- 1Hu Sheep Academy, Nanjing Agricultural University, Nanjing, China
- 2Jiangsu Livestock Embryo Engineering Laboratory, Nanjing Agricultural University, Nanjing, China
- 3Taicang Agricultural and Rural Science and Technology Service Center, and Graduate Workstation, Taicang, China
- 4Animal Production Research Institute, ARC, Ministry of Agriculture, Giza, Egypt
DNA methylation plays an important role in biological processes by affecting gene expression. However, how DNA methylation regulates phenotypic variation in Hu sheep remains unclear. Therefore, we generated genome-wide DNA methylation and transcriptomic profiles in the ovaries of Hu sheep with different prolificacies and genotypes (FecBB and FecB+). Results showed that ovary DNA methylome and transcriptome were significantly different between high prolificacy and low prolificacy Hu sheep. Comparative methylome analyses identified 10,644, 9,594, and 12,214 differentially methylated regions and 87, 1,121, and 2,375 genes, respectively, showing differential expression levels in three different comparison groups. Female reproduction-associated differentially methylated regions-related genes and differentially expressed genes were enriched, thereby the respective interaction networks were constructed. Furthermore, systematical integrative analyses revealed a negative correlation between DNA methylation around the transcriptional start site and gene expression levels, which was confirmed by testing the expression of integrin β2 subunit (ITGB2) and lysosome-associated protein transmembrane-4 beta (LAPTM4B) in vivo and in vitro. These findings demonstrated that DNA methylation influences the propensity for prolificacy by affecting gene expression in the ovaries, which may contribute to a greater understanding of the epigenome and transcriptome that will be useful for animal breeding.
Introduction
Litter size is one of most important traits that determines the fecundity and reproductive efficiency of sheep bred for meat. In China, most sheep species are monotocous and seasonal estrus, although a few are polytocous. Compared with other sheep, Hu sheep is an excellent local breed in China, which is known for its high prolificacy and year-round estrus (Yue, 1996). Therefore, determining the molecular mechanisms associated with fecundity will help accelerate the breeding process of sheep with high prolificacy. Although the existing genetic studies have identified several genes with sheep fecundity, including GDF9, BMP15, and BMPR1B, the underlying genetic mechanisms remain largely unexplored (Chu et al., 2007; Polley et al., 2010; Farhadi et al., 2013; Wang et al., 2015). FecB (mutation in BMPR1B) is one of the key genes associated with sheep prolificacy (Wang et al., 2015). Moreover, evidences revealed that sheep with the homozygous mutation (FecBB; BB) had the greater ovulation rates than those with the heterozygous mutation (FecB+; B+) or the wild-type genotype (++) (Fabre et al., 2006; Gootwine et al., 2008). Thus, Hu sheep with different prolificacies and genotypes (BB and B+) were selected as experimental subjects.
It is widely thought that ovarian dysfunction leads to infertility in mammals. One of the main functions of the ovaries is to produce mature oocytes and secrete reproductive hormones that are involved in the follicular development and ovulation. Therefore, studying the differences in regulatory mechanisms in the ovaries of sheep with different prolificacies may reveal the mechanisms behind the genetic regulation of little size traits.
DNA methylation is an epigenetic modification that regulates gene expression and serves as a key regulator of development (Fan et al., 2020; Yang et al., 2021), differentiation (Khavari et al., 2010), and other processes (Miyakuni et al., 2021). Recent studies have shifted their focus toward how DNA methylation regulates pubertal onset (Lomniczi et al., 2015; Yang et al., 2016; Yuan et al., 2019), estrus (An et al., 2018; Zhou et al., 2018), ovary (Wang et al., 2014; Yu et al., 2015; Sagvekar et al., 2019), oocyte (Mattern et al., 2017; Wei et al., 2019), and embryo development (Salilew-Wondim et al., 2015; Canovas et al., 2017), and reproductive diseases (Vazquez-Martinez et al., 2019; Cao et al., 2021; Zhong et al., 2021). In-depth studies pertaining on epigenetic modifications, such as DNA methylation, are facilitating improvement in animal breeding (Gonzalez-Recio et al., 2015). Several studies have reported the genome-wide methylation profiles associated with milk production-related phenotypes in dairy cows (Singh et al., 2012), and disease resistance in crops (Tirnaz and Batley, 2019), as well as litter size in goats and pigs (Hwang et al., 2017; Kang et al., 2021). Meanwhile, Miao et al. (2017) recently reported the DNA methylation patterns in the ovaries of Dorset sheep and Small Tail Han sheep. These studies have demonstrated that DNA methylation plays an important role in regulating fecundity.
DNA methylation and gene expression profiles have been previously studied in the ovaries of Hu sheep with different prolificacies (the BB genotype only) to elucidate the regulatory mechanisms involved in Hu sheep fecundity (Zhang et al., 2017; Feng et al., 2018). As an extension of this study, here we aimed to systematically investigate both genome-wide DNA methylation and gene expression profiles in the ovaries of Hu sheep with different prolificacies (high prolificacy, HP; low prolificacy, LP) and genotypes (BB and B+) by performing whole-genome bisulfite sequencing (WGBS) and RNA-sequencing (RNA-seq). Furthermore, an integrated analysis of DNA methylation and transcriptome was performed to reveal whether and how DNA methylation mediate prolificacy phenotypic variations by affecting gene expression.
Materials and Methods
Animals and Sample Collection
We previously reported the details of the procedures for handling experimental animals and sample collection (Yao et al., 2021), which were approved by the Institutional Animal Ethics Committee of the Nanjing Agricultural University (SYXK 2011-0036). In brief, we firstly chose twenty non-pregnant ewes (2, 3 years old) based on their litter size numbers of three records, categorizing them as HP ewes (litter size = 3, n = 4) and LP ewes (litter size = 1, n = 16). Meanwhile, BMPR1B polymorphism genotyping of those ewes was detected as described previously (Yao et al., 2021). Finally, nine ewes were selected and divided into HPBB (n = 3), LPBB (n = 3) and LPB+ (n = 3) groups. All ewes were slaughtered during estrus and the ovarian samples were immediately collected and stored at −80°C for WGBS and RNA-seq.
WGBS and RNA-seq
Genomic DNA and total RNA were extracted from ovarian tissue of the nine sheep using a Genomic DNA kit (Cat.#DP304-03,Tiangen, Beijing, China) and TRIzol reagent (Cat.# 15596-026; Invitrogen, Carlsbad, CA), respectively. The concentration and purity of isolated DNA and RNA were determined using NanDrop spectrophotometer (NanoDrop Technologies, Wilmington, DE, United States) and agarose gel electrophoresis. The preparation of nine genome-wide DNA methylation and transcriptome libraries and sequencing (WGBS and RNA-seq), respectively, were performed by Biomarker Technologies Corporation (Beijing, China). Notably, we previously reported the DNA methylation (Zhang et al., 2017) and mRNAs (Feng et al., 2018) profiles in ovaries of only HPBB and LPBB Hu sheep, and using the same described methods, we analyzed the WGBS and RNA-Seq data in this study, with some modifications. Briefly, the raw data were first filtered to remove low-quality reads, and the clean data were then aligned with the reference genome of sheep (Ovis aries v4.0).
Differential methylated regions (DMRs) were defined by the presence of at least three methylation sites in the region, and the difference in methylation levels was >0.2 (>0.3 for CG type) with P-value (Fisher’s) < 0.05. Differentially expressed (DE) mRNAs were identified with the false discovery rate (FDR) < 0.05 and absolute value of log2 (fold change, FC) >1. Primarily, DMGs were detected through mapping the DMRs to genes based on their genomic location. In this study, we defined the genomic region from −3000 bp to the transcription start site (TSS) as the promoter region and from the TSS to the transcriptional termination site (TTS) as the gene body region. To visualize the overlapping gene sets, we generated venn diagrams using Venn diagram online tool (http://bioinformatics.psb.ugent.be/webtools/Venn/).
Integrated Analysis
The correlation between gene expression and methylation was calculated using only differentially expressed genes (DEGs) and DMR-related genes (DMGs). For the global methylation around TSS regions (±2000 bp) and gene expression correlation analysis, the genes was divided into four groups according to their expression level: hightest, lowest, medium-high and medium-low. T visualize the relationship between methylation and gene expression, heatmaps were generated using the R package (heatmap).
Functional Enrichment Analysis
Gene Ontology (GO) enrichment analyses of DMGs and DEGs were implemented by the Wallenius non-central hyper-geometric distribution in the GOseq R packages (Young et al., 2010). Kyoto Encyclopedia of Genes and Genomes (KEGG) analysis of DMGs and DEGs were evaluated using KOBAS software (Mao et al., 2005).
Interaction Network Construction
The interaction network of DMGs and DEGs associated with female reproduction was analyzed using the STRING database (http://string-db.org/) and visualized with Cytoscape software (V3.4.0).
Bisulfite Sequencing PCR
For BSP analysis, genomic DNA of ovaries from each groups was modified with sodium bisulfite using an EZ DNA Methylation-Direct Kit (Cat.#D5020; Zymo Research, Irvine, CA, United States). The PCR products were purified using a gel extraction kit (Cat.#DC301-01; Vazyme, Nanjing, China), then ligated and cloned into the pMD19-T vector (Cat.#6013; Takara, Osaka, Japan). Subsequently, ten positive clones of each sample were randomly selected for DNA sequencing. Data were analyzed and visualized using BIQ Analyzer software. BSP primers are listed in Supplementary Table S1 and all operations were conducted according to the manufacturer’s instructions.
