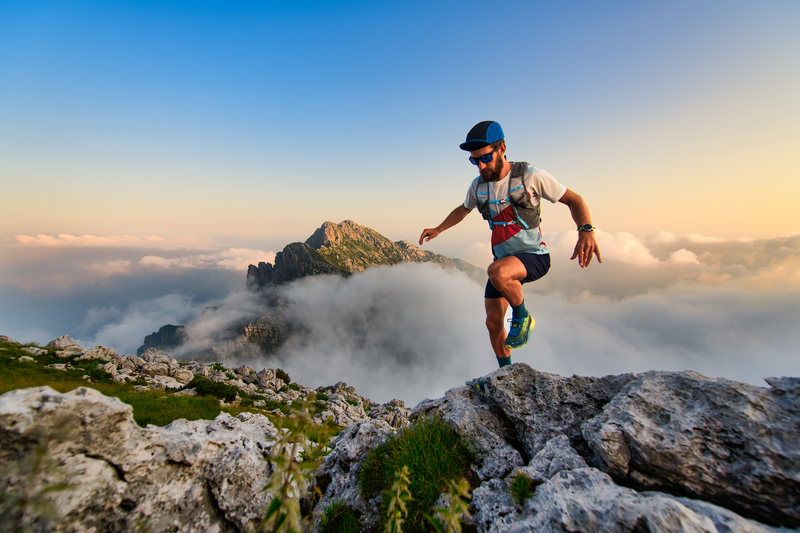
95% of researchers rate our articles as excellent or good
Learn more about the work of our research integrity team to safeguard the quality of each article we publish.
Find out more
REVIEW article
Front. Cell Dev. Biol. , 20 January 2022
Sec. Molecular and Cellular Pathology
Volume 9 - 2021 | https://doi.org/10.3389/fcell.2021.738838
This article is part of the Research Topic Advances in Diagnostics and Treatment of Functional Neurological Disorders: Neurogenomics, Neuromodulation and Machine-Learning View all 26 articles
Depression, a neurological disorder, is a universally common and debilitating illness where social and economic issues could also become one of its etiologic factors. From a global perspective, it is the fourth leading cause of long-term disability in human beings. For centuries, natural products have proven their true potential to combat various diseases and disorders, including depression and its associated ailments. Translational informatics applies informatics models at molecular, imaging, individual, and population levels to promote the translation of basic research to clinical applications. The present review summarizes natural-antidepressant-based translational informatics studies and addresses challenges and opportunities for future research in the field.
Depression is a neurological disorder commonly characterized by emotional and physical health, cognitive abilities, behavioral and sleep patterns affecting populations from all age groups globally (Wang et al., 2007; James et al., 2018). Amongst the major contributing factors are the patient’s family and medical history, traumas in early childhood, the structure of the brain, and drug abuse (Wang et al., 2007; James et al., 2018). At present, the medication for depression management largely relies on the chemical-based drugs that are categorized into selective serotonin reuptake inhibitors (SSRIs), serotonin and norepinephrine reuptake inhibitors (SNRIs), tricyclic antidepressants (TCAs), tetracyclic antidepressants, dopamine reuptake blockers, monoamine oxidase inhibitors (MAOIs), 5-HT receptor (5-HT1A, 5-HT2, and 5-HT3) and noradrenergic antagonists (Alvano and Zieher, 2020). The commercially available anti-depressants include citalopram, fluoxetine (SSRIs), desvenlafaxine, duloxetine (SNRIs), amitriptyline (TCAs), maprotiline (tetracyclic anti-depressants), isocarboxazid (MAOI), bupropion (dopamine reuptake blockers), vilazodone, nefazodone, and vortioxetine (5-HT1A, 5-HT2, and 5-HT3 receptor antagonists), and mirtazapine (noradrenergic antagonist) (Anonymous, 2000; Milev et al., 2016), which are accompanied by potential side-effects. Precisely, these vary from common side-effects (nausea, drowsiness, fatigue, constipation, dry mouth, weight gain, trouble sleeping, nervousness, tremors, and sexual problems) to serious health complications (low blood pressure, irregular heartbeat, seizures) (Ferguson, 2001). It is, therefore, imperative to venture into the natural products-based therapeutic domains.
Plant and natural products-based traditional medicine provides the foundation for numerous commercial drugs and has played a significant role in addressing global primary health requirements, especially, in developing countries (Duke et al., 1993; UNESCO World Decade for Cultural Development, and Organization W.H, 1996; Cragg et al., 1997; Newman et al., 2003; OrganizationW.H, 2013; Jyoti and Kumar., 2019; Mukhtar and Singh, 2020; Newman and Cragg, 2020; Atanasov et al., 2021; Dangar and Patel, 2021; Sathya and Arumugam, 2021). These natural products are isolated from various sources that include animals, fungi, plants, and microbes (Gunatilaka, 2006; Cragg and Newman, 2013; Genilloud, 2014; Atanasov et al., 2015; Katz and Baltz, 2016; Newman and Cragg, 2016; Futamura et al., 2017; Ikeda, 2017; Baltz, 2019; Singla et al., 2020; Apoorva et al., 2021; Bansal et al., 2021; Swarnkar et al., 2021). Unlike the chemical commercial drugs, laboratory synthesis of natural products with a heterogeneous composition and unique structures is an arduous task, albeit, enhanced stability and efficacy are reported for them. Moreover, there is often no evidence of microbial resistance, major toxic or side-effects for natural products-based therapeutics, and formulations (Moore et al., 2017). Essentially, these natural products include plant metabolic extracts and secondary metabolites extracted from different medicinal plants. Together, these are reported to demonstrate neuroprotective effects and reduce the risk of neurodegenerative diseases that strengthen their candidature as potential neuroprotective agents (Farahani et al., 2015; Fajemiroye et al., 2016; Martins, 2018; Bhandari et al., 2019). In light of the above facts, ongoing research efforts for depression management are focused on developing natural products-based anti-depressants.
In the recent past, tremendous progress in the interdisciplinary fields of medicinal science and computer-aided high throughput screening of therapeutic targets has accelerated the ominous process of drug discovery (Singh et al., 2009; Igoli et al., 2014; Singla, 2014; Khan et al., 2020). For this, various therapeutic target databases, models, and tools have been constructed that are essential for the management of vast clinical data (Chen et al., 2020; Liu et al., 2020; Yan et al., 2020; Chen et al., 2021; He et al., 2021). Together, these pave the way to the modern era of drug discovery and “big data,” with a foundation of five pillars,“ namelyValue,” “Variety,” “Velocity,” “Veracity,” and “Volume” (Dhingra and Kumar, 2007). The preclinical and clinical data collected from patients suffering from depression along with the data procured from healthy individuals make up the big depression data. This big data can be utilized for future approaches to data-driven medicine for depression. Figure 1 illustrates the 5 V s of big data characterization for depression. Computer-Aided Drug Discovery (CADD) based on the “big data,” surpasses the traditional methods of drug discovery that rely on ligand and structure-based drug designing tools (Sairam et al., 2002; Hazra et al., 2012; Joon et al., 2021). Howbeit, this demands a standardized multi-tiered data integration with robust algorithms for mining, structuring, and analysis of the accumulated clinical data (Shen et al., 2020; Miao et al., 2021). Also, this is crucial for assessing the precision of drug-target interactions generated by molecular simulation studies. Further, these contemporary drug discovery databases are expected to have ample data storage capacity with proper sources to ascertain the actual data density for the discovery of novel drugs and their targets. Moreover, the appropriate utilization of these modernized computational approaches is crucial for novel drug discovery.
Even though much research has been done on natural product-based therapeutics that strengthen their credibility in the treatment of myriad ailments, the translation of data obtained from basic research to clinical application is still a challenge to the scientific community (Singla and Dubey, 2019; Singla et al., 2021a; Singla et al., 2021b; Singla et al., 2021c; Madaan et al., 2021). With a focus on the antidepressant application of these natural products, here, we propose a paradigm of the informatics-driven research model and summarize the application of informatics models at different levels for augmenting translational research. Since the studies on the application of data models, Bioinformatics, imaging informatics, medical informatics, and health informatics in natural products-based drug discovery is scarce, this review will illuminate developmental insights for future novel translational informatics-based research directions in the field.
Depression pathogenesis involves multiple complex molecular mechanisms (Figure 2). To date, two pathways, including mitogen-activated protein kinase signaling (MAPK) and cyclic adenosine phosphate signaling (cAMP), have been widely reported to be associated with depression development, which has attracted close attention in antidepressant research (Duman and Voleti, 2012).
The Ras-MAPK signaling pathway includes two key kinases, namely extracellular signal-regulated kinase (ERK) and MAP/ERK kinase (MEK). ERK is typically activated by the phosphorylation of various growth factors. The activated ERK will then enter the nucleus and regulate a bunch of transcription factors, promoting specific genes’ transcription and expression. ERK signaling is regulated by protein kinase A (PKA) and protein kinase C (PKC), the activators of which can activate the ERK 1/2 in the hippocampus (Roberson et al., 1999). Accumulating pieces of evidence have demonstrated that the decreased activity of ERK signaling is one of the contributors to depression. Data from studies on depressive suicide victims showed reduced activity of PKA, PKC, and adenylate cyclase (AC) (Dwivedi et al., 2004). Duric et al. sequenced the hippocampus tissues from major depressive disorder (MDD) patients and noticed a significantly elevated expression of MKP1, which is a suppressor for ERK and MEK (Duric et al., 2010). Moreover, Dwivedi et al. revealed the decreased catalytic and phosphorylation activity of ERK 1/2 via direct examination of the expression level of ERK 1/2 in the brain tissues from the suicide victims suffering from depression (Dwivedi et al., 2001). They also noticed the downregulation of Ras, which is an upstream regulator of ERK, and the interaction between Ras and MEK1 is limited as well (Dwivedi et al., 2009). Besides, MAPK suppressors like protein tyrosine phosphatase receptor type R (PTPRR) are also engaged in the ERK-involved depression mechanisms. Overexpression of PTPRR in mice led to their susceptibility to depression (Li et al., 2016). Taken together, ERK signaling plays a crucial role in enhancing neuron plasticity and promoting the release of neural growth factors. The general mechanisms of ERK signaling in depression development are due to its inactivation or suppression. Studies from a system level, however, should be addressed to further explore the landscape of ERK-engaged depression pathogenesis.
The cAMP signaling, or cAMP/AC/PKA signaling, is heavily involved in emotion regulation. A general route of this pathway starts from the activation of AC, which will result in the catalysis of ATP to cAMP, followed by the activation of PKA. The activated PKA will then phosphorylate the cAMP response element-binding protein (CREB) and finally regulate the gene transcription. Studies have noticed that the promotion of cAMP signaling may have antidepressant effects. For instance, Data from Jiang et al. showed that the immobility time of rats was significantly reduced by a PKA agonist named 8-BR-camp (Jang et al., 2018). Nico et al. used a cAMP analog that inhibits PKA but not cAMP and revealed that besides the activation of PKA, the elevation of cAMP can also promote the activity of this signaling pathway and enhance the antidepressant effects (Liebenberg et al., 2011). In addition, Wang et al. also showed similar results. By injection of Raleigh Alvin (rolipram), which is an inhibitor for phosphoric acid lipase 2–4 (PDE4) that can prevent the hydrolysis of cAMP, the researchers found the increased level of cAMP and phosphorylated CREB, and reduce of immobile time of the mice (Wang et al., 2019). Notably, the cAMP and MAPK signaling partially share the same proteins in depression development. There may be interactions between these two pathways, the relationship of which should be paid more attention to, especially for those antidepressant relevant research.
The traditional system of medicine is the bedrock for several commercial drugs for depression and is based on natural products from various sources (Baird-Lambert et al., 1982; England, 1998; Hu et al., 2002; Hedner et al., 2006; Kochanowska et al., 2008; Zhao et al., 2016; Kochanowska-Karamyan et al., 2020; Singla, 2021). There is substantial evidence on the antidepressant activity of metabolic extracts and metabolites isolated from various medicinal plants (Farahani et al., 2015). The metabolic extracts are derived from distinct plant parts, such as leaves, flowers, roots, fruits (powdered or unripe), stem bark, bulb (powdered), whole plant, seed, petal, stigma, rhizome, hypocotyls, and etc. Reportedly, the plant secondary metabolites with anti-depression activity belong to different classes of phytocompounds that mainly comprise alkaloids, flavonoids, furocoumarins, glycosides, polyphenols, saponins, triterpenoids, and xanthones (Farahani et al., 2015). Together, these execute the anti-depression activity or neuroprotective effects through different mechanisms that target neurological signaling pathways or molecules responsible for depressive disorders (Farahani et al., 2015). The antidepressant properties of some important natural products are discussed below.
The methanolic extracts of Asparagus racemosus Willd. (roots) demonstrated in vivo antidepressant effects via MAO (Monoamine oxidases: MAO-A and MAO-B) inhibitory activity and dopaminergic (D2), serotonergic, GABAergic (Gamma-aminobutyric acid), adrenergic (α1), and noradrenergic receptor system interactions (Dhingra and Kumar, 2007; Singh et al., 2009). Similarly, the whole plant extracts of Bacopa monnieri (L.) Wettst. exerted in vivo antidepressant effects mainly through MAO (Monoamine oxidases: MAO-A and MAO-B) inhibitory activity and dopaminergic (D2), noradrenergic, and serotonergic receptor system interactions (Sairam et al., 2002; Maity et al., 2011; Hazra et al., 2012; Singh et al., 2014; Girish et al., 2016). Further, the fruit (methanolic) and seed (aqueous) extracts of Benincasa hispida (Thunb.) Cogn. exhibited MAO-A enzyme inhibition activity and dopaminergic (D2), serotonergic, GABAergic, adrenergic, and noradrenergic receptor system interactions (Dhingra and Joshi, 2012; Bharti and Singh, 2013). Phyllanthus emblica L. aqueous fruit extract showed MAO-A inhibitory activity and dopaminergic (D2), serotonergic, adrenergic receptor system interactions. This antidepressant activity could be due to the ascorbic acid, tannins, flavonoids, and polyphenols present in its fruit (Pemminati et al., 2010; Dhingra et al., 2011). Another plant extract that acts through MAO (MAO-A and MAO-B) activity inhibition is Glycyrrhiza glabra L. The aqueous, hydroalcoholic and ethanolic root extracts of G. glabra L. elevates norepinephrine (NE) and dopamine (DA) levels in the brain (Dhingra and Sharma, 2006; Chowdhury et al., 2011; Biswas et al., 2012). The petroleum ether stem extracts of Tinospora cordifolia (Willd.) Hook. f. and Thomson demonstrated antidepressant activity by MAO (MAO-A and MAO-B) enzyme inhibitory activity and dopaminergic (D2), serotonergic, adrenergic, and noradrenergic receptor interactions (Dhingra and Goyal, 2008a). The ethanolic and aqueous leaf extracts of Rhazya stricta Decne. demonstrated antidepressant effects through MAO-A inhibition (Ali B. et al., 1998; Ali B. H. et al., 1998). Momordica charantia L. relies on dopaminergic (D2), serotonergic (5-HT2), muscarinic, cholinergic, and noradrenergic (α1 and α2) receptor systems for its antidepressant activity (Ishola et al., 2013). Piato et al. and Siqueira et al. showed that Ptychopetalum olacoides Benth. (ethanolic root extract) possess antidepressant activity along with the noradrenergic (β) and dopamine (D1) receptor system interactions (Siqueira et al., 2004; Piato et al., 2009). Pedersen et al. demonstrated that Mondia whitei (Hook.f.) Skeels and Xysmalobium undulatum (L.) W.T.Aiton possess antidepressant activities, which could be attributed to their affinity for the Serotonin transporter (SERT) (Pedersen et al., 2008). Reportedly, the ethanolic root and rhizome extracts of Nardostachys jatamansi (D.Don) DC. showed anti-MAO (MAO-A and MAO-B) activity and GABAB receptor interaction (Dhingra and Goyal, 2008b; Karanth et al., 2012; Deepa et al., 2013). The root and rhizome extracts of (aqueous, dichloromethane, hydroethanolic, oil, and methanolic) Valeriana jatamansi Jones ex Roxb. were reported to have antidepressant effects via inhibition of nitric oxide (Subhan et al., 2010; Sah et al., 2011a; Sah et al., 2011b). Viana et al. demonstrated the antidepressant activity of Schisandra chinensis (Turcz.) Baill. (seeds) to be through its interaction with noradrenergic receptors (Viana et al., 2005). Rodrigues et al. showed that the hydroethanolic stem and leaf extracts of Siphocamphylus verticillatus possess antidepressant activity via synaptosomal inhibitory activity. These include [3H] dopamine [3H] noradrenaline, and [3H] serotonin uptake (Rodrigues et al., 2002). The uptake of serotonin and dopamine was also inhibited by the hydroethanolic bark extract of Trichilia catigua A. Juss. (Campos et al., 2005). Burdette et al. showed antidepressant activity of various extracts (aqueous, ethanol, and isopropanol) of Actaea racemosa L. It was reported to behave as a serotonin (5-HT1A, 5-HT1D, and 5-HT7) receptor agonist (Burdette et al., 2003). Figure 3 shows an interaction analysis map of metabolite extracts from various plant sources with the physiological biomarkers of depression. Except for Rhazya stricta Decne., Mondia whitei (Hook.f.) Skeels, Valeriana jatamansi Jones ex Roxb., Schisandra chinensis (Turcz.) Baill., and Xysmalobium undulatum (L.) W.T.Aiton, the rest all the metabolite extracts were documented to be multimodal in action for their antidepressant activity. To the surprise, all the natural sources belong to a single class (Magnoliopsida). Further, as per the covered data, the family Apocynaceae, Curcurbitaceae, and Caprifoliaceae were of special importance to explore natural antidepressants. In our previous literature study, we have observed that families like Solonaceae and Fabaceae, are important in yielding agents against Parkinson’s disease (Singla et al., 2021a).
