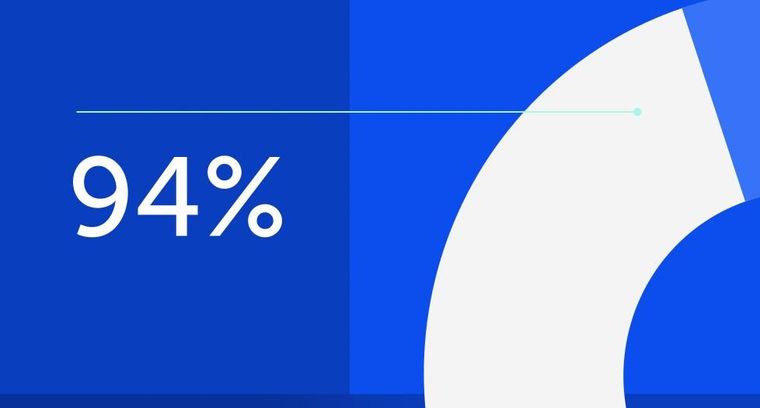
94% of researchers rate our articles as excellent or good
Learn more about the work of our research integrity team to safeguard the quality of each article we publish.
Find out more
ORIGINAL RESEARCH article
Front. Cell Dev. Biol., 17 August 2021
Sec. Cell Growth and Division
Volume 9 - 2021 | https://doi.org/10.3389/fcell.2021.708331
Thrombocytopenia is closely linked with hemorrhagic diseases, for which induction of thrombopoiesis shows promise as an effective treatment. Polyphenols widely exist in plants and manifest antioxidation and antitumour activities. In this study, we investigated the thrombopoietic effect and mechanism of 3,3′,4′-trimethylellagic acid (TMEA, a polyphenol in Sanguisorba officinalis L.) using in silico prediction and experimental validation. A KEGG analysis indicated that PI3K/Akt signalling functioned as a crucial pathway. Furthermore, the virtual molecular docking results showed high-affinity binding (a docking score of 6.65) between TMEA and mTOR, suggesting that TMEA might target the mTOR protein to modulate signalling activity. After isolation of TMEA, in vitro and in vivo validation revealed that this compound could promote megakaryocyte differentiation/maturation and platelet formation. In addition, it enhanced the phosphorylation of PI3K, Akt, mTOR, and P70S6K and increased the expression of GATA-1 and NF-E2, which confirmed the mechanism prediction. In conclusion, our findings are the first to demonstrate that TMEA may provide a novel therapeutic strategy that relies on the PI3K/Akt/mTOR pathway to facilitate megakaryocyte differentiation and platelet production.
Thrombocytopenia is a common hematological disease characterized by a peripheral blood platelet count of less than 100 × 109/L. In the clinic, this disease is divided into primary and secondary subtypes (Ghoshal and Bhattacharyya, 2014; Eto and Kunishima, 2016; Mones and Soff, 2019). Primary thrombocytopenia is usually caused by conditions such as adverse drug reactions, alcohol abuse, surgery, infection, hepatopathy, nephropathy, and pregnancy, which lead to excessive depletion of peripheral blood platelets and megakaryocyte maturation disorder (Stentoft, 2016; Gudbrandsdottir et al., 2020). Secondary thrombocytopenia, also known as acquired thrombocytopenia, follows multifold complex diseases such as chronic lymphocytic leukemia, rheumatoid arthritis, and systemic lupus erythematosus (Mojadidi et al., 2016; Greenberg, 2017; Castro, 2017). Currently, the main therapies for thrombocytopenia comprise drug medications, platelet transfusion and improved diet quality in the clinic (Liebman and Pullarkat, 2011; Lakshmanan and Cuker, 2012). However, platelet transfusion has been associated with allergic reactions and febrile nonhemolytic reactions (Kaufman et al., 2015), while an improved diet might merely as an adjunct to treatment. Drug regimens are still the main therapy for thrombocytopenia. Unfortunately, the agents used to treat thrombocytopenia, such as cytokines, glucocorticoids, and immunoglobulin, have severe adverse reactions, do not prevent high recurrence rates, and show low treatment tolerance (Deshayes and Godeau, 2020). Therefore, it is necessary to explore a novel approach to platelet recovery with high efficacy and low toxicity.
Currently, active compound identification and isolation from traditional Chinese medicinal plants are playing vital roles in novel drug development. As secondary metabolites in raw plant materials, polyphenols are structurally typified by benzene derivatives with multiple hydroxyl substitutions and mainly comprise flavonoids, lignans, tannins, astragalosides, ellagic acid homolog, gallic acid homologs, and curcumin (Croft, 2016; Rothwell et al., 2017). These substances are capable of reducing oxidative stress, suppressing tumour progression, and preventing cardiovascular events, which may indicate their druggability (Scalbert et al., 2005; Poklar Ulrih, 2017; Tresserra-Rimbau et al., 2018; Liang et al., 2020).
Dried root of Sanguisorba officinalis L. (SO), a common herbal material, has hemostatic and astringent efficacies for treating hemorrhagic diseases in the clinic. Increasing pharmacological evidence has shown that an SO regimen can promote megakaryocyte maturation and be beneficial for thrombocytopenia remission (Gao et al., 2014). However, its key component with platelet-elevating activity remains unidentified, impeding further understanding of the mechanism of SO action. However, polyphenols were discovered to moderate irradiation-induced megakaryocyte apoptosis (Xu et al., 2018), suggesting a potential ability of polyphenols to induce thrombopoiesis, which coincides with our preliminary experimental results. Here, 3,3′,4′-trimethylellagic acid (TMEA), a representative SO polyphenol, may exert a therapeutic impact on thrombocytopenia according to the existing evidence. Nevertheless, it is imperative to confirm the prothrombopoietic effect of TMEA and clarify its molecular mechanism.
Network pharmacology offers a feasible approach for systematically investigating the complex relationship between drugs and diseases through systems biology and network analysis methods (Casas et al., 2019; Luo et al., 2020). According to the disease-gene-drug correlation, network pharmacology can be applied to explore potential therapeutic targets for drugs, generally improving the efficiency of drug discovery (Hao and Xiao, 2014; Jiang et al., 2019). In addition, molecular docking simulation is an emerging strategy in computer-aided drug design based on receptor characteristics and receptor and drug molecules interactions (Saikia and Bordoloi, 2019; Santos et al., 2019; Pinzi and Rastelli, 2019; Kaur et al., 2019; Aucar and Cavasotto, 2020). The binding abilities are scored in multiple ways. The hub proteins and signalling pathways can then be deduced by visualizing small molecules interacting with target proteins. In summary, the use of these two in silico techniques may provide reliable insight into and understanding of the mechanism of compound action because of the available sources with large amounts of data.
In the present study, we probed the molecular mechanism of TMEA action on thrombocytopenia via a network pharmacology approach, which might underpin its potential therapeutic effects. The binding of TMEA with the hub protein mTOR was verified by molecular docking simulation. In vitro and in vivo investigations on TMEA activity were designed to verify these predicted results. Our findings showed that TMEA could activate the PI3K/Akt/mTOR signalling pathway to promote platelet production. In summary, this study presents helpful ideas by reporting a combined computational analysis with an experimental trial to explore the thrombopoiesis-promoting effect and underlying mechanism of TMEA in the potential treatment of thrombocytopenia.
As previously reported (Bai et al., 2019), TMEA was prepared by silica gel column chromatography. In brief, 70% ethanol SO extract was partitioned using methylene chloride (CH2Cl2). The CH2Cl2 fraction was subjected to chromatographic isolation by silica gel and eluted with such solution systems in different proportions as petroleum ether (PE)-acetic ether (EAC) (8:2), PE-EAC (6:4), PE-EAC (8:2), and PE-EAC (10:0), successively. TMEA was obtained in PE–EAC (8:2) eluted solution. An UPLC (Exion) – QTOF (X500R) – MS system (SCIEX, Massachusetts, United States) was employed for the analysis of TMEA. The sample injection volume was 5 μL. Phenomenex Kinetex C18 column (100 mm × 2.1 mm, 2.6 μm, 100 Å) was used for the chromatographic separation at column temperature of 40°C. Mobile phase A [0.1% formic acid-water (V/V) mixture] and mobile phase B [0.1% formic acid-acetonitrile (V/V) mixture] were delivered at a flow rate of 0.3 mL/min for gradient elution as follows: 5% B from 0 to 2.00 min, 5% to 70% B from 2.01 to 18.00 min, and 70% to 100% A from 18.01 to 20.00 min. Ultraviolet (UV)-visible (vis) detection was performed at a wavelength of 247 nm. The mass-spectrum assay was optimized in negative ion mode. The ion spray voltage was set at -4.5 kV, and the interface temperature was set at 550°C. Data were obtained in scan mode from m/z 60 to 2000 Da and further analysed using SCIEX OS B.1.4.
Lipinski’s rule-of-five is used for screening potential oral drugs in humans on the basis of a drug-likeness assessment. To explore the drug-like properties of TMEA, we uploaded the SMILES format for TMEA [COC1 = C(OC)C2 = C3C(= C1)C(= O)OC1 = C(OC)C(O) = CC(C(= O)O2) = C31] into the SwissADME server,1 a web-based tool used for evaluating the pharmacokinetics, drug-likeness, and medicinal chemistry friendliness of small molecules (Daina et al., 2017). Screening was performed with the default parameters.
We retrieved TMEA-matching structural information from the PubChem database2 and then input the structural formula into the SwissTargetPrediction database3 to obtained the compound-related targets (Gfeller et al., 2014; Hähnke et al., 2018). Other alternative targets of TMEA action were obtained from the PharmMapper platform4 on the basis of a three-dimensional (3D) TMEA structure (Forouzesh et al., 2019). The gene targets linked to TMEA activity were thereby identified as ‘‘drug targets’’ through target data integration without repetition and nonhuman sources. Thrombocytopenia-relevant targets were obtained as ‘‘disease targets’’ from the GeneCards5 and DisGeNET6 databases after duplicate removal (Rebhan et al., 1998; Piñero et al., 2017). Ultimately, the mapping of “drug targets” onto “disease targets” led to the identification of the shared targets engaging in compound-target-disease interrelations.
