- 1Department of Biochemistry and Molecular Biology, School of Basic Medical Sciences, Southern Medical University, Guangzhou, China
- 2Guangdong Key Laboratory of Biochip Technology, Southern Medical University, Guangzhou, China
- 3Department of Radiology, Nanfang Hospital, Southern Medical University, Guangzhou, China
- 4Institute of Integrated Bioinformedicine and Translational Science, Hong Kong Baptist University, Hong Kong SAR, China
- 5Guangdong-Hong Kong-Macau Joint Lab on Chinese Medicine and Immune Disease Research, Guangzhou, China
Osteoporosis (OP) is a systemic disease susceptible to fracture due to the decline of bone mineral density and bone mass, the destruction of bone tissue microstructure, and increased bone fragility. At present, the treatments of OP mainly include bisphosphonates, hormone therapy, and RANKL antibody therapy. However, these treatments have observable side effects and cannot fundamentally improve bone metabolism. Currently, the prescription of herbal medicine and their derived proprietary Chinese medicines are playing increasingly important roles in the treatment of OP due to their significant curative effects and few side effects. Among these prescriptions, Gushukang Granules (GSK), Xianling Gubao Capsules (XLGB), and Er-xian Decoction (EXD) are widely employed at the clinic on therapy of OP, which also is in line with the compatibility principle of “different treatments for the same disease” in herbal medicine. However, at present, the functional interpretation of “different treatments for the same disease” in herbal medicine still lacks systematic quantitative research, especially on the detection of key component groups and mechanisms. To solve this problem, we designed a new bioinformatics model based on random walk, optimized programming, and information gain to analyze the components and targets to figure out the Functional Response Motifs (FRMs) of different prescriptions for the therapy of OP. The distribution of high relevance score, the number of reported evidence, and coverage of enriched pathways were performed to verify the precision and reliability of FRMs. At the same time, the information gain and target influence of each component was calculated, and the key component groups in all FRMs of each prescription were screened to speculate the potential action mode of different prescriptions on the same disease. Results show that the relevance score and the number of reported evidence of high reliable genes in FRMs were higher than those of the pathogenic genes of OP. Furthermore, the gene enrichment pathways in FRMs could cover 79.6, 81, and 79.5% of the gene enrichment pathways in the component-target (C-T) network. Functional pathway enrichment analysis showed that GSK, XLGB, and EXD all treat OP through osteoclast differentiation (hsa04380), calcium signaling pathway (hsa04020), MAPK signaling pathway (hsa04010), and PI3K-Akt signaling pathway (hsa04151). Combined with experiments, the key component groups and the mechanism of “different treatments for the same disease” in the three prescriptions and proprietary Chinese medicines were verified. This study provides methodological references for the optimization and mechanism speculation of Chinese medicine prescriptions and proprietary Chinese medicines.
Introduction
Osteoporosis (OP) is the most common bone disease characterized by decreased bone mass and degradation of bone microstructure. The main clinical manifestations are decreased bone density, chronic pain, decreased mobility, and so on (Miller, 2016). The common pathogenic factors include aging, decreased estrogen, nutritional disorders, poor living habits, and long-term use of steroids, anti-cancer drugs, diuretics, and so on. The main manifestations of OP are the decline of bone mineral density and bone quality. Its symptoms are most prone to systemic metabolic diseases such as fracture, low back pain, shortening of body length, bone pain, and even weakening of respiratory function (Zhao and Wang, 2003). It has a significant influence on the quality of life of patients and brings a heavy economic burden to families and society. OP is universal and can affect men and women of all races, especially older women who have passed menopause (Lane et al., 2000).
The current treatment drugs for OP mainly include estrogen (Stefanick, 2005), bisphosphonates (Delmas, 2005), calcitonin (Silverman, 2003), and parathyroid hormone (Neer et al., 2001; Srivastava and Deal, 2002). After treatment, the symptoms of osteoporosis patients will be alleviated, but these treatments cannot radically enhance bone metabolism and maintain the balance between osteoclasts and osteogenesis. In addition, the side effects of drugs also bring multiple risks to patients, including some side effects, even toxicity to the kidney, blood, and liver; gastrointestinal side effects; and immunosuppression (Tella and Gallagher, 2014). After taking estrogen receptor modulator drugs, it can also cause endometrial hyperplasia and uterine bleeding (Papapoulos and Makras, 2008).
Herbal medicine has been diffusely used at the clinic on OP therapy due to its fewer side effects and irritation (Zhang et al., 2016). It is known that Gushukang Granules (GSK), Xianling Gubao Capsules (XLGB), Er-xian Decoction (EXD), Liuwei Dihuang Pills, and Guishen Pill are effective in treating OP. In the treatments of OP, different prescriptions have the same and different targets and pathways, which fully figure out the action mode of “multi-components-multi-targets-multi-pathways” on the clinical therapy of complex diseases. How to systematically quantify the hidden mode of action in herbal prescriptions is the foundation and crux to interpret the principle of “different treatments for the same disease” in herbal medicine.
Among these prescriptions, GSK, XLGB, and EXD are widely used in the clinic. GSK contains seven herbs: Epimedium brevicornum Maxim., Radix Rehmanniae Preparata, Auricularia auricular (L.) Underw., Astragalus mongholicus Bunge, Cucumis sativus L., Davallia mariesii Moore ex Bak., and Salvia miltiorrhiza Bge. XLGB consists of six herbs: Epimedium brevicornum Maxim., Rehmannia glutinosa (Gaetn.) Libosch. ex Fisch. et Mey., Salvia miltiorrhiza Bge., Dipsacales, Anemarrhena asphodeloides Bunge, and Psoralea corylifolia Linn. EXD has six kinds of herbs: Curculigo orchioides Gaertn., Epimedium brevicornum Maxim., Angelica sinensis (Oliv.) Diels, Morinda officinalis How., Phellodendron chinense Schneid., and Anemarrhena asphodeloides Bunge. Clinical studies have shown that GSK can increase sex hormones (estrogen and androgen), inhibit bone absorption, effectively improve bone mineral density (BMD), reduce bone loss, increase osteocalcin (OC) and blood alkaline phosphatase (ALP) levels, and enhance osteoblast activity, which can effectively prevent and treat OP (Li et al., 2001; Wang et al., 2007; Wang et al., 2018a). Pharmacological research has shown that XLGB can improve bone metabolism, promote osteogenic effects, inhibit osteoclasts, increase bone density, and facilitate bone formation (Zhu and Hou, 2020). Clinical studies have also shown that XLGB has a therapeutic effect on BMD in patients with OP, which can effectively increase BMD, improve bone metabolism, and control bone loss (Wu et al., 2017). Pharmacological studies have shown that EXD not only increases the proliferation of osteoblasts and alkaline phosphatase (ALP) activity but also reduces the tartrate resistant acid phosphatase (TRAP) activity of osteoclasts (Li et al., 2017). Additionally, EXD affects the calcium signaling pathway and mediates apoptosis by activating the expression of downstream CAMK and activates downstream JNK, AKT, and ERK through upstream TNF-α, affecting the apoptosis process of bone-related cells (Yang et al., 2021). However, the pharmacodynamic material foundation and related molecular mechanism of different prescriptions in the therapy of OP under the concept of “different treatments for same disease” are still indistinct. Therefore, it is necessary to scientifically decipher the material basis and molecular mechanism of the efficacy of different prescriptions on the same disease.
Herbal informatics is an interdisciplinary subject that integrates Chinese medicine, computer science, biology, mathematics, multi-directional pharmacology, and other disciplines. It researches complex herbal medicine systems by systematically observing the response and effect of drugs on pathogenetic gene networks (Wang et al., 2021). Herbal informatics and network pharmacology have been diffusely used in the “same disease and different treatments” of herbal medicine. For example, Wang Kexin et al. clarified the molecular mechanism of DSD, GFD, and HGWD in treating rheumatoid arthritis based on herbal informatics (Wang et al., 2020a). Gao Yao et al. used the method of herbal informatics to analyze the mechanism of Xiaoyao Powder and Kaixin powder in the treatment of depression (Gao et al., 2018). Zhao Can et al. discussed the possible mechanism of Fuling Xingren Gancao Decoction and Ju-Zhijiang Decoction in treating coronary atherosclerotic heart disease based on the herbal informatics method (Zhao et al., 2019). With the continuous in-depth intersection of life sciences, chemistry, computer and information sciences, and other disciplines with drug research and the optimization and upgrading of network visualization tools and network construction analysis technologies, the research ideas and technical methods of herbal informatics will be better used. The study of the mechanism of the “different treatments for the same disease” of Chinese medicine provides more reference for the study of modernization of Chinese medicine (Li and Zhang, 2013).
In this study, a computational model based on herbal informatics was designed to discover the Significant Different Functional Modes (SDFMs) of different prescriptions in the therapy of OP. In order to further decode the key components group of different prescriptions on OP, a genetic algorithm-based optimization model was designed to figure out the FRMs from SDFMs. The distribution of high relevance score, the number of reported evidence, and the coverage of the enrichment pathway of target genes in FRMs were used to evaluate the accuracy and reliability of the FRMs detection model. Then, the effective proteins in the FRMs of each prescription were employed to screen the key components group of each prescription. The importance scores of the components in the key components group of each prescription were obtained based on information gain and target influence, and the effects of these key components were verified by cell experiments. Finally, the potential action mode of different prescriptions in treating the same disease was speculated based on the key components group of each prescription.
In conclusion, according to the herbal informatics strategy proposed in this study, the main mechanisms and relevant pharmacological effects of different treatments of OP can be detected through FRMs, which provide a new network-based method for herbal medicine in the clinic therapy of complex diseases.
Materials and Methods
Flowchart
The schematic diagram of the whole process is shown in Figure 1, and the detailed procedure is described as follows: 1) chemical compounds of GSK, XLGB, and EXD were extracted from the published databases, and a widely used ADME screening model was used to screen potential active components in these compounds. Then, the online webserver is used to predict the targets of these active components. 2) The discovery model of the functional response motif was designed to optimize the C-T network and obtain Significant SDFMs. SDFMs were optimized by a genetic-based optimization model to obtain FRMs. 3) The reliability and accuracy of the FRMs detection model were validated by the distribution of the relevance score, the number of reported evidence, and coverage of functional pathways. 4) The information gain and target influence were combined to score the components in the key components group of each prescription. 5) The effectiveness of high-scored and randomly selected components in the key components group were performed in the in vitro experiments to confirm the precision of our proposed key components group selection model. 6) Finally, the potential action mode of different prescriptions treating the same disease mechanism was inferred by function analysis of the key components group in each prescription.
