- 1Biomedical Informatics Research Lab, School of Basic Medicine and Clinical Pharmacy, China Pharmaceutical University, Nanjing, China
- 2Cancer Genomics Research Center, School of Basic Medicine and Clinical Pharmacy, China Pharmaceutical University, Nanjing, China
- 3Big Data Research Institute, China Pharmaceutical University, Nanjing, China
- 4Jiangsu Cancer Hospital, Jiangsu Institute of Cancer Research, The Affiliated Cancer Hospital of Nanjing Medical University, Nanjing, Jiangsu, China
Splicing abnormality resulting from somatic mutations in key splicing factor genes (SFG) has been detected in various cancers. Hence, an in-depth study of splicing factor genes mutations’ impact on pan-cancer is meaningful. This study investigated associations of splicing factor genes mutations with clinical features, tumor progression phenotypes, genomic integrity, anti-tumor immune responses, and immunotherapy response in 12 common cancer types from the TCGA database. Compared to SFG-wildtype cancers, SFG-mutated cancers displayed worse survival prognosis, higher tumor mutation burden and aneuploidy levels, higher expression of immunosuppressive signatures, and higher levels of tumor stemness, proliferation potential, and intratumor heterogeneity (ITH). However, splicing factor genes-mutated cancers showed higher response rates to immune checkpoint inhibitors than splicing factor genes-wildtype cancers in six cancer cohorts. Single-cell data analysis confirmed that splicing factor genes mutations were associated with increased tumor stemness, proliferation capacity, PD-L1 expression, intratumor heterogeneity, and aneuploidy levels. Our data suggest that the mutation in key splicing factor genes correlates with unfavorable clinical outcomes and disease progression, genomic instability, anti-tumor immunosuppression, and increased immunotherapy response in pan-cancer. Thus, the splicing factor genes mutation is an adverse prognostic factor and a positive marker for immunotherapy response in cancer.
Introduction
RNA splicing is a dynamic process in which pre-mRNA exon-intron borders are identified, and the intervening intronic sequences are removed, leaving coding exons ligated to form mature mRNA (Witten and Ule, 2011). Alternative pre-mRNA splicing is a major cause of transcript diversity in mammalian cells, which is orchestrated by the spliceosome, a megadalton complex to generate tissue- and species-specific differentiation patterns (Papasaikas and Valcarcel, 2016). The spliceosome comprises five small nuclear ribonucleoproteins (snRNPs) and numerous auxiliary proteins (Matera and Wang, 2014). There are two classes of introns: U2-type and U12-type. The U2-type accounts for > 99.5% of introns, while the U12-type is responsible for < .5% of introns and resides in around 700–800 genes. The introns are spliced by U2-type- and U12-type-dependent spliceosomes, also known as “major” and “minor” spliceosomes, respectively (Akinyi and Frilander, 2021). Although the functional roles of most isoforms generated by alternative splicing remain unclear, specific isoforms are selected in cancer to promote neoplastic transformation, cancer progression, and drug resistance (David and Manley, 2010; Zhang and Manley, 2013). In some cases, recurrent somatic point mutations near splice sites may contribute to specific splicing changes that promote cancer development by inducing mis-splicing of tumor suppressor genes (Supek et al., 2014; Jung et al., 2015).
Hotspot mutations in splicing factor genes (SFG) have been recently reported to have a high frequency in hematological malignancies and solid tumors (Patnaik et al., 2013; Landau et al., 2015). It suggests the importance of RNA splicing in cancer. Recurrent somatic mutations of the SFG, such as SF3B1, SRSF2, U2AF1, and ZRSR2, have been uncovered in myelodysplastic syndrome (MDS) (Yoshida et al., 2011), chronic lymphocytic leukemia (Mansouri et al., 2013), acute myeloid leukemia (Cancer Genome Atlas Research et al., 2013), breast cancer (McCullough, 2013), lung adenocarcinoma (Imielinski et al., 2012), and uveal melanoma (Harbour et al., 2013). Given the crucial roles of specific alternatively spliced isoforms in cancer development and the increased sensitivity of cancer cells to global perturbation of splicing efficiency versus normal cells, the pharmacological intervention of splicing may represent an important therapeutic strategy (Hubert et al., 2013; Hsu et al., 2015). Because increased tumor mutation burden (TMB) may generate neoepitopes to render a specific subpopulation of cancer patients sensitive to immunotherapies (Chan et al., 2015; Rizvi et al., 2015), abnormal mRNAs generated by mutations in spliceosomal genes can result in neoepitope production in cancers. Seiler et al. (Seiler et al., 2018) have reported that 119 SFGs have putative driver mutations in 33 TCGA cancer types. They found that the most common mutations were mutually exclusive and were associated with lineage-independent altered splicing. Furthermore, tumors with these mutations were deregulated in cell-autonomous pathways and immune infiltration (Seiler et al., 2018).
Nevertheless, an in-depth study of SFG mutations’ impact on pan-cancer remains insufficient. With the recent development of next-generation sequencing and single-cell sequencing technologies, many cancer-specific multi-omics data have been generated, which provides a unique opportunity to interrogate splicing deregulation due to somatic mutations across human cancers. Here we performed a systematic analysis of the association between SFG mutations and alterations of molecular and clinical features in 12 TCGA cancer types, including those most common human cancer types, such as breast, lung, colon, stomach, and prostate cancers. We also validated our findings in tumors by analyzing single-cell transcriptomes.
Methods
Datasets
We downloaded transcriptome (RNA-Seq, RSEM normalized), somatic mutations, somatic copy number alterations (SCNAs), protein expression profiling, and clinical data for 12 TCGA cancer types from the genomic data commons (GDC) data portal (https://portal.gdc.cancer.gov/). The 12 cancer types included adrenocortical carcinoma (ACC), bladder urothelial carcinoma (BLCA), breast invasive carcinoma (BRCA), colon adenocarcinoma (COAD), head and neck squamous cell carcinoma (HNSC), kidney renal clear cell carcinoma (KIRC), lung adenocarcinoma (LUAD), lung squamous cell carcinoma (LUSC), prostate adenocarcinoma (PRAD), skin cutaneous melanoma (SKCM), stomach adenocarcinoma (STAD), and uterine corpus endometrial carcinoma (UCEC). We obtained 119 key splicing factor genes from the publication by Seiler et al. (Seiler et al., 2018). Moreover, we obtained the datasets for six cancer cohorts treated with ICIs, which involved somatic mutation profiles and immunotherapy response data in cancer patients. These datasets included Hugo (melanoma) (Hugo et al., 2016), Riaz (melanoma) (Riaz et al., 2017), Hellmann (non-small cell lung cancer) (Hellmann et al., 2018), Liu (metastatic melanoma) (Liu et al., 2019), Rizvi (non-small cell lung cancer) (Rizvi et al., 2015), and Lauss (melanoma) (Lauss et al., 2017) cohorts. We defined a tumor as SFG-mutated if at least one of the 119 key splicing factor genes mutated in the tumor; otherwise, the tumor was defined as SFG-wildtype. In addition, we obtained six single-cell RNA sequencing (scRNA-seq) transcriptomic datasets for six cancer cohorts from the NCBI gene expression omnibus (GEO) (https://www.ncbi.nlm.nih.gov/geo/). These cancer cohorts included BRCA (Qian et al., 2020), COAD (Qian et al., 2020), SKCM (Jerby-Arnon et al., 2018), PRAD (Chen et al., 2021), LUAD (Maynard et al., 2020), and HNSC (Puram et al., 2017). Prior to subsequent analyses, we normalized all gene expression values by log2 (RSEM or TPM + 1). A summary of these datasets is shown in Supplementary Table S1.
Gene-set enrichment analysis
We used the single-sample gene-set enrichment analysis (ssGSEA) (Hänzelmann and Guinney, 2013) to score the enrichment of pathways, immune signatures, proliferation, and stemness signatures based on the expression profiles of their pathway or marker genes. The pathway genes were obtained from KEGG (Kanehisa et al., 2017), and the marker genes of immune, proliferation and stemness signatures were obtained from several publications, including CD8+ T cells (Li et al., 2020a), proliferation (Li et al., 2020a), and tumor stemness (Miranda et al., 2019).
