Corrigendum: Single-cell RNA sequencing reveals the difference in human normal and degenerative nucleus pulposus tissue profiles and cellular interactions
- 1Department of Spinal Surgery, The Affiliated Hospital of Qingdao University, Qingdao, China
- 2Department of Medicine, Qingdao University, Qingdao, China
- 3Medical Research Center, Shandong Institute of Orthopaedics and Traumatology, The Affiliated Hospital of Qingdao University, Qingdao, China
- 4Department of Pathogenic Biology, Qingdao University Medical College, Qingdao, China
- 5Department of Spinal Surgery, Qingdao Municipal Hospital, Qingdao, China
- 6970 Hospital of the PLA Joint Logistic Support Force, Weihai, China
The nucleus pulposus (NP), a heterogeneous tissue, is an essential functional component of the intervertebral disc. However, NP cell development route and regulation mechanism in intervertebral disc degeneration (IVDD) remain unknown. Here, we performed single-cell RNA sequencing of six NP samples with normal control, mild degeneration, and severe degeneration. Based on unbiased clustering of gene expression patterns from 30,300 single-cell RNA sequencing, we identified three cell lineage families of macrophages, endothelial, and chondrocyte cells and characterized seven chondrocyte subtypes, and defined two developmental pathways of the chondrocyte cell lineage families in the process of IVDD. Additionally, CellPhoneDB analysis revealed potential interactions between chondrocyte cells and other cells in IVDD. Chondrocytes in one of the differentiated orientations interact with macrophages and endothelial cells and have an inflammatory amplification effect, which were key factors causing IVDD. Collectively, these results revealed the dynamic cell landscape of IVDD development and offered new insights into the influence of NP cells differentiation on extracellular matrix homeostasis during degeneration, providing potential treatment targets for IVDD.
Introduction
IVDD (intervertebral disc degeneration), a persistent age-related disease, has been recognized as a primary source of low back pain (Risbud and Shapiro 2014). The senior population had an IVDD prevalence of more than 90%, resulting in a massive burden on the worldwide healthcare system (Teraguchi et al., 2014; Safiri et al., 2021). The nucleus pulposus (NP), annulus fibrous, and cartilaginous endplate (CEP) of the IVD link adjoining vertebral bodies sustain body load, absorb vertical vibration, and preserve spinal mobility (Humzah and Soames 1988). The NP is a gelatinous substance made up of nucleus pulposus cells (NPCs) and extracellular matrix (ECM). ECM in healthy NP is mostly made up of type II collagen and proteoglycan (Le Maitre et al., 2007). Because of its extremely hydrating characteristics, NP may deform reversibly, which helps to alleviate mechanical conduction and balanced load distribution (Iatridis et al., 1996).
IVDD is defined by changes in the biological structure and function of NP, which are primarily influenced by the phenotype and function of NPCs (Lawson and Harfe 2015; Li et al., 2019). During the development of IVDD, NPCs increased the expression of ECM degrading enzymes, such as matrix metalloproteinases (MMPs) and disintegrin-like and metalloprotease with thrombospondin motifs (ADAMTSs). While the inhibitor protein, tissue inhibitor of metalloproteinases (TIMPs), is not expressed adequately, it fails to buffer the increased catabolism process and preserve ECM homeostasis in NP (Le Maitre et al., 2007; Vo et al., 2013). Cytokines, including interleukin (ILs), tumor necrosis factors (TNFs), and growth factors (GFs), play an important role in regulating NPC activity and attracting inflammatory and endothelial cells (ECs) throughout this process (Risbud and Shapiro 2014; Kwon et al., 2017). The secretion of proteoglycan and type II collagen by NPCs declines as a result of different processes, while the synthesis of type I and III collagen rises, resulting in ECM remodeling, which damages the tissue structure of NP and the ecological balance of IVD (Le Maitre et al., 2007; Yan et al., 2017). As a result, the pathogenic mechanism of IVDD is a complicated network involving many cell connections and dynamic regulatory mechanisms.
The dynamic alterations in the expression profile of NPCs in IVDD development are now well characterized. For example, Risbud et al. (2007) first isolated NPCs with stem/progenitor cell features from IVDD tissues, while Gilson et al. (2010) discovered a notochordal-like cell subpopulation in the NP of adult bovine IVDs. Understanding the variability of NPCs might aid in the design of fundamental strategies for biological and targeted IVDD treatment. Most existing studies, however, have been limited to the bulk level, which fails to explore the heterogeneity of NPCs and their unique roles in IVDD from a high-resolution perspective; additionally, the transcriptional regulation and cellular interactions that contribute to disease progression are unknown.
Single-cell transcriptome sequencing (scRNA-seq) has become increasingly popular in the study of tissue and cell heterogeneity, and its molecular regulatory mechanisms in physiological development, pathological processes, inflammation, and immunity as high-throughput sequencing technology have improved and innovated (Potter 2018). In this study, we performed scRNA-seq of the NP with various degrees of IVDD and explore novel cellular interactions and crucial molecular pathways contributing to the disease development.
Methods
Ethical Approval and Consent
The procedures used in this study were approved by the independent ethics committee of the Affiliated Hospital of Qingdao University (Qingdao, China). All subjects signed a written informed consent form, and all experiments were performed following study protocol.
Clinical Sample Procurement
NP tissue samples were obtained from the Department of Spinal Surgery in the Affiliated Hospital of Qingdao University. NP samples were obtained from patients with disc herniation, lumbar spondylolisthesis, and lumbar spinal stenosis who were undergoing interbody fusion surgery. During the operation, we only took tissue samples from the central area of the intervertebral disc. After that, we further cut the samples to remove residual annulus fibrosus and cartilage endplate to ensure maximum purity of the nucleus pulposus. NP samples were obtained from one patient without IVDD as the normal control group and five patients with IVDD as the IVDD group. We excluded participants who had a tumor or endocrine system diseases. Based on the preoperative lumbar MRI image, Pfirrmann grades (Pfirrmann et al., 2001) were utilized to assess the degree of degeneration. One patient (Ctrl) with spinal cord injury was diagnosed with Pfirrmann I and served as the normal control (NC); three patients (NP4, NP9, and NP10) were diagnosed with Pfirrmann II/III and served as the mild IVDD (IVDD-M); and two patients (NP2, NP8) were diagnosed with Pfirrmann IV/V and served as the severe IVDD (IVDD-S).
NP Sample Processing and Single-Cell Dissociation
The fresh NP sample at different grades was placed into the GEXSCOPE Tissue Preservation Solution (Singleron Biotechnologies) storage and transported at 2–8°C. Firstly, all samples were washed three times with Hanks Balanced Salt Solution (HBSS), cut into pieces (1–2 mm3), and subjected to enzymatic digestion with 2 ml GEXSCOPE Tissue Dissociation Solution (Singleron Biotechnologies) in a 15-ml centrifuge tube at 37°C constant temperature shaker for 15 min. Subsequently, cell suspension was filtered through a 40-μm sterile cell strainer (Corning). Cell suspensions were centrifuged at 500 g for 5 min at 4°C, the suspensions were discarded, and cell pellets were resuspended with 1 ml phosphate buffer saline (PBS) (HyClone). The cell suspension was incubated with 2 ml GEXSCOPE Red Blood Cell Lysis Buffer (Singleron Biotechnologies) at 25°C for 10 min. Then it was centrifuged at 500 g for 5 min, red blood cell was removed, and the suspension was resuspended with PBS. Finally, cells were counted using a TC20 automated cell counter (Singleron), and live cells were determined by trypan blue staining (Sigma). Isolated NP was directly prepared for cDNAs amplification and single-cell RNA-Seq library construction.
Singleron MatrixTM Single-Cell RNA Sequencing
The single-cell suspension with a concentration of 1 × 105 cells/ml was loaded onto the microfluidic chip. According to the manufacturer’s protocols (Singleron GEXSCOPE Single Cell RNA-seq Library Kit, Singleron Biotechnologies), the single-cell RNA-seq library was prepared, which was captured for sequencing by using an Illumina HiSeq X with 150 bp paired-end reads (Dura et al., 2019).
Single-Cell RNA-Seq Data Processing
To quantitatively analyze the gene expression of cells, we first removed low-quality reads by fast QC, fastp, and poly-A tails, and adaptor sequences were removed by cutadapt. After quality control, raw reads were mapped to the reference genome GRCh38 (Ensembl version 92 annotation) via STAR (Liao et al., 2014). Gene counts and unique molecular identifier (UMI) counts were acquired by the featureCounts software. Expression matrix files for subsequent analyses were generated based on gene counts and UMI counts. Cells were filtered by gene counts between 200 and 5,000 and UMI counts below 30,000. Cells with over 30% mitochondrial content were removed. We used functions from Seurat v2.3 for dimension-reduction and clustering (Butler et al., 2018). All gene expression was normalized and scaled using NormalizeData and ScaleData.