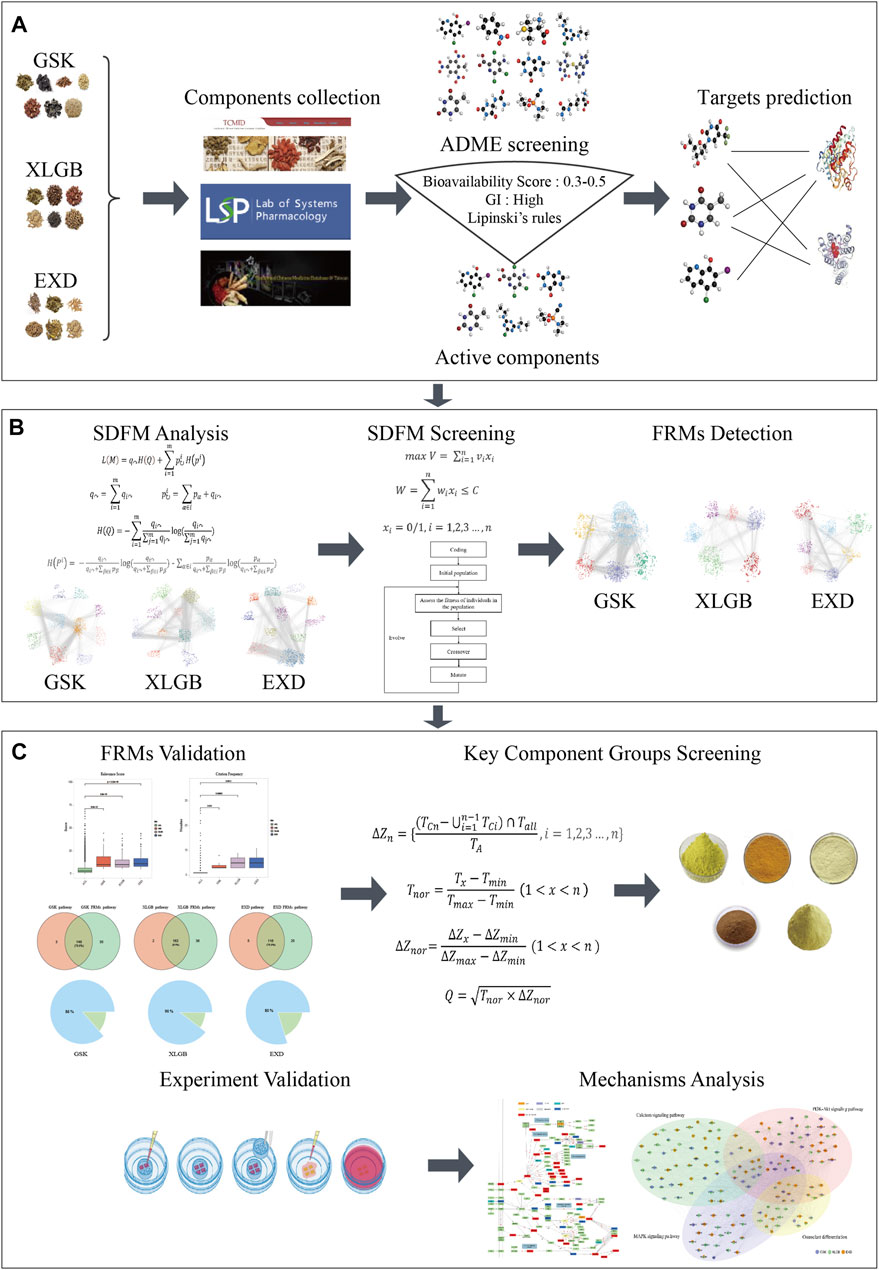
FIGURE 1. The flowchart of our network pharmacology approach, including component collection and target prediction (A). Using the function motif discovery model to find Significant Different Functional Modes (SDFMs) in the three prescriptions and then using the genetic-based optimization model to figure out the Functional Response Motifs (FRMs) (B). Validation of FRMs, screening key component groups, and experiment validation and potential mechanism analysis (C).
Component Collection
The original components in the three prescriptions were extracted by searching the Traditional Chinese Medicine Integrated Database (TCMID 2.0), TCM Database@Taiwan, and Traditional Chinese Medicine Systems Pharmacology Database and Analysis Platform (TCMSP) with the herbs in GSK, XLGB, and EXD as keywords. Open Babel (version 2.4.1) was used to convert the structures of all chemical components into canonical SMILES. The Similarity Ensemble Approach (SEA), Hit Identification and Target Prediction (HitPick), and SwissTargetPrediction were used to predict the drug targets of GSK, XLGB, and EXD.
SwissADME Screening
Lipinski’s Rule of Five is the five elementary principles for selecting drug-like molecules, put forward by the pharmaceutical chemist Christopher Lipinski in 1997. Compounds that conform to the Lipinski Rule of Five will have better pharmacokinetic features and higher bioavailability during metabolism in vivo, so they tend more to become oral drugs. In drug research and development, the Lipinski Rule of Five is used in the preliminary screening of the compound library to exclude those molecules that are unfit for drugs, narrow the range of selecting, and economize the cost of drug research and development. The detailed description of Lipinski’s Rule of Five is that the molecular weight of the compound is less than 500 Da. The number of hydrogen bond donors (including hydroxyl groups and amino groups) in the structure of the compound does not exceed 5. The number of hydrogen bond receptors in the compound does not exceed 10. The logarithmic value (logP) of the lipid-water partition coefficient of the compound is between −2 and 5. The quantity of rotatable bonds in the compound does not exceed 10 (Lipinski et al., 2001). The bioavailability score indicates the probability that a compound has at least 10% oral bioavailability or a measurable Caco-2 permeability in rats. Gastrointestinal absorption (GI absorption) indicates that the drug has good oral bioavailability (Daina et al., 2017). Our research screened the active components of GSK, XLGB, and EXD according to Lipinski’s Rule of Five, bioavailability, and gastrointestinal absorption. Among them, the bioavailability is defined between 0.3 and 0.55.
Network Construction
Cytoscape (version 3.7.2) was employed to build a C-T network, and its plug-in NetworkAnalyzer was used to analyze network topology parameters.
Functional Enrichment Analysis
Function enrichment is conducted based on KEGG (Kyoto Encyclopedia of Genes and Genomes). The hypergeometric distribution model is employed to calculate the significance of the biological pathway containing the target gene. The Benjamini–Hochberg method was used to correct the p-value. All statistical analysis was performed using the R language (version 4.0.5).
Explore the Significant Different Functional Modes
In order to find SDFMs in the three prescriptions for treating OP, we designed the below function motif discovery model.
The function motif discovery model is based on the combination of random walk and information compression. It uses the double-layer Huffman coding method to associate community discovery with information coding, records the paths generated by a random walk in the graph, and finds the community division with the shortest length. The average coding length of each step described in the random walk can be measured by the following prescription:
where
Detection of Functional Response Motifs
In order to further screen the SDFM, we designed a novel genetic-based optimization model, which is described in detail as follows:
The genetic algorithm (GA) is a random walk method that simulates the evolution of the genetic mechanism of the evolutionary laws of nature. It takes all individuals in a population as the object and efficiently searches a coded parameter space through genetic operations of selection, crossover, and mutation. As a new global optimization search algorithm, the genetic algorithm has the obvious characteristics of simplicity and generality, strong robustness, high efficiency, and practicality. It can be applied in all kinds of fields, has achieved good results, and has become one of the critical, intelligent algorithms by degrees. The calculation process is as follows:
1) Random generation of the initial population: the chromosome coding method is represented by a binary column code of length
2) Individual evaluation: under the premise of not exceeding
3) Individual selection: the roulette model is used to convert individual fitness into the area of the roulette wheel in proportion and rotate the roulette wheel. Finally, the individual corresponding to the landing position is selected.
4) Two points cross: two points are randomly set in the individual code, and some genes are exchanged in the middle of the two intersection points.
5) Basic mutation, a number is randomly generated for each chromosome, indicating whether the chromosome needs to be mutated. If a mutation is needed, a random variable is generated, indicating which bit of the chromosome to modify.
6) A new population is formed, and the iteration continues until the termination condition is met:
Pathway Network Integrating
Cytoscape (version 3.7.2) was used to combine osteoclast differentiation, calcium signaling pathway, MAPK signaling pathway, and PI3K-Akt signaling pathway into an integrated pathway.
Key Component Groups Screening
In order to screen the key component groups in different prescriptions, we designed a novel component importance calculation method that combined the information gain and target influence. We sort all the components in descending order of the corresponding target number and calculate the information gain
Experiment Validation
Cell Culture and Drug Treatment
Mouse preosteoblastic MC3T3-E1 cells were purchased from the American Type Culture Collection (ATCC) and stored in Minimal Essential Medium, Alpha (α-MEM), supplemented with 10% fetal bovine serum (FBS), 100 units/mL of penicillin G, and 100 μg/ml of streptomycin at 37°C under 5% CO2. Cells were seeded into 96-well plates (1×103 cells per/well) for 24 h and then treated with 5 μM quercetin, isoliquiritigenin, rutaecarpine, isofraxidin, and secoisolariciresinol for 24 and 48 h. Quercetin, isoliquiritigenin, rutaecarpine, isofraxidin, and secoisolariciresinol (≥98% purity by HPLC) were dissolved in DMSO.
Cell Viability Assay
Cell Count Kit-8 (CCK-8) assay was utilized to measure cell viability. After cell culture, add 10 μl CCK8 to the culture medium and incubate at 37°C for 2 h. The absorbance was measured at 450 nm with a microplate reader (TECAN, infiniteM200).
Results
Collection of Chemical Components and Determination of High-Concentration Components
We collected the herbal components and concentrations of GSK, XLGB, and EXD from the reported literature. The detailed information is shown in Table 1 and Supplementary Table S1. The results show that the chemical composition and concentration of the herbal medicine provide experimental auxiliary evidence for searching for active components and provide a valuable reference for further analysis.
Screening of Active Components for GSK, XLGB, and EXD
Seven herbs of GSK with 672 components, six herbs of XLGB with 540 components, and six herbs of EXD with 752 components were extracted from the Traditional Chinese Medicine Integrated Database (TCMID 2.0), TCM@TAIWAN, and Traditional Chinese Medicine Systems Pharmacology Database and Analysis Platform (TCMSP). Generally speaking, each Chinese medicine compound contains multiple herbal medicines, and each herbal medicine contains a series of chemical components. The pharmacological properties of these components are closely related to their therapeutic effects. Ingredients with better pharmacological properties may have positive therapeutic effects. Therefore, before analyzing the pharmacological effects, we first screen for pharmacological properties. SwissADME was used to screen the components in accordance with Lipinski’s Rule of Five, gastrointestinal absorption, and bioavailability. After SwissADME screening, 271, 242, and 344 active components were obtained in GSK, XLGB, and EXD, respectively (Table 2, Supplementary Table S2). Our further analysis revealed 88 common components in the three prescriptions, 65, 37, and 232 specific components in GSK, XLGB, and EXD, respectively. In addition, we found that each of the herbs from GSK, XLGB, and EXD had its own chemical composition. There were many common components among the three prescriptions, while there were few common components in the prescriptions. Each prescription depended on its unique components (Figure 2). These results indicated that three prescriptions may play a role in treating OP by influencing both the common and specific components.
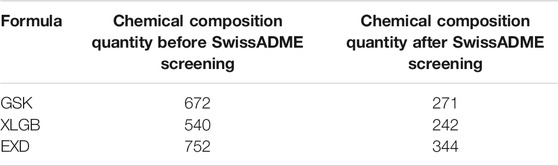
TABLE 2. The number of active components before and after SwissADME screening in GSK, XLGB, and EXD.
