-9
Liu, J., Yue, Y., Han, D., Wang, X., Fu, Y., Zhang, L., et al. (2014). A METTL3-METTL14 Complex Mediates Mammalian Nuclear RNA N6-Adenosine Methylation. Nat. Chem. Biol. 10 (2), 93–95. doi:10.1038/nchembio.1432
Liu X, X., Wang, H., Zhao, X., Luo, Q., Wang, Q., Tan, K., et al. (2021). Arginine Methylation of METTL14 Promotes RNA N6-Methyladenosine Modification and Endoderm Differentiation of Mouse Embryonic Stem Cells. Nat. Commun. 12 (1), 3780. doi:10.1038/s41467-021-24035-6
Ma, J. Z., Yang, F., Zhou, C. C., Liu, F., Yuan, J. H., Wang, F., et al. (2016). METTL14 Suppresses the Metastatic Potential of Hepatocellular Carcinoma by Modulating N 6 -methyladenosine-dependent Primary MicroRNA Processing. Hepatology 65 (2), 529–543. doi:10.1002/hep.28885
MacDonald, B. T., Tamai, K., and He, X. (2009). Wnt/β-Catenin Signaling: Components, Mechanisms, and Diseases. Dev. Cell 17 (1), 9–26. doi:10.1016/j.devcel.2009.06.016
Mathiyalagan, P., Adamiak, M., Mayourian, J., Sassi, Y., Liang, Y., Agarwal, N., et al. (2019). FTO-dependent N 6 -Methyladenosine Regulates Cardiac Function during Remodeling and Repair. Circulation 139 (4), 518–532. doi:10.1161/CIRCULATIONAHA.118.033794
Meyer, K. D., Patil, D. P., Zhou, J., Zinoviev, A., Skabkin, M. A., Elemento, O., et al. (2015). 5′ UTR m6A Promotes Cap-independent Translation. Cell 163 (4), 999–1010. doi:10.1016/j.cell.2015.10.012
Mitropoulos, K., Merkouri Papadima, E., Xiromerisiou, G., Balasopoulou, A., Charalampidou, K., Galani, V., et al. (2017). Genomic Variants in the FTO Gene Are Associated with Sporadic Amyotrophic Lateral Sclerosis in Greek Patients. Hum. Genomics 11 (1), 30. doi:10.1186/s40246-017-0126-2
Miyoshi, J., Bobe, A. M., Miyoshi, S., Huang, Y., Hubert, N., Delmont, T. O., et al. (2017). Peripartum Antibiotics Promote Gut Dysbiosis, Loss of Immune Tolerance, and Inflammatory Bowel Disease in Genetically Prone Offspring. Cell Rep. 20 (2), 491–504. doi:10.1016/j.celrep.2017.06.060
Pertea, M., Kim, D., Pertea, G. M., Leek, J. T., and Salzberg, S. L. (2016). Transcript-level Expression Analysis of RNA-Seq Experiments with HISAT, StringTie and Ballgown. Nat. Protoc. 11 (9), 1650–1667. doi:10.1038/nprot.2016.095
Reya, T., and Clevers, H. (2005). Wnt Signalling in Stem Cells and Cancer. Nature 434 (7035), 843–850. doi:10.1038/nature03319
Roundtree, I. A., Evans, M. E., Pan, T., and He, C. (2017). Dynamic RNA Modifications in Gene Expression Regulation. Cell 169 (7), 1187–1200. doi:10.1016/j.cell.2017.05.045
Sampson, T. R., Debelius, J. W., Thron, T., Janssen, S., Shastri, G. G., Ilhan, Z. E., et al. (2016). Gut Microbiota Regulate Motor Deficits and Neuroinflammation in a Model of Parkinson's Disease. Cell 167 (6), 1469–1480. doi:10.1016/j.cell.2016.11.018
Seki, D., Mayer, M., Hausmann, B., Pjevac, P., Giordano, V., Goeral, K., et al. (2021). Aberrant Gut-Microbiota-Immune-Brain axis Development in Premature Neonates with Brain Damage. Cell Host Microbe 29 (10), 1558–1572. doi:10.1016/j.chom.2021.08.004
Shalem, O., Sanjana, N. E., Hartenian, E., Shi, X., Scott, D. A., Mikkelsen, T. S., et al. (2014). Genome-Scale CRISPR-Cas9 Knockout Screening in Human Cells. Science 343 (6166), 84–87. doi:10.1126/science.1247005
