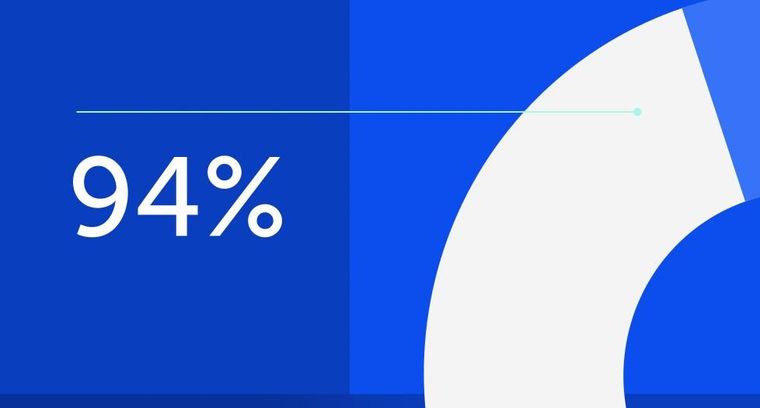
94% of researchers rate our articles as excellent or good
Learn more about the work of our research integrity team to safeguard the quality of each article we publish.
Find out more
ORIGINAL RESEARCH article
Front. Cell Dev. Biol., 04 October 2021
Sec. Epigenomics and Epigenetics
Volume 9 - 2021 | https://doi.org/10.3389/fcell.2021.722671
This article is part of the Research TopicFinding New Epigenomics and Epigenetics Biomarkers for Complex Diseases and Significant Developmental Events with Machine Learning MethodsView all 36 articles
Atrial fibrillation (AF)/paroxysmal AF (PAF) is the main cause of cardiogenic embolism. In recent years, the progression from paroxysmal AF to persistent AF has attracted more and more attention. However, the molecular mechanism of the progression of AF is unclear. In this study, we performed RNA sequencing for normal samples, paroxysmal AF and persistent AF samples to identify differentially expressed gene (DEG) and explore the roles of these DEGs in AF. Totally, 272 differently expressed mRNAs (DEmRNAs) and 286 differentially expressed lncRNAs (DElncRNAs) were identified in paroxysmal AF compared to normal samples; 324 DEmRNAs and 258 DElncRNAs were found in persistent atrial fibrillation compared with normal samples; and 520 DEmRNAs and 414 DElncRNAs were identified in persistent AF compared to paroxysmal AF samples. Interestingly, among the DEGs, approximately 50% were coding genes and around 50% were non-coding RNAs, suggesting that lncRNAs may also have a crucial role in the progression of AF. Bioinformatics analysis demonstrated that these DEGs were significantly related to regulating multiple AF associated pathways, such as the regulation of vascular endothelial growth factor production and binding to the CXCR chemokine receptor. Furthermore, weighted gene co-expression network analysis (WGCNA) was conducted to identify key modules and hub RNAs and lncRNAs to determine their potential associations with AF. Five hub modules were identified in the progression of AF, including blue, brown, gray, turquoise and yellow modules. Interestingly, blue module and turquoise module were significantly negatively and positively correlated to the progression of AF respectively, indicating that they may have a more important role in the AF. Moreover, the hub protein-protein interaction (PPI) networks and lncRNA–mRNA regulatory network were constructed. Bioinformatics analysis on the hub PPI network in turquoise was involved in regulating immune response related signaling, such as leukocyte chemotaxis, macrophage activation, and positive regulation of α-β T cell activation. Our findings could clarify the underlying molecular changes associated fibrillation, and provide a useful resource for identifying AF marker.
Atrial fibrillation (AF) is a common tachyarrhythmia, which had been the main cause of cardiogenic embolic infarction (Abdul-Rahim et al., 2015; Kelley, 2015). AF develop from paroxysmal AF to persistent AF (Shukla and Curtis, 2014). Paroxysmal AF occurs in about 50% of all AF cases (Skaarup et al., 2016). Persistent AF occurs in about 20% of chronic heart failure and is related to a poor prognosis (Cornelis et al., 2018). In the past 10 years, AF ablation has been a common treatment of AF (Pelargonio et al., 2021). Previous studies have shown that AF is a complex disease caused by genetic and environmental factors (Lubitz et al., 2010). In the past few decades, some regulators related to AF have been discovered, such as NLRP3 (Yao et al., 2018), JPH2 (Beavers et al., 2013) and microRNA-26 (miR-26) (Luo et al., 2013). Knockout of NLRP3 inhibits the development of AF (Yao et al., 2018). MicroRNA-26 regulates AF and promotes changes in the inward rectifier potassium current of AF (Luo et al., 2013). However, the underlying mechanism of the progression of AF remains unclear. Understanding the molecular mechanism of AF will help to identify biomarkers for the early diagnosis and treatment for AF.
LncRNA is a set of non-coding transcripts longer than 200 nucleotides (Shi et al., 2020a; Statello et al., 2021). In recent years, lncRNA has been confirmed to have a crucial role in a variety of cell functions, including epigenetic regulation, transcription regulation, etc., and has been potential biomarkers for disease diagnosis and treatment (Cao, 2014; Gu and Chen, 2020). In human cells, it has been identified more than 100,000 lncRNAs (Statello et al., 2021), which play an important role in the cardiovascular system. However, only a small number of lncRNA functions have been studied in AF. For example, in AF, the lncRNA LICPAR modulates atrial fibrosis through Smad signaling (Wang et al., 2020). LncRNA NEAT1 regulates atrial fibrosis during AF through the miR-320/Npas2 axis (Dai et al., 2021). LncRNA-MIAT regulates AF-induced myocardial fibrosis through miR-133a-3p (Yao et al., 2020). LncRNA-PVT1 modulates atrial fibrosis during AF through miR-128 (Cao et al., 2019). To understand the expression patterns and possible functions of lncRNAs in AF could provide helpful information for the treatment of AF.