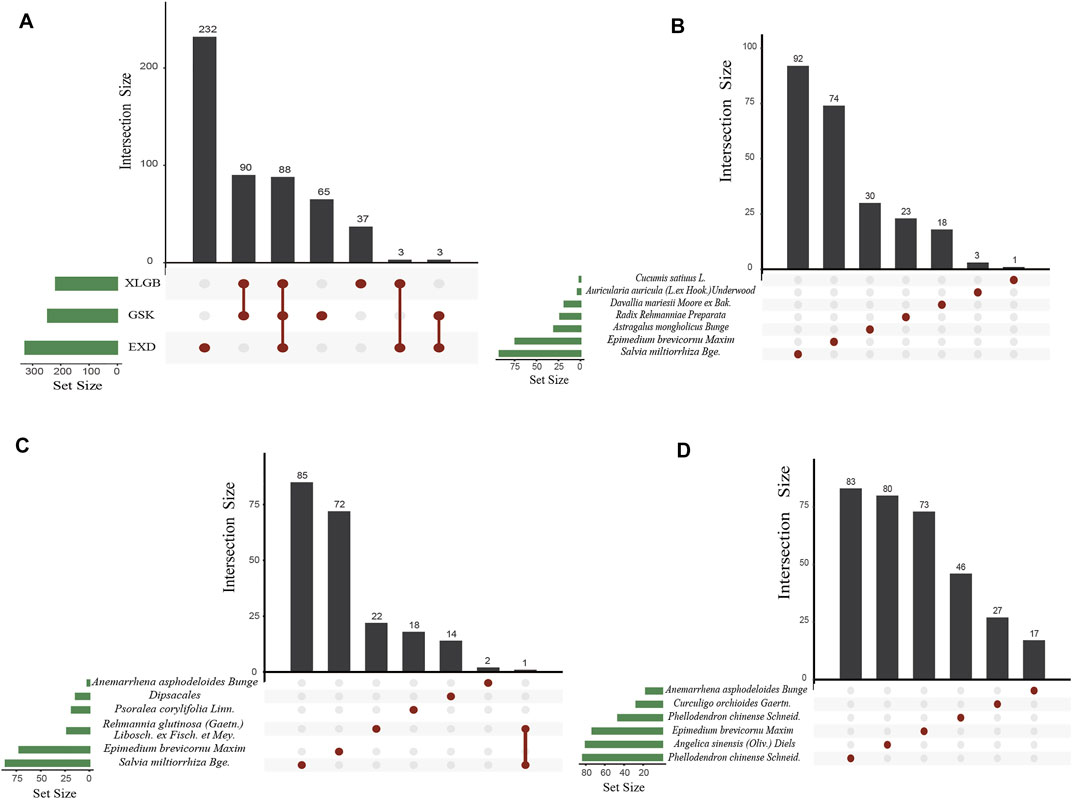
FIGURE 2. Distribution map of active components in GSK, XLGB, and EXD (A). Common components of GSK, XLGB, and EXD (B–D). Distribution map of herbs in GSK (B). XLGB (C). and EXD (D).
Target Prediction and C-T Network Construction
Cytoscape was used to construct a C-T network to analyze the relationships between the active components and targets of the three prescriptions (Supplementary Figure S1). The results showed 271, 240, and 339 active components; 1,264, 1,157, and 1,445 targets; and 9,701, 7,001, and 10,333 interactions in the GSK network, XLGB network, and EXD network, respectively. Then, we use the Cytoscape plug-in tool NetworkAnalyzer to further analyze the topological parameters of the three prescription C-T networks. After analyzing the network topology in the C-T network of GSK, the average degree of the components was 35.80. Among them, phenylalanine had the highest node degree, acting on 207 drug targets (degree: 207), and tyrosine acted on 128 targets in descending order (degree: 128). Quercetin acted on 120 targets (degree: 120). Studies have shown that high phenylalanine levels can affect bones, cause bone-related diseases, and affect bone mineral density (BMD) in a lower way (Mendes et al., 2012). Phosphorylation of tyrosine residues is key to the regulation of osteoclast production and bone resorption activity (Shalev and Elson, 2019). Phenylalanine can be converted to tyrosine, and the metabolic state of phenylalanine is related to normal body growth and maintenance of normal physiological functions (Westbroek et al., 2001). In addition, phenylalanine interacts with the calcium-sensing receptor (CaSR), affecting the body’s calcium metabolism and bone balance (Conigrave et al., 2008). Animal experiments have proved that OP can be prevented by intervening phenylalanine metabolism in rats (Liu et al., 2012). Quercetin can restrain the expression of ERK1/2, MAPK mRNA, and protein, thereby inhibiting the conduction of the ERK1/2-MAPK signaling pathway, promoting the expression of specific genes in bone and osteoblast generation, increasing bone mineral density, and preventing OP (Casado-Díaz et al., 2016). In the C-T network of GSK, the average degree of the target was 7.67. Among them, the highest degree of the node was Microtubule Associated Protein Tau (MAPT), which targeted 172 compounds (degree: 172), followed by Tyrosyl-DNA phosphodiesterase 1 (TDP1), which targeted 135 compounds (degree: 135). Muscleblind Like Splicing Regulator 1 (MBNL1) targeted 83 compounds (degree: 83).
In the C-T network of XLGB, the average degree of the components was 30.48. Among them, phenylalanine had the highest node degree, acting on 202 drug targets (degree: 202), and tyrosine acted on 124 targets in descending order (degree: 124). Quercetin acted on 106 targets (degree: 106). The average degree of the target was 6.05. Among them, the highest degree of the node was Microtubule Associated Protein Tau (MAPT), which targeted 105 compounds (degree: 105), followed by Tyrosyl-DNA phosphodiesterase 1 (TDP1), which targeted 74 compounds (degree: 74). Carbonic anhydrase 7 (CA7) targeted 63 compounds (degree: 63).
In the C-T network of EXD, the average degree of the components was 30.58. Among them, asperglaucide had the highest node degree, acting on 267 drug targets (degree: 267), and phenylalanine acted on 197 targets in descending order (degree: 197). n-cis-Feruloyltyramine acted on 177 targets (degree: 177). The average degree of the target was 7.16. Among them, the highest degree of the node was cytochrome P450 family 1 subfamily B member 1 (CYP1B1), which targeted 94 compounds (degree: 94), followed by carbonic anhydrase 3 (CA3), which targeted 90 compounds (degree: 90). Carbonic anhydrase 7 (CA7) targeted 86 compounds (degree: 86). Through the comparative analysis of the number of targeted genes, it can be seen that GSK had a stronger control rate on the C-T network.
The results showed that, in the three prescriptions, there were a relationship between one component corresponding to multiple targets and a phenomenon of different components acting on the same target, consistent with the function of “multi-components-multi-targets-multi-pathways” in herbal medicine, reflecting the complicacy of the underlying mechanism of herbal medicine.
Screening Significant Different Functional Modes and Functional Response Motifs
Due to the large and complex C-T network of the three prescriptions, it is difficult to quickly extract the most important active ingredient information. Therefore, we designed a novel function motif discovery model to screen the C-T network to filter the modes that can represent the complete C-T network. The results showed that we predicted 22, 23, and 30 SDFM in GSK, XLGB, and EXD, respectively (p < 0.05). In order to figure out the FRMs and remove the noise in the SDFM in each prescription. We designed another genetic-based optimization model to optimize the SDFM and finally obtained 11, 13, and 15 FRMs in GSK, XLGB, and EXD, respectively (Figures 3–5).
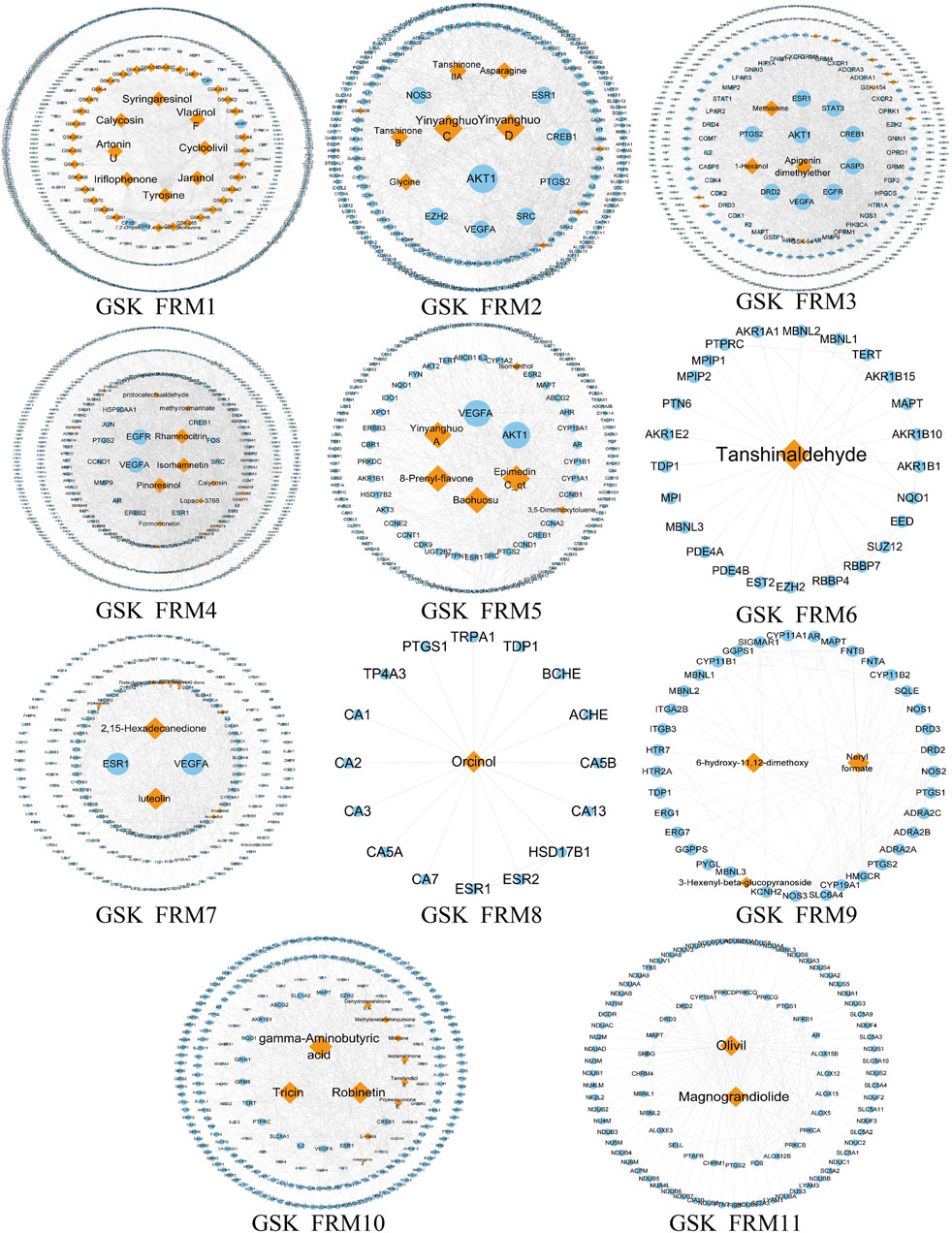
FIGURE 3. The predicated FRMs of the C-T network in GSK. The orange nodes represent the components in GSK, and the blue nodes represent the related targets.