Dimension-Reduction and Clustering
We used principle component analysis (PCA) to analyze the top 2,000 variance genes, which were selected by FindVariableFeautres (Shaath et al., 2020). Cells were separated into three clusters by FindClusters using the top 20 principle components and resolution parameter at 1.0. For subclustering of chondrocyte types, we set the resolution at 1.2. The t-SNE algorithm was applied to visualize cells in a two-dimensional space. According to selected PC dimensions with the Seurat R package8, we performed the t-SNE analysis.
Differentially Expressed Gene Analysis
Differentially expressed genes (DEGs) in each cell cluster of NP were selected by Seurat FindMarkers based on Wilcox likelihood-ratio test with default parameters. Genes expressed in more than 10% of the cells in a cluster and with an average log (Fold Change) of greater than 0.25 were selected as DEGs.
Marker Gene Analysis and Identification of Cell Types
According to Seurat’s FindAllMarkers function, we determined the marker genes of each cell group relative to other cell clusters in NP by “Wilcox” (Wilcoxon rank sum test). The cell-type identity of each cluster was manually annotated with the expression of canonical markers combined with knowledge from literature. Heatmaps/dot/violin plots displaying the expression of markers used to identify each cell type were generated by Seurat DoHeatmap/DotPlot/Vlnplot.
Enrichment and Cell Interaction Analysis
The “clusterProfiler” R package version was used to perform enrichment analysis, including Gene Ontology (GO) and Kyoto encyclopedia of genes and genomes (KEGG) analysis (Yu et al., 2012). Pathways with p_adj value < 0.05 were considered as significantly enriched. Cell–cell interaction (CCI) analyses were predicted based on known ligand–receptor pairs by Cellphone DB (Efremova et al., 2020). Based on the average log gene expression distribution for all genes across each cell type, individual ligand or receptor expression was thresholded by a cutoff. Predicted interaction pairs with p-value < 0.05 and of average log expression >0.1 were considered as significant and visualized by the circlize (0.4.10) R package.
Gene Set Variation Analysis
All canonical pathways in the website of the molecular signature database (MSigDB, version 6.2) were provided by the GSEABase package (version 1.44.0). Next, we applied the gene set variation analysis (GSVA) method with default settings to assign pathway activity estimates for individual cells, as implemented in the GSVA package (version 1.30.0). To quantify the differences in pathway activity between multiple clusters, we used a generalized linear model to contrast the enrichment scores for each cell.
Transcription Factor Analysis
Human transcription factors (TFs) were obtained from AnimalTFDB3.0 for the downstream analysis. Enrichment of the predicted targets was assessed by comparing gene expression in each cluster between different groups, and only significant TFs were selected as candidate TFs. The TF network was constructed by the SCENIC R (Aibar et al., 2017) toolkit using the scRNA expression matrix. The GENIE3 package predicted a regulatory network based on the co-expression of regulators and targets. RcisTarget and AUCell were employed to trim modules for targets and evaluate the activity of the regulatory network on all the cells, respectively.
Monocle Analysis
The Monocle package (version 2.99.0) was used to plot trajectories to illustrate the behavioral similarity and transitions (Qiu et al., 2017). We used an expression matrix derived from Seurat to build a CellDataSet for Monocle pipeline, and partition the cells into supergroups after dimensionality reduction. A simple PPT method was applied in organizing supergroups into a tree-like trajectory. A plot cell trajectory module was used to plot the trajectory and color the cells by subcluster type.
Statistical Analysis
Statistical analyses were performed using R packages. ANOVA or Student’s t-test with Student–Newman–Keuls post hoc test was performed to determine the statistical significance between differences, and p-value < 0.05 was considered as statistically significant. Statistical calculations were performed by SPSS 21.0. Statistical analyses were performed in GraphPad Prism (version 8.3).
Result
Cellular Constitution of Human NP in IVDD
To investigate cellular diversity, we used scRNA-seq analysis on six NP samples from five patients with various degrees of IVDD (IVDD-M: NP4, NP9, and NP10 and IVDD-S: NP2 and NP8) and one spinal cord injury patient without IVDD (NC) (Figure 1A). Clinical information was collected from the patient records as shown in Supplementary Table S1. A total of 30,300 cells were collected after data pre-processing and quality assessment. Each cell had an average of 6,089 UMIs and 1,671 genes. Unsupervised cell clustering revealed three major groups in parallel based on lineage-specific marker gene expression: chondrocytes (particularly expressing SOX9, ACAN, and COMP), endothelial cells (EC, specifically expressing PECAM1, CD34, and PLVAP), and macrophages (specifically expressing CD74, TYROBP, and LAPTM) (Figures 1B,C and Supplementary Figure S1A and Supplementary Table S2). Chondrocytes, which make up the majority of the cells in all samples, are further split into three empirically defined populations: cartilage progenitor cells (CPCs), fibrochondrocyte progenitors (FCPs), and homeostatic chondrocytes (HomCs), as well as four new populations known as C1–4 (Figures 1D,F). Figure 1F and Supplementary Figure S1B show each chondrocyte population based on cell lineage-specific marker gene expression. The top 20 differentially expressed genes (DEGs) are shown in Figure 1G and Supplementary Table S3.
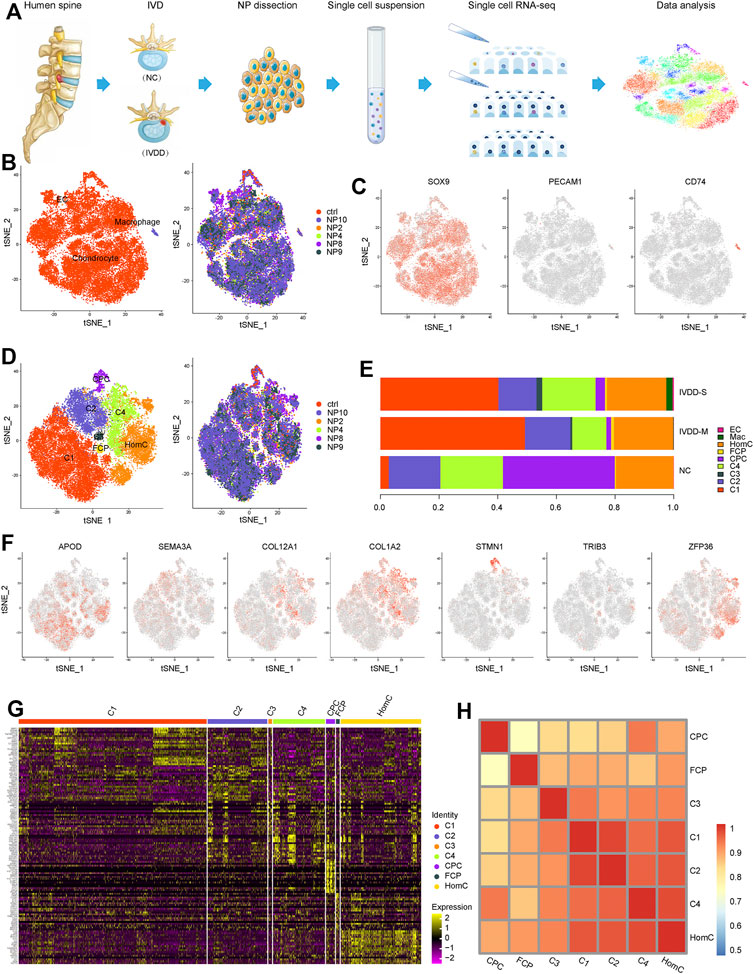
FIGURE 1. Single-cell atlas of healthy and degenerative NP samples. (A) Schematic diagram of sample source and experimental workflow. (B) t-SNE of the 30,300 cells profiled three main cell types (left) and six samples (right) distribution. (C) t-SNE plots showing the expression of marker genes for chondrocyte, macrophage, and EC defined above each panel. (D) t-SNE of the chondrocytes profiled seven subclusters (left) and six samples (right) distribution. (E) Bar plots represented the proportion of the distinct cell clusters in NP from NC, IVDD-M, and IVDD-S. (F) t-SNE plots showing the expression of marker genes for seven chondrocyte subclusters defined above each panel. (G) Heatmap of top 20 DEGs for seven chondrocyte subclusters; each column represents a cell cluster, and each row represents a DEG for a cluster. (H) Heatmap showing pairwise Pearson correlations in each chondrocyte subcluster. NP, nucleus pulposus; t-SNE, t-distributed stochastic neighbor embedding; EC, endothelial cells; CPC, cartilage progenitor cells; FCP, fibrochondrocyte progenitors; HomC, homeostatic chondrocytes; DEGs, differentially expressed genes.
Next, we compared the distribution of subclusters in each group (Figure 1E and Supplementary Table S4) and discovered that chondrocytes were the majority in the samples, whereas EC and macrophage were only slightly present in IVDD-M and IVDD-S, which is consistent with the phenomenon of inflammatory response and vascular growth in IVDD (Kwon et al., 2017; Nakazawa et al., 2018). Interestingly, we discovered that three chondrocyte subclusters are linked to the IVDD process. C1 was seldom in the NC group, whereas it was the most common chondrocyte subtype in the degenerative samples. However, as IVDD progressed, the proportion of CPCs fell dramatically. Furthermore, C3 was only found in degenerative samples, and the proportion was greater in the IVDD-S group than in the IVDD-M group.