Cell Culture and Treatments
Hu sheep granulosa cells (GCs) were isolated from healthy follicles (2–5 mm) and cultured as our previously described (Yao et al., 2021). Briefly, GCs were seeded into different plates (6-well: 1 × 106 cells/well) in culture medium (Dulbecco’s modified Eagle’s medium/nutrient mixture F-12 supplemented with 10% fetal bovine serum, 2 mM L-glutamine, 100 IU/ml penicillin, and 100 μg/ml streptomycin) at 37°C and 5% CO2. When the cultured GCs attained 60–70% confluence, the culture medium was replaced with new medium containing various concentrations (0, 1, 2, 5, 10, and 20 μM) of 5-Aza-deoxycytidine (5-Aza; Cat.#a3656; Sigma-Aldrich, United States), as a DNA methyltransferase inhibitor, and incubated for 48 h.
ITGB2 (integrin β2 subunit) knockdown was achieved using siRNAs, which were synthesized by GenePharma (Shanghai, China) and the sequences listed in Supplementary Table S2. After cultured GCs to 60–70% confluence, siRNA-NC and siRNA-ITGB2 were transfected into GCs with or without 10 μM 5-Aza for 48 h. Subsequently, all treated cells were collected for further analysis. All reagents used in cell culture were purchased from Life Technologies (Pleasanton CA, United States).
Immunohistochemistry
Immunohistochemistry was performed following our previously described method (Yao et al., 2017). Rabbit anti-LAPTM4B (lysosome-associated protein transmembrane-4 beta; Cat.#18895 -1-AP; ProteinTech, Rosemont, IL, United States) were used as primary antibody. For negative control, the primary antibody was replaced with Tris-buffered saline. Digital images were examined using a light microscope (Nikon, Tokyo, Japan).
Quantitative Reverse Transcriptase PCR
For qRT-PCR analysis, cDNA was synthesized using reverse transcription reagent kit with gDNA wiper (Cat.#R323-01; Vazyme, Nanjing, China). Subsequently, qRT-PCR was performed on an ABI 7500 Real-Time PCR System (Applied BioSystems, Carlsbad, CA, United States) using SYBR Green Master Mix (Cat.#Q711-02; Vazyme, Nanjing, China). Relative mRNA expression levels were quantified using 2–ΔΔCT method, with Glyceraldehyde 3-phosphate dehydrogenase (GAPDH) as the internal control. Primers were designed using the Primer 5.0 software and listed in Supplementary Table S3.
Statistical Analysis
Data are presented as means ± SEM based on three independent experiments, with GraphPad Prism v7.0 (GraphPad Software, CA, United States). Statistical analyses were performed by the one-way analysis of variance followed by a post hoc test in the SPSS25.0 software package (Chicago, IL, United States). P-values < 0.05 were considered statistically significant.
Results
Overview of Genome-Wide DNA Methylation Profiling in the Ovaries of Hu Sheep
To determine the effects of DNA methylation on the phenotypic variation of prolificacy, WGBS analysis was performed in the ovaries of HPBB, LPBB and LPB + groups of Hu sheep. After data filtering, each sample had approximately 210 million clean reads. Of mapped reads, 74.26, 73.94, and 66.65% in the HPBB, LPBB and LPB + groups, respectively, were used for subsequent analysis, with an average of 3.58% of methylated cytosine sites (Supplementary Table S4).
Global DNA methylation profiles indicated three contexts, CG, CHH, and CHG (where H is A, C, or T), existed in the ovaries of Hu sheep. The genomic methylation proportions of CG, CHG, and CHH contexts was respectively 72.23, 0.50 and 0.50% in the LPB + group, 69.60, 0.63 and 0.63% in the LPBB group, and 70.77, 0.70 and 0.66% in the HPBB group (Figure 1A). Among the three methylated contexts (CG, CHG and CHH), the methylated CG context represented 95.55, 95.20 and 95.06% in the LPB+, LPBB and HPBB groups, respectively (Figure 1B). In addition, the methylated level of CG context was mainly between 90 and 100%, whereas the methylated levels of CHG and CHH contexts apparently exhibited a more uniform distribution range from 10 to 30% (Figure 1C).
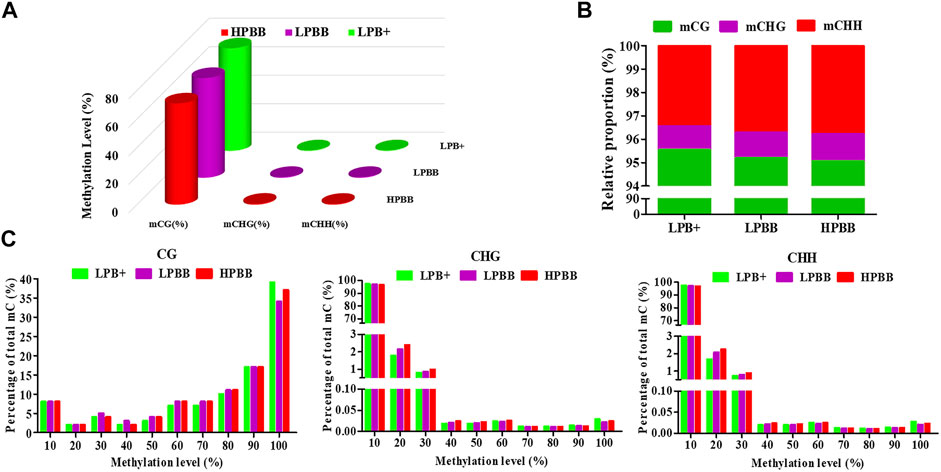
FIGURE 1. DNA methylation levels and distribution in ovaries of Hu sheep with different prolificacies. (A) Genomic methylation levels of CG, CHG, and CHH in different groups. (B) Relative average proportions of CG, CHG, and CHH methylation contexts in ovaries among different groups. (C) The average methylation percentage (y-axis) for different methylation levels (x-axis) of CG, CHG, and CHH methylation in different groups.
Comparison of DMRs in the Ovaries of Hu Sheep with Different Prolificacies
The Model-Based Analysis of Bisulfite Sequencing package was used to identify DMRs and compare the DNA methylation profiles between the different groups. Overall, 10,644 DMRs (6,281 hyper-methylated and 4,363 hypo-methylated; 10,634 CG, 1 CHG and 9 CHH), 9,594 DMRs (4,359 hyper-methylated and 5,235 hypo-methylated; 9,583 CG, 2 CHG and 9 CHH) and 12,214 DMRs (4,562 hyper-methylated and 7,652 hypo-methylated; 12,199 CG, 1 CHG and 14 CHH) were identified in the LPBB vs. HPBB, LPB + vs. HPBB and LPB + vs. LPBB groups, respectively (Figure 2A and Supplementary Tables S5–S7). Moreover, the distribution of DMRs were mainly located at intergenic and intron regions (Figures 2B–D).
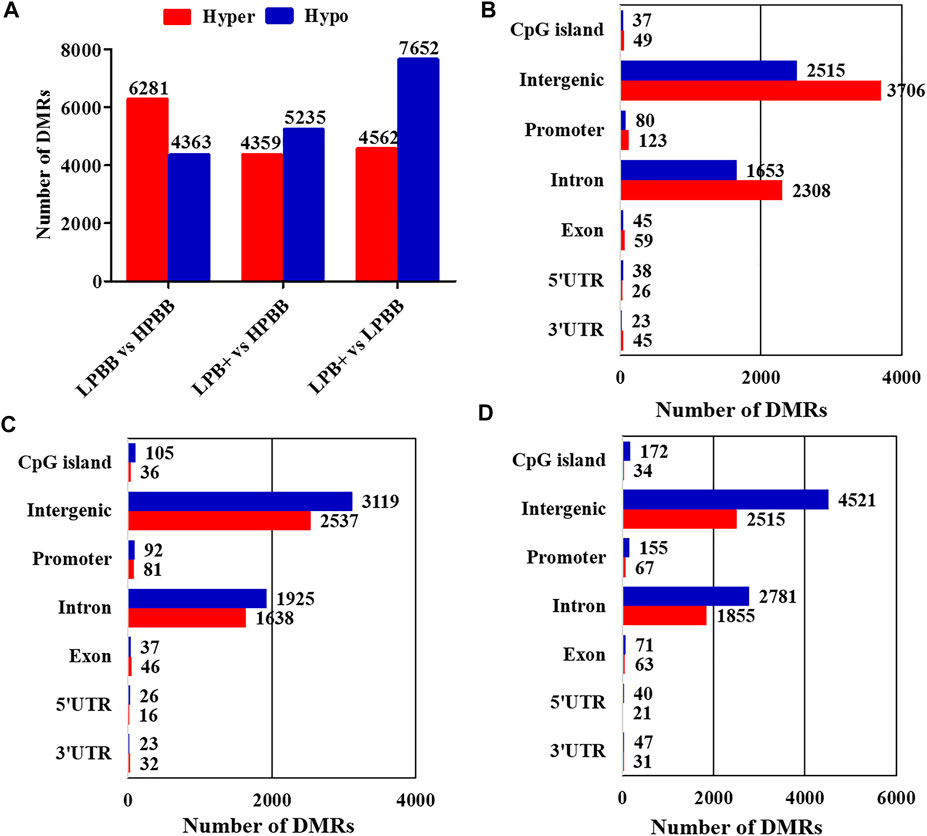
FIGURE 2. Identification of DMRs in different groups. (A) The number of DMRs (hyper- and hypo-methylated) in different comparison groups. (B,C) The distribution of DMRs in different genomic elements in the (B) LPBB vs. HPBB, (C) LPB + vs. HPBB, and (D) LPB + vs. LPBB groups.
