- 1Department of Hepatopancreatobiliary Surgery, Shenzhen Institute of Translational Medicine, Health Science Center, The First Affiliated Hospital of Shenzhen University, Shenzhen Second People’s Hospital, Shenzhen, China
- 2Shenzhen Xenotransplantation Medical Engineering Research and Development Center, Shenzhen Institute of Translational Medicine, Health Science Center, The First Affiliated Hospital of Shenzhen University, Shenzhen Second People’s Hospital, Shenzhen, China
- 3Imaging Department, Shenzhen Institute of Translational Medicine , Health Science Center, Shenzhen Second People’s Hospital, The First Affiliated Hospital of Shenzhen University, Shenzhen, China
Gastric cancer (GC) is a malignant disease of the digestive tract and a life-threatening disease worldwide. Ferroptosis, an iron-dependent cell death caused by lipid peroxidation, is reported to be highly correlated with gastric tumorigenesis and immune cell activity. However, the underlying relationship between ferroptosis and the tumor microenvironment in GC and potential intervention strategies have not been unveiled. In this study, we profiled the transcriptome and prognosis data of ferroptosis-related genes (FRGs) in GC samples of the TCGA-STAD dataset. The infiltrating immune cells in GC were estimated using the CIBERSORT and XCELL algorithms. We found that the high expression of the hub FRGs (MYB, PSAT1, TP53, and LONP1) was positively correlated with poor overall survival in GC patients. The results were validated in an external GC cohort (GSE62254). Further immune cell infiltration analysis revealed that CD4+ T cells were the major infiltrated cells in the tumor microenvironment of GC. Moreover, the hub FRGs were significantly positively correlated with activated CD4+ T cell infiltration, especially Th cells. The gene features in the high-FRG score group were enriched in cell division, DNA repair, protein folding, T cell receptor, Wnt and NIK/NF-kappaB signaling pathways, indicating that the hub FRGs may mediate CD4+ T cell activation by these pathways. In addition, an upstream transcriptional regulation network of the hub FRGs by lncRNAs was also developed. Three lncRNAs (A2M-AS1, C2orf27A, and ZNF667-AS1) were identified to be related to the expression of the hub FRGs. Collectively, these results showed that lncRNA A2M-AS1, C2orf27A, and ZNF667-AS1 may target the hub FRGs and impair CD4+ T cell activation, which finally leads to poor prognosis of GC. Effective interventions for the above lncRNAs and the hub FRGs can help promote CD4+ T cell activation in GC patients and improve the efficacy of immunotherapy. These findings provide a novel idea of GC immunotherapy and hold promise for future clinical application.
1 Introduction
Gastric cancer, as the third leading cause of cancer-related deaths, is responsible for a high burden of disease globally (Sung et al., 2021). In GC, ferroptosis is reported to be related to tumor cell proliferation and cancer therapeutic efficacy (Zhang et al., 2020; Ma et al., 2021). Ferroptosis, a type of programmed cell death, is induced by iron-dependent lipid peroxidation (Dixon et al., 2012). Moreover, increased ferroptosis is reported to contribute to the antitumor efficacy of immunotherapy (Wang et al., 2019a). Thus, in-depth research on ferroptosis in GC will help to clarify the understanding of tumorigenesis and develop effective approaches for GC treatment.
Recent studies have shown the relationship between ferroptosis and the immune microenvironment in the tumorigenesis and prognosis of GC (Zhang et al., 2020). The cells and molecules of the tumor microenvironment play a major role in tumor initiation and progression as well as responses to therapy (Voron et al., 2014; Subhash et al., 2015; Russick et al., 2020; Bejarano et al., 2021). The tumor microenvironment is composed of multiple components, such as macrophages, T cells, B cells, NK cells, tumor parenchymal cells, lymphocytes, fibroblasts, mesenchymal cells, and angiogenic factors (Subhash et al., 2015). Recently, it has been reported that CD4+ T cells might be a potential immunotherapeutic target for GC (Wei et al., 2018; Gu et al., 2020). Accordingly, CD4+ T cells are now commonly divided into two distinct lineages: Treg cells and conventional T helper cells (Corthay, 2009). Conventional Th cells control adaptive immunity by activating other effector cells, such as CD8+ cytotoxic T cells, B cells, and macrophages (Feau et al., 2012). Treg cells are defined as T cells in charge of suppression of potentially deleterious activities of Th cells, regulation of the effector class of the immune response, and suppression of T-cell activation (Corthay, 2009; Arumugam and Sugin Lal Jabaris, 2021). The study of CD4+ T cells has tremendous potential to contribute to cancer immunotherapy (Yuan et al., 2017). It has been previously reported that ferroptosis is involved in regulating CD4+ T cell homeostasis, which enhances the function of follicular helper T cells during infection and following vaccination (Yao et al., 2021). However, the function of ferroptosis in the regulation of the GC microenvironment and the upstream regulators of ferroptosis-related genes (FRGs) are worth further exploration.
In this study, we identified four important FRGs (the hub FRGs: PSAT1, MYB, TP53, and LONP1) that were related to gastric carcinogenesis and prognosis. Based on immune cell infiltration analysis of different immune cell types, we found that the expression of the hub FRGs was negatively correlated with resting CD4+ T cell infiltration but positively correlated with activated CD4+ T cell infiltration in the GC samples of the TCGA-STAD dataset. We indicated that the hub FRGs might participate in CD4+ T cell activation. The T cell receptor signaling pathway, NIK/NF-kappaB signaling pathway and Wnt signaling pathway were shown to be significantly related to the hub FRGs. To assess the potential upstream regulatory mechanism of the hub FRGs, we identified three lncRNAs (A2M-AS1, C2orf27A, and ZNF667-AS1) that might inhibit the expression of the hub FRGs. Collectively, these results revealed that these three lncRNAs are upstream regulators of the hub FRGs and affect the GC microenvironment.
2 Materials and Methods
2.1 Data Preparing and Processing
Ferroptosis-related genes (FRGs): We first obtained 289 FRGs from previous studies (PMID: 33425905, 31634899, 31634900, 31105042, 28985560, and 32760210) (Stockwell et al., 2017; Bersuker et al., 2019; Doll et al., 2019; Hassannia et al., 2019; Liu et al., 2020a; Liang et al., 2020).
TCGA-STAD dataset: The gene expression data of 375 GC patients and 32 adjacent cancer samples and clinical data of matched patients were obtained from The Cancer Genome Atlas (TCGA) data portal (https://TCGAData.nci.nih.gov/TCGA/). For differential expressed gene (DEG) analysis of mRNA, we used the limma package in R 4.0.5, and genes with a p-value <0.05 and fold change >1.5 were selected to be differentially expressed. For Kaplan-Meier (K-M) analysis and Cox regression analysis, a risk score was calculated for each patient, and a median value was identified for all patients. GC patients were then divided into a low group (score below the median) and a high group (score above the median). The high and low groups were stratified and visualized using K-M survival curves and analyzed for statistical significance using the log-rank test. Cox regression analysis and K-M curves with the log-rank test were conducted by the glmnet and survival packages in R 4.0.5.
GSE62254 dataset (validation dataset): K-M analysis of the GEO dataset (GSE62254) was performed by the Kaplan-Meier Plotter database (https://kmplot.com/analysis/) (Cristescu et al., 2015). We used the Auto select best cutoff function to split patients.
LncRNAs: LncRNAs were annotated by GENCODE (version 25). We used the limma package in R 4.0.5 to perform DEG analysis. LncRNAs with a p-value <0.05 and fold change >1.5 were selected to be significantly differentially expressed. K-M analysis and Cox regression analysis were used to calculate median survival.
The heatmap plots were displayed by the scaled FPKM of each gene across the whole GC samples.
2.2 Correlation Analysis of Gene Expression
We applied Pearson’s correlation test to assess the correlations between the hub FRGs and other genes. Pearson’s correlation test was performed in R 4.0.5. Gene pairs with Pearson’s correlation coefficient >0.1 and p-value <0.01 will be considered coexpressed genes.
2.3 Immune Infiltration Analysis
The CIBERSORT and XCELL algorithms, which enable estimation of cell type abundances from bulk tissue transcriptomes, were analyzed to assess the percentage of immune cell types. We applied CIBERSORT and XCELL methods in TIMER (http://timer.comp-genomics.org/) to infer the infiltration fraction of different types of immune cells among GC samples in the TCGA-STAD dataset based on the FPKM of genes in GC samples.
For the correlation between the hub FRGs and immune cell markers, we used the correlation function in TIMER (https://cistrome.shinyapps.io/timer/). The TCGA-STAD dataset was selected for the correlation analysis.
2.4 Gene Ontology Enrichment Analysis
GO terms of selected genes were enriched using DAVID (the database for annotation, visualization, and integrated discovery, https://david.ncifcrf.gov/), which is an online tool for functional annotation and enrichment analysis to reveal biological features related to large gene lists. The visualization of representative biological process GO terms was performed by the ggplot2 package in R4.0.5.