Characterization of Chondrocyte Subtypes
Chondrocytes secrete ECM such as collagen and proteoglycan, which are required for the NP’s structure and function. We performed Gene Ontology (GO) analysis with DEGs to objectively comprehend the heterogeneity and biological function of chondrocyte subgroups (Figures 2A–H). Specifically, we discovered genes like FGF2, HMOX1, CXCL8, and CXCL2 promote C1’s multifunctionality in the BP of positive regulation of vasculature development, antimicrobial humoral response, and transition metal ion homeostasis (Simons 2004; Semple et al., 2010; Loboda et al., 2016) (Figure 2A). Extracellular matrix organization and ossification were considerably enhanced in C2, C3, and C4, while the three chondrocytes expressed a distinct array of ECM proteins and MMPs, such as C2 expressing COL2A1 and VCAN, C3 expressing COL12A1 and MMP2, and C4 expressing COL3A1 and COL1A1. Furthermore, the GO analysis revealed that C2 is related to the cholesterol biosynthetic process, C3 is involved in extracellular matrix disassembly, and C4 is more prone to tissue remodeling (Figures 2B–D). In summary, C1 and C3 were classified as inflammatory response subsets. C2 has a high expression of collagen type II and proteoglycan to keep the ECM’s regular structure. C4 has a phenotype similar to fibroblasts and may contribute to ECM remodeling (Reeves et al., 2014).
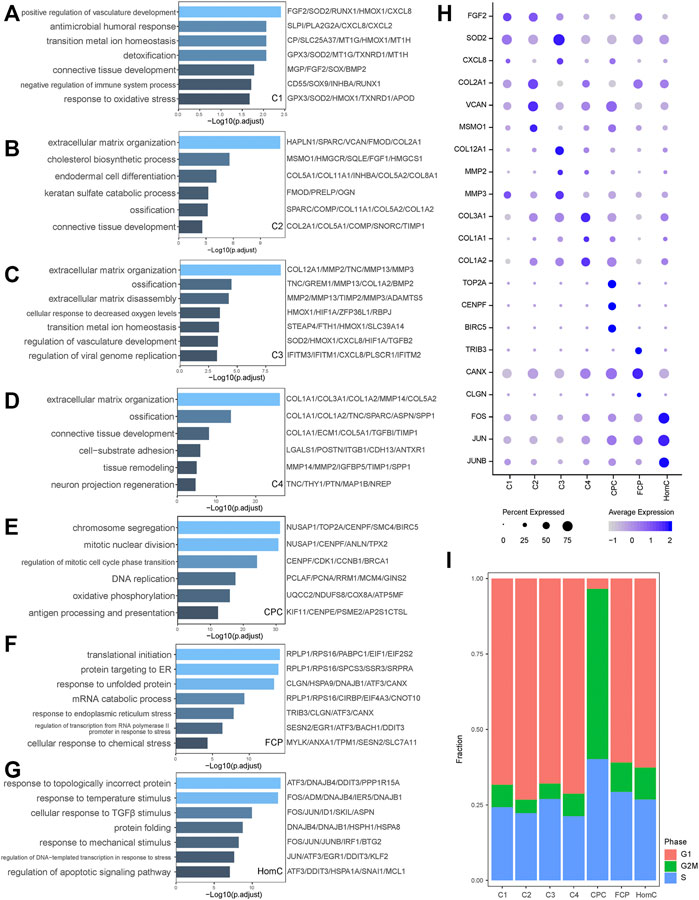
FIGURE 2. Characterization of biological functions of chondrocyte subclusters. (A–G) Bar plots showed the upregulated pathways and representative genes corresponding to seven chondrocyte subclusters of C1, C2, C3, C4, CPC, FCP, and HomC, respectively, by GO analysis. (H) Dot plot showing the expression levels of selected representative genes among seven chondrocyte subclusters. Dot size indicates the percentage of cells expressing the particular genes, and the spectrum of color represents the mean average expression of the genes. (I) The proportion of cells annotated as the different cell cycle phases in seven chondrocytes subclusters. CPC, cartilage progenitor cells; FCP, fibrochondrocyte progenitors; HomC, homeostatic chondrocytes.
We also discovered that CPC, FCP, and HomC display different functions in IVDD. CPC, as NP-derived progenitor/stem cells, specifically expressed TOP2A, CENPF, and BIRC5, all of which are associated with chromosome segregation and mitotic nuclear division, which is consistent with our GO analysis (Bellayr et al., 2016; Kanfer and Kornmann 2016; Wheatley and Altieri 2019; Nielsen et al., 2020) (Figure 2E). Annotation of the cell cycle revealed that the fraction of CPC in the G2/M phase was substantially higher than that of other subpopulations, demonstrating its active proliferative ability (Figure 2I). FCP-related genes TRIB3 and CANX have been linked to protein folding quality control and ER stress (Mondal et al., 2016; Forrester et al., 2019) (Figure 2F). HomC has a significant capacity to respond to stress, such as response to topologically incorrect protein, response to temperature stimulus, and cellular response to TGFβ stimulus. FOS/JUN, as transcription factors (TFs), were crucial in regulating the HomC response to stress (Wagner 2001) (Figure 2G). Above all, these seven chondrocytes perform a variety of tasks while retaining a high degree of connection (Figure 1H).
Transcriptional Regulation in Chondrocyte Subpopulations
The single-cell regulatory network inference and clustering (SCENIC) approach was performed to investigate the TFs that may govern various chondrocyte phenotypes. This analysis revealed that several TF networks were enriched in distinct subclusters (Figure 3A). In C1 and C3, the NF-kappaB family (NFKB1, NFKB2, and REL) shows enhanced regulatory activity, which is consistent with the inflammatory response subgroups, but FOSL1 and its target genes, including MMP2, MMP3, and MMP13, are substantially upregulated in C3 (Chen et al., 2020). TCF4 regulon activity is greater in C4, which may contribute to C4’s fibrous characteristics (Mathew et al., 2011). TFDP1, BRCA1, and MYBL1 are CPC-specific regulons that play important roles in the cell cycle and DNA damage repair control (Qiao et al., 2007; Wu et al., 2010; Xie et al., 2020). Coincidentally, TFDP1 controls BRCA1 transcriptional activity in chondrocytes (Pellicelli et al., 2016). Furthermore, SCENIC results revealed that the regulatory activities of JUN and FOS regulons in HomC were highly elevated, which is consistent with GO analysis (Figures 3B,C and Supplementary Figure S1C). Thus, we investigated the potential upstream regulatory TFs, which will aid in our understanding of the heterogeneity of NPCs and their involvement in IVDD.
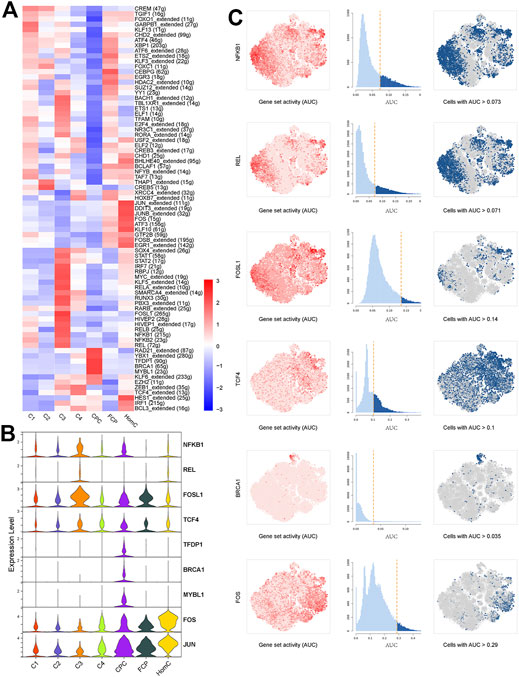
FIGURE 3. Transcriptional regulation of seven chondrocyte subclusters. (A) Heatmap indicating the expression regulation by TFs analyzed with SCENIC in seven chondrocyte subclusters. Numbers between brackets indicate the (extended) regulons for respective TFs. (B) Violin plots showing the expression levels of representative candidate TFs across the seven chondrocyte subclusters. (C) SCENIC analysis predicts selected TFs as central hubs governing the different chondrocyte subclusters. TF regulon activities were quantified using AUCell. TFs, transcription factors; SCENIC, single-cell regulatory network inference and clustering; CPC, cartilage progenitor cells; FCP, fibrochondrocyte progenitors; HomC, homeostatic chondrocytes.
