- 1School of Biochemistry and Cell Biology, University College Cork, Cork, Ireland
- 2Shemyakin-Ovchinnikov Institute of Bioorganic Chemistry, RAS, Moscow, Russia
Detection of translation in so-called non-coding RNA provides an opportunity for identification of novel bioactive peptides and microproteins. The main methods used for these purposes are ribosome profiling and mass spectrometry. A number of publicly available datasets already exist for a substantial number of different cell types grown under various conditions, and public data mining is an attractive strategy for identification of translation in non-coding RNAs. Since the analysis of publicly available data requires intensive data processing, several data resources have been created recently for exploring processed publicly available data, such as OpenProt, GWIPS-viz, and Trips-Viz. In this work we provide a detailed demonstration of how to use the latter two tools for exploring experimental evidence for translation of RNAs hitherto classified as non-coding. For this purpose, we use a set of transcripts with substantially different patterns of ribosome footprint distributions. We discuss how certain features of these patterns can be used as evidence for or against genuine translation. During our analysis we concluded that the MTLN mRNA, previously misannotated as lncRNA LINC00116, likely encodes only a short proteoform expressed from shorter RNA transcript variants.
Introduction
Ribosome profiling, or footprinting (a.k.a. Ribo-seq), has allowed for a detailed assessment of whole cellular transcriptomes (Ingolia et al., 2009). The Ribo-seq technique enables this by generating a snapshot of active ribosome locations at a given moment by only sequencing the parts of RNA molecules protected by the ribosome during translation, which are termed ribosome protected fragments (RPFs) or ribosome footprints (Ingolia, 2014). These data are used for inferring parameters of translation, including translation rates of individual mRNAs, differential translation, ribosome pause detection and identification of translated open reading frames (ORFs), among others (Ingolia, 2014; Brar and Weissman, 2015; Andreev et al., 2017). A plethora of computational approaches and software tools have been developed for the analysis of ribosome profiling data (Kiniry et al., 2020). Among many findings made with the use of ribosome profiling were observations of translation of some of the RNA molecules that were previously classified as non-coding RNA (ncRNA).
The term “non-coding RNA” had classically referred to a very large and diverse group of RNA molecules that number in the thousands (Cheng et al., 2005; Birney et al., 2007; Washietl et al., 2007; van Bakel et al., 2010; Shahrouki and Larsson, 2012). Within non-coding RNA, RNAs longer than 200 nucleotides were classified as long non-coding RNA (lncRNA), while shorter transcripts were referred to as small RNAs. These small RNAs were sub-divided into transfer RNAs (tRNAs), micro RNAs (miRs), short-interfering RNAs (siRNAs), piwi interacting RNAs (piRNAs) etc. (Storz, 2002; Großhans and Filipowicz, 2008; Fang and Fullwood, 2016).
The evidence that lncRNAs can be translated was initially provided by Ingolia et al. (2011). Later, by analyzing available data, Chew et al., demonstrated that the high ribosomal occupancy in many lncRNAs resembles that in 5′ leaders of protein coding mRNAs (Chew et al., 2013). The 5′ leader sequences often contain translated short open reading frames, providing an argument in support of translation within lncRNAs. A counter argument was made by Guttman et al., who used ribosome footprint density at stop codons as a signature of genuine translation and developed ribosome release score (RRS) to measure it (Guttman et al., 2013). High RRSs are observed for long protein coding ORFs, but not for short ORFs in 5′ leaders and lncRNAs. This argument, however, is flawed, as it only shows that re-initiation and leaky scanning are infrequent downstream of long protein coding ORFs. Indeed, translation of downstream ORFs is observed only in rare cases downstream of relatively short ORFs lacking ATG codons within the entire coding sequence (Benitez-Cantos et al., 2020) or during equally infrequent stop codon readthrough (Loughran et al., 2014). However, when ORFs are short and their translation is inefficient, re-initiation (Munzarová et al., 2011) and leaky scanning (Michel et al., 2014a) are possible, so that the 5′ leaders and lncRNAs could have multiple, often overlapping ORFs that are translated. Subsequently, Ingolia et al. (2014) developed an approach for discriminating genuine translation from aberrant RNA protection by the ribosome or other large ribonucleoprotein complexes with the analysis of the distribution of ribosome footprint lengths, called the fragment length organization similarity score, FLOSS. FLOSS scores appear to be similar for protein coding ORFs, 5′ leaders and lncRNAs, but were distinct for the protected fragments derived from RNAs with known non-coding functions (Ingolia et al., 2014). While there is an overwhelming body of evidence that many lncRNAs have translated ORFs, it is unlikely that many of them code for stable protein products because the lack of long ORFs and of nucleotide substitution patterns typical for protein coding evolution. Although the functional significance of the translated ORFs remains largely unclear, emerging data suggest certain possibilities, such as ribosome assisted RNA processing (Sun et al., 2020).
Several mRNAs coding for small proteins were initially misclassified as lncRNAs, and some of them were “upgraded” to the status of mRNAs after their products have been identified and characterized. An example is LINC00116 that was found to code for a 56-amino acid functional microprotein found in mitochondria (Catherman et al., 2013). Later, it was independently rediscovered and characterized by several groups, named mitoregulin and assigned the protein coding gene symbol MTLN (Stein et al., 2018; Chugunova et al., 2019; Lin et al., 2019). Mitoregulin has been shown to enhance mitochondrial respiratory activity (Chugunova et al., 2019) and play a regulatory role in adipocyte metabolism (Friesen et al., 2020).
There are many other examples of microprotein encoding mRNAs misclassified as non-coding; a 46-amino acid microprotein, myoregulin encoded by LINC00948 (Anderson et al., 2015); the 7-kilodalton microprotein, non-annotated P-body dissociating polypeptide (NoBody) encoded by LINC01420 (D’Lima et al., 2017); the microprotein, CIP2A-BP encoded by LINC00665 that inhibits triple negative breast cancer progression (Guo et al., 2020), and the small endogenous peptide, SMIM30, which promotes hepatocellular cancer tumorigenesis, encoded by LINC00998 (Pang et al., 2020) to name a few. Nonetheless, it is likely that more await discovery, and therefore analysis of lncRNA translation and protein coding potential is an active area of research.
