- 1Department of Urology, Shandong Provincial Hospital Affiliated to Shandong University, Jinan, China
- 2Department of Urology, Shandong Provincial Hospital Affiliated to Shandong First Medical University, Jinan, China
- 3Cheeloo College of Medicine, Shandong University, Jinan, China
- 4Department of Nephrology, Shandong Provincial Hospital Affiliated to Shandong University, Jinan, China
- 5Department of Nephrology, Shandong Provincial Hospital Affiliated to Shandong First Medical University, Jinan, China
- 6Department of Urology, Shandong Provincial ENT Hospital Affiliated to Shandong University, Jinan, China
N6-methyladenosine (m6A) is the product of the most prevalent mRNA modification in eukaryotic cells. Accumulating evidence shows that tumor microenvironment (TME) plays a pivotal role in tumor development. However, the underlying relationship between m6A modification and the TME of a papillary renal cell carcinoma (PRCC) is still unclear. To investigate the relationship between m6A modification and prognosis and immunotherapeutic efficacy for PRCC, we looked for distinct m6A modification patterns based on 23 m6A-related genes. Next, the correlation between m6A modification patterns and TME-related characteristics was investigated. Then, the intersected differentially expressed genes were selected and the scoring system, denoted as m6A score, was established to evaluate m6A modification, prognosis, and immunotherapeutic efficacy. In this study, three distinct m6A expression clusters were identified. Based on the results of immune cell infiltration analysis and functional analysis, carcinogenic pathways, TME-related immune cells, and pathways were identified as well. More importantly, the established m6A score showed good value in predicting clinical outcomes according to results using external cohorts. Specifically, PRCC patients with low m6A score value showed better survival, immunotherapeutic response, and higher tumor mutation burden. Furthermore, immunohistochemistry using PRCC clinical samples from our medical center was carried out and verified our results. In conclusion, this study highlights the underlying correlation between m6A modification and the immune landscape and, hence, enhances our understanding of the TME and improved the therapeutic outlook for PRCC patients.
Introduction
Kidney cancer is a heterogenous disease for which several subtypes with different genetic and morphologic characteristics are identified. Renal cell carcinoma (RCC) accounts for the vast majority of histological types of kidney cancer with clear cell renal cell carcinoma (ccRCC) making up 70%–80% and papillary renal cell carcinoma (PRCC) 15%–20% of RCCs (Linehan et al., 2016; Barata and Rini, 2017; Vuong et al., 2019). Although most cases of PRCC are indolent with limited risk of mortality, the overall prognosis for PRCC remains limited (Steffens et al., 19902012).
The tumor microenvironment TME is a cellular environment in which tumor cells and other nonmalignant cells exist, and it is composed of various immune cells and related materials, including lymphocytes, fibroblasts, stromal cells, blood vessels, and so on (Wu and Dai, 2017). The TME acts as the soil of tumor cells, and the great impact of TME on tumorigenesis and tumor immunotherapy has become increasingly evident (Li et al., 2021). In an abnormal TME, immune cells become significantly remodeled, which affects their normal functions, such as proliferation, migration, and differentiation (Binnewies et al., 2018). Therefore, immunosuppression is the essential characteristic of TME. Currently, RCC tumors are considered to be immunogenic, and many studies find that various immune cells could infiltrate into RCC TMEs. However, these immune cells block the effective antitumor responses. Owing to the immunosuppressed state of RCC tumors and the immune-tolerance of TMEs, the response of RCC to immune checkpoint inhibitors (ICIs) is unsatisfactory (Syn et al., 2017).
Due to the advances in RNA sequencing, N6-methyladenosine (m6A), the product of the most common type of mRNA modification in eukaryotic cells, has garnered great interest (Qi et al., 2016; Ke et al., 2017). The m6A modification is regulated by three types of molecules, known as “writer,” “eraser,” and “reader” molecules (Yang et al., 2018). It is reported that m6A modification plays multifaceted roles in tumor development and metastasis (Xiao et al., 2018). Various research investigation indicates that abnormal m6A modification occurs in most immune cells, including dendritic cells, regulatory T cells, macrophages, CD4+ T cells, and CD8+ T cells, and results in tumor escape or immune disorder (Chen et al., 2018; Han et al., 2019; Li et al., 2021). However, it is still unclear whether m6A modification in diverse immune cells in the TME is responsible for tumor progression and the effectiveness of ICIs. Therefore, it is essential to determine the potential effects of m6A modification on the TME and to explore its clinic value as a new therapeutic tool for treatment of PRCC.
Materials and Methods
Data Collection and Processing
The expression data and clinical information for kidney renal papillary cell carcinoma (KIRP) were downloaded directly from the Cancer Genome Atlas (TCGA) (https://cancergenome.nih.gov/), Gene Expression Omnibus (https://www.ncbi.nlm.nih.gov/geo/), and the Cancer Immunome Atlas (TCIA) (https://tcia.at/home). Specific data from 289 KIRP patients and 32 tumor-free patients were obtained from these databases. Copy number variation (CNV) and somatic mutation data were downloaded from TCGA as well. Samples without survival data were removed. The “limma” package was used to normalize gene expression data and transform fragments per kilobase per million (FPKM) values to transcripts per kilobase per million (TPM) value. R (R version 4.0.1) was used to extract and analyze expression data and clinical information. After conducting a comprehensive literature review (Zhang et al., 2020; Gu et al., 2021; Zhong et al., 2021), we identified 23 m6A regulators, including METTL3, METTL14, METTL16, WTAP, VIRMA, ZC3H13, RBM15, RBM15B, YTHDC1, YTHDC2, YTHDF1, YTHDF2, YTHDF3, HNRNPC, FMR1, LRPPRC, HNRNPA2B1, IGFBP1, IGFBP2, IGFBP3, RBMX, FTO, and ALKBH5, representing m6A writers, readers, and erasers.
Identification of Differentially Expressed Genes and Functional Analysis
The “limma” and “ggplot2” packages were used to assess and visualize the differentially expressed genes (DEGs) in KIRP samples and nontumor tissues. Difference with adjust p < .01 were considered to be significant. Gene Ontology (GO) and Kyoto Encyclopedia of Genes and Genomes (KEGG) analyses were performed through the “clusterProfiler” package. To determine the differences in biological processes between various m6A expression clusters, specifically to estimate the variation in biological processes, gene set variation analysis (GSVA) was conducted by using the “GSVA” package (Hänzelmann et al., 2013). We utilized the gene set “c2.cp.kegg.v6.2-symbols” from the MSigDB database (Liberzon et al., 2011). Here, adjusted p < .05 was considered as the threshold.
Estimation of TME Immune Cell Infiltration and Tumor Mutation Burden
Single-sample gene-set enrichment analysis (ssGSEA) was used to quantify the level of immune infiltration into the PRCC TME (Barbie et al., 2009; Charoentong et al., 2017). The relevant gene set, which marks various TME-infiltrated immune cell subtypes, was collected from previous studies (Barbie et al., 2009; Charoentong et al., 2017). The ssGSEA scores represented the enrichment of different immune cell subtypes in each sample. Tumor mutation burden (TMB) was analyzed with the KIRP somatic mutation data by using the “maftools” R package (Chen and Mellman, 2017). Two TMB sets (high and low TMB) were constructed by using an optimal cutoff value of TMB. We evaluated the difference between the m6Ascore values of two TMB sets.
Unsupervised Clustering and the Construction of an m6A Regulators Model
Owing to relatively small sizes of the KIRP data sets in the Gene-Expression Omnibus (GEO) database, we used the GSE2748 cohort and TCGA KIRP data set to perform unsupervised clustering analysis with the “ConsensusClusterPlus” package (Wilkerson and Hayes, 2010). Here, 1000 repetitions were performed. The expression data of 23 m6A genes were extracted from GSE2748. The clustering analysis was performed to classify the KIRP samples into distinct m6A expression clusters based on the expression of 23 m6A regulators.
To quantify the m6A expression cluster of each KIRP sample, the m6A score was applied and established as follows. First, we identified intersected DEGs from the constructed m6A expression clusters. All KIRP patients were divided into diverse groups via unsupervised clustering analysis. Then, the univariate Cox regression analysis was utilized to assess the prognosis of each selected gene. p < .05 was considered as the significance criterion. After extracting the prognosis-related regulators, we applied principal component analysis to establish the m6A gene model, and the principal components 1 and 2 were selected as signature scores. Finally, m6A score was calculated using following formula: m6A score = Σ (PC1i + PC2i) (where i is the expression of the selected m6A related DEGs from the m6A expression cluster) (Sotiriou et al., 2006; Zeng et al., 2019).