The Trajectory of Chondrocytes in NP
Changes in chondrocyte expression patterns are closely linked to the development of IVDD (Li et al., 2019; Tsingas et al., 2020). To study the conversion of chondrocytes in NP during IVDD development, we performed a trajectory analysis of various chondrocyte subtypes by Monocle two method in all samples (Supplementary Figure S2A). However, we discovered that NP10 is not suitable for the current analysis programs because its pseudotime trajectory did not fit well enough with other samples from the IVDD-M group, which could be due to the presence of degenerative spondylolisthesis and the “intervertebral disc vacuum phenomenon” at the sample segment in this patient (Supplementary Figure S2B). Then, except for NP10, we ran a trajectory analysis on chondrocytes and created a pseudotime trajectory axis with two terminals corresponding to two distinct cell fates (Figures 4A–C). The cells in the root of the trajectory were labeled R-C, the cells in fate1 were labeled F1-C, and the cells in fate2 were labeled F2-C (Figure 4A). In the pseudotime trajectory, we compared the dispersion patterns of several chondrocyte subtypes. CPC is mostly found near the root. C1 mostly follows two fates, C3 and FCP primarily occupy the ends of the two fates, and C2, C4, and HomC are scattered along the trajectory (Figure 4C). Figure 4D revealed that the proportion of R-C progressively dropped, while the proportion of F1-C and F2-C steadily rose as the disease progressed. In general, our result indicates that the distribution of chondrocyte subsets in the chondrocyte fate differentiation curve was consistent with the IVDD pathological process.
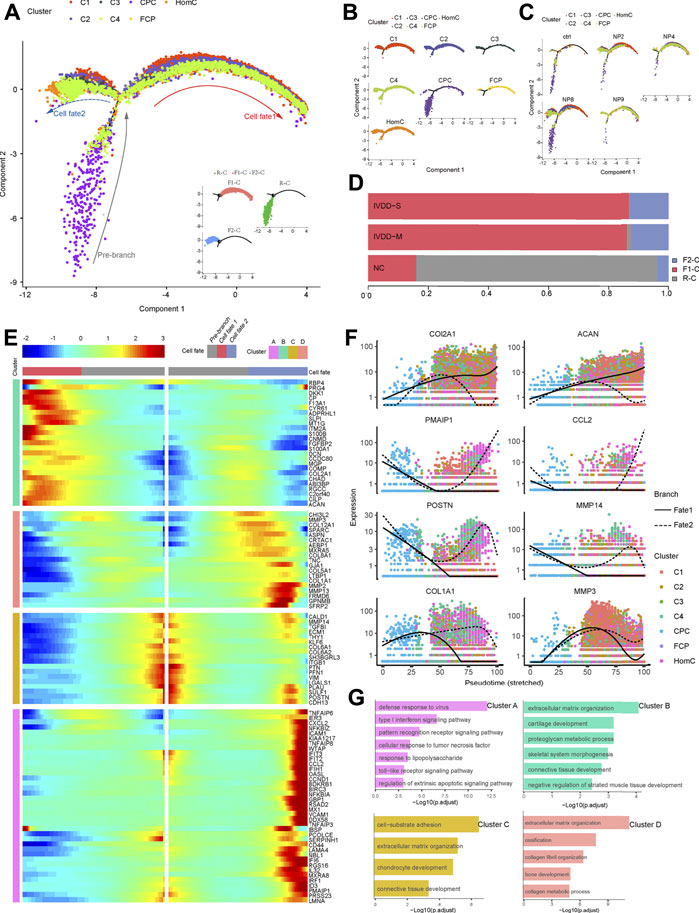
FIGURE 4. Pseudotime trajectory analysis depicted chondrocyte fate differentiation in IVDD. (A) Monocle two trajectory plot contains three branches and one connecting point showing dynamics of chondrocyte subclusters (except NP10). (B,C) Projection of individual cell types and samples onto the trajectory of (A). (D) Bar plots represented the proportion of different cell states corresponded to three branches from NC, IVDD-M, and IVDD-S. (E) Heatmap showing the standardized kinetic curves of the genes in trajectory from root to fate1 or fate2. (F) Pseudotime kinetics of specific representative genes from the root of the trajectory to fate1 (solid line) and fate2 (dashed line). (G) Bar plots showing the top annotated GO terms in four genes sets, which are hierarchically clustered from (E). CPC, cartilage progenitor cells; FCP, fibrochondrocyte progenitors; HomC, homeostatic chondrocytes.
Next, using the results of the trajectory analysis, we deconstructed sample gene transformation patterns to investigate the precise impact of the alterations in cell fate (Figure 4E). These genes were divided into four distinct clusters. Cluster “A” enriched with genes (PMAIP1, CCL2, CXCL2, and ICAM1) linked to defense response to virus, type I interferon signaling pathway, and cellular response to TNF was dramatically elevated in the transition to fate2. Meanwhile, clusters C and D genes enriched in the ECM structure are also expressed in the direction of fate2, including MMP2, MMP3, POSTN, and COL1A1. Cluster “B” genes (COL2A1 and ACAN) highly expressed in normal ECM were dramatically enhanced in the transition to fate1 (Figures 4E–G).
Finally, trajectory analysis revealed that during IVDD, NPC differentiated into two cell fates (R-C to F1-C and R-C to F2-C). The process of chronic inflammation and changes in ECM has a high correlation with the two cell fates, implying that studying the differentiation direction of NPCs, particularly the mechanism network of fate2, may provide an effective strategy for the treatment of IVDD.
The Different Cell Fate Determination of NPCs Reflects a Landscape of IVDD Development
To deeply insight into the mechanism behind IVDD, we utilized GSVA to characterize R-C, F1-C, and F2-C functions, and SCENIC to discover candidate TFs. Figures 5A–D and Supplementary Table S5 depict more precise information about cell distribution, cell cycle annotation, and DEGs from chondrocyte cells in three stages.
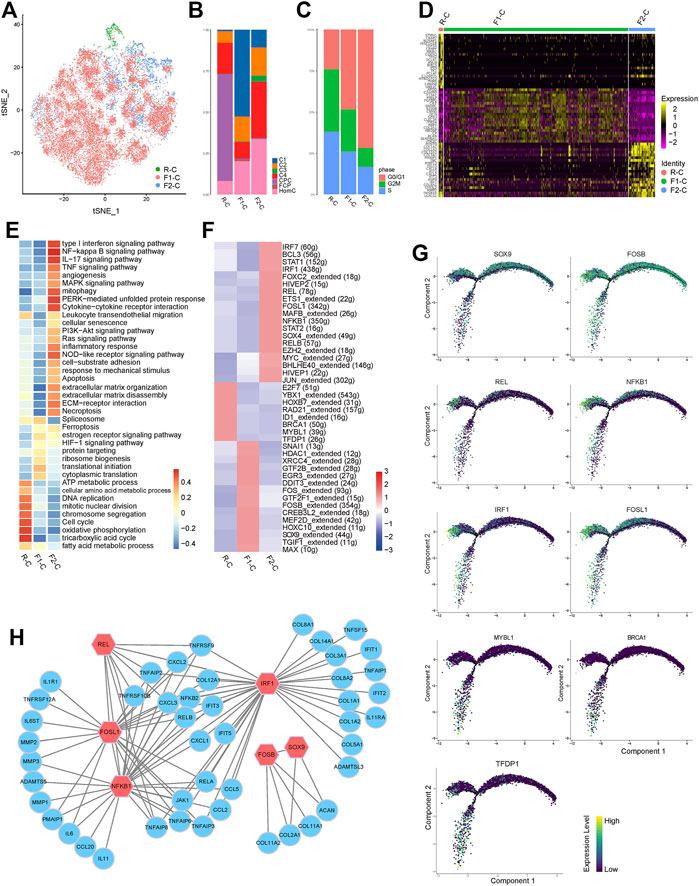
FIGURE 5. Transcriptional regulation related to chondrocyte differentiation fate. (A) t-SNE of the chondrocytes profiled three cell states (R-C, F1-C, and F2-C). (B) Bar plots represented the proportion of seven chondrocyte subclusters in R-C, F1-C, and F2-C. (C) Bar plots represented the proportion of cells annotated as the different cell cycle phases in R-C, F1-C, and F2-C. (D) Heatmap showing top 20 DEGs for R-C, F1-C, and F2-C; each column represents a cell state, and each row represents a DEG for a state. (E) The heatmap of GSVA of the GO and KEGG gene sets related to IVDD among the three cell states. (F) Heatmap indicating the expression regulation by TFs analyzed with SCENIC in three cell states. Numbers between brackets indicate the (extended) regulons for respective TFs. (G) The expression levels of the selected TFs on pseudotime trajectories. (H) Regulatory networks consisting of selected TFs and their target genes drive cell fate differentiation. A node represents a gene and edges represent the interactions. Red nodes are hub TFs. CPC, cartilage progenitor cells; FCP, fibrochondrocyte progenitors; HomC, homeostatic chondrocytes; DEGs, differentially expressed genes; TFs, transcription factors; SCENIC, single-cell regulatory network inference and clustering.