A number of tools have been developed for automatic detection of translated ORFs using Ribo-seq data (Crappé et al., 2015; Fields et al., 2015; Ji et al., 2015; Raj et al., 2016; Reuter et al., 2016; Erhard et al., 2018; Xiao et al., 2018; Brunet et al., 2019), their predictions vary and in the absence of a gold standard, their accuracies are difficult to estimate (Baranov and Michel, 2016). RNA protection from nuclease digestion could also occur from large RNA-protein complexes other than the ribosome. In fact, a tool Rfoot has been developed specifically for identification of such RNase protection due to RNA-binding proteins (Ji, 2018). It has been discussed that ribosomal footprints can be differentiated from non-ribosomal activity via differences in footprint length and lack of triplet periodicity (Ji et al., 2016; Ingolia et al., 2019). Therefore, for accurate and reliable detection of genuinely translated ORFs and protein-coding potential, it is often necessary to carefully examine available data manually. Here, we demonstrate how publicly available ribosome profiling data can be explored using ribosome profiling data resources from RiboSeq. Org portal, Trips-Viz (TRanscriptome-wide Information on Protein Synthesis-Visualized) and GWIPS-viz (Genome Wide Information on Protein Synthesis-visualized).
Trips-Viz is a graphical user interface (GUI) on-line platform that allows for interactive analysis and visualization of Ribo-seq and shotgun RNA sequencing (RNA-seq) data aligned to transcriptomes (Kiniry et al., 2019, 2021). To date Trips-Viz contains 2064 Ribo-seq files and 752 RNA-seq files from 114 studies across nine organisms. In the section “Setup and Configurations,” we describe in detail how to use the relevant functionalities of Trips-Viz. In the section “Data exploration in the context of individual RNA sequences,” we examine a selection of transcripts that illustrate different patterns of ribosomal footprints aligned to them and evaluate these patterns for genuine translation, see Table 1. The GWIPS-viz browser provides visualization of unambiguously mapped footprints to reference genomes (Michel et al., 2014b) and its use is necessary in order to evaluate how well transcript annotations and gene structures are supported by available data. In addition, we use the codon alignment viewer (CodAlignView) that is helpful for visualization of codon substitution that can reflect evolutionary selection acting on protein coding sequences (Jungreis et al., 2021).
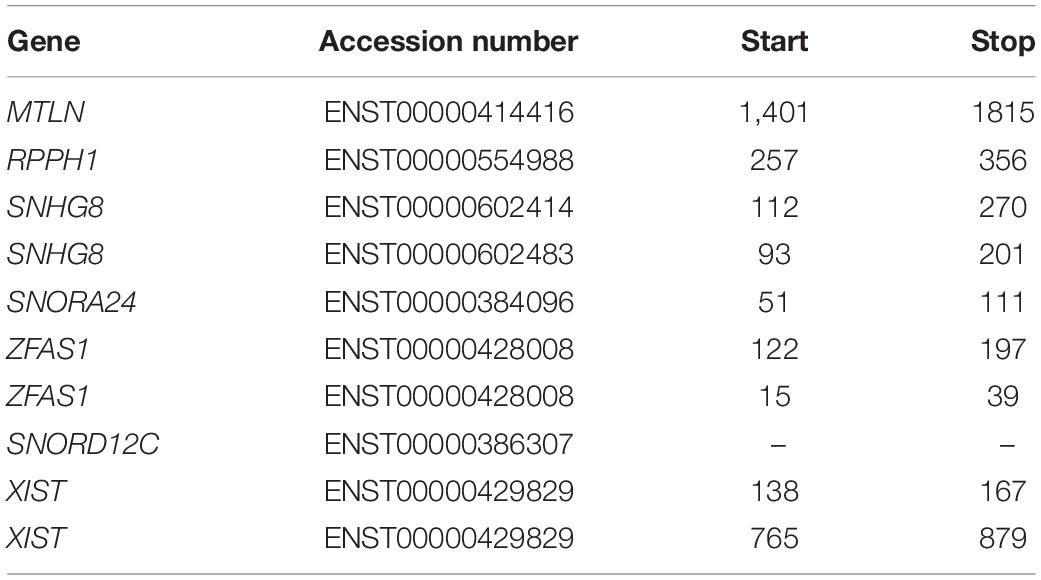
Table 1. List of RNAs examined with the corresponding accession number and transcript coordinates of the ORFs in order of appearance.
Setup and Configurations
Trips-Viz1 provides data aligned to the transcriptomes of several organisms and a rich repertoire of functional visualizations for the analysis of ribosome profiling data. Here we focus on Homo sapiens and the function “Single transcript plot” to manually examine transcripts of interest. For further explanation on the other analyses available within Trips-Viz, please refer to detailed instructions and videos available within the Trips-Viz platform (Kiniry et al., 2019, 2021).
Using prior knowledge, we selected the translated ORF on MTLN mRNA (formerly LINC00116) to serve as an example for genuine translation. As an example of a ncRNA whose ORF translation is unlikely we chose RPPH1 that encodes for the RNA component of RNase P. We further explored ribosome footprints aligned to SNHG8, ZFAS1, and XIST. The translation of all three lncRNAs has been reported previously (Ji et al., 2015; Calviello et al., 2016; Martinez et al., 2020), the translation of SNHG8 and ZFAS1 was also reported in additional studies (van Heesch et al., 2019; Gaertner et al., 2020) and the translation of ZFAS1 was also reported by Chen et al. (2020).
While the default options for “Single transcript plot” are usually adequate for initial analysis, there are several parameters that could affect the analysis and their meaning needs to be explained. “Min triplet periodicity score” is a threshold used to filter the data based on the strength of triplet periodicity signal. Triplet periodicity can be used for identification of the reading frame of translation (Michel et al., 2012). Triplet periodicity, as well as other parameters of ribosome profiling data, vary considerably across different studies (O’Connor et al., 2016). Therefore, not all data offer the same power to accurately identify the translated reading frame. To improve the quality of this parameter we used a triplet periodicity score cutoff of 0.5, meaning any read lengths with a score less than this would not be displayed. In addition to improving detection of the footprints’ frame of origin, good triplet periodicity is also an indirect signature of good data quality. Although reducing the number of reads analyzed does reduce the coverage and potentially exclude the detection of certain lowly translated ORFs, a reasonably large number of Ribo-seq datasets pass the 0.5 threshold, see Table 2.