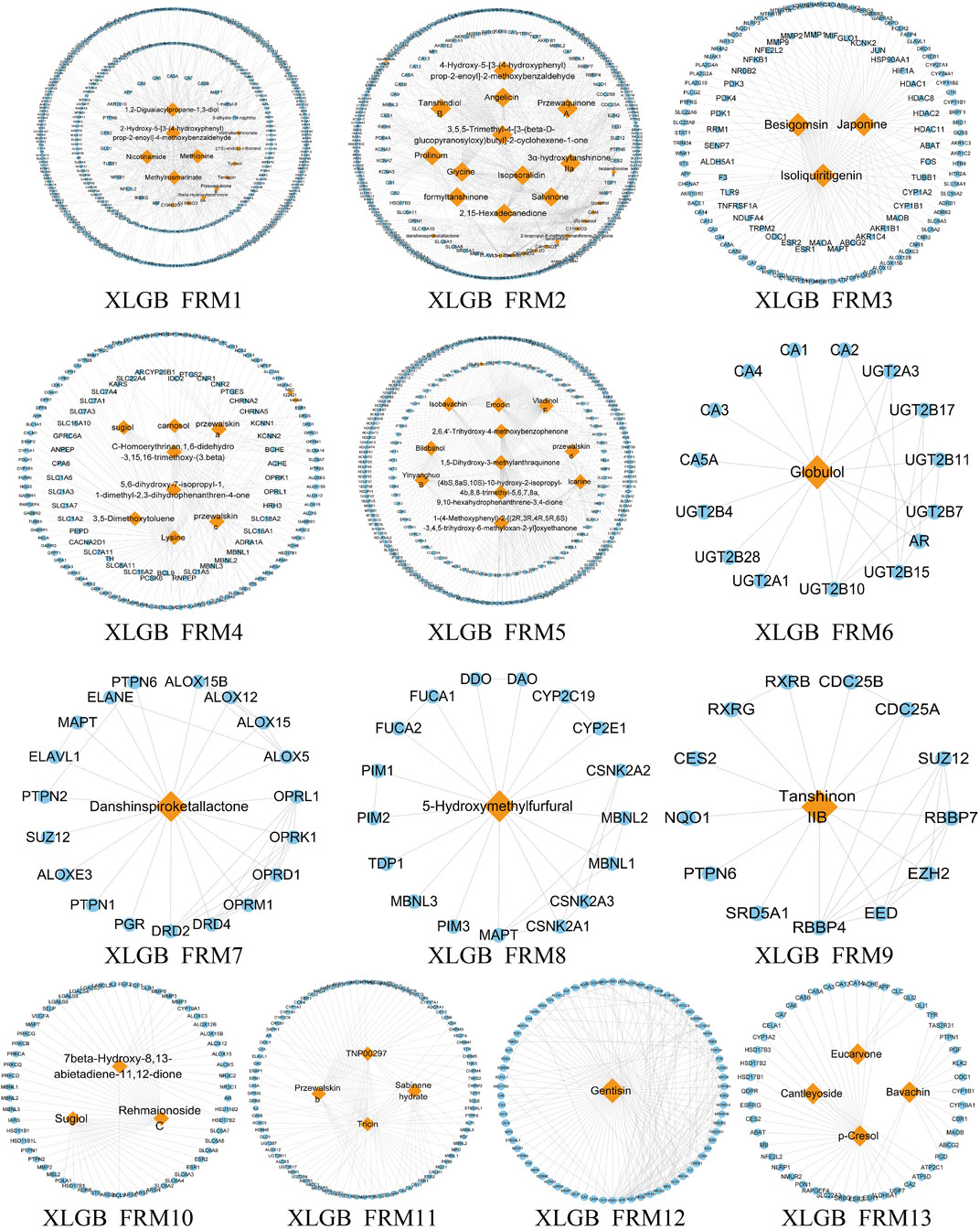
FIGURE 4. The predicated FRMs of the C-T network in XLGB. The orange nodes represent the components in XLGB, and the blue nodes represent the related targets.
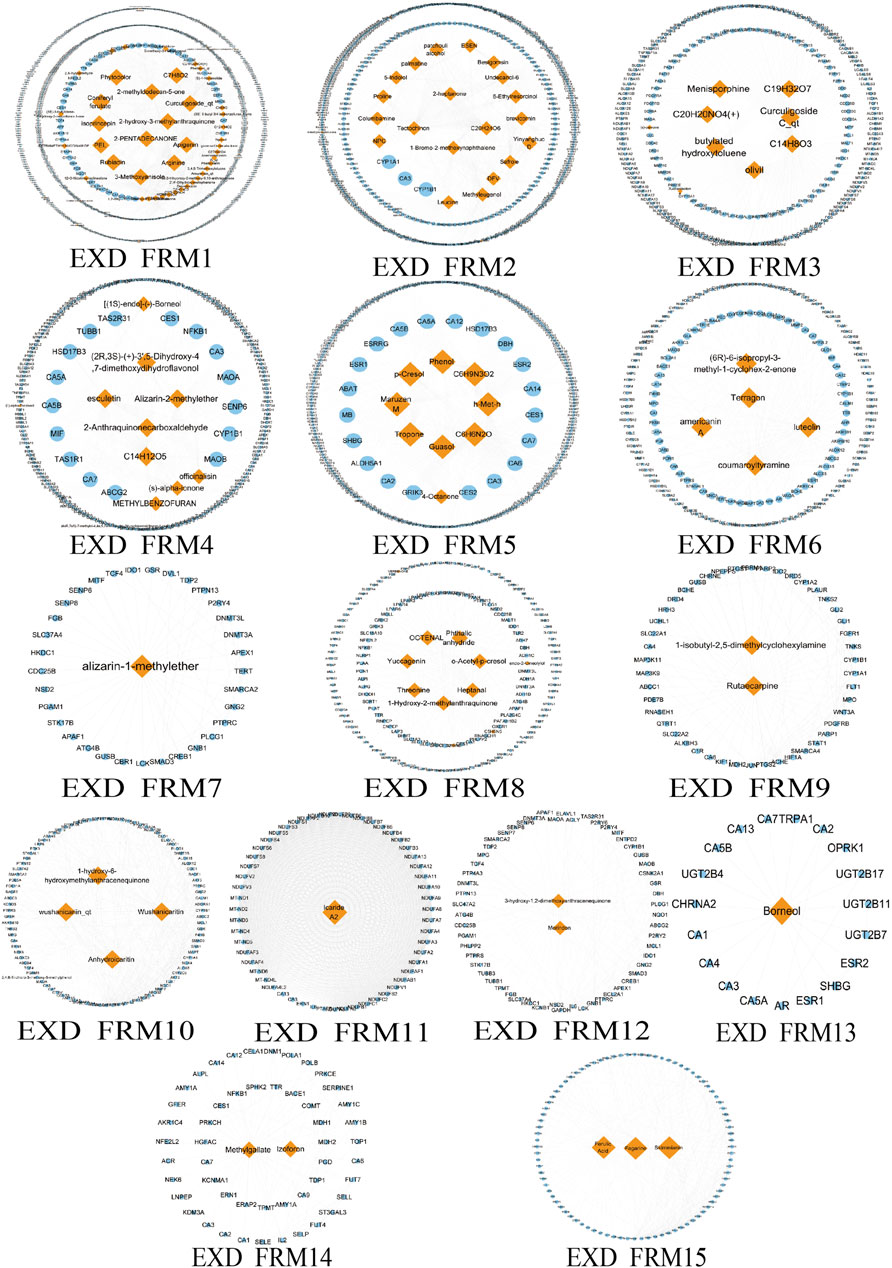
FIGURE 5. The predicated FRMs of the C-T network in EXD. The orange nodes represent the components in EXD, and the blue nodes represent the related targets.
Validating FRMs
In order to verify whether the entire C-T network can be replaced by the predicted FRMs, we propose two criteria to evaluate the accuracy and dependability of FRMs. The first criterion is whether the relevance score and the number of reported evidence of FRMs-related high reliable genes, defined in the following section, are higher than those of the pathogenic genes of OP. High relevance score and the high number of reported evidence indicate that the genes in FRMs can cover the high reliable pathogenic genes to the maximum extent. The second criterion is whether the gene enrichment pathways in FRMs can cover the gene enrichment pathways in the C-T network as much as possible. The high pathway coverage indicates that FRMs can cover most of the gene enrichment pathways in the C-T network and have the most likely function of the prescription. Details of the results are as follows:
Validating FRMs Based Relevance Score and the Number of Reported Evidence
We extracted the pathogenic genes of OP with relevance score and the number of reported evidence from GeneCards database and DisGeNet database.
Herein, we set a criterion for screening two high reliable pathogenic gene sets by calculating the average relevance score and the number of reported evidence of pathogenic genes, respectively. The first high reliable pathogenic gene set was defined as the genes with higher relevance score than the average of all pathogenic genes. The second high reliable pathogenic gene set was defined as the genes with higher number of reported evidence than the average of all pathogenic genes. According to this criterion, the average relevance score and the number of reported evidence of pathogenic genes of OP collected from the database are 5.13 and 2.7, respectively. Thus, in the first high reliable pathogenic gene set, the relevance score of each gene was higher than 5.13. While in the second high reliable pathogenic gene set, the number of reported evidence of each gene was higher than 2.7. The average relevance scores of FRMs in GSK, XLGB, and EXD were 14.22, 12.98, and 14.43, respectively, while the average numbers of reported evidence were 10.5, 7.75, and 8.17, respectively, which were significantly higher than the two high reliable pathogenic gene sets, respectively (Figure 6). The high relevance score and the number of reported evidence can represent a higher degree of association between genes and disease, and the average score and the number of reported evidence of predicted FRMs in three prescriptions were significantly higher than those of the two high reliable pathogenic genes sets. Results showed that the FRMs prediction model could accurately screen genes with higher disease correlation.
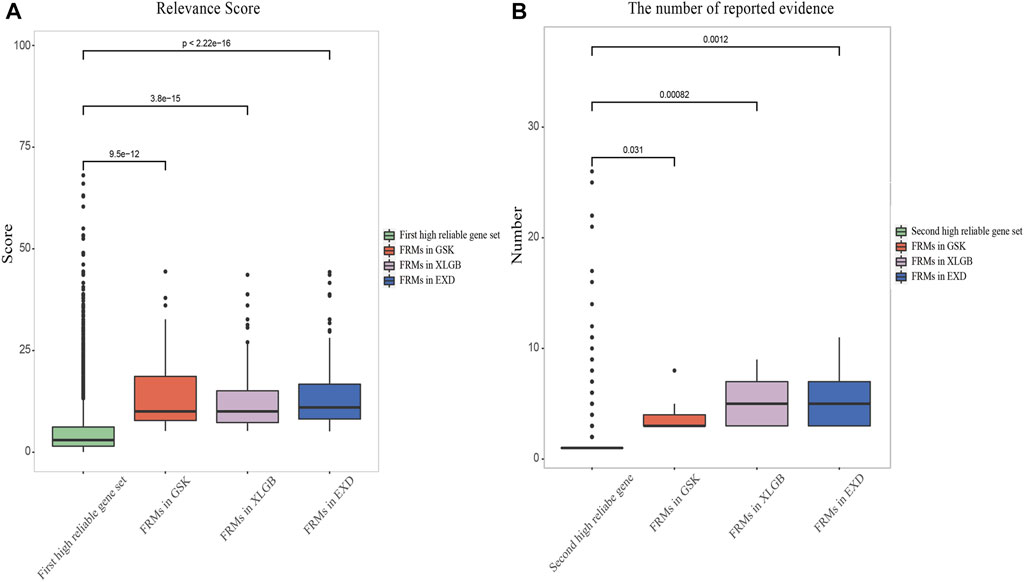
FIGURE 6. Validation of FRMs. Relevance score (A) and the number of reported evidence (B) of FRMs and two high reliable pathogenic gene sets of OP were compared.