R-C is primarily concentrated in the cell cycle and oxidative phosphorylation (Figure 5E). However, when the cells are differentiated into the two cell fates, the expression of TFs (TFDP1, BRCA1, and MYBL1) is reduced (Figures 5F,G). F1-C cells are primarily enriched in protein targeting, translational initiation, and ribosome biogenesis (Figure 5E), indicating that these cells are primarily employed for protein synthesis, processing, and transport. Notably, the ECM produced at this stage was mostly comprised of collagen type II and proteoglycan (coded by COL2A1 and ACAN), with minimal expression of MMPs and ADAMTs (Figure 5D, Supplementary Table S5). We also discovered that FOSB and SOX9, the particular transcription factors predicted by SCENIC to control COL2A1 and ACAN, were significantly expressed in this condition (Figures 5F–H). F2-C genes were enriched in an ECM organization and ECM disassembly, such as COL1A1, COL1A2, COL3A1, and MMP13. Meanwhile, F2-C highly expressed a wide range of inflammatory chemokines, including CXCL8 and CXCL2. Furthermore, F2-C overexpressed several interferon-induced genes, including IFIT1, IFIT2, and IFIT3. Meanwhile, JAK1 and STAT1, which are upstream regulatory components of interferon-induced genes, were also considerably increased (Zhou et al., 2013) (Figures 5D,E and Supplementary Table S5). The interferon signaling pathway is widely recognized to have antiproliferation and pro-apoptotic effects, which may be one of the reasons for the reduction in the number of NPCs in IVDD. We discovered that NFKB1, FOSL1, IRF1, and REL have strong regulatory activity and can control each other at this stage using SCENIC, and interestingly, the predicted target genes of these TFs are the same genes that are highly expressed in F2-C. Furthermore, TNF, NF-κB, MAPK, IL17, and type I interferon signaling pathways enriched in F2-C can cause the upregulation or activation of these TFs (Beg and Baldwin 1994; Wang et al., 2017; Bonelli et al., 2019) (Figures 5E–H). Taken together, these data indicated TFs and signal pathways that govern the fate of various cells in IVDD, contributing to a better understanding of the disease mechanism.
Constructing a Cell Fate-Based Cell Communication Network for IVDD
The ligand–receptor pair facilitates cell contact, influences cell function and destiny, and promotes or inhibits disease development. In our study, macrophages and EC were only found in the IVDD group, and we utilized CellPhoneDB to investigate potential connections between chondrocytes and macrophages and EC at various cell fates (Figure 6A).
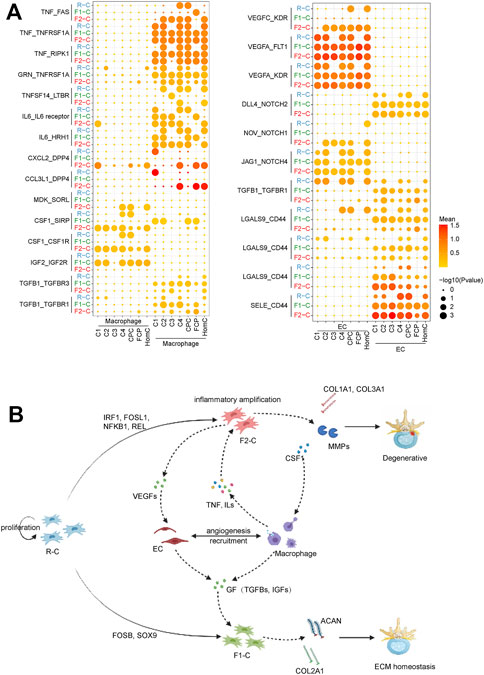
FIGURE 6. Network of regulatory mechanisms for IVDD. (A) Dot plots depicting ligand–receptor pairs between chondrocytes and macrophage (left) and EC (right). Dot size indicates the percentage of cells expressing the particular genes and the spectrum of color represents the mean average expression of the genes. (B) Diagram of the regulatory mechanisms of IVDD found in this study. Solid arrows represent the direction of cell differentiation; dashed arrows represent cytokines and extracellular matrix secreted by cells.
Macrophages secrete a large number of TNF ligand superfamily cytokines, including TNF, TNFSF10, and TNFSF14, whereas chondrocytes express corresponding receptors, including LTBR, TNFRSF1A, RIPK1, and FAS, indicating that the macrophage plays an apoptosis-mediated role in IVDD via the TNF signaling pathway. Surprisingly, our findings revealed that FAS-mediated programmed cell death favors F2-C (Wallach et al., 1999). Meanwhile, F1-C inhibits TNF signal-mediated apoptosis via GRN binding to TNF signaling receptors (Tang et al., 2011). Macrophages produce CCL3L1 and CXCL12, which bind to the DPP4 receptor expressed by chondrocytes. DPP4 is overexpressed in osteoarthritic chondrocytes to have a pro-inflammatory impact (Wang et al., 2020). F2-C, in turn, controls macrophage proliferation, migration, and differentiation via CSF1 and MDK (Sato et al., 2001; Lin et al., 2019). This reveals the interplay between F2-C and macrophages in inflammatory amplification. GFs are cytokines that control cell proliferation and differentiation. Our findings suggest that F1-C interacts more with macrophages via GFs (TGFB1, IGF2). Recent research has demonstrated that the TGF pathway promotes SOX9 production in chondrocytes (Lee et al., 2008), which helps to explain why SOX9 is so abundant in F1-C (Figure 6A).
Chondrocytes express vascular endothelial growth factors (VEGFs), which include VEGFA, VEGFB, and VEGFC, the most potent angiogenesis agents (Dai and Rabie 2007). VEGFs, as ligands, can stimulate EC proliferation, migration, and differentiation by binding to EC-expressed KDR and FLT1. The connection between chondrocytes and EC is also mirrored in the Notch family’s control of cell destiny (including NOTH1, NOTH2, and NOTH4) (Ramasamy et al., 2014), and these interactions are more visible in F2-C. Our findings revealed that R-C chondrocytes influenced EC via TGF-β, whereas EC influenced F1-C chondrocytes via TGF-β. Furthermore, SELE and LGALS9-expressing EC interact with the ligand CD44 produced by chondrocytes, which plays a crucial role in the regulation of cell contact and cell adhesion (Auguste et al., 2007; O'Brien et al., 2018) (Figure 6A).
Finally, examination of intercellular communication indicated signal crosstalk between chondrocytes, macrophages, and EC. F1-C was more active in the GF pathway, which can maintain the normal development of the chondrocytes. In contrast, in F2-C, macrophages and ECs interact with chondrocytes to promote chondrocyte apoptosis and enhance inflammatory response, which may be a key factor for the development of IVDD.
Discussion
NPCs are a crucial functional component of the IVD, and thorough knowledge of their transcriptome profile in degeneration will aid in the development of innovative treatment methods. In this study, we found out several intriguing discoveries by doing single-cell sequencing on NPCs from different phases of IVDD.
Adults believe the healthy IVD to be an immune privilege, an avascular organ whose nutrient supply is largely dependent on fluid diffusion from the CEP (Urban et al., 2004). IVDD is an unavoidable aging process that involves an inflammatory response and subsequent ECM remodeling (Le Maitre et al., 2007; Chen et al., 2017; Tsingas et al., 2020), which is consistent with our results that macrophages and ECs were exclusively detected in the degenerative NP. The uneven distribution of macrophages and EC between IVDD-M and IVDD-S indicates that they play a role in the IVDD process.
Earlier research has demonstrated that the NP develops from the notochord during embryonic development (Lawson and Harfe 2015). As a result, we looked at the expression of the notochord cell markers KRT8, KRT18, KRT19, FOXA2, and TBXT in chondrocytes (Weiler et al., 2010) (Supplementary Figure S2C). Although these cells are sporadic and not clustered by the t-SNE, this result confirms earlier findings that as the NP develops, notochord lineage cells gradually vanish in the IVD, but a small number of cells continue to express notochord cell markers, at the same time indicating the dynamic evolution of the NP phenotype (Stosiek et al., 1988; Weiler et al., 2010).
In this study, we identified three empirically defined and four novel chondrocyte subsets. Our findings revealed that the quantity of C1 and C3 rose significantly in the IVDD group and that they were mostly dispersed in two branches of cell destiny in the trajectory tree. Furthermore, C1 and C3 had greater levels of chemokines (CXCL8 and CXCL2) and matrix-degrading enzymes (MMP2, MMP3, and MMP13), all of which are thought to be important mediators of the inflammatory response. Interestingly, C1 and C3 are also abundant in transition metal ion homeostasis, with genes CP, Hmox1, and STEAP4 playing key roles in iron and copper ion transport and redox processes. According to Jomova and Valko (2011), disturbance of transition metal ion homeostasis can cause oxidative stress and increased generation of reactive oxygen species, which can contribute to the onset of a variety of diseases, including chronic inflammation. Our previous research found that ferroptosis occurred in NPCs and accelerated IVD degeneration (Zhang et al., 2021). However, the underlying mechanism of ion-homeostasis system in IVDD needs to be investigated further. In summary, the functional study of seven chondrocyte subgroups offered more thorough knowledge of the maintenance and control of NP homeostasis by elucidating NP cell heterogeneity.