Calculation of the ratio of two immune signatures
The ratio of two immune signatures in a tumor is the log2-transformed value of the geometric mean expression level of all marker genes in an immune signature over that in another immune signature.
Evaluation of TMB, homologous recombination deficiency (HRD) and SCNAs
We defined a tumor’s TMB as the total number of somatic mutations detected in the tumor. From the publication by Knijnenburg et al. (2018), we obtained HRD scores (aneuploidy levels) of TCGA cancers. Knijnenburg et al. defined HRD scores in 9,125 TCGA cancer samples based on HRD loss of heterozygosity, large-scale state transitions, and the number of telomeric allelic imbalances. We utilized GISTIC2 (Mermel et al., 2011) to calculate G-scores of SFG-mutated and SFG-wildtype tumors with the input of “SNP6” files, representing the amplitude and frequency of SCNAs across a group of samples.
Logistic regression analysis
We predicted the ratios of two immune signatures (high (>median) versus low (<median)) by the logistic regression model with three predictors (SFG mutation, HRD score, and TMB). The SFG mutation was a discrete variable, and the HRD score and TMB were continuous variables. In the logistic regression analysis, we first normalized all values by z-score and then fitted the binary model with the “glm ()” R function. In the function, the parameter “family” was specified as “binomial” and other parameters as default.
Survival analysis
We utilized Kaplan–Meier curves to display the survival time differences between different groups of cancer patients. A total of three survival endpoints were analyzed, including overall survival (OS), progression-free interval (PFI), and disease-specific survival (DSS). The log-rank test was used to assess the significance of survival time differences.
Single-cell data pre-process and visualization
We utilized the R package “Seurat” (v3.2.1) (Butler et al., 2018) to pre-process the scRNA-seq data before subsequent analyses. We first filtered out the genes expressed in less than three tumor cells and deleted the cells expressing less than 200 genes. Next, we normalized the gene expression matrix using the function “NormalizeData” with the default parameters. Furthermore, we identified the genes whose expression had high variations across single cells by the function “FindVariableFeatures” with the default “vst” method in “Seurat.” Based on these genes, we performed the principal component analysis of the gene expression matrix. With the first ten principal components, we built a shared nearest neighbor graph to cluster cells using the “FindClusters” function. Finally, we employed the uniform manifold approximation and projection (UMAP) (Becht et al., 2018) method (the “RunUMAP” R function) to visualize cells in low dimensions.
Inference of SFG mutations in single cells
We identified subpopulations of cancer single cells with a certain phenotype, such as SFG mutations, using the Scissor algorithm (Sun et al., 2022). Scissor defines phenotype-associated subpopulations of single cells by integrating bulk transcriptomes with phenotypic features and single-cell transcriptomes based on the similarity between single cell and bulk tumor expression profiles.
Inference of DNA copy number variations (CNVs) in single cells
We utilized inferCNV (Patel et al., 2014) to infer CNVs in cancer cells relative to normal cells. We converted all CNV values inferred by inferCNV to 0, 1, or 2, where “0” indicates neutral, “1” loss or gain of one copy, and “2” loss or gain of two copies. The re-standardized CNV score of each cell was the sum of the CNV value for each gene.
Clustering single cells
We used the single-cell consensus clustering (SC3) algorithm (Kiselev et al., 2017) to perform unsupervised clustering of single cells.
Statistical analysis
In comparisons of two classes of samples, we used the Student’s t-test if they followed normal distributions; otherwise, we used the Mann–Whitney U test. We employed the Fisher’s exact test to examine the correlation between two categorical variables and the Z-test to compare the population proportions between two groups. We performed all statistical analyses in the R programming environment (version 3.6.1).
Results
Mutations of SFG are associated with unfavorable clinical outcomes in cancer
We found that in six cancer types (BLCA, BRCA, LUSC, SKCM, STAD, and UCEC), mutations of SFG were correlated with worse OS, DSS, and/or PFI (log-rank test, p ≤ .05) (Figure 1A). Furthermore, we found that late-stage (stage III-IV) tumors harbored a significantly higher proportion of SFG-mutated tumors compared with early-stage (stage I-II) tumors in pan-cancer (Fisher’s exact test, p < .001) and in three individual cancer types (ACC, HNSC, and STAD) (p < .05) (Figure 1B). Tumor grade represents the growth speed and spread extent of a tumor on the basis of the abnormality degree of tumor cells relative to normal cells. We found that SFG-mutated tumors constituted a higher proportion in high-grade (G3-4) than in low-grade (G1-2) tumors in pan-cancer (p = .004) (Figure 1B).
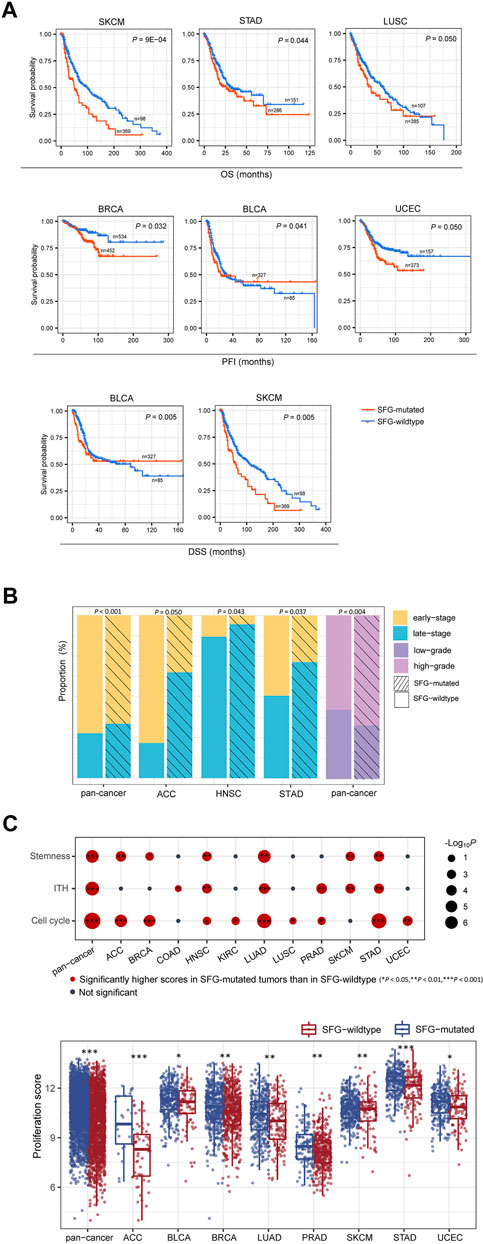
FIGURE 1. SFG mutations correlate with unfavorable clinical outcomes in cancer. (A) Kaplan-Meier curves displaying that SFG-mutated tumors have worse survival prognosis than SFG-wildtype tumors in multiple cancer types. The log-rank test p values are shown. (B) Advanced (late-stage or high-grade) tumors include a significantly higher proportion of SFG-mutated tumors than non-advanced (early-stage or low-grade) tumors in pan-cancer and in multiple cancer types. The Fisher’s exact test p values are shown. (C) The tumor progression phenotypes (stemness, proliferation, intratumor heterogeneity (ITH), and cell cycle activity) have significantly higher scores in SFG-mutated than in SFG-wildtype tumors in pan-cancer and in multiple cancer types. The one-tailed Mann-Whitney U test p values are shown.
We further compared several phenotypes indicating tumor progression or unfavorable prognosis, including tumor stemness, proliferation, and intratumor heterogeneity (ITH). Notably, all these phenotypes showed significantly higher scores in SFG-mutated than in SFG-wildtype tumors in pan-cancer (one-tailed Mann-Whitney U test, p < .001) (Figure 1C). Moreover, in 6, 8, and 6 individual cancer types, SFG-mutated tumors displayed significantly higher scores of stemness, proliferation, and ITH than SFG-wildtype tumors, respectively (Figure 1C). In addition, SFG-mutated tumors showed significantly higher enrichment scores of the cell cycle pathway than SFG-wildtype tumors in pan-cancer and in nine individual cancer types (Figure 1C).
Taken together, these results support that SFG mutations are associated with unfavorable clinical outcomes in various cancers.