2.5 Long Non-Coding RNAs-Hub Ferroptosis-Related Genes Network Analysis
LncRNAs were annotated by GENCODE (version 25) (https://www.gencodegenes.org/). The correlation between lncRNAs and the hub FRGs was calculated by Pearson’s correlation analysis. The connectivity of a given gene was measured by the sum of absolute values of Pearson’s correlation coefficient between lncRNAs and the hub FRGs. The red color represents more connectivity between two genes.
2.6 Statistical Analyses
The statistical analyses, including t-tests and log-rank tests, were performed using R 4.0.5. The limma package in R 4.0.5 was used to detect DEGs with the cutoff of p-value <0.05 and fold change >1.5. Data visualization was performed by the ggplot2 package in R 4.0.5. p < 0.05 was considered to be statistically significant.
3 Results
3.1 Ferroptosis-Related Genes Play Crucial Roles in Gastric Carcinogenesis and Progression
We show the workflow chart of this study in Figure 1. To identify the key genes involved in the process of gastric carcinogenesis as well as prognosis, we conducted an in-depth analysis of public RNA-Seq data from the TCGA-STAD dataset of 375 gastric cancer (GC) and 32 adjacent normal tissues. Based on the expression profile in the GC samples of the TCGA-STAD dataset, 9,712 differentially expressed genes (DEGs) were analyzed in GC tissues compared with adjacent normal tissues (fold change >1.5, p-value <0.05) (Figure 2A; Supplementary Table S1). Among these DEGs, 7015 were upregulated in GC and 2,697 were downregulated in GC (Figure 2A). To assess the expression of FRGs in gastric carcinoma and to determine their roles in gastric carcinogenesis, we obtained 289 FRGs from previous studies (Stockwell et al., 2017; Bersuker et al., 2019; Doll et al., 2019; Hassannia et al., 2019; Liu et al., 2020a; Liang et al., 2020) (Supplementary Table S2). Nearly 65% (187 of 289) of FRGs were differentially expressed between GC tissues and adjacent mucosa in the TCGA-STAD dataset (Figure 2A; Supplementary Table S3). Among them, 133 FRGs were GC-upregulated, and 54 FRGs were GC-downregulated (Figure 2A; Supplementary Table S3). GO enrichment analysis of the above FRGs revealed multiple significantly enriched signaling pathways associated with GC, as well as crucial pathways related to ferroptosis. GC-upregulated FRGs were significantly enriched in the cellular response to hydrogen peroxide and cellular iron ion homeostasis pathways, whereas GC-downregulated FRGs were enriched in the oxidation-reduction process, response to cAMP, and cell cycle arrest GO terms, indicating the crucial roles of ferroptosis in GC (Figure 2B; Supplementary Table S4).
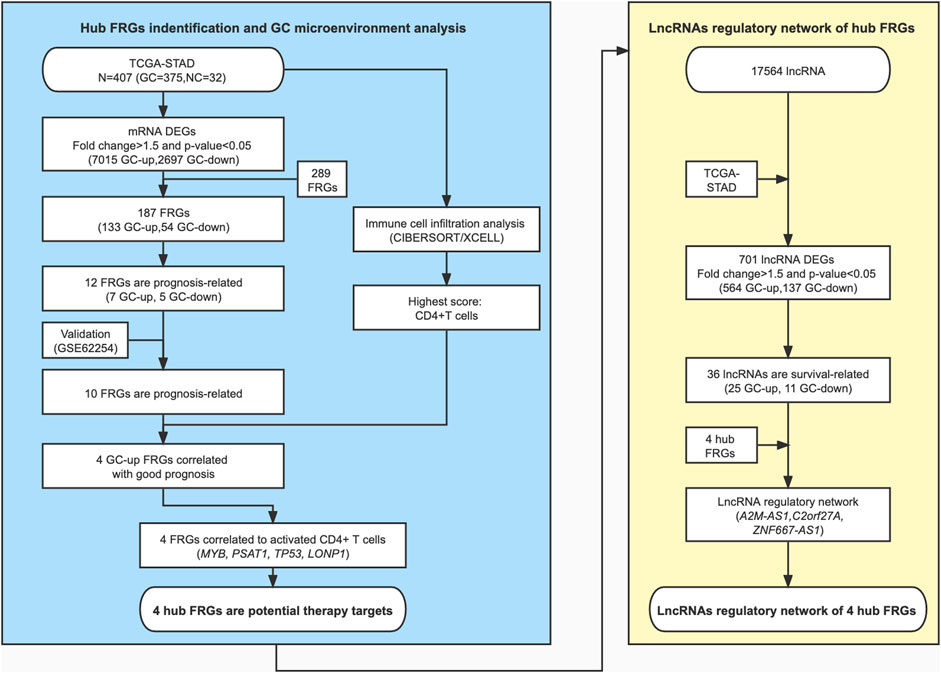
FIGURE 1. A schematic diagram of the study design and patient cohorts for the identification of hub ferroptosis-related genes.
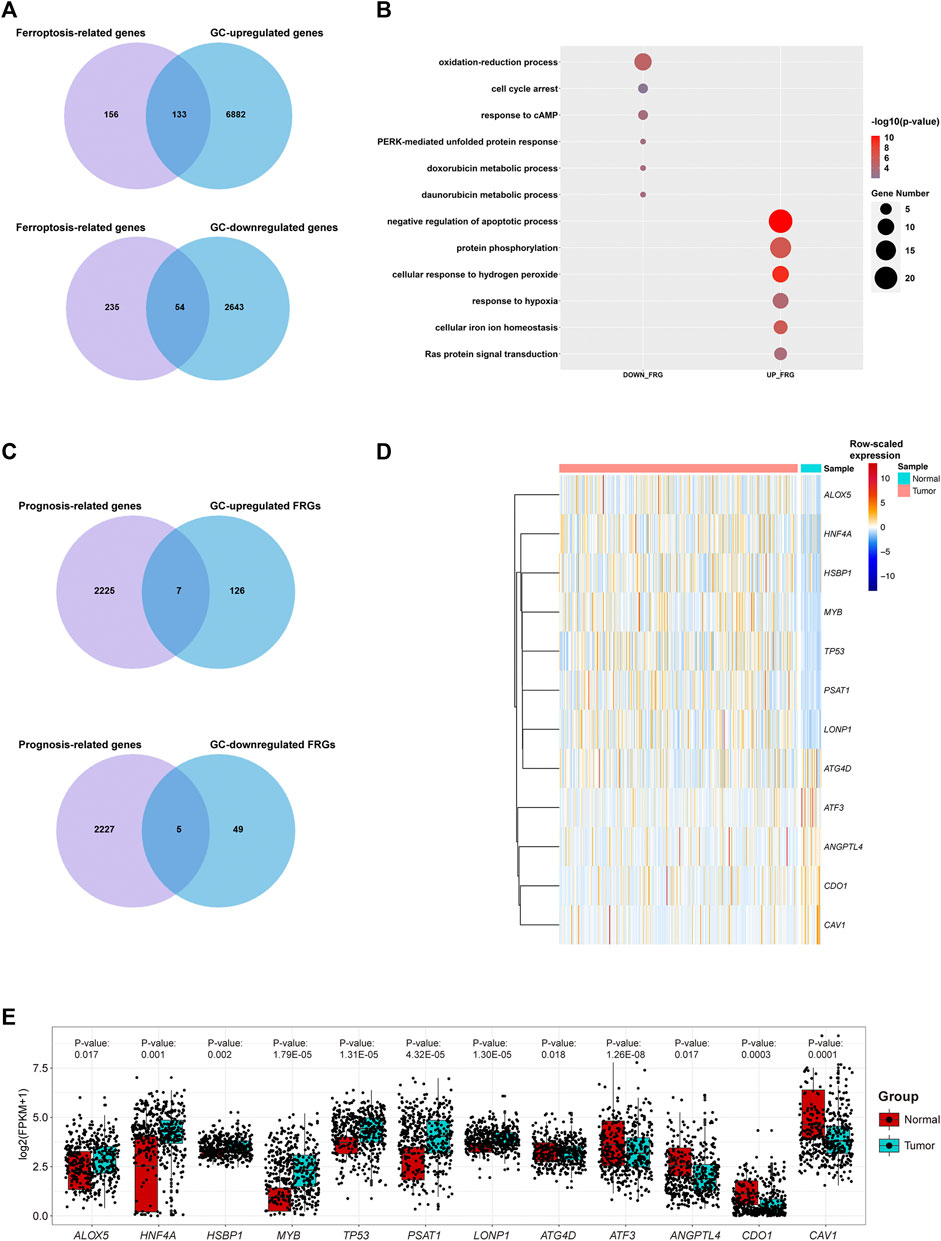
FIGURE 2. Transcriptome profiling of gastric cancer samples in the TCGA dataset. (A) Venn diagram representation of ferroptosis-related genes (FRGs) and differentially expressed genes (DEGs) between GC samples and adjacent cancer samples in the TCGA dataset. (B) Representative GO terms of GC-differentially expressed FRGs in the TCGA dataset. (C) Venn diagram representation of prognosis-related genes and GC-differentially expressed FRGs in the TCGA dataset. (D) Heatmap plot for raw-scaled expression of important FRGs in GC samples and adjacent cancer samples in the TCGA dataset. (E) Boxplot for the expression (log(FPKM+1)) of the 12 differentially expressed FRGs.
