- 1Department of Immunology, CAMS Key Laboratory for T Cell and Cancer Immunotherapy, Institute of Basic Medical Sciences, Chinese Academy of Medical Sciences and School of Basic Medicine, Peking Union Medical College, State Key Laboratory of Medical Molecular Biology, Beijing, China
- 2Cheeloo College of Medicine, Shandong University, Jinan, China
- 3School of Physical Science, University of California, Irvine, Irvine, CA, United States
- 4Weihai Municipal Hospital, Cheeloo College of Medicine, Shandong University, Weihai, China
- 5Department of Translational Molecular Pathology, The University of Texas MD Anderson Cancer Center, Houston, TX, United States
Background: Long noncoding RNAs (lncRNAs) crucially modulate DNA damage responses/repair in cancer cells. However, the underlying regulatory role of genome integrity and its clinical value in colon adenocarcinoma (COAD) remains unclear. This study links genome instability to lncRNA using computational biology techniques, in attempt to propose novel biomarkers of immunotherapy outcome, and investigated a potential competing endogenous RNA (ceRNA) as a molecular regulatory mechanism.
Methods: TCGA-COAD patients were divided into genome unstable (GU)-like and genome stable (GS)-like clusters via hierarchical clustering to predict immunotherapy outcomes. Multivariate Cox model was established to predict the overall survival rate in COAD patients. Additionally, SVM and LASSO algorithms were applied to obtain hub lncRNAs. A novel genome instability-related ceRNA network was predicted with the Starbase 2.0 database. To better understand how these genes fundamentally interact during tumor progression and development, the mutation analysis and single-gene analysis for each gene was performed.
Results: In contrast to those in the GS-like cluster, GU-like-cluster patients demonstrated a higher tumor mutational burden (TMB)/microsatellite instability (MSI), DNA polymerase epsilon (POLE) mutation rate, and immune checkpoint expression, all indicate a greater predictive power for response rate for immunotherapy. The novel prognostic signature demonstrated an outstanding predictive performance (AUC > 0.70). The genes in the genome insatiability-related ceRNA network (including four axes: AL161772.1-has-miR-671-5p (hsa-miR-181d-5p, has-miR-106a-5p)-NINL, AL161772.1-has-miR-106a-5p-TNFSF11, AC124067.4-hsa-miR-92b-3p (hsa-miR-589-5p)-PHYHIPL, and BOLA3-AS1-has-miR-130b-3p-SALL4) were identified as critical regulators of tumor microenvironment infiltration, cancer stemness, and drug resistance. qPCR was performed to validate the expression patterns of these genes. Furthermore, the MSI-high proportion was greater in patients with mutated type than in those with the wild type according to all four target genes, indicating that these four genes modulate genomic integrity and could serve as novel immunotherapy biomarkers.
Conclusion: We demonstrated that genome instability-related lncRNA is a novel biomarker for immunotherapy outcomes and prognosis. A novel ceRNA network that modulates genomic integrity, including four lncRNA-miRNA-mRNA axes, was proposed.
Introduction
Colon adenocarcinoma (COAD) is the primary subtype of colorectal cancer, ranking fourth in lethal malignancies worldwide and second across the United States (Brody, 2015). Although the establishment of a series of molecularly targeted therapies has led to a noticeable increase in the survival of COAD patients, chemotherapy is still the standard and irreplaceable treatment, and the 5-year survival rate for Stage IV COAD patients is less than 10% (Ganesh et al., 2019). Thus, there is an urgency to further develop novel treatment regimens (Garrido-Castro et al., 2019).
In recent years, immunotherapy has become an ideal option for advanced COAD with the emergence and rapid development of immune checkpoint inhibitors (ICIs) (Qin et al., 2019; Hu et al., 2020). Considering that patient response rates to nivolumab and pembrolizumab (both PD-1/PD-L1 inhibitors) are diverse and often less than 50%, additional predictive biomarkers need to be identified (Schrock et al., 2019). Genome instability was reported as one of the top 10 promising discoveries for cancer treatment in the twenty-first century and has elicited a corresponding interest (Hanahan and Weinberg, 2011). Recent clinical practice has demonstrated that genome instability is associated with ICI outcomes. Microsatellite instability (MSI) is a critical biomarker for ICIs (Boland and Goel, 2010). In general, MLH1 promoter hypermethylation or germline mutations in four DNA mismatch repair (dMMR) machinery genes (MSH6, MSH2, PMS2, MLH1) lead to MSI (Dudley et al., 2016). PD-1/PD-L1 inhibitors in MSI-high (MSI-H) metastatic colorectal carcinoma have been confirmed to have a favorable cancer-control effect with high progression-free survival (Schrock et al., 2019).
Long noncoding RNAs (lncRNAs) are nonprotein coding RNAs that are longer than 200 nucleotides. They are involved in a series of diverse biological processes, including cell development, differentiation (Fatica and Bozzoni, 2014), the cell cycle response (Hung et al., 2011), and gene imprinting (Kanduri, 2016). Current studies on these molecules have mainly focused on their dysregulation in cancers, which leads to alterations in tumor behavior (Bhan et al., 2017). Therefore, lncRNAs are promising candidates for clinical cancer biomarker exploration (Li Y. et al., 2020; Goyal et al., 2021). A novel role for lncRNAs is modulating DNA damage response pathways, such as the TP53 and ATM/ATR pathways (Su et al., 2018). This study linked genome instability to lncRNA, which was termed genome instability-related lncRNA (GIRlncR), and hypothesized that GIRlncR could serve as a novel immunotherapy biomarker for COAD.
Advances in RNA sequencing (RNA-seq) techniques have largely promoted the functional annotation and progress on the computational characteristics of lncRNAs (Chen et al., 2016). Yin et al. (2021) identified a genome instability-related lncRNA prognostic signature in COAD using computational biological techniques. However, they did not focus enough on the predictive ability of GIRlncR for immunotherapy outcomes. They also did not explore the regulatory mechanisms of lncRNAs (Yin et al., 2021). This study established a patient stratification clustering method for COAD to predict ICI outcomes in these patients. A novel genome instability-related competing endogenous RNA (ceRNA) network (including four axes: AL161772.1-has-miR-671-5p (hsa-miR-181d-5p, has-miR-106a-5p)-NINL, AL161772.1-has-miR-106a-5p-TNFSF11, AC124067.4-hsa-miR-92b-3p (hsa-miR-589-5p)-PHYHIPL, and BOLA3-AS1-has-miR-130b-3p-SALL4) was constructed using machine learning algorithms. Furthermore, the MSI-H proportion was greater in patients with mutated type than in those with the wild type according to all four target genes, indicating that these genes modulate genomic integrity and could serve as novel immunotherapy biomarkers.
Materials and Methods
Data Selection and Code Availability
The graphical abstract presents the flow chart and online resources for this study. The original data of RNA-seq (FPKM format), miRNA-seq, corresponding clinical characteristics, including gender, age, overall survival (OS), and stage, and simple nucleotide variation (SNV) were downloaded from the TCGA-COAD project (December 24, 2020). The tumor mutational burden (TMB) and MSI data were retrieved from cBioportal (Cerami et al., 2012). The clustering method was used to detect and exclude the outlier RNA-seq samples. Eventually, 453 RNA-seq samples were acquired; however, only 446 patients with both matching RNA-seq data and corresponding clinical features were included in this study. All biological and clinical samples in this study are publicly available. The data availability policies of the open-accessed databases were strictly followed. All code utilized in this study can be acquired via email MjAxODAwNDEzMDQwQG1haWwuc2R1LmVkdS5jbg== with reasonable grounds.
Differential Expression Gene Extraction
The R package “limma” was used to extract differential expression genes (DEGs). All tumor RNA-seq samples were sorted according to their SNV numbers, from largest to smallest. The first 25% and last 25% of samples were named the genome-unstable (GU) group and genome-stable (GS) group. Differentially expressed lncRNAs between the GS and GU groups were considered GIRlncRs. The cutoff value was adjusted a p-Value (adj. p) < 0.05, and | log2 fold-change| > 1. Similarly, genome instability-related mRNA (GIRmR) and miRNA (GIRmiR) were extracted.
Hierarchical Clustering to Establish a Novel Immunotherapy Outcome Predictive Stratification Method
The “sparcl” package was implemented to perform unsupervised hierarchical clustering based on GIRlncRs (k = 2). The clusters with higher and lower SNV numbers were named GU-like and GS-like clusters, respectively. We compared the tumor mutational burden (TMB), MSI, expression, and mutation rates of four MMR genes, expression of six immune-related genes, and the mutation rates of POLE (a novel biomarker for immunotherapy outcomes (Wang et al., 2019)) between the two clusters. The MMR and immune-related genes included in this study are listed in Table 1. Single-sample gene set enrichment analysis (ssGSEA) was used to compare tumor microenvironment (TME) infiltration and functions between the two clusters (Supplementary Table 1). Survival analysis using the log-rank test between the GS-like and GU-like clusters was performed to evaluate the prognostic value of clustering. By calculating the Pearson’s correlation coefficients, we ranked the relevance of associations between each mRNA and GIRlncR expression. The first 10 ranked mRNAs were considered coexpressed with GIRlncR. Cytoscape, a biological network modifying software, was used to visualize the lncRNA-mRNA coexpression network (Shannon et al., 2003). To explore the potential functions of GIRlncRs, functional gene enrichment analysis based on the Gene Ontology (GO) (Ashburner et al., 2000) and Kyoto Encyclopedia of Genes and Genomes (KEGG) (Kanehisa and Goto, 2000) databases was performed.
Multivariate Cox Regression to Construct a Novel Gene Signature
Multivariate Cox regression is the most widely utilized regression model for analyzing medical survival time and survival status data (Bradburn et al., 2003), and it was used to explore the association with event incidence (surviving/deceased in this study). The hazard ratio (HR) represents the probability of event occurrence under the currently observed feature patterns (gene expression pattern in this study). First, we divided 446 patients equally into the training and test cohorts. Chi-square tests were used to detect selection bias for each feature during patient division. Second, univariate Cox regression analysis of each GIRlncR was used for feature selection. Only statistically significant features (p < 0.05) were used for model construction. Third, multivariate Cox regression was performed to construct the genome instability-related lncRNA prognostic signature (GIlncPS) using the training cohort. The constructed model is as follows:
The model was applied to the test cohort. In both training and test cohorts, patients who had a risk score higher than the median value of the risk score were classified as the high-risk group and vice versa. Survival analysis and receiver operating characteristic (ROC) curves and the area under the curve (AUC) were utilized to evaluate the reliability of the GIlncPS. Moreover, given that BRAF is a frequently mutated gene with prognostic value (Sanz-Garcia et al., 2017), we tested whether our signature has better predictive performance than this event. Survival analysis among BRAF mutation-type/high-risk, BRAF mutation-type/low-risk, BRAF wild-type/high-risk, AND BRAF wild-type/low-risk groups was performed using the log-rank test. To demonstrate that our signature has a better predictive performance than other lncRNA signatures, we found two proposed lncRNA signatures for COAD, namely, Li’s signature (Li Z. et al., 2020) and Jin’s signature (Jin et al., 2020). AUC was used to rank the predictive performance of these signatures according to OS at 1, 3, and 5 years for 446 patients. To demonstrate that our signature has prognostic ability independent of general factors, we performed multivariate Cox regression, including clinical features (age, gender stage, T (tumor), M (metastasis), N (lymph node), KRAS mutation type, TP53 mutation type, BRAF mutation type, and POLE mutation type) and the risk score. The nomogram, including our signature, was plotted for clinical reference, which was evaluated using a calibration curve.