Mutations of SFG are associated with genomic instability in cancer
Genomic instability often results in increased TMB and CNAs (Li et al., 2020b). Notably, SFG-mutated tumors had significantly higher TMB than SFG-wildtype tumors in pan-cancer and all the 12 cancer types (one-tailed Mann-Whitney U test, p < .001) (Figure 2A). As expected, SFG-mutated tumors harbored far more neoantigens (Rooney et al., 2015) than SFG-wildtype tumors in pan-cancer and in 10 individual cancer types (p < .05) (Figure 2A). HRD may lead to large-scale genomic instability, namely aneuploidy (Matera and Wang, 2014). We found that SFG-mutated tumors had significantly higher HRD scores (i.e., aneuploidy levels) than SFG-wildtype tumors in pan-cancer and in eight individual cancer types (one-tailed Mann-Whitney U test, p < .05) (Figure 2B). DNA mismatch repair deficiency (dMMR) or microsatellite instability (MSI) is a prevalent pattern of genomic instability in certain cancer types, such as endometrial, colorectal, and gastric cancers. We found that SFG-mutated tumors harbored a significantly higher proportion of MSI tumors than SFG-wildtype tumors in UCEC, COAD, and STAD (Fisher’s exact test, p < .001) (Figure 2C). In addition, we found many DNA repair pathways showing higher enrichment in SFG-mutated than in SFG-wildtype tumors in at least a half of the 12 cancer types. These pathways included DNA replication, base excision repair, nucleotide excision repair, non-homologous end-joining, Fanconi anemia, homologous recombination, and mismatch repair (Figure 2D). Furthermore, the amplitude and frequency of SCNAs, including copy number amplifications and deletions, were higher in SFG-mutated than in SFG-wildtype tumors in pan-cancer across the chromosome (Figure 2E). These results collectively suggest a significant association between SFG mutations and increased genomic instability in diverse cancers.
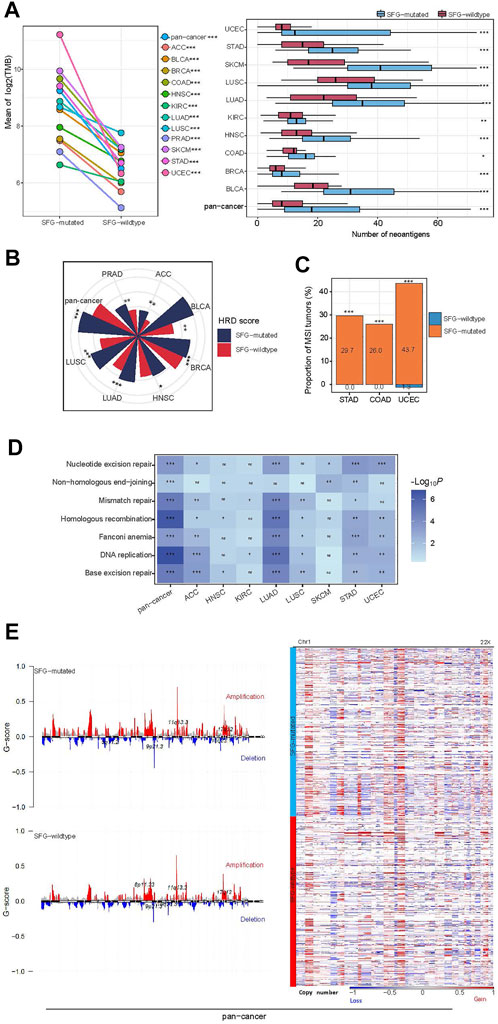
FIGURE 2. SFG mutations correlate with genomic instability in cancer. (A) SFG-mutated tumors have significantly higher tumor mutation burden (TMB) and neoantigens than SFG-wildtype tumors in pan-cancer and in most individual cancer types. (B) SFG-mutated tumors have significantly higher homologous recombination deficiency (HRD) scores than SFG-wildtype tumors in pan-cancer and in seven individual cancer types. (C) SFG-mutated tumors harbor a significantly higher proportion of MSI tumors than SFG-wildtype tumors in the cancer types with a high prevalence of MSI tumors. (D) SFG-mutated tumors have higher enrichment of DNA repair pathways than SFG-wildtype tumors. (E) SFG-mutated tumors have higher levels of copy number alteration (G-scores) than SFG-wildtype tumors in pan-cancer, and heatmap shows that SFG-mutated tumors have higher amplitudes of copy number amplification and deletion across chromosomes than SFG-wildtype tumors. The one-tailed Mann-Whitney U test p values are shown in (A,B,D), and the Fisher’s exact test p values are shown in (C). *p < .05, **p < .01, ***p < .001, ns not significant.
Interestingly, we found smoking to have a significant positive correlation with SFG mutations, as evidenced by the fact that smokers had a significantly higher proportion of SFG mutations than non-smokers in pan-cancer (75.09% versus 55.52%; Z-test, p = 5.8 × 10–13). The reason explaining this result could be that smoking can incite mutations of tumor cells to enhance TMB (Yoshida et al., 2020).
Mutations of SFG are associated with reduced anti-tumor immune responses and increased immunotherapy response
We found that SFG-mutated tumors had significantly higher expression levels of PD-L1 in pan-cancer and in seven individual cancer types (Student’s t-test, p < .05; FC > 1.5) (Figure 3A). Furthermore, in pan-cancer and in seven individual cancer types, the ratios of immune-stimulatory to immune-inhibitory signatures (CD8+ T cells/PD-1) were significantly lower in SFG-mutated than in SFG-wildtype tumors (p < .05) (Figure 3B). Because both TMB and tumor aneuploidy have significant associations with anti-tumor immune responses (Davoli et al., 2017) and meanwhile the SFG mutation has significant associations with TMB and tumor aneuploidy, the significant correlation between the SFG mutation and anti-tumor immune responses could be mediated by TMB and tumor aneuploidy. To clarify this speculation, we performed logistic regression to predict high versus low ratios of CD8+ T cells/PD-1 in pan-cancer using three predictors: SFG mutation, HRD score, and TMB. This analysis demonstrated that the SFG mutation was a significant, negative predictor of ratios of CD8+ T cells/PD-1 (p = 4.2 × 10–8; β = -.33). Taken together, these results suggest a significant association between SFG mutations and reduced anti-tumor immune responses in diverse cancers.
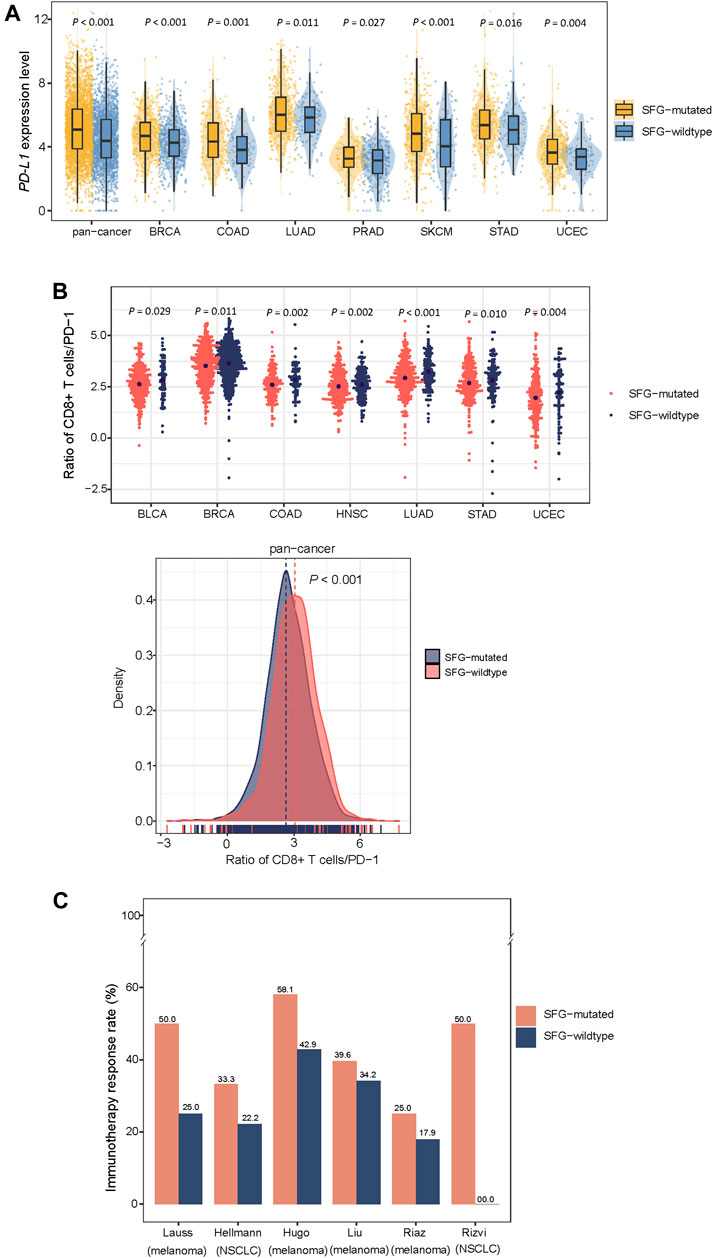
FIGURE 3. SFG mutations correlate with reduced anti-tumor immune responses and increased immunotherapy response in cancer. SFG-mutated tumors have higher expression levels of PD-L1 (A) and the ratios of immune-stimulatory to immune-inhibitory signatures (CD8+ T cells/PD-1) (B) than SFG-wildtype tumors in pan-cancer and in seven cancer types. The Student’s t test p values are shown in (A,B). (C) SFG-mutated tumors have higher response rates to immune checkpoint inhibitors (ICIs) than SFG-wildtype tumors in six cancer cohorts.