To appraise the interactions of the targets in a network, we uploaded the information on the shared targets described above to the STRING_v11.0 database7 with the species defined as “Homo sapiens” (Szklarczyk et al., 2017). In a STRING network analysis, a confidence score of greater than 0.4 indicates credible interrelations among proteins. Accordingly, the eligible PPI data were input into Cytoscape_v3.7.1 software for visibility optimization and topology analysis of the PPI network. The following criteria for determining interconnective nodes assisted in identifying the hub targets (nodes) of TMEA action in thrombocytopenia: degree values greater than the corresponding medians, betweenness centrality and closeness centrality values greater than twice the corresponding medians.
Gene Ontology (GO) term and Kyoto Encyclopedia of Genes and Genomes (KEGG) pathway enrichment analyses were performed to decipher the functions of differentially expressed genes, which were also used to predict the potential mechanism of drug action. We entered the gene symbols of the common targets into the Database for Annotation, Visualization and Integrated Discovery (DAVID)_v6.8, a database with integrated GO and KEGG modules (Huang da et al., 2009). The GO analysis reflected a three-category evaluation of TMEA action, including the TMEA influenced genes in the biological process (BP), molecular function (MF), and cellular component (CC) categories. The KEGG analysis was performed for target-protein-related pathway screening to discover the key mechanism underlying TMEA-driven recovery from thrombocytopenia. The results from the pathway enrichment analyses were prepared for visualization as a bubble diagram using the OmicShare platform.8
The use of virtual molecular docking can help comprehend the interaction process between a small-molecule compound and its target protein via in silico visual simulation. Thus, to observe the direct binding of TMEA with the hub targets identified in the PPI network, we performed the following procedure: First, we derived the 3D TMEA structure and crystal structures of the hub proteins [namely, TLR2 (PDB ID: 1FYW) (Ve et al., 2017), SRC (PDB ID: 1YOJ) (Breitenlechner et al., 2005), mTOR (PDB ID: 4JT6) (Yang et al., 2013), and ABL1 (PDB ID: 5NP2) (Merő et al., 2019)] from the PubChem and RCSB Protein Data Bank9 databases. Second, we removed any co-crystallized ligands from these 4 proteins, and water molecules were removed from the protein structures after assessment of the X-ray diffraction structures. Third, hydrogen atoms were added to the protein structures, in which the amino acid side chains were fixed. Finally, for virtual TMEA-protein interaction imaging, Surflex-Dock (SFXC) was adopted as the docking mode after protein structure preparation. Surflex-Dock scores (total scores) denote binding affinities.
To dynamically observe the efficient flexible docking of each small molecule to its target proteins, we implemented MD simulations using AMBER_18. In preparation for this experiment, the molecular mechanics method was adopted to optimize the protein complex system. The general AMBER force field (GAFF) was used for the ligands, and the ff14SB force field was employed for the protein (Vaijanathappa et al., 2019). All protein-ligand complex systems were immersed in a box (with a 12.0-Å boundary) in the TIP3P water model and then neutralized by the addition of Na+ or Cl- counter ions.
The MD simulations were carried out using the PMEMD.mpi and PMEMD.cuda modules in the AMBER_18 package. Initially, several minimization steps were conducted with these systems in place to prevent possible steric crashes. Then, each system was gradually heated from 0 to 300 K during the heating stage and maintained at 300 K during the subsequent equilibrium and production stages. A time step of 2 fs was used for the heating stage, the equilibrium stage, and the entire production stage. A periodic boundary condition was employed to maintain constant temperature and pressure ensembles. The pressure was set to 1 atm and controlled by the anisotropic (x-, y-, z-) pressure scaling protocol with a pressure relaxation time of 1 ps. The temperature was regulated using Langevin dynamics with a collision frequency of 2.0 ps–1. The particle mesh Ewald (PME) method was adopted to handle long-range electrostatics, and a 10-Å cut-off was set to render real-space interactions. All covalent bonds involving hydrogen atoms were constrained with the SHAKE algorithm. Each system was subjected to a 25-ns MD simulation, and the trajectory of the simulated systems was saved every 100 ps.
For the saved trajectories of MD simulations, the MM/GBSA method was used to calculate the binding energies of the receptors exposed to each ligand. A total of 100 snapshots were extracted from 20 to 25 ns to calculate the mean binding energy. The formula is as follows:
Where ΔEbind is the binding energy and ΔEMM denotes the sum of the molecular mechanical energies in a vacuum, which can be further categorized into contributions from electrostatic, van der Waals, and internal energies. This term was computed using the molecular mechanics method. ΔESOL is the solvation energy, which includes the polar solvation energy (ΔEGB) calculated with the generalized born (GB) approximation model and the nonpolar part (ΔESA) obtained by fitting the solvent accessible surface area (SASA) with linear combinations of pairwise overlaps (LCPOs). Additionally, the energies of each residue were decomposed into the backbone and side-chain atoms. The energy decomposition can be analysed to determine the contributions of the key residues to binding.
HEL cells were purchased from American Type Culture Collection (Manassas, VA, United States). The cells were cultured in complete RPMI 1640 medium (Gibco, Thermo Fisher Scientific, Waltham, MA, States) containing 10% foetal bovine serum (FBS, Gibco, Thermo Fisher Scientific, Waltham, MA, States), 100 U/mL penicillin, and 100 μg/mL streptomycin (Beyotime, Sichuan, China) at 37°C in a humidified incubator with a 5% CO2 atmosphere.
HEL cells were seeded into 6-well flat-bottomed plates at an initial cell density of 4 × 104 cells/well. In addition to being sustained in the complete medium and under the same incubator conditions described above, the cells were harshly treated with 10 μM and 20 μM TMEA to induce their differentiation into megakaryocytes. The medium was replaced every two days, and the TMEA containing medium was replaced to the TMEA group. Cell size increasing is one of the markers of megakaryocyte maturation (Limb et al., 2015; Huang et al., 2016), thus we counted cells that were more than twice larger than undifferentiated HEL cells. The megakaryocyte-like cells whose diameters were twice larger than undifferentiated HEL cells were counted under a microscope at 100× magnification on the 8th and 12th days of treatment. Three fields were randomly selected for mature cell counting and as the basis for statistical analyses.
Giemsa stain was applied to observe the cell morphology under a microscope (Stockert et al., 2014). For that purpose, HEL cells were inoculated in 6-well plates and incubated overnight in fresh complete medium. The cells were treated with 10 μM and 20 μM TMEA in separate batches. After incubation for 8 and 12 days, the cells were washed with PBS and fixed with 100% precooled methanol. Freshly prepared 5% Giemsa solution (Solarbio, Beijing, China, 2018126) was added to each well, and then, the cells were stained at room temperature for 15 min. Multiploid cells were captured at 400× magnification under a microscope.
HEL cells were immobilized at room temperature with 4% paraformaldehyde solution (Biosharp, Anhui, China, 1810473) in phosphate-buffered saline (PBS) on the 8th and 12th days of treatment. The cells were rinsed with PBS and permeated with 0.5% Triton X-100 solution. After washing again with PBS, TRITC-labelled phalloidin working solution was allowed to infiltrate into the cells on glass slides. The cells were incubated in the dark at room temperature and then washed again with PBS. The nuclei were restained with DAPI solution (100 nM). The images were observed under a fluorescence microscope.
After 12 successive days of drug intervention, the HEL cells were centrifuged and prepared for subsequent staining after the supernatant was removed. DAPI staining solution (Beyotime, Sichuan, China, 1207181412) and a 4% paraformaldehyde fixative solution (Biosharp, Anhui, China, 1810473) were mixed at a volumetric ratio of 1:100 for dyeing cell nuclei. The cells were incubated in the dark with the dye solution for 15 min and then analysed with a high-content cell imaging analysis system (Molecular Devices, ImageXpress Micro 4).
HEL cells were seeded into 6-well flat-bottomed plates at an initial cell density of 4 × 104 cells/well. The cells were treated with 10 μM and 20 μM TMEA for 4,8, and 12 days. The medium was added every two days, and the TMEA containing medium was added to the TMEA group. The cells harvested at different time points were washed once with ice-cold PBS and subsequently incubated with 3 μL of CD41-FITC (BioLegend, United States, FHF0411) and 3 μL CD42b-PE (BD, United States, 555473) in 100 μL PBS in the dark for 30 min. Then the cells were re-suspended in 400 μL of PBS, and submitted to the analysis by a FACSVerse flow cytometer (BD Biosciences, SanJose, CA, United States). CD41 expression was assessed using the fluorescein isothiocyanate (FITC) channel, whileCD42b level was examined using the PE channel. The forward and side scatters were used to eliminate the disturbance from cellular fragments. The quantitation of the test biomarker expressions was based on the percentage of CD41+/CD42b+ cells.
HEL cells were seeded into 6-well flat-bottomed plates at an initial cell density of 4 × 104 cells/well. The cells were treated with 10 μM and 20 μM TMEA for 4,8, and 12 days. The cells harvested at different time points were centrifuged at 1500 r/min for 3 min, re-suspended in 0.5 mL of serum-free medium containing 10 μM DCFH-DA (Beyotime, Sichuan, China, S0033), incubated in the dark for 20 min under a gentle mixing at 5 min intervals. The cells were subsequently washed with serum-free medium twice, resuspended in 500 μL of PBS, and submitted to the analysis of intracellular oxidative species (ROS) using a FACSVerse flow cytometer (BD Biosciences, San Jose, CA, United States) under FITC channel. The quantitation of the test DCF was based on the corresponding mean fluorescence.
Cell apoptosis rate was measured by flow cytometry using the Annexin V-FITC/PI Apoptosis Detection Kit (Vazyme, Nanjing, China, A211). Briefly, HEL cells were seeded in 6-well plates at a density of 4 × 104cells/well. The cells were treated with 10 μM and 20 μM TMEA for 4, 8, and 12 days. After treatment, cells were harvested for apoptosis detection according to the manufacturer’s instructions. Flow cytometric analysis was then carried out by using a FACSVerse flow cytometer (BD Biosciences, San Jose, CA, United States). Data acquisition and analysis were performed by using the Flowjo software (BD Biosciences, San Jose, CA, United States).