Significant research has provided insight into the ability of mesenchymal stem cells to heal damaged intervertebral discs (Richardson et al., 2016). Despite the existence of progenitor cells developing into osteogenic, adipogenic, or chondrogenic lineages in degenerative adult IVD discovered in a previous work by Risbud et al. (2007), the monitoring of the resident progenitor cells in the NP has remained a mystery until now. Recently, a collection of single-cell sequencing data on articular cartilage was used to identify the existence of CPC (Ji et al., 2019). Based on our findings, CPC was plentiful in normal IVD and steadily reduced with degeneration, and has a high proliferative capacity and greater energy consumption. Above all, the findings of this study are likely to pave the way for a novel strategy to treating IVDD utilizing NP-derived progenitor cells.
Through pseudotime analysis, we established the trajectory of chondrocyte development toward two cell fates. During the degeneration process, we witnessed chondrocytes transforming into two opposing cell phenotypes. SCENIC revealed the major regulatory function of FOSB and SOX9 for COL2A1 and ACAN from R-C to F1-C (fate1). Furthermore, CellphoneDB indicated that chondrocytes have a higher contact with macrophages and EC in this phase via TGF-β signaling pathways. Previous research has shown that SOX9 stimulates NPC formation and maintains ECM homeostasis (Cucchiarini et al., 2007; Lefebvre and Dvir-Ginzberg 2017; Tsingas et al., 2020). Interestingly, recent studies have shown that the TGF-β signal pathway can increase the transcriptional level of COL2A1 and ACAN by upregulating the expression of SOX9 (Lee et al., 2008; Bozhokin et al., 2020), which explains why COL2A1 and ACAN are abundant at this phase.
On the other hand, our findings show that chondrocyte differentiation to fate2 is the primary factor causing IVDD. Chen et al. (2020) found that FOSL1 can regulate the expression of MMPs to promote the migration of decidua stromal cells. Forero et al. (2019) showed that IRF1 is activated by type I interferons and upregulates the expression of multiple inflammatory factors. Campbell et al. (2000) reported that NFKB1 and REL are essential for mediating inflammatory responses in rheumatoid arthritis. Our data establish that these TFs (NFKB1, IRF1, REL, and FOSL1) increased the production of collagen, inflammatory factors, and matrix-degrading enzymes, all of which are linked with ECM remodeling in IVDD. CellphoneDB research indicated that F2-C interacts with macrophages and EC, causing inflammation to be amplified. Macrophages boost F2-C’s inflammatory response by secreting IL6 and TNF, whereas F2-C’s CSF1 stimulates macrophage proliferation, differentiation, and migration. Furthermore, VEGFs are abundantly expressed in F2-C, where they stimulate EC proliferation, migration, and differentiation while also playing an essential role in the development of microvessels, which can speed up macrophage recruitment (Figure 6B). Thus, our findings indicate that cells from the same subpopulation produced by single-cell clustering have distinct fates in practice. However, the precise causes causing this occurrence must be investigated and debated further.
In conclusion, our single-cell sequencing data provided a detailed inventory of the NPCs in IVDD from a single-cell perspective. Our data corroborated earlier findings and presented fresh study paths with potential therapeutic targets by identifying critical cell subclusters, signal pathways, and TFs, modeling cell–cell interactions, and, most significantly, giving insights into cell fate determination.
Data Availability Statement
The data presented in the study are deposited in the China National GeneBank repository, accession number CNP0002664 (https://db.cngb.org/search/project/CNP0002664/).
Ethics Statement
The studies involving human participants were reviewed and approved by the independent ethics committee of the Affiliated Hospital of Qingdao University (Qingdao, China). The patients/participants provided their written informed consent to participate in this study.
Author Contributions
SH: conception and design, literature search, investigation, drafting of the article; SH and YZ: conception and design, provision of study materials; SH, XZ, HZ, SM, and MK: analysis and interpretation of the data; SH and XL: collection and assembly of data; XM: obtaining of funding, administrative, and final approval of the article.
Funding
This work was supported by the National Natural Science Foundation of China (CN) (Grant Nos. 81871804 and 81672200), National Key R&D Program of China (2019YFC0121400), and Natural Science Foundation of Shandong province (ZR2020QH082).
Conflict of Interest
The authors declare that the research was conducted in the absence of any commercial or financial relationships that could be construed as a potential conflict of interest.
Publisher’s Note
All claims expressed in this article are solely those of the authors and do not necessarily represent those of their affiliated organizations, or those of the publisher, the editors, and the reviewers. Any product that may be evaluated in this article, or claim that may be made by its manufacturer, is not guaranteed or endorsed by the publisher.
Acknowledgments
We thank Singleron Biotechnologies GmbH for technical support.
Supplementary Material
The Supplementary Material for this article can be found online at: https://www.frontiersin.org/articles/10.3389/fcell.2022.824771/full#supplementary-material
References
Aibar, S., González-Blas, C. B., Moerman, T., Huynh-Thu, V. A., Imrichova, H., Hulselmans, G., et al. (2017). SCENIC: Single-Cell Regulatory Network Inference and Clustering. Nat. Methods 14, 1083–1086. doi:10.1038/nmeth.4463
Auguste, P., Fallavollita, L., Wang, N., Burnier, J., Bikfalvi, A., and Brodt, P. (2007). The Host Inflammatory Response Promotes Liver Metastasis by Increasing Tumor Cell Arrest and Extravasation. Am. J. Pathol. 170, 1781–1792. doi:10.2353/ajpath.2007.060886
Beg, A. A., and Baldwin, A. S. (1994). Activation of Multiple NF-Kappa B/Rel DNA-Binding Complexes by Tumor Necrosis Factor. Oncogene 9, 1487–1492.
Bellayr, I. H., Marklein, R. A., Lo Surdo, J. L., Bauer, S. R., and Puri, R. K. (2016). Identification of Predictive Gene Markers for Multipotent Stromal Cell Proliferation. Stem Cell Dev. 25, 861–873. doi:10.1089/scd.2015.0374
Bonelli, M., Dalwigk, K., Platzer, A., Olmos Calvo, I., Hayer, S., Niederreiter, B., et al. (2019). IRF1 Is Critical for the TNF-Driven Interferon Response in Rheumatoid Fibroblast-like Synoviocytes. Exp. Mol. Med. 51, 1–11. doi:10.1038/s12276-019-0267-6
Bozhokin, M. S., Sopova, Y. V., Kachkin, D. V., Rubel, A. A., and Khotin, M. G. (2020). Mechanisms of TGFβ3 Action as a Therapeutic Agent for Promoting the Synthesis of Extracellular Matrix Proteins in Hyaline Cartilage. Biochem. Mosc. 85, 436–447. doi:10.1134/s0006297920040045
Butler, A., Hoffman, P., Smibert, P., Papalexi, E., and Satija, R. (2018). Integrating Single-Cell Transcriptomic Data across Different Conditions, Technologies, and Species. Nat. Biotechnol. 36, 411–420. doi:10.1038/nbt.4096
Campbell, I. K., Gerondakis, S., O’Donnell, K., and Wicks, I. P. (2000). Distinct Roles for the NF-Κb1 (P50) and C-Rel Transcription Factors in Inflammatory Arthritis. J. Clin. Invest. 105, 1799–1806. doi:10.1172/jci8298