Identification of DMGs and DEGs Among Different Comparison Groups
We identified 7,933 DMGs in the ovaries of the different comparison groups, of which 1,944 DMGs were common to all comparison groups (Figure 3A and Supplementary Table S8). Of these DMGs, 4,721 (3,393 hyper-methylated and 2,670 hypo-methylated), 4,426 (2,694 hyper-methylated and 2,951 hypo-methylated) and 5,152 (2,813 hyper-methylated and 3,812 hypo-methylated) were differentially methylated in the LPBB vs. HPBB, LPB + vs. HPBB and LPB + vs. LPBB groups, respectively (Figure 3B). Moreover, DMGs that exhibited DMRs in their promoter and gene body are shown in Figures 3C–E. The LPBB vs. HPBB, LPB + vs. HPBB and LPB + vs. LPBB groups contained 487, 404 and 528 genes, respectively, including both hyper- and hypo-methylated DMRs in their promoter and gene body.
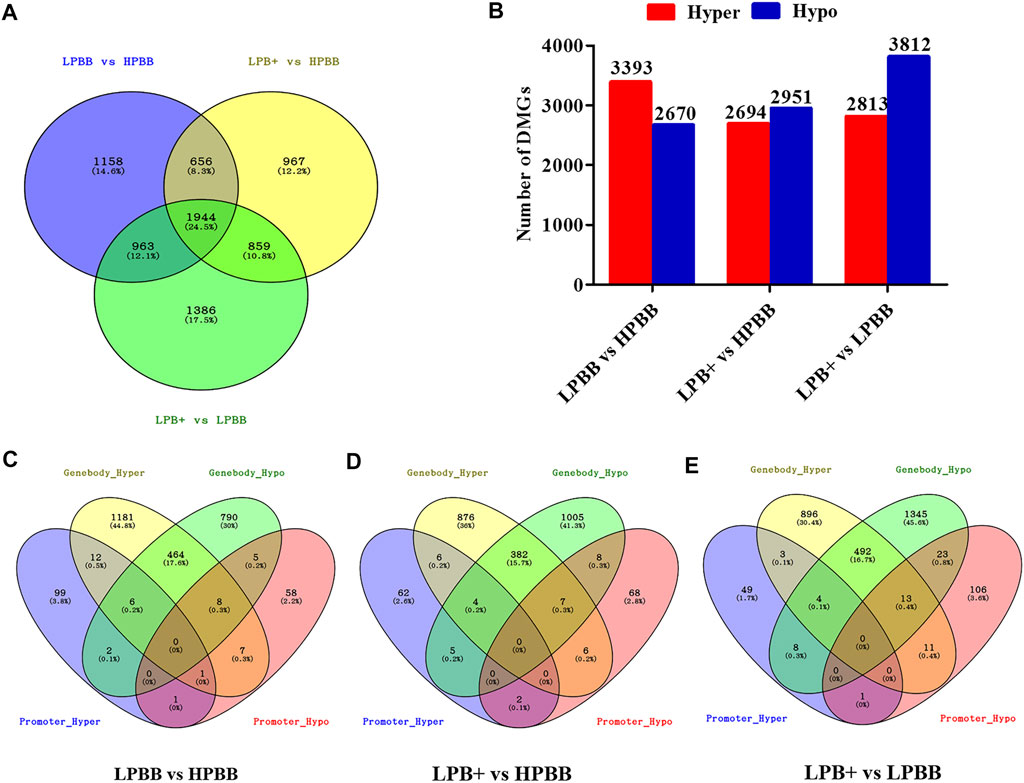
FIGURE 3. DMGs in the ovaries of different comparison groups. (A) DMGs that were unique or common among different comparison groups. (B) Number of hyper- and hypo-methylated genes in different comparison groups. (C–E) Venn diagram of the number of DMGs in promoter and gene body in the LPBB vs. HPBB, LPB + vs. HPBB, and LPB + vs. LPBB groups.
There were 87 (19 up-regulated and 68 down-regulated), 1,121 (662 up-regulated and 459 down-regulated) and 2,375 (1,563 up-regulated and 812 down-regulated) DE mRNAs identified in the LPBB vs. HPBB, LPB + vs. HPBB and LPB + vs. LPBB groups, respectively (Figures 4A–C). Hierarchical clustering of the DE mRNAs (Figures 4D–F) revealed the expression patterns of the individuals for each comparison.
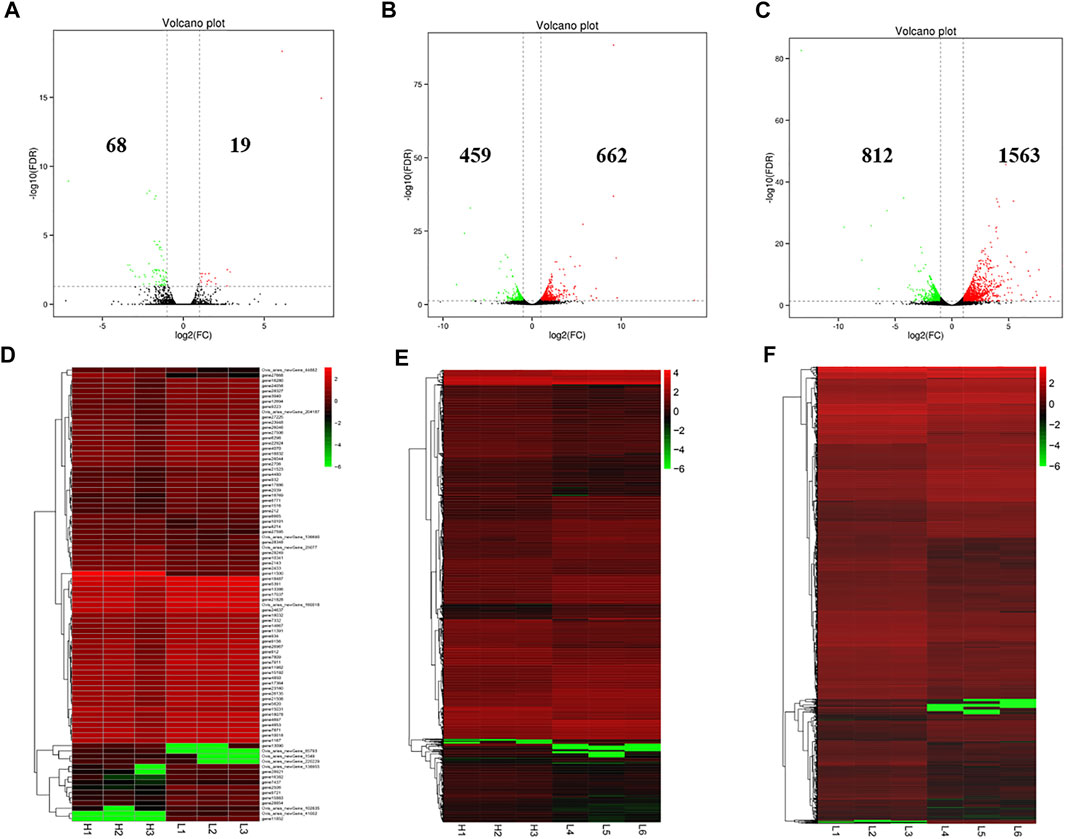
FIGURE 4. Identification of DE mRNAs. (A–C) Volcano plot of DE mRNAs in each group. Red indicates up-regulation and green indicates down-regulation. (D–F) Hierarchical clustering of DE mRNAs in each group. (A,D): LPBB vs. HPBB; (B and E): LPB + vs. HPBB; and (C,F): LPB + vs. LPBB groups. HPBB (H1, H2, H3); LPBB (L1, L2, L3); LPB+ (L4, L5, L6).
To confirm the reliability of the WGBS and RNA-seq data, four regions and seven genes were randomly selected for BSP and qRT-PCR, respectively. The results were consistent with the WGBS and RNA-seq data, suggesting that the WGBS and RNA-seq data were reliable for further study (Figure 5).