FIGURE 3. Interaction analysis map to express association and relationship between class and family of natural sources with the physiological pathways related to depression.
Zhou et al. and Jin et al. demonstrated that antidepressant effects of Akebia trifoliata (Thunb.) Koidz. (ethanolic powdered fruit extract) are due to hederagenin (Supplementary Figure S1A), which has high norepinephrine transporter (NET), dopamine transporter (DAT), and serotonin (SERT) transporter affinity, and inhibitory activity (humans and rats) (Zhou et al., 2010; Jin et al., 2012). Likewise, several studies reported anti-NET, anti-DAT, and anti-SERT activities of alkaloids, namely, buphanamine (Supplementary Figure S1B) and buphanidrine (Supplementary Figure S1C), isolated from the ethanolic bulb extract of Boophone disticha (L.f.) Herb. (Nielsen et al., 2004; Pedersen et al., 2008; Gadaga et al., 2010). Quercetin (Supplementary Figure S1D) isolated from the aqueous powdered bulb extract of Allium cepa L. demonstrated MAO inhibitory activity. An increased metabolite to neurotransmitter ratio was observed in rat models of depression administered with quercetin (Sakakibara et al., 2014). Likewise, paeoniflorin (Supplementary Figure S1E) and albiflorin (Supplementary Figure S1F) isolated from the ethanolic root extract of Paeonia lactiflora Pall. exhibited MAO inhibitory activity. These glycosides also up-regulated the serotonergic systems (Mao Q.-Q. et al., 2008; Mao Q. et al., 2008; Qiu et al., 2013). Ren et al. showed that sarsasapogenin (Supplementary Figure S1G) isolated from Anemarrhena asphodeloides Bunge leaf extracts possessed MAO (MAO-A and MAO-B) inhibitory activity. It was also shown to interact with norepinephrine and serotonin (5-HT) receptor systems (Ren et al., 2006). MAO inhibitory activity was observed with piperine (Supplementary Figure S1H) and methylpiperate (Supplementary Figure S1I) isolated from the ethanolic fruit extract of Piper longum L. (Lee et al., 2005; Lee et al., 2008). Other examples include polygalatenoside A (Supplementary Figure S1J) and polygalatenoside B, YZ-50, and 3,6-disinapoyl sucrose (Supplementary Figure S1K) isolated from the root extracts of Polygala tenuifolia Willd. that caused NE-mediated MAO activity inhibition (Cheng et al., 2006; Hu et al., 2010; Hu et al., 2011). Isoliquiritin (Supplementary Figure S1L) and liquiritin (Supplementary Figure S1M) isolated from the aqueous root extracts of Glycyrrhiza uralensis Fisch. ex DC. demonstrated serotonin (enhanced 5-HT) and NE-mediated antidepressant activity in the mice model of depression (Wang W. et al., 2008; Zhao et al., 2008; Fan et al., 2012). 4-hydroxyisoleucine (Supplementary Figure S1N) isolated from the seed extract of Trigonella foenum-graecum L. exhibited serotonergic system-mediated antidepressant activity (Gaur et al., 2012). Various other metabolites, such as 1 F-fructofuranosylnystose (Supplementary Figure S1O), heptasaccharide (Supplementary Figure S2P), inulin-type hexasaccharide, nystose (Supplementary Figure S2Q), succinic acid (Supplementary Figure S2R) (aqueous root extracts of Gynochthodes officinalis (F.C.How) Razafim. and B. Bremer) (Cui et al., 1995; Zhang et al., 2002), neferine (Supplementary Figure S2S) (seed extracts of Nelumbo nucifera Gaertn) (Dhanarasu and Al-Hazimi, 2013), gingerol (Supplementary Figure S2T) and shogoal (hydromethanolic rhizome extract of Zingiber officinale Roscoe) (Pratap et al., 2012; Sibi and Meera, 2013) were demonstrated to be agonists for serotonin receptor (5-HT1A). Turmerone (Supplementary Figure S2U) (aqueous rhizome extract of Curcuma longa L.) (Yu et al., 2002; Liao et al., 2013) and gentiacaulein (Supplementary Figure S2V) (diethyl ether aerial extract of Gentiana acaulis L.) (Tomic et al., 2005) impeded MAO-A activity while desmethoxyyangonin (Supplementary Figure S2W) and pyrones (Piper methysticum G. Forst.) caused dopaminergic-dependent MAO-B inhibition in vivo (Baum et al., 1998; Uebelhack et al., 2007). Furocoumarins, namely, psoralen (Supplementary Figure S2X) and psoralidin (Supplementary Figure S2Y) isolated from the seed extract of Cullen corylifolium (L.) Medik. possessed serotonergic-dependent MAO inhibitory activity (Xu et al., 2008; Yi et al., 2008). Similar antidepressant activities were observed with rosiridin (Supplementary Figure S2Z) isolated from the aqueous, dichloromethane, and methanolic root extracts of Rhodiola rosea L. (Supplementary Figure S2) (van Diermen et al., 2009; Mannucci et al., 2012). Riparins (Riparin II (Supplementary Figure S2AA) and Riparin III (Supplementary Figure S2AB) (Sousa et al., 2004; Teixeira et al., 2013), lectins (Barauna et al., 2006), and rutin (Supplementary Figure S2AC) (Machado et al., 2007; Machado et al., 2008) isolated from the unripe fruit (ethanolic extract), seed, and stem and leaf (hexane and ethanolic extracts) of Aniba riparia (Nees) Mez, Canavalia brasiliensis Mart. ex Benth., and Schinus molle L. respectively were shown to interact with dopaminergic, noradrenergic, and serotonergic receptor systems. β-amyrin palmitate (Supplementary Figure S2AD) isolated from the methanolic leaf extracts of Lobelia inflata L. showed antidepressant activity via noradrenergic receptor system activation (Subarnas et al., 1992; Subarnas et al., 1993). Flavonoids, precisely, hyperoside (Supplementary Figure S2AE) and isoquercitrin (Supplementary Figure S2AF) isolated from the ethanolic leaf extracts of Apocynum venetum L. caused elevated hippocampus levels of NE and DA. Dopaminergic receptor system (D1 and D2) interactions were observed (Butterweck et al., 2001; Zheng et al., 2013). 1,8-cineole (Supplementary Figure S2AG), betulinic acid (Supplementary Figure S2AH), carnosol (Supplementary Figure S2AI), and ursolic acid (Supplementary Figure S2AJ) isolated from Salvia rosmarinus Spenn. (stem and leaf extracts) demonstrated antidepressant effect via dopamine receptor activation (Machado et al., 2012; Machado et al., 2013; Mukhtar et al., 2013; Singla et al., 2017). 2,4,5-trimethoxycinnamic acid (Supplementary Figure S2AK), apigenin (Supplementary Figure S3AL), and rosmarinic acid (Supplementary Figure S3AM) isolated from the leaves of Perilla frutescens (L.) Britton demonstrated dopaminergic system-dependent antidepressant activity (Nakazawa et al., 2003; Ito et al., 2008; Yi et al., 2013). Carvacrol (Supplementary Figure S3AN) (aromatic plant extract) caused a dopaminergic system-mediated antidepressant effect leading to elevated levels of serotonin (5-HT) and dopamine (Melo et al., 2011; Zotti et al., 2013). On the contrary, curcumin (Supplementary Figure S3AO) exerts its antidepressant activity via the serotonergic receptor system (5-HT1A/1B and 5-HT2C) causing an elevation in the serotonin (5-HT) levels (Wang R. et al., 2008; Kulkarni et al., 2008). The otherwise altered 5-HT1A mRNA (hippocampus) was also reversed in curcumin-treated mice models of depression (Xu et al., 2007). Resveratrol (Supplementary Figure S3AP) showed MAO inhibitory activities and increased dopamine, noradrenaline, and serotonin (5-HT) levels in rat models of depression (Xu et al., 2010; Yu et al., 2013). Crocin (Supplementary Figure S3AQ), kaempferol (Supplementary Figure S3AR), and safranal (Supplementary Figure S3AS) isolated from the petal and stigma extracts (aqueous and ethanolic extracts) of Crocus sativus L. demonstrated a potential antidepressant activity by inducing the release of brain dopamine and glutamine (Hosseinzadeh et al., 2004; Hosseinzadeh et al., 2007; Ettehadi et al., 2013). Forskolin (Supplementary Figure S3AT) isolated from Coleus hadiensis (Forssk.) A.J.Paton showed antidepressant activity by enhancing the availability of cAMP in the brain (Wachtel and Loschmann, 1986; Maeda et al., 1997). Ferulic acid (Supplementary Figure S3AU) increases CREB phosphorylation and mRNA levels of a brain-derived neurotropic factor in mice models of depression (Yabe et al., 2010). The scientific name of the medicinal plants was mentioned as per the universally accepted nomenclature, specified and recommended by the Ethnopharmacology team. So, the names specified in the manuscript will seems to be different from that of cited articles. To cross-check the nomenclature, refer Medicinal Plant Names Service (MPNS) https://mpns.science.kew.org/mpns-portal/and http://www.plantsoftheworldonline.org/. Data for Figures 3, 4 was collected manually by literature search using PubMed and Google Scholar. For Figure 3, the taxonomical class of the biological sources has been retrieved from the NCBI taxonomy browser. For Figure 4, the phytochemical class was mentioned as per the classification mentioned in PubChem, NCBI. Then all these data were transformed as per the Sankey Graph principles to convert into an interactive illustration. Figure 4 shows an interaction analysis map of various potential antidepressant phytochemicals or metabolites with the physiological biomarkers of depression. It has been observed that the majority of natural antidepressants fall under the category of carbohydrates, glycosides, phenols, polyphenolics, flavonoids, carboxylic acids, and terpenes. Figure 5 illustrates the molecular mechanisms mediated by the bioactives for depression management.
FIGURE 4. Interaction analysis map to express association and relationship between phytochemical classifications of antidepressant metabolites with the physiological pathways related to depression.
FIGURE 5. Illustration of the molecular mechanisms mediated by the bioactives for depression management.
Karamyan et al. demonstrated in vivo antidepressant activity for veranamine isolated from the marine sponge (Verongula rigida). Owing to its selective affinity towards sigma-1 and 5HT2B receptors, it could serve as a novel antidepressant drug candidate (Kochanowska-Karamyan et al., 2020). Similarly, barettin, 8,9 dihydrobarettin, gelliusines A and B, and sigma-conotoxin have been reported to possess selective affinity towards the serotonin receptors (England, 1998; Hedner et al., 2006). Further, Lambert et al. reported the MAO inhibitory activity for methylaplysinopsin isolated from Aplysinopsis reticulate (a sponge) (Baird-Lambert et al., 1982). The antagonist binding displacement activity at 5HT2A and 5HT2C receptors have been reported for the compounds isolated from Smenospongia aurea (a sponge) (Hu et al., 2002). There are some other marine natural products reported to possess promising antidepressant activity in vivo (Kochanowska et al., 2008). For example, potential in vivo antidepressant activity was reported for the total sterols and β-sitosterol isolated from Sargassum horneri (a brown seaweed). There occurred a significant increase in NE, 5-HT, and 5-HIAA (5-hydroxyindoleacetic acid) neurotransmitters (Zhao et al., 2016).
With the breakthrough technologies, such as omics and informatics, biomedical research has made significant strides (Canuel et al., 2014; McGuire et al., 2020). Since the collected biomedical information is huge, data management with highly organized databases is enormously important (Harel et al., 2011). Besides, such well-structured databases must be amenable to sharing and integration of the standardized and annotated stored data (Misra et al., 2019). Natural products comprise a spectrum of potential therapeutic compounds for myriad diseases. Owing to the spectacular diversity in natural products, the development of natural-products databases is essential (Mehbub et al., 2014; Atanasov et al., 2015; Cheesman et al., 2017). These databases, in turn, will broaden our horizon on the mechanistic insights of natural products or compounds on a particular disease target and reveal crucial clinical details for ‘precision medicine’. One such example is COCONUT, an acronym for MongoDB COlleCtion of Open Natural prodUcTs (https://coconut.naturalproducts.net) that comprise freely accessible natural products databases, albeit partially (Sorokina et al., 2021).
Based on the geographical location, the traditional medicinal system has various distinct branches with shared attributes. To name a few, these conventional medicinal systems include traditional Indian medicine, traditional Chinese medicine, and traditional Islamic medicine (Pan et al., 2014; Yuan et al., 2016). From a holistic perspective, the development of robust databases and knowledge bases is imperative for a systematic sharing and annotation of these traditional medicinal systems that encompass enormous information on natural products. This indeed is indispensable for an integrated evaluation and screening of natural products (Ikram et al., 2015). Over the years, endeavours have been undertaken to build compendious natural products databases that accommodate essential data on the natural products, their potential targets, and genetic interactions. HERB (http://herb.ac.cn) and SymMap (https://www.symmap.org/) are exemplary traditional Chinese medicine databases. HERB contains elementary information on herbs with their putative targets and genetic interaction mechanisms while SymMap is useful in mapping the disease symptoms disseminating appropriate prescriptions (Wu et al., 2019; Fang et al., 2021). Others in the category are the curated Indian Medical Plants, Phytochemistry And Therapeutics (IMPPAT) (https://cb.imsc.res.in/imppat), and Universal Natural Product Resource (UNaProd) (http://jafarilab.com/unaprod) databases that provide useful information on the nomenclature and medicinal applications of Indian and Iranian herbs, respectively (Mohanraj et al., 2018; Naghizadeh et al., 2020). Noteworthy, data on natural products contained within these traditional medicine databases may provide us with valuable conventional clinical and therapeutic anti-depression prescriptions. Howbeit, a real-time integrated data analysis is all-important for gaining newer clinical insights to the global scientific community dedicated to depression research.
In recent times, there has been a resurgence in interest in natural product-based drug discovery research (Gu et al., 2013; Laganà et al., 2019). However, this demands prompt, apt, and credible screening methods for natural products, followed by their isolation from the heterogeneous extracts and structural characterization to strengthen their therapeutic potential over alternate drug discovery processes. Additionally, these screening tools must be competent for the large-scale production of natural products-based therapeutic compounds (Butler, 2004). It is, therefore, incumbent on the global research community to develop robust and comprehensive natural products databases that comprise crucial information on the species source and quantitative pharmacological activity of all possible natural products, in addition to their structural details and qualitative pharmacological activity. Unfortunately, the existing general and specialized natural products databases disseminate experimental quantitative activity data for the few natural products contained within. The examples include SuperNatural (http://bioinformatics.charite.de/supernatural) (Banerjee et al., 2015), ZINC (http://zinc.docking.org/) (Irwin and Shoichet, 2005), TCM-ID (Traditional Chinese Medicine Information Database) (http://bidd.group/TCMID/) (Wang et al., 2005), TCM@Taiwan/iSMART (SysteMs Biology Associated Research with TCM) (http://ismart.cmu.edu.tw/) (Chang et al., 2011), TCMID (Traditional Chinese Medicines Integrated Database) (http://119.3.41.228:8000/tcmid/) (Huang L. et al., 2018), TCMSP (Traditional Chinese Medicine Systems Pharmacology Database and Analysis Platform) (https://old.tcmsp-e.com/tcmsp.php) (Ru et al., 2014), TM-MC (Northeast Asian traditional medicine) (http://informatics.kiom.re.kr/compound) (Kim et al., 2015), NuBBEDB (Nuclei of Bioassays, Ecophysiology and Biosynthesis of Natural Products Database) (https://nubbe.iq.unesp.br/portal/nubbedb.html) (Pilon et al., 2017), SANCDB (South African Natural Compounds Database) (https://sancdb.rubi.ru.ac.za/) (Hatherley et al., 2015), HIT (Herbal ingredients’ targets databases) (https://bio.tools/hit) (Ye et al., 2010), NPACT (Naturally Occurring Plant-based Anti-cancer Compound-Activity-Target database (http://crdd.osdd.net/raghava/npact/) (Mangal et al., 2013), and BioPhytMol (http://ab-openlab.csir.res.in/biophytmol/) (Sharma et al., 2014). To this end, numerous natural products databases were constructed to complement the existing databases with ample information on species sources and experimental quantitative activity for myriad natural products. These include NPASS (Natural Product Activity and Species Source) (http://bidd2.nus.edu.sg/NPASS/) (Zeng et al., 2018), NANPDB (Northern African Natural Products Database) (http://african-compounds.org/nanpdb/) (Ntie-Kang et al., 2017), and SuperNatural II (Super Natural database) (http://bioinformatics.charite.de/supernatural) (Banerjee et al., 2015). These provide an enhanced knowledge of the structural and physicochemical attributes for a large majority of natural compounds along with their toxicity class prediction, metabolic pathways, pharmacokinetics, biological activity, and related mechanisms, and vendor information (Banerjee et al., 2015; Ntie-Kang et al., 2017; Zeng et al., 2018). Besides, there exist some other natural product databases, such as, NPBS (Natural Products and Biological Sources) (http://www.organchem.csdb.cn/scdb/NPBS) that furnish vital information on the relationship between natural products and their sources (relational data) (Xu et al., 2020). Essentially, the biological source is linked to the natural products derived from it and vice versa. This database with a broader range of natural source species can be exploited to avoid the replication of isolation and characterization of established natural products (Xu et al., 2020). Other examples include CMNPD (Comprehensive Marine Natural Products Database) (https://www.cmnpd.org/) (Lyu et al., 2021) and PAMDB (Pseudomonas Aeruginosa Metabolome Database) (http://pseudomonas.umaryland.edu/) (Huang W. et al., 2018) that encompass data on marine natural products and the metabolic pathway diagrams and metabolomics on Pseudomonas aeruginosa, respectively. There are some others that include, PSC-db (http://pscdb.appsbio.utalca.cl) (Valdés-Jiménez et al., 2021), TeroKit (http://terokit.qmclab.com) (Zeng et al., 2020), MedPServer (http://bif.uohyd.ac.in/medserver) (Potshangbam et al., 2018), TriForC (http://bioinformatics.psb.ugent.be/triforc) (Miettinen et al., 2018), 3DMET (http://www.3dmet.dna.affrc.go.jp) (Maeda and Kondo, 2013), BiG-FAM (https://bigfam.bioinformatics.nl) (Kautsar et al., 2021), DEREP-NP (https://github.com/clzani/DE) (Zani and Carroll, 2017), TMDB (http://pcsb.ahau.edu.cn:8080/TCDB/index.jsp) (Yue et al., 2014). In all, these databases with an enormous wealth of information on natural products and their source species (biological sources) might be instrumental in augmenting the efforts directed towards screening of anti-depression therapeutics, drug discovery, and development. Table 1 outlines the databases comprising natural products-sharing of data and references as discussed above.