Chen, C., Li, C., Liu, W., Guo, F., Kou, X., Sun, S., et al. (2020). Estrogen-induced FOS-like 1 Regulates Matrix Metalloproteinase Expression and the Motility of Human Endometrial and Decidual Stromal Cells. J. Biol. Chem. 295, 2248–2258. doi:10.1074/jbc.RA119.010701
Chen, S., Fu, P., Wu, H., and Pei, M. (2017). Meniscus, Articular Cartilage and Nucleus Pulposus: a Comparative Review of Cartilage-like Tissues in Anatomy, Development and Function. Cell Tissue Res 370, 53–70. doi:10.1007/s00441-017-2613-0
Cucchiarini, M., Thurn, T., Weimer, A., Kohn, D., Terwilliger, E. F., and Madry, H. (2007). Restoration of the Extracellular Matrix in Human Osteoarthritic Articular Cartilage by Overexpression of the Transcription factorSOX9. Arthritis Rheum. 56, 158–167. doi:10.1002/art.22299
Dai, J., and Rabie, A. B. M. (2007). VEGF: an Essential Mediator of Both Angiogenesis and Endochondral Ossification. J. Dent Res. 86, 937–950. doi:10.1177/154405910708601006
Dura, B., Choi, J.-Y., Zhang, K., Damsky, W., Thakral, D., Bosenberg, M., et al. (2019). scFTD-Seq: Freeze-Thaw Lysis Based, Portable Approach toward Highly Distributed Single-Cell 3′ mRNA Profiling. Nucleic Acids Res. 47, e16. doi:10.1093/nar/gky1173
Efremova, M., Vento-Tormo, M., Teichmann, S. A., and Vento-Tormo, R. (2020). CellPhoneDB: Inferring Cell-Cell Communication from Combined Expression of Multi-Subunit Ligand-Receptor Complexes. Nat. Protoc. 15, 1484–1506. doi:10.1038/s41596-020-0292-x
Forero, A., Ozarkar, S., Li, H., Lee, C. H., Hemann, E. A., Nadjsombati, M. S., et al. (2019). Differential Activation of the Transcription Factor IRF1 Underlies the Distinct Immune Responses Elicited by Type I and Type III Interferons. Immunity 51, 451–464.e6. doi:10.1016/j.immuni.2019.07.007
Forrester, A., De Leonibus, C., Grumati, P., Fasana, E., Piemontese, M., Staiano, L., et al. (2019). A Selective ER ‐phagy Exerts Procollagen Quality Control via a Calnexin‐ FAM 134B Complex. Embo J. 38, e99847. doi:10.15252/embj.201899847
Gilson, A., Dreger, M., and Urban, J. P. (2010). Differential Expression Level of Cytokeratin 8 in Cells of the Bovine Nucleus Pulposus Complicates the Search for Specific Intervertebral Disc Cell Markers. Arthritis Res. Ther. 12, R24. doi:10.1186/ar2931
Humzah, M. D., and Soames, R. W. (1988). Human Intervertebral Disc: Structure and Function. Anat. Rec. 220, 337–356. doi:10.1002/ar.1092200402
Iatridis, J. C., Weidenbaum, M., Setton, L. A., and Mow, V. C. (1996). Is the Nucleus Pulposus a Solid or a Fluid? Mechanical Behaviors of the Nucleus Pulposus of the Human Intervertebral Disc. Spine 21, 1174–1184. doi:10.1097/00007632-199605150-00009
Ji, Q., Zheng, Y., Zhang, G., Hu, Y., Fan, X., Hou, Y., et al. (2019). Single-cell RNA-Seq Analysis Reveals the Progression of Human Osteoarthritis. Ann. Rheum. Dis. 78, 100–110. doi:10.1136/annrheumdis-2017-212863
Jomova, K., and Valko, M. (2011). Advances in Metal-Induced Oxidative Stress and Human Disease. Toxicology 283, 65–87. doi:10.1016/j.tox.2011.03.001
Kanfer, G., and Kornmann, B. (2016). Dynamics of the Mitochondrial Network during Mitosis. Biochem. Soc. Trans. 44 (2), 510–516. doi:10.1042/bst20150274
Kwon, W.-K., Moon, H. J., Kwon, T.-H., Park, Y.-K., and Kim, J. H. (2017). The Role of Hypoxia in Angiogenesis and Extracellular Matrix Regulation of Intervertebral Disc Cells during Inflammatory Reactions. Neurosurgery 81, 867–875. doi:10.1093/neuros/nyx149
Lawson, L., and Harfe, B. D. (2015). Notochord to Nucleus Pulposus Transition. Curr. Osteoporos. Rep. 13, 336–341. doi:10.1007/s11914-015-0284-x
Le Maitre, C. L., Pockert, A., Buttle, D. J., Freemont, A. J., and Hoyland, J. A. (2007). Matrix Synthesis and Degradation in Human Intervertebral Disc Degeneration. Biochem. Soc. Trans. 35, 652–655. doi:10.1042/bst0350652
Lee, Y.-J., Kong, M.-H., Song, K.-Y., Lee, K.-H., and Heo, S.-H. (2008). The Relation between Sox9, TGF-Β1, and Proteoglycan in Human Intervertebral Disc Cells. J. Korean Neurosurg. Soc. 43, 149–154. doi:10.3340/jkns.2008.43.3.149
Lefebvre, V., and Dvir-Ginzberg, M. (2017). SOX9 and the many Facets of its Regulation in the Chondrocyte Lineage. Connect. Tissue Res. 58, 2–14. doi:10.1080/03008207.2016.1183667
Li, Z., Chen, X., Xu, D., Li, S., Chan, M. T. V., and Wu, W. K. K. (2019). Circular RNAs in Nucleus Pulposus Cell Function and Intervertebral Disc Degeneration. Cell Prolif 52, e12704. doi:10.1111/cpr.12704
Liao, Y., Smyth, G. K., and Shi, W. (2014). featureCounts: an Efficient General Purpose Program for Assigning Sequence Reads to Genomic Features. Bioinformatics 30 (7), 923–930. doi:10.1093/bioinformatics/btt656
Lin, W., Xu, D., Austin, C. D., Caplazi, P., Senger, K., Sun, Y., et al. (2019). Function of CSF1 and IL34 in Macrophage Homeostasis, Inflammation, and Cancer. Front. Immunol. 10, 2019. doi:10.3389/fimmu.2019.02019
Loboda, A., Damulewicz, M., Pyza, E., Jozkowicz, A., and Dulak, J. (2016). Role of Nrf2/HO-1 System in Development, Oxidative Stress Response and Diseases: an Evolutionarily Conserved Mechanism. Cell. Mol. Life Sci. 73, 3221–3247. doi:10.1007/s00018-016-2223-0
Mathew, S. J., Hansen, J. M., Merrell, A. J., Murphy, M. M., Lawson, J. A., Hutcheson, D. A., et al. (2011). Connective Tissue Fibroblasts and Tcf4 Regulate Myogenesis. Development. 138, 371–384. doi:10.1242/dev.057463
Mondal, D., Mathur, A., and Chandra, P. K. (2016). Tripping on TRIB3 at the junction of Health, Metabolic Dysfunction and Cancer. Biochimie 124, 34–52. doi:10.1016/j.biochi.2016.02.005
Nakazawa, K. R., Walter, B. A., Laudier, D. M., Krishnamoorthy, D., Mosley, G. E., Spiller, K. L., et al. (2018). Accumulation and Localization of Macrophage Phenotypes with Human Intervertebral Disc Degeneration. Spine J. 18, 343–356. doi:10.1016/j.spinee.2017.09.018
Nielsen, C. F., Zhang, T., Barisic, M., Kalitsis, P., and Hudson, D. F. (2020). Topoisomerase IIα Is Essential for Maintenance of Mitotic Chromosome Structure. Proc. Natl. Acad. Sci. USA 117, 12131–12142. doi:10.1073/pnas.2001760117
O’Brien, M. J., Shu, Q., Stinson, W. A., Tsou, P.-S., Ruth, J. H., Isozaki, T., et al. (2018). A Unique Role for Galectin-9 in Angiogenesis and Inflammatory Arthritis. Arthritis Res. Ther. 20, 31. doi:10.1186/s13075-018-1519-x
Pellicelli, M., Picard, C., Wang, D., Lavigne, P., and Moreau, A. (2016). E2F1 and TFDP1 Regulate PITX1 Expression in Normal and Osteoarthritic Articular Chondrocytes. PloS one 11, e0165951. doi:10.1371/journal.pone.0165951
Pfirrmann, C. W. A., Metzdorf, A., Zanetti, M., Hodler, J., and Boos, N. (2001). Magnetic Resonance Classification of Lumbar Intervertebral Disc Degeneration. Spine 26 (17), 1873–1878. doi:10.1097/00007632-200109010-00011
Potter, S. S. (2018). Single-cell RNA Sequencing for the Study of Development, Physiology and Disease. Nat. Rev. Nephrol. 14, 479–492. doi:10.1038/s41581-018-0021-7
Qiao, H., Di Stefano, L., Tian, C., Li, Y.-Y., Yin, Y.-H., Qian, X.-P., et al. (2007). Human TFDP3, a Novel DP Protein, Inhibits DNA Binding and Transactivation by E2F. J. Biol. Chem. 282, 454–466. doi:10.1074/jbc.M606169200
Qiu, X., Hill, A., Packer, J., Lin, D., Ma, Y.-A., and Trapnell, C. (2017). Single-cell mRNA Quantification and Differential Analysis with Census. Nat. Methods 14, 309–315. doi:10.1038/nmeth.4150
Ramasamy, S. K., Kusumbe, A. P., Wang, L., and Adams, R. H. (2014). Endothelial Notch Activity Promotes Angiogenesis and Osteogenesis in Bone. Nature 507, 376–380. doi:10.1038/nature13146