Validating FRMs Based on Gene Enrichment Pathways Analysis
Another criterion for evaluating the accuracy and reliability of FRMs is to evaluate their functional consistency. According to this method, we used the KEGG enrichment analysis to determine whether the gene enrichment pathways in FRMs could cover the gene enrichment pathways in the C-T network as much as possible. The high pathways coverage indicates that FRMs can represent the complete C-T network at the functional level. Our analysis showed that GSK, XLGB, and EXD FRMs gene enrichment pathways accounted for 79.6, 81, and 79.5% of GSK, XLGB, and EXD complete C-T network gene enrichment pathways, respectively (Figure 7). The results showed that the predicted FRMs can represent the complete C-T network at the functional level, and it also indicated that the predicted FRMs can preserve the functional pathway of herbal medicine prescriptions to the maximum extent. Additionally, to examine whether the FRMs in each prescription have the similar therapeutic function as the original prescription, we defined the prescription-related reference pathways by selecting the intersection of target gene and pathogenic gene enriched pathways. After that, we compared the enrichment pathways of genes in FRMs for each prescription to corresponding reference pathways. The results showed that the proportion of GSK, XLGB, and EXD was 86.47, 89.63, and 79.8%, respectively (Figure 8). It showed that screened FRMs can retain the functional information of the original prescriptions and the intervention function of the original prescriptions to the maximum extent.
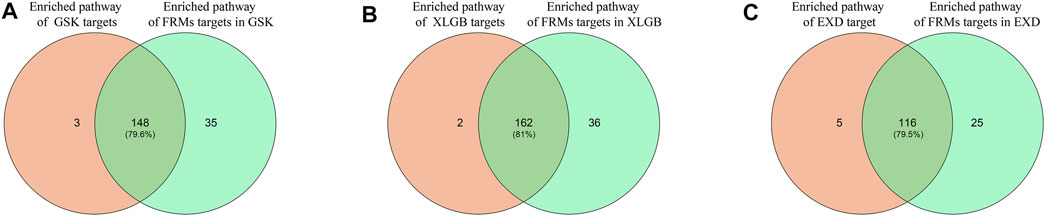
FIGURE 7. Validation of FRMs. The intersection proportion of FRMs gene enrichment pathways and C-T network gene enrichment pathways in GSK(A), XLGB(B), and EXD(C).
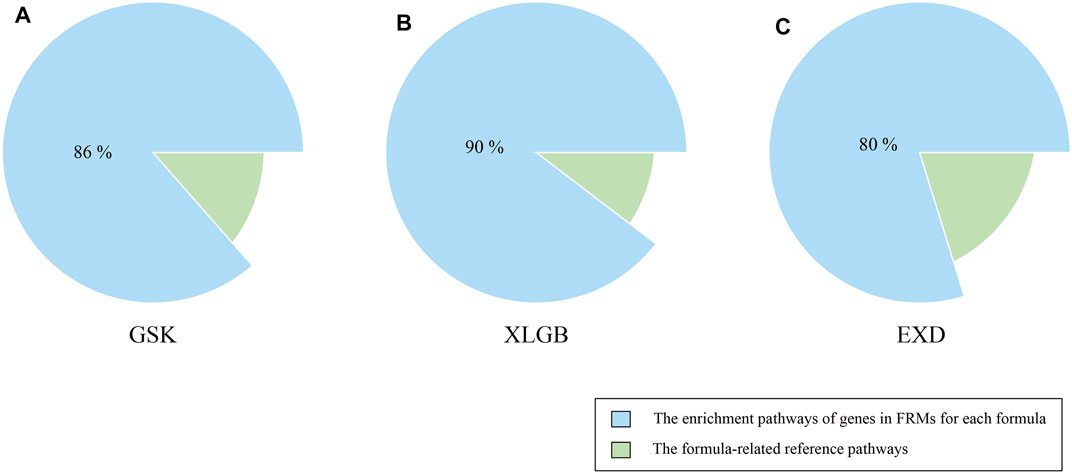
FIGURE 8. Validation of FRMs. The proportion of the enrichment pathways of genes in FRMs for GSK (A), XLGB (B), and EXD (C) to corresponding reference pathways. The formula-related reference pathways were defined as selecting the intersection of the target gene and pathogenic gene enriched pathways.
Potential Mechanism Analysis
In order to reveal the potential action mode underlying the treatment of OP by different prescriptions, we performed a pathway enrichment analysis of FRMs genes. Other disease, virus-related, and drug-resistant pathways were removed, and pathways with a count number greater than 12 were retained for further analysis. We found that 40 pathways were enriched in GSK, 66 pathways in XLGB, and 48 pathways in EXD. After combination, the three prescriptions were found to be enriched in 16 identical pathways (Figure 9), for example, steroid hormone biosynthesis (hsa00140), osteoclast differentiation (hsa04380), calcium signaling pathway (hsa04020), MAPK signaling pathway (hsa04010), and PI3K-Akt signaling pathway (hsa04151). Through PubMed literature retrieval, we found that, among these common pathways, osteoclast differentiation, calcium signaling pathway, MAPK signaling pathway, and PI3K-Akt signaling pathway were most reported. Previous studies have shown that the suppression of RANKL-induced calcium signaling inhibits OP in oophorectomy (OVX) mouse models (Chen et al., 2020). MAPK pathway is a major signaling pathway regulating OP. By restraining the production of RANKL-induced ROS and increasing the expression of antioxidant enzymes, intracellular ROS levels are inhibited and the activation of the MAPK pathway is weakened, resulting in the attenuation of downstream proteins, which contributes to the reduction of OP (Yao et al., 2018; Chen et al., 2019). Meanwhile, the PI3K/AKT signaling pathway has been proven to be essential for all stages of bone maturation, differentiation, and bone growth. Inhabiting the PI3K/AKT signaling pathway not only injures the differentiation of chondrocytes but also inhibits the growth of longitudinal bones (Ye et al., 2019).
Next, osteoclast differentiation, calcium signaling pathway, MAPK signaling pathway, and PI3K-Akt signaling pathway were combined into a gene network (Figure 10). Our analysis found that, in this gene network, GSK, XLGB, and EXD had different and co-functional effects in treating OP. There were 10 genes common to GSK, XLGB, and EXD (ITGB3, IGF1R, CHRM1, GRB2, IL2, BCL2L1, ERBB4, PIK3R1, RPS6KA4, and TACR2). For example, GSK, XLGB, and EXD all have target gene ERBB4, which played a role in OP through PI3K-Akt signaling pathway, MAPK signaling pathway, and calcium signaling pathway, and common gene IL2, which only affected OP through the PI3K-Akt signaling pathway. In the gene network, 27, 37, and 44 genes were unique to GSK, XLGB, and EXD. For example, among the 27 specific genes of GSK, LCK affected OP through osteoclast differentiation; VEGFA played a role in OP through the PI3K-Akt signaling pathway, MAPK signaling pathway, and calcium signaling pathway. Among the 37 unique genes of XLGB, HRH2 simply affected OP through the calcium signaling pathway; JUN impacted OP through the MAPK signaling pathway and osteoclast differentiation pathway. Among the 44 distinct genes of EXD, CACNB1 merely affected OP through the MAPK signaling pathway; CHRM2 influenced OP through the PI3K-Akt signaling pathway and calcium signaling pathway (Figure 10). ITGB3 belongs to the integrin family and is a membrane receptor composed of a subunit and ß subunit, which is involved in cell cycle, cytoskeletal tissue, osteoblast differentiation, and proliferation (Rapisarda et al., 2017; Lopes et al., 2019). ITGB3 can also interact with IL1RN to activate ß-catenin signaling and regulate osteoblast differentiation (Zou et al., 2021). In addition, IGF1R signaling plays an important role in osteoblast-mediated bone formation and promotes osteoblasts differentiation and maturation. During the differentiation of bone marrow stromal cells, IGF1R signaling pathway is controlled by PI3K/Akt and inhibits osteoblast apoptosis (Fang et al., 2019).
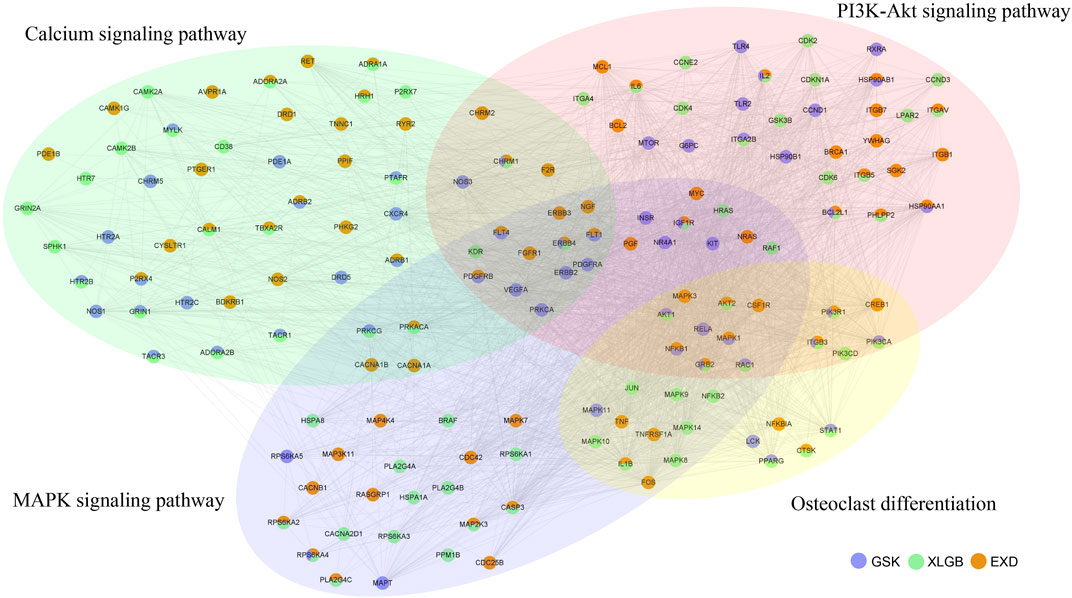
FIGURE 10. Gene network in the osteoclast differentiation, calcium signaling pathway, MAPK signaling pathway, and PI3K-Akt signaling pathway. Blue nodes represent targets in GSK. Green nodes represent targets in XLGB. Orange nodes represent targets in EXD. Nodes with three colors indicate that the gene is shared by three prescriptions. Nodes in light yellow, light green, light blue, and light red circles represent genes of osteoclast differentiation, calcium signaling pathway, MAPK signaling pathway, and PI3K-Akt signaling pathway, respectively.