Weighted gene co-expression network analysis (WGCNA) is used to cluster highly related genes to further understand the hub modules and disease types/clinical phenotypes (Langfelder and Horvath, 2008). In recent years, WGCNA has been used to identify key regulators in disease progression. For example, Ren et al. (2021) performed WGCNA analysis to identify diagnostic genes and important microRNAs associated with rheumatoid arthritis. Here, we performed RNA sequencing to identify differently expressed mRNAs (DEmRNAs) and lncRNAs in normal samples, paroxysmal AF, and persistent AF. In addition, we use bioinformatics methods, such as WGCNA, and PPI network analysis, to identify the hub lncRNAs and mRNAs in AF. Our findings aim to clarify the underlying molecular changes associated fibrillation, and provide a useful resource for identifying AF marker.
In this study, 10 control samples, 10 paroxysmal AF, and 10 persistent AF samples were prepared for RNA sequencing. RNA was extracted from Approximately 500 mg AF and normal samples using the RNeasy mini kit (QIAGEN).
We next applied Corall Total RNA Seq library preparation kit (Lexogen, Vienna, Austria) for RNA Seq library using 150 ng of total RNA. The RiboCop rRNA Depletion Kit (Lexogen, Vienna, Austria) was used to remove rRNA. The quality of the sequencing library was evaluated by D1000 screen tape analysis using the 4200 TapeStation system (Agilent, United States) and quantified using QubitTM dsDNA HS analysis kit (Invitrogen, United States). RNA processing was used by Illumina NextSeq 500 sequencing. The R software package Deseq2 was used for mRNA differential expression analysis (Zhang et al., 2020). The genes with |log2 fold change | ≥ 1 and FDR ≤ 0.1 were considered to be differentially expressed (Gu et al., 2021a,b).
A scale-free co-expression network was constructed by using WGCNA package in R (Langfelder and Horvath, 2008). The appropriate soft threshold power (β) is determined based on a scale-free topology criterion. The result was clustered by topological overlap matrix analysis. In addition, the correlations between module eigengenes (MEs) were calculated via Pearson’s correlation analysis.
In order to explore the functional annotation of DEGs, we performed gene ontology (GO) (Gu et al., 2020a), kyoto encyclopedia of genes and genomes (KEGG) (Gu et al., 2020b; Liang et al., 2020) and UniProt analysis to predict gene functions using DAVID system (Jiao et al., 2012; Shi et al., 2020b).
We used the STRING to construct a PPI network (Shi et al., 2018). The PPI network was visualized through Cytoscape, and further filtered through plug-in molecular complex detection (MCODE) to determine the candidate hub differentially expressed module (DEM) (Feng et al., 2019). The biological significance of gene modules was visualized with MCODE in Cytoscape to identify the most significant module (Gu et al., 2020c).
Cytoscape displays the co-expression network of hub lncRNAs through the topological analysis of lncRNAs, the central nodes of these networks were explored. The significantly co-expression network of hub lncRNA-mRNAs with an absolute Pearson correlation coefficient >0.99 was chosen as the targets to build the network.
The WGCNA package in R was used to generate a co-expression network of DEGs (Langfelder and Horvath, 2008). Pearson’s correlation analysis was conducted as a similarity measure. The soft threshold power (β) of the correlation matrix was used to emphasize strong correlations between genes and penalize weak correlations. Next, the adjacency was used to calculate the topological overlap matrix (TOM).
In this study, 10 control samples, 10 paroxysmal AF, and 10 persistent AF samples were sequenced among their RNA. Then, we identified DEGs in the progression of AF with the R package Limma. 558 genes were identified to be differently expressed in paroxysmal AF compared to control samples (Figures 1A,B); 582 genes were identified to be differently expressed in persistent AF compared to control samples (Figures 1C,D); and 934 genes were identified to be differently expressed in persistent AF compared to paroxysmal AF tissues (Figures 1E,F). The heat map and volcano map of the DEG are shown in Figure 1.
Figure 1. Identification of DEGs among normal, paroxysmal AF and persistent AF samples. (A,B) The scatter plot and volcano plot show the DEGs in paroxysmal AF compared to normal samples. (C,D) The scatter plot and volcano plot show the DEGs in persistent AF compared to normal samples. (E,F) The scatter plot and volcano plot show the DEGs in persistent AF compared to paroxysmal AF samples.
Interestingly, we found that the expression of various lncRNAs in the progression of atrial fibrillation was differently changed. Among the DEGs, about 50% were coding genes and around 50% were non-coding RNAs (Figure 2). Compared with normal samples, 272 DEGs were found in patients with paroxysmal atrial fibrillation, including 100 upregulated and 172 downregulated mRNAs (Figure 2A). Overall, 324 DEmRNAs were found in persistent atrial fibrillation compared with normal samples, including 219 upregulated and 105 downregulated mRNAs (Figure 2B). In addition, compared with paroxysmal atrial fibrillation samples, 520 genes were identified in persistent atrial fibrillation samples, including 281 upregulated genes and 239 downregulated genes (Figure 2C).
Figure 2. Identification of DEmRNAs and lncRNAs among normal, paroxysmal AF and persistent AF samples. (A–C) The heat map shows the DEmRNAs in paroxysmal AF compared to normal samples (A), persistent AF compared to normal samples (B), and persistent AF compared to paroxysmal AF samples (C). (D–F) The heat map shows the differently expressed lncRNAs in paroxysmal AF compared to normal samples (A), persistent AF compared to normal samples (B), and persistent AF compared to paroxysmal AF samples (C).