Reeves, S. R., Kolstad, T., Lien, T.-Y., Elliott, M., Ziegler, S. F., Wight, T. N., et al. (2014). Asthmatic Airway Epithelial Cells Differentially Regulate Fibroblast Expression of Extracellular Matrix Components. J. Allergy Clin. Immunol. 134, 663–670.e1. doi:10.1016/j.jaci.2014.04.007
Richardson, S. M., Kalamegam, G., Pushparaj, P. N., Matta, C., Memic, A., Khademhosseini, A., et al. (2016). Mesenchymal Stem Cells in Regenerative Medicine: Focus on Articular Cartilage and Intervertebral Disc Regeneration. Methods 99, 69–80. doi:10.1016/j.ymeth.2015.09.015
Risbud, M. V., Guttapalli, A., Tsai, T.-T., Lee, J. Y., Danielson, K. G., Vaccaro, A. R., et al. (2007). Evidence for Skeletal Progenitor Cells in the Degenerate Human Intervertebral Disc. Spine 32, 2537–2544. doi:10.1097/BRS.0b013e318158dea6
Risbud, M. V., and Shapiro, I. M. (2014). Role of Cytokines in Intervertebral Disc Degeneration: Pain and Disc Content. Nat. Rev. Rheumatol. 10, 44–56. doi:10.1038/nrrheum.2013.160
Safiri, S., Kolahi, A. A., Cross, M., Hill, C., Smith, E., Carson‐Chahhoud, K., et al. (2021). Prevalence, Deaths, and Disability‐Adjusted Life Years Due to Musculoskeletal Disorders for 195 Countries and Territories 1990-2017. Arthritis Rheumatol. 73, 702–714. doi:10.1002/art.41571
Sato, W., Kadomatsu, K., Yuzawa, Y., Muramatsu, H., Hotta, N., Matsuo, S., et al. (2001). Midkine Is Involved in Neutrophil Infiltration into the Tubulointerstitium in Ischemic Renal Injury. J. Immunol. 167, 3463–3469. doi:10.4049/jimmunol.167.6.3463
Semple, B. D., Kossmann, T., and Morganti-Kossmann, M. C. (2010). Role of Chemokines in CNS Health and Pathology: a Focus on the CCL2/CCR2 and CXCL8/CXCR2 Networks. J. Cereb. Blood Flow Metab. 30, 459–473. doi:10.1038/jcbfm.2009.240
Shaath, H., Vishnubalaji, R., Elkord, E., and Alajez, N. M. (2020). Single-Cell Transcriptome Analysis Highlights a Role for Neutrophils and Inflammatory Macrophages in the Pathogenesis of Severe COVID-19. Cells 9, 2374. doi:10.3390/cells9112374
Simons, M. (2004). Integrative Signaling in Angiogenesis. Mol. Cel Biochem 264, 99–102. doi:10.1023/b:mcbi.0000044379.25823.03
Stosiek, P., Kasper, M., and Karsten, U. (1988). Expression of Cytokeratin and Vimentin in Nucleus Pulposus Cells. Differentiation 39, 78–81. doi:10.1111/j.1432-0436.1988.tb00083.x
Tang, W., Lu, Y., Tian, Q.-Y., Zhang, Y., Guo, F.-J., Liu, G.-Y., et al. (2011). The Growth Factor Progranulin Binds to TNF Receptors and Is Therapeutic against Inflammatory Arthritis in Mice. Science 332, 478–484. doi:10.1126/science.1199214
Teraguchi, M., Yoshimura, N., Hashizume, H., Muraki, S., Yamada, H., Minamide, A., et al. (2014). Prevalence and Distribution of Intervertebral Disc Degeneration over the Entire Spine in a Population-Based Cohort: the Wakayama Spine Study. Osteoarthritis and Cartilage 22, 104–110. doi:10.1016/j.joca.2013.10.019
Tsingas, M., Ottone, O. K., Haseeb, A., Barve, R. A., Shapiro, I. M., Lefebvre, V., et al. (2020). Sox9 Deletion Causes Severe Intervertebral Disc Degeneration Characterized by Apoptosis, Matrix Remodeling, and Compartment-specific Transcriptomic Changes. Matrix Biol. 94, 110–133. doi:10.1016/j.matbio.2020.09.003
Urban, J. P. G., Smith, S., and Fairbank, J. C. T. (2004). Nutrition of the Intervertebral Disc. Spine 29, 2700–2709. doi:10.1097/01.brs.0000146499.97948.52
Vo, N. V., Hartman, R. A., Yurube, T., Jacobs, L. J., Sowa, G. A., and Kang, J. D. (2013). Expression and Regulation of Metalloproteinases and Their Inhibitors in Intervertebral Disc Aging and Degeneration. Spine J. 13, 331–341. doi:10.1016/j.spinee.2012.02.027
Wagner, E. F. (2001). AP-1 - Introductory Remarks. Oncogene 20 (19), 2334–2335. doi:10.1038/sj.onc.1204416
Wallach, D., Varfolomeev, E. E., Malinin, N. L., Goltsev, Y. V., Kovalenko, A. V., and Boldin, M. P. (1999). Tumor Necrosis Factor Receptor and Fas Signaling Mechanisms. Annu. Rev. Immunol. 17, 331–367. doi:10.1146/annurev.immunol.17.1.331
Wang, C., Li, Z., Shao, F., Yang, X., Feng, X., Shi, S., et al. (2017). High Expression of Collagen Triple Helix Repeat Containing 1 (CTHRC1) Facilitates Progression of Oesophageal Squamous Cell Carcinoma through MAPK/MEK/ERK/FRA-1 Activation. J. Exp. Clin. Cancer Res. 36, 84. doi:10.1186/s13046-017-0555-8
Wang, K., Chen, X., Chen, Y., Sheng, S., and Huang, Z. (2020). Grape Seed Procyanidins Suppress the Apoptosis and Senescence of Chondrocytes and Ameliorates Osteoarthritis via the DPP4-Sirt1 Pathway. Food Funct. 11, 10493–10505. doi:10.1039/d0fo01377c
Weiler, C., Nerlich, A. G., Schaaf, R., Bachmeier, B. E., Wuertz, K., and Boos, N. (2010). Immunohistochemical Identification of Notochordal Markers in Cells in the Aging Human Lumbar Intervertebral Disc. Eur. Spine J. 19, 1761–1770. doi:10.1007/s00586-010-1392-z
Wheatley, S. P., and Altieri, D. C. (2019). Survivin at a Glance. J. Cel Sci. 132, jcs223826. doi:10.1242/jcs.223826
Wu, J., Lu, L.-Y., and Yu, X. (2010). The Role of BRCA1 in DNA Damage Response. Protein Cell 1, 117–123. doi:10.1007/s13238-010-0010-5
Xie, B., Liu, Y., Zhao, Z., Liu, Q., Wang, X., Xie, Y., et al. (2020). MYB Proto-oncogene-like 1-TWIST1 Axis Promotes Growth and Metastasis of Hepatocellular Carcinoma Cells. Mol. Ther. - Oncolytics 18, 58–69. doi:10.1016/j.omto.2020.05.016
Yan, Z., Pan, Y., Wang, S., Cheng, M., Kong, H., Sun, C., et al. (2017). Static Compression Induces ECM Remodeling and Integrin α2β1 Expression and Signaling in a Rat Tail Caudal Intervertebral Disc Degeneration Model. Spine 42, E448–E458. doi:10.1097/brs.0000000000001856
Yu, G., Wang, L.-G., Han, Y., and He, Q.-Y. (2012). ClusterProfiler: an R Package for Comparing Biological Themes Among Gene Clusters. OMICS. 16, 284–287. doi:10.1089/omi.2011.0118
Zhang, Y., Han, S., Kong, M., Tu, Q., Zhang, L., and Ma, X. (2021). Single-cell RNA-Seq Analysis Identifies Unique Chondrocyte Subsets and Reveals Involvement of Ferroptosis in Human Intervertebral Disc Degeneration. Osteoarthritis and Cartilage. 29, 1324–1334. doi:10.1016/j.joca.2021.06.010
Keywords: single-cell RNA sequencing, intervertebral disc degeneration, nucleus pulposus, chondrocyte, differentiation and regulation
Citation: Han S, Zhang Y, Zhang X, Zhang H, Meng S, Kong M, Liu X and Ma X (2022) Single-Cell RNA Sequencing of the Nucleus Pulposus Reveals Chondrocyte Differentiation and Regulation in Intervertebral Disc Degeneration. Front. Cell Dev. Biol. 10:824771. doi: 10.3389/fcell.2022.824771
Received: 29 November 2021; Accepted: 10 January 2022;
Published: 21 February 2022.
Edited by:
Lei Ma, Third Hospital of Hebei Medical University, ChinaReviewed by:
Xiaobin Wang, The Second Xiangya Hospital of Central South University, ChinaZengwu Shao, Huazhong University of Science and Technology, China
Copyright © 2022 Han, Zhang, Zhang, Zhang, Meng, Kong, Liu and Ma. This is an open-access article distributed under the terms of the Creative Commons Attribution License (CC BY). The use, distribution or reproduction in other forums is permitted, provided the original author(s) and the copyright owner(s) are credited and that the original publication in this journal is cited, in accordance with accepted academic practice. No use, distribution or reproduction is permitted which does not comply with these terms.
*Correspondence: Xuexiao Ma, bWF4dWV4aWFvc3BpbmFsQDE2My5jb20=
†These authors have contributed equally to this work and share first authorship