Corrigendum: The Regulators Associated With N6-Methyladenosine in Lung Adenocarcinoma and Lung Squamous Cell Carcinoma Reveal New Clinical and Prognostic Markers
- 1School of Laboratory Medicine, Hangzhou Medical College, Hangzhou, China
- 2Southern Marine Science and Engineering Guangdong Laboratory (Zhanjiang), Guangdong Medical University, Zhanjiang, China
- 3Guangdong Provincial Key Laboratory of Systems Biology and Synthetic Biology for Urogenital Tumors, Department of Urology, The First Affiliated Hospital of Shenzhen University, Shenzhen Second People’s Hospital (Shenzhen Institute of Translational Medicine), Shenzhen, China
- 4Department of Radiation Oncology, Hubei Cancer Hospital, Tongji Medical College, Huazhong University of Science and Technology, Wuhan, China
- 5Anhui Clinical and Preclinical Key Laboratory of Respiratory Disease, The First Affiliated Hospital of Bengbu Medical College, Bengbu, China
N6-methyladenosine (m6A) methylation is of significant importance in the initiation and progression of tumors, but how specific genes take effect in different lung cancers still needs to be explored. The aim of this study is to analyze the correlation between the m6A RNA methylation regulators and the occurrence and development of lung cancer. The data of lung adenocarcinoma (LUAD) and lung squamous cell carcinoma (LUSC) were obtained through the TCGA database. We systematically analyzed the related pathological characteristics and prognostic factors by applying univariate and multivariate Cox regression, as well as LASSO Cox regression. Some of 23 m6A regulators are identified as having high expression in lung cancer. In addition, risk score has been shown to be an independent prognostic factor in lung cancer. Our research not only fully reveals that m6A regulators and clinical pathological characteristics are potentially useful with respect to survival and prognosis in different lung tumors but also can lay a theoretical root for the treatment for lung cancer—notably, to point out a new direction for the development of treatment.
Introduction
M6A methylation, as a dynamic reversible process, is regulated by three enzymes: demethylase (erasers), function manager (readers), and methyltransferase complex (writers) (Yang et al., 2018; Zhou et al., 2020). Erasers include FTO and ALKBH5 (Li et al., 2017); readers include YTHDC1, YTHDC2, YTHDF1, YTHDF2, and HNRNPC (Zhang et al., 2019; He et al., 2020); and writers include MTETL3, METTL14, WTAP, KIAA1429, RBM15, and ZC3H13 (Tuncel and Kalkan, 2019; Asada et al., 2020). It is these m6A methylation regulators that are closely correlated with different human diseases, especially with cancer (Wang et al., 2018). A growing majority of evidence indicates that m6A has the capacity to exert a double effect in tumors. On the one hand, m6A regulates the expression of oncogenes and tumor suppressor genes, which act as promoting and suppressing cancer, respectively. On the other hand, the expression level of m6A and the expression and activity of m6A enzyme can be regulated to affect the function of m6A. Ultimately, they achieve the purpose of adjusting the differentiation of cancer stem cells and regulating T cell differentiation and immune homeostasis.
In the LUAD, the increased expression of METTL3 can enhance the translation of oncogene BRD4 by forming an mRNA ring with EIF3. With that, METTL3 accelerates the translation of oncogene by promoting ribosome recycling, thereby providing an easier way for the invasion and metastasis of lung cancer cells (Choe et al., 2018; Ni et al., 2021). The expression of FTO is elevated in the LUSC, and FTO inhibits cancer formation by inhibiting m6A methylation, which inhibits expression of the oncogene MZF1 eventually. Both m6A with high expression and MZF1 with deficient expression inhibit tumor development (Liu et al., 2018).
Generally speaking, patients with early treatment of lung cancer have a good prognosis, but most patients are diagnosed to be in the advanced stage of lung cancer, whose prognosis is poor (Wozniak, 2012; Zhu et al., 2019). Nowadays, TNM staging is still the dominant method to analyze the prognosis of lung cancer, although there is a certain degree of error and lack of individuality (Wozniak and Schwartz, 2018). Our preoccupation is searching for further accurate prognostic factors from the point of view of gene and molecule, for the sake of making accurate prognostic analysis and the individualized treatment selection.
In this article, we acquired the information of patients with lung adenocarcinoma (LUAD) and lung squamous cell carcinoma (LUSC), respectively. Pan-cancer data was the total of LUAD and LUSC data. The expression of m6A methylation regulators was analyzed to shed light on if it was related to LUAD, LUSC, and pan-cancer. Especially significant, based on these data, we try to find out the clinical correlation between m6A methylation regulators and lung cancer (Li et al., 2021).
Results
The Overview of m6A RNA Methylation Regulators in Lung Cancer
We drew a heatmap of LUAD, LUSC, and pan-cancer data after diff analysis firstly. Various gene expression levels were displayed as follows (Figure 1).