Because both PD-L1 expression (Patel and Kurzrock, 2015) and TMB (Goodman et al., 2017) are positive predictors of the response to immune checkpoint inhibitors (ICIs) and SFG mutations have heightened PD-L1 expression and TMB in cancer, we anticipated that SFG-mutated tumors would respond better to ICIs than SFG-wildtype tumors. As expected, in six cancer cohorts receiving ICI treatments, SFG-mutated tumors displayed higher response rates to ICIs than SFG-wildtype tumors (Figure 3C). These cohorts included Hugo (melanoma) (Hugo et al., 2016), Riaz (melanoma) (Riaz et al., 2017), Hellmann (non-small cell lung cancer) (Hellmann et al., 2018), Liu (metastatic melanoma) (Liu et al., 2019), Rizvi (non-small cell lung cancer) (Rizvi et al., 2015), Lauss (melanoma) (Lauss et al., 2017) cohorts, in which the response rates to ICIs in SFG-mutated versus SFG-wildtype tumors were 58.1% versus 42.9%, 25.0% versus 17.9%, 33.3% versus 22.2%, 39.6% versus 34.2%, 50% versus 0%, 50% versus 25%, respectively.
Different cancer subtypes have significantly different mutation rates of SFG
The mutations in certain tumor suppressor genes or oncogenes occur frequently in cancer, and their mutations are associated with cancer onset and development, such as TP53, EGFR, KRAS, and BRAF mutations. Notably, these genes-mutated tumors exhibited significantly higher mutation rates of SFG than these genes-wildtype tumors in pan-cancer and in multiple individual cancer types (Z-test, p < .05) (Figure 4A). For example, in pan-cancer and in six individual cancer types (ACC, BLCA, BRCA, LUAD, LUSC, and SKCM), the TP53-mutated subtype had higher mutation rates of SFG than the TP53-wildtype subtype. The EGFR-mutated subtype displayed higher mutation rates of SFG than the EGFR-wildtype subtype in pan-cancer and in six individual cancer types (ACC, COAD, LUSC, SKCM, STAD, and UCEC). SFG showed significantly higher mutation rates in BRAF-mutated than in BRAF-wildtype tumors in pan-cancer and in four individual cancer types (BRCA, COAD, STAD, and UCEC).
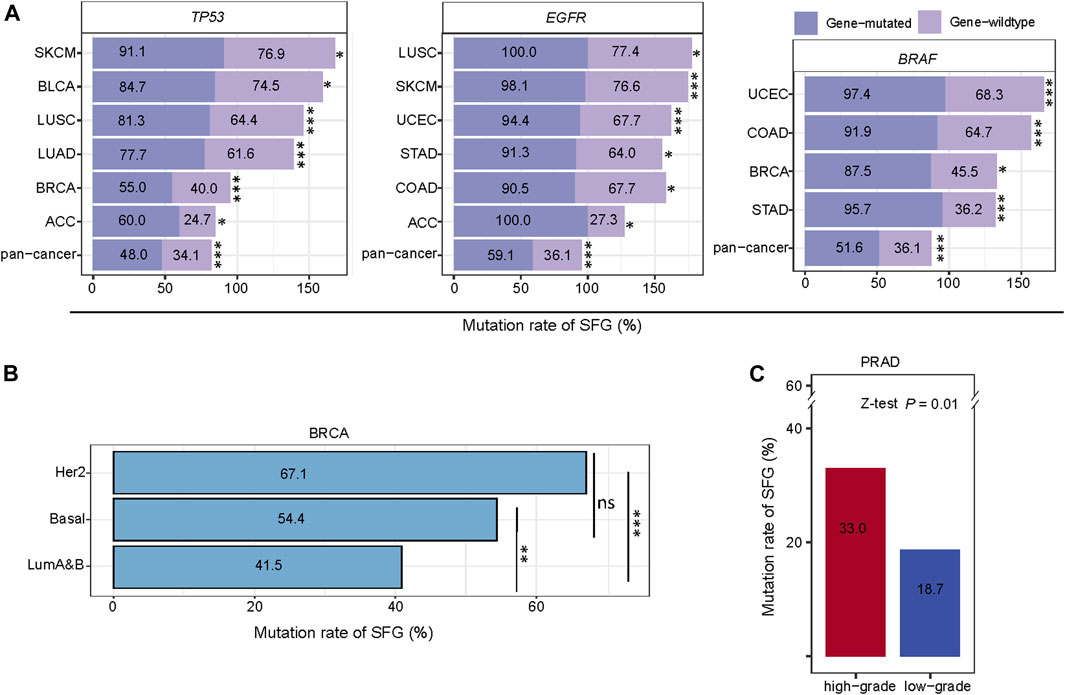
FIGURE 4. Comparisons of mutation rates of SFG between different cancer subtypes. (A) The tumors with mutations in TP53, EGFR, or BRAF have significantly higher mutation rates of SFG than the tumors without such mutations in pan-cancer and in multiple cancer types. (B) Comparisons of mutation rates of SFG among the breast cancer subtypes. (C) High-grade tumors (Gleason score > 7) have a significantly higher mutation rate of SFG than low-grade tumors (Gleason score < 7). *p < .05, **p < .01, ***p < .001, ns not significant. The Z-test p values are shown in (A,B,C).
We further compared mutation rates of SFG among the breast cancer subtypes: luminal A&B, HER2-enriched, and basal-like, which were determined by the PAM50 assay (Parker et al., 2009). Notably, both HER2-enriched and basal-like displayed significantly higher mutation rates of SFG than luminal A&B (p < .01), while the SFG mutation rates showed no significant difference between HER2-enriched and basal-like (p = .07) (Figure 4B). Overall, these results indicate that the SFG mutation is an adverse prognostic factor in breast cancer since luminal A&B often has a better prognosis than HER2-enriched and basal-like breast cancers (Howlader et al., 2018). In PRAD, the Gleason score, ranging from 6 to 10, is the most common system to grade prostate cancer. A higher Gleason score indicates a higher grade of malignancy. We found that high-grade tumors (Gleason score > 7) have a significantly higher mutation rate of SFG than low-grade tumors (Gleason score ≤ 7) in PRAD (33.0% versus 18.7%; Z-test, p = .01) (Figure 4C).
Taken together, these results suggest that SFG was more frequently mutated in aggressive than in unaggressive cancer subtypes.