Computational tools based on multi-scale modeling (MSM) are explicitly efficient, robust, and dynamic in integrating data, testing hypotheses, and comprehensively illuminating the pathophysiological mechanisms underlying depression-related neurological disorders (Ramirez-Mahaluf et al., 2015; Shen et al., 2019). Collectively, these expedite diagnosis and therapy together with antidepressant target identification for drug development (Ramirez-Mahaluf et al., 2015). These putative antidepressant molecules can be explored to ascertain their candidature as a proficient therapeutic target or biomarker for depression disorders by employing amalgamated and synchronized network-based strategies (Zhang T.-T. et al., 2018; Wu et al., 2018). Over the past few years, deep learning has revolutionized the traditional target screening, which embarks on a new age of drug discovery (Schneider, 2017; Kraus, 2019; Kiriiri et al., 2020; Schaduangrat et al., 2020; Gupta et al., 2021; Paul et al., 2021; Singh et al., 2021). For instance, TripletRes and AlphaFold are globally acclaimed contemporary two-dimensional (2D) protein structure prediction tools of deep learning, which have spectacularly boosted the efficacy of classical drug discovery strategies (Kryshtafovych et al., 2019; Li et al., 2019; Senior et al., 2019). A consolidated yet thoughtful utilization of these modern computational tools will be decisive in exploring naturally occurring molecules for depression management and prognosis (Truax and Romo, 2020; Woo and Shenvi, 2021).
In general, the naturally occurring biomolecules are either isolated from microbial fauna or medicinal plants, which is an expensive and labour extensive long rigmarole (Sairam et al., 2002; Sarker and Nahar, 2012; Bucar et al., 2013; Zhang Q.-W. et al., 2018). These cumbersome isolation procedures are oftentimes undermined by inevitable constraints, such as the seasonal variations of plant growth, variations in microbial growth conditions (in the case of microbial biomolecules), the efficacy of the purification procedures employed, and low yields (Sairam et al., 2002; Sarker and Nahar, 2012; Bucar et al., 2013; Zhang Q.-W. et al., 2018). A combination of highly efficient isolation and purification techniques is, therefore, indispensable to obtain these natural products at reasonable yields. Even though there exist a few fully autonomous computational algorithms and tools, these are tarnished by major pitfalls. For instance, these are capable of accomplishing solo commands at a given time and are usually confined to comparatively simple molecular drug targets (Song et al., 2009; Prachayasittikul et al., 2015; Bharatam, 2021). A highly acclaimed synthesis route design tool for complex natural products is Chematica (Mikulak-Klucznik et al., 2020). With the innovation of Chematica, autonomous-computer-aided synthesis pathways are designed swiftly for myriad commercially important natural products and biologically active compounds of medicinal value. In contrast to the previous synthesis pathways, it requires fewer steps with incredible synthetic efficiency and cost-effectiveness evident from its laboratory performance (Klucznik et al., 2018).
It is worth discussing here, that the classical retro-synthesis technique for even simple organic molecules relied on the recursive or repetitive transformation into still smaller entities. This cumbersome task has been hastened by the advent of computer-based retro-synthesis. Quite dismally, these are in their infancy with disappointingly sluggish performance and quality-compromised outputs. Recently, these limitations have been overcome with the introduction of symbolic artificial intelligence (AI) and Monte Carlo tree search-guided revelation of retro-synthesis routes for diverse organic molecules (Segler et al., 2018). This state-of-the-art computer-aided retro-synthesis tool essentially consists of deep neural networks, viz. expansion policy, and filter networks integrated Monte Carlo tree search to allow a guided search with the prior selection of the propitious steps for synthesis route. Further, these deep learning tools are exceptionally swift in their output for diverse molecules over manually designed conventional heuristic methods that rely on extracted rules for synthesis route search. Also, these are well-trained, with an inbuilt knowledge on nearly all the reported organic reactions for myriad molecules as ascertained by a double-blind analysis (Segler et al., 2018).
This spectacular breakthrough in the field of computer-aided retro-synthesis might assist researchers in devising novel techniques to deduce feasible approaches for the optimal synthesis of molecular targets. Also, this does not require any prior knowledge or expertise regarding the existing strategies. Altogether, these contemporary computational tools, perhaps strategize the multi-step complex syntheses route designs for natural products, which are otherwise quite laborious and inefficacious.
The network-based approaches play a cardinal role in numerous scientific fields (Chandran et al., 2017; Guo et al., 2020). Amongst the various crucial applications is in the domain of biomedical sciences, where it assists in the evaluation of diverse systemic molecular interactions (Sonawane et al., 2019; Sheik Amamuddy et al., 2020; Wang et al., 2020). To perform investigations, such as assessing the effects of dysfunctional molecules in the system as a whole, hitherto biological networks were relied upon (Furlong, 2013; Somvanshi and Venkatesh, 2013; Altaf-Ul-Amin et al., 2014; Charitou et al., 2016; Hu et al., 2016; Caldera et al., 2017; Faeder et al., 2020; Silverman et al., 2020; Wang et al., 2020). The discovery of biomarkers together with a screening of putative drug molecules for complex diseases, including depression-related disorders can be accomplished using more utilitarian network-based applications. These include, but are not limited to co-expression, gene-gene, and protein-protein interaction network-based strategies (Khanin et al., 2011; Vella et al., 2017; Myers et al., 2019; Sun et al., 2019; Ovens et al., 2021).
With the innovation of genomics or genomic sequencing (DNA/RNA), the process of novel drug discovery is tremendously accelerated (Xia, 2017; Suwinski et al., 2019; McGuire et al., 2020). Further, genome sequencing was pioneering in introducing the concept of “Drug repurposing” for medically-approved drugs, which was a turning point in treating diverse ailments and a diminished economic burden for developing newer drugs for individual disease treatment (Emilien, 2000; Jarada et al., 2020; Nabirotchkin et al., 2020). Howbeit, their applicability in the therapeutic management of numerous disparate diseases was sceptical. This limitation is overcome by the contemporary and technologically advanced genomic techniques that sped the identification of specific disease-causing key genetic factors or anomalies in an individual, in particular (Dryja, 1997; Lander et al., 2001; Hasin et al., 2017; Horton and Lucassen, 2019). This “Precision medicine” furnishes a mechanistic insight into an individual patient’s disease (customized disease module) and unveils principal disease contributing elements. Eventually, these disease mechanisms could be targeted with “precision” for high-end personalized treatment strategies (Dugger et al., 2017; König et al., 2017). In this direction, a drug repurposing, Genome-wide Positioning Systems network (GPSnet) algorithm that targets genomic sequence profile-derived disease modules from a single patient was developed. These genomic sequencing profiles allow protein-protein interaction mapping for human diseases that reveal the key molecular players in disease pathophysiology. This, in turn, is pivotal in strategizing and prioritizing the selection of repurposed drugs for an effective and customized treatment regimen. Further, disease modules based on the predictions from GPSnet (https://www.gpsnet.com.br/) could accurately predict responses and strategize usage for a reasonable number of approved chemotherapeutic drugs for approximately five thousand cancer patients on prior in silico investigations (transcriptomic profiling and exome sequencing) (Cheng et al., 2019). As a proof of concept, ouabain (cardiac drug) demonstrated an antitumor potency via anti-HIF1α/LEO1 activity in vitro. This in silico tool could perform the dual role of specifically identifying a disease module and repurposing the approved drugs with precise indications for medical applications as observed in the case of cancer. These findings strengthen the candidature of GPSnet as a drug repurposing scaffold for constructing an effective therapeutic screen for various drugs, including identification of naturally occurring potential drug molecules, their synthesis, and precise administration in depression therapies (Cheng et al., 2019).
There is accumulating evidence on the utility of network theory in evaluating the therapeutic potential of natural products in health management. For instance, association network-based novel techniques were developed for scrutinizing and discerning microbes that synthesize biomolecules from those that participate in the biological transformation of natural (or pharmaceutical) products within the human host. Specifically, these association networks rely on the concomitant probing of metabolomics and metagenomics data on diversified human microbial fauna. As a step further, the intended molecules were mapped to their respective clade and finally to the phylogenetic tree to identify the microbial species participating in their synthesis or biotransformation (Cao et al., 2019). As a futuristic approach, these might supersede the available time and cost-extensive characterization techniques, which are solely dependent on methods of microbial cultivation. Further, this limits their efficiency of species identification that partakes in the synthesis or the transformation of the vivid small molecular wealth found within the host system. The feasibility study of metagenomics and metabolomics association networks unveiled the corynomycolenic acid-producing microbial genes amid the human cystic fibrosis microbiome isolates. Additionally, these accurately delineated the associations of quinolone signals (Pseudomonas), phevalin, and tyrvalin to their respective clusters of biosynthetic genes (Cao et al., 2019).
In another study, the mechanisms that govern natural products and synthetic chemotherapeutic synergism were investigated. Accordingly, the information on compounds and their targets was retrieved from the public domain that aided in assessing the targetable space for respective natural products. In the context of the network, their evaluation accentuated the notion that these natural products exhibit groupings of targets in the family, which are disparate as well as share commonness with a synthetic chemotherapeutic. Conclusively, these rational pieces of evidence emphasize the chemotherapeutic efficiency of natural products for developing complementary and combinatorial novel chemotherapies with synthetic anticancer drugs (Chamberlin et al., 2019). Likewise, multi-potent natural chemotherapeutics were screened from Clerodendrum indicum and C. serratum using network pharmacology (Gogoi et al., 2017). By employing an integrative approach, the anticancer effects of a combination of drugs against various cancer targets were determined. Amongst the predicted natural anti-cancer compounds, apigenin 7-glucoside and hispidulin could bind efficiently to reasonable chemotherapeutic targets (seventeen). These findings are crucial milestones in the field of novel anti-cancer drug discovery (Gogoi et al., 2017).
Whilst shreds of evidence on network-based approaches for anti-depression natural product discovery are lacking, the above findings might serve as important milestones in bridging the gap in the identification of natural products and developing these as potent anti-depression therapeutics.
With a global technological advancement in the healthcare sector, depression management strategies now include translational informatics, which has firmly integrated clinical data with basic research (Smith et al., 2007; Unützer and Park, 2012; Tenenbaum, 2016; Robinson, 2018; Kraus et al., 2019). Also, these aim to strive at “precision medicine” for depression care (Nierenberg, 2012; Menke, 2018; Serretti, 2018). Noteworthy, these substantially rely on multi-tiered databases comprising enormous yet, segregated data from various resources, such as clinical, environmental, lifestyle, and natural products data (Herland et al., 2014; Shameer et al., 2017; Seyhan and Carini, 2019). These, in turn, are essential for developing a well-trained AI system with ample inbuilt knowledge. It is contemplated that the AI system, so developed, can be exploited for performing dual tasks of exploring potential natural therapeutic candidates commenced by recommendation of precise drugs for depression management at first. Secondly, the real-time medical status of a single patient can be monitored based on the instantaneous physiological information together with an automated AI system alarm upon encountering health aberrations. Furthermore, healthcare counselling and related advice can be imparted to patients by day-to-day evaluation using cloud computing. Unfortunately, the AI system for depression management is still in its infancy struggling with issues of privacy and confidentiality of patient information amongst myriad other challenges (Grist et al., 2018; Graham et al., 2019; Hategan et al., 2019; Huckvale et al., 2019; Romano and Tatonetti, 2019; Tran et al., 2019; Bickman, 2020; Ke et al., 2020; Mennen et al., 2021).
Figure 6 illustrates the futuristic translational informatics-based model for depression management using natural antidepressants. Presently, the databases on natural products store data on the promising candidates for myriad diseases. With the increasing research data on depression-related therapeutics based on natural products and the complexity associated with the disease, it is important to integrate these databases with systematic analysis. These, in turn, are expected to disseminate the references and knowledge for well-trained AI systems. It is, therefore, contemplated that the AI systems can be exploited for performing two important tasks. Firstly, these can be utilized for natural product screening and for endorsing promising candidates for depression-targeted pre-clinical and clinical trials. Next, the effective anti-depression natural products, as revealed by these studies, are included in the treatment regime of the patients diagnosed with depression. Depending upon their effectiveness, these can be administered either as a stand-alone or in combination with commercially approved drugs. Secondly, AI systems can be used for surveillance as well as monitoring the health status of the patients. Accordingly, patients’ physiological data are acquired in real-time through wearables and cloud platform-assisted technology. This caters to the customized needs of the patients by providing them suggestions for their self-care, and their health status is also reported simultaneously. Although this systemic translational informatics-based model for depression management using natural antidepressants is promising and attractive, various issues are yet to be addressed to implement this futuristic approach.
FIGURE 6. The futuristic translational informatics-based model for depression management using natural antidepressants.
Unfortunately, the available databases for natural products contain scarce anti-depression therapeutic candidates (Varteresian and Lavretsky, 2014; Sorokina et al., 2021). As the scientific intrigue and general concern for depression management have gained momentum, it is imperative to develop anti-depression natural therapeutic candidate databases. For futuristic computational therapeutic screening, these depression-specific databases are essential. In light of the disease complexity, these databases must mandatorily employ an integrated systematic analysis. Further, the construction of knowledge databases is suggested as they undertake the compilation of data obtained from distinct levels. These include, but are not limited to, potential therapeutic biomolecules with the databases of natural products, related biomolecules, and their putative targets within the host system, environmental factors, and various other depression-associated attributes. Eventually, a stratified biomedical landscape for depression management is sketched by these knowledge databases. These knowledge databases, in turn, aid in the construction of knowledge graphs, which are expert-populated data integration biomedical resources. Specifically, the biomedical entities (concepts) are illustrated as nodes while inter-entity associations or relationships are depicted as edges (Yu et al., 2017; Nicholson and Greene, 2020). These might be of immense significance in assisting various biomedical applications capable of comprehending novel clinical, genomic, and pharmaceutical details needed for treatment support decisions.
Since depression is a neurological and debilitating disease with complex traits, which might have genetic roots as observed in the case of other psychiatric disorders such as cystic fibrosis and Huntington’s chorea (Bowcock, 2010; Dunn et al., 2015; Shadrina et al., 2018). Owing to the complexity of contributing factors, the AI system largely reckons on molecular dynamic simulations- and modeling tools-based depression evolutionary analysis for evaluating the implications of natural products for patients with depression disorders (Romano and Tatonetti, 2019). Consequently, unveiling the principal elements and crucial molecular players associated with disease progression and deciphering effective natural products for its management becomes an onerous task. This generates mind-boggling yet critical questions concerning improvisations on the robustness of AI for screening of natural products and precision medicine (Shen et al., 2021). Quite possibly, these can be subdued by employing quality training data, selection, optimization, and validation algorithms, feature extraction as well as standard and validated techniques-based data collection.
Depression-specific medicinal research encompasses complex clinical and molecular phenotypic data types with yet more complicated interconnections (Krishnan and Nestler, 2008; Clark et al., 2017). By and large, the ongoing research emphasizes certain aspects while neglecting other facets, which might be commensurably important in prompt diagnosis, therapy, and management of depressive disorders. Mapping these entwined clinical and molecular phenotypic linkages is cardinal for modeling in systems biology for any disease type (Schumann et al., 2014). For instance, there have been reports on the therapeutic efficacy of some natural products at the molecular level without any marked effect on the clinical phenotypes of patients (Liu et al., 2015; Yeung et al., 2018). This supports the notion that gathering a paired molecular and clinical data for a defined duration can be instrumental in simulating disease progression, depression, in this case, and generating a reliable model for the same. The Cancer Genome Atlas (TCGA) (https://www.cancer.gov/) and the International Cancer Genome Consortium (ICGC) (https://daco.icgc.org/) are exemplary data integration programs that can be viewed as a landmark for constructing similar depression-disorder programs. In conclusion, Figure 6 represents a promising systemic model for depression management, however, the feasibility of this model relies on several challenges, including the scarce quantitative data on natural antidepressants.
RS, SJ, and LS collected the data, analyzed it, and wrote the manuscript. BS generated the idea and outline, fundraiser, and finally revised the manuscript.
This work was supported by the National Natural Science Foundation of China (32070671), the Covid-19 research projects of West China Hospital Sichuan University (Grant No. HX-2019-nCoV-057) as well as the regional innovation cooperation between Sichuan and Guangxi Provinces (2020YFQ0019).