Analysis of Mutation Profile and Identification of Hub-Long Noncoding RNAs in Genome Stable- and Genome Unstable-Like Clusters
To investigate the relationship between the clusters and risk groups, Sankey plots were constructed. Waterfall plots were generated to explore the diversity of mutation profiles between GS- and GU-like clusters. To extract lncRNAs that had the closest relationship with clustering, LASSO and SVM algorithms were applied. The lncRNAs included in LASSO-screened lncRNAs, SVM-screened lncRNAs, and prognostic signatures were considered hub lncRNAs.
Competing Endogenous RNA Network
The hub-lncRNA and target mRNA interactions were predicted using an online comprehensive RNA database, ENCORI1 (Li et al., 2014). The overlapping mRNAs in GIRmRs and hub-lncRNA target mRNAs require further research. Only the statistically significant lncRNA-mRNA pairs (p < 0.05) in COAD remained for further research. We then retrieved the miRNAs mediating the relationships between the lncRNAs and mRNAs. The retrieved miRNAs that were differentially expressed between the GS and GU groups were retained for ceRNA network construction. Figure 4G illustrated the screening process of ceRNA network.
Comprehensive Single-Gene Analysis
It is hypothesized that the genes included in the ceRNA network play a pivotal role in COAD. A comprehensive single-gene analysis was performed. Differential expression analysis was performed between normal and tumor tissues from COAD patients by employing the Mann-Whitney U test. Thorsson et al. (2018) identified six immune subtypes across 33 TCGA cancer types (C1–C6) and compared the expression of each gene among these six subtypes using the Kruskal-Wallis test. Spearman correlation analysis was performed to detect the correlation between each gene and TME infiltration, the expression of MMR genes, immune-related genes (Table 1), and two previously discovered crucial lncRNAs regulating genome instability (NOARD (Munschauer et al., 2018), GUARDIN (Hu et al., 2018)). The TME was evaluated by the estimation of stromal and immune cells in malignant tumor tissues using expression data (ESTIMATE) immune data, stromal score (Yoshihara et al., 2013), and cell-type identification by estimating relative subsets of RNA transcripts (CIBERSORT) and specific immune cell types (Newman et al., 2019). Given that genome instability contributes to tumor heterogeneity, which leads to drug resistance (Morel et al., 2017; Dagogo-Jack and Shaw, 2018; Sansregret et al., 2018), we performed cancer stemness and drug sensitivity analysis for these genes. Malta et al. (2018) identified a novel index to evaluate cancer stemness features based on DNA methylation patterns (DNA stemness score (DNAss)) and mRNA expression patterns (RNA stemness score (RNAss)). We calculated the Spearman correlation between each gene and cancer stemness. Drug sensitivity analysis was also performed using an open-access database, specifically National Cancer Institute (NCI)-60. The Kolmogorov-Smirnov test was applied to verify the normality of the included indexes, including TMB/MSI scores, NCI-60 indexes, TME indexes, and cancer stemness indexes (Supplementary Table 2). We found that the drug sensitivity index was normally distributed. Therefore, the method of Pearson’s correlation to explore the association between gene expression and drug sensitivity is appropriate.
Expression Pattern Validation by qPCR
Total RNA was isolated from COAD cell lines named Caco-2, Lovo, HCT116, and HT29 using the RNeasy Mini Kit (QIAGEN, Hilden, Germany), according to the manufacturer’s instructions. Complementary DNA (cDNA) was produced by RNA using the PrimeScriptTM Reverse Transcription Kit (TakaRa, Maebashi, Japan) in an ABI 7500 System (Applied Biosystems, Thermo Fisher Scientific, Waltham, MA, United States). The primers specific for target genes were designed and synthesized by Tianyi Huiyuan Biotech (Beijing, China). The following procedures were performed: activation of enzymes at 50°C for 2 min and then 95°C for 2 min, 45 cycles of denaturation at 95°C for 15 s, annealing at 58°C for 20 s, and extension at 72°C for 30 s. The relative expression levels of the target genes were calculated using the 2−ΔΔCT method. Glyceraldehyde 3-phosphate dehydrogenase (GAPDH) was used as the internal control. All qPCR reactions were performed in triplicate. The primers used in this study are listed below.
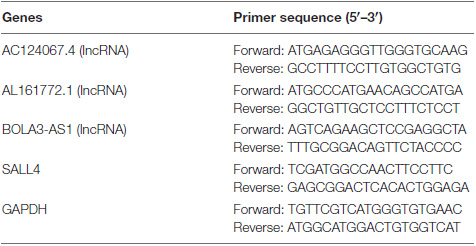
Mutation Analysis
Specific somatic mutations drive cancer development (Koch, 2017). Therefore, the mutation analysis of the target mRNAs was applied, including somatic mutations and copy number variation (CNV) analysis. The R package “RCircos” was used to explore the chromosome location and CNV number for each gene. We then explored the alteration rate, type, and site in the COAD datasets and examined the association between the alteration status and gene expression. We calculated the Spearman’s correlation TMB and MSI scores and assessed the expression of each gene to explore whether the target mRNAs were directly associated with genome instability. Furthermore, the association between target mRNA alteration status and MSI status was explored. All mutation analyses were performed using the online database cBioportal.
Statistics
SPSS Statistics (version 26.0; IBM, Armonk, NY, United States) and Microsoft Excel (Microsoft Corporation, Redmond, WA, United States) were used to present the retrieved data and perform the Chi-square test. R language (version 4.0.3) was used to perform machine learning algorithms and correlation analysis (R Core Team, 2013). The Benjamini and Hochberg method was used to adjust the p-Value for DEG extraction.
Results
Differential Expression Genes Extraction and Hierarchical Clustering
In total, 123 GIRlncRs were extracted between the GS and GU groups (Figure 1A). We constructed a mRNA and GIRlncR coexpression network (Figure 1B) to show the top 10 close interactions between each GIRlncR and corresponding mRNAs, indicating that the GIRlncRs regulate genome instability and complex tumor cell biological processes. Functional analysis further illustrated the relationship between GIRlncRs and tumor immunity (Figures 1C,D). Almost all GO- and KEGG-enriched pathways involved immune responses, such as antigen processing and T-cell activation. The unsupervised hierarchical clustering based on GIRlncRs was employed to divide COAD patients into two clusters with various genome instability statuses and immune therapy response rates. In total, 453 samples were clustered into GS-like (302 samples) and GU-like groups (151 samples; Figure 1E). Surprisingly, the TMB, MSI score, and expression of all immune checkpoint-related genes were higher in the GU-like cluster (p < 0.05), whereas expression of the three MMR genes (MLH1, PMS2, MSH2) was lower (p < 0.05; Figures 1F–I). The mutation rates of all four MMR genes were higher in the GU-like group (p < 0.05). The ssGSEA showed that almost all immune cells and immune functions were increased in the GU-like group (Figures 1J,K). Furthermore, the mutation rate of the novel immunotherapy response rate biomarker POLE was also significantly higher in the GU-like group (p < 0.05; Figure 1L). These findings strongly suggest that our clustering based on GIRlncRs could predict TME infiltration and ICI response rates in COAD patients. However, as shown in Figure 1M, the OS of the two clusters did not differ, indicating that the clustering method could not fulfill the purpose of prognosis.
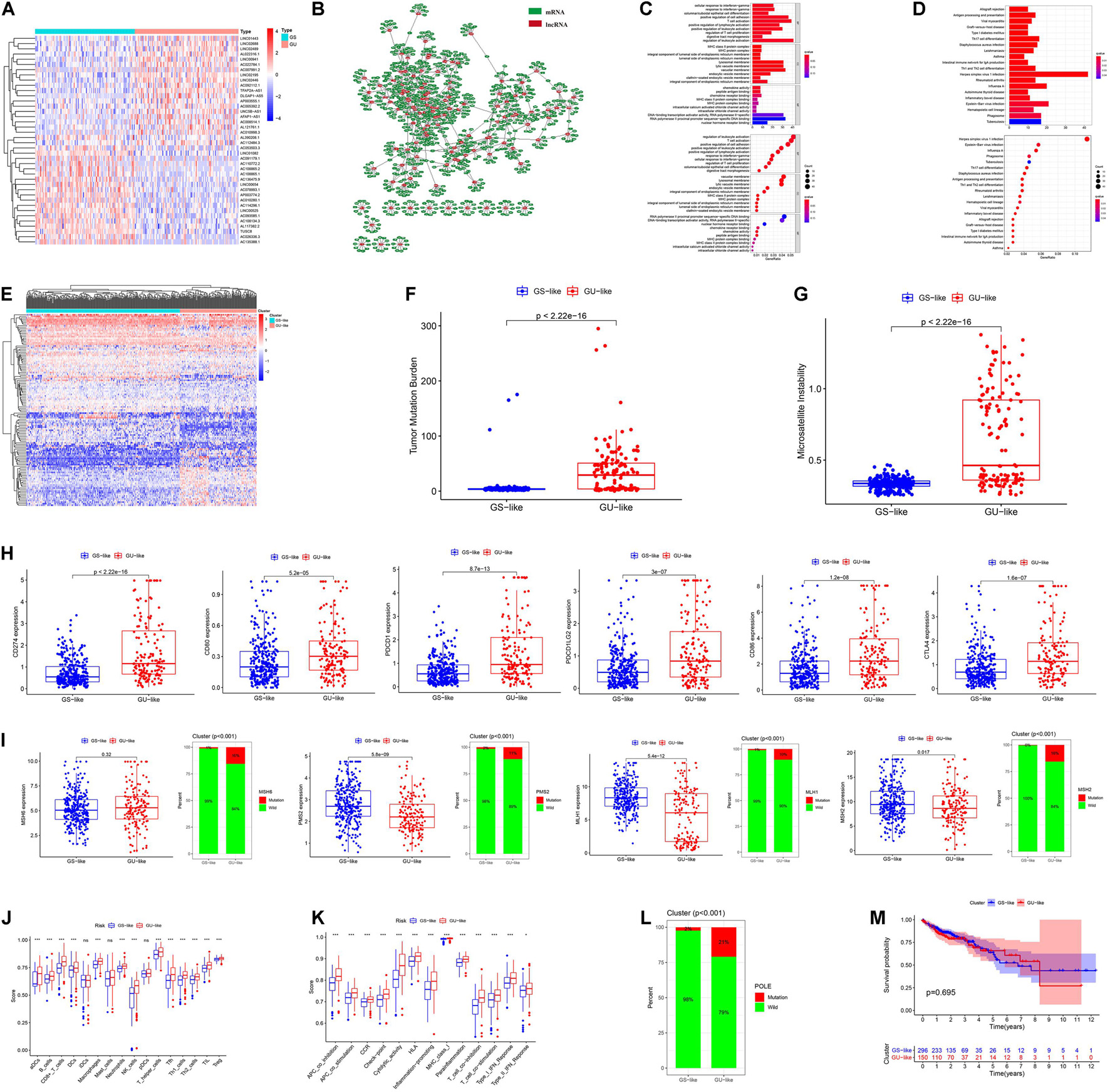
Figure 1. Hierarchical clustering and analysis of the genome stable (GS)-like and genome unstable (GU)-like clusters. (A) The heatmap of 123 genome instability-related lncRNAs (GIRlncRs) between the GS and GU groups. (B) The coexpression network of the GIRlncRs and top-10 correlated mRNAs for each lncRNAs by Pearson’s correlation. The functional enrichment analysis of the coexpressed mRNAs of GIRlncRs based on GO (C) and KEGG (D). (E) The unsupervised hierarchical clustering based on 123 genome instability-related lncRNAs. The comparison of the tumor mutational burden (F), microsatellite instability (G), expression of six immune checkpoint-related genes (CD274 (PD-L1), CD80, PDCD1 (PD-1), PDCD1LG2(PD-L2), CD86, and CTLA4) (H), expression and mutation rate of four DNA mismatch repair protein gene (MLH1, MSH2, MSH6, and PMS2) (I) and mutation rate of POLE (L) between the two clusters by Mann-Whitney U test. The comparison of the immune cell infiltration (J) and Immune functions (K) of the two clusters by ssGSEA. (M) The survival analysis of the two clusters by log-rank test. *p < 0.05; ***p < 0.001; and ns, not significant.