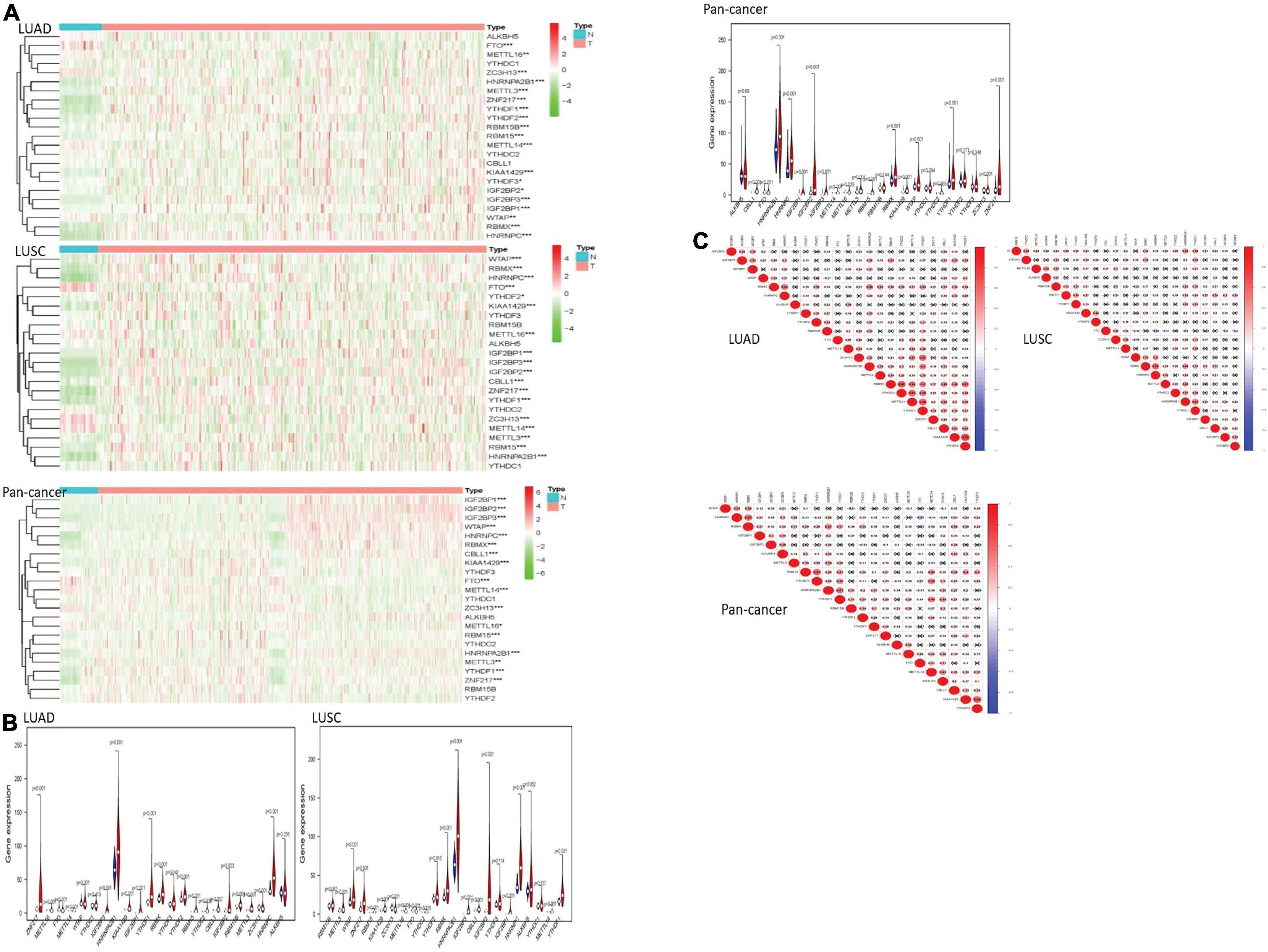
Figure 1. The overview of m6A RNA methylation regulators in lung cancer. (A) The figures reflect the expression levels of 23 m6A RNA methylation regulators in normal tissues and lung cancer tissues. When the gene expression level is high, the color turns red in the picture. However, the color becomes green with the gene expression decreasing. (B) The tree diagram at the top of the figure represents the clustering results of different samples from different experimental groups, and the tree diagram on the left demonstrates the clustering results of different genes from different samples. The “vioplots” clearly show the difference between m6A RNA methylation regulators in lung cancer and normal lung tissue. Blue represents normal lung tissue, and red represents lung cancer. (C) The diagram visually shows the relationship between different m6A RNA methylation regulators through Pearson correlation analysis. Red represents a positive correlation, and blue represents a negative correlation. *P < 0.05, **P < 0.01 and ***P < 0.01.
Most of the m6A RNA methylation regulators showed a weak to moderate positive correlation. KIAA1429 and YTHDF3 were the most correlated in LUAD. In LUSC, METTL3 and RBMX had the most obvious positive correlation. The negative correlation between HNRNPC gene and FTO gene was the most recognizable. In pan-cancer, KIAA1429 and YTHDF3 were the most relevant. HNRNPC and FTO were the most obvious negative correlation.
Consensus Clustering of m6A RNA Methylation Regulators Identified Two Clusters of Lung Cancer
After using “ConsensusClusterPlus” package to group LUAD, LUSC, and pan-cancer, the CDF value was small and the lines were smooth when k = 2. Next, PCA was used to verify whether the classification was correct. It was proved that the classification of cluster 1 and cluster 2 meets the requirements (Figures 2A–C).
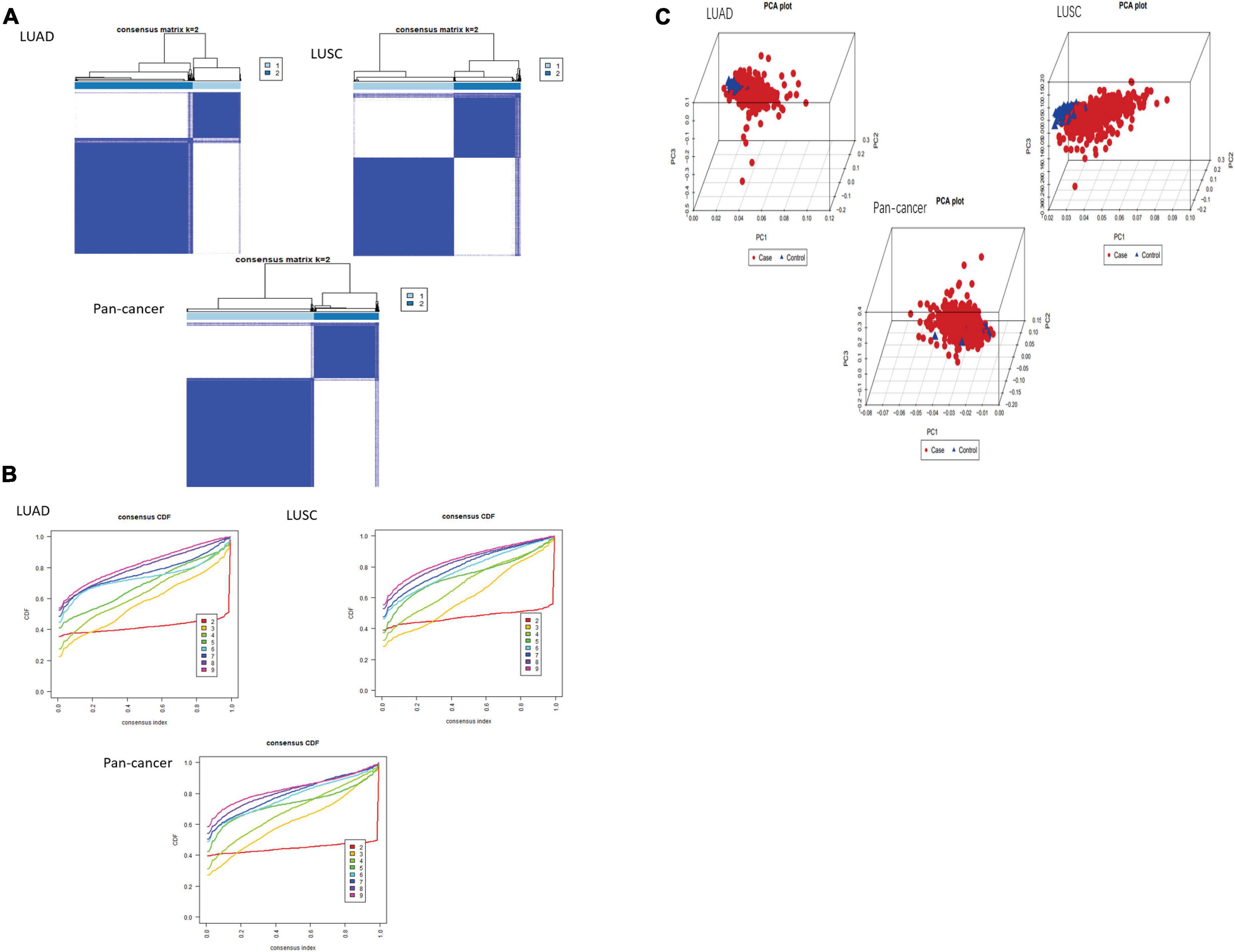
Figure 2. Classification of cluster by m6A RNA methylation regulator. (A,B) As K changed from 2 to 9, clustering cumulative distribution function of different lung cancer groups has been shown discriminatively. The consistent clustering matrix is the most suitable when K = 2. (C) PCR analysis results of the principal components are shown in the RNA expression profile among the TCGA database. Red represents lung cancer cases in cluster 1. Blue represents normal lung tissue of cluster 2.