Proteins upregulated in SFG-mutated cancers are mainly associated with cell cycle regulation and genomic instability
We compared protein expression profiles between SFG-mutated and SFG-wildtype tumors in each of the 12 cancer types. We found 15 proteins having significantly higher expression levels in SFG-mutated than in SFG-wildtype tumors in at least five cancer types (two-tailed Student’s t-test, FDR < .05) (Figure 5). These proteins included Lck, PCNA, ASNS, 4E-BP1, FASN, ACC_pS79, Cyclin_B1, Chk1_pS345, eEF2, eIF4E, FoxM1, Rb_pS807_S811, p90RSK, Src_pY416, and TFRC. Notably, many of these proteins are cell cycle regulators, such as Cyclin_B1, Chk1_pS345, FoxM1, Rb_pS807_S811, and Src_pY416. In addition, some proteins are involved in DNA damage repair, such as PCNA. These results are concordant with the previous analysis showing that SFG mutations are associated with cell cycle activation and genomic instability.
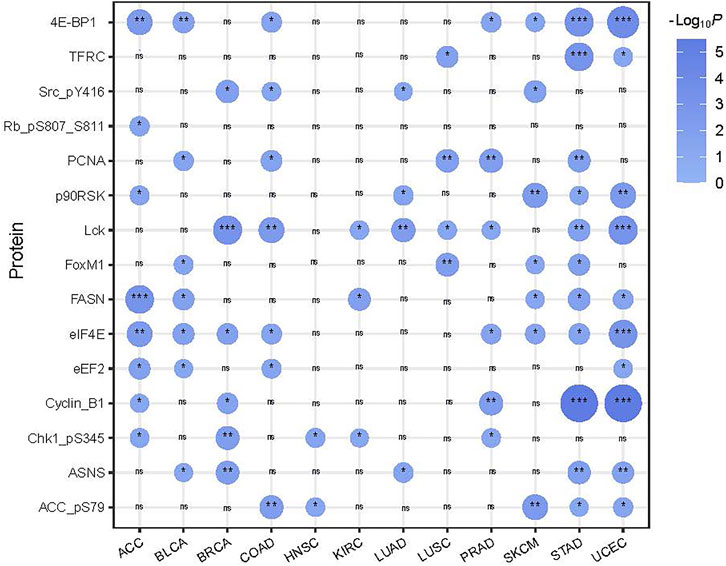
FIGURE 5. 15 proteins showing significantly higher expression levels in SFG-mutated than in SFG-wildtype tumors in at least five cancer types. The two-tailed Student’s t test p values are shown.
Exploration of SFG mutations in cancer single cells
Using the Scissor algorithm (Sun et al., 2022), we identified subpopulations of cancer single cells with phenotypes of SFG mutations and SFG wildtype in six single-cell transcriptomes from BRCA (Qian et al., 2020), COAD (Qian et al., 2020), SKCM (Jerby-Arnon et al., 2018), PRAD (Chen et al., 2021), LUAD (Maynard et al., 2020), and HNSC (Puram et al., 2017), respectively (Figure 6A). In 4 and five cancer types, the SFG-mutated cell subpopulation showed significantly higher scores of stemness and proliferation than the SFG-wildtype cell subpopulation, respectively (p < .01) (Figure 6B). In five cancer types, the SFG-mutated cell subpopulation tended to have significantly higher PD-L1 expression levels compared to than the SFG-wildtype cell subpopulation (p < .05) (Figure 6B). Overall, these results are consistent with those by analyzing tumor tissues. Furthermore, we used the SC3 algorithm (Kiselev et al., 2017) to cluster cancer cells in SFG-mutated and SFG-wildtype cell subpopulations, respectively. Among two cancer types (COAD and BRCA), SC3 uncovered significantly different numbers of cell clusters between SFG-mutated and SFG-wildtype cell subpopulations (Figure 6C). In COAD, the SFG-mutated cell subpopulation involved 49 clusters versus 30 clusters in the SFG-wildtype cell subpopulation. In BRCA, the SFG-mutated cell subpopulation included 80 clusters versus 26 clusters in the SFG-wildtype cell subpopulation. It suggests that SFG-mutated cell subpopulations are more heterogeneous than SFG-wildtype cell subpopulations, supporting the higher ITH of SFG-mutated versus SFG-wildtype tumors. We employed the inferCNV algorithm (Patel et al., 2014) to infer CNVs in cancer cells with reference to normal cells. Notably, in five cancer types, the SFG-mutated cell subpopulation had significantly greater CNV values than the SFG-wildtype cell subpopulation (p < .02) (Figure 6D). These results confirmed that SFG mutations had a significant association with genomic instability at the single-cell level.
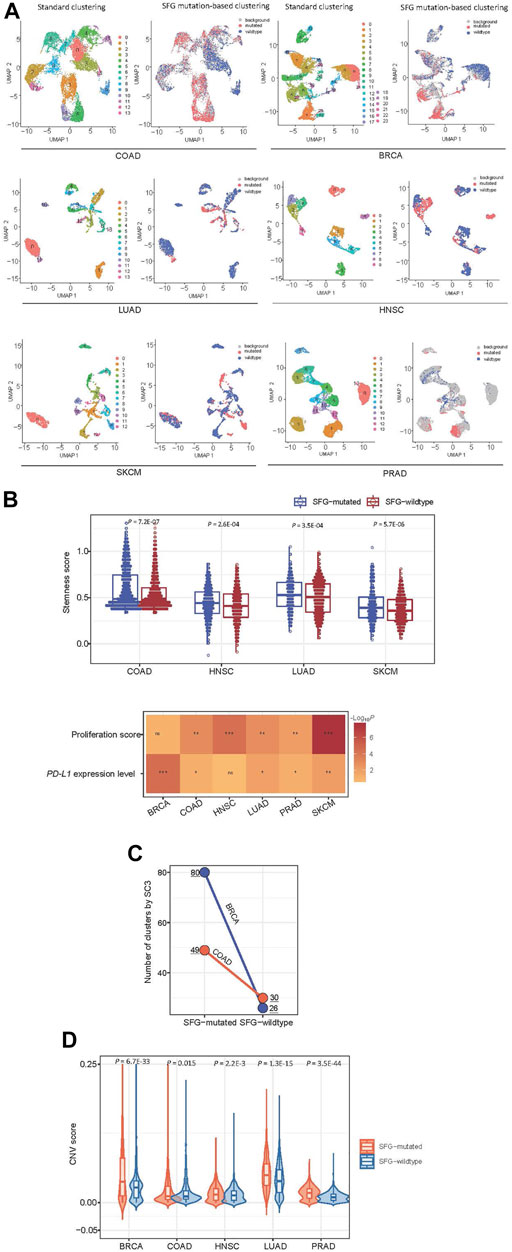
FIGURE 6. Exploration of SFG mutations in cancer single cells. (A) Identification of subpopulations of cancer single cells with phenotypes of SFG mutations and SFG wildtype in six single-cell transcriptomes by the Scissor algorithm (Sun et al., 2022). (B) The SFG-mutated cancer cell subpopulation has significantly higher scores of stemness and proliferation and higher PD-L1 expression levels than the SFG-wildtype cancer cell subpopulation. (C) The single-cell consensus clustering by SC3 (Kiselev et al., 2017) identifying more clusters in SFG-mutated cell subpopulations than in SFG-wildtype cell subpopulations in two cancer types. (D) The SFG-mutated cell subpopulation has significantly greater CNV values than the SFG-wildtype cell subpopulation in five cancer types. The CNV values were inferred by the inferCNV algorithm (Patel et al., 2014).
Discussion
Splicing abnormality caused by somatic mutations in SFG has been detected in a wide variety of human cancers. In this study, we comprehensively investigated associations of SFG mutations with clinical features, genomic integrity, anti-tumor immune responses, and immunotherapy response in 12 common cancer types and their pan-cancer. Our analysis showed that SFG mutations were associated with worse clinical outcomes, higher genomic instability, and anti-tumor immunosuppression but increased immunotherapy response in diverse cancer types. In addition, SFG mutations were associated with tumor progression or unfavorable phenotypes, such as high tumor stemness, proliferation potential, and ITH. It is interesting to observe that SFG mutations are correlated with a better response to ICIs in multiple cancers, although SFG mutations have a significant association with reduced anti-tumor immune responses. Increased TMB (and thus neoantigens) and PD-L1 expression in SFG-mutated cancers could explain why this subtype responds better to ICIs than SFG-wildtype cancers since both TMB and PD-L1 expression are positive predictors of immunotherapy response (Patel and Kurzrock, 2015; Samstein et al., 2019). On the other hand, high levels of genomic instability, i.e., SCNAs or aneuploidy, may lead to reduced anti-tumor immune responses in cancers with SFG mutations since tumor aneuploidy may promote anti-tumor immune evasion (Davoli et al., 2017). Taken together, our results indicate that the relationship between anti-tumor immune responses and immunotherapy responses is not necessarily positive but it even could be negative. Therefore, the association between the tumor immune microenvironment (TIME) and immunotherapy response is complex, although the TIME has been recognized as a critical factor for responses to immunotherapy (Binnewies et al., 2018). Overall, tumors can be classified as “cold” (lack of immune infiltration) and “hot” (with abundant immune infiltration) types in terms of their TIME (Duan et al., 2020). It has formed a common sense that “hot” tumors respond better to immunotherapy than “cold” tumors (Galon and Bruni, 2019). However, our results do not appear to support this conclusion. Thus, further investigations into the association between the TIME and immunotherapy response are warranted.