Multivariate Cox Regression to Construct the Genome Instability-Related LncRNA Prognostic Signature
Since our clustering could not predict OS in COAD patients, we constructed a novel GIlncPS. The basic characteristics of the training and test cohorts are shown in Supplementary Table 3. The Chi-square test showed that the division of training and test cohorts was proper without selection bias (all p > 0.05). The regression results are shown in Supplementary Table 4. An eight-lncRNA GIlncPS was acquired as follows: risk score = 0.239 × LINC01807 + 0.235 × AC009237.14 + 0.310 × LOXL1-AS1 + 0.028 × AC005392.2 + 0.751 × AP003555.1 + 0.384 × BOLA3-AS1 – 0.330 × PTPRD-AS1 + 0.258 × AC004009.1. The high- and low-risk groups were divided according to median risk score. Survival analysis indicated that OS was significantly longer in the low-risk group in the training, test, and entire TCGA cohorts (p < 0.05; Figures 2A–C). Notably, all the included lncRNAs, except PRPRD-AS1, were risk factors for poor prognosis in COAD patients. The heatmap shows that all of these lncRNAs, except PRPRD-AS1, were overexpressed in high-risk groups because only PRPRD-AS1 was a protective factor (Figures 2D–F). The AUCs of the ROC in the three cohorts were acceptable for the lncRNA prognostic signature. Only the AUC at 1 year in the test cohort did not reach 0.7 (Figures 2G–I). Compared with the prognostic models of Li and Jin, our GIlncPS had the best performance (AUC = 0.735, 0.745, and 0.741, respectively; Figures 2J–L). Independent prognostic analysis indicated that old age, advanced stage, and occurrence of metastasis were significantly related to poor prognosis, and a low-risk score was significantly related to good prognosis (p < 0.05; Figures 3A,B). Figure 3C shows the nomogram for clinical use. The calibration curve demonstrated the reliability of the nomogram (Figure 3D). We also compared TMB/MSI and TME infiltration and functions between the high- and low-risk groups. However, the results differed from those of hierarchical clustering. As shown in Supplementary Figures 1A–H, all ICI outcome predictive biomarkers, except MSI, were not significantly different between the two groups, indicating that our prognostic signature behaved poorly in predicting genome instability, TME infiltration, and ICI response rates for COAD patients. Furthermore, Supplementary Figure 1I shows that the mutation rate of BRAF was higher in the high-risk group (19% vs. 11%). The prognostic ability of the GIlncPS was better than that of BRAF (p < 0.01; Supplementary Figure 1J). The OS in the high-risk groups was always lower than that in the low-risk groups, regardless of the BRAF mutation type. To conclude, we constructed a GIlncPS with strong prognostic ability, but it could not predict the TME infiltration and ICI response rates for COAD patients.
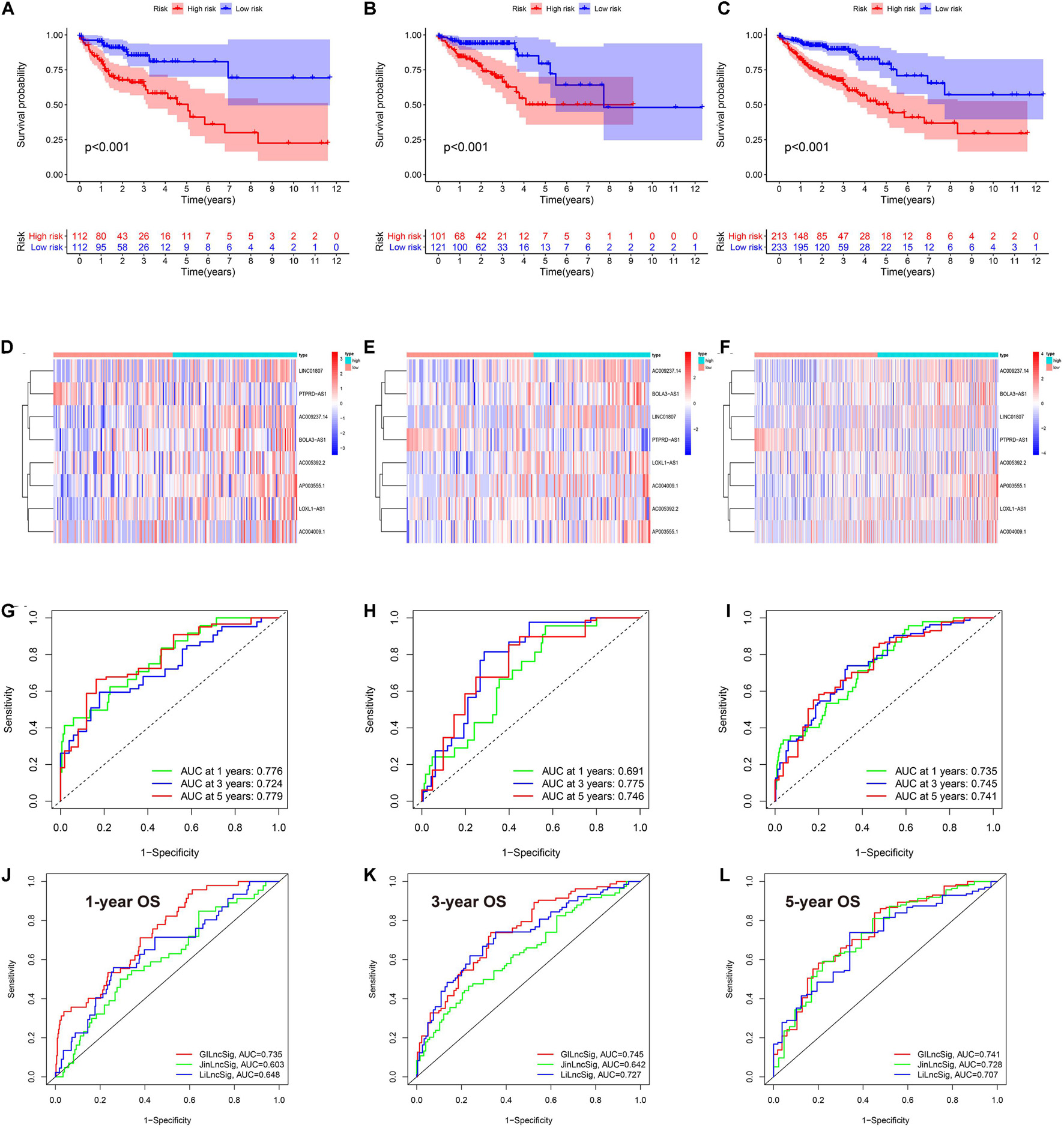
Figure 2. The eigth lncRNA prognostic signature in TCGA cohort. (A–C) Survival analysis between the high- and low-risk groups based on overall survival (D–F). Heatmap of the eight lncRNA between high- and low-risk groups (G–I). rROCeceiver operating characteristics curve of the signature model based on OS at 1-3-5-year. AUC, the area under the curve. (A,D,G) Training cohort; (B,E,H) are test cohort; (C,F,I) are TCGA cohort. Model comparison with two previously published lncRNA prognostic signatures by ROC curve based on overall survival in TCGA cohort at 1-(J), 3-(K), 5-(L) year.