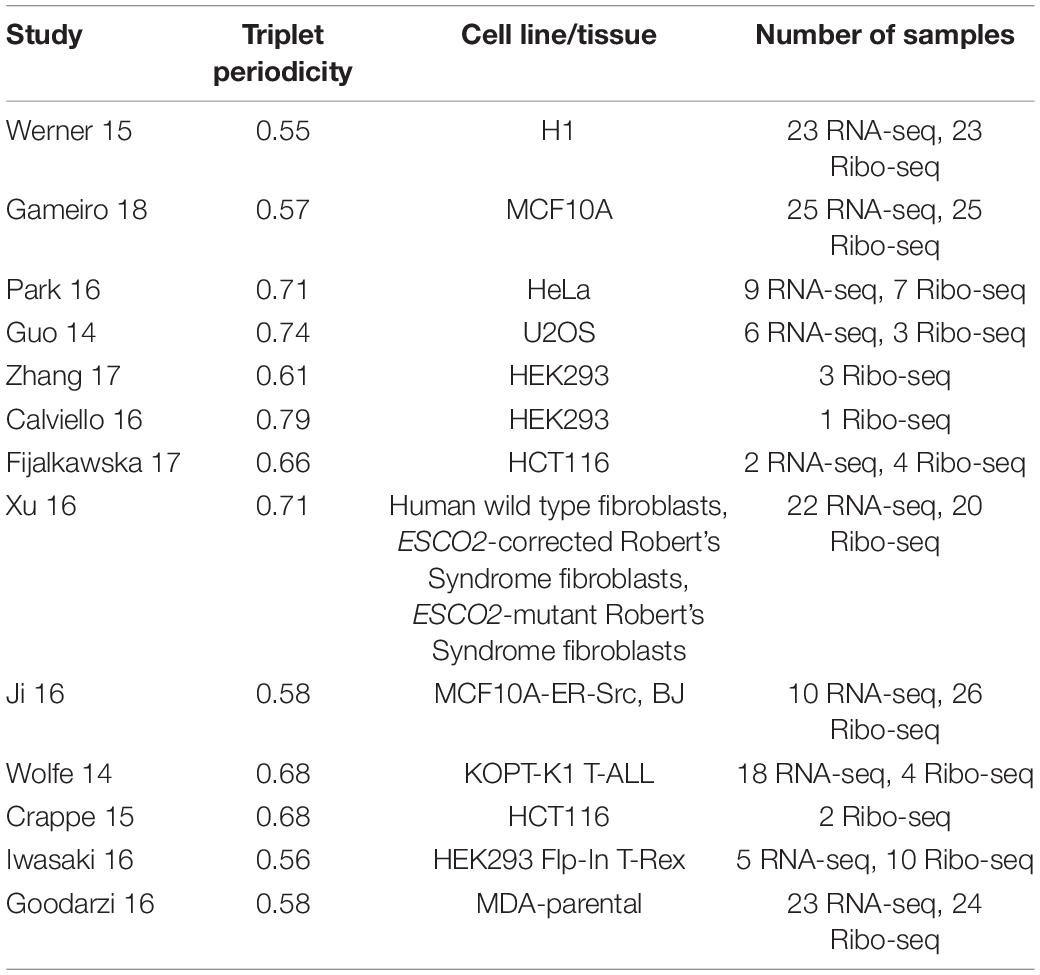
Table 2. List of studies used for the ‘single transcript plot’ analysis with corresponding triplet periodicity scores, cell line/tissue and number of samples for each study (Guo et al., 2014; Wolfe et al., 2014; Crappé et al., 2015; Werner et al., 2015; Calviello et al., 2016; Goodarzi et al., 2016; Iwasaki et al., 2016; Ji et al., 2016; Park et al., 2016; Xu et al., 2016; Fija-Lkowska et al., 2017; Zhang et al., 2017; Gameiro and Struhl, 2018).
Another important parameter is the use of ambiguously mapped reads. Ribosome footprints are short and therefore often cannot be unambiguously aligned. Enabling such multimapping creates an uncertainty regarding the true origin of the footprint. However, disabling multimapping results in a reduction of footprint density in the areas that share similarity with other sequences from the same genome. A number of approaches to mitigate this issue has been developed, see Kiniry, Michel and Baranov for a review (Kiniry et al., 2020). Trips-Viz, however, can either enable or disable ambiguous reads mapping. Here we disable multimapping by default to maximize the specificity, but sometimes explore ribosome profiling density plots under both modes, as this may help in interpretation of data for genes occurring in multiple copies and for closely related paralogs. In addition, when available, the corresponding RNA-seq studies were also enabled. Distribution of RNA-seq reads can be used to assess whether the annotation of a transcript is supported by the data, as well as to assess the mappability of corresponding regions. Changes in RNA-seq coverage could indicate regions that are difficult to sequence or to align, although RNA-seq data can exhibit its own RNA-seq specific biases, such as an increase of density toward the 3′end due to preferential capture of polyadenylated RNA fragments when poly-dT is used for mRNA capture (Weinberg et al., 2016). We visualized exon locations by enabling “Exon Junctions” on the generated plot legends tab which makes it easier to track in conjunction with genomic alignments. Finally in some individual cases, we also used mass spectrometry data available in Trips-Viz.
For visualization of genomic alignments, assessment of gene structures and selection of most appropriate transcript isoforms, we used the GWIPS-viz browser. Unlike Trips-Viz, GWIPS-viz provides ribosome profiling data aligned to the reference genome sequences, instead of transcriptome sequences. GWIPS-viz is based on the UCSC genome browser (Navarro Gonzalez et al., 2021) and is easy to use for anyone familiar with the latter. In addition to ribosome profiling data tracks, GWIPS-viz provides a number of auxiliary tracks that are helpful in the interpretation of ribosome profiling data, such as annotation tracks. Here we used the following tracks: “Basic Annotation Set from Gencode Version 25”; “mRNA-seq coverage from all studies,” which is a global aggregate for the number of RNA-seq reads aligned to each coordinate; “Ribosome profiles from all studies,” which is the visualization of inferred coordinates of ribosome A-sites (elongating ribosomes); “Initiating ribosome profiles from all studies” is the track for P-sites of ribosomes captured with translation inhibitors that preferentially arrest initiating ribosomes (Ingolia et al., 2011; Lee et al., 2012). Finally, we also enabled “Basewise Conservation by PhyloP100way” for assessment of nucleotide sequence conservation (Pollard et al., 2010). The default color scheme: elongating ribosome profiles are shown in red; initiating ribosome profiles are in blue; while, RNA-seq data are in green (Supplementary Figure 1A). It is important to note that while Trips-Viz alignments are strand-specific since transcripts are single stranded, GWIPS-viz alignments are not strand specific. Strand-specificity is provided only for bacterial genomes where a large proportion of genes overlap and the data interpretation would be difficult otherwise. Strand-specificity is provided only for bacterial genomes where a large proportion of genes overlap and the data interpretation would be difficult otherwise. Translation of overlapping antisense lncRNAs has been reported in mammals (Ruiz-Orera and Albà, 2019); hence, to properly analyze the corresponding loci, it is important to explore the corresponding RNAs in Trips-Viz.