To date, the conventional treatment strategies for cancer, including surgery, radiotherapy, chemotherapy, and targeted therapies, remain the major therapeutic approaches for most cancers. However, these strategies often achieve limited efficiency for advanced or recurrent cancers. Immunotherapy, particularly ICIs, has demonstrated success in treating various malignancies, including metastatic cancers, as a recently emerging treatment strategy. Nevertheless, the response rate of cancer patients to ICIs is around 20% to date (Cristescu et al., 2018). Thus, discovering predictive markers for immunotherapy response is significant. Several such markers used in current clinical practice include PD-L1 expression (Patel and Kurzrock, 2015), high tumor mutation burden (TMB) (Allgauer et al., 2018), and dMMR (Oliveira et al., 2019). However, these markers are not perfect in predicting immunotherapy response (Sun et al., 2020). For example, more than 50% of cancer patients with high expression of PD-L1 may not respond to ICIs (Reck et al., 2016). Therefore, identifying novel predictive markers for immunotherapy response is urgently needed. Our analysis suggests that the SFG mutation could be a positive predictor for immunotherapy response.
Among the proteins upregulated in SFG-mutated cancers, ACC (acetyl-CoA carboxylase) is a lipogenic enzyme to promote the synthesis of saturated fatty acids to meet the increasing demands of new membrane phospholipids in cancer cells (Igal, 2010). Thus, ACC is upregulated in various cancers, including breast, prostate, and liver cancers, and plays a critical role in cancer development (Wang C et al., 2010; Fang et al., 2014). It is justified that ACC is more abundant in SFG-mutated than in SFG-wildtype tumors since SFG mutations are associated with unfavorable clinical outcomes in various cancers. The lymphocyte-specific protein tyrosine kinase (Lck) is a member of the Src family of protein tyrosine kinases, whose upregulation may promote the development of diverse cancers, such as breast, colon, liver, and lung cancers (Sugihara et al., 2018; Bommhardt et al., 2019). Again, the positive association between SFG mutations and Lck expression conforms to the fact that the SFG mutation is an adverse prognostic factor in diverse cancers.
This study has several limitations. First, our results were obtained by the bioinformatics analysis. As a result, the associations between SFG mutations and various clinical and molecular characteristics in cancer are a correlation relationship but not a causal relationship. To demonstrate the causal relationship, further experimental and clinical studies are must. Second, in defining the phenotype of SFG mutations, we took the 119 key splicing factor genes as a whole but ignored the difference among the impact of different splicing factor genes’ mutations on splicing deregulation. Finally, in the single-cell data analysis, we defined the phenotype of SFG mutations based on the computational inference but not DNA sequencing, although such inference might result in inaccurate results.
In conclusion, the mutation in key splicing factor genes correlates with unfavorable clinical outcomes and disease progression, genomic instability, anti-tumor immunosuppression, and increased immunotherapy response in pan-cancer. Thus, the SFG mutation is an adverse prognostic factor and a positive marker for immunotherapy response in cancer.
Data availability statement
The original contributions presented in the study are included in the article/Supplementary Material, further inquiries can be directed to the corresponding authors.
Author contributions
JL performed the research, data analyses and visualization. CC performed data analyses. ZL: investigation, funding acquisition, project administration. XW conceived of the research, designed the methods, and wrote the manuscript. All authors read and approved the final manuscript.
Funding
This work was supported by Natural Science Foundation of Jiangsu Province (BK20201090) and China Postdoctoral Science Foundation (2021M691338) to ZL.
Acknowledgments
We thank Zeinab Abdelrahman for editing the manuscript.
Conflict of interest
The authors declare that the research was conducted in the absence of any commercial or financial relationships that could be construed as a potential conflict of interest.
Publisher’s note
All claims expressed in this article are solely those of the authors and do not necessarily represent those of their affiliated organizations, or those of the publisher, the editors and the reviewers. Any product that may be evaluated in this article, or claim that may be made by its manufacturer, is not guaranteed or endorsed by the publisher.
Supplementary material
The Supplementary Material for this article can be found online at: https://www.frontiersin.org/articles/10.3389/fcell.2022.1045130/full#supplementary-material
References
Akinyi, M. V., and Frilander, M. J. (2021). At the intersection of major and minor spliceosomes: Crosstalk mechanisms and their impact on gene expression. Front. Genet. 12, 700744. doi:10.3389/fgene.2021.700744
Allgauer, M., Budczies, J., Christopoulos, P., Endris, V., Lier, A., Rempel, E., et al. (2018). Implementing tumor mutational burden (TMB) analysis in routine diagnostics-a primer for molecular pathologists and clinicians. Transl. Lung Cancer Res. 7 (6), 703–715. doi:10.21037/tlcr.2018.08.14
Becht, E., McInnes, L., Healy, J., Dutertre, C. A., Kwok, I. W. H., Ng, L. G., et al. (2018). Dimensionality reduction for visualizing single-cell data using UMAP. Nat. Biotechnol. 37, 38–44. doi:10.1038/nbt.4314
Binnewies, M., Roberts, E. W., Kersten, K., Chan, V., Fearon, D. F., Merad, M., et al. (2018). Understanding the tumor immune microenvironment (TIME) for effective therapy. Nat. Med. 24 (5), 541–550. doi:10.1038/s41591-018-0014-x
Bommhardt, U., Schraven, B., and Simeoni, L. (2019). Beyond TCR signaling: Emerging functions of Lck in cancer and immunotherapy. Int. J. Mol. Sci. 20 (14), 3500. doi:10.3390/ijms20143500
Butler, A., Hoffman, P., Smibert, P., Papalexi, E., and Satija, R. (2018). Integrating single-cell transcriptomic data across different conditions, technologies, and species. Nat. Biotechnol. 36 (5), 411–420. doi:10.1038/nbt.4096
Cancer Genome Atlas Research Ley, T. J., Miller, C., Ding, L., Raphael, B. J., Mungall, A. J., et al. (2013). Genomic and epigenomic landscapes of adult de novo acute myeloid leukemia. N. Engl. J. Med. 368 (22), 2059–2074. doi:10.1056/NEJMoa1301689
Chan, T. A., Wolchok, J. D., and Snyder, A. (2015). Genetic basis for clinical response to CTLA-4 blockade in melanoma. N. Engl. J. Med. 373 (20), 1984. doi:10.1056/NEJMc1508163