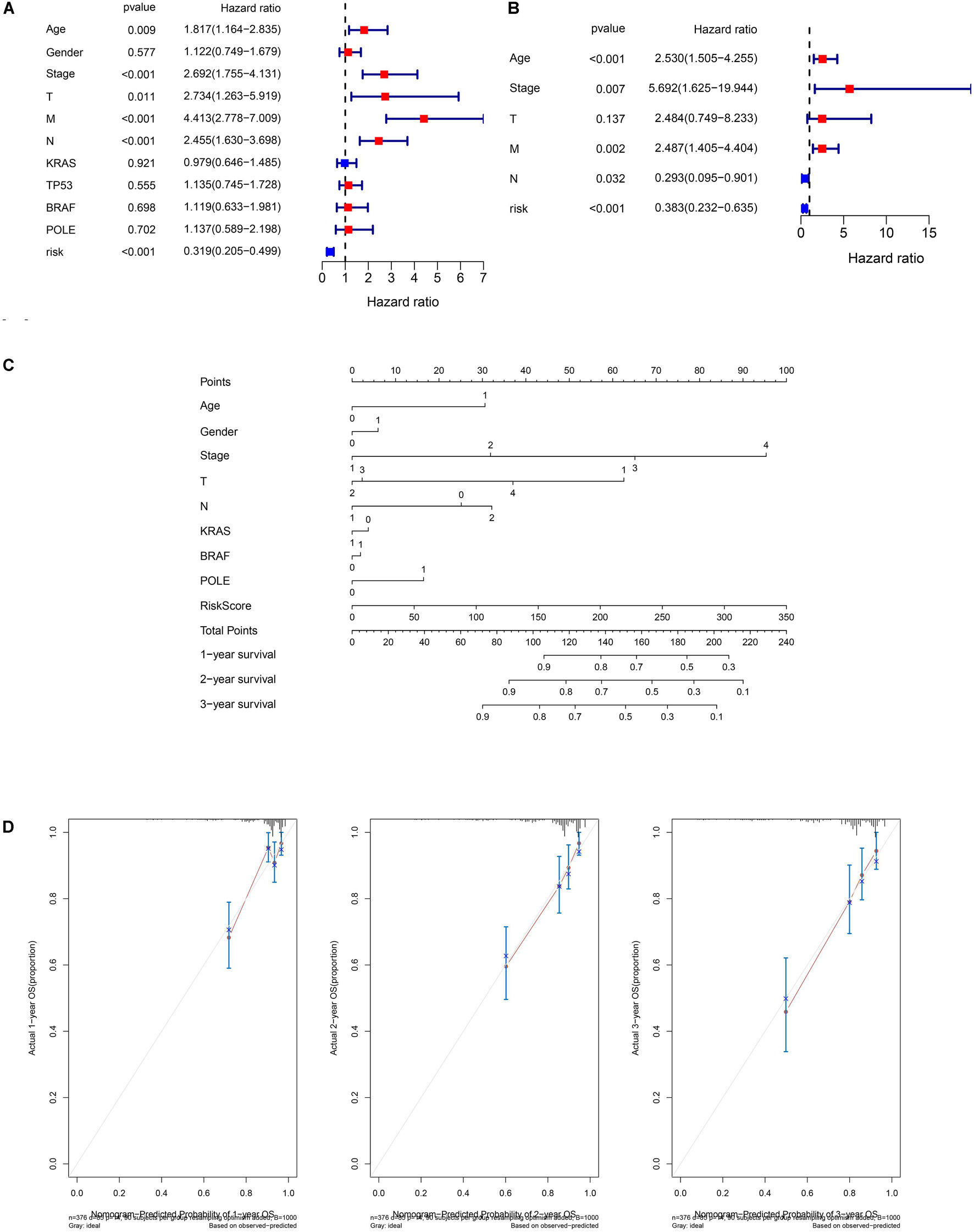
Figure 3. Independent prognostic analysis and nomogram of the 8-lncRNA prognostic signature. (A) Univariate Cox regression of the TCGA cohort. (B) Multivariate Cox regression of the TCGA cohort based on overall survival. Age (>65 vs. ≤65), gender (male vs. female), stage (III/IV vs. I/II), T (III/IV vs. I/II), M (I vs. 0), N (I/II vs. 0), KRAS (mutation vs. wild), TP53 (mutation vs. wild), BRAF (mutation vs. wild), POLE (mutation vs. wild), and risk (low-risk vs. high-risk). (C) Nomogram of the TCGA cohort based on overall survival (M was not included in the nomogram due to only 20 patients with M1). (D) Calibration curve of the TCGA cohort based on overall survival at 1, 2, and 3 years.
Analysis of Mutation Profile and Identification of Hub-Long Noncoding RNAs in Clusters and Risk Groups
The Sankey plot showed the relationship among the GS-and GU-like clusters, risk groups, and survival status (Figure 4A). The results showed no significant difference between the clusters and risk groups, and the death proportion in the high-risk group was higher. The top three genes with the highest mutation frequencies in the GS-like cluster were APC (86%), TNN (40%), and TP53 (65%) (Figure 4B), whereas those in the GU-like cluster were APC (53%), TTN (69%), and TP53 (31%) (Figure 4C). In general, the mutation frequency in the GU-like cluster was higher than that in the GS-like group, and the frequencies of multi-hit and frame-shift mutations were much higher. LASSO and SVM algorithms were utilized to extract hub-lncRNAs in GS- and GU-clusters for further investigation. We selected the minimum value of λ, a penalty parameter of 0.1, and the L1 norm of the default (Figures 4D,E). Here, 43 lncRNAs were screened using LASSO regression. The average weight of each lncRNA acquired from the 10-fold test SVM algorithm is provided in Supplementary Table 5. The cut-off value was set at | 0.28|. The Venn plot of the selection process of hub-lncRNA among SVM, LASSO, and the prognostic signature is presented in Figure 4F. In total, 55 hub lncRNAs were identified. The screening process of the lncRNAs, miRNAs, and mRNAs included in the ceRNA network is presented in Figure 4G.
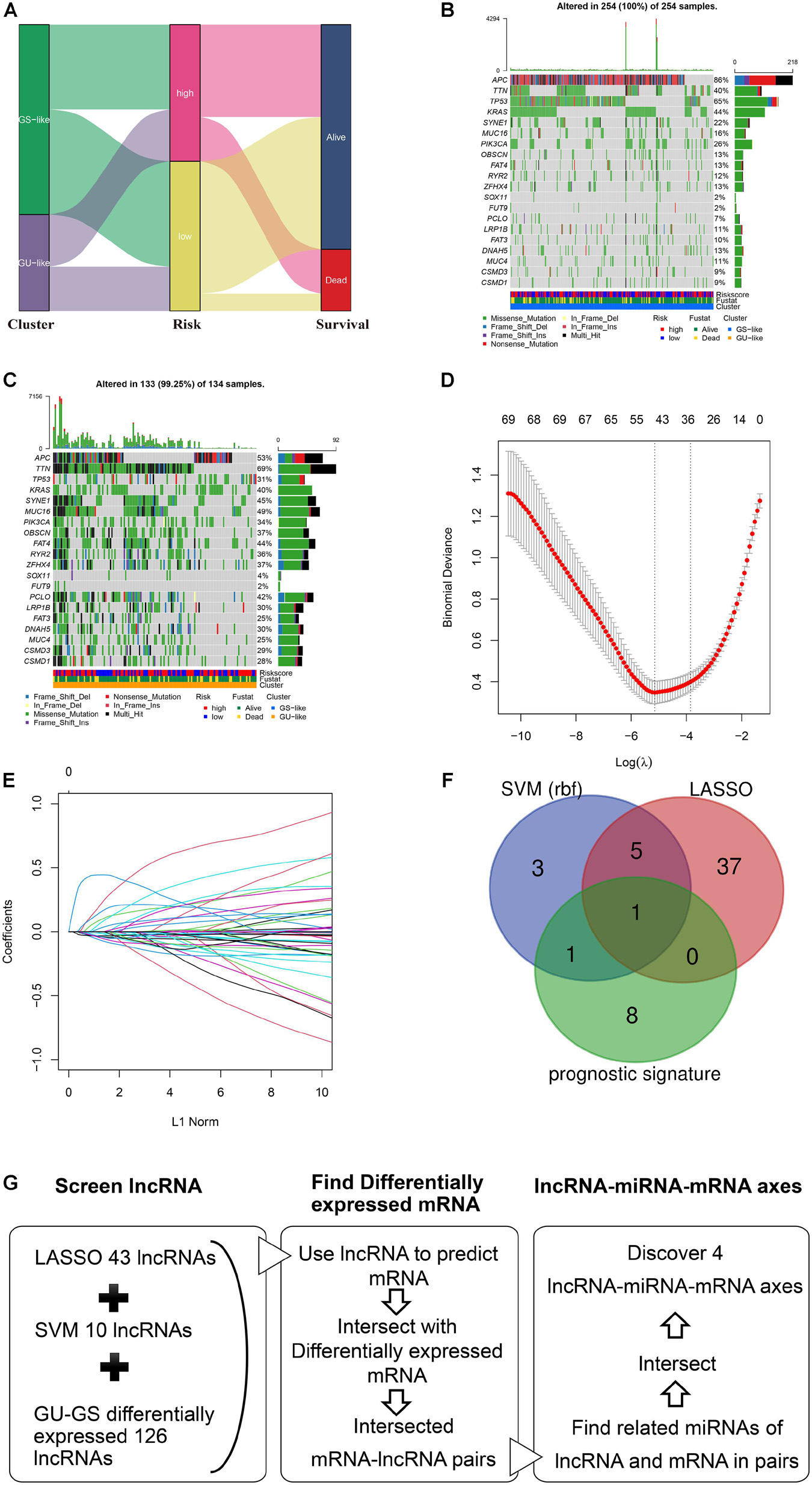
Figure 4. Analysis of mutation profile and identification of hub-lncRNAs in genome stable (GS)- and genome unstable (GU)-like clusters. (A) Sankey plot of the relationship among GS- and GU-like clusters, risk groups, and survival status. The mutation profile of GS-like cluster (B) and GU-like cluster (C). (D) The selection of λ in least absolute shrinkage and selection operator regression. (E) The selection of L1 norm in LASSO regression. (F) The Venn plot of hub-lncRNA in SVM (support vector machine), LASSO, or prognostic signature. (G) Screening process of the lncRNA, miRNA, mRNA included in the ceRNA network.
Genome Instability-Related Competing Endogenous RNA Network and Single-Gene Analysis
The ENCORI database was utilized to predict the interactions between hub lncRNAs and their target mRNAs. The constructed ceRNA network is shown in Figure 5A and Table 2. Seven lncRNA-miRNA-mRNA pairs were found in the ceRNA network. To comprehensively understand these three lncRNAs (BOLA3-AS1, AC124067.4, and AL161772.1) and four target RNAs (NINL, SALL4, TNFSF11, PHYHIPL), we performed differential expression, cancer stemness, immune subtype, TME infiltration, and drug sensitivity analyses.