Shi, H., Wang, X., Lu, Z., Zhao, B. S., Ma, H., Hsu, P. J., et al. (2017). YTHDF3 Facilitates Translation and Decay of N(6)-Methyladenosine-Modified RNA. Cell Res. 27 (3), 315–328. doi:10.1038/cr.2017.15
Shu, X., Cao, J., Cheng, M., Xiang, S., Gao, M., Li, T., et al. (2020). A Metabolic Labeling Method Detects m6A Transcriptome-wide at Single Base Resolution. Nat. Chem. Biol. 16 (8), 887–895. doi:10.1038/s41589-020-0526-9
Su, R., Dong, L., Li, C., Nachtergaele, S., Wunderlich, M., Qing, Y., et al. (2018). R-2HG Exhibits Anti-tumor Activity by Targeting FTO/m6A/MYC/CEBPA Signaling. Cell 172 (1-2), 90–105. doi:10.1016/j.cell.2017.11.031
Su, R., Dong, L., Li, Y., Gao, M., Han, L., Wunderlich, M., et al. (2020). Targeting FTO Suppresses Cancer Stem Cell Maintenance and Immune Evasion. Cancer Cell 38 (1), 79–96. doi:10.1016/j.ccell.2020.04.017
Uddin, M. B., Wang, Z., and Yang, C. (2021). The m6A RNA Methylation Regulates Oncogenic Signaling Pathways Driving Cell Malignant Transformation and Carcinogenesis. Mol. Cancer. 20 (1), 61. doi:10.1186/s12943-021-01356-0
Vu, L. P., Pickering, B. F., Cheng, Y., Zaccara, S., Nguyen, D., Minuesa, G., et al. (2017). The N6-Methyladenosine (m6A)-Forming Enzyme METTL3 Controls Myeloid Differentiation of Normal Hematopoietic and Leukemia Cells. Nat. Med. 23 (11), 1369–1376. doi:10.1038/nm.4416
Vuong, H. E., Pronovost, G. N., Williams, D. W., Coley, E. J. L., Siegler, E. L., Qiu, A., et al. (2020). The Maternal Microbiome Modulates Fetal Neurodevelopment in Mice. Nature 586 (7828), 281–286. doi:10.1038/s41586-020-2745-3
Wang, X., Li, Y., Chen, W., Shi, H., Eren, A. M., Morozov, A., et al. (2019). Transcriptome-wide Reprogramming of N6-Methyladenosine Modification by the Mouse Microbiome. Cell Res. 29 (2), 167–170. doi:10.1038/s41422-018-0127-2
Wang, X., Lu, Z., Gomez, A., Hon, G. C., Yue, Y., Han, D., et al. (2014). N6-methyladenosine-dependent Regulation of Messenger RNA Stability. Nature 505 (7481), 117–120. doi:10.1038/nature12730
Wang, X., Zhao, B. S., Roundtree, I. A., Lu, Z., Han, D., Ma, H., et al. (2015). N6-methyladenosine Modulates Messenger RNA Translation Efficiency. Cell 161 (6), 1388–1399. doi:10.1016/j.cell.2015.05.014
Wang, Y., Li, Y., Toth, J. I., Petroski, M. D., Zhang, Z., and Zhao, J. C. (2014). N6-methyladenosine Modification Destabilizes Developmental Regulators in Embryonic Stem Cells. Nat. Cell Biol. 16 (2), 191–198. doi:10.1038/ncb2902
Wang, Y., Li, Y., Yue, M., Wang, J., Kumar, S., Wechsler-Reya, R. J., et al. (2018). N6-methyladenosine RNA Modification Regulates Embryonic Neural Stem Cell Self-Renewal through Histone Modifications. Nat. Neurosci. 21 (2), 195–206. doi:10.1038/s41593-017-0057-1
Willyard, C. (2021). How Gut Microbes Could Drive Brain Disorders. Nature 590 (7844), 22–25. doi:10.1038/d41586-021-00260-3
Wu, J., Wang, K., Wang, X., Pang, Y., and Jiang, C. (2021). The Role of the Gut Microbiome and its Metabolites in Metabolic Diseases. Protein Cell 12 (5), 360–373. doi:10.1007/s13238-020-00814-7