Chen, S., Zhu, G., Yang, Y., Wang, F., Xiao, Y. T., Zhang, N., et al. (2021). Single-cell analysis reveals transcriptomic remodellings in distinct cell types that contribute to human prostate cancer progression. Nat. Cell Biol. 23 (1), 87–98. doi:10.1038/s41556-020-00613-6
Cristescu, R., Mogg, R., Ayers, M., Albright, A., Murphy, E., Yearley, J., et al. (2018). Pan-tumor genomic biomarkers for PD-1 checkpoint blockade-based immunotherapy. Science 362 (6411), eaar3593. doi:10.1126/science.aar3593
David, C. J., and Manley, J. L. (2010). Alternative pre-mRNA splicing regulation in cancer: Pathways and programs unhinged. Genes Dev. 24 (21), 2343–2364. doi:10.1101/gad.1973010
Davoli, T., Uno, H., Wooten, E. C., and Elledge, S. J. (2017). Tumor aneuploidy correlates with markers of immune evasion and with reduced response to immunotherapy. Science 355 (6322), eaaf8399. doi:10.1126/science.aaf8399
Duan, Q., Zhang, H., Zheng, J., and Zhang, L. (2020). Turning cold into hot: Firing up the tumor microenvironment. Trends Cancer 6 (7), 605–618. doi:10.1016/j.trecan.2020.02.022
Fang, W., Cui, H., Yu, D., Chen, Y., Wang, J., and Yu, G. (2014). Increased expression of phospho-acetyl-CoA carboxylase protein is an independent prognostic factor for human gastric cancer without lymph node metastasis. Med. Oncol. 31 (7), 15. doi:10.1007/s12032-014-0015-7
Galon, J., and Bruni, D. (2019). Approaches to treat immune hot, altered and cold tumours with combination immunotherapies. Nat. Rev. Drug Discov. 18 (3), 197–218. doi:10.1038/s41573-018-0007-y
Goodman, A. M., Kato, S., Bazhenova, L., Patel, S. P., Frampton, G. M., Miller, V., et al. (2017). Tumor mutational burden as an independent predictor of response to immunotherapy in diverse cancers. Mol. Cancer Ther. 16 (11), 2598–2608. doi:10.1158/1535-7163.MCT-17-0386
Hänzelmann, C. R., and Guinney, J. (2013). GSVA: Gene set variation analysis for microarray and RNA-seq data. BMC Bioinforma. 14, 7. doi:10.1186/1471-2105-14-7
Harbour, J. W., Roberson, E. D. O., Anbunathan, H., Onken, M. D., Worley, L. A., and Bowcock, A. M. (2013). Recurrent mutations at codon 625 of the splicing factor SF3B1 in uveal melanoma. Nat. Genet. 45 (2), 133–135. doi:10.1038/ng.2523
Hellmann, M. D., Nathanson, T., Rizvi, H., Creelan, B. C., Sanchez-Vega, F., Ahuja, A., et al. (2018). Genomic features of response to combination immunotherapy in patients with advanced non-small-cell lung cancer. Cancer Cell 33 (5), 843–852. doi:10.1016/j.ccell.2018.03.018
Howlader, N., Cronin, K. A., Kurian, A. W., and Andridge, R. (2018). Differences in breast cancer survival by molecular subtypes in the United States. Cancer Epidemiol. Biomarkers Prev. 27 (6), 619–626. doi:10.1158/1055-9965.EPI-17-0627
Hsu, T. Y., Simon, L. M., Neill, N. J., Marcotte, R., Sayad, A., Bland, C. S., et al. (2015). The spliceosome is a therapeutic vulnerability in MYC-driven cancer. Nature 525 (7569), 384–388. doi:10.1038/nature14985
Hubert, C. G., Bradley, R. K., Ding, Y., Toledo, C. M., Herman, J., Skutt-Kakaria, K., et al. (2013). Genome-wide RNAi screens in human brain tumor isolates reveal a novel viability requirement for PHF5A. Genes Dev. 27 (9), 1032–1045. doi:10.1101/gad.212548.112
Hugo, W., Zaretsky, J. M., Sun, L., Song, C., Moreno, B. H., Hu-Lieskovan, S., et al. (2016). Genomic and transcriptomic features of response to anti-PD-1 therapy in metastatic melanoma. Cell 165 (1), 35–44. doi:10.1016/j.cell.2016.02.065
Igal, R. A. (2010). Stearoyl-CoA desaturase-1: A novel key player in the mechanisms of cell proliferation, programmed cell death and transformation to cancer. Carcinogenesis 31 (9), 1509–1515. doi:10.1093/carcin/bgq131
Imielinski, M., Berger, A. H., Hammerman, P. S., Hernandez, B., Pugh, T. J., Hodis, E., et al. (2012). Mapping the hallmarks of lung adenocarcinoma with massively parallel sequencing. Cell 150 (6), 1107–1120. doi:10.1016/j.cell.2012.08.029
Jerby-Arnon, L., Shah, P., Cuoco, M. S., Rodman, C., Su, M. J., Melms, J. C., et al. (2018). A cancer cell program promotes T cell exclusion and resistance to checkpoint blockade. Cell 175 (4), 984–997. doi:10.1016/j.cell.2018.09.006
Jung, H., Lee, D., Lee, J., Park, D., Kim, Y. J., Park, W. Y., et al. (2015). Intron retention is a widespread mechanism of tumor-suppressor inactivation. Nat. Genet. 47 (11), 1242–1248. doi:10.1038/ng.3414
Kanehisa, M., Furumichi, M., Tanabe, M., Sato, Y., and Morishima, K. (2017). KEGG: New perspectives on genomes, pathways, diseases and drugs. Nucleic Acids Res. 45 (D1), D353–D361. doi:10.1093/nar/gkw1092
Kiselev, V. Y., Kirschner, K., Schaub, M. T., Andrews, T., Yiu, A., Chandra, T., et al. (2017). SC3: Consensus clustering of single-cell RNA-seq data. Nat. Methods 14 (5), 483–486. doi:10.1038/nmeth.4236
Knijnenburg, T. A., Wang, L., Zimmermann, M. T., Chambwe, N., Gao, G. F., Cherniack, A. D., et al. (2018). Genomic and molecular landscape of DNA damage repair deficiency across the cancer Genome Atlas. Cell Rep. 23 (1), 239–254. doi:10.1016/j.celrep.2018.03.076
Landau, D. A., Tausch, E., Taylor-Weiner, A. N., Stewart, C., Reiter, J. G., Bahlo, J., et al. (2015). Mutations driving CLL and their evolution in progression and relapse. Nature 526 (7574), 525–530. doi:10.1038/nature15395
Lauss, M., Donia, M., Harbst, K., Andersen, R., Mitra, S., Rosengren, F., et al. (2017). Mutational and putative neoantigen load predict clinical benefit of adoptive T cell therapy in melanoma. Nat. Commun. 8 (1), 1738. doi:10.1038/s41467-017-01460-0
Li, L., Li, M. Y., and Wang, X. S. (2020). Cancer type-dependent correlations between TP53 mutations and antitumor immunity. DNA Repair (Amst), 88. doi:10.1016/j.dnarep.2020.102785
Li, M., Zhang, Z., Li, L., and Wang, X. (2020). An algorithm to quantify intratumor heterogeneity based on alterations of gene expression profiles. Commun. Biol. 3 (1), 505. doi:10.1038/s42003-020-01230-7
Liu, D., Schilling, B., Liu, D., Sucker, A., Livingstone, E., Jerby-Arnon, L., et al. (2019). Integrative molecular and clinical modeling of clinical outcomes to PD1 blockade in patients with metastatic melanoma. Nat. Med. 25 (12), 1916–1927. doi:10.1038/s41591-019-0654-5
Mansouri, L., Grabowski, P., Degerman, S., Svenson, U., Gunnarsson, R., Cahill, N., et al. (2013). Short telomere length is associated with NOTCH1/SF3B1/TP53 aberrations and poor outcome in newly diagnosed chronic lymphocytic leukemia patients. Am. J. Hematol. 88 (8), 647–651. doi:10.1002/ajh.23466
Matera, A. G., and Wang, Z. (2014). A day in the life of the spliceosome. Nat. Rev. Mol. Cell Biol. 15 (2), 108–121. doi:10.1038/nrm3742
Maynard, A., McCoach, C. E., Rotow, J. K., Harris, L., Haderk, F., Kerr, D. L., et al. (2020). Therapy-induced evolution of human lung cancer revealed by single-cell RNA sequencing. Cell 182 (5), 1232–1251. doi:10.1016/j.cell.2020.07.017
McCullough, A. (2013). Comprehensive molecular portraits of human breast tumours. Yearbook of Pathology and Laboratory Medicine, 286–288.