Furthermore, seven differentially expressed FRGs were significantly associated with good prognosis, and five differentially expressed FRGs were significantly associated with poor prognosis (Figure 2C). The expression levels of these 12 genes in the TCGA-STAD dataset were shown in Figures 2D,E. The TCGA-STAD cohort was stratified into high and low groups according to the median expression level of each gene, and all 12 genes were significantly correlated with prognosis (p < 0.05, Figures 3A,B). Among them, seven GC-upregulated FRGs (LONP1, MYB, PSAT1, TP53, HNF4A, HSBP1, and ALOX5) and five GC-downregulated FRGs (ATF3, CAV1, ANGPTL4, CDO1, and ATG4D) were prognosis-related genes (Figures 3A,B). To validate these results of the GC prognosis-related FRGs, we analyzed another transcriptome dataset (GSE62254) of GC with corresponding survival data (Cristescu et al., 2015). The results showed that 10 (LONP1, MYB, PSAT1, TP53, HNF4A, ALOX5, ATF3, CAV1, ANGPTL4, and CDO1) of these 12 FRGs were also significantly correlated with prognosis in the GSE62254 dataset (Supplementary Figure S1), indicating that these 10 FRGs may influence ferroptosis and GC progression.
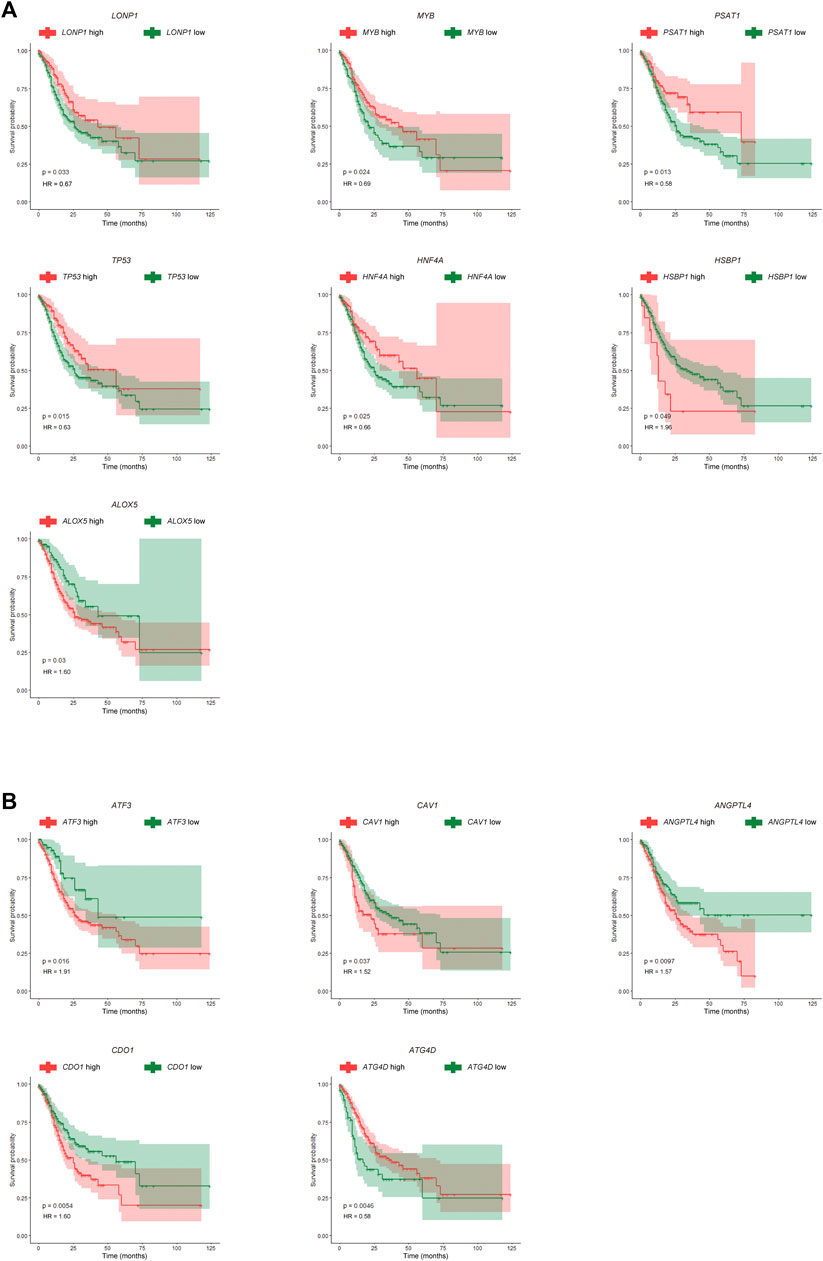
FIGURE 3. Kaplan-Meier (K-M) survival curves for prognosis-related FRGs in GC. (A) K-M survival curves and p-values for the seven GC-upregulated and prognosis-related FRGs. (B) K-M survival curves and p-values for the five GC-downregulated and prognosis-related FRGs.
3.2 Four Hub Ferroptosis-Related Genes May be Related to CD4+ T Cell Activation
To investigate the relationship between the important FRGs and the tumor microenvironment of GC, we calculated the different immune cell infiltration scores of patients in the TCGA-STAD dataset based on CIBERSORT. We found that the infiltrated immune cells in the TCGA-STAD dataset were mainly CD4+ memory T cells, CD8+ T cells, naïve B cells, Tregs, and so on. Among them, CD4+ T cells, including Tregs, follicular helper T cells, activated memory CD4+ T cells, resting memory CD4+ T cells, and naïve CD4+ T cells, had the highest immune cell infiltration score and the largest number of infiltrated patients (Figures 4A,B; Supplementary Table S5). The main type of infiltrated immune cells in GC patients might be CD4+ T cells. Among them, resting or activated memory CD4+ T cells accounted for a large proportion. Subsequently, we explored the Pearson’s correlation coefficient of the expression of prognosis-related FRGs and different immune cell infiltration scores. Interestingly, we found that most GC prognosis-related FRGs were significantly correlated with one or more immune cell infiltration scores (Figure 4C). More interestingly, four of the GC-upregulated FRGs (LONP1, MYB, PSAT1, and TP53) showed a significant positive correlation with activated CD4+ T cell infiltration (Pearson’s correlation coefficient >0.1 and p-value ≤ 0.01, Figures 4C,D; Supplementary Table S6). This indicates that during gastric carcinogenesis, high expression of the hub FRGs (PSAT1, LONP1, MYB, TP53) may promote an increase in activated CD4+ T cells. Since CD4+ T cells help for recruitment, proliferation, and effector function of CD8+ T cells (Bos and Sherman, 2010; Ahrends et al., 2019), we speculate that activated CD4+ T cells could promote the killing efficiency of tumor cells by CD8+ T cells in GC. Our study further showed that the expression of the hub FRGs was negatively correlated with the infiltration level of resting CD4+ T cells (Figure 4C), which suggests that the hub FRGs may promote the conversion of CD4+ T cells from resting to activated, thereby affecting the prognosis of GC patients.