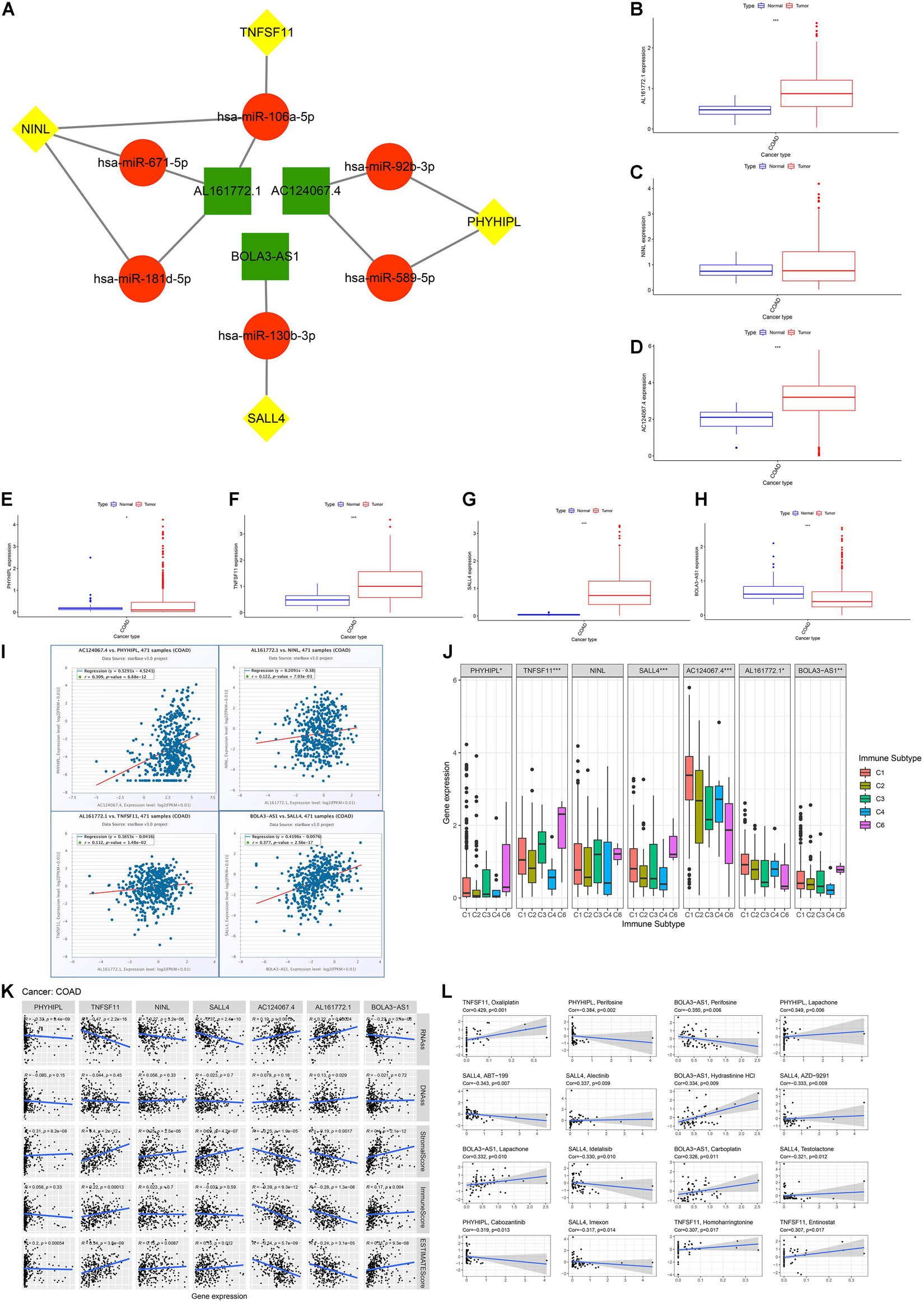
Figure 5. Competing endogenous RNA (ceRNA) network construction and immune, cancer stemness, and drug sensitivity analysis. (A) The genome instability-related ceRNA network in colon adenocarcinoma (COAD) based on ENCORI. (B–H) The differential expression analysis included three lncRNAs (BOLA3-AS1, AC124067.4, AL161772.1) and four target mRNAs (NINL, SALL4, TNFSF11, PHYHIPL) by Mann-Whitney U test. (I) The correlation between the expression of each lncRNA and its target mRNAs based on ENCORI. (J) The association between the seven gene expression and five immune subtypes in COAD. (K) The association between the seven-gene expression and cancer stemness based on mRNA expression (ssRNA) and DNA methylation patterns (DNAss); the association between the seven-gene expression and the ESTIMATE immune score, stromal score, and ESTIMATE score. (L) The association between the seven-gene expression between drug sensitivity based on the NCI60 database (top 16 ranked by correlation). *p < 0.05, ** p < 0.01, and ***p < 0.001.
First, we compared the expression of seven genes between normal and tumor tissues using the Mann-Whitney U test in COAD. As shown in Figures 5B–H, AC124067.4, AL161772.1, SALL4, and TNFSF11 were highly expressed in COAD, whereas BOLA3-AS1 and PHYHIPL were expressed at low levels (p < 0.05). These results indicate that AC124067.4, AL161772.1, SALL4, and TNFSF11 might promote COAD development and that BOLA3-AS1 and PHYHIPL might inhibit its development (Figure 5I). As expected, the four lncRNA-mRNA expression patterns met the ceRNA criterion, especially AC124067.4-PHYHIPL (r = 0.309, p = 6.88e-12) and BOLA3-AS1-SALL4 (r = 0.377, p = 2.56e-17). It has been reported that genome instability is closely related to tumor immunity. ANOVA was used to detect whether the expression of the seven genes was significantly related to the immune subtypes in COAD (Figure 5J). The results showed that six genes (BOLA3-AS1, AC124067.4, AL161772.1, SALL4, PHYHIPL, and TNFSF11) were significantly associated with the COAD immune subtype (p < 0.05), indicating that these seven genes could modulate tumor immune functions at the genetic level. These seven genes were also closely related to genome instability and immune-related genes (Supplementary Figure 2). All seven genes were positively related to MLH1, PMS2, and NORAD (p < 0.01). In addition, most genes (4/7, 57.1%) were negatively correlated with PD-L1 (CD274; p < 0.05). These findings suggest that the high expression of these seven genes contributes to genomic integrity and predicts poor ICI outcomes. Moreover, these seven genes are closely related to COAD TME infiltration and function (Figure 5K). Most genes were positively related to stromal cell infiltration (5/7, 71.4%) and immune cell infiltration (4/7, 57.1%) and negatively related to tumor purity (5/7, 71.4%). According to the Spearman’s correlation between cancer stemness features and these seven genes, we found that most genes were negatively related to cancer stemness (5/7 (71.4%) for RNAss and 4/7 (57.1%) for DNAss; Figure 5K). Finally, drug sensitivity analysis was performed to explore the relationship between the seven genes and drug resistance. The results showed that the expression of these seven genes was positively associated with the sensitivity of drugs regulating DNA replication and synthesis. For example, TNFSF11 expression was positively associated with sensitivity to oxaliplatin, a common platinum-based antineoplastic drug for colorectal cancer, which is believed to function by blocking the duplication of DNA (r = 0.429, p < 0.001). Meanwhile, some protein kinase inhibitors, such as perifosine and cobimetinib, were negatively related to these genes. In conclusion, we proposed a novel ceRNA network that modulates genome instability and found that these seven genes are involved in TME infiltration, cancer stemness, and drug resistance.
Expression Pattern Validation by qPCR
To ascertain these potential prognostic biomarkers, three lncRNAs (AC124067.4, AL161772.1, BOLA3-AS1) with significant expression alterations and one mRNA SALL4 were further performed by qRT-PCR validation. The results showed that AC124067.4, AL161772.1, and SALL4 gene levels were significantly increased in COAD cell lines (Figures 6A–D) whereas BOLA3-AS1 (Figure 6) was significantly reduced, which was consistent with the above analysis. Taken together, the expressions of AC124067.4, AL161772.1, and SALL4 were significantly upregulated, and their expression level was also associated with the OS rate in patients with COAD. These findings indicate the possibility of using the panel as a prognostic biomarker for COAD.
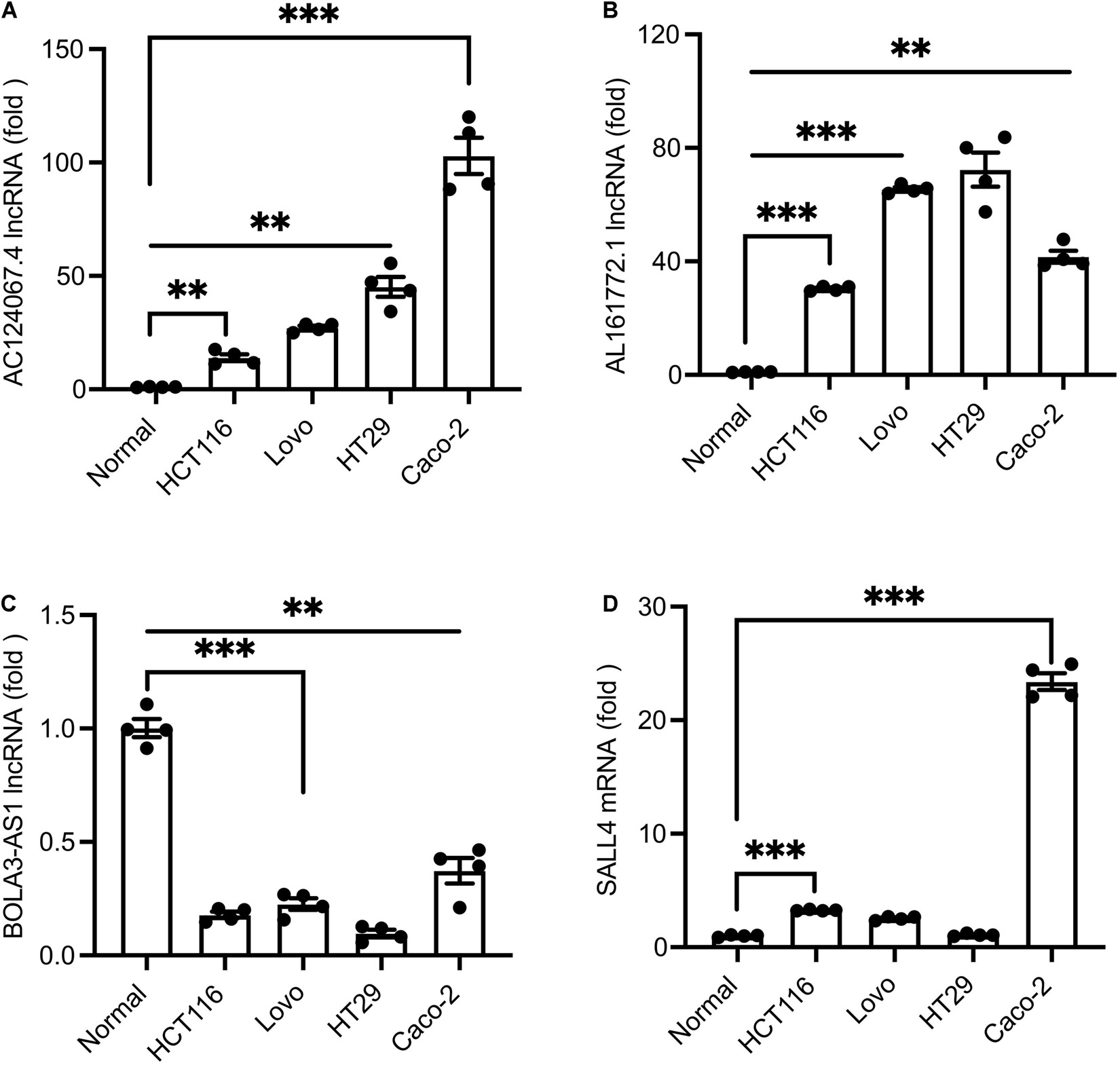
Figure 6. (A–D) qPCR verification of the expression levels of differential lncRNAs in COAD cell lines and normal cells. Values are shown as the mean ± SEM (n = 4). ***p < 0.001 and **p < 0.01 by Student’s t-test. Normal cells were employed as controls.
Mutation Analysis
First, we explored the rates of alteration and types with respect to the four target mRNAs. As shown in Figures 7A–D, the rates of NINL, PHYHIPL, SALL4, and TNFSF11 alteration were 5%, 0.8%, 6%, and 2%, respectively. For NINL and SALL4, somatic mutations and CNVs accounted for almost half of these for each. Regarding PHYHIPL alterations, somatic mutations were predominant, whereas CNVs formed the majority of TNFSF11 alterations. Missense mutations accounted for most somatic mutations among the four gene alterations, and amplification accounted for most CNVs among the NINL, SALL4, and TNFSF11 alterations. The alteration sites of each target gene were also explored. Notably, all four gene alteration sites were dispersed across the entire gene, rather than clustering on specific domains, indicating that the four-gene alterations mainly affect mRNA expression but not the coded protein activity (Figures 7E–H). Therefore, we investigated whether alterations in the four genes were associated with their expression. As expected, three genes (NINL, SALL4, and TNFSF11) were expressed at low levels for mutated types, and the CNVs of the three genes were positively related to mRNA expression. However, the rate of PHYHIPL alterations was only 0.8%, and PHYHIPL expression was not related to its alteration type.