Therefore, we also focused on the expression changes and potential functions in AF. As shown in Figure 1, compared with normal samples, a total of 286 DElncRNAs were identified in paroxysmal atrial fibrillation, and 116 lncRNAs were upregulated and 170 lncRNAs were downregulated (Figure 2D). Overall, compared with normal samples, 124 upregulated and 134 downregulated lncRNAs were identified in persistent atrial fibrillation (Figure 2E). In addition, compared with paroxysmal atrial fibrillation samples, 414 DElncRNAs were identified in persistent atrial fibrillation samples, including 153 upregulated lncRNAs and 261 downregulated lncRNAs (Figure 2F). Of note, several lncRNAs were observed to be differently expressed in multiple stages of AF, such as MTND1P23, RP11-1081M5.2, and XIST.
Then, we conducted an in silico analysis of the DEGs in the progression of AF. Interestingly, we found that the differentially expressed genes in patients with paroxysmal AF compared with control samples are mainly related to the positive regulation of VEGF production, CXCR chemokine receptor binding, VEGF production, and the formation of renal tubules (Figure 3A).
Figure 3. Bioinformatics analysis of DEGs among normal, paroxysmal AF and persistent AF samples. (A,B) GO and KEGG pathway analysis of DEmRNAs in paroxysmal AF compared to normal samples. (C,D) GO and KEGG pathway analysis of DEmRNAs in persistent AF compared to normal samples. (E,F) GO and KEGG pathway analysis of DEmRNAs in persistent AF compared to paroxysmal AF samples.
In addition, we also found that the genes differentially expressed between persistent AF and normal samples were mainly involved in the regulation of myosin filaments, myofilament sliding, actin-myosin filament sliding, scavenger receptor activity, sodium ion homeostasis, negatively regulating blood coagulation, muscle tissue morphogenesis, negatively regulating coagulation, myocardial tissue morphogenesis (Figure 3C).
Finally, we found that the differentially expressed genes between persistent AF and paroxysmal AF were mainly related to the production of interleukin-18 (IL-18), IgG binding, clustering of skeletal muscle acetylcholine-gated channels, positive regulation of renal sodium excretion, fever production, positive regulation of fever production, positive regulation of VEGFR signaling pathway, cell response to leptin stimulation, response to leptin, the regulation of fever, and the positive regulation of the chemotaxis of granulocytes (Figure 3E).
Use the KEGG database to analyze the pathway of DEmRNAs. We found that the genes differentially expressed in paroxysmal AF and normal samples mainly involve phagosomes, whooping cough, pathogenic Escherichia coli infection, complement and coagulation cascade, osteoclast differentiation, tryptophan metabolism, taurine and subcutaneous Taurine metabolism, fatty acid biosynthesis, leishmaniasis, ovarian steroid production and cytokine-cytokine receptor interaction (Figure 3B).
The differentially expressed genes in persistent atrial fibrillation compared with normal atrial fibrillation mainly involved neuroactive ligand-receptor interactions, tight junctions, complement and coagulation cascades, mucin-type O-glycan biosynthesis, calcium signaling pathways, endocrine, and other factors regulating calcium reabsorption, glycosaminoglycan biosynthesis-heparan/heparin sulfate, amyotrophic lateral sclerosis (ALS), cGMP-PKG signaling pathway, and retinol metabolism (Figure 3D).
Compared with paroxysmal AF, the differentially expressed genes in persistent AF mainly involved phagosomes, complement and coagulation cascade, osteoclast differentiation, leishmaniasis, malaria, African trypanosomiasis, neuroactive ligand-receptor interaction, PPAR signaling pathway, whooping cough, and ovarian steroid hormone production (Figure 3F).
Next, we used the WGCNA package in the R software to perform a co-expression network analysis of the gene expression in the progress of AF. In order to identify all co-expressed genes, we chose β = 8 (fit value R2 = 0.85) as the cutoff to build a network (Figures 4A,B).
Figure 4. Weighted gene co-expression network analysis of differently expressed genes. (A) The scale-free fit index (left). (B) Mean connectivity (right) for various soft-thresholding powers. (C) Dendrogram of the DEGs clustered based on a dissimilarity measure (1-TOM). (D) Cluster analysis and heatmap of the genes in different modules. (E) Heatmap showing the relationship between module eigengenes.
Based on these analyses, we initially obtained five gene modules and then used the dynamic tree cutting algorithm in the WGCNA software package to process the hierarchical clustering tree. In the progress of AF, a total of four gene modules were obtained, including blue, brown, turquoise and yellow modules (Figure 4C). In addition, the gray module includes all genes that cannot be put into any other modules. The clustering tree diagram of the module is shown in the Figure 4D, while the intrinsic clustering of the modules is provided in Figure 4E.
We performed the first principal component analysis (PCA) on five gene modules. The PCA results reflected the main trend of gene expression in each module. Our results indicated that blue module was negatively correlated to the AF progression and downregulated in paroxysmal and persistent AF compared to control samples, and downregulated in persistent AF samples compared to paroxysmal AF samples (Figure 5A). The brown module was downregulated in persistent AF samples compared to control and paroxysmal AF samples, and not differently expressed in paroxysmal AF compared to control samples (Figure 5B). The gray module was upregulated in paroxysmal AF compared to control samples, but downregulated in persistent AF samples compared to paroxysmal AF samples (Figure 5C). Of note, our results indicated that turquoise module was significantly positively correlated to the progression of AF, upregulated in paroxysmal and persistent AF compared to control samples, and upregulated in persistent AF samples compared to paroxysmal AF samples (Figure 5D). Finally, our results showed that yellow module was upregulated in persistent AF and paroxysmal AF samples compared to control samples, and not differently expressed in persistent AF compared to paroxysmal AF samples (Figure 5E). These results suggest that blue module and turquoise module may have a crucial role in the occurrence and progression of AF, that the brown module may have a more important role in the progression of AF, and that the yellow module may have a more important role in the occurrence of AF.
Figure 5. The analysis of the correlation between AF progression and module expression. (A–E) The analysis of the correlation between AF progression and the average expression of genes in blue module (A), brown module (B), grey module (C), turquoise module (D), and yellow module (E).