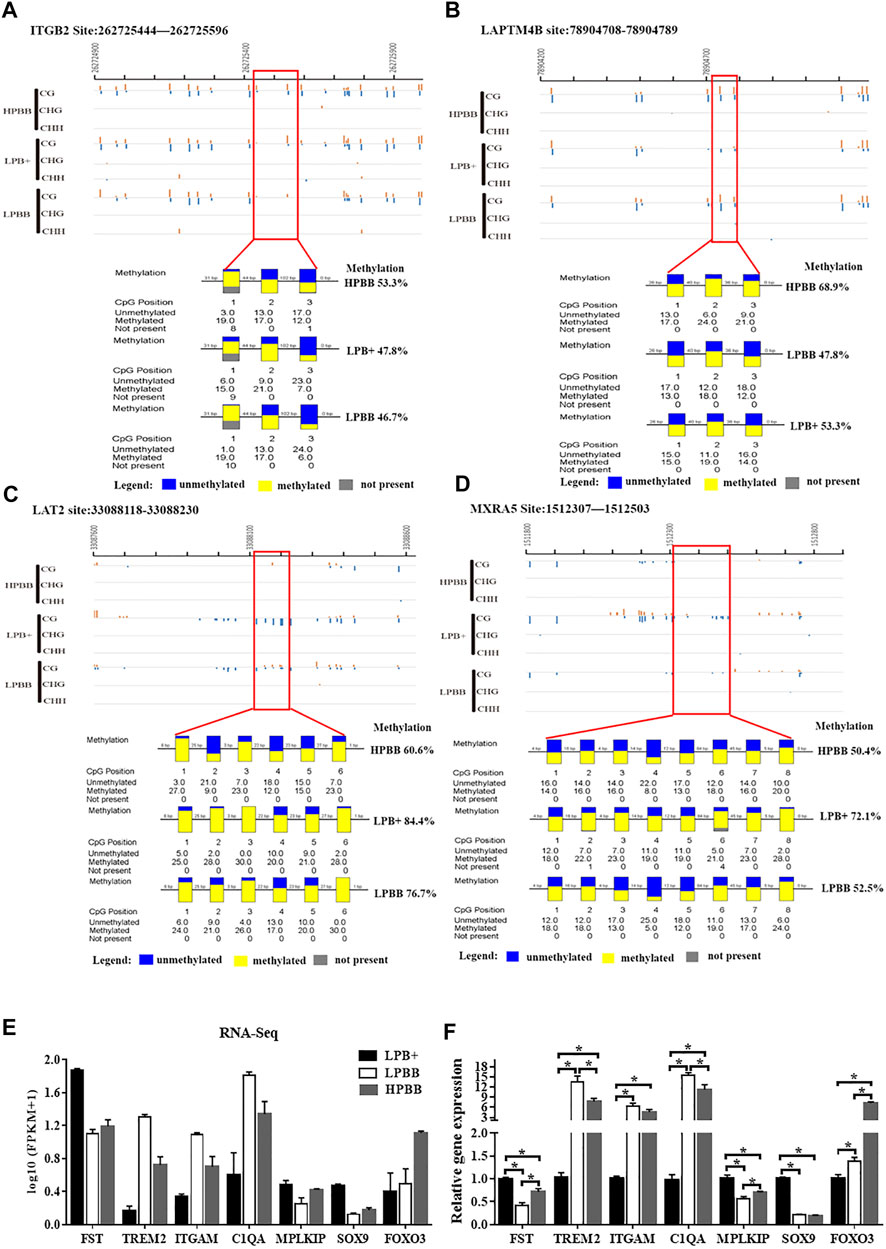
FIGURE 5. Validation of WGBS and RNA-seq data by BSP and qRT-PCR, respectively. (A,B) A DMR across the different groups in the promoter of the ITGB2 and LAPTM4B from 262725444 to 262725596 and from 78904708 to 78904789, respectively. (C) A DMR across the three groups in the intron of LAT2 from 33088118 to 33088230. (D) A DMR across the three groups in the distal intergenic region of MXRA5 from 1512307 to 1512503. Each box corresponds to one CpG position in the genomic sequence. Blue indicates unmethylated and yellow indicates methylated. (E) Seven mRNAs were randomly selected from the RNA-seq data. RNA-seq data are presented as log10 (FPKM+1). FPKM: Fragments Per Kilobase of transcript per Million fragments mapped. (F) Validation of the DEGs using qRT-PCR.
Functional Enrichment and Interaction Network Construction
As previously mentioned, the majority of methylated cytosines were of the CG type; thus, we focused on DMGs of methylated CG for functional enrichment analysis. GO and KEGG analyses were performed to evaluate the functions of DMGs and DEGs in the ovaries of Hu sheep with different prolificacies. Across all comparisons, the DMGs were significantly enriched in the TGF-β and Wnt signaling pathways (Supplementary Figure S1). Furthermore, we selected the female reproduction associated DMGs (Supplementary Tables S9–S11) and DEGs (Supplementary Tables S12–S14) for functional enrichment and the interaction networks construction for each comparison (Figure 6). Interestingly, most of DMGs from all the comparison groups were enriched in the ovarian follicle development/rupture, BMP signaling pathway and ovulation GO terms, as well as the Wnt and TGF-β signaling pathways among all comparison groups (Supplementary Figure S2). Specifically, INHBA, TGFBR2 and SMAD7 genes of the TGF-β pathway and SFRP1, FZD1 and MAP3K7 genes of the Wnt pathway were differentially methylated among all comparison groups.
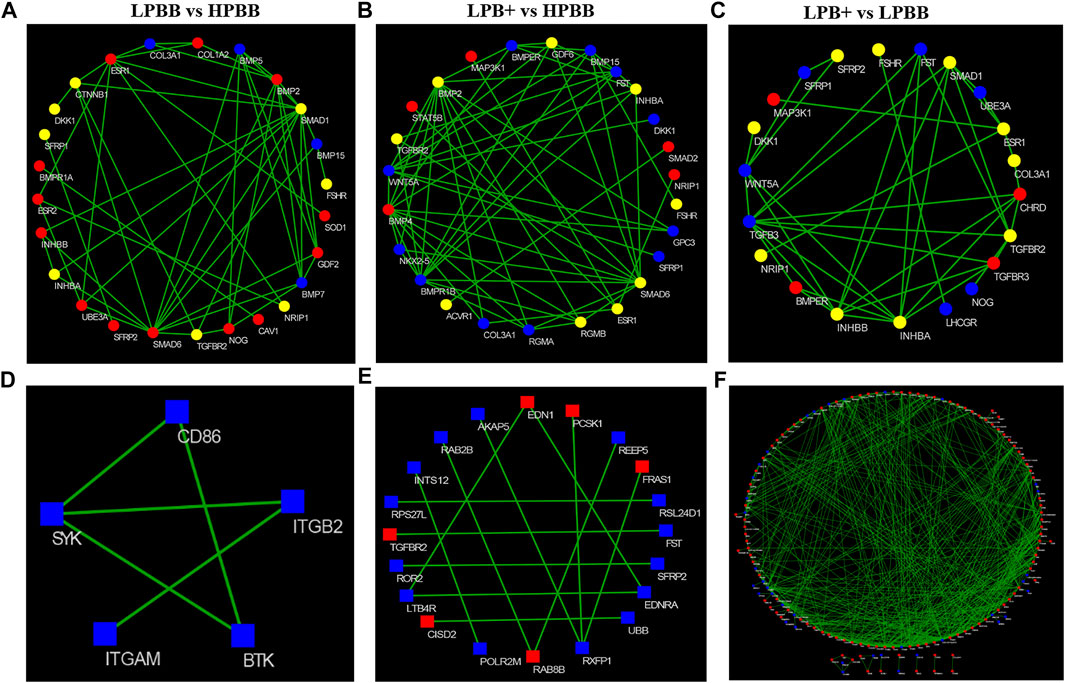
FIGURE 6. Construction of the network of DMGs and DEGs related to female reproduction. (A–C) Red and blue colors represent strong-hyper and strong-hypo, respectively. Yellow color represents the coexisting strong-hyper and strong-hypo. (D–F) Red and blue colors represent up- and down-regulated, respectively. (A,D) LPBB vs. HPBB; (B and E) LPB + vs. HPBB; and (C,F) LPB + vs. LPBB groups.
Similarly, the female reproduction associated DEGs from the LPBB vs. HPBB group were enriched in the hormone biosynthetic process and embryo implantation in GO terms (Supplementary Figure S3A), ae well as the ovarian steroidogenesis, PI3K-Akt and Rap1 signaling pathways (Supplementary Figure S3B). Meanwhile, female reproduction associated DEGs from both the LPB + vs. HPBB and LPB + vs. LPBB groups were enriched in the female gonad and ovarian follicle development GO terms (Supplementary Figures S3C,E), as well as the ovarian steroidogenesis, PI3K-Akt, TGF-β and Wnt signaling pathways (Supplementary Figures S3D,F).
Correlation Analysis Between DNA Methylation and Gene Expression
As shown in Figures 7A–C, a significant negative correlation was observed between DNA methylation level around the TSS and gene expression. In the LPBB vs. HPBB, LPB + vs. HPBB and LPB + vs. LPBB groups, we found that 13, 195 and 530 of the DMGs, respectively, associated with DEGs (Figures 7D–F and Supplementary Tables S15–S17). Moreover, the heatmap was constructed to visualize the relationship between DMGs and DEGs in the different comparison groups (Figures 7G–I). Many DMGs contained more than one DMR. For example, five DMRs in NDST4 were hyper-methylated and one DMR in NDST4 was hypo-methylated in the HPBB group compared to that in the LPBB group. In the LPBB vs. HPBB group, we identified 10 hyper-methylated genes with down-regulated expression levels in the HPBB group, including genes related to the Hippo (ITGB2), PI3K-Akt (SYK) and Rap1 (ITGB2) signaling pathways, while three genes were hypo-methylated and up-regulated in the HPBB group compared to that in the LPBB group (Figure 7G and Supplementary Table S15). In the LPB + vs. HPBB group, 77 genes were hyper-methylated and down-regulated in the HPBB group, and these genes were related to the regulation of the TGF-β (ID2), estrogen (LOC101114987) and oocyte meiosis (LOC101112318 and PTTG1) signaling pathways. In contrast, 49 genes were hyper-methylated and down-regulated in the LPB + group, and these genes were related to the TGF-β (TGFBR2), PI3K-Akt (COL4A1, LOC101115805 and COL24A1), FoxO (TGFBR2 and FOXO3) and insulin (CBLB, PDE3A and RIMS2) signaling pathways (Figure 7H and Supplementary Table S16). In the LPB + vs. LPBB group, 153 genes were hyper-methylated and down-regulated in the LPBB group, and these genes were related to the PI3K-Akt (PRKAA2, FGF10, FGF12 and LOC101103187), TGF-β (ID2) and ovarian steroidogenesis (FSHR) pathways. We found that 201 genes were hypo-methylated and up-regulated in the LPBB group compared to that in the LPB + group, and these genes were related to the PI3K-Akt (COL24A1, TLR4, SYK, ITGAV, ITGA7, VWF, RELN, FLT4, KIT, SGK1, THBS2, COL4A1 and LOC101115805), TGF-β (CDKN2B and TGFBR2) and ovarian steroidogenesis (STAR and PRKX) pathways (Figure 7I and Supplementary Table S17). In addition, some genes exhibited coinciding methylation and expression patterns in three comparison groups (Figures 7G–I and Supplementary Tables S15–S17). Interestingly, we found that ITGB2 and LAPTM4B genes, which were hyper-methylated (DMRs in their promoter) and down-regulated in LPBB vs. HPBB group and both LPB + vs. HPBB and LPB + vs. LPBB group, respectively, to confirm the negative correlation between DNA methylation and gene expression.