The Categories Determined by Consensus Clustering Are Closely Related to Clinical Results
In the cleaned LUAD data, the survival probability of cluster 2 was greater than that of cluster 1. In assessing the relationship between clustering and clinical pathological features, the number of cluster 1 was significantly less than that of cluster 2. The result revealed that cluster 1 and cluster 2 had significant differences in M stage, T stage, total stage, and survival status. In LUSCs after washing, the difference in overall survival between the two clusters was not apparent. In the heatmap, the length of cluster 1 was slightly longer than that of cluster 2. There were differences in total stage, gender, and age between the two clusters. Of the cleaned pan-cancer, cluster 1 had a higher survival probability than cluster 2 when the patients had the same survival time. In the heatmap, the length of cluster 1 was also slightly longer than that of cluster 2, and there were differences in N stage, T stage, total stage, and gender (Figure 3).
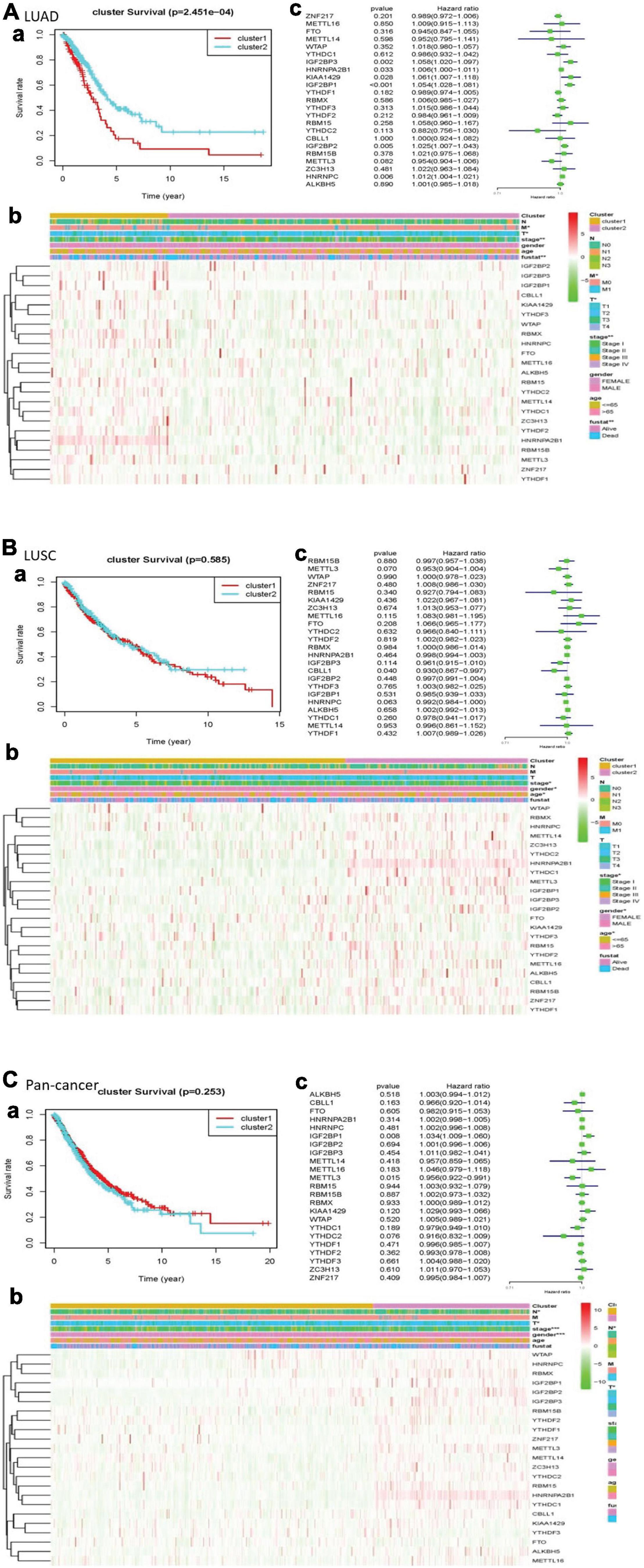
Figure 3. Clinical pathological characteristics and overall survival rate of cluster 1 and cluster 2 in different lung cancers, as well as the risk signature with m6A RNA methylation regulators. (A) LUAD; (B) LUSC; (C) pan-cancer. (a) Survival plot. The graph plots the overall survival curves of cluster 1 and cluster 2 in different lung cancers. Red represents cluster 1, and blue represents cluster 2. (b) Heatmap. The heat map shows the expression of m6A RNA methylation regulator in two clusters and its clinical pathological characteristics. When P < 0.05, the result is meaningful. (c) Univariate Cox regression. The graph records the hazard ratio and 95% confidence interval calculated by univariate Cox regression. The univariate analysis is beneficial to select the meaningful genes.
Prognostic Values of Risk Signature and m6A RNA Methylation Regulators
We used univariate Cox regression to get that, with these dangerous genes named IGF2BP3, HNRNPA2B1, KIAA1429, IGF2BP1, IGF2BP2, and HNRNPC, patients hardly survived. In LUSC, CBLL1 is a risk gene. In pan-cancer, IGF2BP1 was a risk gene, while METTL3 was a protective gene (Figure 3).