Genomic and Clinical Data for ICI Therapy
Then, we investigated whether the established m6A expression cluster could predict the response of PRCC to ICI therapy based on two immunotherapy cohorts. After a comprehensive search for gene expression data and complete clinical information of patients treated with ICIs, we finally included two related cohorts. The first cohort involved metastatic melanoma patients treated with the anti-PD-1 drug (pembrolizumab) from the GEO database (GSE78220). Moreover, genomic and clinical data for mTOR inhibitor (everolimus) therapy was downloaded from the Supplementary File appended to published study (Barbie et al., 2009; Charoentong et al., 2017). All raw expression data were normalized using the “limma” package and transformed into the more comparable TPM value.
Immunohistochemistry
Five pairs of PRCC and adjacent normal tissues were collected from May 2021 to October 2021 from Shandong Provincial Hospital affiliated with Shandong First University. The study was approved by the Ethics Committee of Shandong Provincial Hospital (Approval No. SWYX: NO. 2021-491). IHC was performed according to published method (Wang et al., 2020). All samples were incubated with rabbit polyclonal anti-CD8 (ab101500), anti-CD69 (ab233396), anti-CD163 (ab182422), anti-YTHDF1 (ab252346), anti-YTHDF2 (ab220163), anti-YTHDF3 (ab220161), anti-ZC3H13 (IHC0104123), anti-HNRNPA2B1 (ab31645), and anti-IGFBP2 (ab188200) antibodies overnight at 4°C and then washed. Two pathologists independently assessed the IHC slides.
Statistical Analysis
The Kruskal–Wallis test was used to estimate the significance of differences between values of three or more groups. Spearman’s correlation analysis was applied to calculate the correlation coefficient between number of TME-infiltrated immune cells and the expression level of m6A regulators. We employed the “survminer” package to determine the optimal cutoff value. Based on the optimal cutoff point, all PRCC patients were grouped into high or low m6A score sets. Then, the Kaplan–Meier analysis with a log-rank test was conducted to test the prognosis of patients. The mutation landscape of KIRP cohorts was depicted by using the “maftools” package (Mayakonda et al., 2018). Statistical analysis was performed with R packages (version 4.0.1). A two-tailed p < .05 was considered to be significant.
Results
Genetic Variation and Clinical Relevance of m6A Genes in PRCC
Based on the transcriptomic profiles of 23 m6A regulators, we investigated the expression pattern of all m6A regulators in PRCC and normal samples from TCGA (Figure 1A). Then, we integrated CNV as well as somatic mutations and illustrated the prevalence of alteration of m6A genes in PRCC. Only 22 of 281 samples (7.83%) showed m6A regulator mutations. Specifically, 8 out of 23 m6A regulators experienced mutations (Figure 1B). Afterward, we investigated the CNV frequency of 23 m6A genes, which identified that most CNV alterations in 23 genes were focused on the CNV deletion (Figure 1C). Moreover, we determined the locations of the CNV alteration on human chromosomes as well (Figure 1D). These results indicate that genetic variation commonly occurs in PRCC cells and is heterogeneous between PRCC and normal tissues, exhibiting the potential role for the aberrant expression of m6A genes in tumorigenesis and development as well as progression. Finally, when investigating the potential clinical relevance of 23 m6A regulators, we found that three types of m6A regulators were positively correlated with patient prognosis and interacted with each other (Figure 1E and Supplementary Figure S1A). In addition, most of the genes were indicated to be risk factors for overall survival (OS) of PRCC patients; only YTHDC1, ALKBH5, FTO, RBM15B, METTL14, and METTL16 were out.
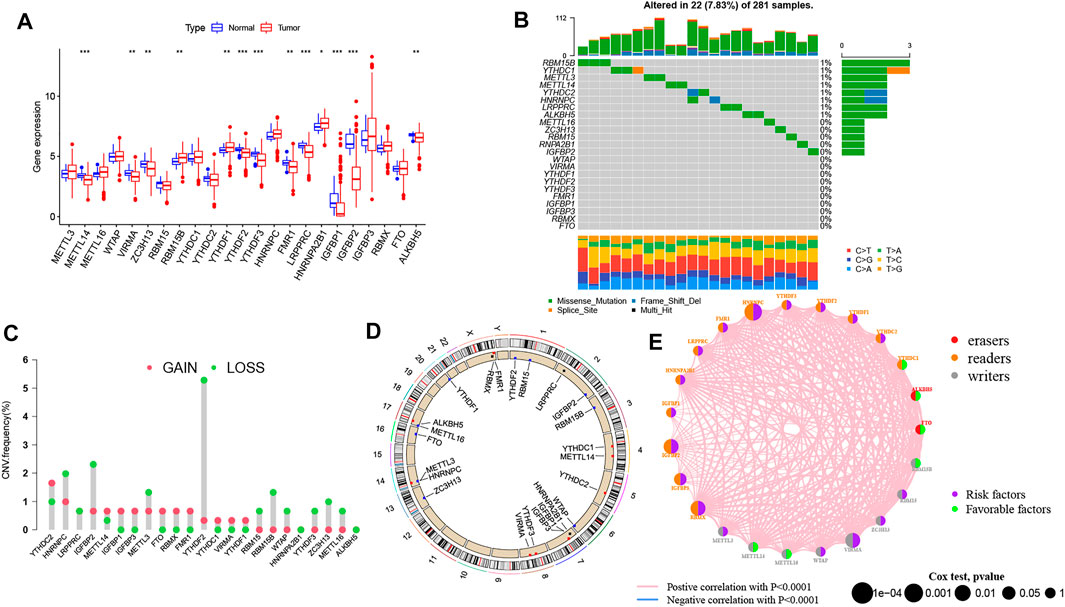
FIGURE 1. Characteristics of genetic and variation of m6A genes in pRCC. (A) Transcriptomic profile of 23 m6A genes among normal and PRCC tumor tissues. The upper and lower ends of the boxes indicate interquartile range (IQR) of values. The lines in the boxes represent each the median value. The asterisks represented the p value. (*p < .05; **p < .01; ***p < .001) (B) Mutation frequency of 23 m6A genes in in 289 PRCC patients. The bar plot at the upper part of the figure shows TMB. Numbers on the right part of the figure represent the mutation frequency. (C) CNV alteration frequency of 23 m6A genes in the 289-patient PRCC cohort. The height of each column indicates the alteration frequency. The red dot represents the amplification frequency; the green dot represents the deletion frequency. (D) Location of CNV alterations of 23 m6A genes on human chromosomes in PRCC cohort. (E) Interaction network of 23 m6A genes in the PRCC cohort. The size of each circle is indicative of the magnitude of the effect on the prognosis. The green dots mean the protective factors, and the purple dots represent the risk factors. The thickness of each line indicates the degree of correlation between each gene.
We also determined whether genetic variations of “writer,” “reader,” and “eraser” genes were associated with the expression other m6A regulators’ (Supplementary Figures S1B–L). The results demonstrate that only YTHDF1 was upregulated in METTL14 mutated PRCC samples while other m6A genes highly expressed in wild-type ALKBH5, HNRNPC, METTL14, YTHDC1, and YTHDC2.
Different m6A Modification Patterns Mediated by 23 m6A Genes and Its Clinical Relevance
Based on the expression levels of the 23 m6A genes, we classified the PRCC patients by carrying out unsupervised clustering analysis (Supplementary Figures S2A–E). We finally identified three patterns, termed as m6A expression clusters A, B and C, which included 56 cases in m6A expression cluster A, 128 cases in m6A expression cluster B, and 127 cases in m6A expression cluster C (Figure 2A). Then, we determined the prognostic values of the three m6A modification patterns. According to this analysis, m6A expression cluster A showed the most favorable survival (Figure 2B). After combing the TCGA and GEO data sets for comprehensive clinical data from PRCC patients, we made a heat map to visualize the correlation between the three m6A expression clusters and clinical characteristics. As shown in the Figure 2A, m6A expression cluster C was associated with poor prognosis and enriched in metastatic tumors as well as being associated with patient old age. By comparison, m6A expression clusters A and B showed relatively better prognose. We also noted that 23 m6A-related genes had relatively high expression levels in m6A expression cluster C, followed by m6A expression clusters B and A (Figure 2C).