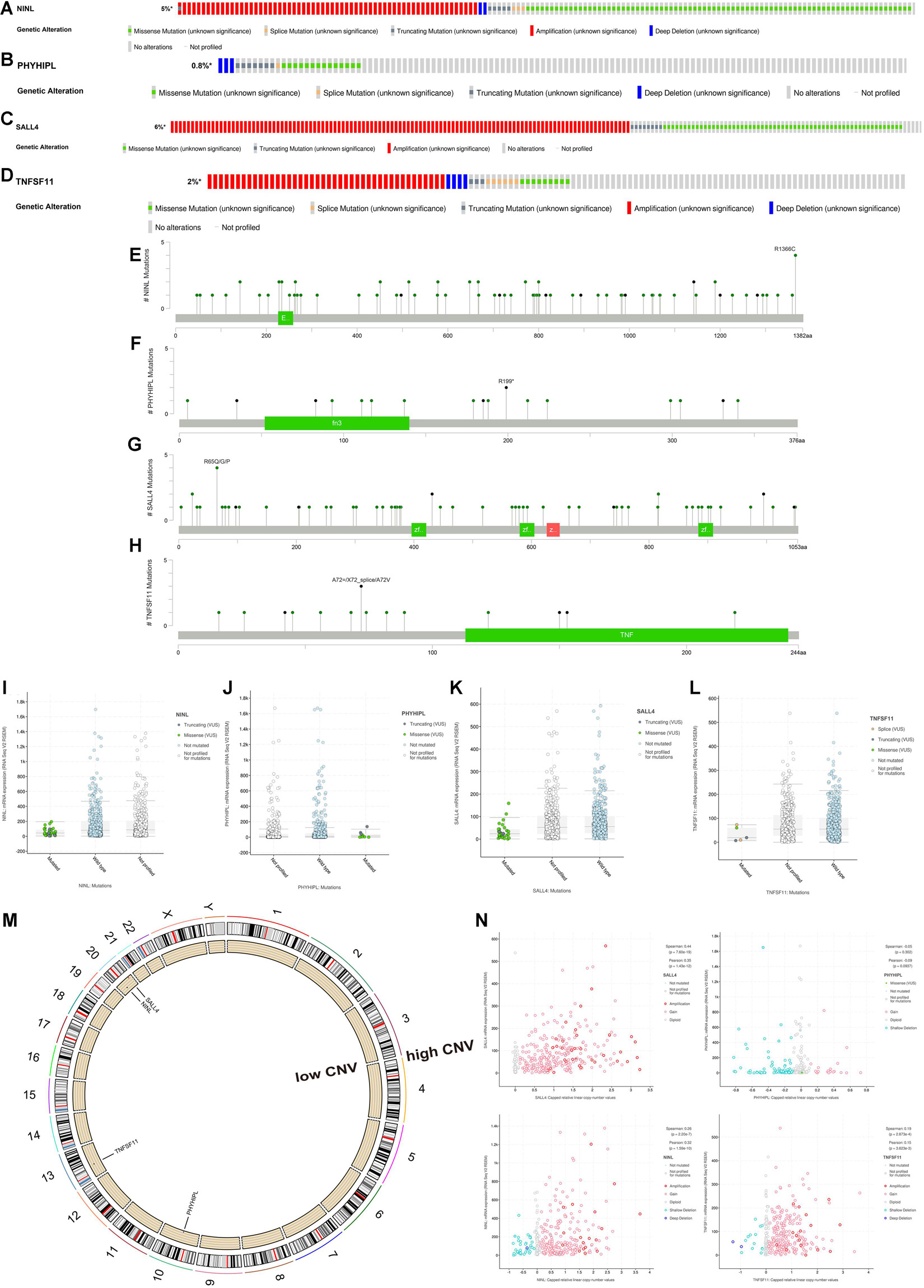
Figure 7. Somatic mutation and copy number variation (CNV) analysis of three lncRNAs (BOLA3-AS1, AC124067.4, AL161772.1) and four target mRNAs (NINL, SALL4, TNFSF11, PHYHIPL). The alteration rates and types of NINL (A), PHYHIPL (B), SALL4 (C), and TNFSF11 (D). The alteration sits of each gene on the coding protein domains for NINL (E), PHYHIPL (F), SALL4 (G), and TNFSF11 (H). The comparison of gene expression between wild type and mutated type for NINL (I), PHYHIPL (J), SALL4 (K), and TNFSF11 (L). (M) The location on a chromosome and the most common CNV status of the four target mRNAs. (N) The association between gene expression and CNV for NINL, PHYHIPL, SALL4, and TNFSF11.
Microsatellite instability has become a recognized biomarker for immunotherapy and has been widely used in clinical practice. It is speculated that the seven genes proposed in this study could serve as clinically novel TMB and MSI biomarkers. As shown in Figures 8A–D, we explored the association between each gene and TMB/MSI in the pan-cancer database (the abbreviations are provided in Supplementary Table 6). Surprisingly, all the expression of all seven genes was negatively related to TMB and MSI scores in COAD (p < 0.05), indicating that these seven genes could maintain genomic integrity in COAD, which was particularly apparent for AC124067.4 (correlation coefficient R reached approximately –0.4 for TMB and –0.5 for MSI) and SALL4 (R reached approximately –0.3 for TMB and –0.35 for MSI; Figures 8A–D). Moreover, we compared MSI status between patients with mutated and wild-type disease using cBioPortal. The MSI-H proportions were greater in patients with the mutated type than in those with the wild type according to all four genes (Figure 8E). Especially, the MSI-H proportion reached almost 60% in patients with mutated SALL4, whereas only 10% of wild-type patients had MSI-H. We next explored the association between the seven CNVs and the MSI status. As shown in Figure 8F, the CNVs of NINL, SALL4, and TNFSF11 were fewer in patients with MSI-H COAD. In conclusion, the seven genes proposed in this study were closely related to genome instability in COAD and could serve as novel immunotherapy outcome biomarkers.
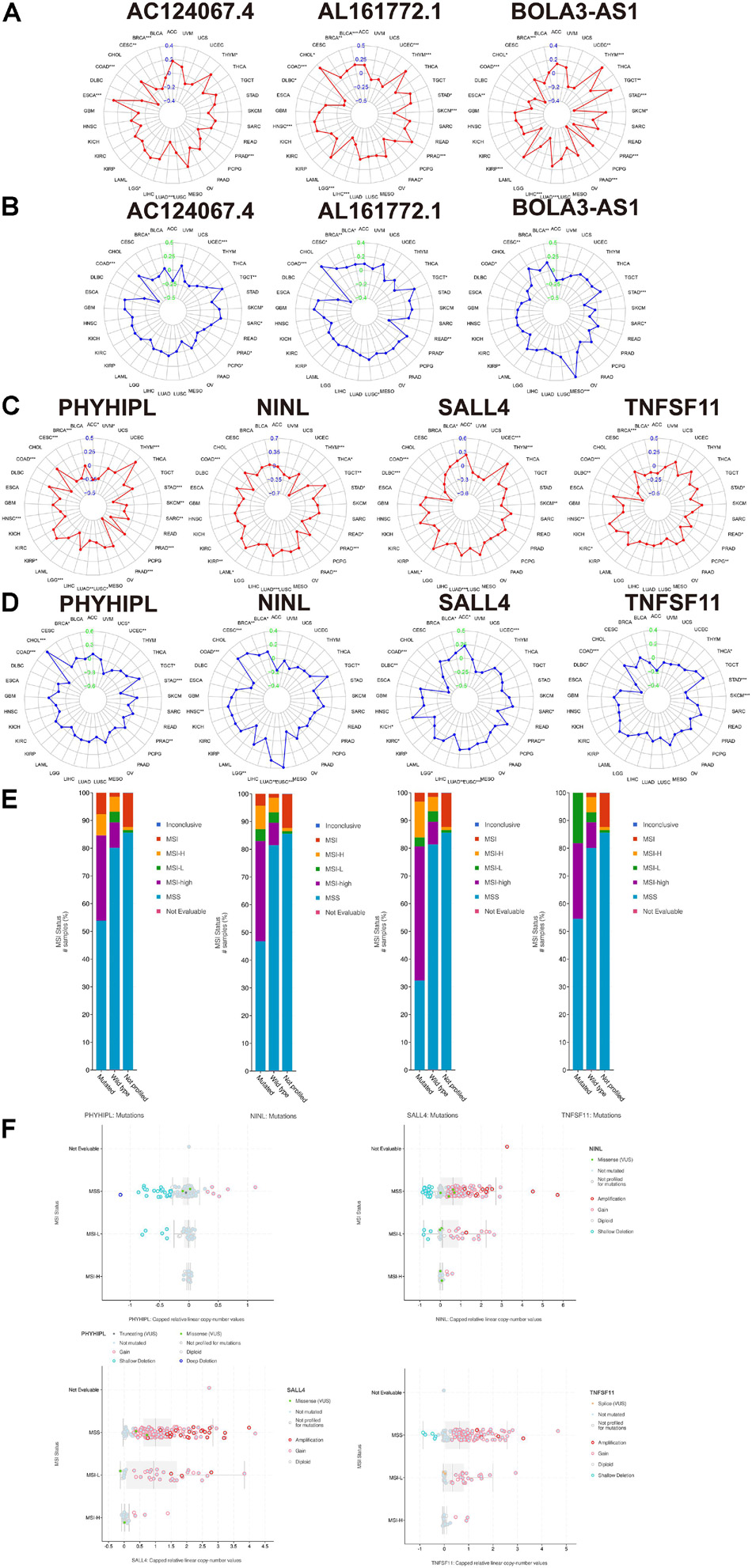
Figure 8. The association between the three lncRNAs (BOLA3-AS1, AC124067.4, and AL161772.1), four target mRNAs (NINL, SALL4, TNFSF11, and PHYHIPL), and the tumor mutational burden (TMB), microsatellite instability (MSI) score, and MSI status. (A) The association between the three lncRNA expression and the TMB in pan-cancer using Spearman’s correlation. (B) The association between the three lncRNA expression and the MSI score in pan-cancer. The association between the four target mRNAs and the TMB (C) and MSI score (D) in pan-cancer. (E) The comparison of the MSI status between the wild type and mutated type of the four target mRNAs (adjusted by each gene mutation rate) in COAD based on cBioPortal. (F) The association between the MSI status and the copy number variation of the four target mRNAs in COAD based on cBioPortal.
Discussion
This study presented primary work comprising a computational bioinformatics analysis to identify the critical genetic/epigenetic biomarkers from the genome instability-related ceRNA network involved in COAD. This study first provided a hierarchical clustering method to stratify patients for immunotherapy and a prognostic signature for clinical reference. Subsequently, genome insatiability-related ceRNA network genes were identified as critical regulators modulating TME infiltration, cancer stemness, and drug resistance. The identification of the lncRNA-miRNA-mRNA axes AL161772.1-has-miR-671-5p (hsa-miR-181d-5p, has-miR-106a-5p)-NINL, AL161772.1-has-miR-106a-5p-TNFSF11, AC124067.4-hsa-miR-92b-3p (hsa-miR-589-5p)-PHYHIPL, and BOLA3-AS1-has-miR-130b-3p-SALL4 and the construction of a ceRNA network based on these four axes were the most significant findings of this study and are the main object of this discussion.