The least absolute shrinkage and selection operator (LASSO) Cox regression algorithm was implemented to analyze 23 genes. According to the minimum standard, 5 genes were selected for LUAD, 3 for LUSC, and 3 for pan-cancer. Five genes in LUAD are IGF2BP1, IGF2BP2, HNRNPC, KIAA1429, and HNRNPA2B1. Three genes are CBLL1, HNRNPC, and METTL3 in LUSC. In pan-cancer, three genes are IGF2BP1, METTL3, and YTHDC2. Then, risk characteristics were established, respectively. The coefficients were used to calculate the risk scores of each group. Among LUAD, LUSC, and pan-cancer, the survival rate of patients in the high-risk group is both lower than that in the low-risk group (Figures 4A–C).
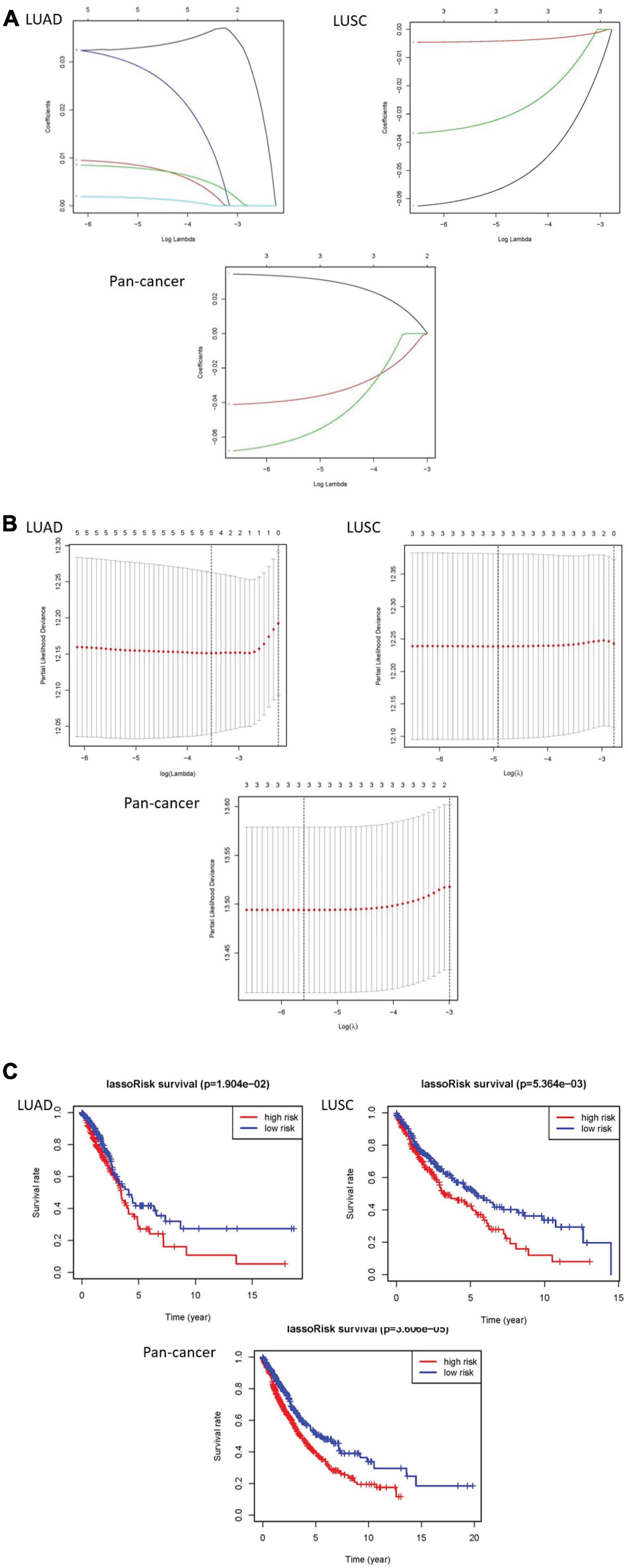
Figure 4. The results of LASSO Cox regression algorithm and survival curves. (A,B) Show coefficient results derived from LASSO Cox regression algorithm. (C) There are distinct differences of overall survival curves in the high-risk group and low-risk group which are distributed by risk score.
Prognostic Risk Scores Are Related to the Characteristics of Lung Cancer Clinical Cases
We made a ROC curve to get the results that the risk score can be served as an independent prognostic factor. Next, a heatmap showed that there is a significant difference in survival status in LUAD. Similarly, the pan-cancer data have obvious differences in N stage, T stage, total stage, and survival status. However, there are no significant differences in any pathological features of LUSC (Figures 5A–C).
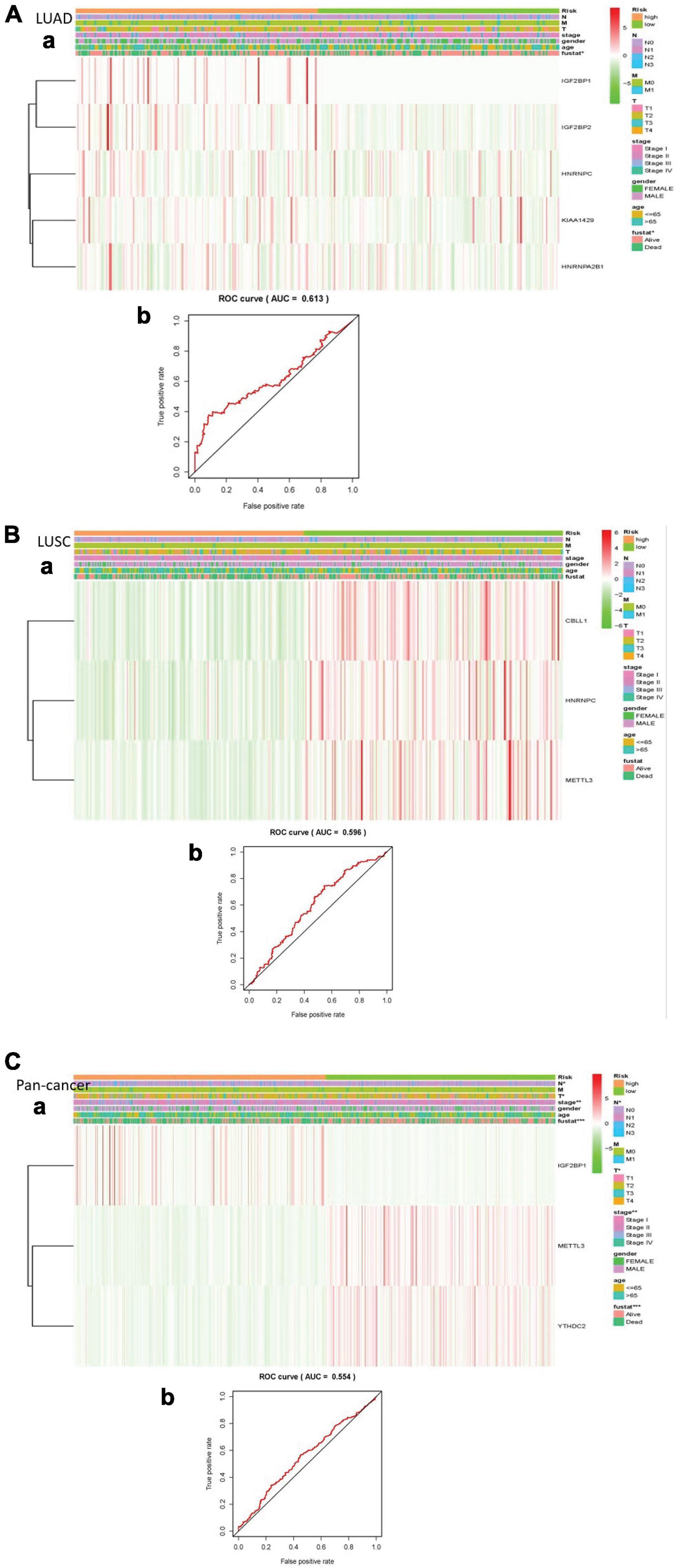
Figure 5. Explain the relationship between risk score, m6A RNA methylation regulator expression level, clinical pathological characteristics, risk group, and overall survival rate. (A) LUAD; (B) LUSC; (C) pan-cancer. (a) Every ROC curve indicates the relationship between risk score and survival rate, reflecting the predictive efficiency of prognostic signatures. (b) Each heatmap shows the expression level of m6A RNA methylation regulator in lung cancer of two clusters. In addition, there are apparent differences in the distribution of clinical pathological characteristics between the two groups. When P < 0.001, the result is significant.