HEL cells were collected after drug intervention and fixed with 4% paraformaldehyde fixative solution at room temperature. Subsequently, we washed the cells three times with PBS, added 0.5% Triton X-100 to perforate the cell membranes within 10 min, and blocked this process by adding 5% BSA at room temperature. Then, anti-GATA-1 and anti-NF-E2 antibodies were incubated with the cells overnight at 4°C. After washing with PBS, the cells were co-incubated with DAPI to observe the nuclear translocation of the transcription factors GATA-1 and NF-E2 via fluorescence confocal microscopy.
On the 4th, 8th, and 12th days of TMEA treatment, the HEL cells were harvested and lysed with 1× RIPA lysis buffer (Cell Signaling Technology, Beverly, MA, United States) containing EDTA-free protease inhibitor cocktail (TargetMol, Shanghai, China). Total proteins were extracted from the cells. After heating at 95°C for 10 min, the protein samples were electrophoresed and transferred to PVDF membranes (Millipore, Darmstadt, Germany). Then, the membranes were rinsed twice in PBS-Tween 20 (PBST) and immersed in 5% skim milk powder solution in PBST with gentle shaking. After 2 h of blocking, the membranes were washed in PBST 3 times and incubated overnight at 4°C separately with the indicated primary antibodies: anti-PI3K, anti-phosphorylated-(p-)PI3K, anti-Akt, anti-p-Akt, anti-mTOR, anti-p-mTOR, anti-P70S6K, anti-p-P70S6K, anti-GATA-1, or anti-NF-E2, and anti-β-actin (Cell Signaling Technology, Danvers, MA, United States). Subsequently, these membranes were washed 3 times and incubated with horseradish peroxidase-labelled secondary antibody. After 1 h of sealing, protein bands in the membranes were visualized using UltraSignalTM ECL western blotting detection reagents (4A Biotech Co., Ltd, Beijing, China) and a ChemiDoc MP Imaging System (Bio-Rad, Hercules, CA, United States). These bands were quantitatively analysed by ImageJ software (National Institutes of Health, Bethesda, MD, United States). The relative image intensities of the target proteins to β-actin positively manifested their expression.
Specific pathogen-free (SPF) Kunming mice, evenly composed of one-half male and one-half female mice weighing 20.0 ± 2.3 g, were purchased from Dashuo Experimental Animal Research Center (Chengdu, China). These mice were bred in specific-pathogen-free laminar flow cabinets under a 12-h light/dark cycle. The mice were fed standard diets and allowed to drink freely. The ambient temperature was controlled at (24 ± 2) °C. All experimental processes were approved by the Ethics Committee of Southwest Medical University (Licence No. 20170341). After acclimation for 7 days, the animals were randomly assigned to 4 groups: the control group, X-ray (model) group, X-ray + TPO (3000 U/kg, positive control) group, and X-ray + TMEA (10 mg/kg, treatment) group. Except for those in the control groups, the mice were X-ray-irradiated at a single 4-Gy dose to induce experimental haematopoietic dysfunction. Notably, the mice in the X-ray-exposed groups were tested to ensure relative uniformity of baseline levels of peripheral blood cells before drug administration.
After irradiation-induced modelling, the animals in the control and model groups were intraperitoneally injected with normal saline. The mice in the positive control group were administered recombinant human TPO injection intraperitoneally. TMEA was intraperitoneally injected into the mice in the treatment group for therapeutic evaluation. All these injections were performed once per day. Subsequently, we collected 40-μL whole blood samples from the retro-orbital venous plexus of each mouse initially and on the 4th, 7th, 10th, 14th, and 17th day, and these samples were mixed and diluted by a 160-μL diluent for blood cell analysis. Complete blood cell counts were determined using an automatic haematology analyser (Sysmex XT-2000iV, Kobe, Japan).
The data acquired in this study were statistically analysed using GraphPad Prism 8.0 (GraphPad Software Inc., La Jolla, CA, United States). The data are expressed as the means ± standard deviation (). Statistical significance was assessed using two-tailed Student’s t-tests or one-way analysis of variance (ANOVA). In all cases, a P-value < 0.05 was considered to indicate a significant difference.
UPLC-QTOF-MS was used to confirm the molecular information on TMEA obtained from analyses of the SO extract. As shown in Figure 1, the retention time of TMEA was 3.785 min, and its relative molecular mass was 343.0481 [M-H]–, which partially reflects the structural characteristics of the TMEA molecule. This result suggests that TMEA may undertake SO activity in part, serving as an active component in this herbal material.
Figure 1. Identification of TMEA from SO roots using UPLC-QTOF-MS. (A) Total ion chromatogram (TIC) with 247-nm UV for TMEA. (B) UV chromatogram at 247 nm for TMEA. The retention time of TMEA was 3.782 min. (C) Diode array detection (DAD)-coupled chromatogram with 247-nm UV for TMEA. The relative molecular mass was 343.0481 [M-H]–. The structure of TMEA is shown in this figure panel (C).
Drug-likeness assessment is vital for predicting latent therapeutic compounds. From Lipinski’s rule of five (Ro5), a druggable compound likely meets the following requirements: molecular weight from 180 to 500 Daltons, polar surface area (PSA) less than or equal to 140 Å2, a n-octanol/water partition coefficient (XLogP3) less than 5, and the number of hydrogen-bond donors and acceptors less than 5 and 10, respectively (Chagas et al., 2018). Our results showed that the properties of the TMEA molecule were in line with those of the Ro5. It may be considered a lead compound for structurally optimized drug development (Table 1).
To acquire the “drug targets” and the “disease targets,” we separately integrated the TMEA-related targets obtained from SwissTargetPrediction with PharmMapper analyses and the thrombocytopenia-associated targets obtained from GeneCards and DisGeNET. The results revealed that 105 targets were found in the SwissTargetPrediction, and 110 targets were identified with PharmMapper. We ultimately obtained 205 drug targets after data integration. Similarly, 2401 and 340 targets linked with thrombocytopenia were retrieved from GeneCards and DisGeNET, respectively. Ultimately, we retained 295 disease targets after removing the duplicate targets obtained of these two database analyses. The drug targets and the disease targets intersected to generate 17 potential therapeutic targets for TMEA treatment of thrombocytopenia. These targets are shown in Figure 2A: mTOR, SRC, ABL1, TLR2, PRKCQ, FLI1, MAD2L1, B2M, IVD, E2F1, MS4A1, CA2, PDGFRB, ALOX5, XDH, BCL2L1, and ACHE.
Figure 2. Network pharmacology analysis of TMEA activity in thrombocytopenia treatment. (A) Venn diagram of the shared targets of TMEA and in thrombocytopenia. (B) Protein-interactive (PPI) network based on the targets for TMEA in the treatment of thrombocytopenia. (C) GO enrichment analysis of the hub targets for thrombocytopenia treatment with TMEA. The 15 BP, 5 CC, and 5 MF terms with the greatest enrichment are listed in ascending order of P-values. (D) The 10 KEGG pathways enriched with hub targets for TMEA in treating thrombocytopenia. The bubble size indicates the number of enriched genes; its colour signifies the corresponding P-value. The rich factor denotes the ratio of the target genes in a pathway to all the annotated genes in this pathway. The colour of the bubble is related to the P-value.
The 17 therapeutic targets were analysed using the STRING database. Meeting the criterion of a confidence score greater than 0.4, the desired target data were imported into Cytoscape_v3.7.1 to generate an interaction network, which contained 10 nodes and 21 edges. In the network, a node represented a target. The smaller the degree value was, the closer the node colour was to orange. Moreover, the larger the degree value was, the closer the node colour was to blue. Additionally, the edges among the nodes represented PPIs. The thickness of the line denoted the intensity of the PPI, as shown in Figure 2B.
Then, we performed a topology analysis off all nodes in the network using Cytoscape to identify the hub targets. For each node (target), the degree value was set to be greater than 4.5, the betweenness centrality was determined to be greater than 0.08842593, and the closeness centrality was determined to be greater than 0.518092105. With these criteria, 4 hub targets, namely, mTOR, SRC, ABL1, and TLR2, were identified, as shown in Table 2.
To predict the mechanism underlying TMEA action on thrombocytopenia, we conducted GO and KEGG analyses through the DAVID platform. The GO analysis results showed that a total of 75 GO terms were related to thrombocytopenia treatment with TMEA. Of these GO annotations, 54 terms were linked to the BP category and mainly involve protein autophosphorylation, regulation of actin cytoskeleton organization, intrinsic apoptotic signalling pathway in response to DNA damage, positive regulation of fibroblast proliferation, and positive regulation of apoptotic process. Nine terms were related to the CC category and mainly involve the cytosol, extracellular space, perinuclear region of cytoplasm, nuclear membrane, and intrinsic component of plasma membrane. Twelve terms were associated with the MF category and mainly involve protein binding, protein kinase activity, protein kinase binding, protein tyrosine kinase activity, and protein homodimerization activity. In total, 15 BP terms, 5 CC terms, and 5 MF terms showing the greatest enrichment with TMEA-related processes were ranked separately in ascending order of P-values, as shown in Figure 2C. Furthermore, the results of the KEGG analysis revealed that 14 pathways might be involved in TMEA regulatory action in thrombocytopenia, mainly the PI3K-Akt signalling pathway; pathways in glioma, cancer, and chronic myeloid leukaemia; the ErbB signalling pathway; and pathways in prostate cancer and HTLV-I infection. The 10 most-enriched pathways were arranged in ascending order of P-values, as shown in Figure 2D.
Molecular docking stimulation was used to estimate the binding abilities of TMEA to the 4 hub targets (mTOR, SRC, ABL1, and TLR2). In this study, a docking score (total score) ≥4.52 indicated that the molecule showed binding affinity for the target, and a docking score >5.0 was deemed to indicate high binding activity (Hsin et al., 2013, 2016; Gu et al., 2020). As shown in Figure 3, mTOR and SRC exhibited strong affinities for TMEA, with docking scores of 6.65 and 6.00, respectively.
Figure 3. In silico molecular docking of TMEA to hub targets. (A) TMEA bound to TLR2 protein (1FYW) via the active residue LYS695. (B) TMEA bound to the SRC protein (1YOJ) via active sites ARG462, LYS353, LYS358, and LYS460. (C) TMEA bound to mTOR protein (4JT6) via the active residues ASP2357 and TYR2225. (D) TMEA bound to ABL1 protein (5NP2) at the active site LEU69.