Among the specific genes of GSK, cyclin D1 (CCND1) is a member of the protein kinases family associated with cell cycle regulation (Guo et al., 2013). In addition, CCND1 is often overexpressed in human diseases through translocation, amplification, or post-transcriptional regulation (Xu and Lin, 2018). Studies have found that controlling the expression of CCND1 can regulate the proliferation, differentiation, mineralization, and apoptosis of osteoblasts, therefore treating OP (Wang and Cai, 2020). In addition, it was shown that chemokine receptor-4 (CXCR4) overexpression in mesenchymal stem cells can promote BMD increase, improve MSC migration to bones, enhance MSC effects, and prevent bone loss in OVX mice after oophorectomy (Cho et al., 2009).
Among the specific genes of XLGB, CD38 has been shown to play a role in bone remodeling. It is expressed in osteoclasts, and when activated, it increases IL-6 release and inhibits bone resorption (Sun et al., 1999). Meanwhile, osteoblasts and osteoclasts express P2X7R, which regulates differentiation, function, and longevity of both cell types (Agrawal and Gartland, 2015). In the mouse model of postmenopausal osteoporosis, P2X7 has been shown to hinder bone loss. After the deletion of P2X7R in ovariectomized mice, significant bone loss, osteoblast reduction, and osteoclast increase were observed. Under normal physiological conditions, the loss of P2X7R leads to osteopenic-like bone phenotype (Wang et al., 2018b).
Among the specific genes of EXD, BCL2 is a gene that inhibits apoptosis. Studies have shown that upregulation of BCL2 may inhibit bone loss, while upregulation of BCL2 in osteoblasts can restrain osteoblast differentiation and cause osteocyte apoptosis (Moriishi et al., 2011). Colony stimulating factor 1 receptor (CSF-1R or c-FMS) is a tyrosine kinase receptor, a receptor for CSF-1, which is delivered by osteoblasts and promotes the proliferation of osteoclast progenitor cells through the combination of CSF-1R and receptor activator of nuclear factor-kappaB ligand (RANKL), leading to the formation of mature osteoclasts (Wittrant et al., 2009). Previous studies have shown that the CSF-1/CSF-1R signaling pathway affects the expression of RANK in osteoclasts and RANKL binds to RANK to induce the cascade of MAPKs, PI3K, and NF-κB signaling and finally generates NFATc1, which is the major regulator of osteoclast differentiation. The inhibition of CSF-1R on osteoclasts hinders the proliferation, differentiation, and survival of osteoclasts and downregulates the formation of osteoclast markers (TRAP) (Zinnia and Khademul Islam, 2021).
The above results indicate that the FRMs of different prescriptions have different and co-functional effects in treating OP. They provide a possible mechanism reference for us to reveal multiple treatments for the same disease.
Screen Key Component Groups
Osteoclast differentiation, calcium signaling pathway, PI3K-Akt signaling pathway, and MAPK signaling pathway were combined into an integrated pathway. A novel component importance calculation method that combined the information gain and target influence was designed and employed to screen the key component groups in different prescriptions. The components with a
Experimental Validation In Vitro
MC3T3-E1 is an osteoblast strain constructed from C57BL/6 mouse cranial parietal cells, which has biological characteristics of osteoblasts, such as ALP active type Ⅰ collagen synthesis and matrix calcification, and is often used as a cellular model for bone metabolism studies. In order to assess the reliability of our model, the CCK8 method was used to validate the effects of the key components (quercetin, isoliquiritigenin, rutaecarpine, isofraxidin, and secoisolariciresinol) on the MC3T3-E1 cells at different time points (Day 0, Day 1, Day 2). The results showed that the cell viabilities of MC3T3-E1 cells were 115.93, 119.74, 111.27, 115.11, and 117.02% after exposure to 5 μM quercetin, isoliquiritigenin, rutaecarpine, isofraxidin, and secoisolariciresinol on Day 1, respectively (Figure 11). On Day 2, the cell viabilities of five components were 147.88, 149.88, 132.34, 131.87, and 133.02%, respectively (Figure 11). Compared with the control group (DMSO), the cell viability of the MC3T3-E1 cells markedly increased after treatment with five components at Day 1 and Day 2. Our results suggested that quercetin, isoliquiritigenin, rutaecarpine, isofraxidin, and secoisolariciresinol could increase the viabilities of MC3T3-E1 cells.
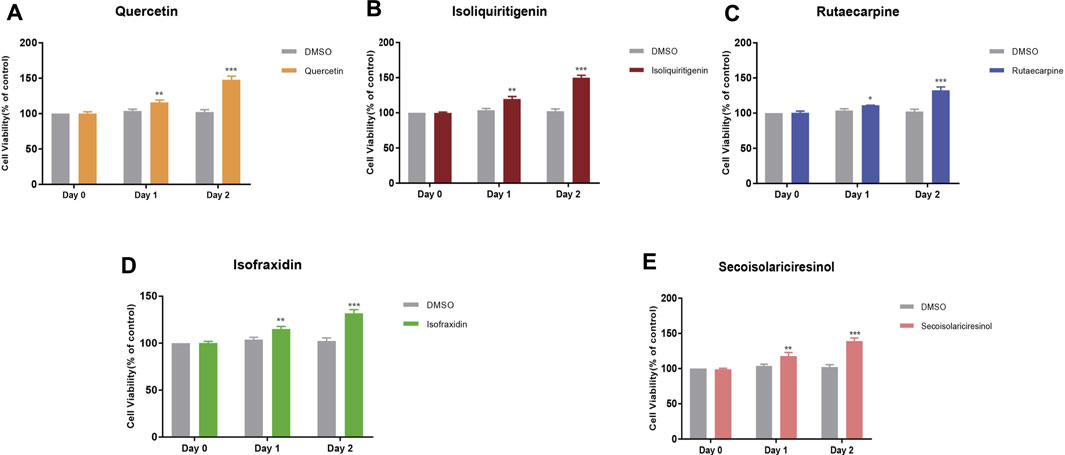
FIGURE 11. Effects of quercetin (A), isoliquiritigenin (B), rutaecarpine (C), isofraxidin (D), and secoisolariciresinol (E) on cell viabilities on MC3T3-E1 cells. *p < 0.05, **p < 0.01, ***p < 0.001 compared with DMSO group.
Discussion
OP causes fractures and other complications, which increase the physical pain of patients and bring great pressure and burden to the society and family. For the treatments of OP, drugs that promote bone mineralization, inhibit bone resorption, and promote bone formation are widely used (Das and Crockett, 2013). However, these drugs are expensive and have side effects. Thus, it is desirable to choose an alternative medicine to address this issue. Herbal medicine prescriptions have significant advantages in the treatment of OP because of their remarkable efficacy and few side effects. However, due to the characteristic of “multi-components-multi-targets-multi-pathways,” their mechanism of action is not yet clear. Therefore, it is required to exploit new methods to study the pharmacological material basis and molecular mechanism of herbal medicine prescriptions and make a scientific interpretation.
Herbal informatics is an interdisciplinary subject that integrates Chinese medicine, computer science, biology, mathematics, multi-directional pharmacology, and other disciplines. It researches complex herbal medicine systems by systematically observing the intervention and influence of drugs on disease networks (Guo et al., 2021). Herbal informatics has been widely used in herbal medicine. For example, Wang Kexin et al. systematically interpreted the compatibility of Huanglian Jiedu Decoction in treating diseases (Wang et al., 2020b). Gao Yao et al. used the method of herbal informatics to analyze the mechanism of LCW in treating Systemic Lupus Erythematosus (Gao et al., 2020). Yang Lang et al. designed a new network pharmacology method to decode the mechanism of EXD in treating OP (Yang et al., 2021). These studies demonstrate the reliability of herbal informatics.
In this study, after ADME screening, 517 active components were obtained. Among the three prescriptions, there were 88 common components; there were 65, 37, and 232 specific components in GSK, XLGB, and EXD, respectively. This suggests that the three prescriptions impact OP treatment through common and specific components.
To quickly extract vital information from the complex C-T networks, we designed a novel function motif discovery model and a genetic-based optimization model and obtained 11, 13, and 15 FRMs (p < 0.05). The FRMs were used to detect the latent action mode of GSK, XLGB, and EXD in the clinical therapeutics of OP.
The function motif discovery model based on a random walk adopts the idea of double-layer coding, which can greatly simplify the coding length. Nodes are divided into N categories with different numbers, and the same coding is used in each category so that the coding length can be saved. The genetic-based optimization model has good global search ability and can quickly search for all solutions in the solution space without falling into the trap of fast descent of local optimal solutions. Moreover, it can conveniently carry out distributed calculations, accelerate the solving speed, and have strong convergence using its inherent parallelism. At the same time, searching from the group has the potential to be parallel. It can compare multiple individuals at the same time and use the evaluation function to evaluate individuals. The process is simple. In the iterative process, the probability mechanism is used to iterate, and the selection of individuals is random to avoid accidental results. The genetic algorithm (GA) can retain good individuals and maintain the group’s diversity. The information gain inspects the feature’s contribution to the whole system and considers both the occurrence and nonoccurrence of the feature. Meanwhile, it considers comprehensively and uses the statistical attributes of all samples to reduce the sensitivity to noise.
The accuracy and reliability of FRMs were evaluated using the relevance score, the number of reported evidence, and the coverage of functional pathways of FRMs. Results showed that the predicted FRMs overlapped with the C-T network in pathogenic genes and functional pathways at high coverage.
Next, we conducted pathway enrichment analysis for each FRMs and found that 16 enriched pathways were shared by three prescriptions. Through a literature search, we found that osteoclast differentiation, calcium signaling pathway, MAPK signaling pathway, and PI3K-Akt signaling pathway were most reported. Recent research has shown that RANKL-induced ROS signaling regulates the MAPK and NF-κB activity, and the loss of NF-κB signaling in mouse models causes bone defect formation, leading to osteoporosis (Iotsova et al., 1997). RANKL can stimulate the three main members of the MAPK signaling pathway, JNK, ERK, and P38, therefore impacting the differentiation of osteoclasts (Boyle et al., 2003). In the OVX mouse model, the reduction of ROS level and the inhibition of the MAPK signaling pathway can inhibit osteoclast differentiation and reduce bone loss (Xiao et al., 2020). PI3K/AKT signaling pathway exists in mammalian cells; is involved in cell proliferation, metastasis, and apoptosis; and regulates the functions of osteoblasts and osteoclasts by affecting their formation, proliferation, differentiation, and apoptosis (Agas et al., 2013) (Qu et al., 2014). Activating the PI3K/AKT signaling pathway affects the upregulation of the expression of osteogenic differentiation marker genes, thereby promoting the proliferation and differentiation of osteoblasts (Lu et al., 2017). Inhibition of the PI3K/AKT signaling pathway can reduce the bone resorption ability of osteoclasts (Zhang et al., 2020), thus inhibiting the occurrence of osteoporosis.
Furthermore, we found that some enriched genes were shared by four pathways, but others were specific to one pathway, and these genes all played different roles in the influence of OP, indicating that the FRMs of different prescriptions have different and co-functional effects in the treatment of OP. It provides a possible mechanism reference for us to reveal multiple treatments for the same disease.