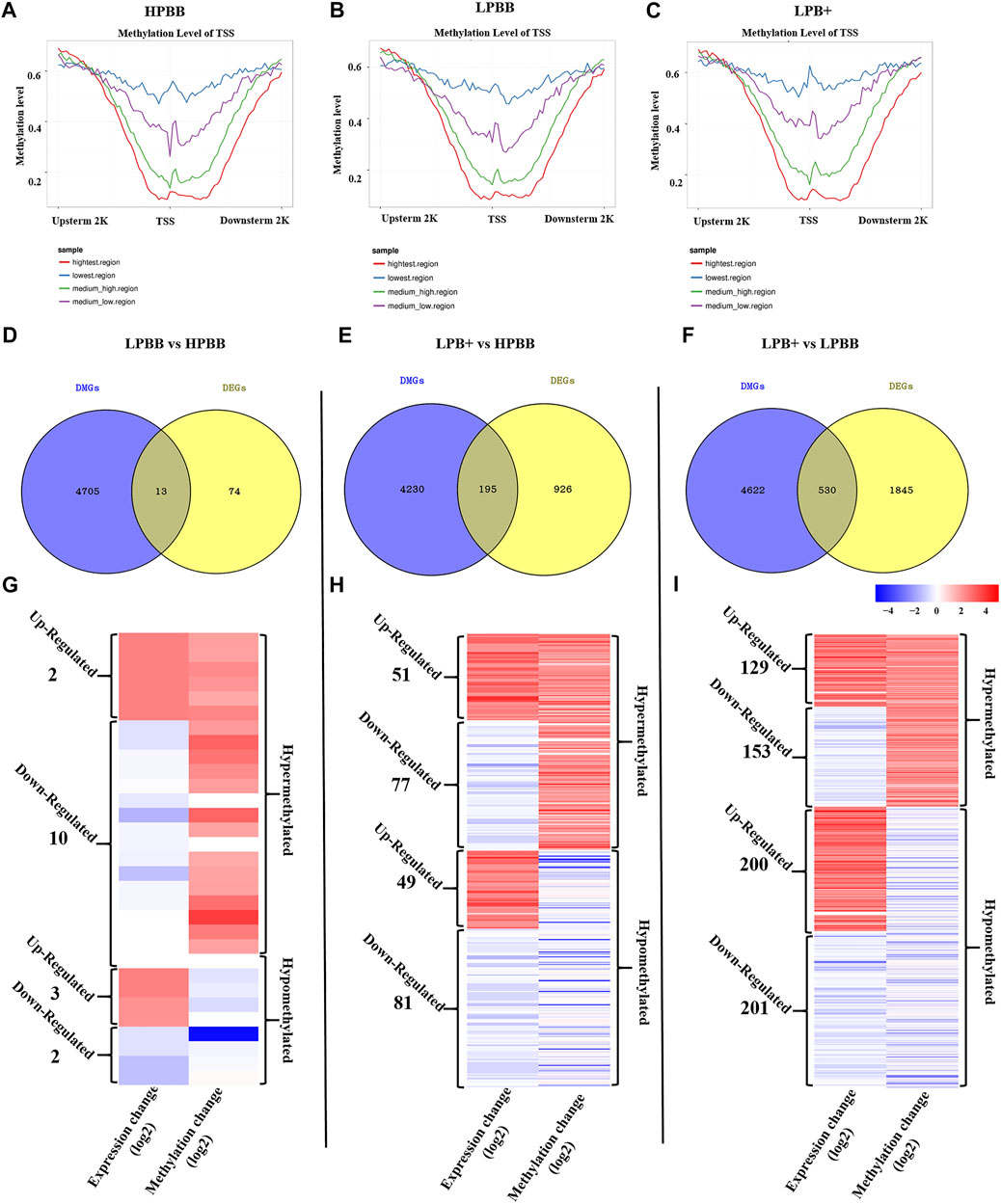
FIGURE 7. Integrative analysis of the DNA methylome and transcriptome in the ovaries of different groups. (A–C) DNA methylation level distribution around TSS of four levels of gene expression in the different groups. (D–F) Venn diagram shows the common DMGs and DEGs. (G–I) Heatmap showing the differentially methylated levels and corresponding genes change in the different comparison groups.
Integrative Analysis of ITGB2 and LAPTM4B Methylation and Expression
ITGB2 mRNA expression level in the LPBB group was significantly higher than that in the LPB+ and HPBB groups; with the expression being higher in the HPBB group than in the LPB + group (Figure 8A). LAPTM4B mRNA expression level in the LPB + group was significantly higher than that in the LPBB and HPBB groups (Figure 8B). These results were consistent with the RNA-seq data. Meanwhile, LAPTM4B protein was predominantly localized in the GCs of the antral follicle (Figure 8C).
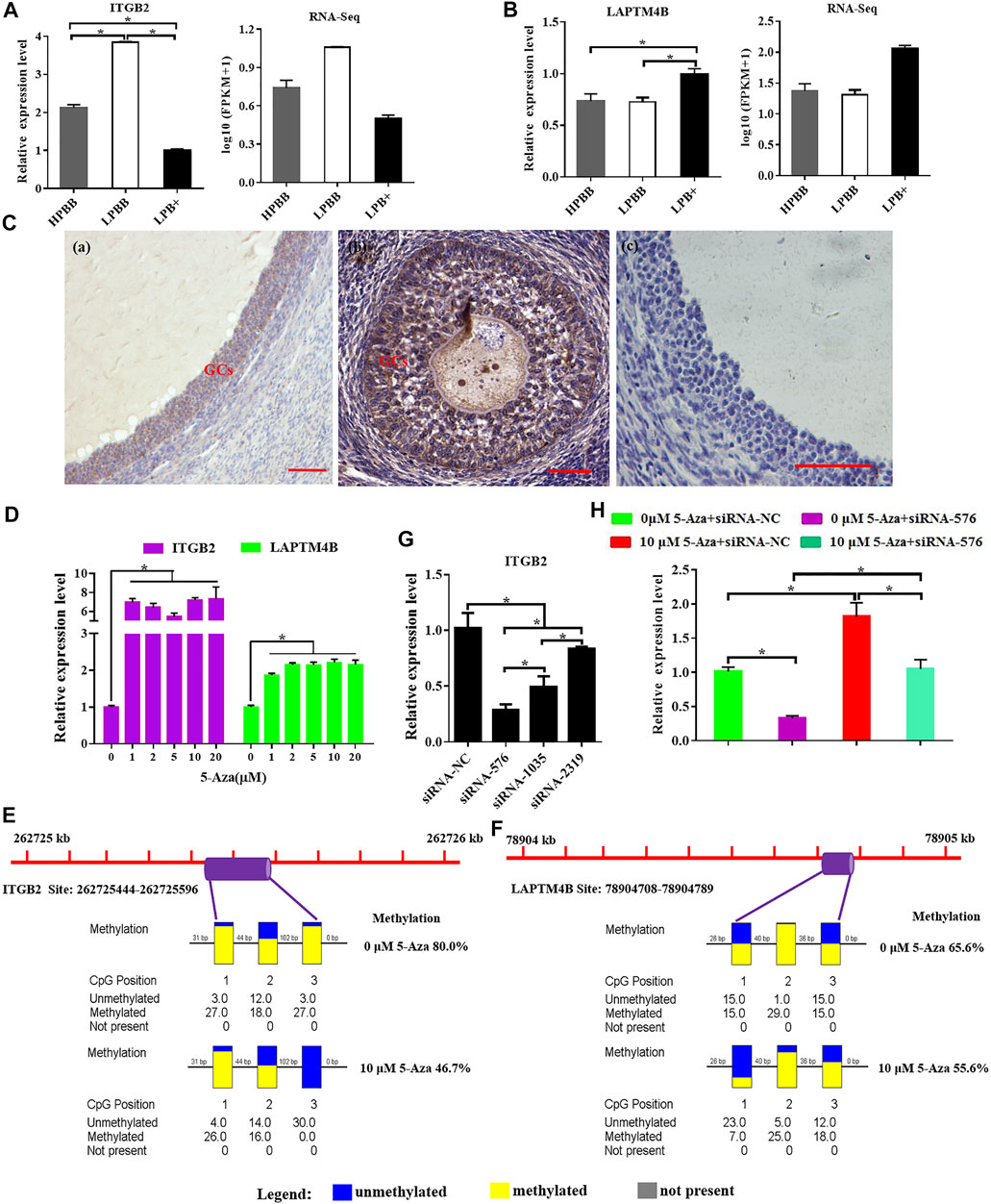
FIGURE 8. Integrative analysis of methylation and expression levels of ITGB2 and LAPTM4B. (A,B) ITGB2 and LAPTM4B mRNA expression in the ovaries were detected by qRT-PCR. (C) Localization of LAPTM4B in the ovaries using immunohistochemistry. Scale bars = 50 μm. (D) ITGB2 and LAPTM4B mRNA expression levels in cultured GCs were detected after treatment with various concentrations (0, 1, 2, 5, 10, and 20 μM) of 5-Aza treatment. (E,F) Methylation levels of ITGB2 and LAPTM4B in cultured GCs after 10 μM 5-Aza treatment. (G) Suppression efficiency of ITGB2 was evaluated by qRT-PCR. (H) mRNA level of ITGB2 in cultured GCs stimulated by 5-Aza with or without siRNA-ITGB2.