In addition to ribosome profiling data resources, we took advantage of CodAlignView2, which differentially colors synonymous and non-synonymous codon substitutions, while also differentially coloring the latter depending on whether they lead to similar or radical changes according to BLOSUM62 (Jungreis et al., 2021). Such visualizations enable manual exploration of evolutionary selection acting on potential protein coding sequences, as synonymous and conservative non-synonymous substitutions are more frequent in protein coding sequences than radical, non-synonymous substitutions (M. F. Lin et al., 2011). The tool also differentially highlights stop codons and ATG codons, and visualizes other features such as predicted splice sites (Supplementary Figure 1B).
Data Exploration in the Context of Individual RNA Sequences
MTLN mRNA as an Example of Genuine Protein-Producing Translation
As mentioned earlier, MTLN was previously misannotated as lncRNA LINC00116. Since its productive translation has been extensively characterized, we used it as a “gold standard” example.
Figure 1A shows RNA-seq and Ribo-seq data aligned to the sequence of longest MTLN mRNA isoform (ENST00000414416). However, there appears to be no RNA-seq coverage for most of the annotated sequence up to ∼1500 nucleotides (nt) downstream of the annotated transcript 5′ end. For reference, we have also included a Trips-Viz visualization with ambiguously mapped reads enabled (Supplementary Figure 2A). When ambiguous mapping is allowed, only an isolated peak of RNA-seq emerges in the region of the second exon, ∼500 nt. The discontinuous RNA-seq coverage strongly suggest that this peak is an artifact of ambiguous mapping. Thus, the data suggest that a much shorter transcript is transcribed in all cells that were used for producing these data (Table 2). Consistent with that, ribosome profiling data appears only downstream of the fifth ATG in the annotated CDS (third in CDS frame). Indeed, if the entire annotated transcript were to be translated, how would preinitiation ribosome complexes reach the annotated coding sequence (CDS), bypassing ∼25 ATGs upstream? Existence of a shorter transcript explains this conundrum as the fifth ATG in the annotated CDS appears to be the first ATG in the truncated transcript supported by both the RNA-seq and Ribo-seq data, furthermore initiation at this ATG would be expected under the classic scanning model of translation initiation. Triplet periodicity strongly supports translation of the annotated CDS frame (frame three) indicating the genuine “translational” nature of Ribo-seq reads. Trips-Viz also contains proteomics data on the peptide masses that can be matched in mass-spectrometry datasets using MSFragger (Kong et al., 2017) and Philosopher (da Veiga Leprevost et al., 2020). Supplementary Figure 2B shows a screenshot of available data. Interestingly, while the most abundant peptides match MTLN CDS (in blue), there are also peptides whose masses matches products of conceptual translation of other reading frames (green and red), they may represent false positives.
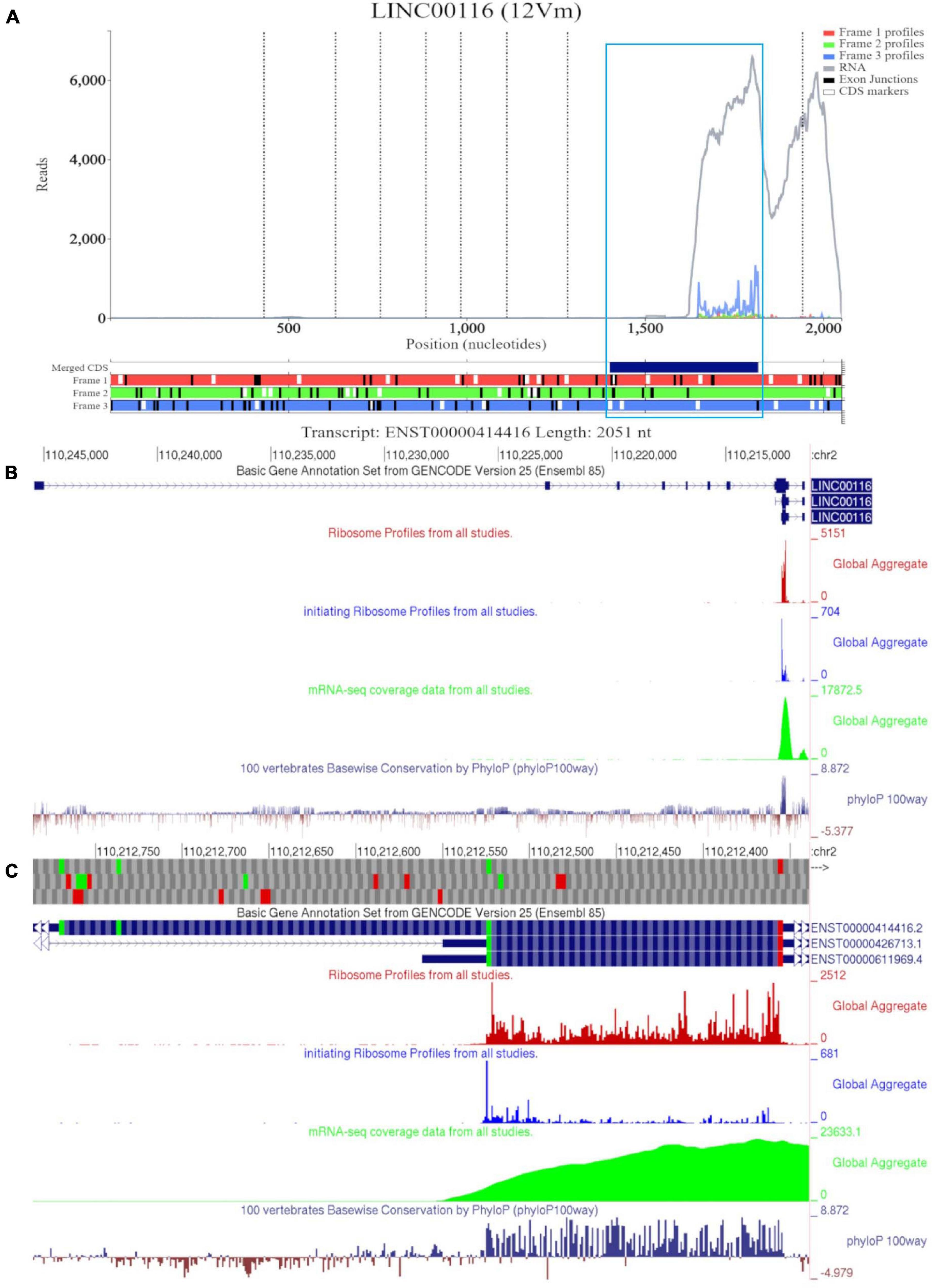
Figure 1. Visualization of MTLN/LINC00116 in Trips-Viz and GWIPS-Viz. (A) Whole transcript view of MTLN in Trips-Viz with data indicating the protein product is shorter than the annotated coding sequence. (B) Visualization of MTLN/LINC00116 in GWIPS-Viz with the ‘Basic Gene Annotation Set from Gencode Version 25,’ ‘Ribosome Profiles from all studies,’ ‘initiating Ribosome Profiles from all studies,’ ‘mRNA-seq coverage data from all studies’ and ‘100 vertebrates Basewise Conservation by PhyloP100way’ tracks enabled. (C) Coding sequence on MTLN in GWIPS-viz with the tracks described in Setup and Configurations enabled.