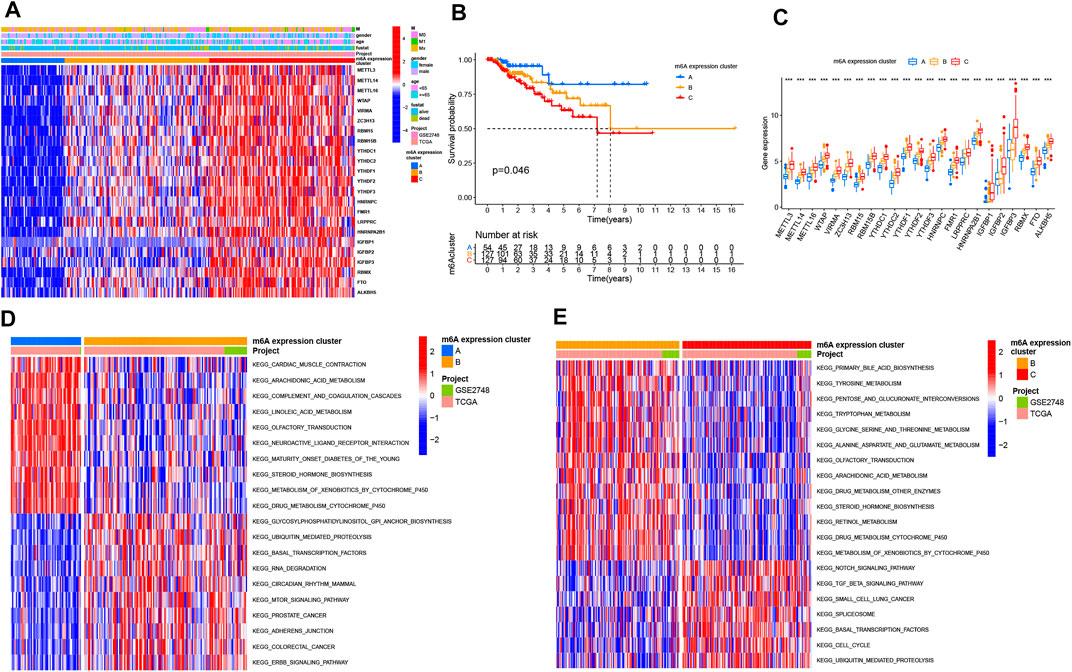
FIGURE 2. Distinct m6A modification patterns and biological features of each cluster. (A) Unsupervised clustering of 23 m6A genes in TCGA PRCC and GSE2748 data sets. The m6A expression cluster, tumor M stage, survival status, gender, and age were used as annotations. Red means high expression of m6A genes, and blue represents low expression. The M means metastasis. (B) Kaplan–Meier survival analysis showing the OS for the three m6A expression clusters based on TCGA PRCC and GSE2748 data sets. A log-rank test was performed. (C) Expression pattern of 23 m6A genes among three m6A expression clusters. The upper and lower ends of the boxes mean interquartile range (IQR) of the values. The lines in the boxes represent each the median value. The asterisks represent the p value. (*p < .05; **p < .01; ***p < .001) (D,E) GSVA enrichment analysis depicting the activation states of the biological processes in three m6A expression clusters. In the heat map, red means activated pathways, and blue means inhibited pathways. (D) m6A expression clusters B vs. A (E) m6A expression clusters B vs. C.
Biological and TME Cell Infiltration Characteristics in Three m6A Modification Patterns
To investigate the biological processes associated with the three types of m6A modification patterns, we performed a GSVA analysis. The m6A expression cluster A was found to be associated with immune activation processes, such as complement and coagulation cascades. The m6A expression cluster B was found to be associated with oncogenic and stromal signaling pathways, including mTOR signaling pathways, ERBB signaling pathways, and adherens junction. The m6A expression cluster C was also found to be related with immune-related pathways, such as the Notch signaling pathway (Figures 2D,E). Then, we explored the TME cell infiltration for the different m6A expression clusters. The ssGSEA analysis presented that activated CD8+ T cells, myeloid-derived suppressor cells, and several innate immune cells, such as macrophages and monocytes, were enriched in m6A expression cluster A (Figure 3A). Moreover, m6A expression cluster C was associated with natural killer cells, plasmacytoid dendritic cells, and type 2 T helper cells. Afterward, we determined the proportion of immune cells in the three m6A expression clusters by using the CIBERSORT algorithm (Figure 3B). However, a significant difference between the different immune cells was not observed. Finally, we used principal component analysis (PCA), which verified significant differences between the three distinct clusters of PRCC patients (Figure 3C).
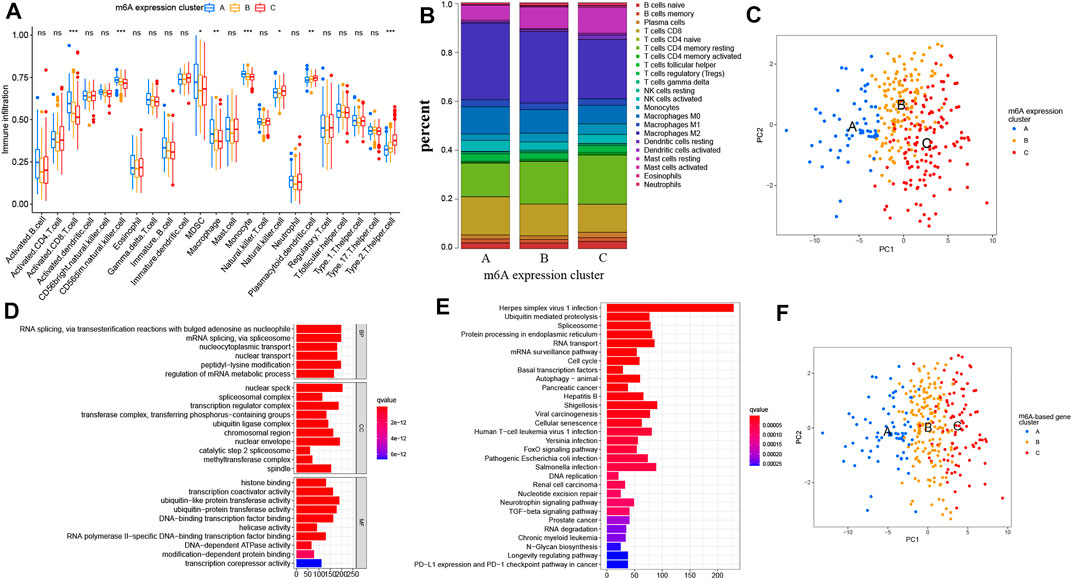
FIGURE 3. Characteristics of TME cell infiltration in diverse m6A expression clusters and the construction of m6A gene signatures. (A) Abundance of TME-related immune cells in three m6A patterns. The histogram shows the expression difference of 23 kinds of immune cells between m6A expression cluster A, B, and C. The upper and lower ends of the boxes mean interquartile range (IQR) of the values. The lines in the boxes represent each the median value. The asterisks represent the p value. (*p < .05; **p < .01; ***p < .001) (B) Proportion of different TME-related immune cells in the three m6A patterns as analyzed by CIBERSORT. (C) Principal component analysis of PRCC patients in three m6A expression clusters, which indicates a remarkable difference between the different modification patterns. (D,E) Functional analysis for m6A-related genes. (D) GO enrichment analysis. (E) KEGG enrichment analysis. MF means molecular function, CC means cellular component, and BP means biological process. (F) Principal component analysis of PRCC patients for the three m6A-based gene expression clusters. The left part of each figure indicates the biological functions and signaling pathways. The degree of enrichment is represented by the color depth of each bar plot.
Model and Biological Characteristics of the m6A Regulators
To further describe the features of the three m6A expression clusters, we identified 4780 intersected m6A DEGs among the three clusters (Supplementary Figure S2E). Afterward, we analyzed these phenotype-related genes by carrying out KEGG and GO enrichment analyses. The GO analysis revealed a significant enrichment (FDR <0.01) of the methyltransferase complex, RNA methyltransferase activity, and activation of innate immune response (Figure 3D and Supplementary Table S1). The KEGG pathway analysis also indicated that RCC, PD-L1 expression, and the PD-1 checkpoint pathway in cancer were enriched in these selected m6A DEGs (Figure 3E and Supplementary Table S2). The above analysis further confirmed the pivotal role played by m6A modification in immune regulation as well as RCC. Next, univariate Cox regression analysis was carried out to determine the prognosis-related m6A genes. Here, 1285 prognosis-related m6A regulators were extracted for unsupervised clustering analysis. With the optimal k = 3, three genomic clusters were constructed and named m6A-based gene expression clusters A–C (Supplementary Figures S3A–E). A PCA analysis found difference between these three m6A-based gene expression clusters as well (Figure 3F). Once again, these results confirmed that diverse m6A modification patterns occurred for PRCC.