In order to determine the hub-mRNAs associated with AF, we built a PPI network and used the MCODE scoring system. A total of 4 hub networks were identified in the AF related modules. As presented in Figure 6, a hub network including 19 nodes and 57 edges were identified in gray module (Figure 6A); a hub network including 6 nodes and 15 edges were identified in blue module (Figure 6B); a hub network including 38 nodes and 464 edges were identified in turquoise module (Figure 6C); a hub network including eight nodes and 13 edges were identified in grown module (Figure 6D).
Figure 6. Construction of hub PPI networks in the hub modules. (A–D) The hub PPI network was constructed in gray module (A), blue module (B), turquoise module (C), and brown module (D).
Through the correlation analysis of the DEG and differentially expressed lncRNA (DEL) of the turquoise module, the brown module and all the green and yellow modules, we constructed the DEG-DEL co-expression network. As shown in Figure 7, the blue module includes 35 lncRNAs and 51 mRNAs; the brown module includes five lncRNAs and 19 mRNAs; the turquoise module includes six lncRNAs and 34 mRNAs; and the yellow module includes two lncRNAs and 21 mRNAs.
Figure 7. Construction of lncRNA-mRNA interaction network in the hub modules. (A–D) The lncRNA-mRNA interaction network was built in blue module (A), brown module (B), turquoise module (C), and yellow module (D).
Therefore, we found that some DEGs and DElncRNAs act as hub regulators (connection degree ≥ 5) in the lncRNA-mRNA regulatory network. For example, HSPB6 in the blue module interacts with 11 LncRNAs while CTC-251I16.1 in the blue module connects more than 10 mRNAs and 20 lncRNAs; in the brown module PPP1R1a connects 17 mRNAs and 5 lncRNAs; EDN1, CALCR, COL13A1, and ETV5 in the turquoise module act as the hub genes and connect with more than 30 mRNAs and five lncRNAs; C3 in the yellow module is connected to 20 mRNAs and 2 lncRNAs.
In order to understand the biological effects of hub genes and hub lncRNAs, we performed function prediction via the plug-in CLUEGO in Cytoscape. Our results showed that hub genes in turquoise module was significantly related to cell extravasation, leukocyte chemotaxis, macrophage activation, response to bacteria-derived molecules and positive regulation of α-β T cell activation (Figure 8A). Next, we predicted the potential function of hub lncRNA to in the turquoise module using its co-expressing mRNA. The hub lncRNA in the turquoise module was significantly related to the mineralocorticoid response (Figure 8B).
Figure 8. The function prediction of hub gene and lncRNA of turquoise module. (A,B) The function prediction of Hub mRNAs (A) and lncRNAs (B) of turquoise module.
Atrial fibrillation/paroxysmal AF (PAF) is the main cause of cardiogenic embolism. In recent years, the progression from paroxysmal AF to persistent AF has attracted more and more attention (Proietti et al., 2015). Emerging data indicated a significant association between the morbidity and the transition. However, the molecular mechanism of the progression of AF is unclear. Therefore, we performed RNA sequencing for normal samples, paroxysmal AF and persistent AF samples to identify DEG and explore the roles of these DEGs in AF. In our current research, it is very interesting that we found that the expression of various lncRNAs in the progression of atrial fibrillation was differently changed. Totally, 272 DEmRNAs and 286 DElncRNAs were identified in paroxysmal atrial fibrillation compared to normal samples; 324 DEmRNAs and 258 DElncRNAs found in persistent atrial fibrillation compared with normal samples; and 520 DEmRNAs and 414 DElncRNAs were identified to be differently expressed in persistent AF compared to paroxysmal AF samples (Figures 1E,F). Among the DEGs, about 50% were coding genes and around 50% were non-coding RNAs, suggesting that lncRNAs may also have a crucial role in the progression of AF.
Considering that potential functions of these DEmRNAs in AF remained to be unclear, we performed the enrichment analysis of DEGS. we found that DEGs were involved in the regulation of multiple biological processes and pathways in the progression of AF. It is worth noting that the DEGs between normal and paroxysmal atrial fibrillation was most related to the regulation of VEGF production and the binding to the CXCR chemokine receptor. The VEGF-VEGFR system is essential in angiogenesis and lymphangiogenesis (Shibuya, 2015). Studies have shown that VEGFs play a crucial role in the occurrence, and development of AF (Chung et al., 2002). Multiple studies in patients with early atrial fibrillation reported elevated levels of VEGFA. Vascular endothelial growth factor promotes atrial arrhythmia by inducing acute intercalary disk remodeling (Mezache et al., 2020). It is very interesting that our research is consistent with previous reports that intracardiac VEGF levels increased in patients with paroxysmal, but not persistent AF (Scridon et al., 2012). CXCR family members also play an important role in AF. For example, the chemokine receptor CXCR-2 is a key regulator of monocyte mobilization in hypertension and heart remodeling; and blocking the activation of CXCR-2 can be used as a new treatment strategy for AF (Zhang et al., 2020). We also revealed that DEG between normal and sustained AF was most significantly correlated with mucin-type O-glycan biosynthesis. So far, our research reveals the relationship between this pathway and AF for the first time. Finally, we found that DEG between paroxysmal and persistent atrial fibrillation was most significantly associated with the interleukin-18, coagulation, and complement cascade. Interleukin-18 plays a central role in the regulation of both innate and adaptive immunity (Dinarello, 2018). A previous study showed that AF patients have higher levels of IL-18. IL-18 is positively related to the inner diameter of the left atrium (Luan et al., 2010). A recent genetic study showed that genetic variation of interleukin-18 is related to a lower risk of atrial fibrillation among people in the Northeast China (Wang et al., 2017). In addition, Kornej et al. (2018) reported that complement and coagulation cascades were also related to AF. These reports, together with our findings, further prove the key role of these signals in AF.