To validate these molecular docking stimulation results, we performed 25-ns molecular dynamics simulations and calculated the binding free energies for each complex formation. In this study, the most favourable docking conformations were selected for use in the MD simulations. A 25-ns MD simulation for each system was performed to determine the stable conformation. The RMSDs of mTOR and TMEA fluctuated near ∼4 Å and ∼0.5 Å after 15 ns and 3 ns, respectively (Figure 4A). SRC and TMEA remained stable at ∼5 Å and ∼0.5 Å after 15 ns and 5 ns, respectively (Figure 4B). For the system of ABL1 complexed with TMEA, equilibrium was observed after 20 ns and 1 ns, and the RMSD values were ∼4 Å and ∼0.5 Å (Figure 4C). Similarly, the RMSD values for TLR2 and TMEA fluctuated between ∼7 and 0.8 Å after 10 ns and 5 ns, respectively, which indicated that the complex reached a stable state (Figure 4D). Moreover, for subsequent analyses of residue contribution to each system in the simulation, the root-mean-square fluctuation (RMSF) values versus the residue numbers of all the compounds are shown in Figures 4E–H (Supplementary Videos 1–4). The key residues, including TYR2225, ASP2357, LYS353, LYS358, LYS460, ARG462, LEU69, and LYS695, exhibited rigid behaviours with low RMSF values (approximately 1 A ng), which indicated stable complex properties. These results support the reliability of the MD simulation.
Figure 4. Molecular dynamics simulations (25 ns) used to evaluate TMEA-target interactions. (A–D) RMSD values of TMEA binding to mTOR, SRC, ABL1, and TLR2, respectively. (E–H) RMSF values of TMEA binding to mTOR, SRC, ABL1, and TLR2, respectively.
On the basis of the MD simulation, binding free energies were calculated using MM-GBSA methods to assess the energy parameters of the four representative complexes. The binding free energy vales for the complexes are listed in Table 3. The results showed that ΔGbind of TMEA binding with mTOR, SRC, ABL1, and TLR2 as determined by MM/GBSA was –35.08, –29.95, –25.09, and –19.02 kcal/mol, respectively.
To appraise the overall effect of TMEA on promoting megakaryocyte differentiation, we randomly selected 3 fields viewed under an ordinary microscope to count the megakaryocyte-like cells. As shown in Figure 5A, obvious megakaryocyte-like cells were observed. On the 8th and 12th days, the cells treated with TMEA manifested statistically significant differences (P < 0.001) in terms cytomorphology compared to the control cells (Figure 5B).
Figure 5. TMEA-induced HEL cell differentiation/polyploidization (megakaryocytic commitment). (A) Megakaryocyte-like HEL cell count on the 8th and 12th days of treatment using a microscope at 100× magnification. The megakaryocyte-like HEL cells in the TMEA treated groups were significantly greater than that in the control cells on these two indicated days. (B) The histogram displays the statistical result of megakaryocyte-like HEL cell count. *** P < 0.001, vs. the control. (C) HEL cell nucleotype as determined with Giemsa staining under a microscope at 400× magnification. (D) F-actin cytoskeletons of HEL cells are shown with phalloidin staining 8 days and 12 days after treatment with 10 μM or 20 μM TMEA. Arrows designate the salient regions with heavy phalloidin staining. Mean fluorescence intensities in the treated cells at both TMEA concentrations were significantly higher than those in the control cells on the 8th day and 12th day (E). The histograms display the mean fluorescence intensities as determined by statistical analysis. * P < 0.05, ** P < 0.01, and *** P < 0.001, vs. the control.
Giemsa staining was used for observing nucleotypes in the HEL cells treated with TMEA. Figure 5C shows apparent nuclear division of the cells treated with TMEA at 10 and 20 μM. This result demonstrates that nuclear division characterizes HEL cell differentiation into megakaryocytes upon TMEA triggering.
HEL cells were treated with 10 or 20 μM TMEA for 12 days. The polyploid proportion in these cells was then detected with a high-content cell imaging analysis system, as shown in Figure 6. Compared with that in the control, the proportion of polyploid cells significantly increased in a concentration-dependent manner after 12 days of TMEA intervention. However, the proportion of diploid cells decreased with increasing TMEA concentration. These results are shown in Figures 6D,E.
Figure 6. Regulatory effect of TMEA on the cell cycle and DNA ploidy in HEL cells. (A) Fluorescence imaging by DAPI staining at 200×. (B) and (C) Cell cycle classification with DNA ploidy analysis. Blue, aquamarine blue, green, orange, and red cell labels represent the different phases of the cell cycle: G0/G1, S, G2 (≥4 N), early M (≥4 N), and late M phases, respectively. (D) The proportion of polyploid cells increased in a concentration-dependent manner 12 days post-TMEA intervention; (E) the proportion of diploid cells decreased with increasing TMEA concentration. * P < 0.05 and **P < 0.01, vs. the control.
Actin cytoskeleton organization can drive dynamic processes in megakaryocyte maturation, inducing morphological changes such as parapodium and proplatelet formation. In this study, morphological alterations of the HEL cells were evident within 8 to 12 days of TMEA intervention, as determined by filamentous (F-) actin staining and exhibited in Figure 7. Arrows show actin bundles protruding from the cell membrane (Figures 5D,E). These protrusions reflected potential megakaryocyte lineage commitment and proplatelet formation of HEL cells. In contrast, these membrane protrusions were almost absent in the untreated HEL cells (Figure 6).
Figure 7. CD41 and CD42b levels in megakaryocytes upon TMEA treatment. (A) On the 4th, 8th, and 12th days of TMEA stimulation, the CD41 and CD42b levels were significantly elevated in the treated cells. (B) The histograms show the statistical analysis of the cells percent with CD41+/CD42b+. ** P < 0.01, and *** P < 0.001, vs. the control.
Changes in the expression of characteristic molecular markers are commonly used to evaluate differentiation and maturation of megakaryocytes (Zhang et al., 2019). The expressions of the cell surface CD41 and the megakaryocytic maturation associated antigen ofCD42b were thus analyzed, and the results showed TMEA obviously improved CD41 and CD42bantibody-stained cell population (Figure 7), confirming the increase ofCD41 and CD42b expressions during the differentiation process of megakaryocytes.
ROS generation could play pivotal roles in regulating the differentiation of megakaryocytes (Yang et al., 2019). The results from flow cytometry assay using DCFH-DA probe showed that TMEA induced excessive ROS production in megakaryocytes on the 8th and 12th days (Figure 8A),which was in a concentration-dependent manner (Figure 8C). This result consistently confirmed oxidative stress could be induced by TMEA in megakaryocytes, thus resulting in the accelerated thrombopoiesis.
Figure 8. Apoptosis and ROS level by TMEA in HEL cells. (A) Apoptotic cells of HEL cells treated TMEA concentrations were measured by flow cytometry using an Annexin V-FITC/PI apoptotic detecting kit. (B) Intracellular ROS generation in HEL cells using flow cytometry analysis. (C) Quantitative analysis of Annexin V+ in different treatments on the 4th, 8th, and 12th days. * P < 0.05, ** P < 0.01, and *** P < 0.001, vs. the control. (D) Quantitative analysis of ROS generation in different treatments on the 4th, 8th and 12th days. *** P < 0.001, vs. the control.
Proplatelet formation requires localized apoptosis to facilitate the cytoskeletal rearrangements required for platelet shedding (De et al., 2002). The results from flow cytometry assay using Annexin V/PI staining showed that TMEA induced excessive apoptosis in megakaryocytes on the 8th and 12th days (Figure 8B), which was in a concentration-dependent manner (Figure 8D).
The transcription factors GATA-1 and NF-E2 play essential roles in megakaryocytic differentiation and maturation as well as proplatelet formation, associating upstream molecular signalling with downstream transcript expression. After TMEA treatment at 10 μM or 20 μM, GATA-1 and NF-E2 expression, quantified by fluorescence intensity, was significantly increased in the treated HEL cells on the 12th day compared with that in the control cells. The arrows show the protein expression of GATA-1 and NF-E2 (Figures 9A,B). This result warrants to subsequent investigation on the molecular mechanism of TMEA in promoting HEL cell (megakaryocyte) differentiation.
Figure 9. Transcription factor regulation by TMEA in HEL cells. GATA-1 (A) and NF-E2 (B) expression in HEL cells is shown by fluorescence intensity. After stimulation with 10 μM or 20 μM TMEA, GATA-1, and NF-E2 expression was significantly increased in the treated cells on the 12th day compared with the control. Arrows show the distinct regions with heavy anti-GATA-1 and anti-NF-E2 staining separately. The histograms show the statistical analysis of cells with GATA-1 or NF-E2 fluorescence signal. * P < 0.05, ** P < 0.01, and *** P < 0.001, vs. the control.
Since the in silico prediction results emphasized the PI3K/Akt signalling pathway involvement in a latent regulatory mechanism of TMEA action, we measured the expression of the hub proteins in this pathway, which are diverse and embody TMEA-prompted pathway regulation: (p-)PI3K, (p-)Akt, (p-)mTOR, (p-)P70S6K, GATA-1, and NF-E2 (Figure 10). On the 4th day, phosphorylation levels of PI3K, AKT, and mTOR were no significant changes in the TMEA treated cells. On the 8th and 12th days of TMEA stimulation, phosphorylation levels of PI3K, AKT, and mTOR, P70S6K markedly increased in the treated cells, showing the same trend as transcription factor expression (P < 0.05 or 0.001). These results indicate that TMEA stimulation can promote megakaryocyte differentiation/maturation by activating the PI3K/AKT/mTOR/P70S6K/GATA-1/NF-E2 cascade.
Figure 10. TMEA-triggered activation of the signalling cascade in HEL cell differentiation. (A) The hub protein expression in the theoretical pathway was validated by western blot analysis and denoted by relative density. On the 4th, 8th, and 12th days of TMEA stimulation, the phosphorylation levels of PI3K, AKT, mTOR, and P70S6K were significantly elevated in the treated cells. Additionally, the expression of transcription factors GATA-1 and NF-E2 was markedly increased in the treated cells compared with the control cells. (B) The histograms show the statistical analysis of the relative densities. * P < 0.05 and *** P < 0.001, vs. the control.