Finally, we performed univariate and multivariate Cox regression analysis. In univariate analysis, total stage, T stage, N stage, and risk scores are all related to overall survival in LUAD. Age, total stage, and risk scores are connected with overall survival in LUSC. Total staging, T staging, M staging, N staging, and risk scores have something to do with overall survival in pan-cancer. When all factors were incorporated into the multivariate analysis, the total stage and risk scores were still significantly correlated with the overall survival rate in LUAD. Age and risk scores are related to overall survival in LUSC. T staging and risk scores were in connection with overall survival in pan-cancer (Figure 6).
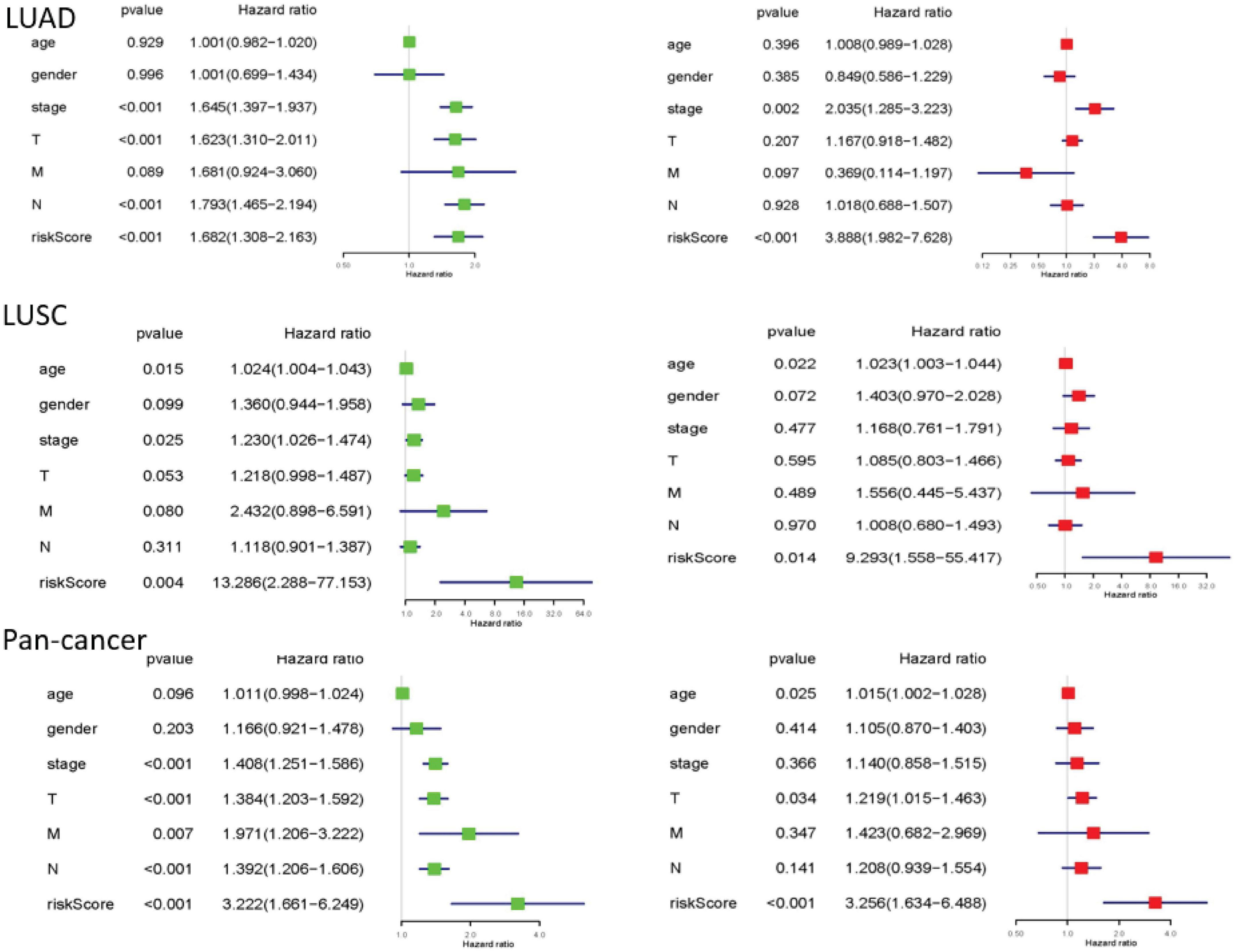
Figure 6. The results of univariate and multivariate Cox regression analysis. Univariate and multivariate Cox regression analysis between overall survival rate and clinical pathological characteristics was performed for each type of lung cancer. They could identify the independent prognostic factors. When P < 0.05, the result is meaningful.