Weighted gene co-expression network analysis has been applied to identify the core genes in AF. For example, Zou et al. (2018) reported that LEP, FOS, EDN1, NMU, CALB2, TAC1 may be related to the occurrence and maintenance of AF using WGCNA method and public dataset GSE41177. Li et al. (2020) used GSE79768 to perform a WGCNA analysis to determine the key modules related to atrial fibrillation. However, these reports were based on online public databases and the clinical information of the samples used in these reports remained unclear. In this study, we collected 10 control samples, 10 paroxysmal AF and 10 persistent AF to perform RNA-sequencing analysis. Moreover, we performed PPI network and WGCNA analysis to reveal the biological mechanisms related to the progression of atrial fibrillation. Five modules were identified in the progress of AF, including blue, brown, gray, turquoise, and yellow modules. By analyzing the correlation between these modules and the progression of atrial fibrillation, we found that the turquoise module was significantly positively correlated with the progression of atrial fibrillation while the blue module was significantly negatively correlated with the progression of AF. Moreover, a PPI network was used to identify functional gene connections through MCODE based on a scoring system. Several hub genes were also found in different modules, such as KIAA0101, UHRF1, CDCA2, HJURP, NCAPG, SGOL1, and CENPA in grey module, GPR37L1 in blue module, CD163, CD28 and CX3CR1 in turquoise module, and KNG1 and GRM1 in brown module. It is worth noting that several of these hub genes are reported to be significantly associated with AF. For example, interleukin 10 treatment improves inflammatory atrial remodeling and fibrillation induced by a high-fat diet (Kondo et al., 2018). The plasma concentration of IL-10 in the acute phase is associated with high risk sources of cardiogenic stroke. The serum soluble CD163 level in AF was significantly higher than that in patients with sinus rhythm (Zhong et al., 2016).
Recently, accumulating evidence indicates a link between immune response and AF (Liu et al., 2018). Previous studies demonstrated that marcrophages lead to both structure and electric atrial remodeling in AF (Liu et al., 2018). In addition, a large number of studies have shown that T cells are closely related to cardiovascular diseases, including AF (Liu et al., 2018). For instance, a significant high CD4 + CD28null T cells was found in patient with AF (Sulzgruber et al., 2017). However, the precise mechanism remains unclear. In this study, we conducted a bioinformatics analysis on the turquoise module and reported that the Hub PPI network in the turquoise module significantly participates in regulating leukocyte chemotaxis and macrophage activity and positively regulates α-β T cell activation, indicating that immune pathways have a key role in AF.
LncRNA plays an important role in the regulation of cardiovascular diseases. LncRNA HOTAIR, as a ceRNA, regulates the remodeling of connexin 43 during AF by sponging microRNA-613 (Dai et al., 2020). LncRNA TCONS-00106987 stimulates miR-26 to regulate KCNJ2 to promote atrial electrical remodeling during AF (Du et al., 2020). LncRNA-LINC00472 reduces the expression of JP2 and RyR2 through miR-24, thereby participating in the pathogenesis of AF (Wang et al., 2019). In this study, we identified 286 DElncRNAs in paroxysmal AF and 258 DElncRNAs in persistent AF. Also 414 DElncRNA were identified between persistent and paroxysmal AF samples. Of note, several lncRNAs were observed to differently express in multiple stages of AF, such as MTND1P23, RP11-1081M5.2, XIST and BANCR. Interestingly, several of them had been reported to have key roles in human disease, such as XIST and BANCR. For example, BANCR was previously identified as a cancer-promoting lncRNA and was also significantly related to the pathogenesis of multiple cardiovascular diseases (Li et al., 2017; Wilson et al., 2020). For example, Wilson et al. (2020) found that BANCR promotes cardiomyocyte migration in humans. BANCR promotes vascular smooth muscle cell proliferation via JNK pathway (Li et al., 2017). XIST has a regulatory role in cardiomyocyte function, modulates cardiomyocyte apoptosis via miR-873 (Cai et al., 2020), promotes cardiac fibroblasts proliferation by sponging miR-155-5p (Zhang et al., 2021), and protects the hypoxia-induced cardiomyocyte injury via HK2 (Fan et al., 2020), indicating that XIST’s key roles in heart development. Also, in this study we identified several central lncRNA-mRNA co-expression networks during AF, such as CTC-251I16.1 in the blue module, which connects to more than 10 mRNAs and more than 20 lncRNAs. Bioinformatics analysis showed that the central lncRNA in the turquoise module is significantly related to the mineralocorticoid response.
Our research has several limitations. First, the size of clinical samples used in this study was limited. In the future study, we plan to collect more AF samples and comprehensive clinical information to confirm the correlation between hub genes and AF progression. Secondly, we did not perform gain/loss of function analysis to explore the potential role of hub gene and lncRNA in AF.
In this study, the WGCNA algorithm was for the first time to systematically explore the roles of DEGs in AF. Bioinformatics analysis demonstrated that these DEGs were significantly related to regulate multiple AF related pathways, such as the regulation of VEGF production and binding to the CXCR chemokine receptor. Furthermore, five hub modules were identified in the progression of AF, including blue, brown, gray, turquoise and yellow modules. These results provide new information for further understanding of the pathogenesis and differential diagnosis of AF.
The data presented in the study could be accessed by requesting to the corresponding author.
The studies involving human participants were reviewed and approved by the Chongqing General Hospital, University of Chinese Academy of Sciences. The patients/participants provided their written informed consent to participate in this study.
HC designed the project. PY and YC performed bioinformatics analysis. PY and HJ prepared the manuscript. All authors read and approved the final manuscript.