To confirm the in vivo platelet-increasing effect of TMEA, we injected X-ray-exposed mice intraperitoneally with TMEA. Owing to the X-ray exposure, the blood platelet count in the model mice declined to a minimum on the 7th day after irradiation, while that of the control mice was unchanged. TMEA administration notably inhibited X-ray-induced blood platelet reduction (i.e., thrombocytopenia) and boosted platelet recovery from days 7 to 14 (P < 0.001), as shown in Figure 11A.
Figure 11. In vivo platelet recovery by TMEA administration and its underlying molecular mechanism. (A) The blood platelet count (PLT) in the treated mice reflected the therapeutic effect of TMEA on X-ray-induced thrombocytopenia. Compared to that in the control, the PLT in the model mice was reduced to the minimum on the 7th day post-irradiation. From the 7th to 14th days, the PLT in the TMEA-injected mice was higher than that in the model mice. The line chart exhibits the statistical analysis of PLT. * P < 0.05, ** P < 0.01, and *** P < 0.001, vs. the X-ray group. (B) The PI3K/Akt/mTOR/P70S6K/GATA-1/NF-E2 pathway may be part of the key molecular mechanism of TMEA action on megakaryocytic differentiation and platelet production.
Given the physiological function of platelets in blood coagulation, haemorrhage is the primary concomitant symptom of thrombocytopenia. Although routine clinical remedies in response to thrombocytopenic bleeding are capable of boosting platelet recovery and inhibiting haemorrhagic progression, serious side effects, high medical expenses and high recurrence rates caused by these therapies may place large burdens on the patient population (Delaney et al., 2016). In this regard, phytoproduct intervention as a highlighted alternative may create opportunities for achieving better outcomes of thrombocytopenia treatment (Gao and Chong, 2012; Sun et al., 2018).
Traditional Chinese herbs comprise a treasure trove of natural medicinal products. TMEA, an SO polyphenol, was deduced to be a core compound that might govern the thrombopoiesis-augmenting mechanism of a TCM since the chemical traits of this compound meet the aforementioned criteria. To probe this assumption, in this study, we employed in silico analysis (combining a network pharmacology approach and molecular docking simulation) followed by experimental investigations (in vivo and in vitro).
Useful for the multitargeted regulatory patterns of natural products, the network pharmacology method enabled the integration multiple of multiple factors for determining a TMEA-mediated signalling map, similar to that in other reported studies (Lv et al., 2014; Zhu et al., 2018; Tu et al., 2020; Wang Z. Y. et al., 2020; Wang W. et al., 2020). Using target prediction, we identified 17 latent target proteins in the compound-target-disease interrelated network: mTOR, SRC, ABL1, TLR2, PRKCQ, FLI1, MAD2L1, B2M, IVD, E2F1, MS4A1, CA2, PDGFRB, ALOX5, XDH, BCL2L1, and ACHE. Among these targets, 4 diverse hub targets, mTOR, SRC, ABL1, and TLR2, were identified in the PPI network, which was constructed with 10 nodes and 21 edges. Moreover, the in silico docking data of TMEA with the hub targets were used in modelling simulations to estimate binding affinities, showing that the docking score of mTOR with TMEA was 6.65, the highest appetency among the tested compounds. These findings laid the foundation for signalling pathway inference and follow-up experimental validation. We conjectured that the molecular mechanism of TMEA action in thrombocytopenia is likely related to the TMEA interaction with the hub protein mTOR.
mTOR, mechanistic target of rapamycin, is a serine/threonine protein kinase that regulates various cellular functions, including cell survival, growth, proliferation, differentiation, apoptosis, and homeostasis maintenance (Laplante and Sabatini, 2012). Especially during multilineage haematopoiesis, mTOR exhibits distinct functions, even playing opposite roles in different phases. For example, mTOR activation was indispensable for the maintenance of haematopoietic stem cells (HSCs) but not haematopoietic progenitors (Zhou et al., 2016). In terms of erythroid cell commitment, mTOR inhibition by rapamycin significantly improved erythroid cell maturation and anaemia in a β-thalassemia mouse model (Zhang et al., 2014), whereas suppression of mTOR signalling markedly reduced the proportion of erythroid progenitor cells in a population of wild-type bone marrow cells (Liu et al., 2020). During megakaryocytic development, mTOR overexpression might inhibit megakaryocyte differentiation by triggering abnormal autophagy, as indicated when the autophagy-related gene ATG7 was knocked out (Drayer et al., 2006; Liu et al., 2011; Wang et al., 2016; Malik et al., 2018; Sun and Shan, 2019). Additionally, mTOR blockade with rapamycin suppressed the maturation of both adult and neonatal megakaryocytes; the former showed inhibited polyploidization and cytoplasmic maturation, and the latter showed no ploidy changes (Hou et al., 2018). These outcomes emphasize the significance of mTOR biofunction in haematopoiesis, notably megakaryocyte maturation and platelet production related to our study. Therefore, it is easy to understand that mTOR modulation by TMEA may be a pivotal point in TMEA-controlled signal transduction.
In addition to identifying the targets of TMEA action, we obtained 14 potential pathways using KEGG pathway enrichment analysis. Of these pathways, the PI3K/Akt signalling pathway caught our attention not only because of its top rank among the enriched pathways but also because of its linkage to mTOR as a downstream signalling molecule. PI3K/Akt is an essential pathway mediating cell survival, differentiation, proliferation, apoptosis and migration (Hers et al., 2011). Disorders of this pathway can be found in various human diseases, including cancer, diabetes, cardiovascular diseases, nervous system diseases, and haematopoietic diseases (Buitenhuis and Coffer, 2009; Polak and Buitenhuis, 2012). For platelet formation, the intracellular PI3K/Akt pathway can be activated by multiple haematopoietic factors, such as stem cell factor, platelet-derived growth factor, interleukins, and thrombopoietin (TPO). These extracellular regulators combine with their membrane receptors to maintain homeostasis and promote committed differentiation of HSCs and secondary progenitors, leading to megakaryopoiesis and thrombopoiesis (Nakao et al., 2008; Vainchenker and Raslova, 2020). As intracellular signal transducers, PI3K and Akt together constitute a signalling cascade transmitting a stimulative signal from the activated receptor to subsequent signalling molecules. mTOR, one of the downstream signalling molecules of Akt, is activated by its interaction with Akt, which phosphorylates P70S6K and further genetically upregulates the expression of transcription factors, including GATA-1 and NF-E2. Expression of GATA-1, an important transcription activator in megakaryocytes, is conducive to NF-E2 expression and governs the transcriptional activity of FOG-1 through interaction. These regulatory processes positively affect megakaryocyte maturation and proplatelet formation by amplifying F-actin expression (important in cytoskeleton organization/remodelling) and assembly in a PDK1-dependent manner (Bury et al., 2016; Johnson et al., 2016; Geue et al., 2019). Accordingly, it is essential and feasible to augment megakaryopoiesis/thrombopoiesis cells to enhance the PI3K/Akt/mTOR/P70S6K/GATA-1/NF-E2 signalling pathway. Moreover, from the pathological perspective, thrombocytopenia development correlates with abnormalities in megakaryocyte production, growth, and differentiation (Flaumenhaft et al., 2009; Wang et al., 2019). These cytological variations result from cell signalling dysfunction, particularly affecting the PI3K/Akt/mTOR pathway and its downstream cascades (Raslova et al., 2003). We can thereby surmise that this pathway may play a critical role in thrombocytopenia treatment with TMEA, acting in a core molecular mechanism. In addition, the GO analysis revealed BPs such as protein autophosphorylation, regulation of actin cytoskeleton organization, and platelet-derived growth factor receptor-beta signalling pathway (Figure 2), which also affect megakaryopoiesis/thrombopoiesis-associated signalling pathways, supporting the stated importance of the PI3K/Akt/mTOR pathway.
The evidence from in vitro and in vivo experiments corroborates the predicted results. Because platelets originate from the differentiation of megakaryocytes, polyploidy occurs, and multilobulated nuclei appear when megakaryocytes mature (Cullmann et al., 2021). These phenotypes were observed by differentiated cell counting, Giemsa staining and DAPI staining after TMEA intervention. Furthermore, cytoskeletal staining with phalloidin also showed the ability of TMEA to promote megakaryocyte commitment to proplatelets in our study on the basis of the close association between actin and cytoplasmic division (Rojnuckarin and Kaushansky, 2001). Cell shape maintenance and change are dependent on 2 cytoskeleton systems, the microtubule cytoskeleton and F-actin cytoskeleton. In mature megakaryocytes, the microtubule cytoskeleton propels proplatelet formation through microtubule polymerization or reorganization, while the F-actin cytoskeleton is critical for stabilizing cell shape at a resting state, organizing the topology of surface receptors, and driving cytoplasm fragmentation as well as platelet spreading. Hence, F-actin expression can be used to quantitatively estimate megakaryocyte differentiation/maturation. Previous ultrastructural analysis of proplatelet-committed megakaryocytes by phalloidin staining helped reveal the dispersion of actin filaments, showing a similar tendency to our data (Rojnuckarin and Kaushansky, 2001). All platelets are the progeny of megakaryocytes, and megakaryocytes that produce functional platelets are marked as CD41+CD42b+(Schulze and Italiano, 2016; Zhang et al., 2019). In this study, the count of CD41+CD42b+cells were upregulated during megakaryocyte differentiation/maturation for the 12 consecutive days.
The following investigations were based on the in vitro activity verification above. We further tested the therapeutic effect of TMEA on irradiation-induced thrombocytopenia in mice. In this in vivo study, TMEA injection resulted in a significant increase in peripheral platelet count, especially 7 and 10 days after X-ray irradiation of the mice. On account of bone marrow haematopoietic dysfunction after whole-body irradiation, the results reveal TMEA action on thrombopoiesis and may partly reflect its linkage to megakaryocyte differentiation/maturation. Because TMEA treatment for thrombocytopenia was experimentally verified to be effective, we explored the key regulatory mechanism of TMEA in thrombopoiesis. As predicted in silico, the adjustment to the PI3K/Akt/mTOR/P70S6K/GATA-1/NF-E2 signalling pathway may be instrumental in facilitating thrombopoiesis by TMEA (Figure 11B). Therefore, we detected critical protein phosphorylation to decipher whether TMEA functioned through this proposed pathway. The results suggest that phosphorylated proteins such as p-PI3K, p-Akt, p-mTOR, and p-P70S6K were significantly upregulated in TMEA-treated HEL cells compared to those in the control cells. Moreover, TMEA intervention enhanced the expression of the transcription factors NF-E2 and GATA-1 in HEL cells, which was confirmed by both western blot analysis and immunofluorescence staining. These findings not only demonstrate a bridge created between TMEA activity and the predicted mechanism but also demonstrate the indispensable functions of NF-E2 and GATA-1 as transcriptional regulators in megakaryocyte development (Takayama et al., 2010; Rost et al., 2018).