Next, we evaluated the effect of 5-Aza on ITGB2 and LAPTM4B expression in cultured GCs, and the results showed that ITGB2 and LAPTM4B mRNA expression were significantly higher in the 5-Aza treatment group (Figure 8D). Moreover, following the treatment of GCs with 5-Aza, the methylation level of the LAPTM4B and ITGB2 promoter decreased compared to that of the control cells (Figures 8E,F). Additionally, the mRNA expression of ITGB2 was significantly decreased using siRNA-specific ITGB2 (Figure 8G), subsequently, we demonstrated that the treatment of 5-Aza inhibited ITGB2-specific siRNA-reduced ITGB2 expression in GCs (Figure 8H).
Discussion
Fecundity is an economically important trait in the sheep industry. Therefore, investigating the molecular mechanisms of sheep fecundity may assist in accelerating the breeding process. Herein, we systematically investigated the genome-wide DNA methylation and gene expression profiles in the ovaries of Hu sheep with different prolificacies and genotypes by WGBS and RNA-seq, respectively. Although it has been demonstrated that the homozygous mutation (BB) had the higher fecundity than the heterozygous mutation (B+) or wild-type (++), significant differences in prolificacy phenotypes were found in the same genotype (BB) of Hu sheep with under the same feeding conditions. Therefore, we conducted an integrated analysis of DNA methylation and gene expression patterns was performed to reveal the manner in which DNA methylation may regulate prolificacy by affecting gene expression.
WGBS revealed that approximately 3.58% of cytosine sites were methylated in the ovaries of Hu sheep, with the highest proportion of CG methylation context. This finding is corroborated by previous reports in other animals (Yuan et al., 2016; Hwang et al., 2017; Fan et al., 2020). Moreover, the DNA methylation of the CG context from the ovaries of Hu sheep exhibited significant hyper-methylation levels (90–100%), but CHH and CHG exhibited hypo-methylation levels (10–30%), which is consistent with the results of a previous study in the ovaries of pigs (Yuan et al., 2016). These results suggest that the CG methylation was high-efficiently maintained in the ovaries of Hu sheep.
The majority of DMRs in the ovaries of Hu sheep exhibit a similar distribution to that observed in the ovaries or muscle of pigs and Hu sheep (Hwang et al., 2017; Yang et al., 2017; Fan et al., 2020), with the mainly located at the intergenic and intron elements. Accordingly, we identified numerous DMGs among the three comparison groups. As described above, focusing on the CG methylation context of DMGs, and we found that the common DMGs were significantly enriched in the TGF-β and Wnt signaling pathways, which have been confirmed to be involved in fecundity (Chu et al., 2007; Gao et al., 2020). For example, INHBA is associated with follicle development (Cui et al., 2020; Li et al., 2021), oocyte maturation (Tesfaye et al., 2009) and fecundity (Hiendleder et al., 1996; Yu et al., 2019). In the present study, INHBA was found to contain five distal intergenic DMRs, with four DMRs being hyper-methylated and one being hypo-methylated in the LPB + vs. HPBB group. Moreover, six DMRs (five distal intergenic and one intron) were identified in INHBA, of which five were hypo-methylated and one was hyper-methylated in the LPB + vs. LPBB group. FZD1 has been confirmed to regulate certain biological processes, including oocyte maturation, female fertility (Lapointe et al., 2012), embryonic development (Tribulo et al., 2017) and ovary development (Tepekoy et al., 2019). The methylation level of the 3′-UTR of FZD1 in the HPBB group was higher than that in the LPB + group. In addition, most DMGs were enriched in the ovulation and ovarian follicle development biological processes. These results supported the hypothesis that DNA methylation as a regulator of epigenetic modification could influence the prolificacy phenotype (Hwang et al., 2017; Miao et al., 2017; Zhang et al., 2017).
To understand how DNA methylation influences the prolificacy of Hu sheep, here, for the first time, we systematically analyzed and compared the genome-wide DNA methylation and transcriptome of ovaries from Hu sheep with different prolificacies and genotypes. The number of DMGs-DEGs in the LPBB vs. HPBB group was lower than that in both the LPB + vs. HPBB and LPB + vs. LPBB groups. This finding suggests that, although changes in DNA methylation may influence fecundity, the differences are mainly attributed to the genotype. The most significant results of this study showed that an inverse relationship was observed between DNA methylation and gene expression in the HPBB, LPBB, and LPB + groups, which is in agreement with the results of previous studies (Jadhao et al., 2017; Wang et al., 2017; Yang et al., 2017).
DNA methylation status in the promoter and gene body regions regulates gene expression by changing transcription efficiency or chromatin structure (Jones 2012; Yang et al., 2014). Therefore, we further selected the genes (ITGB2 and LAPTM4B) that exhibited inverse changes in DNA methylation and gene expression, and those with DMRs in the promoter or gene body for subsequent analyses. For example, the promoter region of ITGB2, an important regulator of embryo implantation (Guo et al., 2018), oocyte maturation (Antosik et al., 2016) and follicle development (Kisliouk et al., 2007; Antosik et al., 2016), was hyper-methylated in the HPBB group compared to that in the LPBB group; however, its expression was higher in the LPBB group than in the HPBB group. Meanwhile, ITGB2 expression level in atretic follicles was higher than in healthy follicles (Kisliouk et al., 2007). Up-regulated expression of ITGB2 in the LPBB group may be responsible for the increased number of atretic follicles. Previous studies have reported that LAPTM4B is expressed in the reproductive organs and reproductive diseases in bovine or human (Yang et al., 2008; Ndiaye et al., 2015; Meng et al., 2016). Ndiaye et al. (2015) reported that LAPTM4B expression was higher in large dominant follicles than in small antral follicles. The results of the present study showed that the LAPTM4B promoter was hyper-methylated and LAPTM4B expression was down-regulated in the HPBB group compared to that in the LPB + group. Moreover, the relationship between ITGB2 and LPATM4B expression and epigenetic modifications in the ovaries and cultured GCs were assessed, and the results showed an inverse relationships occurred for both. These findings may, to some extent, explain the significant differences in phenotypic variation among Hu sheep.
In summary, the present study systematically integrated DNA methylation and gene expression profiles in the ovaries of Hu sheep with different phenotypes and genotypes, indicating the potential mechanisms underlying on prolificacy phenotypic variation and providing new insights into the genetic mechanism responsible for the excellent fecundity of Hu sheep. As several factors, including physiological, environmental and diet, have been shown to affect phenotypic variation (Peaston and Whitelaw, 2006). Therefore, further studies are needed to fully understand the effects of epigenetic modification on the fecundity of Hu sheep, which may contribute to better reproductive efficiency.
Data Availability Statement
The raw data of transcriptome and DNA methylome have been deposited into the Sequence Read Archive database (https://www.ncbi.nlm.nih.gov/sra) under accession numbers are PRJNA681364 and PRJNA758179, respectively.
Ethics Statement
The animal study was reviewed and approved by Institutional Animal Ethics Committee of the Nanjing Agricultural University (SYXK2011-0036).
Author Contributions
Bioinformatics analysis and methodology were performed by JY, XY, and FL. Investigation and resources were done by FW and ZW. Under the supervision of FW. Experiments validation were performed by XY and FY. Animal feeding and sample collection were done by FL, ZW, and XF. The original draft of the manuscript was written by XY. The manuscript was reviewed and edited by FW and ME-S.
Funding
This work was supported by the National Natural Science Foundation of China (32002174; 31872357), Fundamental Research Funds for the Central Universities (KJQN202130), Jiangsu Agricultural Industry Technology System (JATS(2021)141) and Jiangsu Planned Projects for Postdoctoral Research Funds (2021K254B).
Conflict of Interest
The authors declare that the research was conducted in the absence of any commercial or financial relationships that could be construed as a potential conflict of interest.
Publisher’s Note
All claims expressed in this article are solely those of the authors and do not necessarily represent those of their affiliated organizations, or those of the publisher, the editors and the reviewers. Any product that may be evaluated in this article, or claim that may be made by its manufacturer, is not guaranteed or endorsed by the publisher.
Acknowledgments
We sincerely thank Jinyu Yang from the Biomarker Technologies Corporation for help in bioinformatic analysis.
Supplementary Material
The Supplementary Material for this article can be found online at: https://www.frontiersin.org/articles/10.3389/fcell.2022.820558/full#supplementary-material
Supplementary Figure S1 | KEGG pathway enrichment analysis of DMGs. (A) LPBB vs. HPBB, (B) LPB+ vs. HPBB, and (C) LPB+ vs. LPBB groups.
Supplementary Figure S2 | GO and KEGG enrichment analysis of DMGs related to female reproduction. (A) LPBB vs. HPBB, (B) LPB+ vs. HPBB, and (C) LPB+ vs. LPBB groups.
Supplementary Figure S3 | GO and KEGG pathway enrichment analysis of DEGs related to female reproduction. (A,B) LPBB vs. HPBB, (C,D) LPB+ vs. HPBB, and (E,F) LPB+ vs. LPBB groups.