RS and SJ are honorary-based associated with the iGlobal Research and Publishing Foundation (iGRPF), New Delhi, India. The remaining authors declare that the research was conducted in the absence of any commercial or financial relationships that could be construed as a potential conflict of interest.
All claims expressed in this article are solely those of the authors and do not necessarily represent those of their affiliated organizations, or those of the publisher, the editors and the reviewers. Any product that may be evaluated in this article, or claim that may be made by its manufacturer, is not guaranteed or endorsed by the publisher.
The Supplementary Material for this article can be found online at: https://www.frontiersin.org/articles/10.3389/fcell.2021.738838/full#supplementary-material
Ali, B., Bashir, A. K., and Tanira, M. O. (1998a). The Effect of Rhazya Stricta Decne, a Traditional Medicinal Plant, on the Forced Swimming Test in Rats. Pharmacol. Biochem. Behav. 59 (2), 547–550. doi:10.1016/s0091-3057(97)00470-x
Ali, B. H., Bashir, A. K., Tanira, M. O. M., Medvedev, A. E., Jarrett, N., and Sandler, M. (1998b). Effect of Extract of Rhazya Stricta, a Traditional Medicinal Plant, on Rat Brain Tribulin. Pharmacol. Biochem. Behav. 59(3), 671–675. doi:10.1016/s0091-3057(97)00464-4
Altaf-Ul-Amin, M., Afendi, F. M., Kiboi, S. K., and Kanaya, S. (2014). Systems Biology in the Context of Big Data and Networks. Biomed. Res. Int. 2014, 1–11. doi:10.1155/2014/428570
Alvano, S. A., and Zieher, L. M. (2020). An Updated Classification of Antidepressants: A Proposal to Simplify Treatment. Personalized Med. Psychiatry, 19–20. doi:10.1016/j.pmip.2019.04.002
Anonymous (2000). Practice Guideline for the Treatment of Patients with Major Depressive Disorder (Revision). American Psychiatric Association. Am. J. Psychiatry 157 (4 Suppl. l), 1–45.
Apoorva, M., Suryawanshi, P., and Vidyasagar, G. M. (2021). Phytochemical Screening for Secondary Metabolites and Nutraceutical Value of Sesbania Grandiflora (L) Pers Leaf Extract. Indo Glob. J. Pharm. Sci. 11 (01), 28–32. doi:10.35652/igjps.2021.111004
Atanasov, A. G., Waltenberger, B., Pferschy-Wenzig, E.-M., Linder, T., Wawrosch, C., Uhrin, P., et al. (2015). Discovery and Resupply of Pharmacologically Active Plant-Derived Natural Products: A Review. Biotechnol. Adv. 33 (8), 1582–1614. doi:10.1016/j.biotechadv.2015.08.001
Atanasov, A. G., Zotchev, S. B., Dirsch, V. M., and Supuran, C. T. (2021). Natural Products in Drug Discovery: Advances and Opportunities. Nat. Rev. Drug Discov. 20 (3), 200–216. doi:10.1038/s41573-020-00114-z
Baird-Lambert, J., Davis, P. A., and Taylor, K. M. (1982). Methylaplysinopsin: A Natural Product of Marine Origin with Effects on Serotonergic Neurotransmission. Clin. Exp. Pharmacol. Physiol. 9 (2), 203–212. doi:10.1111/j.1440-1681.1982.tb00798.x
Baltz, R. H. (2019). Natural Product Drug Discovery in the Genomic Era: Realities, Conjectures, Misconceptions, and Opportunities. J. Ind. Microbiol. Biotechnol. 46 (3-4), 281–299. doi:10.1007/s10295-018-2115-4
Banerjee, P., Erehman, J., Gohlke, B.-O., Wilhelm, T., Preissner, R., and Dunkel, M. (2015). Super Natural II—A Database of Natural Products. Nucleic Acids Res. 43 (D1), D935–D939. doi:10.1093/nar/gku886
Bansal, H., Singla, R. K., Behzad, S., Chopra, H., Grewal, A. S., and Shen, B. (2021). Unleashing the Potential of Microbial Natural Products in Drug Discovery: Focusing on Streptomyces as Antimicrobials Goldmine. Curr. Top. Med. Chem. doi:10.2174/1568026621666210916170110
Barauna, S., Kaster, M., Heckert, B., Donascimento, K., Rossi, F., Teixeira, E., et al. (2006). Antidepressant-like Effect of Lectin from Canavalia Brasiliensis (ConBr) Administered Centrally in Mice. Pharmacol. Biochem. Behav. 85 (1), 160–169. doi:10.1016/j.pbb.2006.07.030
Baum, S. S., Hill, R., and Rommelspacher, H. (1998). Effect of Kava Extract and Individual Kavapyrones on Neurotransmitter Levels in the Nucleus Accumbens of Rats. Prog. Neuro-Psychopharmacology Biol. Psychiatry 22 (7), 1105–1120. doi:10.1016/s0278-5846(98)00062-1
Bhandari, K., Satyanarayana, S., De, B., Katakam, P., and Saha, G. (2019). Variance in Antioxidant Potentials and Neuroprotective Effect of Black Tea Due to Seasonal Effect. Indo Glob. J. Pharm. Sci. 09 (01), 54–59. doi:10.35652/igjps.2019.9107
Bharatam, P. V. (2021). Computer-Aided Drug Design. Drug Discov. Dev., 137. doi:10.1007/978-981-15-5534-3_6
Bharti, V., Kiran, S. K., and Singh, S. (2013). Evaluation of the Memory and Learning Improving Effects of Benincasa Hispida Seeds in Mice. Pharmacologia 4 (3), 249–253. doi:10.5567/pharmacologia.2013.249.253
Bickman, L. (2020). Improving Mental Health Services: A 50-Year Journey from Randomized Experiments to Artificial Intelligence and Precision Mental Health. Adm. Pol. Ment. Health Ment. Health Serv. Res. 47 (5), 795–843. doi:10.1007/s10488-020-01065-8
Biswas, U. K., Choudhry, B., and Amrita, K. (2012). Comparative Evaluation of the Antidepressant Effects of Ethanolic and Aqueous Extract of Glycyrrhiza Glabra in Rats and Mice. J. Glob. Trends Pharm. Sci. 3, 585–601.
Bowcock, A. M. (2010). Genome-Wide Association Studies and Infectious Disease. Crit. Reviews™ Immunol. 30 (3), 305–309. doi:10.1615/CritRevImmunol.v30.i3.80
Bucar, F., Wube, A., and Schmid, M. (2013). Natural Product Isolation – How to Get from Biological Material to Pure Compounds. Nat. Product. Rep. 30, 525–545. doi:10.1039/c3np20106f
Burdette, J. E., Liu, J., Chen, S.-n., Fabricant, D. S., Piersen, C. E., Barker, E. L., et al. (2003). Black Cohosh Acts as a Mixed Competitive Ligand and Partial Agonist of the Serotonin Receptor. J. Agric. Food Chem. 51 (19), 5661–5670. doi:10.1021/jf034264r
Butler, M. S. (2004). The Role of Natural Product Chemistry in Drug Discovery. J. Nat. Prod. 67 (12), 2141–2153. doi:10.1021/np040106y
Butterweck, V., Nishibe, S., Sasaki, T., and Uchida, M. (2001). Antidepressant Effects of Apocynum Venetum Leaves in a Forced Swimming Test. Biol. Pharm. Bull. 24 (7), 848–851. doi:10.1248/bpb.24.848
Caldera, M., Buphamalai, P., Müller, F., and Menche, J. (2017). Interactome-based Approaches to Human Disease. Curr. Opin. Syst. Biol. 3, 88–94. doi:10.1016/j.coisb.2017.04.015
Campos, M. M., Fernandes, E. S., Ferreira, J., Santos, A. R. S., and Calixto, J. B. (2005). Antidepressant-like Effects of Trichilia Catigua (Catuaba) Extract: Evidence for Dopaminergic-Mediated Mechanisms. Psychopharmacology 182 (1), 45–53. doi:10.1007/s00213-005-0052-1
Canuel, V., Rance, B., Avillach, P., Degoulet, P., and Burgun, A. (2014). Translational Research Platforms Integrating Clinical and Omics Data: a Review of Publicly Available Solutions. Brief. Bioinform. 16 (2), 280–290. doi:10.1093/bib/bbu006
Cao, L., Shcherbin, E., Mohimani, H., and Lax, S. (2019). A Metabolome- and Metagenome-wide Association Network Reveals Microbial Natural Products and Microbial Biotransformation Products from the Human Microbiota. mSystems 4. doi:10.1128/mSystems.00387-19
Chamberlin, S. R., Blucher, A., Wu, G., Shinto, L., Choonoo, G., Kulesz-Martin, M., et al. (2019). Natural Product Target Network Reveals Potential for Cancer Combination Therapies. Front. Pharmacol. 10. doi:10.3389/fphar.2019.00557
Chandran, U., Mehendale, N., Patil, S., Chaguturu, R., and Patwardhan, B. (2017). Network Pharmacology. Innovative Approaches Drug Discov., 127. doi:10.1016/b978-0-12-801814-9.00005-2
Chang, K.-W., Tsai, T.-Y., Chen, K.-C., Yang, S.-C., Huang, H.-J., Chang, T.-T., et al. (2011). iSMART: An Integrated Cloud Computing Web Server for Traditional Chinese Medicine for Online Virtual Screening,de novoEvolution and Drug Design. J. Biomol. Struct. Dyn. 29 (1), 243–250. doi:10.1080/073911011010524988
Charitou, T., Bryan, K., and Lynn, D. J. (2016). Using Biological Networks to Integrate, Visualize and Analyze Genomics Data. Genet. Selection Evol. 48. doi:10.1186/s12711-016-0205-1
Chen, J., Liu, X., Shen, L., Lin, Y., and Shen, B. (2021). CMBD: A Manually Curated Cancer Metabolic Biomarker Knowledge Database. Oxford: Oxford University Press. doi:10.1093/database/baaa094
Chen, Y., Liu, X., Yu, Y., Yu, C., Yang, L., Lin, Y., et al. (2020). PCaLiStDB: A Lifestyle Database for Precision Prevention of Prostate Cancer. Oxford: Oxford University Press. doi:10.1093/database/baz154
Cheng, F., Lu, W., Liu, C., Fang, J., Hou, Y., Handy, D. E., et al. (2019). A Genome-wide Positioning Systems Network Algorithm for In Silico Drug Repurposing. Nat. Commun. 10. doi:10.1038/s41467-019-10744-6
Cheng, M.-C., Li, C.-Y., Ko, H.-C., Ko, F.-N., Lin, Y.-L., and Wu, T.-S. (2006). Antidepressant Principles of the Roots of Polygala Tenuifolia. J. Nat. Prod. 69 (9), 1305–1309. doi:10.1021/np060207r
Chowdhury, B., Bhattamisra, S. K., and Das, M. C. (2011). Involvement of Monoaminergic System in Antidepressant-like Activity of Glycyrrhiza Glabra Root Extracts in Rat. Pharmacologyonline 2, 405–415.
Clark, L. A., Cuthbert, B., Lewis-Fernández, R., Narrow, W. E., and Reed, G. M. (2017). Three Approaches to Understanding and Classifying Mental Disorder: ICD-11, DSM-5, and the National Institute of Mental Health’s Research Domain Criteria (RDoC). Psychol. Sci. Public Interest 18 (2), 72–145. doi:10.1177/1529100617727266
Cheesman, M., Cock, I., Ilanko, A., Blonk, B., and Cock, I. E. (2017). Developing New Antimicrobial Therapies: Are Synergistic Combinations of Plant Extracts/Compounds with Conventional Antibiotics the Solution?. Pharmacogn. Rev. 11(22), 57–72. doi:10.4103/phrev.phrev_21_17
Cragg, G. M., and Newman, D. J. (2013). Natural Products: A Continuing Source of Novel Drug Leads. Biochim. Biophys. Acta (Bba) - Gen. Subjects 1830 (6), 3670–3695. doi:10.1016/j.bbagen.2013.02.008
Cragg, G. M., Newman, D. J., and Snader, K. M. (1997). Natural Products in Drug Discovery and Development. J. Nat. Prod. 60 (1), 52–60. doi:10.1021/np9604893
Cui, C., Yang, M., Yao, Z., Cao, B., Luo, Z., Xu, Y., et al. (1995). [Antidepressant Active Constituents in the Roots of Morinda Officinalis How]. Zhongguo Zhong Yao Za Zhi, 36-39, 62–33.
Dangar, D. K., and Patel, N. J. (2021). Anti-Inflammatory Effect of Neuracanthus Sphaerostachyus Dalz. Leaves on Experimental Colitis in Rats. Indo Glob. J. Pharm. Sci. 11 (01), 07–14. doi:10.35652/igjps.2021.111002
Deepa, B., Suchetha, K., and Rao, S. (2013). Antidepressant Activity of Nardostachys Jatamansi in Electron Beam Irradiated Mice. Int. J. Res. Ayurveda Pharm. 4 (1), 101–103. doi:10.7897/2277-4343.04135
Dhanarasu, S., and Al-Hazimi, A. (2013). Phytochemistry, Pharmacological and Therapeutic Applications of Nelumbo nucifera. Asian J. Phytomed. Clin. Res. 1, 123–136.
Dhingra, D., and Goyal, P. K.. (2008a). Evidences for the Involvement of Monoaminergic and GABAergic Systems in Antidepressant-like Activity ofTinospora Cordifoliain Mice. Indian J. Pharm. Sci. 70(6). doi:10.4103/0250-474x.49118
Dhingra, D., and Goyal, P. K. (2008b). Inhibition of MAO and GABA: Probable Mechanisms for Antidepressant-like Activity of Nardostachys Jatamansi DC. In Mice. Indian J. Exp. Biol. 46 (4), 212–218.
Dhingra, D., and Joshi, P. (2012). Antidepressant-like Activity of Benincasa Hispida Fruits in Mice: Possible Involvement of Monoaminergic and GABAergic Systems. J. Pharmacol. Pharmacotherapeutics 3. doi:10.4103/0976-500x.92521
Dhingra, D., Joshi, P., Gupta, A., and Chhillar, R. (2011). Possible Involvement of Monoaminergic Neurotransmission in Antidepressant-like Activity of Emblica Officinalis Fruits in Mice. CNS Neurosci. Ther. 18 (5), 419–425. doi:10.1111/j.1755-5949.2011.00256.x
Dhingra, D., and Kumar, V. (2007). Pharmacological Evaluation for Antidepressant-like Activity of Asparagus racemosus Willd. In Mice. Pharmacologyonline 3, 133–152.
Dhingra, D., and Sharma, A. (2006). Antidepressant-like Activity of Glycyrrhiza Glabra L. In Mouse Models of Immobility Tests. Prog. Neuro-Psychopharmacology Biol. Psychiatry 30 (3), 449–454. doi:10.1016/j.pnpbp.2005.11.019
Dryja, T. P. (1997). Gene-based Approach to Human Gene-Phenotype Correlations. Proc. Natl. Acad. Sci. 94 (22), 12117–12121. doi:10.1073/pnas.94.22.12117
Dugger, S. A., Platt, A., and Goldstein, D. B. (2017). Drug Development in the Era of Precision Medicine. Nat. Rev. Drug Discov. 17 (3), 183–196. doi:10.1038/nrd.2017.226
Duke, J. A., Janick, J., and Simon, J. E. (1993). Medicinal Plants and the Pharmaceutical Industry.. New York: Wiley, New crops.
Duman, R. S., and Voleti, B. (2012). Signaling Pathways Underlying the Pathophysiology and Treatment of Depression: Novel Mechanisms for Rapid-Acting Agents. Trends Neurosci. 35 (1), 47–56. doi:10.1016/j.tins.2011.11.004
Dunn, E. C., Brown, R. C., Dai, Y., Rosand, J., Nugent, N. R., Amstadter, A. B., et al. (2015). Genetic Determinants of Depression. Harv. Rev. Psychiatry 23 (1), 1–18. doi:10.1097/hrp.0000000000000054
Duric, V., Banasr, M., Licznerski, P., Schmidt, H. D., Stockmeier, C. A., Simen, A. A., et al. (2010). A Negative Regulator of MAP Kinase Causes Depressive Behavior. Nat. Med. 16 (11), 1328–1332. doi:10.1038/nm.2219
Dwivedi, Y., Rizavi, H. S., Roberts, R. C., Conley, R. C., Tamminga, C. A., and Pandey, G. N. (2001). Reduced Activation and Expression of ERK1/2 MAP Kinase in the post-mortem Brain of Depressed Suicide Subjects. J. Neurochem. 77 (3), 916–928. doi:10.1046/j.1471-4159.2001.00300.x
Dwivedi, Y., Rizavi, H. S., Shukla, P. K., Lyons, J., Faludi, G., Palkovits, M., et al. (2004). Protein Kinase A in Postmortem Brain of Depressed Suicide Victims: Altered Expression of Specific Regulatory and Catalytic Subunits. Biol. Psychiatry 55 (3), 234–243. doi:10.1016/j.biopsych.2003.11.003
Dwivedi, Y., Rizavi, H. S., Zhang, H., Roberts, R. C., Conley, R. R., and Pandey, G. N. (2009). Aberrant Extracellular Signal-Regulated Kinase (ERK)1/2 Signalling in Suicide Brain: Role of ERK Kinase 1 (MEK1). Int. J. Neuropsychopharmacol. 12 (10), 1337–1354. doi:10.1017/S1461145709990575
Emilien, G. (2000). Impact of Genomics on Drug Discovery and Clinical Medicine. Qjm 93 (7), 391–423. doi:10.1093/qjmed/93.7.391
England, L. J. (1998). Inactivation of a Serotonin-Gated Ion Channel by a Polypeptide Toxin from Marine Snails. Science 281 (5376), 575–578. doi:10.1126/science.281.5376.575
Ettehadi, H., Mojabi, S. N., Ranjbaran, M., Shams, J., Sahraei, H., Hedayati, M., et al. (2013). Aqueous Extract of Saffron (Crocus Sativus) Increases Brain Dopamine and Glutamate Concentrations in Rats. J. Behav. Brain Sci. 03 (03), 315–319. doi:10.4236/jbbs.2013.33031
Faeder, J. R., Rivas-Barragan, D., Mubeen, S., Guim Bernat, F., Hofmann-Apitius, M., and Domingo-Fernández, D. (2020). Reasoning over Causal Paths in Biological Networks for Drug Discovery. PLOS Comput. Biol. 16. doi:10.1371/journal.pcbi.1008464
Fajemiroye, J. O., da Silva, D. M., de Oliveira, D. R., and Costa, E. A. (2016). Treatment of Anxiety and Depression: Medicinal Plants in Retrospect. Fundam. Clin. Pharmacol. 30 (3), 198–215. doi:10.1111/fcp.12186
Fan, Z. Z., Zhao, W. H., Guo, J., Cheng, R. F., Zhao, J. Y., Yang, W. D., et al. (2012). Antidepressant Activities of Flavonoids from Glycyrrhiza Uralensis and its Neurogenesis Protective Effect in Rats. Yao Xue Xue Bao 47 (12), 1612–1617.