Among the four identified lncRNA-miRNA-mRNA signaling axes, BOLA3-AS1-has-miR-130b-3p-SALL4 was considered the most valuable in modulating the tumorigenesis of COAD. SALL4 and BOLA3-AS1 were identified and defined as the most promising biomarkers, not only because of their respective high correlations with the same miRNA, which plays a role as a sponge and an intermediate, but also because of their highly consistent and synergic features. The explicit role of SALL4 in COAD can be attributed to its pivotal role in cell proliferation, apoptosis, invasive migration, chemoresistance, and the maintenance of cancer stem cells (Zhang et al., 2015). First, SALL4 is highly expressed and positively associated with BOLA3-AS1 in COAD tumor tissues (Figure 5I), suggesting a positive regulation between them. Second, this work provides a novel viewpoint on how SALL4 interacts with cancer cell stemness, thereby participating in tumor metastasis and progression. This work resulted in an estimate stemness score to contrast and evaluate the degree of stemness associated with genes (Figure 5K). SALL4 and BOLA3-AS1 both yielded impressive results in the multidimensional grading scale of the stemness score. SALL4 has long been regarded as an essential modulator in maintaining embryonic stem cell (ESC) self-renewal and pluripotency (Miettinen et al., 2014). Considering that it is largely considered that ESCs and cancer stem cells share similar metabolic states, SALL4 regulates the activation of several critical signaling pathways in stem cells by upregulating the expression of target genes in the Wnt/β-catenin pathway, and thus, its powerful regulatory role in cancer stemness might be explained in a similar way (Ma et al., 2006; Jang et al., 2015). Third, SALL4 and BOLA3-AS1 were associated with significant drug sensitivity (Figure 5l). Among the selected panels, filtered based on significance ranking, SALL4-related and BOLA3-AS1-related drugs accounted for the largest proportion. Through a literature search on PubMed, almost all related drugs are associated with their outstanding function as inhibitors of oxidative phosphorylation (de Witte et al., 2004; Burgeiro et al., 2013; Hammadi et al., 2015). Tan et al. (2019) reported the high-throughput screening of inhibitors of oxidative phosphorylation and SALL4, activating the transcription of genes that regulate oxidative phosphorylation to increase oxygen consumption, mitochondrial membrane potential, and ATP generation in cancer cells, which is the most predominant scientific finding on SALL4. Oxidative phosphorylation plays a critical role in the repair of DNA damage repair (dMMR). This is associated with the genome instability discussed in this article because dMMR is one of the culprits regulating genome instability. It is important to consider how SALL4 was selected at first; specifically, it comprises one end of an axis, and at the other end is one of the most core genome instability-related lncRNAs, BOLA3-AS1. An entire ceRNA network was constructed on this basis. Therefore, although we still do not know the precise role of SALL4 in COAD, the mechanism by which it influences genome instability and further promotes tumor progression via such a BOLA3-AS1-miRNA-SALL4 axis is very likely to exist and could be explained.
Another important line of evidence when discussing these axes is the pan-cancer TMB/MSI correlation. For the AL161772.1-miRNA-NINL axes, both AL161772.1 and NINL showed a significantly negative correlation with TMB and MSI in COAD, which indicates a function in maintaining genomic integrity. Similarly, AL161772.1-has-miR-106a-5p-TNFSF11 and AC124067.4-hsa-miR-92b-3p(hsa-miR-589-5p)-PHYHIPL both reduce the TMB and MSI in COAD, decreasing the risk of alterations and genome instability.
Diverse degrees of mutations were noted for NINL, TNFSF11, and PHYHIPL. NINL and TNFSF11 have relatively high genetic alteration rates, and the type is primarily amplification. The sites of NINL alterations were the coding protein domains, which were scattered and concentrated in segments. The main mutation site of NINL is on chromosome 22, which is associated with relatively lower CNV, which is the same as that for SALL4, but SALL4 has a higher CNV, and the mutation rate of PHYHIPL is not high. The genetic alteration site was also not dense. It is reasonable to speculate that major alterations do not include PHYHIPL mutations.
Two major weaknesses of this study should be further improved. One is that even though a novel ceRNA mechanism, regulating certain lncRNA-miRNA-mRNA axes and cancer promotion in COAD, was suggested, whether these genes could play a synergistic role in regulating tumor progression needs to be confirmed. More work also needs to be done to provide more details on how these genes interact with each other at the cellular level, with validation based on a clinical cohort. Another limitation is that the crucial genes screened have been rarely reported and researched previously. Although it is easy to find clues relevant to this topic, it is difficult to find direct support of the importance of these genes in the literature. In fact, this finding underlies the importance of this work, which suggests valuable biomarkers for COAD treatment for further research.
This study constructed a prognostic signature of genome instability-related lncRNA and immunotherapy for clinical reference. It also provided a potential ceRNA mechanism through which lncRNAs play a role via specific lncRNA-miRNA-mRNA axes to participate in the process of cancer development.
Data Availability Statement
The original contributions presented in the study are included in the article/Supplementary Material, further inquiries can be directed to the corresponding author/s.
Author Contributions
ZR and ZW performed the data analysis and wrote the first draft. DG and HM revised the first and the second drafts. MC and YZ performed the experiments. YZ revised the second draft. JZ designed and supervised the study. All authors contributed to the article and approved the submitted version.
Funding
This work was supported by the National Natural Science Foundation of China (82071791 and 31471016), the CAMS Initiative for Innovative Medicine (2016-I2 M-1-008), the National Key Research and Development Program of China (2016YFA0101001), and the CAMS Basic Research Expenses (2018PT31052).
Conflict of Interest
The authors declare that the research was conducted in the absence of any commercial or financial relationships that could be construed as a potential conflict of interest.
Publisher’s Note
All claims expressed in this article are solely those of the authors and do not necessarily represent those of their affiliated organizations, or those of the publisher, the editors and the reviewers. Any product that may be evaluated in this article, or claim that may be made by its manufacturer, is not guaranteed or endorsed by the publisher.
Acknowledgments
We would like to thank Dr. Jonathan Josephs-Spaulding for his linguistics assistance during the preparation of this manuscript.
Supplementary Material
The Supplementary Material for this article can be found online at: https://www.frontiersin.org/articles/10.3389/fcell.2021.740455/full#supplementary-material
Supplementary Figure 1 | Genome instability and immune analysis of high- and low-risk groups. (A) Association between somatic mutation count and risk score. Comparison of tumor mutational burden (B), microsatellite instability (C), expression and mutation rate of four DNA mismatch repair protein genes (MLH1, MSH2, MSH6, and PMS2) (D), and expression of six immune checkpoint-related genes (CD274 (PD-L1), CD80, PDCD1 (PD-1), PDCD1LG2 (PD-L2), CD86, CTLA4) (E) between the two groups using the Mann-Whitney U test. Comparison of immune cell infiltration (F) and immune functions (G) of the two groups using single-sample gene set enrichment analysis (ssGSEA). ∗p < 0.05; ∗∗p < 0.01; and ∗∗∗p < 0.001. (H) Comparison of the mutation rate of POLE between the two groups using the Mann-Whitney U-test. (I) Comparison of BRAF mutation rates. (J) Survival analysis among BRAF mutated/high-risk, BRAF mutated/low-risk, BRAF wild/high-risk, BRAF wild/low-risk groups by Kaplan-Meier curve and log-rank test.
Supplementary Figure 2 | Supplemental gene correlation analysis of the three lncRNAs (BOLA3-AS1, AC124067.4, and AL161772.1) and four target mRNAs (NINL, SALL4, TNFSF11, and PHYHIPL). (A) The association between the expression of seven genes and genome instability and immune-related genes (PDCD1, CD274, PDCD1LG2, CTLA4, CD80, CD86, MSH2, MLH1, PMS2, MSH6, NOARD, GUARDIN) by Spearman’s correlation. (B) Correlation between the expression of each miRNA and its paired lncRNAs based on ENCORI. (C) Correlation between the expression of each miRNA and its paired mRNAs based on ENCORI. ∗p < 0.05; ∗∗p < 0.01; and ∗∗∗p < 0.001.
Supplementary Figure 3 | The association between the three lncRNAs (BOLA3-AS1, AC124067.4, and AL161772.1), four target mRNAs (NINL, SALL4, TNFSF11, PHYHIPL), and each specific type of immune cell based on the CIBERSORT database.
Footnotes
References
Ashburner, M., Ball, C. A., Blake, J. A., Botstein, D., Butler, H., Cherry, J. M., et al. (2000). Gene ontology: tool for the unification of biology. Gene Ontol. Consort. Nat. Genet. 25, 25–29. doi: 10.1038/75556
Bhan, A., Soleimani, M., and Mandal, S. S. (2017). Long noncoding RNA and cancer: a new paradigm. Cancer Res. 77, 3965–3981. doi: 10.1158/0008-5472.Can-16-2634
Boland, C. R., and Goel, A. (2010). Microsatellite instability in colorectal cancer. Gastroenterology 138, 2073–2087.e3. doi: 10.1053/j.gastro.2009.12.064
Bradburn, M. J., Clark, T. G., Love, S. B., and Altman, D. G. (2003). Survival analysis part II: multivariate data analysis–an introduction to concepts and methods. Br. J. Cancer 89, 431–436. doi: 10.1038/sj.bjc.6601119
Burgeiro, A., Pereira, C. V., Carvalho, F. S., Pereira, G. C., Mollinedo, F., and Oliveira, P. J. (2013). Edelfosine and perifosine disrupt hepatic mitochondrial oxidative phosphorylation and induce the permeability transition. Mitochondrion 13, 25–35. doi: 10.1016/j.mito.2012.11.003
Cerami, E., Gao, J., Dogrusoz, U., Gross, B. E., Sumer, S. O., Aksoy, B. A., et al. (2012). The cBio cancer genomics portal: an open platform for exploring multidimensional cancer genomics data. Cancer Dis. 2, 401–404. doi: 10.1158/2159-8290.Cd-12-0095
Chen, J., Shishkin, A. A., Zhu, X., Kadri, S., Maza, I., Guttman, M., et al. (2016). Evolutionary analysis across mammals reveals distinct classes of long non-coding RNAs. Genome Biol. 17:19. doi: 10.1186/s13059-016-0880-9
Dagogo-Jack, I., and Shaw, A. T. (2018). Tumour heterogeneity and resistance to cancer therapies. Nat. Rev. Clin. Oncol. 15, 81–94. doi: 10.1038/nrclinonc.2017.166
de Witte, N. V., Stoppani, A. O., and Dubin, M. (2004). 2-Phenyl-beta-lapachone can affect mitochondrial function by redox cycling mediated oxidation. Arch. Biochem. Biophys. 432, 129–135. doi: 10.1016/j.abb.2004.09.020
Dudley, J. C., Lin, M. T., Le, D. T., and Eshleman, J. R. (2016). Microsatellite instability as a biomarker for PD-1 blockade. Clin. Cancer Res. 22, 813–820. doi: 10.1158/1078-0432.Ccr-15-1678
Fatica, A., and Bozzoni, I. (2014). Long non-coding RNAs: new players in cell differentiation and development. Nat. Rev. Genet. 15, 7–21.