To determine the clinical relevance of these clusters, we evaluated the healthy status among the three m6A-based gene expression clusters. The m6A-based gene cluster C showed a worse prognosis than did m6A-based gene expression clusters A and B (Figure 4A). As shown in Figure 4B, m6A-based gene expression cluster C was mainly enriched in metastatic tumors. However, the other clusters were related with alive status as well as nonmetastatic tumor (Figure 4B). The results of the differential analysis of the three clusters validated the pattern of m6Agene signatures as well (Figure 4C).
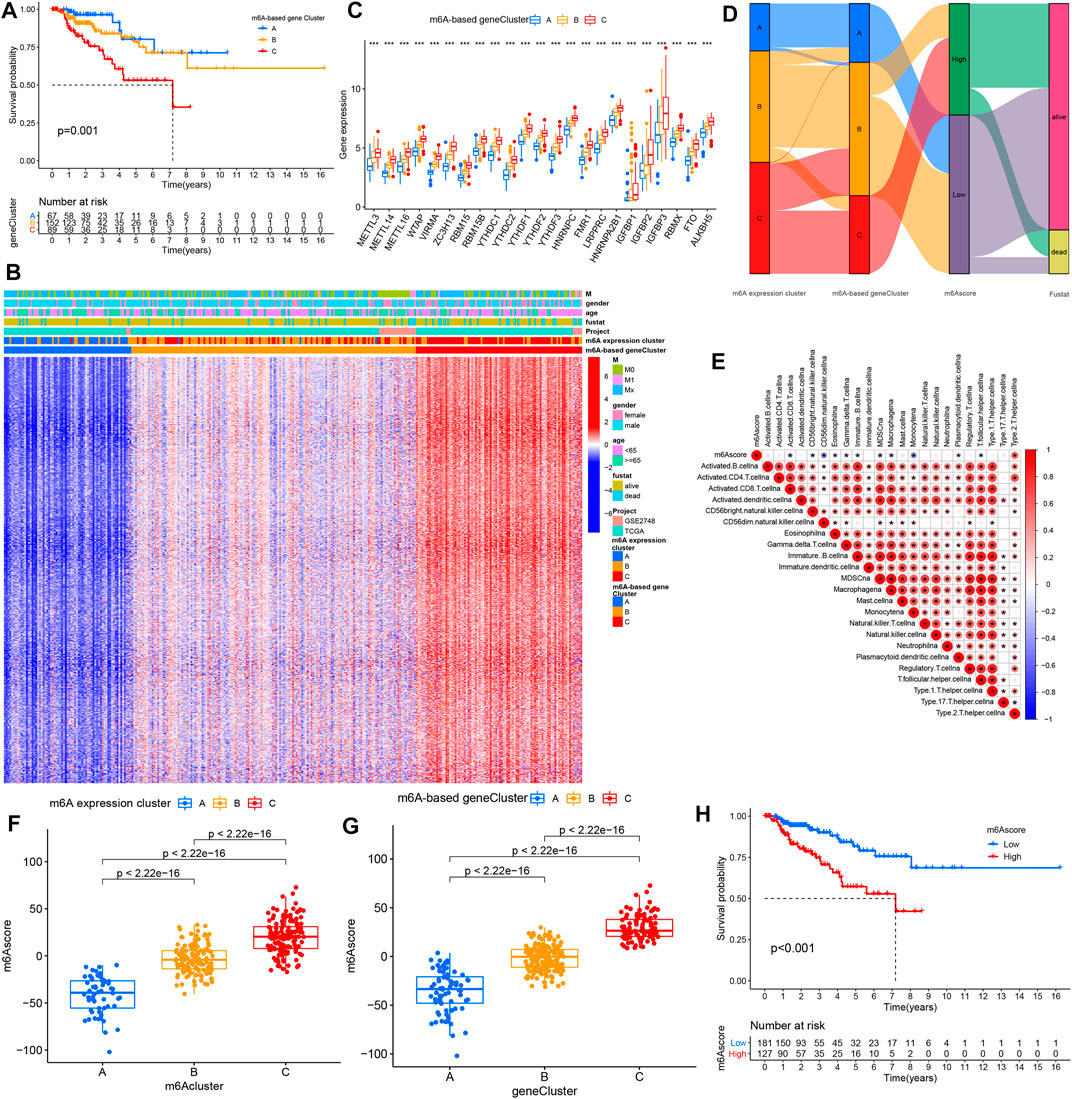
FIGURE 4. Characteristics of diverse m6A-based gene expression clusters. (A) Kaplan–Meier survival analysis showed the OS for the three m6A-based gene expression clusters based on TCGA PRCC and GSE2748 data set with PRCC. (B) Unsupervised clustering of the intersected m6A phenotype-related genes in PRCC, which classifies patients into several clusters, termed m6A-based gene expression clusters. The m6A cluster, tumor M stage, survival status, gender, and age are used as annotations. Red means high expression of m6A genes, and blue represents low expression. The M means metastasis. (C) Expression pattern of 23 m6A genes for the three m6A-based gene expression clusters. The histogram indicates the expression level of 23 m6A genes between m6A-based gene cluster A, B, and C. The upper and lower ends of the boxes mean each the interquartile range (IQR) of values. The lines in the boxes represent median value. The asterisks represented the p value. (*p < .05; **p < .01; ***p < .001). (D) Alluvial diagram displaying the differences in m6A expression clusters, m6A-based gene expression clusters, and m6Ascore. (E) Spearman analyses of the correlations between m6Ascore and biological characteristics in the PRCC cohort. (F,G) Differential analysis of m6A score values among (F) m6A expression clusters and (G) m6A-based gene expression clusters in TCGA PRCC and GSE2748 data sets. (H) K–M analyses for the OS of PRCC patients in high and low m6A score groups.
Evaluation of the m6A Modification Patterns Among the m6A Regulator Signatures
We employed m6A score (a scoring methodology) to quantify and evaluate m6A modification patterns. Alterations of each of the PRCC patient’s attributes were visualized by producing and inspecting an alluvial diagram. The results suggest that most of the PRCC samples showing the m6A-based gene expression cluster C were marked with a high m6A score and showed poor patient survival (Figure 4D). Then, we assessed the correlations between m6A score and biological processes. The m6A score was only positively associated with processes involving type 2 T helper cells but negatively correlated with processes involving other immune cells (Figure 4E). Significant differences in the m6A score were observed between the three m6A-based gene expression clusters as well as between the m6A expression clusters. Both of these results presented that m6A expression cluster C and m6A-based gene expression cluster C have the highest m6Ascore (Figures 4F,G). Afterward, PRCC patients were divided into two distinct groups with an optimal cutoff value. As shown in Figure 4H, patients with low m6A scores showed relatively good survival compared with the high m6A score group.
The m6A Modification Model in the Role of Tumor Somatic Mutation and Immunotherapy
We also analyzed and visualized the somatic mutation profiles of PRCC patients of the high and low m6A score groups by using the “maftools” package. Compared with the high m6A score set, the low m6A score group showed a higher percentage of somatic mutations (Figures 5A,B). A previous study shows an association of high TMB with better survival for most cancers (Xie et al., 2020). Still, a high TMB could improve the prognosis for patients treated with ICIs (Samstein et al., 2019). Considering the significant role of TMB, we tested its prognosis value for PRCC. As observed in the survival plot, the high-TMB set presented improved survival (Figure 5C). Moreover, we found the worst survival for the PRCC patients with both a low-TMB and high m6A score (Figure 5D). The above outcome implies that TMB as well as m6A score could potentially be used as predictive biomarkers.