This work was supported by the China Postdoctoral Science Foundation (2020M670111ZX), Chongqing Municipal Natural Science Foundation (cstc2020jcyj-bshX0068), and Special Fund for Young and Middle-aged Medical Top Talents of Chongqing (ZQNYXGDRCGZS2019005) and Kuanren Talents Program of the Second affiliated Hospital of Chongqing Medical University.
The authors declare that the research was conducted in the absence of any commercial or financial relationships that could be construed as a potential conflict of interest.
All claims expressed in this article are solely those of the authors and do not necessarily represent those of their affiliated organizations, or those of the publisher, the editors and the reviewers. Any product that may be evaluated in this article, or claim that may be made by its manufacturer, is not guaranteed or endorsed by the publisher.
Abdul-Rahim, A. H., Fulton, R. L., Frank, B., Tatlisumak, T., Paciaroni, M., Caso, V., et al. (2015). Association of improved outcome in acute ischaemic stroke patients with atrial fibrillation who receive early antithrombotic therapy: analysis from VISTA. Eur. J. Neurol. 22, 1048–1055. doi: 10.1111/ene.12577
Beavers, D. L., Wang, W., Ather, S., Voigt, N., Garbino, A., Dixit, S. S., et al. (2013). Mutation E169K in junctophilin-2 causes atrial fibrillation due to impaired RyR2 stabilization. J. Am. Coll. Cardiol. 62, 2010–2019. doi: 10.1016/j.jacc.2013.06.052
Cai, C. L., Jin, L., Lang, X. L., and Li, B. L. (2020). Long noncoding RNA XIST regulates cardiomyocyte apoptosis by targeting miR-873-5p/MCL1 axis. Eur. Rev. Med. Pharmacol. Sci. 24, 12878–12886.
Cao, F., Li, Z., Ding, W. M., Yan, L., and Zhao, Q. Y. (2019). LncRNA PVT1 regulates atrial fibrosis via miR-128-3p-SP1-TGF-beta1-Smad axis in atrial fibrillation. Mol. Med. 25:7.
Cao, J. (2014). The functional role of long non-coding RNAs and epigenetics. Biol. Proced. Online 16:11.
Chung, N. A., Belgore, F., Li-Saw-Hee, F. L., Conway, D. S., Blann, A. D., and Lip, G. Y. (2002). Is the hypercoagulable state in atrial fibrillation mediated by vascular endothelial growth factor? Stroke 33, 2187–2191. doi: 10.1161/01.str.0000023889.84649.3d
Cornelis, J., Myers, J., Heidbuchel, H., Vrints, C., and Beckers, P. (2018). Exercise training in heart failure patients with persistent atrial fibrillation: a practical approach. Card Fail Rev. 4, 107–111. doi: 10.15420/cfr.2018.19.2
Dai, H., Zhao, N., Liu, H., Zheng, Y., and Zhao, L. (2021). LncRNA nuclear-enriched abundant transcript 1 regulates atrial fibrosis via the miR-320/NPAS2 axis in atrial fibrillation. Front. Pharmacol. 12:647124. doi: 10.3389/fphar.2021.647124
Dai, W., Chao, X., Li, S., Zhou, S., Zhong, G., and Jiang, Z. (2020). Long Noncoding RNA HOTAIR functions as a competitive endogenous RNA to Regulate Connexin43 remodeling in atrial fibrillation by sponging MicroRNA-613. Cardiovasc. Ther. 2020:5925342.
Dinarello, C. A. (2018). Overview of the IL-1 family in innate inflammation and acquired immunity. Immunol. Rev. 281, 8–27. doi: 10.1111/imr.12621
Du, J., Li, Z., Wang, X., Li, J., Liu, D., Wang, X., et al. (2020). Long noncoding RNA TCONS-00106987 promotes atrial electrical remodelling during atrial fibrillation by sponging miR-26 to regulate KCNJ2. J. Cell. Mol. Med. 24, 12777–12788. doi: 10.1111/jcmm.15869
Fan, J. L., Zhu, T. T., Xue, Z. Y., Ren, W. Q., Guo, J. Q., Zhao, H. Y., et al. (2020). lncRNA-XIST protects the hypoxia-induced cardiomyocyte injury through regulating the miR-125b-hexokianse 2 axis. In Vitro Cell Dev. Biol. Anim. 56, 349–357. doi: 10.1007/s11626-020-00459-0
Feng, H., Gu, Z. Y., Li, Q., Liu, Q. H., Yang, X. Y., and Zhang, J. J. (2019). Identification of significant genes with poor prognosis in ovarian cancer via bioinformatical analysis. J. Ovarian. Res. 12:35.
Gu, C., and Chen, C. (2020). Methylation in lung cancer: a brief review. Methods Mol. Biol. 2204, 91–97. doi: 10.1007/978-1-0716-0904-0_8
Gu, C., Chen, J., Dang, X., Chen, C., Huang, Z., Shen, W., et al. (2021a). Hippo pathway core genes based prognostic signature and immune infiltration patterns in lung squamous cell carcinoma. Front. Oncol. 11:680918. doi: 10.3389/fonc.2021.680918
Gu, C., Huang, Z., Chen, X., Liu, C., Rocco, G., Zhao, S., et al. (2020a). TEAD4 promotes tumor development in patients with lung adenocarcinoma via ERK signaling pathway. Biochim. Biophys. Acta Mol. Basis Dis. 1866:165921. doi: 10.1016/j.bbadis.2020.165921
Gu, C., Shi, X., Dang, X., Chen, J., Chen, C., Chen, Y., et al. (2020b). Identification of common genes and pathways in eight fibrosis diseases. Front. Genet. 11:627396. doi: 10.3389/fgene.2020.627396
Gu, C., Shi, X., Huang, Z., Chen, J., Yang, J., Shi, J., et al. (2020c). A comprehensive study of construction and analysis of competitive endogenous RNA networks in lung adenocarcinoma. Biochim. Biophys. Acta Proteins Proteom. 1868:140444. doi: 10.1016/j.bbapap.2020.140444
Gu, C., Shi, X., Qiu, W., Huang, Z., Yu, Y., Shen, F., et al. (2021b). Comprehensive analysis of the prognostic role and mutational characteristics of m6A-related genes in lung squamous cell carcinoma. Front. Cell Dev. Biol. 9:661792. doi: 10.3389/fcell.2021.661792
Jiao, X., Sherman, B. T., Huang da, W., Stephens, R., Baseler, M. W., Lane, H. C., et al. (2012). DAVID-WS: a stateful web service to facilitate gene/protein list analysis. Bioinformatics 28, 1805–1806. doi: 10.1093/bioinformatics/bts251
Kelley, R. E. (2015). Combined antithrombotic therapy of potential value in acute atrial fibrillation related stroke. Eur. J. Neurol. 22, 1027–1028. doi: 10.1111/ene.12588
Kondo, H., Abe, I., Gotoh, K., Fukui, A., Takanari, H., Ishii, Y., et al. (2018). Interleukin 10 treatment ameliorates high-fat diet-induced inflammatory atrial remodeling and fibrillation. Circ. Arrhythm Electrophysiol. 11:e006040.