References
An, X., Ma, H., Han, P., Zhu, C., Cao, B., and Bai, Y. (2018). Genome-wide Differences in DNA Methylation Changes in Caprine Ovaries between Oestrous and Dioestrous Phases. J. Anim. Sci Biotechnol 9, 85. doi:10.1186/s40104-018-0301-x
Antosik, P., Jeseta, M., Kranc, W., Chachuła, A., Bryja, A., Budna, J., et al. (2016). Expression of Integrins and GDF9 mRNAs Is Associated with Ovarian Follicle Size and Donor Puberty Status in Pigs. Med. Weter 72, 750–754. doi:10.21521/mw.5601
Canovas, S., Ross, P. J., Kelsey, G., and Coy, P. (2017). DNA Methylation in Embryo Development: Epigenetic Impact of ART (Assisted Reproductive Technologies). Bioessays 39, 1700106. doi:10.1002/bies.201700106
Cao, P., Yang, W., Wang, P., Li, X., and Nashun, B. (2021). Characterization of DNA Methylation and Screening of Epigenetic Markers in Polycystic Ovary Syndrome. Front. Cel Dev. Biol. 9, 664843. doi:10.3389/fcell.2021.664843
Chu, M. X., Liu, Z. H., Jiao, C. L., He, Y. Q., Fang, L., Ye, S. C., et al. (2007). Mutations in BMPR-IB and BMP-15 Genes Are Associated with Litter Size in Small Tailed Han Sheep (Ovis aries)1. J. Anim. Sci. 85, 598–603. doi:10.2527/jas.2006-324
Cui, Z., Liu, L., Zhu, Q., Wang, Y., Yin, H., Li, D., et al. (2020). Inhibin A Regulates Follicular Development via Hormone Secretion and Granulosa Cell Behaviors in Laying Hens. Cell Tissue Res 381, 337–350. doi:10.1007/s00441-020-03207-8
Fabre, S., Pierre, A., Mulsant, P., Bodin, L., Di Pasquale, E., Persani, L., et al. (2006). Regulation of Ovulation Rate in Mammals: Contribution of Sheep Genetic Models. Reprod. Biol. Endocrinol. 4, 20. doi:10.1186/1477-7827-4-20
Fan, Y., Liang, Y., Deng, K., Zhang, Z., Zhang, G., Zhang, Y., et al. (2020). Analysis of DNA Methylation Profiles during Sheep Skeletal Muscle Development Using Whole-Genome Bisulfite Sequencing. BMC genomics 21, 327. doi:10.1186/s12864-020-6751-5
Fang, X., Zhao, Z., Yu, H., Li, G., Jiang, P., Yang, Y., et al. (2017). Comparative Genome-wide Methylation Analysis of Longissimus Dorsi Muscles between Japanese Black (Wagyu) and Chinese Red Steppes Cattle. PloS one 12, e0182492. doi:10.1371/journal.pone.0182492
Farhadi, A., Genualdo, V., Perucatti, A., Hafezian, S. H., Rahimi-Mianji, G., Lorenzi, L. D., et al. (2013). Comparative FISH Mapping of BMPR1B, BMP15 and GDF9 Fecundity Genes on Cattle, River buffalo, Sheep and Goat Chromosomes. J. Genet. 92, 595–597. doi:10.1007/s12041-013-0301-8
Feng, X., Li, F., Wang, F., Zhang, G., Pang, J., Ren, C., et al. (2018). Genome-wide Differential Expression Profiling of mRNAs and lncRNAs Associated with Prolificacy in Hu Sheep. Biosci. Rep. 38, BSR20171350. doi:10.1042/BSR20171350
Gao, X., Yao, X., Wang, Z., Li, X., Li, X., An, S., et al. (2020). Long Non-coding RNA366.2 Controls Endometrial Epithelial Cell Proliferation and Migration by Upregulating WNT6 as a ceRNA of miR-1576 in Sheep Uterus. Biochim. Biophys. Acta (Bba) - Gene Regul. Mech. 1863, 194606. doi:10.1016/j.bbagrm.2020.194606
González-Recio, O., Toro, M. A., and Bach, A. (2015). Past, Present, and Future of Epigenetics Applied to Livestock Breeding. Front. Genet. 6, 305. doi:10.3389/fgene.2015.00305
Gootwine, E., Reicher, S., and Rozov, A. (2008). Prolificacy and Lamb Survival at Birth in Awassi and Assaf Sheep Carrying the FecB (Booroola) Mutation. Anim. Reprod. Sci. 108, 402–411. doi:10.1016/10.1016/j.anireprosci.2007.09.009
Guo, F., Si, C., Zhou, M., Wang, J., Zhang, D., Leung, P. C. K., et al. (2018). Decreased PECAM1-Mediated TGF-β1 Expression in the Mid-secretory Endometrium in Women with Recurrent Implantation Failure. Hum. Reprod. 33, 832–843. doi:10.1093/humrep/dey022
Hiendleder, S., Leyhe, B., Jaeger, C., Erhardt, G., and Wassmuth, R. (1996). Molecular Characterization of Ovine α-, βA and βB-inhibin/activin Alleles. J. Anim. Breed. Genet. 113, 363–372. doi:10.1111/j.1439-0388.1996.tb00627.x
Hwang, J. H., An, S. M., Kwon, S., Park, D. H., Kim, T. W., Kang, D. G., et al. (2017). DNA Methylation Patterns and Gene Expression Associated with Litter Size in Berkshire Pig Placenta. PloS one 12, e0184539. doi:10.1371/journal.pone.0184539
Jones, P. A. (2012). Functions of DNA Methylation: Islands, Start Sites, Gene Bodies and beyond. Nat. Rev. Genet. 13, 484–492. doi:10.1038/nrg3230
Kang, B., Wang, J., Zhang, H., Shen, W., El‐Mahdy Othman, O., Zhao, Y., et al. (2021). Genome‐wide Profile in DNA Methylation in Goat Ovaries of Two Different Litter Size Populations. J. Anim. Physiol. Anim. Nutr., 1–11. doi:10.1111/jpn.13600
Khavari, D. A., Sen, G. L., and Rinn, J. L. (2010). DNA Methylation and Epigenetic Control of Cellular Differentiation. Cell cycle 9, 3880–3883. doi:10.4161/cc.9.19.13385
Kisliouk, T., Friedman, A., Klipper, E., Zhou, Q.-Y., Schams, D., Alfaidy, N., et al. (2007). Expression Pattern of Prokineticin 1 and its Receptors in Bovine Ovaries during the Estrous Cycle: Involvement in Corpus Luteum Regression and Follicular Atresia. Biol. Reprod. 76, 749–758. doi:10.1095/biolreprod.106.054734
Lapointe, E., Boyer, A., Rico, C., Paquet, M., Franco, H. L., Gossen, J., et al. (2012). FZD1 Regulates Cumulus Expansion Genes and Is Required for Normal Female Fertility in Mice1. Biol. Reprod. 87, 104. doi:10.1095/biolreprod.112.102608
Li, Z., Wang, J., Zhao, Y., Ma, D., Zhao, M., Li, N., et al. (2021). scRNA‐Seq of Ovarian Follicle Granulosa Cells from Different Fertility Goats Reveals Distinct Expression Patterns. Reprod. Dom Anim. 56, 801–811. doi:10.1111/rda.13920
Lomniczi, A., Wright, H., and Ojeda, S. R. (2015). Epigenetic Regulation of Female Puberty. Front. Neuroendocrinology 36, 90–107. doi:10.1016/j.yfrne.2014.08.003
Mao, X., Cai, T., Olyarchuk, J. G., and Wei, L. (2005). Automated Genome Annotation and Pathway Identification Using the KEGG Orthology (KO) as a Controlled Vocabulary. Bioinformatics 21, 3787–3793. doi:10.2307/159221510.1093/bioinformatics/bti430
Mattern, F., Heinzmann, J., Herrmann, D., Lucas-Hahn, A., Haaf, T., and Niemann, H. (2017). Gene-specific Profiling of DNA Methylation and mRNA Expression in Bovine Oocytes Derived from Follicles of Different Size Categories. Reprod. Fertil. Dev. 29, 2040–2051. doi:10.1071/RD16327
Meng, F., Chen, X., Song, H., Lou, G., and Fu, S. (2016). Lentivirus-mediated RNA Interference Targeting LAPTM4B Inhibits Human Ovarian Cancer Cell InvasionIn Vitro. Chem. Biol. Drug Des. 87, 121–130. doi:10.1111/cbdd.12632
Miao, X., Luo, Q., Zhao, H., and Qin, X. (2017). An Integrated Analysis of miRNAs and Methylated Genes Encoding mRNAs and lncRNAs in Sheep Breeds with Different Fecundity. Front. Physiol. 8, 1049. doi:10.3389/fphys.2017.01049
Miyakuni, K., Nishida, J., Koinuma, D., Nagae, G., Aburatani, H., Miyazono, K., et al. (2021). Genome-wide Analysis of DNA Methylation Identifies the Apoptosis-Related Gene UQCRH in Renal Cancer. Mol. Oncol. 16. doi:10.21203/rs.3.rs-147857/v1
Ndiaye, K., Carrière, P. D., Sirois, J., Silversides, D. W., and Lussier, J. G. (2015). Differential Expression of Lysosome-Associated Protein Transmembrane-4 Beta (LAPTM4B) in Granulosa Cells of Ovarian Follicles and in Other Bovine Tissues. J. Ovarian Res. 8, 12. doi:10.1186/s13048-015-0148-0
Peaston, A. E., and Whitelaw, E. (2006). Epigenetics and Phenotypic Variation in Mammals. Mamm. Genome 17, 365–374. doi:10.1007/s00335-005-0180-2
Polley, S., De, S., Brahma, B., Mukherjee, A., P.V., V., Batabyal, S., et al. (2010). Polymorphism of BMPR1B, BMP15 and GDF9 Fecundity Genes in Prolific Garole Sheep. Trop. Anim. Health Prod. 42, 985–993. doi:10.1007/s11250-009-9518-1