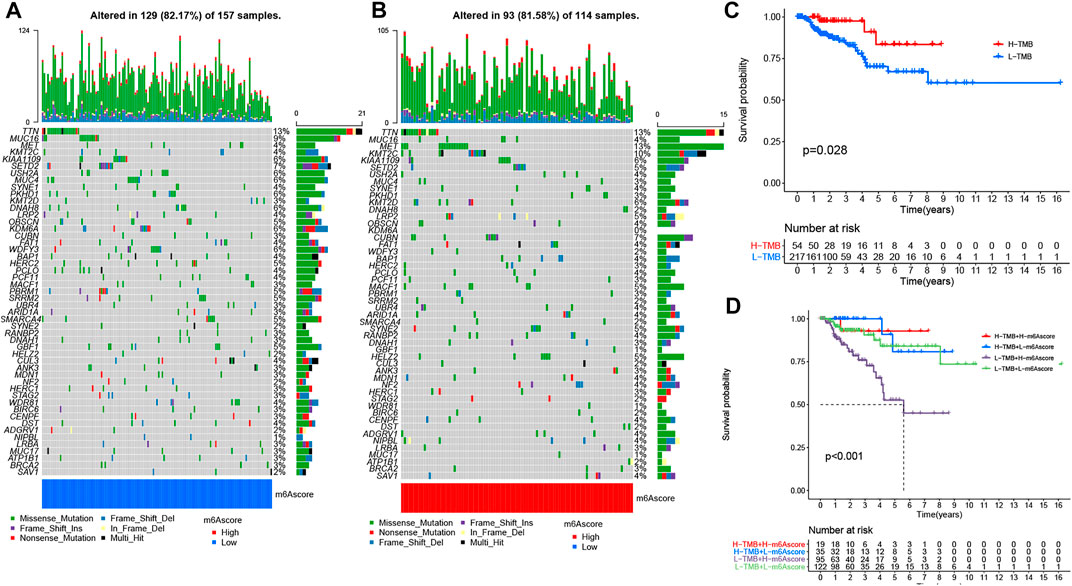
FIGURE 5. The changes of somatic mutations among distinct m6A score groups. (A,B) Waterfall plot showing The changes of somatic mutations in (A) the low-m6A score set and (B) high-m6A score group. The bar plot at the upper part of the figure shows TMB. The numbers on the right part of the figure represent the mutation frequency. (C) Survival analysis for the OS of patients in high- and low-TMB groups. (D) K-M analysis for PRCC patients stratified by both m6A score and TMB. MSI-H, high microsatellite instability; MSI-L, low microsatellite instability.
Next, we interrogated the clinical value of the m6A modification model in immunotherapy (including PD-1 blockade and mTOR inhibitor). In the PD-1 blockade cohort (GSE78220), patients with low m6A scores showed improved overall survival (OS) (Figure 6A). In addition, in the anti-mTOR group, there was a significant difference in OS as well as progression free survival (PFS) between low and high m6A score groups. The therapeutic advantages of the mTOR inhibitor was observed in the low m6A score group (Figures 6B,C). Moreover, in light of unsatisfactory outcomes from tumor therapy, we queried whether m6A score could affect the therapeutic efficacy. The poor outcome of overall response rate and clinical benefit was correlated with high m6A score (Figures 6D,E). Finally, we used the m6A score to predict the reaction to immunotherapy efficacy. After downloading the immunotherapy fraction data from the Cancer Immunome Database (TCIA), we compared the predictive abilities of the m6A scores of the two m6A score groups. Patients with low m6A score values showed significantly better reactions to anti-CTLA-4 and anti-PD-1 therapy (Figures 6F–I).
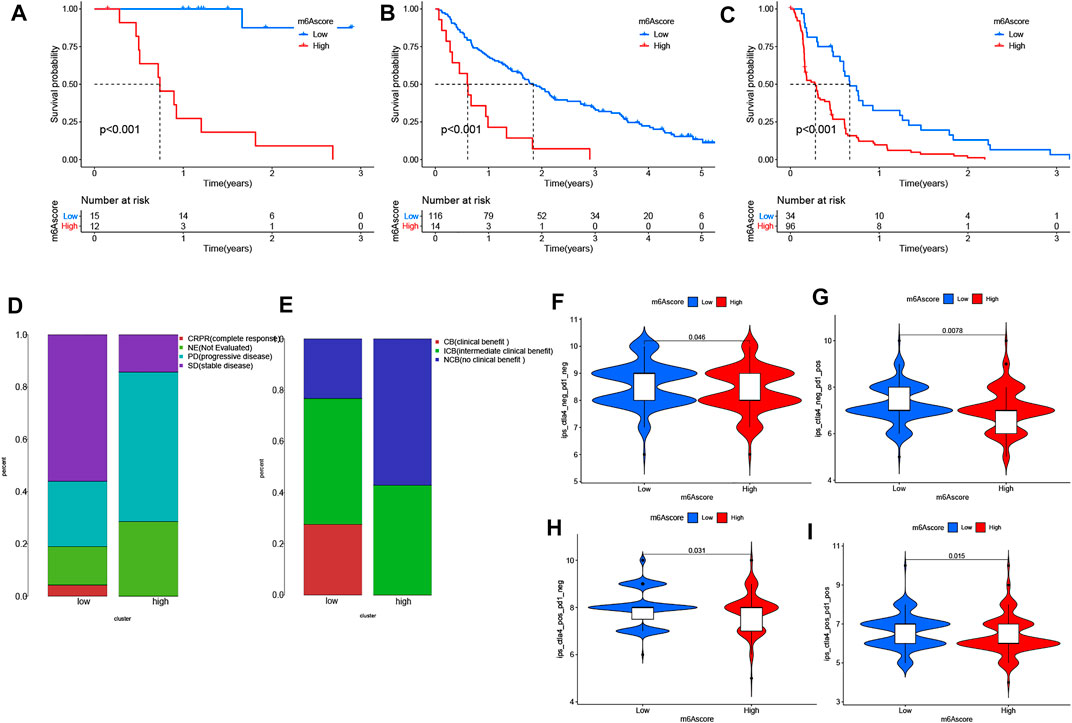
FIGURE 6. The role of distinct m6A modification patterns in immunotherapy. (A–C) Results showing the associations of m6A score was negatively associated with OS and PFS following (A,B) anti-PD-1 therapy or (C) use of mTOR inhibitors. Negative associations were observed in both cases. (D,E) Proportions of PRCC patients with (D) an immunotherapy response and (E) clinical benefit in the two m6Ascore sets. (F–I) Relationship between m6A score and immunotherapeutic response under PD-1 and CTLA-4 expressions: (F) negative PD-1 and CTLA-4. (G) positive PD-1 and negative CTLA-4. (H) positive CTLA-4 and negative PD-1. (I) positive PD-1 and CTLA-4.
Biological Validation of Significant m6A Regulators and Immune Cell Markers
The robustness of m6A regulators as biomarkers was verified using primary PRCC clinical samples from the Shandong Provincial Hospital affiliated with Shandong First Medical University. We selected six m6A genes from the DEGs and five immune cell markers for the following validation. The IHC images acquired of immune cell markers showed weak staining for CD8, CD69, and CD163 in normal renal tissue (Figures 7G–I). Tumor tissue staining of YTHDF1 and HNRNPA2B1 showed moderate staining in the nucleus, and negative staining was observed in the normal tissues (Figures 7A,D). In normal kidney samples, moderate staining for ZC3H13 and YTHDF2 were observed in the nucleus. Regarding the YTHDF3 and IGFBP2, strong staining was positive on the cytoplasm (Figures 7B,C,E,F). However, weak staining patterns for ZC3H13, YTHDF2, YTHDF3, and IGFBP2 were observed in PRCC tissues (Figures 7B,C,E,F). These unique IHC staining patterns further confirmed the above results and illustrated that these selected m6A regulators could be used to predict clinical outcomes.
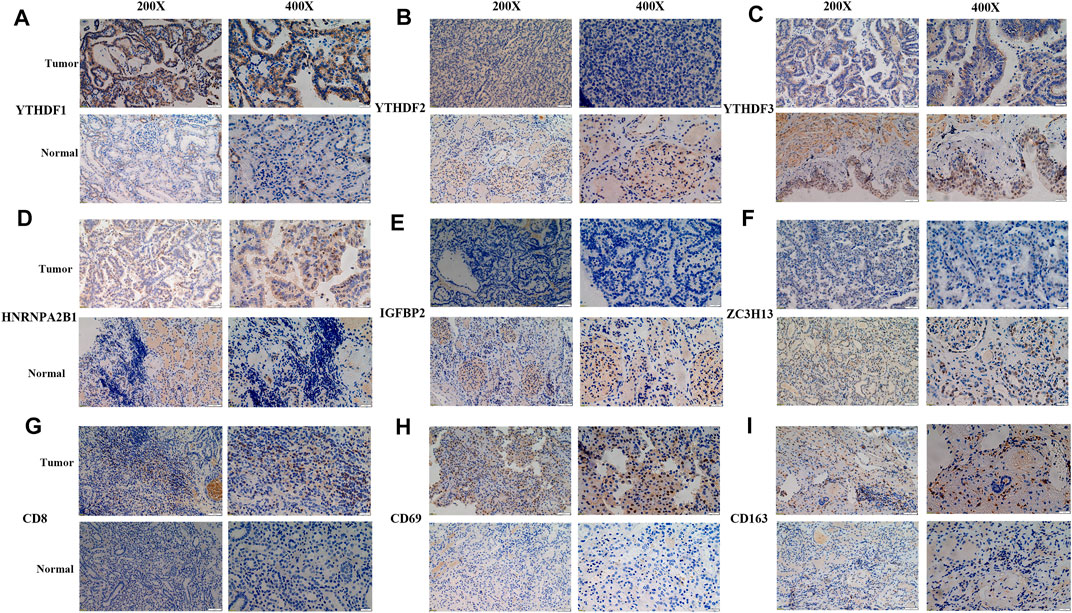
FIGURE 7. Representative IHC images of significant m6A regulators and immune cell markers in PRCC samples. (A–F) IHC patterns for selected m6A regulators in normal and tumor samples. (G–I) IHC patterns for three immune cell markers in normal and tumor samples. Bar, 50 and 200 μm.