GWIPS-viz can be used to further explore whether the annotated transcript is supported by available RNA-seq and Ribo-seq data. For example, it is possible that some of the annotated introns are retained in mature RNA transcripts and would not be represented in Gencode and subsequently in Trips-Viz. Since the data are aligned to the genomes in GWIPS-viz, such problems with transcript annotations can be spotted. GWIPS-viz also provides an easy way to examine which RNA isoform is best supported by the data when multiple isoforms are present. The analysis of the MTLN locus on GWIPS-viz (Figure 1B) did not reveal the presence of RNA-seq or Ribo-seq reads in addition to what is seen in Trips-Viz. Further, it can be seen that in addition to the long isoform, there are two additional short isoforms (ENST00000426713 and ENST00000611969), with annotated CDS starts from the same start codon that we proposed on the analysis of data in Trips-Viz. Figure 1C shows an enlarged view of this area. A high peak of footprints obtained by enriching ribosomes at the initiating sites can be seen to match the same ATG. The same region also displays high nucleotide conservation in the PhyloP track with a pattern of triplet periodicity typical to protein coding regions due to higher frequency of substitutions in the third subcodon position relative to the first and second subcodon positions. The substitution patterns can be explored more reliably with CodAlignView (Figure 2), where a white color indicates absolute nucleotide conservation; while predominance of green (synonymous or conservative substitutions) is reflective of protein coding evolution. Yet again, the “green” region coincides with the region of high Ribo-seq density observed in the CDSs of shorter RNA isoforms. For reference, the alignment set used in CodAlignView was the 24-mammal subset of the 100-way vertebrate alignment using the hg38 human genome assembly (Rosenbloom et al., 2015). Of note, it was the shorter proteoform that was detected and characterized in previous studies (Catherman et al., 2013; Stein et al., 2018; Chugunova et al., 2019; Lin et al., 2019).
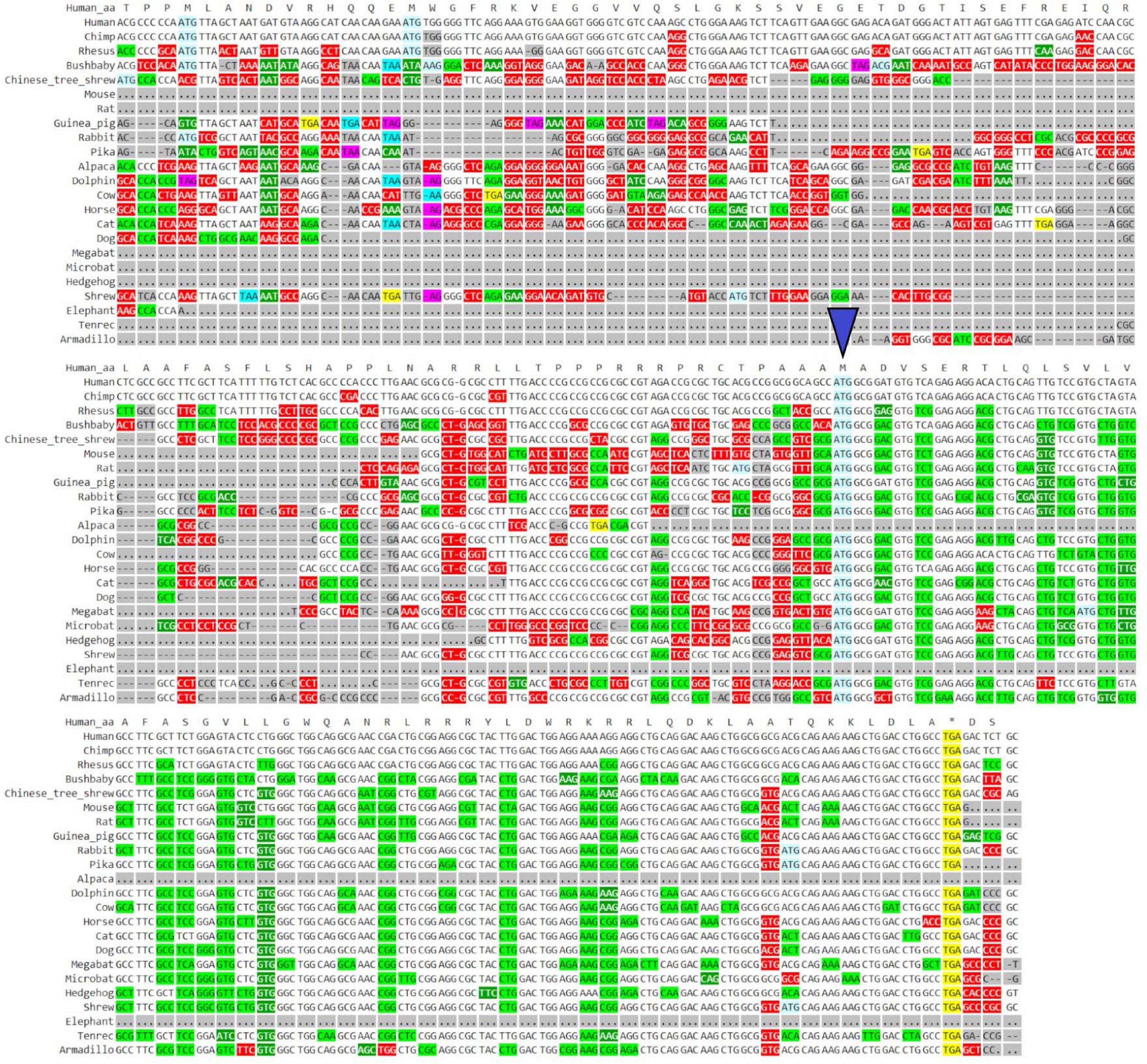
Figure 2. Visualization of MTLN/LINC00116 in CodAlignView. Coding sequence of MTLN visualized in CodAlignView with a blue triangle pointing toward the third ATG start site which correlates with the region where a high ratio of synonymous codon substitutions begins.