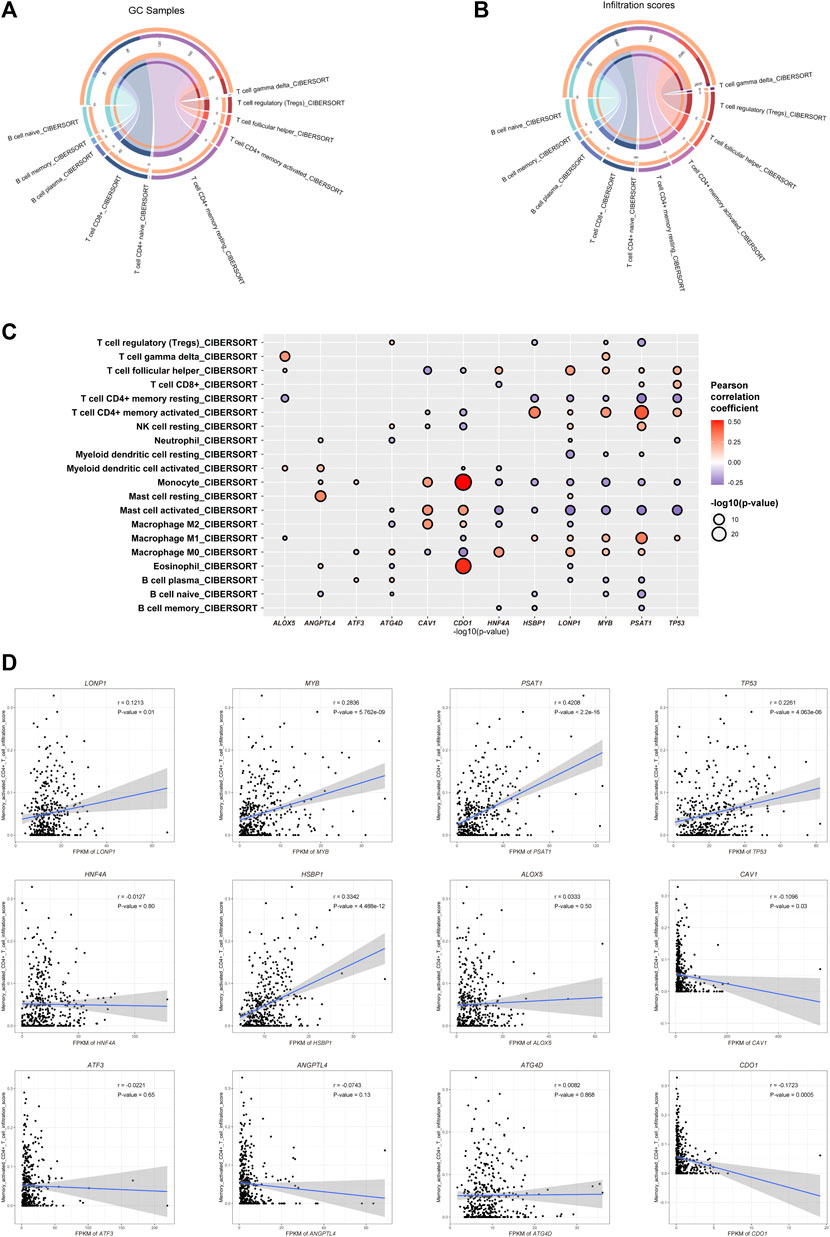
FIGURE 4. Ferroptosis-related genes are related to immune cell infiltration. (A) Counts of each infiltrated immune cell type in GC. (B) Total infiltrated scores of each infiltrated immune cell type in GC. (C) Correlation between the infiltration scores of each immune cell type and the expression of GC-differentially expressed and prognosis-related FRGs. (D) Dot plot showing the positive correlation between the hub FRGs (MYB, LONP1, TP53, and PSAT1) and infiltration scores of CD4+ T cells.
We found that the gene expression of the hub FRGs was significantly related to the marker genes of some CD4+ T cells (Supplementary Figure S2A). Due to the diversity of CD4+ T cell types, we further selected markers of different types of CD4+ T cells to explore the correlation between the hub FRGs and the expression of markers of different types of CD4+ T cells. We found that the expression of TP53 was significantly positively correlated with CCR8, which is a member of the beta chemokine receptor family and serves as one of the Th2 cell markers. LONP1 was positively correlated with IL21R, which is an important molecule involved in many cytokine-induced immune and inflammatory responses and acts as a Th17 cell marker. TP3 is significantly positively correlated with KLRD1, CXCR3, CXCR6, CCR5, and IL12RB1, which serve as Th1 cell markers. Other important co-expression gene pairs also showed a strong relationship among the hub FRGs and multiple markers of different types of CD4+ T cells. In addition, we found that CD25, the main marker of Tregs, did not show much correlation with PSAT1, LONP1, and MYB, indicating that Th cells, not Tregs, were related to the expression of the hub FRGs (Supplementary Figure S2B). To further prove this, we calculated the infiltration scores of cell types of GC using the XCELL algorithm based on the expression data of GC samples in the TCGA-STAD dataset, which allowed us to identify more infiltrated cell types of GC. We also found that the infiltration scores of Th1 (25.1) and Th2 (31.3) cells were much higher than that of Tregs (4.1), indicating that the most dominant types of infiltrated CD4+ T cells in GC are Th1 and Th2 cells, instead of Tregs (Supplementary Figure S2C). In addition, a number of cancer-associated fibroblasts (CAFs) and endothelial cells were also observed, which suggested diverse cell types in the tumor microenvironment of GC. Therefore, we demonstrated that the hub FRGs might promote the activation of different types of CD4+ T cells, especially Th cells, thereby promoting a good prognosis for GC patients.
3.3 Subgroup Analysis of the Hub Ferroptosis-Related Genes, Tumor Microenvironment, and Clinical Characteristics
We initially realized that the hub FRGs may promote the activation of CD4+ T cells, and the activated CD4+ T cells may correlate with good prognosis of GC patients. To further explore the effects of the hub FRGs on the classification, prognosis, and treatment of GC samples, we classified TCGA-STAD GC patients into high-FRG score and low-FRG score groups according to the average expression levels of the hub FRG genes (Figure 5A; Supplementary Table S7). Additionally, to evaluate the relationship between FRGs and the tumor microenvironment, we used the CIBERSORT algorithm to examine the proportions of immune cells. The infiltration score for each patient was calculated, and the results showed that the resting CD4+ T cell infiltration scores in the high-FRG score group were significantly lower than those in the low-FRG score group. The activated CD4+ T cell infiltration scores in the high-FRG group score were significantly higher than those in the low-FRG score group (Figure 5A). Furthermore, the Kaplan-Meier curve indicated that the high-FRG group score had a significantly longer overall survival time than the low-FRG score group (Figure 5B).
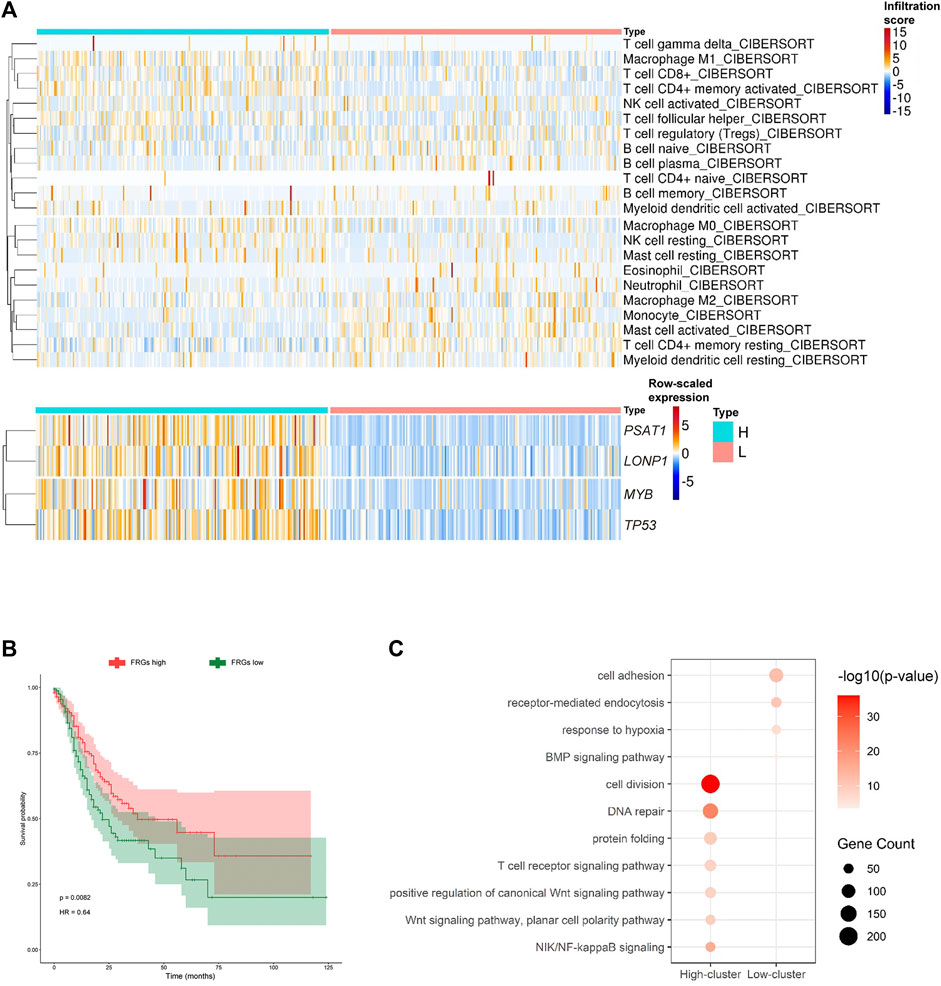
FIGURE 5. MYB, LONP1, TP53, and PSAT1 are related to CD4+ T cell activation. (A) Heatmap showing the infiltration scores of each immune cell type in the two subgroups of GC divided by the average expression of the hub FRGs (high-FRG score and low-FRG score group). (B) K-M survival curves and p-values for the two subgroups divided by the average expression of the hub FRGs. (C) Representative GO terms enriched by DEGs between the two subgroups divided by the average expression of the hub FRGs.
3.4 Identification of Pathways Related to CD4+ T Cell Activation by the Hub Ferroptosis-Related Genes
To identify the pathways related to CD4+ T cell activation by the hub FRGs, we analyzed the enriched pathways of GO terms in the high-FRG score group and low-FRG score group. The highly expressed genes in the high-FRG score group were significantly enriched in cell division, DNA repair, protein folding, T cell receptor, Wnt and NIK/NF-kappaB signaling pathways. In contrast, the low-FRG score group was significantly enriched in cell adhesion, receptor-mediated endocytosis, response to hypoxia, and the BMP signaling pathway (Figure 5C; Supplementary Table S8). As a result, it was possible that the hub FRGs mediated CD4+ T cell activation by cell division, DNA repair, protein folding, T cell receptor, Wnt and NIK/NF-kappaB signaling pathways.