Discussion
The m6A modification plays a pivotal role in tumorigenesis, tumor development, progression, and prognosis (He et al., 2019). Previous studies show the m6A modification displaying dual suppressive and promotive functions in various tumors (He et al., 2018; Wang et al., 2018). However, there are few studies of the m6A modification for RCC (especially PRCC) initiation, progression, and therapy. The TME is a potential regulator of cancer progression and a source of therapeutic targets. In the complex TME, immune and stromal cells play significant roles in cancer development (Quail and Joyce, 2013; Ho et al., 2020). Currently, knowledge of the kidney TME is restricted to only a few different tumor types and lacks comprehensive analysis. Therefore, in this study, we focused our attention on the role of m6A modification in the TME of PRCC and aimed to unravel the potential functions of this modification and contribute to obtaining a deeper understanding of antitumor immune effects of the TME in PRCC.
CNV is one of the most important somatic aberrations in cancer, and several studies find significant associations between CNVs and cancers (Speleman et al., 2008; Shlien and Malkin, 2009; Beroukhim et al., 2010). Based on 23 m6A genes and PRCC copy-number profiles, we explored the alteration of m6A genes in PRCC. The mutations of the m6A regulators occurred relatively infrequently in PRCC, but CNV deletion was a common event. Then, on the basis of clustering analysis, we identified three different m6A expression clusters in PRCC. In 2017, Chen DS et al. proposed three types of cancer-immune phenotypes, namely, immune-inflamed, immune-excluded, and immune-desert phenotypes (Speleman et al., 2008; Shlien and Malkin, 2009; Beroukhim et al., 2010). The immune-inflamed phenotype is characterized by the presence of CD4+ T, CD8+ T, myeloid, and monocytc cells in the TME, which is positioned near the tumor cells (Herbst et al., 2014; Turley et al., 2015). The immune-excluded phenotype also involves the presence of many immune cells, but with these cell, located mainly surrounding the stroma instead of the nest of the tumor (Joyce and Fearon, 2015; Hegde et al., 2016). The immune-desert phenotype presents a paucity of CD8+ T cells in both tumor parenchyma and stroma with this paucity being a feature of a noninflamed TME (Gajewski et al., 2013; Kim and Chen, 2016). In our current study, we found an enrichment of activated CD8+ T cells, myeloid-derived suppressor cells, macrophages, and monocytes in m6A expression cluster A, an association of the m6A expression cluster B with adherens junction, and m6A expression cluster C showing the presence of natural killer and plasmacytoid dendritic cells. Due to the presence of CD8 expressing T cells and other myeloid cells as well as monocytes, the m6A expression cluster A showed improved survival.
Then, we identified the intersected DEGs between diverse m6A expression clusters and assessed the potential biological functions of these genes and the pathways used by them. Our results show a significant enrichment of these DEGs in m6A-, immune- and immunotherapy-related biological functions and pathways. Moreover, we chose T cell (CD8, CD69) and macrophage markers (CD163) as well as differentially expressed m6A regulators to validate the clinical application using primary PRCC samples from our hospital, and the results further confirm the prognostic value in clinical application. To limit the individual heterogeneity, we utilized m6A score to quantify and evaluate m6A modification patterns. Similar to the results of previous research, the m6A expression cluster C and m6A expression cluster A in the current work presented, respectively, the highest and lowest m6A score in PRCC. The K-M survival curve illustrates a better OS and better prognosis associated with m6A-based gene expression cluster A than with m6A-based gene expression cluster C. These results suggest that the m6A scoring system could be applied to determine distinct immune phenotypes and m6A modification patterns.
Somatic mutation was detected between high- and low-m6A score groups as well. The low m6A score group had a high TMB with high TMB associated with better survival for PRCC patients. A similar trend was found in studies involving melanoma and osteosarcoma (Aoude et al., 2020; Xie et al., 2020). Still, a high TMB appears to indicate a better prognosis for patients receiving ICIs for treating various types of tumors (Snyder et al., 2014; Rizvi et al., 2015; Van Allen et al., 2015; Rosenberg et al., 2016). These findings suggest better immunotherapeutic outcomes for the low m6A score group than for the high m6A score group. In light of the disappointing outcomes from immunotherapy (including anti-PD-1 therapy and mTOR inhibitors) to date (Larkin et al., 2015; Postow et al., 2015; Rotte et al., 2018; de Vries-Brilland et al., 2020), we sought to determine whether m6A score could serve as a biomarker to stratify patients with different levels of immune-responsiveness to tumors. By utilizing GSE78220 (PD-1 blockade cohort) and the anti-mTOR group (Hugo et al., 2016; Braun et al., 2020), we showed an association between a low m6A score and improve OS and PFS time. Thus, distinct m6A modification patterns may impact the efficacy of immunotherapy, and m6A score has potential clinical value in evaluating the efficacy of therapeutic.
To improve the outcome for PRCC patients, access to accurate and efficient biomarkers is indispensable. Therefore, we investigated the TME and m6A-related genes to reveal the associated immune cells and molecular mechanism as well as clinical value. This investigation suggests that diverse m6A modification patterns could affect the complexity of the PRCC TME. Moreover, the established m6A score was indicated by our results to have great potential as a predictive indicator to assess the distinct m6A modification patterns and prognose of PRCC patients. More importantly, given the high variety of responses to immunotherapy, the m6A score may be utilized to evaluate how tumors might react to being exposed to an immunotherapy (including anti-PD-1 therapy and mTOR inhibitors). We do note that the relatively small number of PRCC patients receiving immunotherapy may affect the predictive ability of m6A score. Therefore, in future investigations, expression data and clinical information from our medical center will be collected. Further experiments in vivo and in vitro will also be implemented to confirm the molecular mechanism of m6A-related regulators in the PRCC TME. Nevertheless, the study we carried out has enhanced our understanding of TME characteristics and improved the therapeutic landscape for PRCC patients.
Data Availability Statement
The original contributions presented in the study are included in the article/Supplementary Material, further inquiries can be directed to the corresponding authors.
Ethics Statement
The studies involving human participants were reviewed and approved by the Ethics Committee of Shandong Provincial Hospital. The patients/participants provided their written informed consent to participate in this study.
Author Contributions
BZ and WH contributed to the study conception; FC, BZ, JW, ZY, YZ, and ZC conducted the data analysis and were responsible for writing the first draft of the paper. WH, FC, and ZN revised the paper; and all authors read and approved the final version of the manuscript.
Conflict of Interest
The authors declare that the research was conducted in the absence of any commercial or financial relationships that could be construed as a potential conflict of interest.
Publisher’s Note
All claims expressed in this article are solely those of the authors and do not necessarily represent those of their affiliated organizations, or those of the publisher, the editors and the reviewers. Any product that may be evaluated in this article, or claim that may be made by its manufacturer, is not guaranteed or endorsed by the publisher.
Supplementary Material
The Supplementary Material for this article can be found online at: https://www.frontiersin.org/articles/10.3389/fcell.2022.818194/full#supplementary-material
Abbreviations
ccRCC, clear cell renal cell carcinoma; CNV, Copy number variation; DEGs, Differentially expressed genes; GEO, Gene-Expression Omnibus; GSVA, Gene set variation analysis; ICI, Immunological checkpoint inhibitor; m6A, N6-methyladenosine; PCA, Principal component analysis; PRCC, papillary renal cell carcinoma; ssGSEA, Single-sample gene-set enrichment analysis; TCGA, The Cancer Genome Atlas; TCIA, The Cancer Immunome Atlas; TMB, Tumor mutation burden; TME, tumor microenvironment.