Moreover, the ROS generation could play pivotal roles in regulating the megakaryocyte differentiation (Chen et al., 2013; Yang et al., 2019). In the present study, the elevation in cellular ROS level due to TMEA treatment, confirming the differentiation and maturation of TMEA-induced megakaryocytes were accompanied by the increase of ROS. Also, apoptosis is important for megakaryocyte development and thrombopoiesis. Some scholars believed that it was necessary to inhibit the apoptosis of megakaryocytes in order to survive and proceed safely through the process of platelet shedding (Josefsson et al., 2011), while some others believed that megakaryocytes could shed platelets by promoting apoptosis (De et al., 2002). A preliminary study found no obvious apoptosis on the 4th day, whereas on the 8th and 12th days, the cells treated with TMEA showed concentration-dependent apoptosis. We suspected that TMEA had a protective effect on megakaryocytes in the early stage of TMEA intervention. Over time, megakaryocytes shed proplatelets by increasing apoptosis.
Taken together, our investigations profile the characteristics of TMEA action on megakaryocyte maturation/platelet production, from in vitro to in vivo experiments and from virtual prediction to experimental validation. However, in-depth explorations still need to be carried out, including pharmacokinetic analysis of TMEA administration and human primary megakaryocytes studies of TMEA therapy for thrombocytopenia.
In conclusion, the present study for the first time predicted the targets and signalling cascades of TMEA action against thrombocytopenia and determined conclusively that the regulation of the PI3K/Akt/mTOR/P70S6K/GATA-1/NF-E2 signalling pathway lies at a nexus of TMEA targeting and megakaryocytic differentiation into proplatelets. Our findings provide novel insight into potential thrombopoiesis-stimulating medications.
The raw data supporting the conclusions of this article will be made available by the authors, without undue reservation.
The animal study was reviewed and approved by the Ethics Committee of Southwest Medical University.
HL and XS performed the network pharmacological analysis. HL and XJ wrote the manuscript. HL, LW, and FH performed the in vivo experiments. YS, XJ, HL, and JL performed the in vitro experiments. XJ, NJ, and HL performed the statistical analysis. YL, AW, and DQ provided useful suggestions on the methodology. JZ and LY carried out UPLC-MS analysis. JW, QM, and JY designed the study, revised the manuscript, and approved the final proof as corresponding authors. All authors read and approved the final manuscript.
This research was funded by grants from the National Natural Science Foundation of China [Grant Nos. 81774013, 81804221, and 82074129], the National Major Science and Technology Project of the Ministry of Science and Technology of China [Grant No. 2018ZX09721004-006-004], the Science and Technology Planning Project of Sichuan Province, China [Grant Nos. 2018JY0237, 2019JDPT0010, 2019YJ0473, and 19PTDJ0026], the Educational Commission of Sichuan Province, China [Grant Nos. 18TD0051 and 18ZA0525], the Science and Technology Program of Luzhou, China [Grant Nos. 2017-S-39(3/5), 2020LZXNYDZ03, 2018LZXNYD-ZK49, and 2019ZZD006], the School-level Fund of Southwest Medical University [Grant Nos. 2017-ZRZD-017, 2017-ZRQN-081, 2020LZXNYDP01, 2019LZXNYDJ11, and 2018-JYJ-34].
The authors declare that the research was conducted in the absence of any commercial or financial relationships that could be construed as a potential conflict of interest.
All claims expressed in this article are solely those of the authors and do not necessarily represent those of their affiliated organizations, or those of the publisher, the editors and the reviewers. Any product that may be evaluated in this article, or claim that may be made by its manufacturer, is not guaranteed or endorsed by the publisher.
The Supplementary Material for this article can be found online at: https://www.frontiersin.org/articles/10.3389/fcell.2021.708331/full#supplementary-material
Aucar, M. G., and Cavasotto, C. N. (2020). Molecular docking using quantum mechanical-based methods. Methods Mol. Biol. 2114, 269–284. doi: 10.1007/978-1-0716-0282-9_17
Bai, C. F., Sun, Y. S., Pan, X. C., Yang, J., Li, X. X., Wu, A. G., et al. (2019). Antitumor effects of trimethylellagic acid isolated from Sanguisorba officinalis L. on colorectal cancer via angiogenesis inhibition and apoptosis induction. Front. Pharmacol. 10:1646. doi: 10.3389/fphar.2019.01646
Breitenlechner, C. B., Kairies, N. A., Honold, K., Scheiblich, S., Koll, H., Greiter, E., et al. (2005). Crystal structures of active SRC kinase domain complexes. J. Mol. Biol. 353, 222–231. doi: 10.1016/j.jmb.2005.08.023
Buitenhuis, M., and Coffer, P. J. (2009). The role of the PI3K-PKB signaling module in regulation of hematopoiesis. Cell Cycle. 8, 560–566. doi: 10.4161/cc.8.4.7654
Bury, L., Falcinelli, E., Chiasserini, D., Springer, T. A., Italiano, J. E., and Gresele, P. (2016). Cytoskeletal perturbation leads to platelet dysfunction and thrombocytopenia in variant forms of Glanzmann thrombasthenia. Haematologica 101, 46–56. doi: 10.3324/haematol.2015.130849
Casas, A. I., Hassan, A. A., Larsen, S. J., Gomez-Rangel, V., Elbatreek, M., Kleikers, P. W. M., et al. (2019). From single drug targets to synergistic network pharmacology in ischemic stroke. Proc. Natl. Acad. Sci. U.S.A. 116, 7129–7136. doi: 10.1073/pnas.1820799116
Castro, V. (2017). Human platelet antigens and primary immune thrombocytopenia. Rev. Bras. Hematol. Hemoter. 39, 95–97. doi: 10.1016/j.bjhh.2017.02.008
Chagas, C. M., Moss, S., and Alisaraie, L. (2018). Drug metabolites and their effects on the development of adverse reactions: revisiting Lipinski’s rule of five. Int. J. Pharmaceutics. 549, 133–149. doi: 10.1016/j.ijpharm.2018.07.046
Chen, S., Su, Y., and Wang, J. (2013). ROS-mediated platelet generation: a microenvironment-dependent manner for megakaryocyte proliferation, differentiation, and maturation. Cell Death Dis. 4:e722. doi: 10.1038/cddis.2013.253
Croft, K. D. (2016). Dietary polyphenols: antioxidants or not? Arch. BiocheIuistly Biophys. 5, 120–124. doi: 10.1016/j.abb.2015.11.014
Cullmann, K., Jahn, M., Spindler, M., Schenk, F., Manukjan, G., Mucci, A., et al. (2021). Forming megakaryocytes from murine-induced pluripotent stem cells by the inducible overexpression of supporting factors. Res. Pract. Thromb. Haemost. 5, 111–124. doi: 10.1002/rth2.12453
Daina, A., Michielin, O., and Zoete, V. (2017). SwissADME: a free web tool to evaluate pharmacokinetics, drug-likeness and medicinal chemistry friendliness of small molecules. Sci. Rep. 7:42717. doi: 10.1038/srep42717
De, B. S., Sabri, S., Daugas, E., Zermati, Y., Guidotti, J. E., Hermine, O., et al. (2002). Platelet formation is the consequence of caspase activation within megakaryocytes. Blood 100, 1310–1317. doi: 10.1182/blood-2002-03-0686
Delaney, M., Wendel, S., Bercovitz, R. S., Cid, J., Cohn, C., Dunbar, N. M., et al. (2016). Transfusion reactions: prevention, diagnosis, and treatment. Lancet 388, 2825–2836. doi: 10.1016/S0140-6736(15)01313-6
Deshayes, S., and Godeau, B. (2020). Second-line and beyond: treatment options for primary persistent and chronic immune thrombocytopenia. Platelets 31, 291–299. doi: 10.1080/09537104.2019.1636018
Drayer, A. L., Olthof, S. G., and Vellenga, E. (2006). Mammalian target of rapamycin is required for thrombopoietin-induced proliferation of megakaryocyte progenitors. Stem. Cells. 24, 105–114. doi: 10.1634/stemcells.2005-0062
Eto, K., and Kunishima, S. (2016). Linkage between the mechanisms of thrombocytopenia and thrombopoiesis. Blood 127, 1234–1241. doi: 10.1182/blood-2015-07-607903
Flaumenhaft, R., Dilks, J. R., Richardson, J., Alden, E., Patel-Hett, S. R., Battinelli, E., et al. (2009). Megakaryocyte-derived microparticles: direct visualization and distinction from platelet-derived microparticles. Blood 113, 1112–1121. doi: 10.1182/blood-2008-06-163832
Forouzesh, A., Samadi Foroushani, S., Forouzesh, F., and Zand, E. (2019). Reliable target prediction of bioactive molecules based on chemical similarity without employing statistical methods. Front. Pharmacol. 10:835. doi: 10.3389/fphar.2019.00835
Gao, R. L., and Chong, B. H. (2012). Research and development of the effective components of panaxdiol saponin as new Chinese patent medicine for treating hemocytopenia. Chin. J. Integr. Med. 18, 897–902. doi: 10.1007/s11655-012-1292-4