Discussion
With the increasing incidence and mortality of lung cancer today, there are quite in-depth discoveries in DNA and protein modification, so the treatment of lung cancer is also updated at an alarming rate (Karlsson et al., 2019; Siegel et al., 2020). This study explored the clinical prognostic effect of m6A in LUAD, LUSC, and pan-cancer for the purpose of laying the foundation for treatment strategies based on RNA methylation.
In this study, we found that most of the 23 m6A RNA methylation regulators were abnormally expressed in LUAD, LUSC, and pan-cancer. Among them, KIAA1429 and YTHDF3 showed a significant positive correlation. YTHDF3 is a direct m6A reader, which can promote the translation of target mRNAs and improve their stability. The proliferation, migration, and invasion of cancer cells were enhanced with the upregulated expression of METTL3 (Cheng et al., 2019). When the expression level of METTL3 is upregulated, it enhances the proliferation, migration, and invasion of cancer cells in vitro and the growth of tumors in vivo. Meanwhile, YTHDF3 collaborates with YTHDF1 to promote protein synthesis and influence methylated mRNA decay mediated by YTHDF2. Together with YTHDF1 and YTHDF2, YTHDF3 plays a key role in accelerating metabolism of mRNAs modified by m6A in the cytoplasm (Wang X. et al., 2014; Li et al., 2017; Shi et al., 2017).
In the LUAD, LUSC, and pan-cancer, there are significant differences in the overall survival of the two different clusters. They also have significant correlation in different clinical pathological features, indicating that the level of m6A RNA methylation regulator is distinctly related to the poor prognosis of lung cancer.
In the LUAD, KIAA1429 is associated with the formation of m6A methyltransferase complex (MTC), which promotes the formation of m6A (Bokar et al., 1997; Ping et al., 2014; Wang Y. et al., 2014). HNRNPC is considered as the promoter of tumorigenesis, and overexpression of HNRNPC can be found in the main regulators of various cancer progression-related genes, which is closely connected to the poor clinical prognosis of cancer (Huang et al., 2016; Fischl et al., 2019). The risk gene for LUSC is CBLL1. In addition, pan-cancer’s risk gene is METTL3 and the protective gene is IGF2BP1. METTL3 can participate in the composition of MTC as well. Cytoplasmic METTL3 is served as a m6A reader to promote the translation of target mRNA (Liu et al., 2014; Cheng et al., 2019; Ru et al., 2020; Shi et al., 2020). This result is used as a basis for predicting the survival rate of lung cancer according to the genetic differences of patients in the future. Even the known gene variation could be used to fundamentally treat lung cancer from the genetic perspective, for example, some medicine will target m6A methylation (Deng et al., 2018).
We selected the appropriate gene according to the minimum standard and calculated their respective risk scores in the wake of dividing lung cancer into high-risk and low-risk categories by the LASSO algorithm. Then, the ROC curve and heatmap are used to verify that the risk scores can effectively predict the survival of lung cancer patients. Above all, risk scores provide an important basis for clinical evaluation of lung cancer prognosis and provide personalized treatment options.
In summary, this study systematically described the differences in the expression levels of m6A RNA methylation regulators between LUAD, LUSC, and pan-cancer data, revealing the prognostic role of their expressions in relation to the clinical pathological characteristics of lung cancer. M6A RNA methylation regulator obviously not only plays a vital role in the occurrence and development of lung cancer but also affects the clinical manifestations and prognostic development of lung cancer (Wang et al., 2021). Besides, risk scores can become a far-reaching factor in predicting the prognosis of lung cancer, providing essentially for clinical treatment guidance.
Materials and Methods
Data Acquisition
A total of 1037 cases of lung cancer were obtained from the TCGA database,1 including 535 cases of LUAD and 502 cases of LUSC. In addition, there were 108 cases in the control group, containing 59 cases of LUAD and 49 cases of LUSC.
Selection of m6A RNA Methylation Regulators
Depending upon the literature (Shi et al., 2018; Li et al., 2019, 2020; Sun et al., 2019; Liu et al., 2020; Meng et al., 2020; Muthusamy, 2020; Zhou et al., 2020; Zhang et al., 2021; Zou et al., 2021), 23 tumor-related m6A RNA methylated modulators were selected. These 23 genes were extracted and systematically analyzed with the data of LUAD, LUSC, and pan-cancer in TCGA database. Through these procedures, we could search the relationship between the expression of 23 m6A-related genes and the clinical prognosis of lung cancer patients.
Bioinformatic Analysis
In order to examine the role of m6A RNA methylation regulator in lung cancer, we first used “limma” package to analyze 23 m6A RNA methylation regulator related genes in each lung cancer group. In the next step, expression levels of 23 m6A RNA methylation regulators had been distinctly demonstrated by “diff” analysis, “pheatmap” package, and “vioplot” package. Correlation figures were drawn to illustrate the relationship between different m6A RNA methylation regulators. Besides, we utilized the “ConsensusClusterPlus” package to delete the normal lung tissue data in each lung cancer group and divide the remaining lung cancer data into two groups. PCA could verify whether the classification was correct. The survival software package had the capability to study the survival of grouped lung cancers and draw a clinical heatmap of the correlation between different clusters and the relationship between survival and clinical data.
More than anything, for the purpose of finding the relationship between the gene and survival and its effect on the prognosis, the m6A RNA methylation Regulator genes were conducted by univariate Cox regression analysis and LASSO Cox regression algorithm (Bovelstad et al., 2007; Sauerbrei et al., 2007).
In the end, we could construct the survival-related linear risk assessment model—risk scores. Calculation formula: Risk score = . Exp in the formula represents the expression level of the gene, and w represents the coefficient of the gene in the univariate or multivariate Cox regression analysis. ROC curve and heatmap were assessed if risk score could be a prognostic factor in lung cancer (Figure 7).
Statistical Analyses
“Diff” package was used to analyze the expression levels of 23 m6ARNA methylation modulator related genes in LUAD, LUSC, and pan-cancer. Then, the “ConsensusClusterPlus” software package was used to treat LUSC, LUSC, and total lung cancer. Also, each group of lung cancer was divided into two clusters. With the help of univariate Cox regression analysis, the relationship between different clusters and clinical data was clarified. At the same time, the prognostic effect of related genes was analyzed in combination with multivariate regression analysis. Finally, LASSO Cox regression algorithm was performed to analyze related genes and divide each group of lung cancer into high-risk group and low-risk group to find independent prognostic factors. Overall survival referred to the time from the date of diagnosis to death for any reason. This study used Practical Extraction and Report Language (Perl) and R version 3.5.3 for statistical analysis. The results were statistically significant when P < 0.05.
Data Availability Statement
The original contributions presented in the study are included in the article/supplementary material, further inquiries can be directed to the corresponding author/s.
Ethics Statement
The studies involving human participants were reviewed and approved by Guangdong Medical University Ethics committee. The ethics committee waived the requirement of written informed consent for participation.