In summary, the translation of a short proteoform from the short RNA isoforms of MTLN gene is supported by all types of data explored here. This provides a good reference point for the case of genuine translation resulting in production of a stable protein.
RNase P RNA as an Example of Untranslated RNA
RNase P is a large nucleoprotein complex responsible for processing many RNA molecules (Evans et al., 2006). The RNA component of RNase P is transcribed by polymerase III and therefore is not capped (Schramm and Hernandez, 2002). Thus, it is extremely unlikely to be translated, yet fragments of RNase P RNA could contaminate Ribo-Seq data due to protection within the complex and co-isolation with ribosomes. Therefore, we chose the RNA component of RNase P as an example of an untranslated non-coding RNA. In humans it is encoded by the RPPH1 gene.
Figure 3A shows a Trips-Viz screenshot displaying the data aligned to the long RNA isoform RPPH1 (ENST00000554988). Like in the previous case, only part of the annotated transcript is supported by RNA-seq data as visualized in the GWIPS-viz browser (Figure 3C), indicating the presence of the shorter transcript isoform (ENST00000516869). There are several isolated peaks of ribosome footprint density across the transcript that do not correspond to a single ORF. One of the longest ORFs, with the largest number of footprints aligned to it, is in the second (green) reading frame and is depicted within a blue rectangle on Figure 3A. It can be explored at higher magnification in Figure 3B. The mapped reads do not show any triplet periodicity, indicating there is no preferential support for a specific reading frame. The PhyloP track in GWIPS-viz (Figure 3C) indicates high, nucleotide conservation expected for the sequence of this important housekeeping RNA molecule. However, it does not exhibit a pattern characteristic for protein coding evolution (prevalence of synonymous and positive non-synonymous codon substitutions over radical non-synonymous substitutions, see Figure 3D). Thus, RPPH1 represents a genuine example of an untranslated non-coding RNA, with aligned Ribo-seq data that most likely has origins other than protection by translating ribosomes.
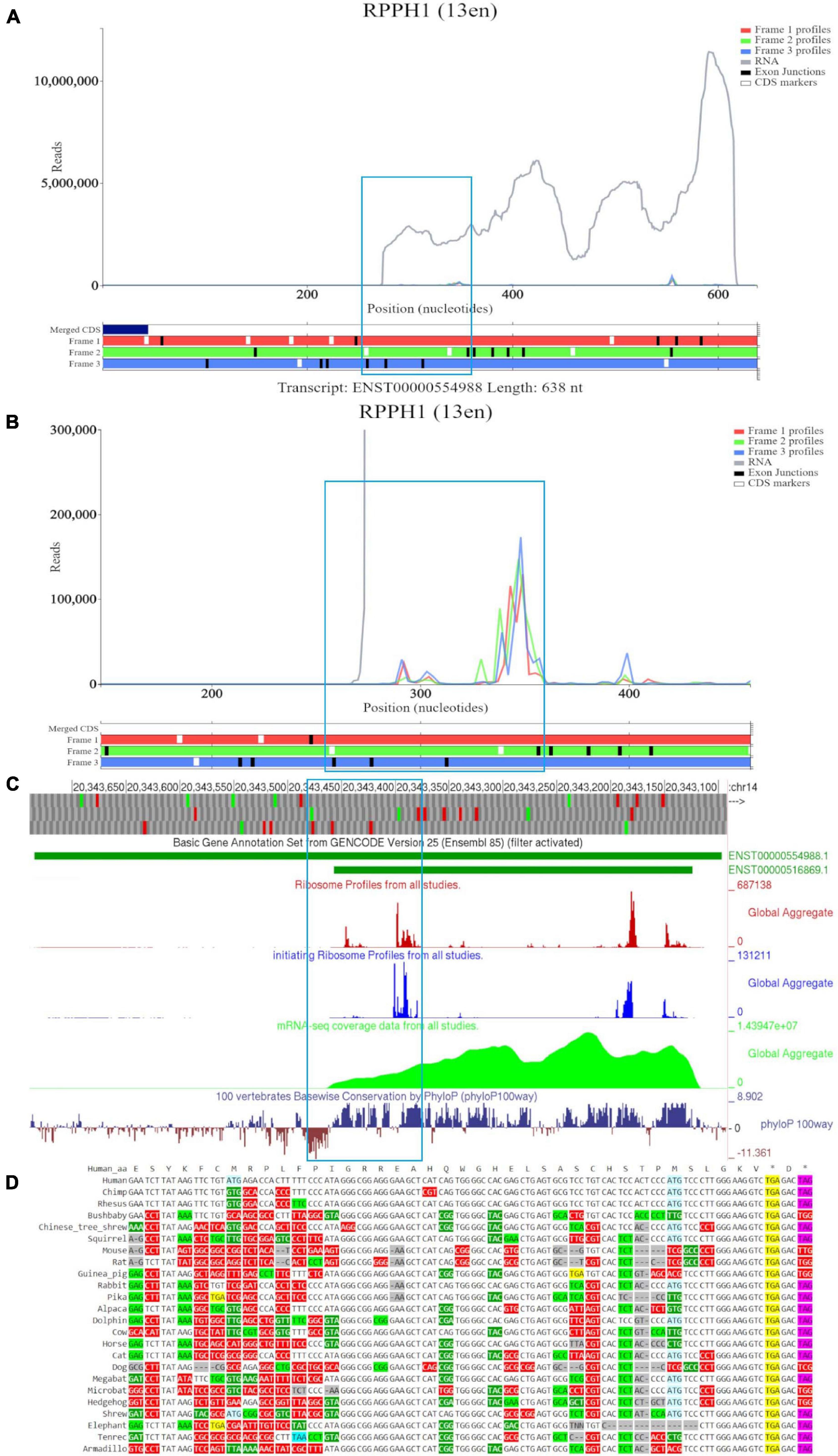
Figure 3. Visualization of RPPH1 in Trips-Viz, GWIPS-Viz and CodAlignView. (A) Whole transcript view of RPPH1 in Trips-Viz with the ORF outlined in blue. (B) Enlarged view of the ORF on RPPH1 in Trips-Viz (C) Visualization of the RPPH1 in GWIPS-viz with the tracks described in Setup and Configurations enabled. (D) CodAlignView of the ORF on RPPH1 showing predominantly absolute codon conservation.