References
Aoude, L. G., Bonazzi, V. F., Brosda, S., Patel, K., Koufariotis, L. T., Oey, H., et al. (2020). Pathogenic Germline Variants Are Associated with Poor Survival in Stage III/IV Melanoma Patients. Sci. Rep. 10 (1), 17687. doi:10.1038/s41598-020-74956-3
Barata, P. C., and Rini, B. I. (2017). Treatment of Renal Cell Carcinoma: Current Status and Future Directions. CA: a Cancer J. clinicians 67 (6), 507–524. doi:10.3322/caac.21411
Barbie, D. A., Tamayo, P., Boehm, J. S., Kim, S. Y., Moody, S. E., Dunn, I. F., et al. (2009). Systematic RNA Interference Reveals that Oncogenic KRAS-Driven Cancers Require TBK1. Nature 462 (7269), 108–112. doi:10.1038/nature08460
Beroukhim, R., Mermel, C. H., Porter, D., Wei, G., Raychaudhuri, S., Donovan, J., et al. (2010). The Landscape of Somatic Copy-Number Alteration across Human Cancers. Nature 463 (7283), 899–905. doi:10.1038/nature08822
Binnewies, M., Roberts, E. W., Kersten, K., Chan, V., Fearon, D. F., Merad, M., et al. (2018). Understanding the Tumor Immune Microenvironment (TIME) for Effective Therapy. Nat. Med. 24 (5), 541–550. doi:10.1038/s41591-018-0014-x
Braun, D. A., Hou, Y., Bakouny, Z., Ficial, M., Sant’ Angelo, M., Forman, J., et al. (2020). Interplay of Somatic Alterations and Immune Infiltration Modulates Response to PD-1 Blockade in Advanced clear Cell Renal Cell Carcinoma. Nat. Med. 26 (6), 909–918. doi:10.1038/s41591-020-0839-y
Charoentong, P., Finotello, F., Angelova, M., Mayer, C., Efremova, M., Rieder, D., et al. (2017). Pan-cancer Immunogenomic Analyses Reveal Genotype-Immunophenotype Relationships and Predictors of Response to Checkpoint Blockade. Cel Rep. 18 (1), 248–262. doi:10.1016/j.celrep.2016.12.019
Chen, D. S., and Mellman, I. (2017). Elements of Cancer Immunity and the Cancer-Immune Set point. Nature 541 (7637), 321–330. doi:10.1038/nature21349
Chen, M., Wei, L., Law, C.-T., Tsang, F. H.-C., Shen, J., Cheng, C. L.-H., et al. (2018). RNA N6-Methyladenosine Methyltransferase-like 3 Promotes Liver Cancer Progression through YTHDF2-dependent Posttranscriptional Silencing of SOCS2. Hepatology 67 (6), 2254–2270. doi:10.1002/hep.29683
de Vries-Brilland, M., Gross-Goupil, M., Seegers, V., Boughalem, E., Beuselinck, B., Thibault, C., et al. (2020). Are Immune Checkpoint Inhibitors a Valid Option for Papillary Renal Cell Carcinoma? A Multicentre Retrospective Study, 136. Oxford, United Kingdom: European journal of cancer, 76–83.
Gajewski, T. F., Woo, S.-R., Zha, Y., Spaapen, R., Zheng, Y., Corrales, L., et al. (2013). Cancer Immunotherapy Strategies Based on Overcoming Barriers within the Tumor Microenvironment. Curr. Opin. Immunol. 25 (2), 268–276. doi:10.1016/j.coi.2013.02.009
Gu, Y., Wu, X., Zhang, J., Fang, Y., Pan, Y., Shu, Y., et al. (2021). The Evolving Landscape of N6-Methyladenosine Modification in the Tumor Microenvironment. Mol. Ther. 29 (5), 1703–1715. doi:10.1016/j.ymthe.2021.04.009
Han, D., Liu, J., Chen, C., Dong, L., Liu, Y., Chang, R., et al. (2019). Anti-tumour Immunity Controlled through mRNA m6A Methylation and YTHDF1 in Dendritic Cells. Nature 566 (7743), 270–274. doi:10.1038/s41586-019-0916-x
Hänzelmann, S., Castelo, R., and Guinney, J. (2013). GSVA: Gene Set Variation Analysis for Microarray and RNA-Seq Data. BMC bioinformatics 14, 7. doi:10.1186/1471-2105-14-7
He, L., Li, H., Wu, A., Peng, Y., Shu, G., and Yin, G. (2019). Functions of N6-Methyladenosine and its Role in Cancer. Mol. Cancer 18 (1), 176. doi:10.1186/s12943-019-1109-9
He, L., Li, J., Wang, X., Ying, Y., Xie, H., Yan, H., et al. (2018). The Dual Role of N6‐methyladenosine Modification of RNAs Is Involved in Human Cancers. J. Cel Mol Med 22 (10), 4630–4639. doi:10.1111/jcmm.13804
Hegde, P. S., Karanikas, V., and Evers, S. (2016). The where, the when, and the How of Immune Monitoring for Cancer Immunotherapies in the Era of Checkpoint Inhibition. Clin. Cancer Res. 22 (8), 1865–1874. doi:10.1158/1078-0432.ccr-15-1507
Herbst, R. S., Soria, J.-C., Kowanetz, M., Fine, G. D., Hamid, O., Gordon, M. S., et al. (2014). Predictive Correlates of Response to the Anti-PD-L1 Antibody MPDL3280A in Cancer Patients. Nature 515 (7528), 563–567. doi:10.1038/nature14011
Ho, W. J., Jaffee, E. M., and Zheng, L. (2020). The Tumour Microenvironment in Pancreatic Cancer - Clinical Challenges and Opportunities. Nat. Rev. Clin. Oncol. 17 (9), 527–540. doi:10.1038/s41571-020-0363-5
Hugo, W., Zaretsky, J. M., Sun, L., Song, C., Moreno, B. H., Hu-Lieskovan, S., et al. (2016). Genomic and Transcriptomic Features of Response to Anti-PD-1 Therapy in Metastatic Melanoma. Cell 165 (1), 35–44. doi:10.1016/j.cell.2016.02.065
Joyce, J. A., and Fearon, D. T. (2015). T Cell Exclusion, Immune Privilege, and the Tumor Microenvironment. Science 348 (6230), 74–80. doi:10.1126/science.aaa6204
Ke, S., Pandya-Jones, A., Saito, Y., Fak, J. J., Vågbø, C. B., Geula, S., et al. (2017). m6A mRNA Modifications Are Deposited in Nascent Pre-mRNA and Are Not Required for Splicing but Do Specify Cytoplasmic turnoverA mRNA Modifications Are Deposited in Nascent Pre-mRNA and Are Not Required for Splicing but Do Specify Cytoplasmic Turnover. Genes Dev. 31 (10), 990–1006. doi:10.1101/gad.301036.117
Kim, J. M., and Chen, D. S. (2016). Immune Escape to PD-L1/pd-1 Blockade: Seven Steps to success (Or Failure). Ann. Oncol. 27 (8), 1492–1504. doi:10.1093/annonc/mdw217
Larkin, J., Chiarion-Sileni, V., Gonzalez, R., Grob, J. J., Cowey, C. L., Lao, C. D., et al. (2015). Combined Nivolumab and Ipilimumab or Monotherapy in Untreated Melanoma. N. Engl. J. Med. 373 (1), 23–34. doi:10.1056/nejmoa1504030
Li, M., Zha, X., and Wang, S. (2021). The Role of N6-Methyladenosine mRNA in the Tumor Microenvironment. Biochim. Biophys. Acta (Bba) - Rev. Cancer 1875 (2), 188522. doi:10.1016/j.bbcan.2021.188522
Liberzon, A., Subramanian, A., Pinchback, R., Thorvaldsdóttir, H., Tamayo, P., and Mesirov, J. P. (2011). Molecular Signatures Database (MSigDB) 3.0. Bioinformatics 27 (12), 1739–1740. doi:10.1093/bioinformatics/btr260
Linehan, W. M., Linehan, W. M., Spellman, P. T., Ricketts, C. J., Creighton, C. J., Fei, S. S., et al. (2016). Comprehensive Molecular Characterization of Papillary Renal-Cell Carcinoma. N. Engl. J. Med. 374 (2), 135–145. doi:10.1056/NEJMoa1505917
Mayakonda, A., Lin, D.-C., Assenov, Y., Plass, C., and Koeffler, H. P. (2018). Maftools: Efficient and Comprehensive Analysis of Somatic Variants in Cancer. Genome Res. 28 (11), 1747–1756. doi:10.1101/gr.239244.118
Postow, M. A., Chesney, J., Pavlick, A. C., Robert, C., Grossmann, K., McDermott, D., et al. (2015). Nivolumab and Ipilimumab versus Ipilimumab in Untreated Melanoma. N. Engl. J. Med. 372 (21), 2006–2017. doi:10.1056/nejmoa1414428