Author Contributions
XZ and GP: conceptualization, methodology, supervision, funding, and software. ST: data curation and writing—original draft preparation. ST, KL, GL, ZT, ZZ, and XZ: visualization and investigation. ST, ZL, YL, JK, WC, ML, and XZ: writing—reviewing and editing. XZ: checked the statistical accuracy as an expert in statistics and bioinformatics. All authors read and approved the final version of the manuscript.
Funding
This work was supported partly by the National Natural Science Foundation of China (Nos. 81541153 and 81972366), the 7th Wuhan Young and Middle-aged Backbone Talent of Medical Training Project 2019 (2019 No. 87), and the Guangdong Key Laboratory funds of Systems Biology and Synthetic Biology for Urogenital Tumors (No. 2017B030301015). The funders had no role in the design of the study; the collection, analysis, and interpretation of the data; the writing of the manuscript; and the decision to submit the manuscript for publication.
Conflict of Interest
The authors declare that the research was conducted in the absence of any commercial or financial relationships that could be construed as a potential conflict of interest.
Publisher’s Note
All claims expressed in this article are solely those of the authors and do not necessarily represent those of their affiliated organizations, or those of the publisher, the editors and the reviewers. Any product that may be evaluated in this article, or claim that may be made by its manufacturer, is not guaranteed or endorsed by the publisher.
Footnotes
References
Asada, K., Bolatkan, A., Takasawa, K., Komatsu, M., Kaneko, S., and Hamamoto, R. (2020). Critical Roles of N(6)-methyladenosine (m(6)A) in cancer and virus infection. Biomolecules 10:1071. doi: 10.3390/biom10071071
Bokar, J. A., Shambaugh, M. E., Polayes, D., Matera, A. G., and Rottman, F. M. (1997). Purification and cDNA cloning of the AdoMet-binding subunit of the human mRNA (N6-adenosine)-methyltransferase. RNA 3, 1233–1247.
Bovelstad, H. M., Nygard, S., Storvold, H. L., Aldrin, M., Borgan, O., Frigessi, A., et al. (2007). Predicting survival from microarray data–a comparative study. Bioinformatics 23, 2080–2087. doi: 10.1093/bioinformatics/btm305
Cheng, M., Sheng, L., Gao, Q., Xiong, Q., Zhang, H., Wu, M., et al. (2019). The m(6)A methyltransferase METTL3 promotes bladder cancer progression via AFF4/NF-kappaB/MYC signaling network. Oncogene 38, 3667–3680. doi: 10.1038/s41388-019-0683-z
Choe, J., Lin, S., Zhang, W., Liu, Q., Wang, L., Ramirez-Moya, J., et al. (2018). mRNA circularization by METTL3-eIF3h enhances translation and promotes oncogenesis. Nature 561, 556–560. doi: 10.1038/s41586-018-0538-8
Deng, X., Su, R., Weng, H., Huang, H., Li, Z., and Chen, J. (2018). RNA N(6)-methyladenosine modification in cancers, current status and perspectives. Cell Res. 28, 507–517. doi: 10.1038/s41422-018-0034-6
Fischl, H., Neve, J., Wang, Z., Patel, R., Louey, A., Tian, B., et al. (2019). hnRNPC regulates cancer-specific alternative cleavage and polyadenylation profiles. Nucleic Acids Res. 47, 7580–7591. doi: 10.1093/nar/gkz461
He, J. J., Li, Z., Rong, Z. X., Gao, J., Mu, Y., Guan, Y. D., et al. (2020). m(6)A reader YTHDC2 promotes radiotherapy resistance of nasopharyngeal carcinoma via activating IGF1R/AKT/S6 signaling axis. Front. Oncol. 10:1166.
Huang, H., Han, Y., Zhang, C., Wu, J., Feng, J., Qu, L., et al. (2016). HNRNPC as a candidate biomarker for chemoresistance in gastric cancer. Tumour. Biol. 37, 3527–3534. doi: 10.1007/s13277-015-4144-1
Karlsson, A., Cirenajwis, H., Ericson-Lindquist, K., Brunnstrom, H., Reutersward, C., Jonsson, M., et al. (2019). A combined gene expression tool for parallel histological prediction and gene fusion detection in non-small cell lung cancer. Sci. Rep. 9:5207.
Li, A., Chen, Y. S., Ping, X. L., Yang, X., Xiao, W., Yang, Y., et al. (2017). Cytoplasmic m(6)A reader YTHDF3 promotes mRNA translation. Cell Res. 27, 444–447. doi: 10.1038/cr.2017.10
Li, K., Luo, H., Luo, H., and Zhu, X. (2020). Clinical and prognostic pan-cancer analysis of m6A RNA methylation regulators in four types of endocrine system tumors. Aging (Albany NY) 12, 23931–23944. doi: 10.18632/aging.104064
Li, X., Feng, Z., Wang, R., Hu, J., He, X., and Shen, Z. (2021). Expression status and prognostic value of m(6)A RNA methylation regulators in lung adenocarcinoma. Life (Basel) 11:619. doi: 10.3390/life11070619
Li, Y., Xiao, J., Bai, J., Tian, Y., Qu, Y., Chen, X., et al. (2019). Molecular characterization and clinical relevance of m(6)A regulators across 33 cancer types. Mol. Cancer 18:137.
Liu, J., Ren, D., Du, Z., Wang, H., Zhang, H., and Jin, Y. (2018). m(6)A demethylase FTO facilitates tumor progression in lung squamous cell carcinoma by regulating MZF1 expression. Biochem. Biophys. Res. Commun. 502, 456–464. doi: 10.1016/j.bbrc.2018.05.175
Liu, J., Yue, Y., Han, D., Wang, X., Fu, Y., Zhang, L., et al. (2014). A METTL3-METTL14 complex mediates mammalian nuclear RNA N6-adenosine methylation. Nat. Chem. Biol. 10, 93–95. doi: 10.1038/nchembio.1432
Liu, S., Li, Q., Chen, K., Zhang, Q., Li, G., Zhuo, L., et al. (2020). The emerging molecular mechanism of m(6)A modulators in tumorigenesis and cancer progression. Biomed. Pharmacother. 127:110098. doi: 10.1016/j.biopha.2020.110098
Meng, Y., Li, S., Gu, D., Xu, K., Du, M., Zhu, L., et al. (2020). Genetic variants in m6A modification genes are associated with colorectal cancer risk. Carcinogenesis 41, 8–17.