Examples of Translation That Are Unlikely to Produce Proteins
For the exploration of translation of lncRNAs whose translational status is less clear, we chose SNHG8, ZFAS1, and XIST. Their translation has been previously reported by several independent ribosome profiling studies (Ji et al., 2015; Calviello et al., 2016; van Heesch et al., 2019; Chen et al., 2020; Gaertner et al., 2020; Martinez et al., 2020) using different methods for automatic detection of translated ORFs (Fields et al., 2015; Calviello et al., 2016; Ji, 2018).
For this analysis, we started with small nucleolar RNA host gene 8 (SNHG8), a lncRNA located on human chromosome 4q26. This lncRNA hosts the H/ACA-box small nucleolar RNA (snoRNA), SNORA24. Non-coding genes that host snoRNAs were found to have short, poorly conserved ORFs and were believed to serve little function outside of carrying snoRNAs in their introns (Tycowski et al., 1996; Smith and Steitz, 1998).
Examination of SNHG8 in GWIPS-viz reveals three isoforms ENST00000602414, ENST00000602483, and ENST00000602819 (Figure 4A). The first two ATGs match with high footprint peaks of initiating ribosomes and are outlined in blue. Nucleotide conservation at this locus is poor, and a signature of accelerated evolution is seen on the PhyloP track. RNA-seq data suggests that the long isoform ENST00000602414 is most likely transcribed. The eighth ATG (outlined in orange) also matches a high footprint peak of initiating ribosomes. Nucleotide conservation for this ORF is similarly poor as visualized on the PhyloP track. It should be noted that all three RNA isoforms contain this ORF. However, initiation at the eighth ATG is more likely under the classical scanning model on the shorter isoform ENST00000602483, as it is the second ATG from the 5′ end. We also note another footprint peak of initiating ribosomes that matches with the ATG (sixth ATG site) located on SNORA24 (ENST00000384096); yet, elongating ribosome footprints would not fully encompass the ORF situated at this locus. The PhyloP tracks reveals high nucleotide conservation at SNORA24 indicating its important functional role.
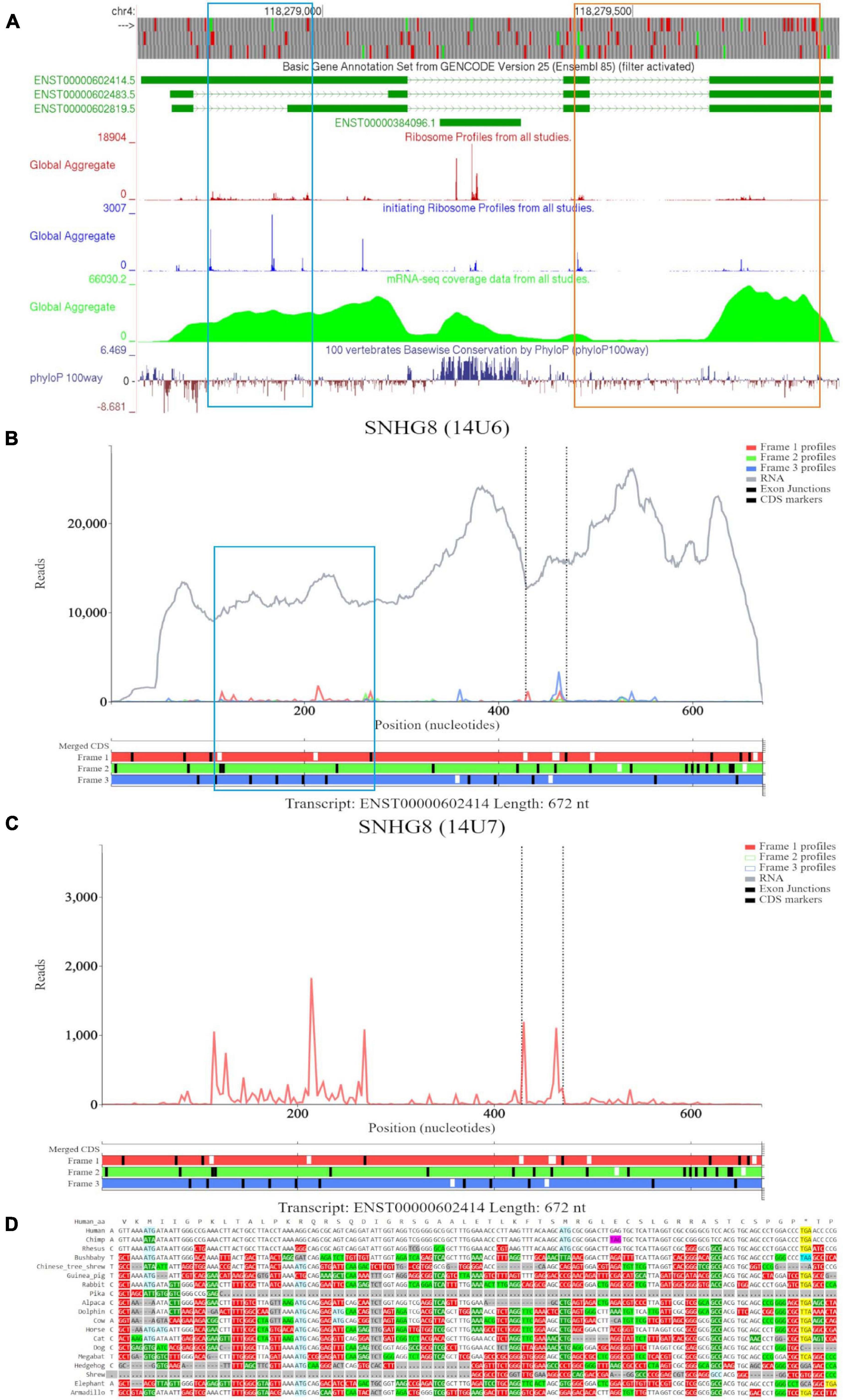
Figure 4. Visualization of SNHG8 in Trips-Viz, GWIPS-Viz and CodAlignView. (A) Visualization of SNHG8 in GWIPS-viz with the tracks described in Setup and Configurations enabled. Two translated ORFs are outlined in blue and orange, respectively. (B) Visualization of SNHG8 isoform ENST00000602414 in Trips-viz. The ORF previously outlined in blue in GWIPS-viz is similarly outlined again. (C) Visualization of transcript ENST00000602414 without RNA-seq data enabled and only reading frame 1 enabled. (D) CodAlignView for the ORF previously outlined in blue showing a high ratio of radical non-synonymous codon substitutions.