Wu, Q., Liang, X., Wang, K., Lin, J., Wang, X., Wang, P., et al. (2021). Intestinal Hypoxia-Inducible Factor 2α Regulates Lactate Levels to Shape the Gut Microbiome and Alter Thermogenesis. Cell Metab. 33 (10), 1988–2003. doi:10.1016/j.cmet.2021.07.007
Wu, T., Hu, E., Xu, S., Chen, M., Guo, P., Dai, Z., et al. (2021). ClusterProfiler 4.0: A Universal Enrichment Tool for Interpreting Omics Data. Innovation 2 (3), 100141. doi:10.1016/j.xinn.2021.100141
Xiao, S., Cao, S., Huang, Q., Xia, L., Deng, M., Yang, M., et al. (2019). The RNA N6-Methyladenosine Modification Landscape of Human Fetal Tissues. Nat. Cell Biol. 21 (5), 651–661. doi:10.1038/s41556-019-0315-4
Xiao, W., Adhikari, S., Dahal, U., Chen, Y.-S., Hao, Y.-J., Sun, B.-F., et al. (2016). Nuclear m6A Reader YTHDC1 Regulates mRNA Splicing. Mol. Cell 61 (4), 507–519. doi:10.1016/j.molcel.2016.01.012
Yoon, K.-J., Ringeling, F. R., Vissers, C., Jacob, F., Pokrass, M., Jimenez-Cyrus, D., et al. (2017). Temporal Control of Mammalian Cortical Neurogenesis by m6A Methylation. Cell 171 (4), 877–889. doi:10.1016/j.cell.2017.09.003
Zhang, X., Chen, B.-d., Zhao, L.-d., and Li, H. (2020). The Gut Microbiota: Emerging Evidence in Autoimmune Diseases. Trends Mol. Med. 26 (9), 862–873. doi:10.1016/j.molmed.2020.04.001
Zhao, B. S., Roundtree, I. A., and He, C. (2017a). Post-transcriptional Gene Regulation by mRNA Modifications. Nat. Rev. Mol. Cell Biol. 18 (1), 31–42. doi:10.1038/nrm.2016.132
Zhao, B. S., Wang, X., Beadell, A. V., Lu, Z., Shi, H., Kuuspalu, A., et al. (2017b). m6A-dependent Maternal mRNA Clearance Facilitates Zebrafish Maternal-To-Zygotic Transition. Nature 542 (7642), 475–478. doi:10.1038/nature21355
Zheng, G., Dahl, J. A., Niu, Y., Fedorcsak, P., Huang, C.-M., Li, C. J., et al. (2013). ALKBH5 Is a Mammalian RNA Demethylase that Impacts RNA Metabolism and Mouse Fertility. Mol. Cell 49 (1), 18–29. doi:10.1016/j.molcel.2012.10.015
Keywords: maternal microbiome, m6A, fetal development, Wnt signaling pathway, METTL3
Citation: Xiao Z, Liu S, Li Z, Cui J, Wang H, Wang Z, Ren Q, Xia L, Wang Z and Li Y (2022) The Maternal Microbiome Programs the m6A Epitranscriptome of the Mouse Fetal Brain and Intestine. Front. Cell Dev. Biol. 10:882994. doi: 10.3389/fcell.2022.882994
Received: 24 February 2022; Accepted: 06 June 2022;
Published: 07 July 2022.
Edited by:
Huilin Huang, Sun Yat-sen University Cancer Center (SYSUCC), ChinaReviewed by:
Huizhe Wu, China Medical University, ChinaPengze Yan, Dana–Farber Cancer Institute, United States
Yu Hou, Chongqing Medical University, China
Copyright © 2022 Xiao, Liu, Li, Cui, Wang, Wang, Ren, Xia, Wang and Li. This is an open-access article distributed under the terms of the Creative Commons Attribution License (CC BY). The use, distribution or reproduction in other forums is permitted, provided the original author(s) and the copyright owner(s) are credited and that the original publication in this journal is cited, in accordance with accepted academic practice. No use, distribution or reproduction is permitted which does not comply with these terms.
*Correspondence: Laixin Xia, eGlhbHhAc211LmVkdS5jbg==; Zhijian Wang, d3pqbmZ5eUAxNjMuY29t; Yuan Li, Z2F2aW5Ac211LmVkdS5jbg==
†These authors have contributed equally to this work