Muthusamy, S. (2020). m(6)A mRNA methylation, A pleiotropic regulator of cancer. Gene 736:144415. doi: 10.1016/j.gene.2020.144415
Ni, X. F., Xie, Q. Q., Zhao, J. M., Xu, Y. J., Ji, M., Hu, W. W., et al. (2021). The hepatic microenvironment promotes lung adenocarcinoma cell proliferation, metastasis, and epithelial-mesenchymal transition via METTL3-mediated N6-methyladenosine modification of YAP1. Aging (Albany NY) 13, 4357–4369. doi: 10.18632/aging.202397
Ping, X. L., Sun, B. F., Wang, L., Xiao, W., Yang, X., Wang, W. J., et al. (2014). Mammalian WTAP is a regulatory subunit of the RNA N6-methyladenosine methyltransferase. Cell Res. 24, 177–189. doi: 10.1038/cr.2014.3
Ru, W., Zhang, X., Yue, B., Qi, A., Shen, X., Huang, Y., et al. (2020). Insight into m(6)A methylation from occurrence to functions. Open Biol. 10:200091. doi: 10.1098/rsob.200091
Sauerbrei, W., Royston, P., and Binder, H. (2007). Selection of important variables and determination of functional form for continuous predictors in multivariable model building. Stat. Med. 26, 5512–5528. doi: 10.1002/sim.3148
Shi, H., Wang, X., Lu, Z., Zhao, B. S., Ma, H., Hsu, P. J., et al. (2017). YTHDF3 facilitates translation and decay of N(6)-methyladenosine-modified RNA. Cell Res. 27, 315–328. doi: 10.1038/cr.2017.15
Shi, H., Zhang, X., Weng, Y. L., Lu, Z., Liu, Y., Lu, Z., et al. (2018). m(6)A facilitates hippocampus-dependent learning and memory through YTHDF1. Nature 563, 249–253. doi: 10.1038/s41586-018-0666-1
Shi, Y., Zheng, C., Jin, Y., Bao, B., Wang, D., Hou, K., et al. (2020). Reduced expression of METTL3 promotes metastasis of triple-negative breast cancer by m6A Methylation-Mediated COL3A1 Up-regulation. Front. Oncol. 10:1126.
Siegel, R. L., Miller, K. D., and Jemal, A. (2020). Cancer statistics, 2020. CA Cancer J. Clin. 70, 7–30.
Sun, T., Wu, R., and Ming, L. (2019). The role of m6A RNA methylation in cancer. Biomed. Pharmacother. 112:108613. doi: 10.1016/j.biopha.2019.108613
Tuncel, G., and Kalkan, R. (2019). Importance of m N(6)-methyladenosine (m(6)A) RNA modification in cancer. Med. Oncol. 36:36.
Wang, H., Zhao, X., and Lu, Z. (2021). m(6)A RNA methylation regulators act as potential prognostic biomarkers in lung adenocarcinoma. Front. Genet. 12:622233.
Wang, S., Chai, P., Jia, R., and Jia, R. (2018). Novel insights on m(6)A RNA methylation in tumorigenesis, a double-edged sword. Mol. Cancer 17:101.
Wang, X., Lu, Z., Gomez, A., Hon, G. C., Yue, Y., Han, D., et al. (2014). N6-methyladenosine-dependent regulation of messenger RNA stability. Nature 505, 117–120. doi: 10.1038/nature12730
Wang, Y., Li, Y., Toth, J. I., Petroski, M. D., Zhang, Z., and Zhao, J. C. (2014). N6-methyladenosine modification destabilizes developmental regulators in embryonic stem cells. Nat. Cell Biol. 16, 191–198. doi: 10.1038/ncb2902
Wozniak, A. (2012). Challenges in the current antiangiogenic treatment paradigm for patients with non-small cell lung cancer. Crit. Rev. Oncol. Hematol. 82, 200–212. doi: 10.1016/j.critrevonc.2011.05.003
Wozniak, A. J., and Schwartz, A. G. (2018). Risk of second lung cancer in patients with previously treated lung cancer, analysis of surveillance, epidemiology, and end results data. J. Thorac. Oncol. 13, e106–e107.
Yang, Y., Hsu, P. J., Chen, Y. S., and Yang, Y. G. (2018). Dynamic transcriptomic m(6)A decoration: writers, erasers, readers and functions in RNA metabolism. Cell Res. 28, 616–624. doi: 10.1038/s41422-018-0040-8
Zhang, C., Fu, J., and Zhou, Y. (2019). A review in research progress concerning m6A methylation and immunoregulation. Front. Immunol. 10:922.
Zhang, X., Zhong, L., Zou, Z., Liang, G., Tang, Z., Li, K., et al. (2021). Clinical and prognostic pan-cancer analysis of N6-methyladenosine regulators in two types of hematological Malignancies, A Retrospective Study Based on TCGA and GTEx Databases. Front. Oncol. 11:623170.
Zhou, Y., Kong, Y., Fan, W., Tao, T., Xiao, Q., Li, N., et al. (2020). Principles of RNA methylation and their implications for biology and medicine. Biomed. Pharmacother. 131:110731. doi: 10.1016/j.biopha.2020.110731
Zhu, X., Luo, H., and Xu, Y. (2019). Transcriptome analysis reveals an important candidate gene involved in both nodal metastasis and prognosis in lung adenocarcinoma. Cell Biosci. 9:92.
Keywords: lung cancer, N6-methyladenosine, prognosis, regulators, risk scores
Citation: Tan S, Li Z, Li K, Li Y, Liang G, Tang Z, Kang J, Chen W, Li M, Zou Z, Pi G and Zhu X (2021) The Regulators Associated With N6-Methyladenosine in Lung Adenocarcinoma and Lung Squamous Cell Carcinoma Reveal New Clinical and Prognostic Markers. Front. Cell Dev. Biol. 9:741521. doi: 10.3389/fcell.2021.741521
Received: 14 July 2021; Accepted: 07 September 2021;
Published: 09 December 2021.
Edited by:
Jian-ye Zhang, Guangzhou Medical University, ChinaReviewed by:
Xiang Zhiming, South China Sea Institute of Oceanology, Chinese Academy of Sciences (CAS), ChinaLiming Mao, Nantong University, China
Copyright © 2021 Tan, Li, Li, Li, Liang, Tang, Kang, Chen, Li, Zou, Pi and Zhu. This is an open-access article distributed under the terms of the Creative Commons Attribution License (CC BY). The use, distribution or reproduction in other forums is permitted, provided the original author(s) and the copyright owner(s) are credited and that the original publication in this journal is cited, in accordance with accepted academic practice. No use, distribution or reproduction is permitted which does not comply with these terms.
*Correspondence: Guoliang Pi, piguoliang_2004@163.com; Xiao Zhu, bioxzhu@yahoo.com
†These authors have contributed equally to this work