Sagvekar, P., Kumar, P., Mangoli, V., Desai, S., and Mukherjee, S. (2019). DNA Methylome Profiling of Granulosa Cells Reveals Altered Methylation in Genes Regulating Vital Ovarian Functions in Polycystic Ovary Syndrome. Clin. Epigenet 11, 61. doi:10.1186/s13148-019-0657-6
Salilew-Wondim, D., Fournier, E., Hoelker, M., Saeed-Zidane, M., Tholen, E., Looft, C., et al. (2015). Genome-Wide DNA Methylation Patterns of Bovine Blastocysts Developed In Vivo from Embryos Completed Different Stages of Development In Vitro. PloS one 10, e0140467. doi:10.1371/journal.pone.0140467
Singh, K., Molenaar, A. J., Swanson, K. M., Gudex, B., Arias, J. A., Erdman, R. A., et al. (2012). Epigenetics: a Possible Role in Acute and Transgenerational Regulation of Dairy Cow Milk Production. Animal 6, 375–381. doi:10.1017/S1751731111002564
Tepekoy, F., Uysal, F., Acar, N., Ustunel, I., and Akkoyunlu, G. (2019). The Effect of GnRH Antagonist Cetrorelix on Wnt Signaling Members in Pubertal and Adult Mouse Ovaries. Histochem. Cel Biol 152, 423–437. doi:10.1007/s00418-019-01817-0
Tesfaye, D., Ghanem, N., Carter, F., Fair, T., Sirard, M.-A., Hoelker, M., et al. (2009). Gene Expression Profile of Cumulus Cells Derived from Cumulus - Oocyte Complexes Matured Either In Vivo or In Vitro. Reprod. Fertil. Dev. 21, 451–461. doi:10.1071/rd08190
Tirnaz, S., and Batley, J. (2019). DNA Methylation: Toward Crop Disease Resistance Improvement. Trends Plant Sci. 24, 1137–1150. doi:10.1016/j.tplants.2019.08.007
Tribulo, P., Moss, J. I., Ozawa, M., Jiang, Z., Tian, X., and Hansen, P. J. (2017). WNT Regulation of Embryonic Development Likely Involves Pathways Independent of Nuclear CTNNB1. Reproduction 153, 405–419. doi:10.1530/REP-16-0610
Vázquez-Martínez, E. R., Gómez-Viais, Y. I., García-Gómez, E., Reyes-Mayoral, C., Reyes-Muñoz, E., Camacho-Arroyo, I., et al. (2019). DNA Methylation in the Pathogenesis of Polycystic Ovary Syndrome. Reproduction 158, R27–R40. doi:10.1530/REP-18-0449
Wang, H., Wang, J., Ning, C., Zheng, X., Fu, J., Wang, A., et al. (2017). Genome-wide DNA Methylation and Transcriptome Analyses Reveal Genes Involved in Immune Responses of Pig Peripheral Blood Mononuclear Cells to Poly I:C. Sci. Rep. 7, 9709. doi:10.1038/s41598-017-10648-9
Wang, W., Liu, S., Li, F., Pan, X., Li, C., Zhang, X., et al. (2015). Polymorphisms of the Ovine BMPR-IB, BMP-15 and FSHR and Their Associations with Litter Size in Two Chinese Indigenous Sheep Breeds. Ijms 16, 11385–11397. doi:10.3390/ijms160511385
Wang, X. X., Wei, J. Z., Jiao, J., Jiang, S. Y., Yu, D. H., and Li, D. (2014). Genome-wide DNA Methylation and Gene Expression Patterns Provide Insight into Polycystic Ovary Syndrome Development. Oncotarget 5, 6603–6610. doi:10.18632/oncotarget.2224
Wei, Y., Lang, J., Zhang, Q., Yang, C. R., Zhao, Z. A., Zhang, Y., et al. (2019). DNA Methylation Analysis and Editing in Single Mammalian Oocytes. Proc. Natl. Acad. Sci. USA 116, 9883–9892. doi:10.1073/pnas.1817703116
Yang, C., Ye, J., Li, X., Gao, X., Zhang, K., Luo, L., et al. (2016). DNA Methylation Patterns in the Hypothalamus of Female Pubertal Goats. PloS one 11, e0165327. doi:10.1371/journal.pone.0165327
Yang, X., Han, H., De Carvalho, D. D., Lay, F. D., Jones, P. A., and Liang, G. (2014). Gene Body Methylation Can Alter Gene Expression and Is a Therapeutic Target in Cancer. Cancer cell 26, 577–590. doi:10.1016/j.ccr.2014.07.028
Yang, Y., Yang, H., McNutt, M. A., Xiong, F., Nie, X., Li, L., et al. (2008). LAPTM4B Overexpression Is an Independent Prognostic Marker in Ovarian Carcinoma. Oncol. Rep. 20, 1077–1083. doi:10.3892/or_00000112
Yang, Y., Fan, X., Yan, J., Chen, M., Zhu, M., Tang, Y., et al. (2021). A Comprehensive Epigenome Atlas Reveals DNA Methylation Regulating Skeletal Muscle Development. Nucleic Acids Res. 49, 1313–1329. doi:10.1093/nar/gkaa1203
Yang, Y., Liang, G., Niu, G., Zhang, Y., Zhou, R., Wang, Y., et al. (2017). Comparative Analysis of DNA Methylome and Transcriptome of Skeletal Muscle in Lean-, Obese-, and Mini-type Pigs. Sci. Rep. 7, 39883. doi:10.1038/srep39883
Yao, X., Gao, X., Bao, Y., El-Samahy, M. A., Yang, J., Wang, Z., et al. (2021). lncRNA FDNCR Promotes Apoptosis of Granulosa Cells by Targeting the miR-543-3p/DCN/TGF-β Signaling Pathway in Hu Sheep. Mol. Ther. - Nucleic Acids 24, 223–240. doi:10.1016/j.omtn.2021.02.030
Yao, X., Yang, H., Zhang, Y., Ren, C., Nie, H., Fan, Y., et al. (2017). Characterization of GALNTL5 Gene Sequence and Expression in Ovine Testes and Sperm. Theriogenology 95, 54–61. doi:10.1016/j.theriogenology.2017.02.018
Young, M. D., Wakefield, M. J., Smyth, G. K., and Oshlack, A. (2010). Gene Ontology Analysis for RNA-Seq: Accounting for Selection Bias. Genome Biol. 11, R14. doi:10.1186/gb-2010-11-2-r14
Yu, D. Y., Wu, R. Z., Zhao, Y., Nie, Z. H., Wei, L., Wang, T. Y., et al. (2019). Polymorphisms of Four Candidate Genes and Their Correlations with Growth Traits in Blue Fox (Alopex lagopus). Gene 717, 143987. doi:10.1016/j.gene.2019.143987
Yu, Y. Y., Sun, C. X., Liu, Y. K., Li, Y., Wang, L., and Zhang, W. (2015). Genome-wide Screen of Ovary-specific DNA Methylation in Polycystic Ovary Syndrome. Fertil. Sterility 104, 145–153. doi:10.1016/j.fertnstert.2015.04.005
Yuan, X. L., Gao, N., Xing, Y., Zhang, H. B., Zhang, A. L., Liu, J., et al. (2016). Profiling the Genome-wide DNA Methylation Pattern of Porcine Ovaries Using Reduced Representation Bisulfite Sequencing. Sci. Rep. 6, 22138. doi:10.1038/srep22138
Yuan, X., Ye, S., Chen, Z., Pan, X., Huang, S., Li, Z., et al. (2019). Dynamic DNA Methylation of Ovaries during Pubertal Transition in Gilts. BMC genomics 20, 510. doi:10.1186/s12864-019-5884-x
Yue, G. H. (1996). Reproductive Characteristics of Chinese Hu Sheep. Anim. Reprod. Sci. 44, 223–230. doi:10.1016/0378-4320(96)01562-X
Zhang, Y., Li, F., Feng, X., Yang, H., Zhu, A., Pang, J., et al. (2017). Genome-wide Analysis of DNA Methylation Profiles on Sheep Ovaries Associated with Prolificacy Using Whole-Genome Bisulfite Sequencing. BMC genomics 18, 759. doi:10.1186/s12864-017-4068-9
Zhong, X., Jin, F., Huang, C., Du, M., Gao, M., and Wei, X. (2021). DNA Methylation of AMHRII and INSR Gene Is Associated with the Pathogenesis of Polycystic Ovary Syndrome (PCOS). Thc 29, 11–25. doi:10.3233/THC-218002
Keywords: DNA methylation, gene expression, ovary, prolificacy, Hu sheep
Citation: Yao X, Li F, Wei Z, EI-Samahy MA, Feng X, Yang F and Wang F (2022) Integrative Genome-Wide DNA Methylome and Transcriptome Analysis of Ovaries from Hu Sheep with High and Low Prolific. Front. Cell Dev. Biol. 10:820558. doi: 10.3389/fcell.2022.820558
Received: 23 November 2021; Accepted: 14 January 2022;
Published: 03 February 2022.
Edited by:
George E. Liu, Agricultural Research Service (USDA), United StatesReviewed by:
Xiao Wang, Konge Larsen ApS, DenmarkMingxing Chu, Institute of Animal Sciences (CAAS), China
Hui Li, Guangxi University, China
Copyright © 2022 Yao, Li, Wei, EI-Samahy, Feng, Yang and Wang. This is an open-access article distributed under the terms of the Creative Commons Attribution License (CC BY). The use, distribution or reproduction in other forums is permitted, provided the original author(s) and the copyright owner(s) are credited and that the original publication in this journal is cited, in accordance with accepted academic practice. No use, distribution or reproduction is permitted which does not comply with these terms.
*Correspondence: Feng Wang, Y2FlZXRAbmphdS5lZHUuY24=