Fang, S., Dong, L., Liu, L., Guo, J., Zhao, L., Zhang, J., et al. (2021). HERB: a High-Throughput experiment- and Reference-Guided Database of Traditional Chinese Medicine. Nucleic Acids Res. 49 (D1), D1197–D1206. doi:10.1093/nar/gkaa1063
Farahani, M. S., Bahramsoltani, R., Farzaei, M. H., Abdollahi, M., and Rahimi, R. (2015). Plant-derived Natural Medicines for the Management of Depression: an Overview of Mechanisms of Action. Rev. Neurosciences 26, 58. doi:10.1515/revneuro-2014-0058
Ferguson, J. M. (2001). SSRI Antidepressant Medications. Prim. Care Companion J. Clin. Psychiatry 03 (01), 22–27. doi:10.4088/PCC.v03n0105
Furlong, L. I. (2013). Human Diseases through the Lens of Network Biology. Trends Genet. 29 (3), 150–159. doi:10.1016/j.tig.2012.11.004
Futamura, Y., Yamamoto, K., and Osada, H. (2017). Phenotypic Screening Meets Natural Products in Drug Discovery†. Biosci. Biotechnol. Biochem. 81 (1), 28–31. doi:10.1080/09168451.2016.1248365
Gadaga, L. L., Tagwireyi, D., Dzangare, J., and Nhachi, C. F. B. (2010). Acute Oral Toxicity and Neurobehavioural Toxicological Effects of Hydroethanolic Extract of Boophone Disticha in Rats. Hum. Exp. Toxicol. 30 (8), 972–980. doi:10.1177/0960327110384524
Gaur, V., Bodhankar, S. L., Mohan, V., and Thakurdesai, P. (2012). Antidepressant-like Effect of 4-hydroxyisoleucine from Trigonella Foenum Graecum L. Seeds in Mice. Biomed. Aging Pathol. 2 (3), 121–125. doi:10.1016/j.biomag.2012.07.002
Genilloud, O. (2014). The Re-emerging Role of Microbial Natural Products in Antibiotic Discovery. Antonie van Leeuwenhoek 106 (1), 173–188. doi:10.1007/s10482-014-0204-6
Girish, C., Oommen, S., and Vishnu, R. (2016). Evidence for the Involvement of the Monoaminergic System in the Antidepressant-like Activity of Methanolic Extract of Bacopa Monnieri in Albino Mice. Int. J. Basic Clin. Pharmacol., 914–922. doi:10.18203/2319-2003.ijbcp20161545
Gogoi, B., Gogoi, D., Silla, Y., Kakoti, B. B., and Bhau, B. S. (2017). Network Pharmacology-Based Virtual Screening of Natural Products from Clerodendrum Species for Identification of Novel Anti-cancer Therapeutics. Mol. BioSystems 13 (2), 406–416. doi:10.1039/c6mb00807k
Graham, S., Depp, C., Lee, E. E., Nebeker, C., Tu, X., Kim, H.-C., et al. (2019). Artificial Intelligence for Mental Health and Mental Illnesses: an Overview. Curr. Psychiatry Rep. 21. doi:10.1007/s11920-019-1094-0
Grist, R., Croker, A., Denne, M., and Stallard, P. (2018). Technology Delivered Interventions for Depression and Anxiety in Children and Adolescents: A Systematic Review and Meta-Analysis. Clin. Child Fam. Psychol. Rev. 22 (2), 147–171. doi:10.1007/s10567-018-0271-8
Gu, J., Gui, Y., Chen, L., Yuan, G., Lu, H. Z., and Xu, X. (2013). Use of Natural Products as Chemical Library for Drug Discovery and Network Pharmacology. PLoS ONE 8, 839. doi:10.1371/journal.pone.0062839
Gunatilaka, A. A. L. (2006). Natural Products from Plant-Associated Microorganisms: Distribution, Structural Diversity, Bioactivity, and Implications of Their Occurrence. J. Nat. Prod. 69 (3), 509–526. doi:10.1021/np058128n
Guo, Z.-H., You, Z.-H., Huang, D.-S., Yi, H.-C., Chen, Z.-H., and Wang, Y.-B. (2020). A Learning Based Framework for Diverse Biomolecule Relationship Prediction in Molecular Association Network. Commun. Biol. 3, 8. doi:10.1038/s42003-020-0858-8
Gupta, R., Srivastava, D., Sahu, M., Tiwari, S., Ambasta, R. K., and Kumar, P. (2021). Artificial Intelligence to Deep Learning: Machine Intelligence Approach for Drug Discovery. Mol. Divers. 25 (3), 1315–1360. doi:10.1007/s11030-021-10217-3
Harel, A., Dalah, I., Pietrokovski, S., Safran, M., and Lancet, D. (2011). Omics Data Management and Annotation. Bioinformatics for Omics Data. 71. doi:10.1007/978-1-61779-027-0_3
Hasin, Y., Seldin, M., and Lusis, A. (2017). Multi-omics Approaches to Disease. Genome Biol. 18, 83–98. doi:10.1186/s13059-017-1215-1
Hategan, A., Giroux, C., and Bourgeois, J. A. (2019). Digital Technology Adoption in Psychiatric Care: an Overview of the Contemporary Shift from Technology to Opportunity. J. Techn. Behav. Sci. 4 (3), 171–177. doi:10.1007/s41347-019-00086-x
Hatherley, R., Brown, D. K., Musyoka, T. M., Penkler, D. L., Faya, N., Lobb, K. A., et al. (2015). SANCDB: a South African Natural Compound Database. J. Cheminformatics 7, 29–38. doi:10.1186/s13321-015-0080-8
Hazra, S., Banerjee, R., Das, B. K., Ghosh, A. K., Banerjee, T. K., Hazra, U. S., et al. (2012). Evaluation of Antidepressant Activity of Bacopa Monnieri in Rat: a Study in Animal Model of Depression. Drug Discov. 2 (4), 8–13.
He, H., Shi, M., Lin, Y., Zhan, C., Wu, R., Bi, C., et al. (2021). HFBD: a Biomarker Knowledge Database for Heart Failure Heterogeneity and Personalized Applications. Bioinformatics. doi:10.1093/bioinformatics/btab470
Hedner, E., Sjögren, M., Frändberg, P.-A., Johansson, T., Göransson, U., Dahlström, M., et al. (2006). Brominated Cyclodipeptides from the Marine Sponge Geodia Barretti as Selective 5-HT Ligands. J. Nat. Prod. 69 (10), 1421–1424. doi:10.1021/np0601760
Herland, M., Khoshgoftaar, T. M., and Wald, R. (2014). A Review of Data Mining Using Big Data in Health Informatics. J. Big Data 1. doi:10.1186/2196-1115-1-2
Horton, R. H., and Lucassen, A. M. (2019). Recent Developments in Genetic/genomic Medicine. Clin. Sci. 133 (5), 697–708. doi:10.1042/cs20180436
Hosseinzadeh, H., Karimi, G., and Niapoor, M. (2004). Antidepressant Effect of Crocus Sativus L. Stigma Extracts and Their Constituents, Crocin and Safranal, in Mice. Acta Horticulturae (650), 435–445. doi:10.17660/ActaHortic10.17660/actahortic.2004.650.54
Hosseinzadeh, H., Motamedshariaty, V., and Hadizadeh, F. (2007). Antidepressant Effect of Kaempferol, a Constituent of Saffron (Crocus Sativus) Petal, in Mice and Rats. Pharmacologyonline 2, 367–370.
Hu, J.-F., Schetz, J. A., Kelly, M., Peng, J.-N., Ang, K. K. H., Flotow, H., et al. (2002). New Antiinfective and Human 5-HT2 Receptor Binding Natural and Semisynthetic Compounds from the Jamaican Sponge Smenospongia Aurea. J. Nat. Prod. 65 (4), 476–480. doi:10.1021/np010471e
Hu, J. X., Thomas, C. E., and Brunak, S. (2016). Network Biology Concepts in Complex Disease Comorbidities. Nat. Rev. Genet. 17 (10), 615–629. doi:10.1038/nrg.2016.87
Hu, Y., Liu, M., Liu, P., Guo, D.-H., Wei, R.-B., and Rahman, K. (2011). Possible Mechanism of the Antidepressant Effect of 3,6′-disinapoyl Sucrose from Polygala Tenuifolia Willd. J. Pharm. Pharmacol. 63 (6), 869–874. doi:10.1111/j.2042-7158.2011.01281.x
Hu, Y., Liu, P., Guo, D.-H., Rahman, K., Wang, D.-X., and Xie, T.-T. (2010). Antidepressant Effects of the Extract YZ-50 fromPolygala Tenuifoliain Chronic Mild Stress Treated Rats and its Possible Mechanisms. Pharm. Biol. 48 (7), 794–800. doi:10.3109/13880200903280034
Huang, L., Xie, D., Yu, Y., Liu, H., Shi, Y., Shi, T., et al. (2018a). TCMID 2.0: a Comprehensive Resource for TCM. Nucleic Acids Res. 46 (D1), D1117–D1120. doi:10.1093/nar/gkx1028
Huang, W., Brewer, L. K., Jones, J. W., Nguyen, A. T., Marcu, A., Wishart, D. S., et al. (2018b). PAMDB: a Comprehensive Pseudomonas aeruginosa Metabolome Database. Nucleic Acids Res. 46 (D1), D575–D580. doi:10.1093/nar/gkx1061
Huckvale, K., Venkatesh, S., and Christensen, H. (2019). Toward Clinical Digital Phenotyping: a Timely Opportunity to Consider Purpose, Quality, and Safety. npj Digital Med. 2, 88. doi:10.1038/s41746-019-0166-1
Igoli, J., Gray, A., Clements, C., Kantheti, P., and Singla, R. (2014). Antitrypanosomal Activity & Docking Studies of Isolated Constituents from the Lichen Cetraria Islandica: Possibly Multifunctional Scaffolds. Curr. Top. Med. Chem. 14 (8), 1014–1021. doi:10.2174/1568026614666140324122323
Ikeda, H. (2017). Natural Products Discovery from Micro-organisms in the post-genome Era†. Biosci. Biotechnol. Biochem. 81 (1), 13–22. doi:10.1080/09168451.2016.1248366
Ikram, R. R. R., Ghani, M. K. A., and Abdullah, N. (2015). An Analysis of Application of Health Informatics in Traditional Medicine: A Review of Four Traditional Medicine Systems. Int. J. Med. Inform. 84 (11), 988–996. doi:10.1016/j.ijmedinf.2015.05.007
Irwin, J. J., and Shoichet, B. K. (2005). ZINC–a Free Database of Commercially Available Compounds for Virtual Screening. J. Chem. Inf. Model. 45 (1), 177–182. doi:10.1021/ci04971410.1021/ci049714++
Ishola, I., Akinyede, A., and Sholarin, A. (2013). Antidepressant and Anxiolytic Properties of the Methanolic Extract of Momordica Charantia Linn (Cucurbitaceae) and its Mechanism of Action. Drug Res. 64 (07), 368–376. doi:10.1055/s-0033-1358712
Ito, N., Yabe, T., Gamo, Y., Nagai, T., Oikawa, T., Yamada, H., et al. (2008). Rosmarinic Acid from Perillae Herba Produces an Antidepressant-like Effect in Mice through Cell Proliferation in the Hippocampus. Biol. Pharm. Bull. 31 (7), 1376–1380. doi:10.1248/bpb.31.1376
James, S. L., Abate, D., Abate, K. H., Abay, S. M., Abbafati, C., Abbasi, N., et al. (2018). Global, Regional, and National Incidence, Prevalence, and Years Lived with Disability for 354 Diseases and Injuries for 195 Countries and Territories, 1990–2017: a Systematic Analysis for the Global Burden of Disease Study 2017. The Lancet 392 (10159), 1789–1858. doi:10.1016/s0140-6736(18)32279-7
Jang, H. J., Suh, P. G., Lee, Y. J., Shin, K. J., Cocco, L., and Chae, Y. C. (2018). PLCgamma1: Potential Arbitrator of Cancer Progression. Adv. Biol. Regul. 67, 179–189. doi:10.1016/j.jbior.2017.11.003
Jarada, T. N., Rokne, J. G., and Alhajj, R. (2020). A Review of Computational Drug Repositioning: Strategies, Approaches, Opportunities, Challenges, and Directions. J. Cheminformatics 12. doi:10.1186/s13321-020-00450-7
Jin, Z.-L., Gao, N., Zhou, D., Chi, M.-G., Yang, X.-M., and Xu, J.-P. (2012). The Extracts of Fructus Akebiae, a Preparation Containing 90% of the Active Ingredient Hederagenin: Serotonin, Norepinephrine and Dopamine Reuptake Inhibitor. Pharmacol. Biochem. Behav. 100 (3), 431–439. doi:10.1016/j.pbb.2011.10.001
Joon, S., Singla, R. K., Shen, B., and Kamal, M. A. (2021). QSCR Analysis of Cytotoxicity of 6-Fluoro-3-(4h-1,2,4-Triazol-3-Yl)quinolin-4(1h)-Ones on Chinese Hamster Ovary Cell Line: Design of REPUBLIC1986. Curr. Med. Chem. doi:10.2174/0929867328666210623150552
Jyoti, P., and Kumar, D. (2019). A Novel Therapy for the Treatment of Depression. Indo Glob. J. Pharm. Sci. 09 (02), 138. doi:10.35652/igjps.2019.92s36
Karanth, K. S., Karkada, G., Shenoy, K. B., and Halahalli, H. (2012). Nardostachys Jatamansi Extract Prevents Chronic Restraint Stress-Induced Learning and Memory Deficits in a Radial Arm Maze Task. J. Nat. Sci. Biol. Med. 3. doi:10.4103/0976-9668.101879
Katz, L., and Baltz, R. H. (2016). Natural Product Discovery: Past, Present, and Future. J. Ind. Microbiol. Biotechnol. 43 (2-3), 155–176. doi:10.1007/s10295-015-1723-5
Kautsar, S. A., Blin, K., Shaw, S., Weber, T., and Medema, M. H. (2021). BiG-FAM: the Biosynthetic Gene Cluster Families Database. Nucleic Acids Res. 49 (D1), D490–D497. doi:10.1093/nar/gkaa812
Ke, H., Chen, D., Shah, T., Liu, X., Zhang, X., Zhang, L., et al. (2020). Cloud-aided Online EEG Classification System for Brain Healthcare: A Case Study of Depression Evaluation with a Lightweight CNN. Softw. Pract. Experience 50 (5), 596–610. doi:10.1002/spe.2668
Khan, S. L., Sonwane, G. M., Siddiqui, F. A., Jain, S. P., Kale, M. A., and Borkar, V. S. (2020). Discovery of Naturally Occurring Flavonoids as Human Cytochrome P450 (CYP3A4) Inhibitors with the Aid of Computational Chemistry. Indo Glob. J. Pharm. Sci. 10 (04), 58–69. doi:10.35652/igjps.2020.10409
Khanin, R., Bauer-Mehren, A., Bundschus, M., Rautschka, M., Mayer, M. A., Sanz, F., et al. (2011). Gene-Disease Network Analysis Reveals Functional Modules in Mendelian, Complex and Environmental Diseases. PLoS ONE 6. doi:10.1371/journal.pone.0020284
Kim, S.-K., Nam, S., Jang, H., Kim, A., and Lee, J.-J. (2015). TM-MC: a Database of Medicinal Materials and Chemical Compounds in Northeast Asian Traditional Medicine. BMC Complement. Altern. Med. 15. doi:10.1186/s12906-015-0758-5
Kiriiri, G. K., Njogu, P. M., and Mwangi, A. N. (2020). Exploring Different Approaches to Improve the success of Drug Discovery and Development Projects: a Review. Future J. Pharm. Sci. 6(1). doi:10.1186/s43094-020-00047-9
Klucznik, T., Mikulak-Klucznik, B., McCormack, M. P., Lima, H., Szymkuć, S., Bhowmick, M., et al. (2018). Efficient Syntheses of Diverse, Medicinally Relevant Targets Planned by Computer and Executed in the Laboratory. Chem 4 (3), 522–532. doi:10.1016/j.chempr.2018.02.002
Kochanowska, A. J., Rao, K. V., Childress, S., El-Alfy, A., Matsumoto, R. R., Kelly, M., et al. (2008). Secondary Metabolites from Three Florida Sponges with Antidepressant Activity. J. Nat. Prod. 71 (2), 186–189. doi:10.1021/np070371u
Kochanowska-Karamyan, A. J., Araujo, H. C., Zhang, X., El-Alfy, A., Carvalho, P., Avery, M. A., et al. (2020). Isolation and Synthesis of Veranamine, an Antidepressant Lead from the Marine Sponge Verongula Rigida. J. Nat. Prod. 83 (4), 1092–1098. doi:10.1021/acs.jnatprod.9b01107
König, I. R., Fuchs, O., Hansen, G., von Mutius, E., and Kopp, M. V. (2017). What Is Precision Medicine? Eur. Respir. J. 50. doi:10.1183/13993003.00391-2017
Kraus, C., Kadriu, B., Lanzenberger, R., Zarate, C. A., and Kasper, S. (2019). Prognosis and Improved Outcomes in Major Depression: a Review. Translational Psychiatry 9. doi:10.1038/s41398-019-0460-3