Kornej, J., Buttner, P., Hammer, E., Engelmann, B., Dinov, B., Sommer, P., et al. (2018). Circulating proteomic patterns in AF related left atrial remodeling indicate involvement of coagulation and complement cascade. PLoS One 13:e0198461. doi: 10.1371/journal.pone.0198461
Langfelder, P., and Horvath, S. (2008). WGCNA: an R package for weighted correlation network analysis. BMC Bioinformatics 9:559. doi: 10.1186/1471-2105-9-559
Li, H., Liu, X., Zhang, L., and Li, X. (2017). LncRNA BANCR facilitates vascular smooth muscle cell proliferation and migration through JNK pathway. Oncotarget 8, 114568–114575. doi: 10.18632/oncotarget.21603
Li, W., Wang, L., Wu, Y., Yuan, Z., and Zhou, J. (2020). Weighted gene coexpression network analysis to identify key modules and hub genes associated with atrial fibrillation. Int. J. Mol. Med. 45, 401–416.
Liang, S., Shi, X., Yu, C., Shao, X., Zhou, H., Li, X., et al. (2020). Identification of novel candidate genes in heterotaxy syndrome patients with congenital heart diseases by whole exome sequencing. Biochim. Biophys. Acta Mol. Basis Dis. 1866:165906. doi: 10.1016/j.bbadis.2020.165906
Liu, Y., Shi, Q., Ma, Y., and Liu, Q. (2018). The role of immune cells in atrial fibrillation. J. Mol. Cell Cardiol. 123, 198–208. doi: 10.1016/j.yjmcc.2018.09.007
Luan, Y., Guo, Y., Li, S., Yu, B., Zhu, S., Li, S., et al. (2010). Interleukin-18 among atrial fibrillation patients in the absence of structural heart disease. Europace 12, 1713–1718. doi: 10.1093/europace/euq321
Lubitz, S. A., Yi, B. A., and Ellinor, P. T. (2010). Genetics of atrial fibrillation. Heart Fail Clin. 6, 239–247.
Luo, X., Pan, Z., Shan, H., Xiao, J., Sun, X., Wang, N., et al. (2013). MicroRNA-26 governs profibrillatory inward-rectifier potassium current changes in atrial fibrillation. J. Clin. Invest. 123, 1939–1951. doi: 10.1172/jci62185
Mezache, L., Struckman, H. L., Greer-Short, A., Baine, S., Gyorke, S., Radwanski, P. B., et al. (2020). Vascular endothelial growth factor promotes atrial arrhythmias by inducing acute intercalated disk remodeling. Sci. Rep. 10:20463.
Pelargonio, G., Di Monaco, A., Guida, P., Pellegrino, P. L., Vergara, P., Grimaldi, M., et al. (2021). Atrial fibrillation ablation: is common practice far from guidelines’ world? The Italian experience from a national survey. J. Interv. Card. Electrophysiol. doi: 10.1007/s10840-021-00955-7 [Epub ahead of print].
Proietti, R., Hadjis, A., AlTurki, A., Thanassoulis, G., Roux, J. F., Verma, A., et al. (2015). A systematic review on the progression of paroxysmal to persistent atrial fibrillation: shedding new light on the effects of catheter ablation. JACC Clin. Electrophysiol. 1, 105–115.
Ren, C., Li, M., Zheng, Y., Wu, F., Du, W., and Quan, R. (2021). Identification of diagnostic genes and vital microRNAs involved in rheumatoid arthritis: based on data mining and experimental verification. PeerJ 9:e11427. doi: 10.7717/peerj.11427
Scridon, A., Morel, E., Nonin-Babary, E., Girerd, N., Fernandez, C., and Chevalier, P. (2012). Increased intracardiac vascular endothelial growth factor levels in patients with paroxysmal, but not persistent atrial fibrillation. Europace 14, 948–953. doi: 10.1093/europace/eur418
Shi, X., Huang, T., Wang, J., Liang, Y., Gu, C., Xu, Y., et al. (2018). Next-generation sequencing identifies novel genes with rare variants in total anomalous pulmonary venous connection. EBioMedicine 38, 217–227. doi: 10.1016/j.ebiom.2018.11.008
Shi, X., Shao, X., Liu, B., Lv, M., Pandey, P., Guo, C., et al. (2020a). Genome-wide screening of functional long noncoding RNAs in the epicardial adipose tissues of atrial fibrillation. Biochim. Biophys. Acta Mol. Basis Dis. 1866:165757. doi: 10.1016/j.bbadis.2020.165757
Shi, X., Zhang, L., Bai, K., Xie, H., Shi, T., Zhang, R., et al. (2020b). Identification of rare variants in novel candidate genes in pulmonary atresia patients by next generation sequencing. Comput. Struct. Biotechnol. J. 18, 381–392. doi: 10.1016/j.csbj.2020.01.011
Shibuya, M. (2015). VEGF-VEGFR system as a target for suppressing inflammation and other diseases. Endocr. Metab. Immune Disord. Drug Targets 15, 135–144. doi: 10.2174/1871530315666150316121956
Shukla, A., and Curtis, A. B. (2014). Avoiding permanent atrial fibrillation: treatment approaches to prevent disease progression. Vasc. Health Risk Manag. 10, 1–12. doi: 10.2147/vhrm.s49334
Skaarup, K. G., Christensen, H., Host, N., Mahmoud, M. M., Ovesen, C., Olsen, F. J., et al. (2016). Diagnosing paroxysmal atrial fibrillation in patients with ischemic strokes and transient ischemic attacks using echocardiographic measurements of left atrium function. Am. J. Cardiol. 117, 91–99. doi: 10.1016/j.amjcard.2015.10.022
Statello, L., Guo, C. J., Chen, L. L., and Huarte, M. (2021). Gene regulation by long non-coding RNAs and its biological functions. Nat. Rev. Mol. Cell. Biol. 22, 96–118.