3.5 Long Non-Coding RNAs can Regulate the Hub Ferroptosis-Related Genes and Act as Potential Intervention Targets
We identified the hub FRGs that might contribute to the good prognosis of GC by promoting the activation of CD4+ T cells. However, their potential upstream regulation mechanism is still unknown. As the “dark matter of the genome,” long noncoding RNAs (lncRNAs) have been reported to regulate gene expression through recruiting regulatory complexes, interacting with RNA binding proteins, interfering with transcription, and other mechanisms (Chang and Han, 2016; Shankaraiah et al., 2018; Gao et al., 2021). To investigate the possible regulatory mechanisms of the hub FRGs and lncRNAs, we further analyzed the lncRNAs related to the hub FRGs based on Pearson’s correlation coefficient of gene expression. We first screened the expression of 17,564 lncRNAs (from GENCODE, version 25) in the TCGA-STAD dataset. The results showed that 701 lncRNAs were differentially expressed (564 were significantly upregulated and 137 were downregulated in the GC group) (Figure 6A). A total of 36 of them were significantly related to the prognosis of GC patients (25 GC upregulated genes and 11 GC downregulated genes) (Figures 6B,C; Supplementary Table S9). Subsequently, we constructed a lncRNA-FRG interaction network based on the connectivity of genes in the interaction network (Figures 6D,E). Among them, three lncRNAs with the strongest regulatory relationship with the hub FRGs were A2M-AS1, C2orf27A, and ZNF667-AS1 (Figure 6E). Since they were also negatively correlated with activated CD4+ T cell infiltration (Supplementary Figure S3), we demonstrated that the three lncRNAs might potentially negatively regulate the hub FRGs, which in turn inhibit the activation of CD4+ T cells. High expression of these three lncRNAs was associated with poor prognosis in GC patients (Figure 6F). Therefore, lncRNAs A2M-AS1, C2orf27A, and ZNF667-AS1 may serve as potential lncRNA targets for regulating ferroptosis, CD4+ T cell activation, and the prognosis of GC. Effective interventions can help promote CD4+ T cell activation in GC patients and improve the efficacy of immunotherapy.
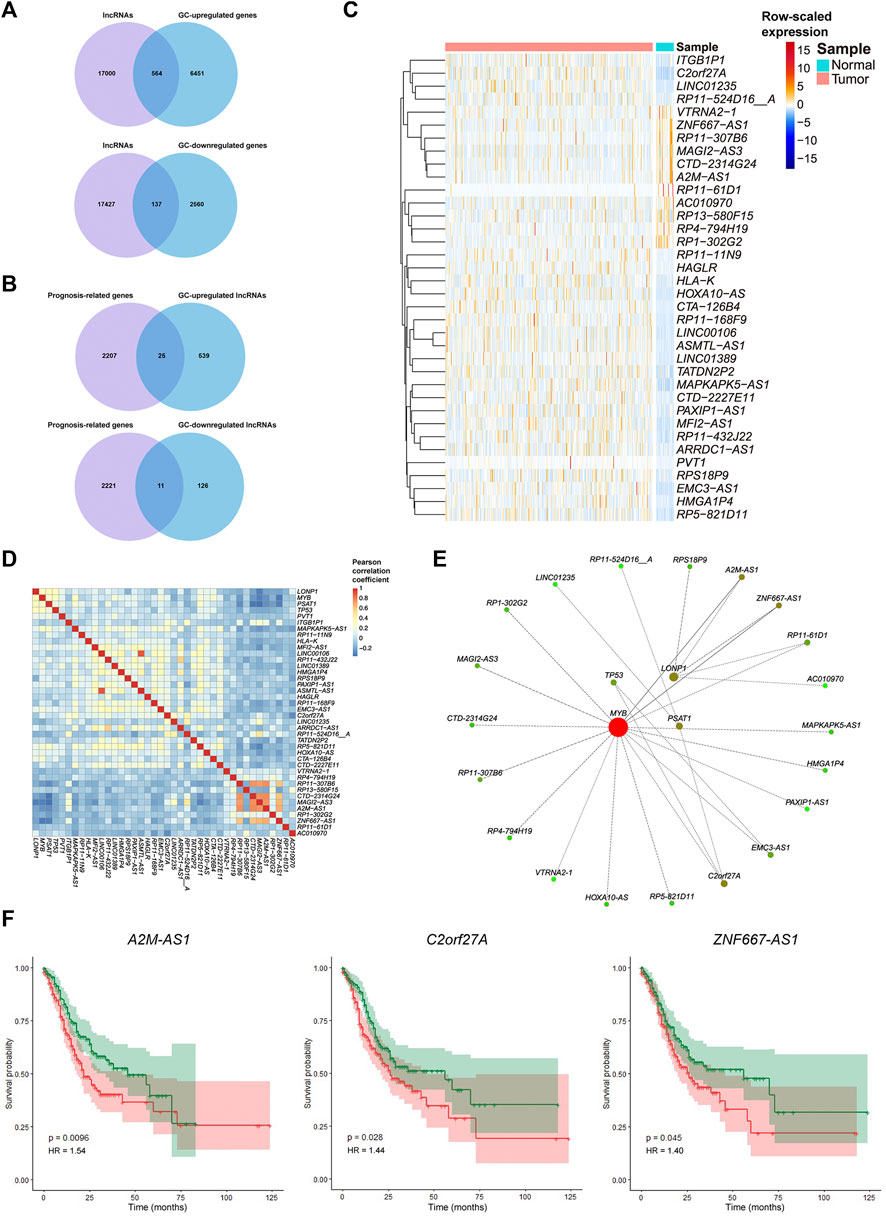
FIGURE 6. Relationships of the expression of the hub FRGs and their correlated lncRNAs involved in tumorigenesis and prognosis of GC. (A) Venn diagram representation of lncRNAs obtained from GENCODE (version 25) and DEGs between GC samples and adjacent cancer samples in the TCGA dataset. (B) Venn diagram representation of prognosis-related genes and GC-differentially expressed lncRNAs in the TCGA dataset. (C) Heatmap plot for the expression of important lncRNAs in GC samples and adjacent cancer samples in the TCGA dataset. (D) Correlation heatmap for Pearson’s coefficient of the expression of important lncRNAs and hub FRGs. (E) Interaction network of important lncRNAs and hub FRGs based on their connectivity. Red represents more connectivity between two genes. (F) K-M survival curves and p-values for the three important lncRNAs (A2M-AS1, C2orf27A, and ZNF667-AS1). Patients with high or low expression of one of the three lncRNAs are labeled with a red line or green line, respectively.
4 Discussion
In this study, we provide a comprehensive transcriptome-wide analysis of ferroptosis-related genes (FRGs) in gastric cancer (GC). Twelve GC-differentially expressed and prognosis-related FRGs were identified as important genes in the TCGA-STAD dataset. Ten of these twelve GC-differentially expressed and prognosis-related FRGs were further validated in the GSE62254 dataset. Interestingly, the high expression of the good-prognosis-related hub FRGs (PSAT1, MYB, TP53, and LONP1) was positively correlated with activated CD4+ T cell infiltration, while negatively correlated with resting CD4+ T cell infiltration in the TCGA-STAD dataset. Thus, we suggest that the hub FRGs have the potential to be involved in CD4+ T cell activation, especially Th cells. The activated Th cells may promote antitumor immunity in GC (Dadaglio et al., 2020). PSAT1 (phospholipid sterol acyltransferase 1) has been reported to be critically important for the transition from p-Pyr to p-Ser (Mullarky et al., 2016) and further affects ferroptosis (Zhang et al., 2019). MYB (C-Myb) can interact directly with CDO1 and play a significant role in ferroptosis (Hao et al., 2017). TP53 serves as a vital factor in cellular ferroptosis in response to various damages (Wang et al., 2016; Wang et al., 2019b). LONP1 has also been reported to be involved in ferroptosis by regulating GPX4 (Wang et al., 2020a). It has been previously reported that ferroptosis is involved in regulating CD4+ T cell homeostasis (Yao et al., 2021). Our results further revealed that the hub FRGs mainly regulate the T cell receptor signaling pathway, NIK/NF-kappaB signaling pathway, and Wnt signaling pathway. To our knowledge, NIK/NF-kappaB regulates the balance of different types of CD4+ T cells (Rowe et al., 2013). NIK/NF-kappaB activation is also involved in T helper (Th) cell differentiation, including Th17 cell differentiation (Park et al., 2014). The Wnt signaling pathway is essential for activating CD4+ T cells and restoring immune homeostasis (Schenkel et al., 2010; Sun et al., 2017). Although these three significant pathways might be the mechanisms of the activation of CD4+ T cells induced by the hub FRGs in GC, further studies are needed to provide conclusive evidence for the specific mechanisms in the tumor microenvironment (TME).