Gao, X. P., Wu, J. M., Zou, W. J., and Dai, Y. (2014). Two ellagic acids isolated from roots of Sanguisorba officinalis L. promote hematopoietic progenitor cell proliferation and megakaryocyte differentiation. Molecules 19, 5448–5458. doi: 10.3390/molecules19045448
Geue, S., Aurbach, K., Manke, M. C., Manukjan, G., Münzer, P., Stegner, D., et al. (2019). Pivotal role of PDK1 in megakaryocyte cytoskeletal dynamics and polarization during platelet biogenesis. Blood 134, 1847–1858. doi: 10.1182/blood.2019000185
Gfeller, D., Grosdidier, A., Wirth, M., Daina, A., Michielin, O., and Zoete, V. (2014). SwissTargetPrediction: a web server for target prediction of bioactive small molecules. Nucleic Acids Res. 42, W32–W38. doi: 10.1093/nar/gku293
Ghoshal, K., and Bhattacharyya, M. (2014). Overview of platelet physiology: its hemostatic and nonhemostatic role in disease pathogenesis. Sci. World J. 2014:781857. doi: 10.1155/2014/781857
Greenberg, E. M. (2017). Thrombocytopenia: a destruction of platelets. J. Infus. Nurs. 40, 41–50. doi: 10.1097/NAN.0000000000000204
Gu, L., Lu, J., Li, Q., Wu, N., Zhang, L., Li, H., et al. (2020). A network-based analysis of key pharmacological pathways of Andrographis paniculata acting on Alzheimer’s disease and experimental validation. J. Ethnopharmacol. 251:112488. doi: 10.1016/j.jep.2019.112488
Gudbrandsdottir, S., Leven, E., Imahiyerobo, A., Lee, C. S., and Bussel, J. (2020). Combination of thrombopoietin receptor agonists, immunosuppressants and intravenous immunoglobulin as treatment of severe refractory immune thrombocytopenia in adults and children. Br. J. Haematol. 189, e37–e40. doi: 10.1111/bjh.16426
Hähnke, V. D., Kim, S., and Bolton, E. E. (2018). PubChem chemical structure standardization. J. Cheminform. 10:36. doi: 10.1186/s13321-018-0293-8
Hao, D. C., and Xiao, P. G. (2014). Network pharmacology: a rosetta stone for traditional Chinese medicine. Drug Dev. Res. 75, 299–312. doi: 10.1002/ddr.21214
Hers, I., Vincent, E. E., and Tavaré, J. M. (2011). Akt signalling in health and disease. Cell. Signal. 23, 1515–1527. doi: 10.1016/j.cellsig.2011.05.004
Hou, X., Xiao, H., Zhang, Y., Zeng, X., Huang, M., Chen, X., et al. (2018). Transient receptor potential channel 6 knockdown prevents apoptosis of renal tubular epithelial cells upon oxidative stress via autophagy activation. Cell Death Dis. 9:1015. doi: 10.1038/s41419-018-1052-5
Hsin, K. Y., Ghosh, S., and Kitano, H. (2013). Combining machine learning systems and multiple docking simulation packages to improve docking prediction reliability for network pharmacology. PLoS. One 12:e83922. doi: 10.1371/journal.pone.0083922
Hsin, K. Y., Matsuoka, Y., Asai, Y., Kamiyoshi, K., Watanabe, T., Kawaoka, Y., et al. (2016). SystemsDock: a web server for network pharmacology-based prediction and analysis. Nucleic Acids Res. 44, 507–513. doi: 10.1093/nar/gkw335
Huang, N., Lou, M., Liu, H., Avila, C., and Ma, Y. P. (2016). Identification of a potent small molecule capable of regulating polyploidization, megakaryocyte maturation, and platelet production. J. Hematol. Oncol. 9:136. doi: 10.1186/s13045-016-0358-y
Huang da, W., Sherman, B. T., and Lempicki, R. A. (2009). Systematic and integrative analysis of large gene lists using DAVID bioinformatics resources. Nat. Protoc. 4, 44–57. doi: 10.1038/nprot.2008.211
Jiang, Y. Y., Liu, N., Zhu, S. R., Hu, X. M., Chang, D., and Liu, J. X. (2019). Elucidation of the mechanisms and molecular targets of Yiqi Shexue formula for treatment of primary immune thrombocytopenia based on network pharmacology. Front. Pharmacol. 10:1136. doi: 10.3389/fphar.2019.01136
Johnson, B., Fletcher, S. J., and Morgan, N. V. (2016). Inherited thrombocytopenia: novel insights into megakaryocyte maturation, proplatelet formation and platelet lifespan. Platelets 27, 519–525. doi: 10.3109/09537104.2016.1148806
Josefsson, E. C., James, C., Henley, K. J., Debrincat, M. A., Rogers, K. L., Dowling, M. R., et al. (2011). Megakaryocytes possess a functional intrinsic apoptosis pathway that must be restrained to survive and produce platelets. J. Exp. Med. 208, 2017–2031. doi: 10.1084/jem.20110750
Kaufman, R. M., Djulbegovic, B., Gernsheimer, T., Kleinman, S., Tinmouth, A. T., Capocelli, K. E., et al. (2015). Platelet transfusion: a clinical practice guideline from the AABB. Ann. Intern. Med. 162, 205–213. doi: 10.7326/M14-1589
Kaur, T., Madgulkar, A., Bhalekar, M., and Asgaonkar, K. (2019). Molecular docking in formulation and development. Curr. Drug Discov. Technol. 16, 30–39. doi: 10.2174/1570163815666180219112421
Lakshmanan, S., and Cuker, A. (2012). Contemporary management of primary immune thrombocytopenia in adults J. Thromb. Haemost. 10, 1988–1998. doi: 10.1111/j.1538-7836.2012.04876.x
Laplante, M., and Sabatini, D. M. (2012). mTOR signaling in growth control and disease. Cell 149, 274–293. doi: 10.1016/j.cell.2012.03.017
Liang, J. X., Bai, W. D., Yang, J., Tu, J. C., Charles Stephen, B., Deng, Z. H., et al. (2020). Research progress on plant polyphenols. Farm. Pmducts. Pmcessing. 515, 85–90.
Liebman, H. A., and Pullarkat, V. (2011). Diagnosis and management of immune thrombocytopenia in the era of thrombopoietin mimetics. Hematology Am. Soc. Hematol. Educ. Program. 2011, 384–390. doi: 10.1182/asheducation-2011.1.384
Limb, J. K., Song, D., Jeon, M., Han, S. Y., Han, G., Jhon, G. J., et al. (2015). 2- (trimethylammonium) ethyl (R)-3-methoxy-3-oxo-2-stearamidopropyl phosphate promotes megakaryocytic differentiation of myeloid leukaemia cells and primary human CD34+ haematopoietic stem cells. J. Tissue Eng. Regen. Med. 9, 435–446. doi: 10.1002/term.1628
Liu, Q., Luo, L., Ren, C., Zou, M., Yang, S., Cai, B., et al. (2020). The opposing roles of the mTOR signaling pathway in different phases of human umbilical cord blood-derived CD34+ cell erythropoiesis. Stem Cells 38, 1492–1505. doi: 10.1002/stem.3268
Liu, Z. J., Italiano, J. Jr., Ferrer-Marin, F., Gutti, R., Bailey, M., Poterjoy, B., et al. (2011). Developmental differences in megakaryocytopoiesis are associated with up-regulated TPO signaling through mTOR and elevated GATA-1 levels in neonatal megakaryocytes. Blood 117, 4106–4117. doi: 10.1182/blood-2010-07-293092
Luo, T. T., Lu, Y., Yan, S. K., Xiao, X., Rong, X. L., and Guo, J. (2020). Network pharmacology in research of Chinese medicine formula: methodology, application and prospective. Chin. J. Integr. Med. 26, 72–80. doi: 10.1007/s11655-019-3064-0
Lv, Y. N., Li, S. X., Zhai, K. F., Kou, J. P., and Yu, B. Y. (2014). Network pharmacology-based prediction and verification of the molecular targets and pathways for schisandrin against cerebrovascular disease. Chin. J. Nat. Med. 12, 251–258. doi: 10.1016/S1875-5364(14)60051-0
Malik, N., Sansom, O. J., and Michie, A. M. (2018). The role of mTOR-mediated signals during haemopoiesis and lineage commitment. Biochem. Soc. Trans. 46, 1313–1324. doi: 10.1042/BST20180141
Merő, B., Radnai, L., Gógl, G., Tőke, O., Leveles, I., Koprivanacz, K., et al. (2019). Structural insights into the tyrosine phosphorylation-mediated inhibition of SH3 domain-ligand interactions. J. Biol. Chem. 294, 4608–4620. doi: 10.1074/jbc.RA118.004732
Mojadidi, M. K., Galeas, J. N., Goodman-Meza, D., Eshtehardi, P., Msaouel, P., Kelesidis, I., et al. (2016). Thrombocytopaenia as a prognostic indicator in heart failure with reduced ejection fraction. Heart Lung Circ. 25, 568–575. doi: 10.1016/j.hlc.2015.11.010
Mones, J. V., and Soff, G. (2019). Management of thrombocytopenia in cancer patients. Cancer Treat. Res. 179, 139–150. doi: 10.1007/978-3-030-20315-3_9
Nakao, T., Geddis, A. E., Fox, N. E., and Kaushansky, K. (2008). PI3K/Akt/FOXO3a pathway contributes to thrombopoietin-induced proliferation of primary megakaryocytes in vitro and in vivo via modulation of p27Kip1. Cell Cycle. 7, 257–266. doi: 10.4161/cc.7.2.5148
Piñero, J., Bravo, A., Queralt-Rosinach, N., Gutiérrez-Sacristán, A., Deu-Pons, J., Centeno, E., et al. (2017). DisGeNET: a comprehensive platform integrating information on human disease-associated genes and variants. Nucleic Acids Res. 45, D833–D839. doi: 10.1093/nar/gkw943
Pinzi, L., and Rastelli, G. (2019). Molecular docking: Shifting paradigms in drug discovery. Int. J. Mol. Sci. 20:4331. doi: 10.3390/ijms20184331
Poklar Ulrih, N. (2017). Analytical techniques for the study of polyphenol-protein interactions. Crit. Rev. Food Sci. Nutr. 57, 2144–2161. doi: 10.1080/10408398.2015.1052040
Polak, R., and Buitenhuis, M. (2012). The PI3K/PKB signaling module as key regulator of hematopoiesis: implications for therapeutic strategies in leukemia. Blood 119, 911–923. doi: 10.1182/blood-2011-07-366203