Sulzgruber, P., Koller, L., Winter, M. P., Richter, B., Blum, S., Korpak, M., et al. (2017). The impact of CD4(+)CD28(null) T-lymphocytes on atrial fibrillation and mortality in patients with chronic heart failure. Thromb. Haemost. 117, 349–356. doi: 10.1160/th16-07-0531
Wang, H., Song, T., Zhao, Y., Zhao, J., Wang, X., and Fu, X. (2020). Long non-coding RNA LICPAR regulates atrial fibrosis via TGF-beta/Smad pathway in atrial fibrillation. Tissue Cell 67:101440. doi: 10.1016/j.tice.2020.101440
Wang, L. Y., Shen, H., Yang, Q., Min, J., Wang, Q., Xi, W., et al. (2019). LncRNA-LINC00472 contributes to the pathogenesis of atrial fibrillation (Af) by reducing expression of JP2 and RyR2 via miR-24. Biomed. Pharmacother. 120:109364. doi: 10.1016/j.biopha.2019.109364
Wang, Y. H., Fu, L., Wang, B., Li, S. F., Sun, Z., and Luan, Y. (2017). Genetic variants of interleukin-18 are associated with reduced risk of atrial fibrillation in a population from Northeast China. Gene 626, 269–274. doi: 10.1016/j.gene.2017.05.034
Wilson, K. D., Ameen, M., Guo, H., Abilez, O. J., Tian, L., Mumbach, M. R., et al. (2020). Endogenous retrovirus-derived lncRNA BANCR promotes cardiomyocyte migration in humans and non-human primates. Dev. Cell 54, 694–709e9.
Yao, C., Veleva, T., Scott, L. Jr., Cao, S., Li, L., Chen, G., et al. (2018). Enhanced Cardiomyocyte NLRP3 inflammasome signaling promotes atrial fibrillation. Circulation 138, 2227–2242. doi: 10.1161/circulationaha.118.035202
Yao, L., Zhou, B., You, L., Hu, H., and Xie, R. (2020). LncRNA MIAT/miR-133a-3p axis regulates atrial fibrillation and atrial fibrillation-induced myocardial fibrosis. Mol. Biol. Rep. 47, 2605–2617. doi: 10.1007/s11033-020-05347-0
Zhang, H., Ma, J., Liu, F., and Zhang, J. (2021). Long non-coding RNA XIST promotes the proliferation of cardiac fibroblasts and the accumulation of extracellular matrix by sponging microRNA-155-5p. Exp. Ther. Med. 21:477.
Zhang, L., Shi, X., Gu, C., Chen, B., Wang, M., Yu, Y., et al. (2020). Identification of cell-to-cell interactions by ligand-receptor pairs in human fetal heart. Biochim. Biophys. Acta Mol. Basis Dis. 1866:165917. doi: 10.1016/j.bbadis.2020.165917
Zhong, S. M., Qin, Y. H., Li, Z. C., and Wei, Y. S. (2016). [Clinical value of detecting serum soluble CD163 level in patients with atrial fibrillation]. Nan Fang Yi Ke Da Xue Xue Bao 36, 1406–1409.
Keywords: atrial fibrillation, WGCNA, bioinformatics analysis, PPI network, RNA sequencing
Citation: Yang P, Cao Y, Jian H and Chen H (2021) Identification of Hub mRNAs and lncRNAs in Atrial Fibrillation Using Weighted Co-expression Network Analysis With RNA-Seq Data. Front. Cell Dev. Biol. 9:722671. doi: 10.3389/fcell.2021.722671
Received: 09 June 2021; Accepted: 09 July 2021;
Published: 04 October 2021.
Edited by:
Tao Huang, Shanghai Institute of Nutrition and Health, ChinaReviewed by:
Yuan-Lin Zheng, Jiangsu Normal University, ChinaCopyright © 2021 Yang, Cao, Jian and Chen. This is an open-access article distributed under the terms of the Creative Commons Attribution License (CC BY). The use, distribution or reproduction in other forums is permitted, provided the original author(s) and the copyright owner(s) are credited and that the original publication in this journal is cited, in accordance with accepted academic practice. No use, distribution or reproduction is permitted which does not comply with these terms.
*Correspondence: Hao Chen, Q2hlbmhhbzkzNjRAMTYzLmNvbQ==
Disclaimer: All claims expressed in this article are solely those of the authors and do not necessarily represent those of their affiliated organizations, or those of the publisher, the editors and the reviewers. Any product that may be evaluated in this article or claim that may be made by its manufacturer is not guaranteed or endorsed by the publisher.
Research integrity at Frontiers
Learn more about the work of our research integrity team to safeguard the quality of each article we publish.