The TME plays a crucial role in the progression and treatment outcomes of tumors. However, the establishment and function of the TME remain obscure due to its complex cellular composition (Yao et al., 2020). The TME consists of multiple cell types, including blood vessels, fibroblasts, immune cells, and other tissue-resident cells (Dai et al., 2017). In addition, the immune status of the TME is extremely complex (Paudel et al., 2019). It has been reported that the infiltration of cytotoxic T cells and memory T cells was associated with good prognosis in gastric patients (Chang et al., 2014). Although the current tumor immunotherapy targets mainly focus on CD8+ T cells, the role of conventional CD4+ T cells in tumor immunity has gradually attracted attention (Nishikawa and Koyama, 2021). CD4+ effector T cells can enhance immunity by regulating dendritic cells or stimulating other proinflammatory cells of the myeloid lineage (Behrens et al., 2004). Since we found that CD4+ T cells are the main type of infiltrated immune cells in GC, our study mainly investigates the regulatory relationships and potential mechanism linking FRGs and CD4+ T cell activation in GC. Our results showed that activation of some subtypes of CD4+ T cells, especially Th1 cells, can promote T cell responses and enhance the effect of immunotherapy.
We demonstrated that FRGs play crucial roles in CD4+ T cell activation. However, the lack of knowledge of CD4+ T cells in immunotherapy limits their application. In this study, we found that the activation of Th cells, instead of Tregs, is related to the hub FRGs. The activation of Th cells can provide cytokines to support important regulatory and effector functions of T cells (Subhash et al., 2015). Since PSAT1, MTB, TP53, and LONP1 were all suggested to be related to CD4+ T cell activation, especially Th cells, we speculate that they have the potential for adjuvant immunotherapy based on CD4+ T cells and improve survival in patients with GC. This further supports the suggestion that these genes have the potential to serve as targets for adjuvant immunotherapy.
We constructed the upstream regulatory network of the hub FRGs by lncRNA. Three lncRNAs (A2M-AS1, C2orf27A, and ZNF667-AS1) were identified to be related to the hub FRGs. Among them, A2M-AS1 was reported to be involved in the invasion, migration, and progression of breast cancer (Fang et al., 2020; Liu et al., 2020b). In addition, A2M-AS1 was also reported to lessen cardiomyocyte injury caused by hypoxia/reoxygenation (H/R) by regulating IL1R2 (Song et al., 2020). Since hypoxia and reoxygenation are also reported to be related to ferroptosis (Li et al., 2020; Eleftheriadis et al., 2021), our study provides a further potential mechanism of A2M-AS1, ferroptosis, and even H/R. C2orf27A is reported to be associated with sorafenib resistance, clinical cancer stages, and pathological tumor grades in liver cancer (Yuan et al., 2021). Our study confirms the role of C2orf27A in tumor progression and provides a new view of it in gastric carcinogenesis and prognosis, as well as its potential relationship with FRGs. ZNF667-AS1 is also a tumor-related lncRNA. Previous studies have reported that ZNF667-AS1 can suppress the progression of nasopharyngeal carcinoma colorectal cancer and cervical cancer (Li et al., 2019; Chen et al., 2020; Zhuang et al., 2021). However, there are also some reports that indicate that ZNF667-AS1 can inhibit the inflammatory response and promote liver metastasis in acute myeloid leukemia (AML) (Wang et al., 2020b). We further identified that it can promote the progression of GC, probably by regulating FRGs and CD4+ T cell activation. These studies provide strong evidence of our lncRNA regulatory network analysis. Their biological roles in GC need further experimental validation. Thus, the regulation of these essential lncRNAs has the potential to act as a novel intervention strategy for antitumor immunotherapy mediated by enhanced CD4+ T cell activation, especially Th cells.
Taken together, our study indicates the relationship between ferroptosis and the TME in GC. We propose that ferroptosis is related to CD4+ T cell activation in GC. We identified three lncRNAs were predicted to regulate the hub FRGs. The hub FRGs (PSAT1, MYB, TP53, and LONP1) and three lncRNAs (A2M-AS1, C2orf27A, and ZNF667-AS1) have the potential to serve as novel targets for adjuvant immunotherapy. Further studies on ferroptosis-induced CD4+ T cell activation will provide a new perspective on the complexity of the TME and diverse strategies for cancer immunotherapy.
Data Availability Statement
The original contributions presented in the study are included in the article/Supplementary Material, further inquiries can be directed to the corresponding authors.
Author Contributions
FY and YZ initiated the study, performed the analysis, prepared the figures, and wrote the manuscript. ZP, YL, JC, ZW, BC, JC, and KT proposed useful comments, suggestions, and revised the manuscript. JD discussed and optimized the pictures in this manuscript. ZP, YN and LM designed the structure and revised the manuscript. All authors contributed to the article and approved the submitted version.
Funding
This study was supported by Shenzhen Foundation of Science and Technology (grant number JCYJ20170817172116272), Sanming Project of Medicine in Shenzhen (SZSM201812079) and Shenzhen High-level Hospital Construction Fund (2019).
Conflict of Interest
The authors declare that the research was conducted in the absence of any commercial or financial relationships that could be construed as a potential conflict of interest.
Publisher’s Note
All claims expressed in this article are solely those of the authors and do not necessarily represent those of their affiliated organizations, or those of the publisher, the editors and the reviewers. Any product that may be evaluated in this article, or claim that may be made by its manufacturer, is not guaranteed or endorsed by the publisher.
Supplementary Material
The Supplementary Material for this article can be found online at: https://www.frontiersin.org/articles/10.3389/fcell.2021.797339/full#supplementary-material
Supplementary Figure 1 | K-M survival curves and p-values for the 12 GC-differentially expressed and prognosis-related FRGs based on the GSE62254 dataset.
Supplementary Figure 2 | Correlation between expression of important FRGs and markers of different types of CD4+ T cells. (A) Correlation between the expression of important FRGs and classical markers of CD4+ T cells. (B) Correlation between the expression of important FRGs and markers of Tfh cells, Th1 cells, Treg cells, Th2 cells, and Th17 cells. (C) Infiltration scores of different cell types in the GC environment based on the XCELL method.
Supplementary Figure 3 | Correlation scores among the three lncRNAs, FRGs, and activated CD4+ T cell infiltration scores.