Kraus, J.-l. (2019). Can Artificial Intelligency Revolutionize Drug Discovery? Ai Soc. 35 (2), 501–504. doi:10.1007/s00146-019-00892-0
Krishnan, V., and Nestler, E. J. (2008). The Molecular Neurobiology of Depression. Nature 455 (7215), 894–902. doi:10.1038/nature07455
Kryshtafovych, A., Schwede, T., Topf, M., Fidelis, K., and Moult, J. (2019). Critical Assessment of Methods of Protein Structure Prediction (CASP)—Round XIII. Proteins: Struct. Funct. Bioinformatics 87 (12), 1011–1020. doi:10.1002/prot.25823
Kulkarni, S. K., Bhutani, M. K., and Bishnoi, M. (2008). Antidepressant Activity of Curcumin: Involvement of Serotonin and Dopamine System. Psychopharmacology 201 (3), 435–442. doi:10.1007/s00213-008-1300-y
Laganà, P., Anastasi, G., Marano, F., Piccione, S., Singla, R. K., Dubey, A. K., et al. (2019). Phenolic Substances in Foods: Health Effects as Anti-inflammatory and Antimicrobial Agents. J. AOAC Int. 102 (5), 1378–1387. doi:10.5740/jaoacint.19-0131
Lander, E. S., Linton, L. M., Birren, B., Nusbaum, C., Zody, M. C., Baldwin, J., et al. (2001). Initial Sequencing and Analysis of the Human Genome. Nature 409 (6822), 860–921. doi:10.1038/35057062
Lee, S. A., Hong, S. S., Han, X. H., Hwang, J. S., Oh, G. J., Lee, K. S., et al. (2005). Piperine from the Fruits of Piper Longum with Inhibitory Effect on Monoamine Oxidase and Antidepressant-like Activity. Chem. Pharm. Bull. 53 (7), 832–835. doi:10.1248/cpb.53.832
Lee, S. A., Hwang, J. S., Han, X. H., Lee, C., Lee, M. H., Choe, S. G., et al. (2008). Methylpiperate Derivatives from Piper Longum and Their Inhibition of Monoamine Oxidase. Arch. Pharmacal Res. 31 (6), 679–683. doi:10.1007/s12272-001-1212-7
Li, X., Liu, Z., Li, W., Sun, N., Xu, Y., Xie, Z., et al. (2016). PTPRR Regulates ERK Dephosphorylation in Depression Mice Model. J. Affect Disord. 193, 233–241. doi:10.1016/j.jad.2015.12.049
Li, Y., Zhang, C., Bell, E. W., Yu, D. J., and Zhang, Y. (2019). Ensembling Multiple Raw Coevolutionary Features with Deep Residual Neural Networks for Contact-Map Prediction in CASP13. Proteins: Struct. Funct. Bioinformatics 87 (12), 1082–1091. doi:10.1002/prot.25798
Liao, J.-C., Tsai, J.-C., Liu, C.-Y., Huang, H.-C., Wu, L.-Y., and Peng, W.-H. (2013). Antidepressant-like Activity of Turmerone in Behavioral Despair Tests in Mice. BMC Complement. Altern. Med. 13. doi:10.1186/1472-6882-13-299
Liebenberg, N., Muller, H. K., Fischer, C. W., Harvey, B. H., Brink, C. B., Elfving, B., et al. (2011). An Inhibitor of cAMP-dependent Protein Kinase Induces Behavioural and Neurological Antidepressant-like Effects in Rats. Neurosci. Lett. 498 (2), 158–161. doi:10.1016/j.neulet.2011.05.004
Liu, L., Liu, C., Wang, Y., Wang, P., Li, Y., and Li, B. (2015). Herbal Medicine for Anxiety, Depression and Insomnia. Curr. Neuropharmacology 13 (4), 481–493. doi:10.2174/1570159x1304150831122734
Liu, X., Zhang, X., Chen, J., Ye, B., Ren, S., Lin, Y., et al. (2020). CRC-EBD: Epigenetic Biomarker Database for Colorectal Cancer. Front. Genet. 11, 907. doi:10.3389/fgene.2020.00907
Lyu, C., Chen, T., Qiang, B., Liu, N., Wang, H., Zhang, L., et al. (2021). CMNPD: a Comprehensive marine Natural Products Database towards Facilitating Drug Discovery from the Ocean. Nucleic Acids Res. 49 (D1), D509–D515. doi:10.1093/nar/gkaa763
Machado, D. G., Bettio, L. E. B., Cunha, M. P., Santos, A. R. S., Pizzolatti, M. G., Brighente, I. M. C., et al. (2008). Antidepressant-like Effect of Rutin Isolated from the Ethanolic Extract from Schinus Molle L. In Mice: Evidence for the Involvement of the Serotonergic and Noradrenergic Systems. Eur. J. Pharmacol. 587 (1-3), 163–168. doi:10.1016/j.ejphar.2008.03.021
Machado, D. G., Cunha, M. P., Neis, V. B., Balen, G. O., Colla, A., Bettio, L. E. B., et al. (2013). Antidepressant-like Effects of Fractions, Essential Oil, Carnosol and Betulinic Acid Isolated from Rosmarinus Officinalis L. Food Chem. 136 (2), 999–1005. doi:10.1016/j.foodchem.2012.09.028
Machado, D. G., Kaster, M. P., Binfaré, R. W., Dias, M., Santos, A. R. S., Pizzolatti, M. G., et al. (2007). Antidepressant-like Effect of the Extract from Leaves of Schinus Molle L. In Mice: Evidence for the Involvement of the Monoaminergic System. Prog. Neuro-Psychopharmacology Biol. Psychiatry 31 (2), 421–428. doi:10.1016/j.pnpbp.2006.11.004
Machado, D. G., Neis, V. B., Balen, G. O., Colla, A., Cunha, M. P., Dalmarco, J. B., et al. (2012). Antidepressant-like Effect of Ursolic Acid Isolated from Rosmarinus Officinalis L. In Mice: Evidence for the Involvement of the Dopaminergic System. Pharmacol. Biochem. Behav. 103 (2), 204–211. doi:10.1016/j.pbb.2012.08.016
Madaan, R., Singla, R. K., Kumar, S., Dubey, A. K., Kumar, D., Sharma, P., et al. (2021). Bergenin - a Biologically Active Scaffold: Nanotechnological Perspectives. Curr. Top. Med. Chem. doi:10.2174/1568026621666211015092654
Maeda, H., Ozawa, H., Saito, T., Irie, T., and Takahata, N. (1997). Potential Antidepressant Properties of Forskolin and a Novel Water-Soluble Forskolin (NKH477) in the Forced Swimming Test. Life Sci. 61 (25), 2435–2442. doi:10.1016/s0024-3205(97)00978-8
Maeda, M. H., and Kondo, K. (2013). Three-Dimensional Structure Database of Natural Metabolites (3DMET): A Novel Database of Curated 3D Structures. J. Chem. Inf. Model. 53 (3), 527–533. doi:10.1021/ci300309k
Maity, T., Adhikari, A., Bhattacharya, K., Biswas, S., Debnath, P. K., and Maharana, C. S. (2011). A Study on Evalution of Antidepressant Effect of Imipramine Adjunct with Aswagandha and Bramhi. Nepal Med. Coll. J. 13 (4), 250–253.
Mangal, M., Sagar, P., Singh, H., Raghava, G. P. S., and Agarwal, S. M. (2013). NPACT: Naturally Occurring Plant-Based Anti-cancer Compound-Activity-Target Database. Nucleic Acids Res. 41 (D1), D1124–D1129. doi:10.1093/nar/gks1047
Mannucci, C., Navarra, M., Calzavara, E., Caputi, A. P., and Calapai, G. (2012). Serotonin Involvement in Rhodiola Rosea Attenuation of Nicotine Withdrawal Signs in Rats. Phytomedicine 19 (12), 1117–1124. doi:10.1016/j.phymed.2012.07.001
Mao, Q.-Q., Ip, S.-P., Tsai, S.-H., and Che, C.-T. (2008a). Antidepressant-like Effect of Peony Glycosides in Mice. J. Ethnopharmacology 119 (2), 272–275. doi:10.1016/j.jep.2008.07.008
Mao, Q., Huang, Z., Ip, S., and Che, C. (2008b). Antidepressant-like Effect of Ethanol Extract fromPaeonia Lactiflorain Mice. Phytotherapy Res. 22 (11), 1496–1499. doi:10.1002/ptr.2519
Martins, J. (2018). Phytochemistry and Pharmacology of Anti-depressant Medicinal Plants: A Review. Biomed. Pharmacother. 104, 343–365. doi:10.1016/j.biopha.2018.05.044
McGuire, A. L., Gabriel, S., Tishkoff, S. A., Wonkam, A., Chakravarti, A., Furlong, E. E. M., et al. (2020). The Road Ahead in Genetics and Genomics. Nat. Rev. Genet. 21 (10), 581–596. doi:10.1038/s41576-020-0272-6
Mehbub, M., Lei, J., Franco, C., and Zhang, W. (2014). Marine Sponge Derived Natural Products between 2001 and 2010: Trends and Opportunities for Discovery of Bioactives. Mar. Drugs 12 (8), 4539–4577. doi:10.3390/md12084539
Melo, F. H. C., Moura, B. A., de Sousa, D. P., de Vasconcelos, S. M. M., Macedo, D. S., Fonteles, M. M. d. F., et al. (2011). Antidepressant-like Effect of Carvacrol (5-Isopropyl-2-Methylphenol) in Mice: Involvement of Dopaminergic System. Fundam. Clin. Pharmacol. 25 (3), 362–367. doi:10.1111/j.1472-8206.2010.00850.x
Menke, A. (2018). Precision Pharmacotherapy: Psychiatry’s Future Direction in Preventing, Diagnosing, and Treating Mental Disorders. Pharmacogenomics Personalized Med. 11, 211–222. doi:10.2147/pgpm.S146110
Mennen, A. C., Turk-Browne, N. B., Wallace, G., Seok, D., Jaganjac, A., Stock, J., et al. (2021). Cloud-Based Functional Magnetic Resonance Imaging Neurofeedback to Reduce the Negative Attentional Bias in Depression: A Proof-Of-Concept Study. Biol. Psychiatry Cogn. Neurosci. Neuroimaging 6 (4), 490–497. doi:10.1016/j.bpsc.2020.10.006
Miao, Z., Bai, J., Shen, L., and Singla, R. K. (2021). The Combination of Tradition and Future: Data-Driven Natural-Product-Based Treatments for Parkinson's Disease. Evid. Based Complement. Alternat Med. 2021, 9990020. doi:10.1155/2021/9990020
Miettinen, K., Iñigo, S., Kreft, L., Pollier, J., De Bo, C., Botzki, A., et al. (2018). The TriForC Database: a Comprehensive Up-To-Date Resource of Plant Triterpene Biosynthesis. Nucleic Acids Res. 46 (D1), D586–D594. doi:10.1093/nar/gkx925
Mikulak-Klucznik, B., Gołębiowska, P., Bayly, A. A., Popik, O., Klucznik, T., Szymkuć, S., et al. (2020). Computational Planning of the Synthesis of Complex Natural Products. Nature 588 (7836), 83–88. doi:10.1038/s41586-020-2855-y
Milev, R. V., Giacobbe, P., Kennedy, S. H., Blumberger, D. M., Daskalakis, Z. J., Downar, J., et al. (2016). Canadian Network for Mood and Anxiety Treatments (CANMAT) 2016 Clinical Guidelines for the Management of Adults with Major Depressive Disorder. Can. J. Psychiatry 61 (9), 561–575. doi:10.1177/0706743716660033
Misra, B. B., Langefeld, C., Olivier, M., and Cox, L. A. (2019). Integrated Omics: Tools, Advances and Future Approaches. J. Mol. Endocrinol. 62 (1), R21–R45. doi:10.1530/jme-18-0055
Mohanraj, K., Karthikeyan, B. S., Vivek-Ananth, R. P., Chand, R. P. B., Aparna, S. R., Mangalapandi, P., et al. (2018). IMPPAT: A Curated Database of Indian Medicinal Plants, Phytochemistry and Therapeutics. Scientific Rep. 8. doi:10.1038/s41598-018-22631-z
Moore, B. S., Carter, G. T., and Brönstrup, M. (2017). Editorial: Are Natural Products the Solution to Antimicrobial Resistance? Nat. Product. Rep. 34 (7), 685–686. doi:10.1039/c7np90026k
Mukhtar, E. J. A., Selman, S. M., Sahib, Z. H., and Naji, H. (2013). Antidepressant-like Effect of Rosmarinus Officinalis Extract in Male Mice. Med. J. Babylon. 10, 803–808.
Mukhtar, H. M., and Singh, H. (2020). Pharmacological and Phytochemical Updates on Citrullus colocynthis & Citrullus Lanata. Indo Glob. J. Pharm. Sci. 10 (04), 10–18. doi:10.35652/igjps.2020.10403
Myers, A. J., Gerring, Z. F., Gamazon, E. R., and Derks, E. M. (2019). A Gene Co-expression Network-Based Analysis of Multiple Brain Tissues Reveals Novel Genes and Molecular Pathways Underlying Major Depression. PLOS Genet. 15. doi:10.1371/journal.pgen.1008245
Nabirotchkin, S., Peluffo, A. E., Rinaudo, P., Yu, J., Hajj, R., and Cohen, D. (2020). Next-generation Drug Repurposing Using Human Genetics and Network Biology. Curr. Opin. Pharmacol. 51, 78–92. doi:10.1016/j.coph.2019.12.004
Naghizadeh, A., Hamzeheian, D., Akbari, S., Mohammadi, F., Otoufat, T., Asgari, S., et al. (2020). UNaProd: A Universal Natural Product Database for Materia Medica of Iranian Traditional Medicine. Evidence-Based Complement. Altern. Med. 2020, 1–14. doi:10.1155/2020/3690781
Nakazawa, T., Yasuda, T., Ueda, J., and Ohsawa, K. (2003). Antidepressant-Like Effects of Apigenin and 2,4,5-Trimethoxycinnamic Acid from Perilla Frutescens in the Forced Swimming Test. Biol. Pharm. Bull. 26 (4), 474–480. doi:10.1248/bpb.26.474
Newman, D. J., and Cragg, G. M. (2016). Natural Products as Sources of New Drugs from 1981 to 2014. J. Nat. Prod. 79 (3), 629–661. doi:10.1021/acs.jnatprod.5b01055
Newman, D. J., and Cragg, G. M. (2020). Natural Products as Sources of New Drugs over the Nearly Four Decades from 01/1981 to 09/2019. J. Nat. Prod. 83 (3), 770–803. doi:10.1021/acs.jnatprod.9b01285
Newman, D. J., Cragg, G. M., and Snader, K. M. (2003). Natural Products as Sources of New Drugs over the Period 1981−2002. J. Nat. Prod. 66 (7), 1022–1037. doi:10.1021/np030096l
Nicholson, D. N., and Greene, C. S. (2020). Constructing Knowledge Graphs and Their Biomedical Applications. Comput. Struct. Biotechnol. J. 18, 1414–1428. doi:10.1016/j.csbj.2020.05.017
Nielsen, N. D., Sandager, M., Stafford, G. I., van Staden, J., and Jäger, A. K. (2004). Screening of Indigenous Plants from South Africa for Affinity to the Serotonin Reuptake Transport Protein. J. Ethnopharmacology 94 (1), 159–163. doi:10.1016/j.jep.2004.05.013
Nierenberg, A. A. (2012). Advancing the Treatment of Depression with Personalized Medicine. J. Clin. Psychiatry 73. doi:10.4088/JCP.11031tx3c
Ntie-Kang, F., Telukunta, K. K., Döring, K., Simoben, C. V., Moumbock, A. F., Malange, Y. I., et al. (2017). NANPDB: A Resource for Natural Products from Northern African Sources. J. Nat. Prod. 80 (7), 2067–2076. doi:10.1021/acs.jnatprod.7b00283
Ovens, K., Maleki, F., Eames, B. F., and McQuillan, I. (2021). A Gene-Embedding Approach for Comparing Co-expression Networks. BMC Bioinformatics 22. doi:10.1186/s12859-021-04055-1
Pan, S.-Y., Litscher, G., Gao, S.-H., Zhou, S.-F., Yu, Z.-L., Chen, H.-Q., et al. (2014). Historical Perspective of Traditional Indigenous Medical Practices: The Current Renaissance and Conservation of Herbal Resources. Evidence-Based Complement. Altern. Med. 2014, 1–20. doi:10.1155/2014/525340
Paul, D., Sanap, G., Shenoy, S., Kalyane, D., Kalia, K., and Tekade, R. K. (2021). Artificial Intelligence in Drug Discovery and Development. Drug Discov. Today 26 (1), 80–93. doi:10.1016/j.drudis.2020.10.010
Pedersen, M. E., Szewczyk, B., Stachowicz, K., Wieronska, J., Andersen, J., Stafford, G. I., et al. (2008). Effects of South African Traditional Medicine in Animal Models for Depression. J. Ethnopharmacology 119 (3), 542–548. doi:10.1016/j.jep.2008.08.030
Pemminati, S., Gopalakrishna, H. N., Shenoy, A. K., Sahu, S. S., Mishra, S., Meti, V., et al. (2010). Antidepressant Activity of Aqueous Extract of Fruits of Emblica Officinalis in Mice. Int. J. Appl. Biol. Pharm. 1 (2), 448–454.