Then, we devised a novel component importance calculation method that combined the information gain and target influence to screen the key component groups in different prescriptions. The components with a
In vitro cell experiments also showed that these components can increase the viability of MC3T3-E1 cells, speculating that these components have a certain role in treating OP. Additionally, to better evaluate the dependability of our proposed network pharmacological model, we will conduct in vivo studies in future studies.
However, there are still some limitations in this study. First, more ingredients from key component groups should be selected for validating the reliability of our method and model. Second, the action mode of GSK, XLGB, and EXD in the clinic therapy of OP is not verified in this study. Meanwhile, the issue of drug dose should be considered in future studies.
In conclusion, we propose a new network pharmacology strategy that reveals the hidden mechanisms of different prescriptions for OP through new bioinformatics models and experimental validation, providing a new web-based approach for herbal medicine treatment of complex diseases.
Data Availability Statement
The original contributions presented in the study are included in the article/Supplementary Material, further inquiries can be directed to the corresponding authors.
Author Contributions
DG and AL provided the concept and designed the study. YL and DG conducted the analyses and wrote the manuscript. YL, QL, YC, YL, HY, JW, CY, QC, HY, and DG participated in data analysis. DG and YL contributed to revising and proofreading the manuscript. All authors read and approved the final manuscript.
Funding
This study is financially supported by the Startup fund from Southern Medical University (grant no. G619280010), the Natural Science Foundation Council of China (grant no. 32070676, 31501080), the National Key R&D Program of China (2018YFC1705205), Natural Science Foundation of Guangdong Province (grant no. 2021A1515010737), Hong Kong Baptist University Strategic Development Fund (grant no. SDF13-1209-P01, SDF15-0324-P02(b) and SDF19-0402-P02), Hong Kong Baptist University Interdisciplinary Research Matching Scheme (grant no. RC/IRCs/17-18/04), the 2020 Guangdong Provincial Science and Technology Innovation Strategy Special Fund (grant no. 2020B1212030006).
Conflict of Interest
The authors declare that the research was conducted in the absence of any commercial or financial relationships that could be construed as a potential conflict of interest.
Publisher’s Note
All claims expressed in this article are solely those of the authors and do not necessarily represent those of their affiliated organizations, or those of the publisher, the editors, and the reviewers. Any product that may be evaluated in this article, or claim that may be made by its manufacturer, is not guaranteed or endorsed by the publisher.
Supplementary Material
The Supplementary Material for this article can be found online at: https://www.frontiersin.org/articles/10.3389/fcell.2022.831894/full#supplementary-material
Abbreviations
OP, osteoporosis; GSK, Gushukang Granules; XLGB, Xianling Gubao Capsules; EXD, Er-xian Decoction; SDFM, Significant Different Functional Modes; FRMs, FRM, Functional Response Motif; C-T network, component-target network.
References
Agas, D., Sabbieti, M. G., Marchetti, L., Xiao, L., and Hurley, M. M. (2013). FGF-2 Enhances Runx-2/Smads Nuclear Localization in BMP-2 Canonical Signaling in Osteoblasts. J. Cel. Physiol. 228 (11), 2149–2158. doi:10.1002/jcp.24382
Agrawal, A., and Gartland, A. (2015). P2X7 Receptors: Role in Bone Cell Formation and Function. J. Mol. Endocrinol. 54 (2), R75–R88. doi:10.1530/jme-14-0226
Boyle, W. J., Simonet, W. S., and Lacey, D. L. (2003). Osteoclast Differentiation and Activation. Nature 423 (6937), 337–342. doi:10.1038/nature01658
Casado-Díaz, A., Anter, J., Dorado, G., and Quesada-Gómez, J. M. (2016). Effects of Quercetin, a Natural Phenolic Compound, in the Differentiation of Human Mesenchymal Stem Cells (MSC) into Adipocytes and Osteoblasts. J. Nutr. Biochem. 32, 151–162. doi:10.1016/j.jnutbio.2016.03.005
Chen, D., Ye, Z., Wang, C., Wang, Q., Wang, H., Kuek, V., et al. (2020). Arctiin Abrogates Osteoclastogenesis and Bone Resorption via Suppressing RANKL-Induced ROS and NFATc1 Activation. Pharmacol. Res. 159, 104944. doi:10.1016/j.phrs.2020.104944
Chen, K., Qiu, P., Yuan, Y., Zheng, L., He, J., Wang, C., et al. (2019). Pseurotin A Inhibits Osteoclastogenesis and Prevents Ovariectomized-Induced Bone Loss by Suppressing Reactive Oxygen Species. Theranostics 9 (6), 1634–1650. doi:10.7150/thno.30206
Cho, S. W., Sun, H. J., Yang, J.-Y., Jung, J. Y., An, J. H., Cho, H. Y., et al. (2009). Transplantation of Mesenchymal Stem Cells Overexpressing RANK-Fc or CXCR4 Prevents Bone Loss in Ovariectomized Mice. Mol. Ther. 17 (11), 1979–1987. doi:10.1038/mt.2009.153
Conigrave, A. D., Brown, E. M., and Rizzoli, R. (2008). Dietary Protein and Bone Health: Roles of Amino Acid-Sensing Receptors in the Control of Calcium Metabolism and Bone Homeostasis. Annu. Rev. Nutr. 28, 131–155. doi:10.1146/annurev.nutr.28.061807.155328
Daina, A., Michielin, O., and Zoete, V. (2017). SwissADME: a Free Web Tool to Evaluate Pharmacokinetics, Drug-Likeness and Medicinal Chemistry Friendliness of Small Molecules. Sci. Rep. 7, 42717. doi:10.1038/srep42717
Das, S., and Crockett, J. C. (2013). Osteoporosis - a Current View of Pharmacological Prevention and Treatment. Drug Des. Devel Ther. 7, 435–448. doi:10.2147/DDDT.S31504
Delmas, P. D. (2005). The Use of Bisphosphonates in the Treatment of Osteoporosis. Curr. Opin. Rheumatol. 17 (4), 462–466. doi:10.1097/01.bor.0000163448.51661.87
Fang, Y., Xue, Z., Zhao, L., Yang, X., Yang, Y., Zhou, X., et al. (2019). Calycosin Stimulates the Osteogenic Differentiation of Rat Calvarial Osteoblasts by Activating the IGF1R/PI3K/Akt Signaling Pathway. Cell Biol Int 43 (3), 323–332. doi:10.1002/cbin.11102
Gao, Y., Wang, K.-x., Wang, P., Li, X., Chen, J.-j., Zhou, B.-y., et al. (2020). A Novel Network Pharmacology Strategy to Decode Mechanism of Lang Chuang Wan in Treating Systemic Lupus Erythematosus. Front. Pharmacol. 11, 512877. doi:10.3389/fphar.2020.512877
Gao, Y., Wu, D., Tian, J.-S., Zhou, Y.-Z., Gao, X., Qin, X., et al. (2018). Mechanism of Network Pharmacology of Xiaoyao Powder and Kaixin Powder in Treating Depression with "Same Disease with Different Treatments. Chin. Traditional Herbal Drugs 49 (15), 3483–3492. doi:10.7501/j.issn.0253-2670.2018.15.004
Guo, S., Zhou, J. Y., Tan, C., Shi, L., Shi, Y., and Shi, J. (2021). Network Pharmacology-Based Analysis on the Mechanism of Action of Ephedrae Herba-Cinnamomi Ramulus Couplet Medicines in the Treatment for Psoriasis. Med. Sci. Monit. 27, e927421. doi:10.12659/MSM.927421
Guo, Y., Yan, K., Fang, J., Qu, Q., Zhou, M., and Chen, F. (2013). Let-7b Expression Determines Response to Chemotherapy through the Regulation of Cyclin D1 in Glioblastoma. J. Exp. Clin. Cancer Res. 32, 41. doi:10.1186/1756-9966-32-41
Iotsova, V., Caamaño, J., Loy, J., Yang, Y., Lewin, A., and Bravo, R. (1997). Osteopetrosis in Mice Lacking NF-Κb1 and NF-Κb2. Nat. Med. 3 (11), 1285–1289. doi:10.1038/nm1197-1285
Lane, J. M., Russell, L., and Khan, S. N. (2000). Osteoporosis. Clin. Orthopaedics Relat. Res. 372 (372), 139–150. doi:10.1097/00003086-200003000-00016
Li, J.-Y., Jia, Y.-S., Chai, L.-M., Mu, X.-H., Ma, S., Xu, L., et al. (2017). Effects of Chinese Herbal Formula Erxian Decoction for Treating Osteoporosis: a Systematic Review. Clin. Interv. Aging 12, 45–53. doi:10.2147/cia.s117597
Li, S. Q., Pei, Z. G., and Liu, Y. M. (2001). Clinical Study on Effect of Gushukang Granule in Preventing and Treating Primary Osteoporosis. Zhongguo Zhong Xi Yi Jie He Za Zhi 21, 265–268.