References
Ahrends, T., Busselaar, J., Severson, T. M., Bąbała, N., de Vries, E., Bovens, A., et al. (2019). CD4+ T Cell Help Creates Memory CD8+ T Cells with Innate and Help-independent Recall Capacities. Nat. Commun. 10 (1), 5531. doi:10.1038/s41467-019-13438-1
Arumugam, M., and Sugin Lal Jabaris, S. (2021). Recent Preclinical Study Offers a Promising Clue: Role of Regulatory T Cells as Biomarkers in Migraine. Immunol. Lett. 240, 9. doi:10.1016/j.imlet.2021.09.004
Behrens, G., Li, M., Smith, C. M., Belz, G. T., Mintern, J., Carbone, F. R., et al. (2004). Helper T Cells, Dendritic Cells and CTL Immunity. Immunol. Cel Biol 82 (1), 84–90. doi:10.1111/j.1440-1711.2004.01211.x
Bejarano, L., Jordāo, M. J. C., and Joyce, J. A. (2021). Therapeutic Targeting of the Tumor Microenvironment. Cancer Discov. 11 (4), 933–959. doi:10.1158/2159-8290.CD-20-1808
Bersuker, K., Hendricks, J. M., Li, Z., Magtanong, L., Ford, B., Tang, P. H., et al. (2019). The CoQ Oxidoreductase FSP1 Acts Parallel to GPX4 to Inhibit Ferroptosis. Nature 575 (7784), 688–692. doi:10.1038/s41586-019-1705-2
Bos, R., and Sherman, L. A. (2010). CD4+ T-Cell Help in the Tumor Milieu Is Required for Recruitment and Cytolytic Function of CD8+ T Lymphocytes. Cancer Res. 70 (21), 8368–8377. doi:10.1158/0008-5472.CAN-10-1322
Chang, C. P., and Han, P. (2016). Epigenetic and lncRNA Regulation of Cardiac Pathophysiology. Biochim. Biophys. Acta 1863 (7 Pt B), 1767–1771. doi:10.1016/j.bbamcr.2016.03.005
Chang, W.-J., Du, Y., Zhao, X., Ma, L. Y., and Cao, G. W. (2014). Inflammation-related Factors Predicting Prognosis of Gastric Cancer. Wjg 20 (16), 4586–4596. doi:10.3748/wjg.v20.i16.4586
Chen, X., Huang, Y., Shi, D., Nie, C., Luo, Y., Guo, L., et al. (2020). LncRNA ZNF667-AS1 Promotes ABLIM1 Expression by Adsorbing microRNA-1290 to Suppress Nasopharyngeal Carcinoma Cell Progression. Ott 13, 4397–4409. doi:10.2147/OTT.S245554
Corthay, A. (2009). How Do Regulatory T Cells Work? Scand. J. Immunol. 70 (4), 326–336. doi:10.1111/j.1365-3083.2009.02308.x
Cristescu, R., Lee, J., Nebozhyn, M., Kim, K.-M., Ting, J. C., Wong, S. S., et al. (2015). Molecular Analysis of Gastric Cancer Identifies Subtypes Associated with Distinct Clinical Outcomes. Nat. Med. 21 (5), 449–456. doi:10.1038/nm.3850
Dadaglio, G., Fayolle, C., Oberkampf, M., Tang, A., Rudilla, F., Couillin, I., et al. (2020). IL-17 Suppresses the Therapeutic Activity of Cancer Vaccines through the Inhibition of CD8+ T-Cell Responses. Oncoimmunology 9 (1), 1758606. doi:10.1080/2162402X.2020.1758606
Dai, Y., Xu, C., Sun, X., and Chen, X. (2017). Nanoparticle Design Strategies for Enhanced Anticancer Therapy by Exploiting the Tumour Microenvironment. Chem. Soc. Rev. 46 (12), 3830–3852. doi:10.1039/c6cs00592f
Dixon, S. J., Lemberg, K. M., Lamprecht, M. R., Skouta, R., Zaitsev, E. M., Gleason, C. E., et al. (2012). Ferroptosis: an Iron-dependent Form of Nonapoptotic Cell Death. Cell 149 (5), 1060–1072. doi:10.1016/j.cell.2012.03.042
Doll, S., Freitas, F. P., Shah, R., Aldrovandi, M., da Silva, M. C., Ingold, I., et al. (2019). FSP1 Is a Glutathione-independent Ferroptosis Suppressor. Nature 575 (7784), 693–698. doi:10.1038/s41586-019-1707-0
Eleftheriadis, T., Pissas, G., Filippidis, G., Liakopoulos, V., and Stefanidis, I. (2021). Reoxygenation Induces Reactive Oxygen Species Production and Ferroptosis in Renal Tubular Epithelial Cells by Activating Aryl Hydrocarbon Receptor. Mol. Med. Rep. 23, 23. doi:10.3892/mmr.2020.11679
Fang, K., Caixia, H., Xiufen, Z., Zijian, G., and Li, L. (2020). Screening of a Novel Upregulated lncRNA, A2M-AS1, that Promotes Invasion and Migration and Signifies Poor Prognosis in Breast Cancer. Biomed. Res. Int. 2020, 9747826. doi:10.1155/2020/9747826
Feau, S., Garcia, Z., Arens, R., Yagita, H., Borst, J., and Schoenberger, S. P. (2012). The CD4+ T-Cell Help Signal Is Transmitted from APC to CD8+ T-Cells via CD27-CD70 Interactions. Nat. Commun. 3, 948. doi:10.1038/ncomms1948
Gao, J., Chen, X., Wei, P., Wang, Y., Li, P., and Shao, K. (2021). Regulation of Pyroptosis in Cardiovascular Pathologies: Role of Noncoding RNAs. Mol. Ther. - Nucleic Acids 25, 220–236. doi:10.1016/j.omtn.2021.05.016
Gu, Y., Chen, Y., Jin, K., Cao, Y., Liu, X., Lv, K., et al. (2020). Intratumoral CD103+CD4+ T Cell Infiltration Defines Immunoevasive Contexture and Poor Clinical Outcomes in Gastric Cancer Patients. Oncoimmunology 9 (1), 1844402. doi:10.1080/2162402X.2020.1844402
Hao, S., Yu, J., He, W., Huang, Q., Zhao, Y., Liang, B., et al. (2017). Cysteine Dioxygenase 1 Mediates Erastin-Induced Ferroptosis in Human Gastric Cancer Cells. Neoplasia 19 (12), 1022–1032. doi:10.1016/j.neo.2017.10.005
Hassannia, B., Vandenabeele, P., and Vanden Berghe, T. (2019). Targeting Ferroptosis to Iron Out Cancer. Cancer Cell 35 (6), 830–849. doi:10.1016/j.ccell.2019.04.002
Li, W., Li, W., Leng, Y., Xiong, Y., and Xia, Z. (2020). Ferroptosis Is Involved in Diabetes Myocardial Ischemia/Reperfusion Injury through Endoplasmic Reticulum Stress. DNA Cel Biol. 39 (2), 210–225. doi:10.1089/dna.2019.5097
Li, Y. J., Yang, Z., Wang, Y. Y., and Wang, Y. (2019). Long Noncoding RNA ZNF667‐AS1 Reduces Tumor Invasion and Metastasis in Cervical Cancer by Counteracting microRNA‐93‐3p‐dependent PEG3 Downregulation. Mol. Oncol. 13 (11), 2375–2392. doi:10.1002/1878-0261.12565
Liang, J.-Y., Wang, D.-S., Lin, H.-C., Chen, X.-X., Yang, H., Zheng, Y., et al. (2020). A Novel Ferroptosis-Related Gene Signature for Overall Survival Prediction in Patients with Hepatocellular Carcinoma. Int. J. Biol. Sci. 16 (13), 2430–2441. doi:10.7150/ijbs.45050
Liu, Y., Zhang, Q., Wu, J., Zhang, H., Li, X., Zheng, Z., et al. (2020). Long Non-coding RNA A2M-AS1 Promotes Breast Cancer Progression by Sponging microRNA-146b to Upregulate MUC19. Ijgm 13, 1305–1316. doi:10.2147/IJGM.S278564
Liu, Y., Zhang, X., Zhang, J., Tan, J., Li, J., and Song, Z. (2020). Development and Validation of a Combined Ferroptosis and Immune Prognostic Classifier for Hepatocellular Carcinoma. Front. Cel Dev. Biol. 8, 596679. doi:10.3389/fcell.2020.596679
Ma, R., Shimura, T., Yin, C., Okugawa, Y., Kitajima, T., Koike, Y., et al. (2021). Antitumor Effects of Andrographis via Ferroptosis-associated G-enes in G-astric C-ancer. Oncol. Lett. 22 (1), 523. doi:10.3892/ol.2021.12784
Mullarky, E., Lucki, N. C., Beheshti Zavareh, R., Anglin, J. L., Gomes, A. P., Nicolay, B. N., et al. (2016). Identification of a Small Molecule Inhibitor of 3-phosphoglycerate Dehydrogenase to Target Serine Biosynthesis in Cancers. Proc. Natl. Acad. Sci. USA 113 (7), 1778–1783. doi:10.1073/pnas.1521548113
Nishikawa, H., and Koyama, S. (2021). Mechanisms of Regulatory T Cell Infiltration in Tumors: Implications for Innovative Immune Precision Therapies. J. Immunother. Cancer 9, e002591. doi:10.1136/jitc-2021-002591
Park, S.-H., Cho, G., and Park, S.-G. (2014). NF-κB Activation in T Helper 17 Cell Differentiation. Immune Netw. 14 (1), 14–20. doi:10.4110/in.2014.14.1.14
Paudel, S., Mehtani, D., and Puri, N. (2019). Mast Cells May Differentially Regulate Growth of Lymphoid Neoplasms by Opposite Modulation of Histamine Receptors. Front. Oncol. 9, 1280. doi:10.3389/fonc.2019.01280
Rowe, A. M., Murray, S. E., Raué, H.-P., Koguchi, Y., Slifka, M. K., and Parker, D. C. (2013). A Cell-Intrinsic Requirement for NF-Κb-Inducing Kinase in CD4 and CD8 T Cell Memory. J.I. 191 (7), 3663–3672. doi:10.4049/jimmunol.1301328
Russick, J., Joubert, P. E., Gillard-Bocquet, M., Torset, C., Meylan, M., Petitprez, F., et al. (2020). Natural Killer Cells in the Human Lung Tumor Microenvironment Display Immune Inhibitory Functions. J. Immunother. Cancer 8, 8. doi:10.1136/jitc-2020-001054