Piato, Â. L., Rizon, L. P., Martins, B. S., Nunes, D. S., and Elisabetsky, E. (2009). Antidepressant Profile of Ptychopetalum Olacoides Bentham (Marapuama) in Mice. Phytotherapy Res. 23 (4), 519–524. doi:10.1002/ptr.2664
Pilon, A. C., Valli, M., Dametto, A. C., Pinto, M. E. F., Freire, R. T., Castro-Gamboa, I., et al. (2017). NuBBEDB: an Updated Database to Uncover Chemical and Biological Information from Brazilian Biodiversity. Scientific Rep. 7. doi:10.1038/s41598-017-07451-x
Potshangbam, A. M., Polavarapu, R., Rathore, R. S., Naresh, D., Prabhu, N. P., Potshangbam, N., et al. (2018). MedPServer: A Database for Identification of Therapeutic Targets and Novel Leads Pertaining to Natural Products. Chem. Biol. Drug Des. 93 (4), 438–446. doi:10.1111/cbdd.13430
Prachayasittikul, V., Worachartcheewan, A., Shoombuatong, W., Songtawee, N., Simeon, S., Prachayasittikul, V., et al. (2015). Computer-Aided Drug Design of Bioactive Natural Products. Curr. Top. Med. Chem. 15 (18), 1780–1800. doi:10.2174/1568026615666150506151101
Pratap, S. R., Ritesh, J., Rahul, M., and Prashant, T. (2012). Antidepressant Activity of Hydroalcoholic Extract of Zingiber Officinale. Int. Res. J. Pharm. 3, 149–151.
Qiu, F., Zhong, X., Mao, Q., and Huang, Z. (2013). The Antidepressant-like Effects of Paeoniflorin in Mouse Models. Exp. Ther. Med. 5 (4), 1113–1116. doi:10.3892/etm.2013.925
Ramirez-Mahaluf, J. P., Roxin, A., Mayberg, H. S., and Compte, A. (2015). A Computational Model of Major Depression: the Role of Glutamate Dysfunction on Cingulo-Frontal Network Dynamics. Cereb. Cortex 27 (1), 660–679. doi:10.1093/cercor/bhv249
Ren, L.-X., Luo, Y.-F., Li, X., Zuo, D.-Y., and Wu, Y.-L. (2006). Antidepressant-Like Effects of Sarsasapogenin from Anemarrhena Asphodeloides BUNGE (Liliaceae). Biol. Pharm. Bull. 29 (11), 2304–2306. doi:10.1248/bpb.29.2304
Roberson, E. D., English, J. D., Adams, J. P., Selcher, J. C., Kondratick, C., and Sweatt, J. D. (1999). The Mitogen-Activated Protein Kinase cascade Couples PKA and PKC to cAMP Response Element Binding Protein Phosphorylation in Area CA1 of hippocampus. J. Neurosci. 19 (11), 4337–4348. doi:10.1523/jneurosci.19-11-04337.1999
Robinson, E. S. J. (2018). Translational New Approaches for Investigating Mood Disorders in Rodents and what They May Reveal about the Underlying Neurobiology of Major Depressive Disorder. Philosophical Trans. R. Soc. B: Biol. Sciences(1742) 373, 20170036–20170047. doi:10.1098/rstb.2017.0036
Rodrigues, A. L. S., da Silva, G. L., Mateussi, A. S., Fernandes, E. S., Miguel, O. G., Yunes, R. A., et al. (2002). Involvement of Monoaminergic System in the Antidepressant-like Effect of the Hydroalcoholic Extract of Siphocampylus Verticillatus. Life Sci. 70 (12), 1347–1358. doi:10.1016/s0024-3205(01)01498-9
Romano, J. D., and Tatonetti, N. P. (2019). Informatics and Computational Methods in Natural Product Drug Discovery: A Review and Perspectives. Front. Genet. 10, 368. doi:10.3389/fgene.2019.00368
Ru, J., Li, P., Wang, J., Zhou, W., Li, B., Huang, C., et al. (2014). TCMSP: a Database of Systems Pharmacology for Drug Discovery from Herbal Medicines. J. Cheminformatics 6, 13. doi:10.1186/1758-2946-6-13
Sah, S. P., Mathela, C. S., and Chopra, K. (2011a). Antidepressant Effect of Valeriana Wallichii Patchouli Alcohol Chemotype in Mice: Behavioural and Biochemical Evidence. J. Ethnopharmacology 135 (1), 197–200. doi:10.1016/j.jep.2011.02.018
Sah, S. P., Mathela, C. S., and Chopra, K. (2011b). Involvement of Nitric Oxide (NO) Signalling Pathway in the Antidepressant Activity of Essential Oil of Valeriana Wallichii Patchouli Alcohol Chemotype. Phytomedicine 18 (14), 1269–1275. doi:10.1016/j.phymed.2011.06.009
Sairam, K., Dorababu, M., Goel, R. K., and Bhattacharya, S. K. (2002). Antidepressant Activity of Standardized Extract of Bacopa Monniera in Experimental Models of Depression in Rats. Phytomedicine 9 (3), 207–211. doi:10.1078/0944-7113-00116
Sakakibara, H., Yoshino, S., Kawai, Y., and Terao, J. (2014). Antidepressant-Like Effect of Onion (Allium cepaL.) Powder in a Rat Behavioral Model of Depression. Biosci. Biotechnol. Biochem. 72 (1), 94–100. doi:10.1271/bbb.70454
Sarker, S. D., and Nahar, L. (2012). An Introduction to Natural Products Isolation. Nat. Prod. Isolation 1. doi:10.1007/978-1-61779-624-1_1
Sathya, R., and Arumugam, R. (2021). Phenolics, Antioxidant and Radical Scavenging Properties of Acanthus ilicifolius L. And Heliotropium Curassavicum L., of the Palk Bay Region, Tamilnadu. Indo Glob. J. Pharm. Sci. 11 (02), 92–99. doi:10.35652/igjps.2021.112003
Schaduangrat, N., Lampa, S., Simeon, S., Gleeson, M. P., Spjuth, O., and Nantasenamat, C. (2020). Towards Reproducible Computational Drug Discovery. J. Cheminformatics 12(1). doi:10.1186/s13321-020-0408-x
Schneider, G. (2017). Automating Drug Discovery. Nat. Rev. Drug Discov. 17 (2), 97–113. doi:10.1038/nrd.2017.232
Schumann, G., Binder, E. B., Holte, A., de Kloet, E. R., Oedegaard, K. J., Robbins, T. W., et al. (2014). Stratified Medicine for Mental Disorders. Eur. Neuropsychopharmacol. 24 (1), 5–50. doi:10.1016/j.euroneuro.2013.09.010
Segler, M. H. S., Preuss, M., and Waller, M. P. (2018). Planning Chemical Syntheses with Deep Neural Networks and Symbolic AI. Nature 555 (7698), 604–610. doi:10.1038/nature25978
Senior, A. W., Evans, R., Jumper, J., Kirkpatrick, J., Sifre, L., Green, T., et al. (2019). Protein Structure Prediction Using Multiple Deep Neural Networks in the 13th Critical Assessment of Protein Structure Prediction (CASP13). Proteins: Struct. Funct. Bioinformatics 87 (12), 1141–1148. doi:10.1002/prot.25834
Serretti, A. (2018). The Present and Future of Precision Medicine in Psychiatry: Focus on Clinical Psychopharmacology of Antidepressants. Clin. Psychopharmacol. Neurosci. 16 (1), 1–6. doi:10.9758/cpn.2018.16.1.1
Seyhan, A. A., and Carini, C. (2019). Are Innovation and New Technologies in Precision Medicine Paving a new era in Patients Centric Care? J. Translational Med. 17, 9. doi:10.1186/s12967-019-1864-9
Shadrina, M., Bondarenko, E. A., and Slominsky, P. A. (2018). Genetics Factors in Major Depression Disease. Front. Psychiatry 9, 334. doi:10.3389/fpsyt.2018.00334
Shameer, K., Badgeley, M. A., Miotto, R., Glicksberg, B. S., Morgan, J. W., and Dudley, J. T. (2017). Translational Bioinformatics in the Era of Real-Time Biomedical, Health Care and Wellness Data Streams. Brief. Bioinform. 18 (1), 105–124. doi:10.1093/bib/bbv118
Sharma, A., Dutta, P., Sharma, M., Rajput, N. K., Dodiya, B., Georrge, J. J., et al. (2014). BioPhytMol: a Drug Discovery Community Resource on Anti-mycobacterial Phytomolecules and Plant Extracts. J. Cheminformatics 6. doi:10.1186/s13321-014-0046-2
Sheik Amamuddy, O., Veldman, W., Manyumwa, C., Khairallah, A., Agajanian, S., Oluyemi, O., et al. (2020). Integrated Computational Approaches and Tools for Allosteric Drug Discovery. Int. J. Mol. Sci. 21(3). doi:10.3390/ijms21030847
Shen, B., Lin, Y., Bi, C., Zhou, S., Bai, Z., Zheng, G., et al. (2019). Translational Informatics for Parkinson's Disease: from Big Biomedical Data to Small Actionable Alterations. Genomics Proteomics Bioinformatics 17 (4), 415–429. doi:10.1016/j.gpb.2018.10.007
Shen, L., Bai, J., Wang, J., and Shen, B. (2021). The Fourth Scientific Discovery Paradigm for Precision Medicine and Healthcare: Challenges Ahead. Precision Clin. Med. 4 (2), 80–84. doi:10.1093/pcmedi/pbab007
Shen, L., Shen, K., Bai, J., Wang, J., Singla, R. K., and Shen, B. (2020). Data-driven Microbiota Biomarker Discovery for Personalized Drug Therapy of Cardiovascular Disease. Pharmacol. Res. 161, 105225. doi:10.1016/j.phrs.2020.105225
Sibi, P. I., and Meera, P. (2013). In Silico docking Analysis of Constituents of Zingiber Officinale as Antidepressant. J. Pharmacognosy Phytotherapy 5 (6), 101–105. doi:10.5897/jpp2013.0280
Silverman, E. K., Schmidt, H. H. H. W., Anastasiadou, E., Altucci, L., Angelini, M., Badimon, L., et al. (2020). Molecular Networks in Network Medicine: Development and Applications. WIREs Syst. Biol. Med. 12 (6). doi:10.1002/wsbm.1489
Singh, G. K., Garabadu, D., Muruganandam, A. V., Joshi, V. K., and Krishnamurthy, S. (2009). Antidepressant Activity of Asparagus racemosus in Rodent Models. Pharmacol. Biochem. Behav. 91 (3), 283–290. doi:10.1016/j.pbb.2008.07.010
Singh, N., Chaput, L., and Villoutreix, B. O. (2021). Virtual Screening Web Servers: Designing Chemical Probes and Drug Candidates in the Cyberspace. Brief. Bioinform. 22 (2), 1790–1818. doi:10.1093/bib/bbaa034
Singh, R., Ramakrishna, R., Bhateria, M., and Bhatta, R. S. (2014). In Vitro Evaluation of Bacopa Monniera Extract and Individual Constituents on Human Recombinant Monoamine Oxidase Enzymes. Phytotherapy Res. 28 (9), 1419–1422. doi:10.1002/ptr.5116
Singla, R. K. (2014). Mechanistic Evidence to Support the Anti-hepatitis B Viral Activity of Multifunctional Scaffold & Conformationally Restricted Magnolol. Natl. Acad. Sci. Lett. 37 (1), 45–50. doi:10.1007/s40009-013-0195-2
Singla, R. K. (2021). Secondary Metabolites as Treatment of Choice for Metabolic Disorders and Infectious Diseases and Their Metabolic Profiling - Part 3. Curr. Drug Metab. 22 (6), 412–414. doi:10.2174/138920022206210708103019
Singla, R. K., Agarwal, T., He, X., and Shen, B. (2021a). Herbal Resources to Combat a Progressive & Degenerative Nervous System Disorder- Parkinson’s Disease. Curr. Drug Targets 22 (6), 609–630. doi:10.2174/1389450121999201013155202
Singla, R. K., and Dubey, A. K. (2019). Molecules and Metabolites from Natural Products as Inhibitors of Biofilm in Candida Spp. Pathogens. Curr. Top. Med. Chem. 19 (28), 2567–2578. doi:10.2174/1568026619666191025154834
Singla, R. K., Guimaraes, A. G., and Zengin, G. (2020). Editorial: Application of Plant Secondary Metabolites to Pain Neuromodulation. Front. Pharmacol. 11, 623399. doi:10.3389/fphar.2020.623399
Singla, R. K., Gupta, R., Joon, S., Gupta, A. K., and Shen, B. (2021b). Isolation, Docking and In Silico ADME-T Studies of Acacianol: Novel Antibacterial Isoflavone Analogue Isolated from Acacia Leucophloea Bark. Curr. Drug Metab. doi:10.2174/1389200222666211005091417
Singla, R. K., Scotti, L., and Dubey, A. K. (2017). Silico Studies Revealed Multiple Neurological Targets for the Antidepressant Molecule Ursolic Acid. Curr. Neuropharmacology 15. doi:10.2174/1570159x14666161229115508
Singla, R. K., Sharma, P., Dubey, A. K., Gundamaraju, R., Kumar, D., Kumar, S., et al. (2021c). Natural Product-Based Studies for the Management of Castration-Resistant Prostate Cancer: Computational to Clinical Studies. Front. Pharmacol. 12. doi:10.3389/fphar.2021.732266
Siqueira, I. R., Cimarosti, H., Fochesatto, C. n., Nunes, D. S., Salbego, C., Elisabetsky, E., et al. (2004). Neuroprotective Effects of Ptychopetalum Olacoides Bentham (Olacaceae) on Oxygen and Glucose Deprivation Induced Damage in Rat Hippocampal Slices. Life Sci. 75 (15), 1897–1906. doi:10.1016/j.lfs.2004.06.001
Smith, G. S., Gunning-Dixon, F. M., Lotrich, F. E., Taylor, W. D., and Evans, J. D. (2007). Translational Research in Late-Life Mood Disorders: Implications for Future Intervention and Prevention Research. Neuropsychopharmacology 32 (9), 1857–1875. doi:10.1038/sj.npp.1301333
Somvanshi, P. R., and Venkatesh, K. V. (2013). A Conceptual Review on Systems Biology in Health and Diseases: from Biological Networks to Modern Therapeutics. Syst. Synth. Biol. 8 (1), 99–116. doi:10.1007/s11693-013-9125-3
Sonawane, A. R., Weiss, S. T., Glass, K., and Sharma, A. (2019). Network Medicine in the Age of Biomedical Big Data. Front. Genet. 10. doi:10.3389/fgene.2019.00294
Song, C. M., Lim, S. J., and Tong, J. C. (2009). Recent Advances in Computer-Aided Drug Design. Brief. Bioinform. 10 (5), 579–591. doi:10.1093/bib/bbp023
Sorokina, M., Merseburger, P., Rajan, K., Yirik, M. A., and Steinbeck, C. (2021). COCONUT Online: Collection of Open Natural Products Database. J. Cheminformatics 13, 9. doi:10.1186/s13321-020-00478-9
Sousa, F. C. F., Melo, C. T. V., Monteiro, A. P., Lima, V. T. M., Gutierrez, S. J. C., Pereira, B. A., et al. (2004). Antianxiety and Antidepressant Effects of Riparin III from Aniba Riparia (Nees) Mez (Lauraceae) in Mice. Pharmacol. Biochem. Behav. 78 (1), 27–33. doi:10.1016/j.pbb.2004.01.019
Subarnas, A., Oshima, Y., and Ohizumi, Y. (1992). An Antidepressant Principle of Lobelia Inflata L. (Campanulaceae). J. Pharm. Sci. 81 (7), 620–621. doi:10.1002/jps.2600810705
Subarnas, A., Tadano, T., Nakahata, N., Arai, Y., Kinemuchi, H., Oshima, Y., et al. (1993). A Possible Mechanism of Antidepresant Activity of Beta-Amyrin Palmitate Isolated from lobelia Inflata Leaves in the Forced Swimming Test. Life Sci. 52 (3), 289–296. doi:10.1016/0024-3205(93)90220-w
Subhan, F., Karim, N., Gilani, A. H., and Sewell, R. D. E. (2010). Terpenoid Content of Valeriana Wallichii Extracts and Antidepressant-like Response Profiles. Phytotherapy Res. 24 (5), 686–691. doi:10.1002/ptr.2980
Sun, Y., Lin, J., and Zhang, L. (2019). The Application of Weighted Gene Co-expression Network Analysis in Identifying Key Modules and Hub Genes Associated with Disease Status in Alzheimer’s Disease. Ann. Translational Med. 7 (24), 800. doi:10.21037/atm.2019.12.59
Suwinski, P., Ong, C., Ling, M. H. T., Poh, Y. M., Khan, A. M., and Ong, H. S. (2019). Advancing Personalized Medicine through the Application of Whole Exome Sequencing and Big Data Analytics10. Front. Genet. doi:10.3389/fgene.2019.00049
Swarnkar, S. K., Khunteta, A., Gupta, M. K., Jain, P., Sharma, S., and Paliwal, S. (2021). Antinociceptive Activity Shown by Aerva Javanica Flowering Top Extract and its Mechanistic Evaluation. Indo Glob. J. Pharm. Sci. 11 (01), 33–41. doi:10.35652/igjps.2021.111005
Teixeira, C. P. L., de Melo, C. T. V., de Araújo, F. L. O., de Carvalho, A. M. R., Silva, M. I. G., Barbosa-Filho, J. M., et al. (2013). Antidepressant-like Effect of Riparin II fromAniba Ripariain Mice: Evidence for the Involvement of the Monoaminergic System. Fundam. Clin. Pharmacol. 27 (2), 129–137. doi:10.1111/j.1472-8206.2011.00973.x
Tenenbaum, J. D. (2016). Translational Bioinformatics: Past, Present, and Future. Genomics, Proteomics & Bioinformatics 14 (1), 31–41. doi:10.1016/j.gpb.2016.01.003
Tomic, M., Tovilovic, G., Butorovic, B., Krstic, D., Jankovic, T., Aljancic, I., et al. (2005). Neuropharmacological Evaluation of Diethylether Extract and Xanthones of. Pharmacol. Biochem. Behav. 81 (3), 535–542. doi:10.1016/j.pbb.2005.03.019
Tran, B. X., McIntyre, R. S., Latkin, C. A., Phan, H. T., Vu, G. T., Nguyen, H. L. T., et al. (2019). The Current Research Landscape on the Artificial Intelligence Application in the Management of Depressive Disorders: A Bibliometric Analysis. Int. J. Environ. Res. Public Health 16(12). doi:10.3390/ijerph16122150
Truax, N. J., and Romo, D. (2020). Bridging the gap between Natural Product Synthesis and Drug Discovery. Nat. Product. Rep. 37 (11), 1436–1453. doi:10.1039/d0np00048e
Uebelhack, R., Franke, L., and Schewe, H. J. (2007). Inhibition of Platelet MAO-B by Kava Pyrone-Enriched Extract from Piper Methysticum Forster (Kava-Kava). Pharmacopsychiatry 31 (05), 187–192. doi:10.1055/s-2007-979325
UNESCO World Decade for Cultural Development, and Organization W.H (1996). World Decade for Cultural Development 1988-1997. Culture and Health: Orientation Texts on the 1996 Theme. Paris, France: UNESCO.