Raslova, H., Roy, L., Vourc’h, C., Le Couedic, J. P., Brison, O., Metivier, D., et al. (2003). Megakaryocyte polyploidization is associated with a functional gene amplification. Blood 101, 541–544. doi: 10.1182/blood-2002-05-1553
Rebhan, M., Chalifa-Caspi, V., Prilusky, J., and Lancet, D. (1998). GeneCards: a novel functional genomics compendium with automated data mining and query reformulation support. Bioinformatics 14, 656–664. doi: 10.1093/bioinformatics/14.8.656
Rojnuckarin, P., and Kaushansky, K. (2001). Actin reorganization and proplatelet formation in murine megakaryocytes: the role of protein kinase c alpha. Blood 97, 154–161. doi: 10.1182/blood.v97.1.154
Rost, M. S., Shestopalov, I., Liu, Y., Vo, A. H., Richter, C. E., Emly, S. M., et al. (2018). Nfe2 is dispensable for early but required for adult thrombocyte formation and function in zebrafish. Blood Adv. 2, 3418–3427. doi: 10.1182/bloodadvances.2018021865
Rothwell, J. A., Knaze, V., and Zamora-Ros, R. (2017). Polyphenols: dietary assessment and role in the prevention of cancers. Curr. Opin. Clin. Nutr. Metab. Care. 20, 512–521. doi: 10.1097/MCO.0000000000000424
Saikia, S., and Bordoloi, M. (2019). Molecular docking: challenges, advances and its use in drug discovery perspective. Curr. Drug Targets 20, 501–521. doi: 10.2174/1389450119666181022153016
Santos, L. H. S., Ferreira, R. S., and Caffarena, E. R. (2019). Integrating molecular docking and molecular dynamics simulations. Methods Mol. Biol. 2053, 13–34. doi: 10.1007/978-1-4939-9752-7_2
Scalbert, A., Manach, C., Morand, C., Remésy, C., and Jiménez, L. (2005). Dietary polyphenols and the prevention of diseases. Crit. Rev. Food Sci. Nutr. 45, 287–306. doi: 10.1080/1040869059096
Schulze, H., and Italiano, J. (2016). Molecular and Cellular Biology of Platelet Formation. Salmon Tower Building, NY: Springer International Publishing, doi: 10.1007/978-3-319-39562-3
Stentoft, J. (2016). ITP: from idiopathic purpura to immune thrombocytopenia and back. Br. J. Haematol. 175, 755–756. doi: 10.1111/bjh.14414
Stockert, J. C., Blázquez-Castro, A., and Horobin, R. W. (2014). Identifying different types of chromatin using Giemsa staining. Methods Mol. Biol. 1094, 25–38. doi: 10.1007/978-1-62703-706-8_3
Sun, R. J., and Shan, N. N. (2019). Megakaryocytic dysfunction in immune thrombocytopenia is linked to autophagy. Cancer Cell Int. 19:59. doi: 10.1186/s12935-019-0779-0
Sun, X., Zhao, Y. N., Qian, S., Gao, R. L., Yin, L. M., Wang, L. P., et al. (2018). Ginseng-derived panaxadiol saponins promote hematopoiesis recovery in cyclophosphamide-induced myelosuppressive mice: potential novel treatment of chemotherapy-induced cytopenias. Chin. J. Integr. Med. 24, 200–206. doi: 10.1007/s11655-017-2754-8
Szklarczyk, D., Morris, J. H., Cook, H., Kuhn, M., Wyder, S., Simonovic, M., et al. (2017). The STRING database in 2017: quality-controlled protein-protein association networks, made broadly accessible. Nucleic Acids Res. 45, D362–D368. doi: 10.1093/nar/gkw937
Takayama, M., Fujita, R., Suzuki, M., Okuyama, R., Aiba, S., Motohashi, H., et al. (2010). Genetic analysis of hierarchical regulation for Gata1 and NF-E2 p45 gene expression in megakaryopoiesis. Mol. Cell Biol. 30, 2668–2680. doi: 10.1128/MCB.01304-09
Tresserra-Rimbau, A., Lamuela-Raventos, R. M., and Moreno, J. J. (2018). Polyphenols, food and pharma. Current knowledge and directions for future research. Biochem. Pharmacol. 156, 186–195. doi: 10.1016/j.bcp.2018.07.050
Tu, Y., Wang, K., Tan, L., Han, B., Hu, Y., Ding, H., et al. (2020). Dolichosin A, a coumestan isolated from Glycine tabacina, inhibits IL-1β-induced inflammation in SW982 human synovial cells and suppresses RANKL-induced osteoclastogenesis: From network pharmacology to experimental pharmacology. J. Ethnopharmacol. 258:112855. doi: 10.1016/j.jep.2020.112855
Vaijanathappa, J., Puttaswamygowda, J., Bevanhalli, R., Dixit, S., and Prabhakaran, P. (2019). Molecular docking_antiproliferative and anticonvulsant activities of Swertia marin isolated from Enicostemma axillare. Bioorg. Chem. 94:103428. doi: 10.1016/j.bioorg.2019.103428
Vainchenker, W., and Raslova, H. (2020). Megakaryocyte polyploidization: role in platelet production. Platelets 31, 707–716. doi: 10.1080/09537104.2019.1667497
Ve, T., Vajjhala, P. R., Hedger, A., Croll, T., DiMaio, F., Horsefield, S., et al. (2017). Structural basis of TIR-domain-assembly formation in MAL- and MyD88-dependent TLR4 signaling. Nat. Struct. Mol. Biol. 24, 743–751. doi: 10.1038/nsmb.3444
Wang, C. Y., Ma, S., Bi, S. J., Su, L., Huang, S. Y., Miao, J. Y., et al. (2019). Enhancing autophagy protects platelets in immune thrombocytopenia patients. Ann. Transl. Med. 7:134. doi: 10.21037/atm.2019.03.04
Wang, W., Wang, S., Liu, T., Ma, Y., Huang, S., Lei, L., et al. (2020). Resveratrol: multi-targets mechanism on neurodegenerative diseases based on network pharmacology. Front. Pharmacol. 11:694. doi: 10.3389/fphar.2020.00694
Wang, X. M., Chu, Y. J., Wang, W. L., and Yuan, W. P. (2016). mTORC signaling in hematopoiesis. Int. J. Hematol. 103, 510–518. doi: 10.1007/s12185-016-1944-z
Wang, Z. Y., Jiang, Z. M., Xiao, P. T., Jiang, Y. Q., Liu, W. J., and Liu, E. H. (2020). The mechanisms of baicalin ameliorate obesity and hyperlipidemia through a network pharmacology approach. Eur. J. Pharmacol. 878:173103. doi: 10.1016/j.ejphar.2020.173103
Xu, Y., Hu, M., Chen, S., Chen, F., Wang, C., Tang, Y., et al. (2018). Tannic acid attenuated irradiation-induced apoptosis in megakaryocytes. Exp. Cell Res. 370, 409–416. doi: 10.1016/j.yexcr.2018.07.003
Yang, H., Rudge, D. G., Koos, J. D., Vaidialingam, B., Yang, H. J., and Pavletich, N. P. (2013). mTOR kinase structure, mechanism and regulation. Nature 497, 217–223. doi: 10.1038/nature12122
Yang, J., Zhao, S., and Ma, D. (2019). Biological characteristics and regulation of early megakaryocytopoiesis. Stem. Cell Rev. Rep. 15, 652–663. doi: 10.1007/s12015-019-09905-3
Zhang, N. Y., Santoso, S., Aster, R. H., Curtis, B. R., and Newman, P. J. (2019). Bioengineered iPSC-derived megakaryocytes for the detection of platelet-specific patient alloantibodies. Blood 134, e1–e8. doi: 10.1182/blood.2019002225
Zhang, X., Campreciós, G., Rimmelé, P., Liang, R., Yalcin, S., Mungamuri, S. K., et al. (2014). FOXO3-mTOR metabolic cooperation in the regulation of erythroid cell maturation and homeostasis. Am. J. Hematol. 89, 954–963. doi: 10.1002/ajh.23786
Zhou, F., Li, X., Wang, W., Zhu, P., Zhou, J., He, W., et al. (2016). Tracing haematopoietic stem cell formation at single-cell resolution. Nature 533, 487–492. doi: 10.1038/nature17997
Keywords: thrombocytopenia, Sanguisorba officinalis L., PI3K, megakaryocyte differentiation, 3, 3′, 4′-trimethylellagic acid
Citation: Li H, Jiang X, Shen X, Sun Y, Jiang N, Zeng J, Lin J, Yue L, Lai J, Li Y, Wu A, Wang L, Qin D, Huang F, Mei Q, Yang J and Wu J (2021) TMEA, a Polyphenol in Sanguisorba officinalis, Promotes Thrombocytopoiesis by Upregulating PI3K/Akt Signaling. Front. Cell Dev. Biol. 9:708331. doi: 10.3389/fcell.2021.708331
Received: 11 May 2021; Accepted: 28 July 2021;
Published: 17 August 2021.
Edited by:
Yibo Luo, University of Toledo, United StatesReviewed by:
Camelia Iancu-Rubin, Icahn School of Medicine at Mount Sinai, United StatesCopyright © 2021 Li, Jiang, Shen, Sun, Jiang, Zeng, Lin, Yue, Lai, Li, Wu, Wang, Qin, Huang, Mei, Yang and Wu. This is an open-access article distributed under the terms of the Creative Commons Attribution License (CC BY). The use, distribution or reproduction in other forums is permitted, provided the original author(s) and the copyright owner(s) are credited and that the original publication in this journal is cited, in accordance with accepted academic practice. No use, distribution or reproduction is permitted which does not comply with these terms.
*Correspondence: Qibing Mei, cWJtZWlAbndwdS5lZHUuY24=; Jing Yang, eWFuZ2ppbmdfMDYxN0AxNjMuY29t; Jianming Wu, amlhbm1pbmd3dUBzd211LmVkdS5jbg==
†These authors have contributed equally to this work and share first authorship
Disclaimer: All claims expressed in this article are solely those of the authors and do not necessarily represent those of their affiliated organizations, or those of the publisher, the editors and the reviewers. Any product that may be evaluated in this article or claim that may be made by its manufacturer is not guaranteed or endorsed by the publisher.
Research integrity at Frontiers
Learn more about the work of our research integrity team to safeguard the quality of each article we publish.