Schenkel, J. M., Zloza, A., Li, W., Narasipura, S. D., and Al-Harthi, L. (2010). β-Catenin Signaling Mediates CD4 Expression on Mature CD8+T Cells. J.I. 185 (4), 2013–2019. doi:10.4049/jimmunol.0902572
Shankaraiah, R. C., Veronese, A., Sabbioni, S., and Negrini, M. (2018). Non-coding RNAs in the Reprogramming of Glucose Metabolism in Cancer. Cancer Lett. 419, 167–174. doi:10.1016/j.canlet.2018.01.048
Song, X.-L., Zhang, F.-F., Wang, W.-J., Li, X.-N., Dang, Y., Li, Y.-X., et al. (2020). LncRNA A2M-AS1 Lessens the Injury of Cardiomyocytes Caused by Hypoxia and Reoxygenation via Regulating IL1R2. Genes Genom 42 (12), 1431–1441. doi:10.1007/s13258-020-01007-6
Stockwell, B. R., Friedmann Angeli, J. P., Bayir, H., Bush, A. I., Conrad, M., Dixon, S. J., et al. (2017). Ferroptosis: A Regulated Cell Death Nexus Linking Metabolism, Redox Biology, and Disease. Cell 171 (2), 273–285. doi:10.1016/j.cell.2017.09.021
Subhash, V. V., Yeo, M. S., Tan, W. L., and Yong, W. P. (2015). Strategies and Advancements in Harnessing the Immune System for Gastric Cancer Immunotherapy. J. Immunol. Res. 2015, 308574. doi:10.1155/2015/308574
Sun, X., Liu, S., Wang, D., Zhang, Y., Li, W., Guo, Y., et al. (2017). Colorectal Cancer Cells Suppress CD4+ T Cells Immunity through Canonical Wnt Signaling. Oncotarget 8 (9), 15168–15181. doi:10.18632/oncotarget.14834
Sung, H., Ferlay, J., Siegel, R. L., Laversanne, M., Soerjomataram, I., Jemal, A., et al. (2021). Global Cancer Statistics 2020: GLOBOCAN Estimates of Incidence and Mortality Worldwide for 36 Cancers in 185 Countries. CA A. Cancer J. Clin. 71 (3), 209–249. doi:10.3322/caac.21660
Voron, T., Marcheteau, E., Pernot, S., Colussi, O., Tartour, E., Taieb, J., et al. (2014). Control of the Immune Response by Pro-angiogenic Factors. Front. Oncol. 4, 70. doi:10.3389/fonc.2014.00070
Wang, H., Liu, C., Zhao, Y., Zhang, W., Xu, K., Li, D., et al. (2020). Inhibition of LONP1 Protects against Erastin-Induced Ferroptosis in Pancreatic Ductal Adenocarcinoma PANC1 Cells. Biochem. Biophysical Res. Commun. 522 (4), 1063–1068. doi:10.1016/j.bbrc.2019.11.187
Wang, L., Zhang, Z., Li, M., Wang, F., Jia, Y., Zhang, F., et al. (2019). P53-dependent Induction of Ferroptosis Is Required for Artemether to Alleviate Carbon Tetrachloride-Induced Liver Fibrosis and Hepatic Stellate Cell Activation. IUBMB Life 71 (1), 45–56. doi:10.1002/iub.1895
Wang, N., Feng, Y., Xie, J., Han, H., Dong, Q., and Wang, W. (2020). Long Non-coding RNA ZNF667-AS1 Knockdown Curbs Liver Metastasis in Acute Myeloid Leukemia by Regulating the microRNA-206/AKAP13 Axis. Cmar 12, 13285–13300. doi:10.2147/CMAR.S269258
Wang, S.-J., Li, D., Ou, Y., Jiang, L., Chen, Y., Zhao, Y., et al. (2016). Acetylation Is Crucial for P53-Mediated Ferroptosis and Tumor Suppression. Cel Rep. 17 (2), 366–373. doi:10.1016/j.celrep.2016.09.022
Wang, W., Green, M., Choi, J. E., Gijón, M., Kennedy, P. D., Johnson, J. K., et al. (2019). CD8+ T Cells Regulate Tumour Ferroptosis during Cancer Immunotherapy. Nature 569 (7755), 270–274. doi:10.1038/s41586-019-1170-y
Wei, M., Shen, D., Mulmi Shrestha, S., Liu, J., Zhang, J., and Yin, Y. (2018). The Progress of T Cell Immunity Related to Prognosis in Gastric Cancer. Biomed. Res. Int. 2018, 3201940. doi:10.1155/2018/3201940
Yao, M., Ventura, P. B., Jiang, Y., Rodriguez, F. J., Wang, L., Perry, J. S. A., et al. (2020). Astrocytic Trans-differentiation Completes a Multicellular Paracrine Feedback Loop Required for Medulloblastoma Tumor Growth. Cell 180 (3), 502–520. doi:10.1016/j.cell.2019.12.024
Yao, Y., Chen, Z., Zhang, H., Chen, C., Zeng, M., Yunis, J., et al. (2021). Selenium-GPX4 axis Protects Follicular Helper T Cells from Ferroptosis. Nat. Immunol. 22 (9), 1127–1139. doi:10.1038/s41590-021-00996-0
Yuan, L., Xu, B., Yuan, P., Zhou, J., Qin, P., Han, L., et al. (2017). Tumor-infiltrating CD4+ T Cells in Patients with Gastric Cancer. Cancer Cel Int 17, 114. doi:10.1186/s12935-017-0489-4
Yuan, W., Tao, R., Huang, D., Yan, W., Shen, G., and Ning, Q. (2021). Transcriptomic Characterization Reveals Prognostic Molecular Signatures of Sorafenib Resistance in Hepatocellular Carcinoma. Aging 13 (3), 3969–3993. doi:10.18632/aging.202365
Zhang, H., Deng, T., Liu, R., Ning, T., Yang, H., Liu, D., et al. (2020). CAF Secreted miR-522 Suppresses Ferroptosis and Promotes Acquired Chemo-Resistance in Gastric Cancer. Mol. Cancer 19 (1), 43. doi:10.1186/s12943-020-01168-8
Zhang, X., Du, L., Qiao, Y., Zhang, X., Zheng, W., Wu, Q., et al. (2019). Ferroptosis Is Governed by Differential Regulation of Transcription in Liver Cancer. Redox Biol. 24, 101211. doi:10.1016/j.redox.2019.101211
Zhuang, L., Ding, W., Ding, W., Zhang, Q., Xu, X., and Xi, D. (2021). lncRNA ZNF667‐AS1 (NR_036521.1) Inhibits the Progression of Colorectal Cancer via Regulating ANK2/JAK2 Expression. J. Cel Physiol 236 (3), 2178–2193. doi:10.1002/jcp.30004
Glossary
A2M-AS1 A2M Antisense RNA 1
ALOX5 Arachidonate 5-Lipoxygenase
ANGPTL4 Angiopoietin Like 4
ATF3 activating transcription factor 3
ATG4D Autophagy Related 4D Cysteine Peptidase
C2orf27A Chromosome 2 Open Reading Frame 27A
CAFs cancer associated fibroblasts
CAV1 Caveolin 1
CCR5 C-C Motif Chemokine Receptor 5
CCR8 C-C Motif Chemokine Receptor 8
CDO1 Cysteine Dioxygenase Type 1
CTL cytotoxic T lymphocytes
CXCR3 C-X-C Motif Chemokine Receptor 3
CXCR6 C-X-C Motif Chemokine Receptor 6
DAVID the database for annotation, visualization and integrated discovery
FPKM fragments per kilobase per million
FRGs ferroptosis-related genes
GC gastric cancer
GO gene ontology
GPX4 glutathione peroxidase 4
HNF4A hepatocyte nuclear factor 4 alpha
HSBP1 heat shock factor binding protein 1
IL12RB1 Interleukin 12 Receptor Subunit Beta 1
IL21R Interleukin 21 Receptor
KLRD1 Killer Cell Lectin Like Receptor D1
lncRNAs long non-coding RNAs
LONP1 Lon Peptidase 1, Mitochondrial
MYB MYB Proto-Oncogene, Transcription Factor
PPI Protein–Protein Interaction
PSAT1 Phosphoserine Aminotransferase 1
STAD stomach adenocarcinoma
STRING search tool for the retrieval of interacting genes/proteins
TCGA the cancer genome atlas
Th T helper
TME tumor microenvironment
TP53 Tumor Protein P53
Treg regulatory T cells
ZNF667-AS1 ZNF667 Antisense RNA 1 (Head To Head)
Keywords: ferroptosis, CD4+ T cell activation, long non-coding RNAs, tumor microenvironment, gastric cancer, TCGA, immune infiltration, lncRNA
Citation: Yao F, Zhan Y, Pu Z, Lu Y, Chen J, Deng J, Wu Z, Chen B, Chen J, Tian K, Ni Y and Mou L (2021) LncRNAs Target Ferroptosis-Related Genes and Impair Activation of CD4+ T Cell in Gastric Cancer. Front. Cell Dev. Biol. 9:797339. doi: 10.3389/fcell.2021.797339
Received: 18 October 2021; Accepted: 22 November 2021;
Published: 13 December 2021.
Edited by:
Jiakai Hou, University of Houston, United StatesReviewed by:
Leilei Shi, University of Texas MD Anderson Cancer Center, United StatesMing Sun, Nanjing Medical University, China
Copyright © 2021 Yao, Zhan, Pu, Lu, Chen, Deng, Wu, Chen, Chen, Tian, Ni and Mou. This is an open-access article distributed under the terms of the Creative Commons Attribution License (CC BY). The use, distribution or reproduction in other forums is permitted, provided the original author(s) and the copyright owner(s) are credited and that the original publication in this journal is cited, in accordance with accepted academic practice. No use, distribution or reproduction is permitted which does not comply with these terms.
*Correspondence: Zuhui Pu, pupeter190@163.com; Yong Ni, szniyong@sina.com; Lisha Mou, lishamou@gmail.com
†These authors have contributed equally to this work