Mermel, C. H., Schumacher, S. E., Hill, B., Meyerson, M. L., Beroukhim, R., and Getz, G. (2011). GISTIC2.0 facilitates sensitive and confident localization of the targets of focal somatic copy-number alteration in human cancers. Genome Biol. 12 (4), R41. doi:10.1186/gb-2011-12-4-r41
Miranda, A., Hamilton, P. T., Zhang, A. W., Pattnaik, S., Becht, E., Mezheyeuski, A., et al. (2019). Cancer stemness, intratumoral heterogeneity, and immune response across cancers. Proc. Natl. Acad. Sci. U. S. A. 116 (18), 9020–9029. doi:10.1073/pnas.1818210116
Oliveira, A. F., Bretes, L., and Furtado, I. (2019). Review of PD-1/PD-L1 inhibitors in metastatic dMMR/MSI-H colorectal cancer. Front. Oncol. 9, 396. doi:10.3389/fonc.2019.00396
Papasaikas, P., and Valcarcel, J. (2016). The spliceosome: The ultimate RNA chaperone and sculptor. Trends Biochem. Sci. 41 (1), 33–45. doi:10.1016/j.tibs.2015.11.003
Parker, J. S., Mullins, M., Cheang, M. C. U., Leung, S., Voduc, D., Vickery, T., et al. (2009). Supervised risk predictor of breast cancer based on intrinsic subtypes. J. Clin. Oncol. 27 (8), 1160–1167. doi:10.1200/JCO.2008.18.1370
Patel, A. P., Tirosh, I., Trombetta, J. J., Shalek, A. K., Gillespie, S. M., Wakimoto, H., et al. (2014). Single-cell RNA-seq highlights intratumoral heterogeneity in primary glioblastoma. Science 344 (6190), 1396–1401. doi:10.1126/science.1254257
Patel, S. P., and Kurzrock, R. (2015). PD-L1 expression as a predictive biomarker in cancer immunotherapy. Mol. Cancer Ther. 14 (4), 847–856. doi:10.1158/1535-7163.MCT-14-0983
Patnaik, M. M., Lasho, T. L., Finke, C. M., Hanson, C. A., Hodnefield, J. M., Knudson, R. A., et al. (2013). Spliceosome mutations involving SRSF2, SF3B1, and U2AF35 in chronic myelomonocytic leukemia: Prevalence, clinical correlates, and prognostic relevance. Am. J. Hematol. 88 (3), 201–206. doi:10.1002/ajh.23373
Puram, S. V., Tirosh, I., Parikh, A. S., Patel, A. P., Yizhak, K., Gillespie, S., et al. (2017). Single-cell transcriptomic analysis of primary and metastatic tumor ecosystems in head and neck cancer. Cell 171 (7), 1611–1624. doi:10.1016/j.cell.2017.10.044
Qian, J., Olbrecht, S., Boeckx, B., Vos, H., Laoui, D., Etlioglu, E., et al. (2020). A pan-cancer blueprint of the heterogeneous tumor microenvironment revealed by single-cell profiling. Cell Res. 30 (9), 745–762. doi:10.1038/s41422-020-0355-0
Reck, M., Rodriguez-Abreu, D., Robinson, A. G., Hui, R., Csoszi, T., Fulop, A., et al. (2016). Pembrolizumab versus chemotherapy for PD-L1-positive non-small-cell lung cancer. N. Engl. J. Med. 375 (19), 1823–1833. doi:10.1056/NEJMoa1606774
Riaz, N., Havel, J. J., Makarov, V., Desrichard, A., Urba, W. J., Sims, J. S., et al. (2017). Tumor and microenvironment evolution during immunotherapy with nivolumab. Cell 171 (4), 934–949. e16. doi:10.1016/j.cell.2017.09.028
Rizvi, N. A., Hellmann, M. D., Snyder, A., Kvistborg, P., Makarov, V., Havel, J. J., et al. (2015). Cancer immunology. Mutational landscape determines sensitivity to PD-1 blockade in non-small cell lung cancer. Science 348 (6230), 124–128. doi:10.1126/science.aaa1348
Rooney, M. S., Shukla, S. A., Wu, C. J., Getz, G., and Hacohen, N. (2015). Molecular and genetic properties of tumors associated with local immune cytolytic activity. Cell 160 (1-2), 48–61. doi:10.1016/j.cell.2014.12.033
Samstein, R. M., Lee, C. H., Shoushtari, A. N., Hellmann, M. D., Shen, R., Janjigian, Y. Y., et al. (2019). Tumor mutational load predicts survival after immunotherapy across multiple cancer types. Nat. Genet. 51 (2), 202–206. doi:10.1038/s41588-018-0312-8
Seiler, M., Peng, S., Agrawal, A. A., Palacino, J., Teng, T., Zhu, P., et al. (2018). Somatic mutational landscape of splicing factor genes and their functional consequences across 33 cancer types. Cell Rep. 23 (1), 282–296. doi:10.1016/j.celrep.2018.01.088
Sugihara, T., Werneburg, N. W., Hernandez, M. C., Yang, L., Kabashima, A., Hirsova, P., et al. (2018). YAP tyrosine phosphorylation and nuclear localization in cholangiocarcinoma cells are regulated by LCK and independent of LATS activity. Mol. Cancer Res. 16 (10), 1556–1567. doi:10.1158/1541-7786.MCR-18-0158
Sun, D., Guan, X., Moran, A. E., Wu, L. Y., Qian, D. Z., Schedin, P., et al. (2022). Identifying phenotype-associated subpopulations by integrating bulk and single-cell sequencing data. Nat. Biotechnol. 40 (4), 527–538. doi:10.1038/s41587-021-01091-3
Sun, J. Y., Zhang, D., Wu, S., Xu, M., Zhou, X., Lu, X. J., et al. (2020). Resistance to PD-1/PD-L1 blockade cancer immunotherapy: Mechanisms, predictive factors, and future perspectives. Biomark. Res. 8, 35. doi:10.1186/s40364-020-00212-5
Supek, F., Minana, B., Valcarcel, J., Gabaldon, T., and Lehner, B. (2014). Synonymous mutations frequently act as driver mutations in human cancers. Cell 156 (6), 1324–1335. doi:10.1016/j.cell.2014.01.051
Wang C, R. S., Watabe, K., Liao, D. F., and Cao, D. (2010). Acetyl-CoA carboxylase-a as a novel target for cancer therapy. Front. Biosci. Sch. Ed. 2 (2), 515–526. doi:10.2741/s82
Witten, J. T., and Ule, J. (2011). Understanding splicing regulation through RNA splicing maps. Trends Genet. 27 (3), 89–97. doi:10.1016/j.tig.2010.12.001
Yoshida, K., Gowers, K. H. C., Lee-Six, H., Chandrasekharan, D. P., Coorens, T., Maughan, E. F., et al. (2020). Tobacco smoking and somatic mutations in human bronchial epithelium. Nature 578 (7794), 266–272. doi:10.1038/s41586-020-1961-1
Yoshida, K., Sanada, M., Shiraishi, Y., Nowak, D., Nagata, Y., Yamamoto, R., et al. (2011). Frequent pathway mutations of splicing machinery in myelodysplasia. Nature 478 (7367), 64–69. doi:10.1038/nature10496
Keywords: somatic mutations, splicing factor genes, pan-cancer, cancer prognosis, anti-tumor immune responses, cancer immunotherapy, genomic instability
Citation: Luo J, Chen C, Liu Z and Wang X (2023) The mutation in splicing factor genes correlates with unfavorable prognosis, genomic instability, anti-tumor immunosuppression and increased immunotherapy response in pan-cancer. Front. Cell Dev. Biol. 10:1045130. doi: 10.3389/fcell.2022.1045130
Received: 15 September 2022; Accepted: 21 December 2022;
Published: 06 January 2023.
Edited by:
Yong Teng, Emory University, United StatesReviewed by:
Nisargbhai Shah, Memorial Sloan Kettering Cancer Center, United StatesHauke Thomsen, ProtaGene CGT GmbH, Germany
Copyright © 2023 Luo, Chen, Liu and Wang. This is an open-access article distributed under the terms of the Creative Commons Attribution License (CC BY). The use, distribution or reproduction in other forums is permitted, provided the original author(s) and the copyright owner(s) are credited and that the original publication in this journal is cited, in accordance with accepted academic practice. No use, distribution or reproduction is permitted which does not comply with these terms.
*Correspondence: Zhixian Liu, bGl1emhpeGlhbkBuam11LmVkdS5jbg==; Xiaosheng Wang, eGlhb3NoZW5nLndhbmdAY3B1LmVkdS5jbg==