Qi, S. T., Ma, J. Y., Wang, Z. B., Guo, L., Hou, Y., Sun, Q. Y., et al. (2016). N6-Methyladenosine Sequencing Highlights the Involvement of mRNA Methylation in Oocyte Meiotic Maturation and Embryo Development by Regulating Translation in Xenopus laevis. J. Biol. Chem. 291 (44), 23020–23026. doi:10.1074/jbc.m116.748889
Quail, D. F., and Joyce, J. A. (2013). Microenvironmental Regulation of Tumor Progression and Metastasis. Nat. Med. 19 (11), 1423–1437. doi:10.1038/nm.3394
Rizvi, N. A., Hellmann, M. D., Snyder, A., Kvistborg, P., Makarov, V., Havel, J. J., et al. (2015). Mutational Landscape Determines Sensitivity to PD-1 Blockade in Non-small Cell Lung Cancer. Science 348 (6230), 124–128. doi:10.1126/science.aaa1348
Rosenberg, J. E., Hoffman-Censits, J., Powles, T., van der Heijden, M. S., Balar, A. V., Necchi, A., et al. (2016). Atezolizumab in Patients with Locally Advanced and Metastatic Urothelial Carcinoma Who Have Progressed Following Treatment with Platinum-Based Chemotherapy: a Single-Arm, Multicentre, Phase 2 Trial. The Lancet 387 (10031), 1909–1920. doi:10.1016/s0140-6736(16)00561-4
Rotte, A., Jin, J. Y., and Lemaire, V. (2018). Mechanistic Overview of Immune Checkpoints to Support the Rational Design of Their Combinations in Cancer Immunotherapy. Ann. Oncol. 29 (1), 71–83. doi:10.1093/annonc/mdx686
Samstein, R. M., Lee, C.-H., Shoushtari, A. N., Hellmann, M. D., Shen, R., Janjigian, Y. Y., et al. (2019). Tumor Mutational Load Predicts Survival after Immunotherapy across Multiple Cancer Types. Nat. Genet. 51 (2), 202–206. doi:10.1038/s41588-018-0312-8
Shlien, A., and Malkin, D. (2009). Copy Number Variations and Cancer. Genome Med. 1 (6), 62. doi:10.1186/gm62
Snyder, A., Makarov, V., Merghoub, T., Yuan, J., Zaretsky, J. M., Desrichard, A., et al. (2014). Genetic Basis for Clinical Response to CTLA-4 Blockade in Melanoma. N. Engl. J. Med. 371 (23), 2189–2199. doi:10.1056/nejmoa1406498
Sotiriou, C., Wirapati, P., Loi, S., Harris, A., Fox, S., Smeds, J., et al. (2006). Gene Expression Profiling in Breast Cancer: Understanding the Molecular Basis of Histologic Grade to Improve Prognosis. J. Natl. Cancer Inst. 98 (4), 262–272. doi:10.1093/jnci/djj052
Speleman, F., Kumps, C., Buysse, K., Poppe, B., Menten, B., and De Preter, K. (2008). Copy Number Alterations and Copy Number Variation in Cancer: Close Encounters of the Bad Kind. Cytogenet. Genome Res. 123 (1-4), 176–182. doi:10.1159/000184706
Steffens, S., Janssen, M., Roos, F. C., Becker, F., Schumacher, S., Seidel, C., et al. (19902012)., 48. Oxford, England, 2347–2352. doi:10.1016/j.ejca.2012.05.002Incidence and Long-Term Prognosis of Papillary Compared to clear Cell Renal Cell Carcinoma-Aa Multicentre StudyEur. J. Cancer15
Syn, N. L., Teng, M. W. L., Mok, T. S. K., and Soo, R. A. (2017). De-novo and Acquired Resistance to Immune Checkpoint Targeting. Lancet Oncol. 18 (12), e731–e741. doi:10.1016/s1470-2045(17)30607-1
Turley, S. J., Cremasco, V., and Astarita, J. L. (2015). Immunological Hallmarks of Stromal Cells in the Tumour Microenvironment. Nat. Rev. Immunol. 15 (11), 669–682. doi:10.1038/nri3902
Van Allen, E. M., Miao, D., Schilling, B., Shukla, S. A., Blank, C., Zimmer, L., et al. (2015). Genomic Correlates of Response to CTLA-4 Blockade in Metastatic Melanoma. Science 350 (6257), 207–211. doi:10.1126/science.aad0095
Vuong, L., Kotecha, R. R., Voss, M. H., and Hakimi, A. A. (2019). Tumor Microenvironment Dynamics in Clear-Cell Renal Cell Carcinoma. Cancer Discov. 9 (10), 1349–1357. doi:10.1158/2159-8290.cd-19-0499
Wang, Q., Chen, C., Ding, Q., Zhao, Y., Wang, Z., Chen, J., et al. (2020). METTL3-mediated m6A Modification of HDGF mRNA Promotes Gastric Cancer Progression and Has Prognostic Significance. Gut 69 (7), 1193–1205. doi:10.1136/gutjnl-2019-319639
Wang, S., Chai, P., Jia, R., and Jia, R. (2018). Novel Insights on m6A RNA Methylation in Tumorigenesis: a Double-Edged Sword. Mol. Cancer 17 (1), 101. doi:10.1186/s12943-018-0847-4
Wilkerson, M. D., and Hayes, D. N. (2010). ConsensusClusterPlus: a Class Discovery Tool with Confidence Assessments and Item Tracking. Bioinformatics (Oxford, England) 26 (12), 1572–1573. doi:10.1093/bioinformatics/btq170
Wu, T., and Dai, Y. (2017). Tumor Microenvironment and Therapeutic Response. Cancer Lett. 387, 61–68. doi:10.1016/j.canlet.2016.01.043
Xiao, C.-L., Zhu, S., He, M., Chen, D., Zhang, Q., Chen, Y., et al. (2018). N6-Methyladenine DNA Modification in the Human Genome. Mol. Cel. 71 (2), 306–318. doi:10.1016/j.molcel.2018.06.015
Xie, L., Yang, Y., Guo, W., Che, D., Xu, J., Sun, X., et al. (2020). The Clinical Implications of Tumor Mutational Burden in Osteosarcoma. Front. Oncol. 10, 595527. doi:10.3389/fonc.2020.595527
Yang, Y., Hsu, P. J., Chen, Y.-S., and Yang, Y.-G. (2018). Dynamic Transcriptomic m6A Decoration: Writers, Erasers, Readers and Functions in RNA Metabolism. Cell Res 28 (6), 616–624. doi:10.1038/s41422-018-0040-8
Zeng, D., Li, M., Zhou, R., Zhang, J., Sun, H., Shi, M., et al. (2019). Tumor Microenvironment Characterization in Gastric Cancer Identifies Prognostic and Immunotherapeutically Relevant Gene Signatures. Cancer Immunol. Res. 7 (5), 737–750. doi:10.1158/2326-6066.cir-18-0436
Zhang, B., Wu, Q., Li, B., Wang, D., Wang, L., and Zhou, Y. L. (2020). m6A Regulator-Mediated Methylation Modification Patterns and Tumor Microenvironment Infiltration Characterization in Gastric cancerA Regulator-Mediated Methylation Modification Patterns and Tumor Microenvironment Infiltration Characterization in Gastric Cancer. Mol. Cancer 19 (1), 53. doi:10.1186/s12943-020-01170-0
Keywords: m 6 A, tumor microenvironment, immunotherapy, mutation burden, survival
Citation: Zheng B, Cheng F, Yao Z, Zhang Y, Cong Z, Wang J, Niu Z and He W (2022) Identification of New m6A Methylation Modification Patterns and Tumor Microenvironment Infiltration Landscape that Predict Clinical Outcomes for Papillary Renal Cell Carcinoma Patients. Front. Cell Dev. Biol. 10:818194. doi: 10.3389/fcell.2022.818194
Received: 19 November 2021; Accepted: 11 February 2022;
Published: 17 March 2022.
Edited by:
Ângela Sousa, University of Beira Interior, PortugalReviewed by:
Yan Chun Li, University of Chicago, United StatesXiuli Liu, University of Texas Southwestern Medical Center, United States
Copyright © 2022 Zheng, Cheng, Yao, Zhang, Cong, Wang, Niu and He. This is an open-access article distributed under the terms of the Creative Commons Attribution License (CC BY). The use, distribution or reproduction in other forums is permitted, provided the original author(s) and the copyright owner(s) are credited and that the original publication in this journal is cited, in accordance with accepted academic practice. No use, distribution or reproduction is permitted which does not comply with these terms.
*Correspondence: Zhihong Niu, nzh1789@163.com; Wei He, Hewei@bjmu.edu.cn
†These authors have contributed equally to this work