Li, S., and Zhang, B. (2013). Traditional Chinese Medicine Network Pharmacology: Theory, Methodology and Application. Chin. J. Nat. Medicines 11 (2), 110–120. doi:10.1016/s1875-5364(13)60037-0
Lipinski, C. A., Lombardo, F., Dominy, B. W., and Feeney, P. J. (2001). Experimental and Computational Approaches to Estimate Solubility and Permeability in Drug Discovery and Development Settings. Adv. Drug Deliv. Rev. 46 (1-3), 3–26. doi:10.1016/s0169-409x(00)00129-0
Liu, X., Zhang, S., Lu, X., Zheng, S., Li, F., and Xiong, Z. (2012). Metabonomic Study on the Anti-osteoporosis Effect of Rhizoma Drynariae and its Action Mechanism Using Ultra-performance Liquid Chromatography-Tandem Mass Spectrometry. J. Ethnopharmacology 139 (1), 311–317. doi:10.1016/j.jep.2011.11.017
Lopes, H. B., Freitas, G. P., Elias, C. N., Tye, C., Stein, J. L., Stein, G. S., et al. (2019). Participation of Integrin β3 in Osteoblast Differentiation Induced by Titanium with Nano or Microtopography. J. Biomed. Mater. Res. 107 (6), 1303–1313. doi:10.1002/jbm.a.36643
Lu, S.-Y., Wang, C.-Y., Jin, Y., Meng, Q., Liu, Q., Liu, Z.-h., et al. (2017). The Osteogenesis-Promoting Effects of Alpha-Lipoic Acid against Glucocorticoid-Induced Osteoporosis through the NOX4, NF-kappaB, JNK and PI3K/AKT Pathways. Sci. Rep. 7 (1), 3331. doi:10.1038/s41598-017-03187-w
Mendes, A. B., Martins, F. F., Cruz, W. M. S., da Silva, L. E., Abadesso, C. B. M., and Boaventura, G. T. (2012). Bone Development in Children and Adolescents with PKU. J. Inherit. Metab. Dis. 35 (3), 425–430. doi:10.1007/s10545-011-9412-7
Miller, P. D. (2016). Management of Severe Osteoporosis. Expert Opin. Pharmacother. 17 (4), 473–488. doi:10.1517/14656566.2016.1124856
Moriishi, T., Maruyama, Z., Fukuyama, R., Ito, M., Miyazaki, T., Kitaura, H., et al. (2011). Overexpression of Bcl2 in Osteoblasts Inhibits Osteoblast Differentiation and Induces Osteocyte Apoptosis. PLoS One 6 (11), e27487. doi:10.1371/journal.pone.0027487
Neer, R. M., Arnaud, C. D., Zanchetta, J. R., Prince, R., Gaich, G. A., Reginster, J.-Y., et al. (2001). Effect of Parathyroid Hormone (1-34) on Fractures and Bone mineral Density in Postmenopausal Women with Osteoporosis. N. Engl. J. Med. 344 (19), 1434–1441. doi:10.1056/nejm200105103441904
Papapoulos, S., and Makras, P. (2008). Selection of Antiresorptive or Anabolic Treatments for Postmenopausal Osteoporosis. Nat. Rev. Endocrinol. 4 (9), 514–523. doi:10.1038/ncpendmet0941
Qu, X., Zhai, Z., Liu, X., Li, H., Ouyang, Z., Wu, C., et al. (2014). Dioscin Inhibits Osteoclast Differentiation and Bone Resorption Though Down-Regulating the Akt Signaling Cascades. Biochem. Biophysical Res. Commun. 443 (2), 658–665. doi:10.1016/j.bbrc.2013.12.029
Rapisarda, V., Borghesan, M., Miguela, V., Encheva, V., Snijders, A. P., Lujambio, A., et al. (2017). Integrin Beta 3 Regulates Cellular Senescence by Activating the TGF-β Pathway. Cel Rep. 18 (10), 2480–2493. doi:10.1016/j.celrep.2017.02.012
Shalev, M., and Elson, A. (2019). The Roles of Protein Tyrosine Phosphatases in Bone-Resorbing Osteoclasts. Biochim. Biophys. Acta (Bba) - Mol. Cel Res. 1866 (1), 114–123. doi:10.1016/j.bbamcr.2018.07.005
Silverman, S. L. (2003). Calcitonin. Endocrinol. Metab. Clin. North America 32 (1), 273–284. doi:10.1016/s0889-8529(02)00060-9
Srivastava, M., and Deal, C. (2002). Osteoporosis in Elderly: Prevention and Treatment. Clin. Geriatr. Med. 18 (3), 529–555. doi:10.1016/s0749-0690(02)00022-8
Stefanick, M. L. (2005). Estrogens and Progestins: Background and History, Trends in Use, and Guidelines and Regimens Approved by the US Food and Drug Administration. Am. J. Med. 118 (Suppl. 12B), 64–73. doi:10.1016/j.amjmed.2005.09.059
Sun, L., Adebanjo, O. A., Moonga, B. S., Corisdeo, S., Anandatheerthavarada, H. K., Biswas, G., et al. (1999). Cd38/Adp-Ribosyl Cyclase. J. Cel Biol 146 (5), 1161–1172. doi:10.1083/jcb.146.5.1161
Tella, S. H., and Gallagher, J. C. (2014). Prevention and Treatment of Postmenopausal Osteoporosis. J. Steroid. Biochem. Mol. Biol. 142, 155–170. doi:10.1016/j.jsbmb.2013.09.008
Wang, K.-X., Gao, Y., Gong, W.-X., Ye, X.-F., Fan, L.-Y., Wang, C., et al. (2020). A Novel Strategy for Decoding and Validating the Combination Principles of Huanglian Jiedu Decoction from Multi-Scale Perspective. Front. Pharmacol. 11, 567088. doi:10.3389/fphar.2020.567088
Wang, K.-x., Gao, Y., Lu, C., Li, Y., Zhou, B.-y., Qin, X.-m., et al. (2020). Uncovering the Complexity Mechanism of Different Formulas Treatment for Rheumatoid Arthritis Based on a Novel Network Pharmacology Model. Front. Pharmacol. 11, 1035. doi:10.3389/fphar.2020.01035
Wang, K., Li, K., Chen, Y., Wei, G., Yu, H., Li, Y., et al. (2021). Computational Network Pharmacology–Based Strategy to Capture Key Functional Components and Decode the Mechanism of Chai-Hu-Shu-Gan-San in Treating Depression. Front. Pharmacol, 12, 782060. doi:10.3389/fphar.2021.782060
Wang, L. J., and Cai, H. Q. (2020). Let‐7b Downgrades CCND1 to Repress Osteogenic Proliferation and Differentiation of MC3T3‐E1 Cells: An Implication in Osteoporosis. Kaohsiung J. Med. Sci. 36 (10), 775–785. doi:10.1002/kjm2.12236
Wang, N., Agrawal, A., Jørgensen, N. R., and Gartland, A. (2018). P2X7 Receptor Regulates Osteoclast Function and Bone Loss in a Mouse Model of Osteoporosis. Sci. Rep. 8 (1), 3507. doi:10.1038/s41598-018-21574-9
Wang, Q., Zhao, Y., Sha, N., Zhang, Y., Li, C., Zhang, H., et al. (2018). The Systemic Bone Protective Effects of Gushukang Granules in Ovariectomized Mice by Inhibiting Osteoclastogenesis and Stimulating Osteoblastogenesis. J. Pharmacol. Sci. 136 (3), 155–164. doi:10.1016/j.jphs.2018.01.007
Wang, X. J., Liang, R. X., and Zhao, L. (2007). Progress of Study on Prevention and Treatment of Osteoporosis by Compound Gushukang. Zhongguo Zhong Xi Yi Jie He Za Zhi 27, 282–285.
Westbroek, I., van der Plas, A., de Rooij, K. E., Klein-Nulend, J., and Nijweide, P. J. (2001). Expression of Serotonin Receptors in Bone. J. Biol. Chem. 276 (31), 28961–28968. doi:10.1074/jbc.m101824200
Wittrant, Y., Gorin, Y., Mohan, S., Wagner, B., and Abboud-Werner, S. L. (2009). Colony-stimulating Factor-1 (CSF-1) Directly Inhibits Receptor Activator of Nuclear Factor-Κb Ligand (RANKL) Expression by Osteoblasts. Endocrinology 150 (11), 4977–4988. doi:10.1210/en.2009-0248
Wu, Z., Zhu, X., Xu, C., Chen, Y., Zhang, L., and Zhang, C. (2017). Effect of Xianling Gubao Capsules on Bone mineral Density in Osteoporosis Patients. J. Biol. Regul. Homeost. Agents 31 (2), 359.
Xiao, L., Zhong, M., Huang, Y., Zhu, J., Tang, W., Li, D., et al. (2020). Puerarin Alleviates Osteoporosis in the Ovariectomy-Induced Mice by Suppressing Osteoclastogenesis via Inhibition of TRAF6/ROS-dependent MAPK/NF-κB Signaling Pathways. Aging 12 (21), 21706–21729. doi:10.18632/aging.103976
Xu, J., and Lin, D. I. (2018). Oncogenic C-Terminal Cyclin D1 (CCND1) Mutations Are Enriched in Endometrioid Endometrial Adenocarcinomas. PLoS One 13 (7), e0199688. doi:10.1371/journal.pone.0199688
Yang, L., Fan, L., Wang, K., Chen, Y., Liang, L., Qin, X., et al. (2021). Analysis of Molecular Mechanism of Erxian Decoction in Treating Osteoporosis Based on Formula Optimization Model. Oxid Med. Cel Longev 2021, 6641838. doi:10.1155/2021/6641838
Yao, H., Yao, Z., Zhang, S., Zhang, W., and Zhou, W. (2018). Upregulation of SIRT1 Inhibits H2O2-induced O-steoblast A-poptosis via FoxO1/β-catenin P-athway. Mol. Med. Rep. 17 (5), 6681–6690. doi:10.3892/mmr.2018.8657
Ye, C., Zhang, W., Hang, K., Chen, M., Hou, W., Chen, J., et al. (2019). Extracellular IL-37 Promotes Osteogenic Differentiation of Human Bone Marrow Mesenchymal Stem Cells via Activation of the PI3K/AKT Signaling Pathway. Cell Death Dis 10 (10), 753. doi:10.1038/s41419-019-1904-7
Zhang, N.-D., Han, T., Huang, B.-K., Rahman, K., Jiang, Y.-P., Xu, H.-T., et al. (2016). Traditional Chinese Medicine Formulas for the Treatment of Osteoporosis: Implication for Antiosteoporotic Drug Discovery. J. Ethnopharmacology 189, 61–80. doi:10.1016/j.jep.2016.05.025
Zhang, Y., Cao, X., Li, P., Fan, Y., Zhang, L., Li, W., et al. (2020). PSMC6 Promotes Osteoblast Apoptosis through Inhibiting PI3K/AKT Signaling Pathway Activation in Ovariectomy-Induced Osteoporosis Mouse Model. J. Cel Physiol 235 (7-8), 5511–5524. doi:10.1002/jcp.29261
Zhao, C., Yang, J., Wu, Y., and Yu, R.Hunan University of Chinese Medicine (2019). Fuling Xingren Gancao Decoction and Ju-Zhi-Jiang Decoction in Treating Coronary Heart Disease with "Treating Same Disease with Different Treatments. Acta Chin. Med. doi:10.16368/j.issn.1674-8999.2019.11.556
Zhao, Y. H., and Wang, M. W. (2003). Progress of Experimental Research on Prevention and Treatment of Osteoporosis by Traditional Chinese Medicine. Zhongguo Zhong Xi Yi Jie He Za Zhi 23 (12), 943–945.
Zhu, N., and Hou, J. (2020). Exploring the Mechanism of Action Xianlingubao Prescription in the Treatment of Osteoporosis by Network Pharmacology. Comput. Biol. Chem. 85 (Suppl. 4), 107240. doi:10.1016/j.compbiolchem.2020.107240
Zinnia, M. A., and Khademul Islam, A. B. M. M. (2021). Fenugreek Steroidal Saponins Hinder Osteoclastogenic Bone Resorption by Targeting CSF-1R Which Diminishes the RANKL/OPG Ratio. Int. J. Biol. Macromolecules 186, 351–364. doi:10.1016/j.ijbiomac.2021.06.197
Keywords: osteoporosis, Gushukang Granules, Xianling Gubao Capsules, Er-xian Decoction, herbal medicine, functional response motif
Citation: Liu Y, Liu Q, Yin C, Li Y, Wu J, Chen Q, Yu H, Lu A and Guan D (2022) Uncovering Hidden Mechanisms of Different Prescriptions Treatment for Osteoporosis via Novel Bioinformatics Model and Experiment Validation. Front. Cell Dev. Biol. 10:831894. doi: 10.3389/fcell.2022.831894
Received: 09 December 2021; Accepted: 06 January 2022;
Published: 08 February 2022.
Edited by:
Airong Qian, Northwestern Polytechnical University, ChinaReviewed by:
Youqiang Sun, Guangzhou University of Chinese Medicine, ChinaChunli Zheng, Northwest University, China
Copyright © 2022 Liu, Liu, Yin, Li, Wu, Chen, Yu, Lu and Guan. This is an open-access article distributed under the terms of the Creative Commons Attribution License (CC BY). The use, distribution or reproduction in other forums is permitted, provided the original author(s) and the copyright owner(s) are credited and that the original publication in this journal is cited, in accordance with accepted academic practice. No use, distribution or reproduction is permitted which does not comply with these terms.
*Correspondence: Aiping Lu, aipinglu@hkbu.edu.hk; Daogang Guan, guandg0929@hotmail.com
†These authors have contributed equally to this work