Ganesh, K., Stadler, Z. K., Cercek, A., Mendelsohn, R. B., Shia, J., Segal, N. H., et al. (2019). Immunotherapy in colorectal cancer: rationale, challenges and potential. Nat. Rev. Gastroenterol. Hepatol. 16, 361–375. doi: 10.1038/s41575-019-0126-x
Garrido-Castro, A. C., Lin, N. U., and Polyak, K. (2019). Insights into molecular classifications of triple-negative breast cancer: improving patient selection for treatment. Cancer Dis. 9, 176–198. doi: 10.1158/2159-8290.Cd-18-1177
Goyal, B., Yadav, S. R. M., Awasthee, N., Gupta, S., Kunnumakkara, A. B., and Gupta, S. C. (2021). Diagnostic, prognostic, and therapeutic significance of long non-coding RNA MALAT1 in cancer. Biochim. et Biophy. Acta Rev. Cancer 1875:188502. doi: 10.1016/j.bbcan.2021.188502
Hammadi, S. A., Almarzooqi, S., Abdul-Kader, H. M., Saraswathiamma, D., and Souid, A. K. (2015). The PI3Kδ inhibitor idelalisib suppresses liver and lung cellular respiration. Int. J. Physiol. Pathophysiol. Pharmacol. 7, 115–125.
Hanahan, D., and Weinberg, R. A. (2011). Hallmarks of cancer: the next generation. Cell 144, 646–674. doi: 10.1016/j.cell.2011.02.013
Hu, F., Wang, Q., Yang, Z., Zhang, Z., and Liu, X. (2020). Network-based identification of biomarkers for colon adenocarcinoma. BMC Cancer 20:668. doi: 10.1186/s12885-020-07157-w
Hu, W. L., Jin, L., Xu, A., Wang, Y. F., Thorne, R. F., Zhang, X. D., et al. (2018). GUARDIN is a p53-responsive long non-coding RNA that is essential for genomic stability. Nat. Cell. Biol. 20, 492–502. doi: 10.1038/s41556-018-0066-7
Hung, T., Wang, Y., Lin, M. F., Koegel, A. K., Kotake, Y., Grant, G. D., et al. (2011). Extensive and coordinated transcription of noncoding RNAs within cell-cycle promoters. Nat. Genet. 43, 621–629. doi: 10.1038/ng.848
Jang, H., Yang, J., Lee, E., and Cheong, J. H. (2015). Metabolism in embryonic and cancer stemness. Arch. Pharm. Res. 38, 381–388. doi: 10.1007/s12272-015-0558-y
Jin, L., Li, C., Liu, T., and Wang, L. (2020). A potential prognostic prediction model of colon adenocarcinoma with recurrence based on prognostic lncRNA signatures. Hum. Genom. 14:24. doi: 10.1186/s40246-020-00270-8
Kanduri, C. (2016). Long noncoding RNAs: Lessons from genomic imprinting. Biochim. et Biophys. Acta (BBA) Gene Regulatory Mech. 1859, 102–111.
Kanehisa, M., and Goto, S. (2000). KEGG: kyoto encyclopedia of genes and genomes. Nucleic Acids Res. 28, 27–30. doi: 10.1093/nar/28.1.27
Koch, L. (2017). The driving force of cancer evolution. Nat. Rev. Genet. 18:703. doi: 10.1038/nrg.2017.95
Li, J. H., Liu, S., Zhou, H., Qu, L. H., and Yang, J. H. (2014). starBase v2.0: decoding miRNA-ceRNA, miRNA-ncRNA and protein-RNA interaction networks from large-scale CLIP-Seq data. Nucleic Acids Res. 42, D92–D97. doi: 10.1093/nar/gkt1248
Li, Y., Jiang, T., Zhou, W., Li, J., Li, X., Wang, Q., et al. (2020). Pan-cancer characterization of immune-related lncRNAs identifies potential oncogenic biomarkers. Nat. Commun. 11:1000. doi: 10.1038/s41467-020-14802-2
Li, Z., Wang, D., and Yin, H. (2020). A seven immune-related lncRNA signature predicts the survival of patients with colon adenocarcinoma. Am. J. Trans. Res. 12, 7060–7078.∗b-Z
Ma, Y., Cui, W., Yang, J., Qu, J., Di, C., Amin, H. M., et al. (2006). SALL4, a novel oncogene, is constitutively expressed in human acute myeloid leukemia (AML) and induces AML in transgenic mice. Blood 108, 2726–2735. doi: 10.1182/blood-2006-02-001594
Malta, T. M., Sokolov, A., Gentles, A. J., Burzykowski, T., Poisson, L., Weinstein, J. N., et al. (2018). Machine learning identifies stemness features associated with oncogenic dedifferentiation. Cell 173, 338–354.e15.
Miettinen, M., Wang, Z., McCue, P. A., Sarlomo-Rikala, M., Rys, J., Biernat, W., et al. (2014). SALL4 expression in germ cell and non-germ cell tumors: a systematic immunohistochemical study of 3215 cases. Am. J. Surg. Pathol. 38, 410–420. doi: 10.1097/pas.0000000000000116
Morel, A. P., Ginestier, C., Pommier, R. M., Cabaud, O., Ruiz, E., Wicinski, J., et al. (2017). A stemness-related ZEB1-MSRB3 axis governs cellular pliancy and breast cancer genome stability. Nat. Med. 23, 568–578. doi: 10.1038/nm.4323
Munschauer, M., Nguyen, C. T., Sirokman, K., Hartigan, C. R., Hogstrom, L., Engreitz, J. M., et al. (2018). The NORAD lncRNA assembles a topoisomerase complex critical for genome stability. Nature 561, 132–136. doi: 10.1038/s41586-018-0453-z
Newman, A. M., Steen, C. B., Liu, C. L., Gentles, A. J., Chaudhuri, A. A., Scherer, F., et al. (2019). Determining cell type abundance and expression from bulk tissues with digital cytometry. Nat. Biotechnol. 37, 773–782. doi: 10.1038/s41587-019-0114-2
Qin, S., Xu, L., Yi, M., Yu, S., Wu, K., and Luo, S. (2019). Novel immune checkpoint targets: moving beyond PD-1 and CTLA-4. Mol. Cancer 18:155. doi: 10.1186/s12943-019-1091-2
R Core Team (2013). R: A Language and Environment for Statistical Computing. Vienna: R Foundation for Statistical Computing.
Sansregret, L., Vanhaesebroeck, B., and Swanton, C. (2018). Determinants and clinical implications of chromosomal instability in cancer. Nat. Rev. Clin. Oncol. 15, 139–150. doi: 10.1038/nrclinonc.2017.198
Sanz-Garcia, E., Argiles, G., Elez, E., and Tabernero, J. (2017). BRAF mutant colorectal cancer: prognosis, treatment, and new perspectives. Ann. Oncol. Off. J. Eur. Soc. Med. Oncol. 28, 2648–2657. doi: 10.1093/annonc/mdx401
Schrock, A. B., Ouyang, C., Sandhu, J., Sokol, E., Jin, D., Ross, J. S., et al. (2019). Tumor mutational burden is predictive of response to immune checkpoint inhibitors in MSI-high metastatic colorectal cancer. Ann. Oncol. 30, 1096–1103. doi: 10.1093/annonc/mdz134
Shannon, P., Markiel, A., Ozier, O., Baliga, N. S., Wang, J. T., Ramage, D., et al. (2003). Cytoscape: a software environment for integrated models of biomolecular interaction networks. Genome Res. 13, 2498–2504. doi: 10.1101/gr.1239303
Su, M., Wang, H., Wang, W., Wang, Y., Ouyang, L., Pan, C., et al. (2018). LncRNAs in DNA damage response and repair in cancer cells. Acta Biochim. et Biophys. Sin. 50, 433–439. doi: 10.1093/abbs/gmy022
Tan, J. L., Li, F., Yeo, J. Z., Yong, K. J., Bassal, M. A., Ng, G. H., et al. (2019). New high-throughput screening identifies compounds that reduce viability specifically in liver cancer cells that express high levels of SALL4 by inhibiting oxidative phosphorylation. Gastroenterology 157, 1615–1629.e17. doi: 10.1053/j.gastro.2019.08.022
Thorsson, V., Gibbs, D. L., Brown, S. D., Wolf, D., Bortone, D. S., Yang, T.-H. O., et al. (2018). The immune landscape of cancer. Immunity 48, 812–830.e14.
Wang, F., Zhao, Q., Wang, Y. N., Jin, Y., He, M. M., Liu, Z. X., et al. (2019). Evaluation of POLE and POLD1 mutations as biomarkers for immunotherapy outcomes across multiple cancer types. JAMA Oncol. 5, 1504–1506. doi: 10.1001/jamaoncol.2019.2963
Yin, T., Zhao, D., and Yao, S. (2021). Identification of a genome instability-associated LncRNA signature for prognosis prediction in colon cancer. Front. Genet. 12:679150. doi: 10.3389/fgene.2021.679150
Yoshihara, K., Shahmoradgoli, M., Martínez, E., Vegesna, R., Kim, H., Torres-Garcia, W., et al. (2013). Inferring tumour purity and stromal and immune cell admixture from expression data. Nat. Commun. 4:2612. doi: 10.1038/ncomms3612
Keywords: genome instability, lncRNA, ceRNA, immune checkpoint inhibitor, MSI, tumor heterogeneity, colon adenocarcinoma
Citation: Ren Z, Wang Z, Gu D, Ma H, Zhu Y, Cai M and Zhang J (2021) Genome Instability and Long Noncoding RNA Reveal Biomarkers for Immunotherapy and Prognosis and Novel Competing Endogenous RNA Mechanism in Colon Adenocarcinoma. Front. Cell Dev. Biol. 9:740455. doi: 10.3389/fcell.2021.740455
Received: 13 July 2021; Accepted: 16 September 2021;
Published: 20 October 2021.
Edited by:
Na Luo, Nankai University, ChinaReviewed by:
Simin Li, Southern Medical University, ChinaSheik Pran Babu Sardar Pasha, University of California, Davis, United States
Copyright © 2021 Ren, Wang, Gu, Ma, Zhu, Cai and Zhang. This is an open-access article distributed under the terms of the Creative Commons Attribution License (CC BY). The use, distribution or reproduction in other forums is permitted, provided the original author(s) and the copyright owner(s) are credited and that the original publication in this journal is cited, in accordance with accepted academic practice. No use, distribution or reproduction is permitted which does not comply with these terms.
*Correspondence: Menghua Cai, bWVuZ2h1YWNhaUBpYm1zLnB1bWMuZWR1LmNu; Jianmin Zhang, anpoYW5nNDJAMTYzLmNvbQ==
†These authors have contributed equally to this work and share first authorship