Unützer, J., and Park, M. (2012). Strategies to Improve the Management of Depression in Primary Care. Prim. Care Clin. Off. Pract. 39 (2), 415–431. doi:10.1016/j.pop.2012.03.010
Valdés-Jiménez, A., Peña-Varas, C., Borrego-Muñoz, P., Arrue, L., Alegría-Arcos, M., Nour-Eldin, H., et al. (2021). PSC-db: A Structured and Searchable 3D-Database for Plant Secondary Compounds Molecules 26 (4), 1124. doi:10.3390/molecules26041124
van Diermen, D., Marston, A., Bravo, J., Reist, M., Carrupt, P.-A., and Hostettmann, K. (2009). Monoamine Oxidase Inhibition by Rhodiola Rosea L. Roots. J. Ethnopharmacology 122 (2), 397–401. doi:10.1016/j.jep.2009.01.007
Varteresian, T., and Lavretsky, H. (2014). Natural Products and Supplements for Geriatric Depression and Cognitive Disorders: An Evaluation of the Research. Curr. Psychiatry Rep. 16, 456–465. doi:10.1007/s11920-014-0456-x
Vella, D., Zoppis, I., Mauri, G., Mauri, P., and Di Silvestre, D. (2017). From Protein-Protein Interactions to Protein Co-expression Networks: a New Perspective to Evaluate Large-Scale Proteomic Data. EURASIP J. Bioinformatics Syst. Biol. 2017(1). doi:10.1186/s13637-017-0059-z
Viana, A., do Rego, J.-C., von Poser, G., Ferraz, A., Heckler, A. P., Costentin, J., et al. (2005). The Antidepressant-like Effect of Hypericum Caprifoliatum Cham & Schlecht (Guttiferae) on Forced Swimming Test Results from an Inhibition of Neuronal Monoamine Uptake. Neuropharmacology 49 (7), 1042–1052. doi:10.1016/j.neuropharm.2005.06.002
Wachtel, H., and Loschmann, P. (1986). Effects of Forskolin and Cyclic Nucleotides in Animal Models Predictive of Antidepressant Activity: Interactions with Rolipram. Psychopharmacol. 90, 430–435. doi:10.1007/bf00174056
Wang, F., Cui, P., Zhu, Y., Tong, J., and Su, C. (2020). Network Embedding in Biomedical Data Science. Brief. Bioinform. 21 (1), 182–197. doi:10.1093/bib/bby117
Wang, J., Zhou, H., Han, L., Chen, X., Chen, Y., and Cao, Z. (2005). Traditional Chinese Medicine Information Database. Clin. Pharmacol. Ther. 78 (1), 92–93. doi:10.1016/j.clpt.2005.03.010
Wang, M. J., Jiang, L., Chen, H. S., and Cheng, L. (2019). Levetiracetam Protects against Cognitive Impairment of Subthreshold Convulsant Discharge Model Rats by Activating Protein Kinase C (PKC)-Growth-Associated Protein 43 (GAP-43)-Calmodulin-Dependent Protein Kinase (CaMK) Signal Transduction Pathway. Med. Sci. Monit. 25, 4627–4638. doi:10.12659/MSM.913542
Wang, P. S., Aguilar-Gaxiola, S., Alonso, J., Angermeyer, M. C., Borges, G., Bromet, E. J., et al. (2007). Use of Mental Health Services for Anxiety, Mood, and Substance Disorders in 17 Countries in the WHO World Mental Health Surveys. The Lancet 370 (9590), 841–850. doi:10.1016/s0140-6736(07)61414-7
Wang, R., Xu, Y., Wu, H.-L., Li, Y.-B., Li, Y.-H., Guo, J.-B., et al. (2008a). The Antidepressant Effects of Curcumin in the Forced Swimming Test Involve 5-HT1 and 5-HT2 Receptors. Eur. J. Pharmacol. 578 (1), 43–50. doi:10.1016/j.ejphar.2007.08.045
Wang, W., Hu, X., Zhao, Z., Liu, P., Hu, Y., Zhou, J., et al. (2008b). Antidepressant-like Effects of Liquiritin and Isoliquiritin from Glycyrrhiza Uralensis in the Forced Swimming Test and Tail Suspension Test in Mice. Prog. Neuro-Psychopharmacology Biol. Psychiatry 32 (5), 1179–1184. doi:10.1016/j.pnpbp.2007.12.021
Woo, S., and Shenvi, R. A. (2021). Natural Product Synthesis through the Lens of Informatics. Acc. Chem. Res. 54 (5), 1157–1167. doi:10.1021/acs.accounts.0c00791
World Health Organization (2013). WHO Traditional Medicine Strategy: 2014-2023. World Health Organization.
Wu, Y., Zhang, F., Yang, K., Fang, S., Bu, D., Li, H., et al. (2019). SymMap: an Integrative Database of Traditional Chinese Medicine Enhanced by Symptom Mapping. Nucleic Acids Res. 47 (D1), D1110–D1117. doi:10.1093/nar/gky1021
Wu, Z., Li, W., Liu, G., and Tang, Y. (2018). Network-Based Methods for Prediction of Drug-Target Interactions. Front. Pharmacol. 9. doi:10.3389/fphar.2018.01134
Xia, X. (2017). Bioinformatics and Drug Discovery. Curr. Top. Med. Chem. 17 (15), 1709–1726. doi:10.2174/1568026617666161116143440
Xu, Q., Pan, Y., Yi, L.-T., Li, Y.-C., Mo, S.-F., Jiang, F.-X., et al. (2008). Antidepressant-Like Effects of Psoralen Isolated from the Seeds of Psoralea Corylifolia in the Mouse Forced Swimming Test. Biol. Pharm. Bull. 31 (6), 1109–1114. doi:10.1248/bpb.31.1109
Xu, T., Chen, W., Zhou, J., Dai, J., Li, Y., and Zhao, Y. (2020). NPBS Database: A Chemical Data Resource with Relational Data between Natural Products and Biological Sources. Oxford: Oxford University Press. doi:10.1093/database/baaa102
Xu, Y., Ku, B., Cui, L., Li, X., Barish, P. A., Foster, T. C., et al. (2007). Curcumin Reverses Impaired Hippocampal Neurogenesis and Increases Serotonin Receptor 1A mRNA and Brain-Derived Neurotrophic Factor Expression in Chronically Stressed Rats. Brain Res. 1162, 9–18. doi:10.1016/j.brainres.2007.05.071
Xu, Y., Wang, Z., You, W., Zhang, X., Li, S., Barish, P. A., et al. (2010). Antidepressant-like Effect of Trans-resveratrol: Involvement of Serotonin and Noradrenaline System. Eur. Neuropsychopharmacol. 20 (6), 405–413. doi:10.1016/j.euroneuro.2010.02.013
Yabe, T., Hirahara, H., Harada, N., Ito, N., Nagai, T., Sanagi, T., et al. (2010). Ferulic Acid Induces Neural Progenitor Cell Proliferation In Vitro and In Vivo. Neuroscience 165 (2), 515–524. doi:10.1016/j.neuroscience.2009.10.023
Yan, W., Yu, C., Chen, J., Zhou, J., and Shen, B. (2020). ANCA: A Web Server for Amino Acid Networks Construction and Analysis. Front. Mol. Biosci. 7, 582702. doi:10.3389/fmolb.2020.582702
Ye, H., Ye, L., Kang, H., Zhang, D., Tao, L., Tang, K., et al. (2010). HIT: Linking Herbal Active Ingredients to Targets. Nucleic Acids Res. 39 (Database), D1055–D1059. doi:10.1093/nar/gkq1165
Yeung, K. S., Hernandez, M., Mao, J. J., Haviland, I., and Gubili, J. (2018). Herbal Medicine for Depression and Anxiety: A Systematic Review with Assessment of Potential Psycho-Oncologic Relevance. Phytotherapy Res. 32 (5), 865–891. doi:10.1002/ptr.6033
Yi, L.-T., Li, J., Geng, D., Liu, B.-B., Fu, Y., Tu, J.-Q., et al. (2013). Essential Oil of Perilla Frutescens-Induced Change in Hippocampal Expression of Brain-Derived Neurotrophic Factor in Chronic Unpredictable Mild Stress in Mice. J. Ethnopharmacology 147 (1), 245–253. doi:10.1016/j.jep.2013.03.015
Yi, L.-T., Li, Y.-C., Pan, Y., Li, J.-M., Xu, Q., Mo, S.-F., et al. (2008). Antidepressant-like Effects of Psoralidin Isolated from the Seeds of Psoralea Corylifolia in the Forced Swimming Test in Mice. Prog. Neuro-Psychopharmacology Biol. Psychiatry 32 (2), 510–519. doi:10.1016/j.pnpbp.2007.10.005
Yu, X., Zhang, J., Sun, S., Zhou, X., Zeng, T., and Chen, L. (2017). Individual-specific Edge-Network Analysis for Disease Prediction. Nucleic Acids Res. 45 (20), e170. doi:10.1093/nar/gkx787
Yu, Y., Wang, R., Chen, C., Du, X., Ruan, L., Sun, J., et al. (2013). Antidepressant-like Effect of Trans-resveratrol in Chronic Stress Model: Behavioral and Neurochemical Evidences. J. Psychiatr. Res. 47 (3), 315–322. doi:10.1016/j.jpsychires.2012.10.018
Yu, Z. F., Kong, L. D., and Chen, Y. (2002). Antidepressant Activity of Aqueous Extracts of Curcuma Longa in Mice. J. Ethnopharmacology 83 (1-2), 161–165. doi:10.1016/s0378-8741(02)00211-8
Yuan, H., Ma, Q., Ye, L., and Piao, G. (2016). The Traditional Medicine and Modern Medicine from Natural Products 21(5). Molecules. doi:10.3390/molecules21050559
Yue, Y., Chu, G.-X., Liu, X.-S., Tang, X., Wang, W., Liu, G.-J., et al. (2014). TMDB: A Literature-Curated Database for Small Molecular Compounds Found from tea. BMC Plant Biol. 14, 243–251. doi:10.1186/s12870-014-0243-1
Zani, C. L., and Carroll, A. R. (2017). Database for Rapid Dereplication of Known Natural Products Using Data from MS and Fast NMR Experiments. J. Nat. Prod. 80 (6), 1758–1766. doi:10.1021/acs.jnatprod.6b01093
Zeng, T., Liu, Z., Zhuang, J., Jiang, Y., He, W., Diao, H., et al. (2020). TeroKit: A Database-Driven Web Server for Terpenome Research. J. Chem. Inf. Model. 60 (4), 2082–2090. doi:10.1021/acs.jcim.0c00141
Zeng, X., Zhang, P., He, W., Qin, C., Chen, S., Tao, L., et al. (2018). NPASS: Natural Product Activity and Species Source Database for Natural Product Research, Discovery and Tool Development. Nucleic Acids Res. 46 (D1), D1217–D1222. doi:10.1093/nar/gkx1026
Zhang, Q.-W., Lin, L.-G., and Ye, W.-C. (2018a). Techniques for Extraction and Isolation of Natural Products: a Comprehensive Review. (1). Chin. Med. 13, 20–46. doi:10.1186/s13020-018-0177-x
Zhang, T.-T., Xue, R., Wang, X., Zhao, S.-W., An, L., Li, Y.-F., et al. (2018b). Network-based Drug Repositioning: A Novel Strategy for Discovering Potential Antidepressants and Their Mode of Action. Eur. Neuropsychopharmacol. 28 (10), 1137–1150. doi:10.1016/j.euroneuro.2018.07.096
Zhang, Z.-Q., Yuan, L., Yang, M., Luo, Z.-P., and Zhao, Y.-M. (2002). The Effect of Morinda Officinalis How, a Chinese Traditional Medicinal Plant, on the DRL 72-s Schedule in Rats and the Forced Swimming Test in Mice. Pharmacol. Biochem. Behav. 72 (1-2), 39–43. doi:10.1016/s0091-3057(01)00730-4
Zhao, D., Zheng, L., Qi, L., Wang, S., Guan, L., Xia, Y., et al. (2016). Structural Features and Potent Antidepressant Effects of Total Sterols and β-sitosterol Extracted from Sargassum Horneri. Mar. Drugs 14(7). doi:10.3390/md14070123
Zhao, Z., Wang, W., Guo, H., and Zhou, D. (2008). Antidepressant-like Effect of Liquiritin from Glycyrrhiza Uralensis in Chronic Variable Stress Induced Depression Model Rats. Behav. Brain Res. 194 (1), 108–113. doi:10.1016/j.bbr.2008.06.030
Zheng, M., Fan, Y., Shi, D., and Liu, C. (2013). Antidepressant-like Effect of Flavonoids Extracted from Apocynum Venetum Leaves on Brain Monoamine Levels and Dopaminergic System. J. Ethnopharmacology 147 (1), 108–113. doi:10.1016/j.jep.2013.02.015
Zhou, D., Jin, H., Lin, H.-B., Yang, X.-M., Cheng, Y.-F., Deng, F.-J., et al. (2010). Antidepressant Effect of the Extracts from Fructus Akebiae. Pharmacol. Biochem. Behav. 94 (3), 488–495. doi:10.1016/j.pbb.2009.11.003
Keywords: depression, natural products, translational informatics, clinical applications, natural antidepressants, neurological disorder
Citation: Singla RK, Joon S, Shen L and Shen B (2022) Translational Informatics for Natural Products as Antidepressant Agents. Front. Cell Dev. Biol. 9:738838. doi: 10.3389/fcell.2021.738838
Received: 09 July 2021; Accepted: 13 December 2021;
Published: 20 January 2022.
Edited by:
Guohui Lu, The First Affiliated Hospital of Nanchang University, ChinaReviewed by:
Monica Trif, Centre for innovative process engineering, GermanyCopyright © 2022 Singla, Joon, Shen and Shen. This is an open-access article distributed under the terms of the Creative Commons Attribution License (CC BY). The use, distribution or reproduction in other forums is permitted, provided the original author(s) and the copyright owner(s) are credited and that the original publication in this journal is cited, in accordance with accepted academic practice. No use, distribution or reproduction is permitted which does not comply with these terms.
*Correspondence: Bairong Shen, YmFpcm9uZy5zaGVuQHNjdS5lZHUuY24=
†These authors have contributed equally to this work and share first authorship
Disclaimer: All claims expressed in this article are solely those of the authors and do not necessarily represent those of their affiliated organizations, or those of the publisher, the editors and the reviewers. Any product that may be evaluated in this article or claim that may be made by its manufacturer is not guaranteed or endorsed by the publisher.
Research integrity at Frontiers
Learn more about the work of our research integrity team to safeguard the quality of each article we publish.