Based on the features seen on GWIPS-viz, we first examined transcript ENST00000602414 on Trips-Viz (Figure 4B). Footprints aligned at the first ATG show good triplet periodicity with the reads biased to reading frame one (red). This signal is better visualized in Figure 4C with removal of the footprints supporting other reading frames. The corresponding region is shown in CodAlignView in the reading frame matching the ORF (Figure 4D), the high density of radical codon substitutions is not supportive of protein coding evolution.
For the ORF at the eighth ATG noted on GWIPS-viz, we examined transcript ENST00000602483 in Trips-Viz (Figure 5A). Ribosomal footprints appear aligned to the second ATG and support reading frame three (blue). This region is shown at close zoom on Figure 5B. However, codon substitution pattern (Figure 5C) is not supportive of translation.
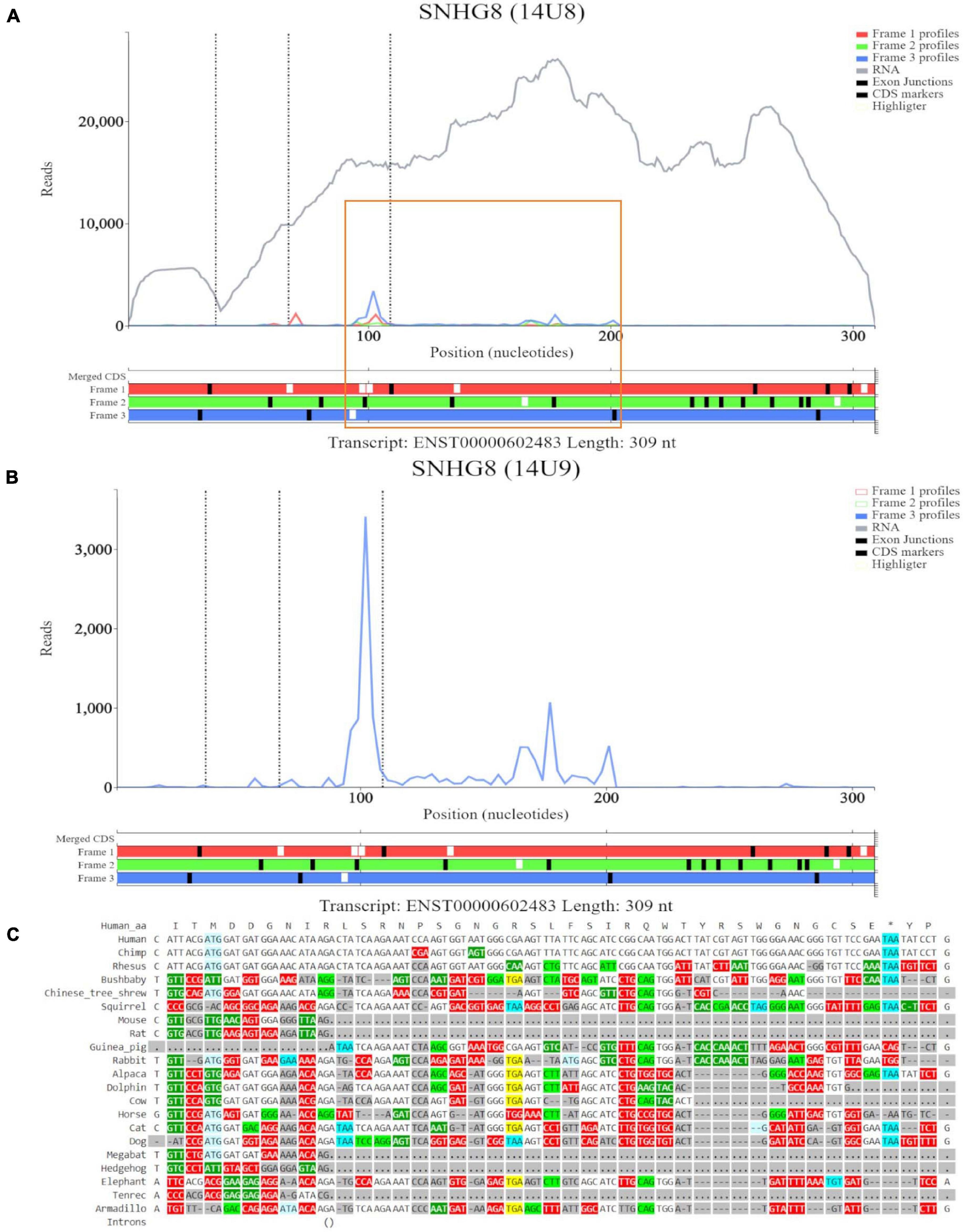
Figure 5. Visualization of SNHG8 in Trips-Viz and CodAlignView. (A) Visualization of SNHG8 isoform ENST00000602483 in Trips-viz. The ORF previously outlined in orange in GWIPS-viz is similarly outlined again. (B) Visualization of transcript ENST00000602483 without RNA-seq data enabled and only reading frame 3 enabled. (C) CodAlignView for the ORF previously outlined in orange showing a high ratio of radical non-synonymous codon substitutions.
For completion, we visualized SNORA24 in Trips-Viz (Supplementary Figure 3A). Although there are footprints aligned to the ATG site that are biased to a single reading frame (blue), they do not encompass the length of the ORF. Small nucleolar RNAs function in ribosome biogenesis and therefore are likely to be isolated as parts of inactive ribosomal complexes. It is also possible that they are protected within other RNA-protein complexes (Ji et al., 2016).
The next RNA examined was zinc finger antisense 1 transcript (ZFAS1), a lncRNA located on human chromosome 20q13.13. It is positioned at the antisense strand of the 5′ end of the protein coding ZNFX1 gene. ZFAS1 also hosts three C/D-box snoRNAs namely SNORD12C, SNORD12B, and SNORD12 in sequential introns (Askarian-Amiri et al., 2011).
In Figure 6A, we observed that there is a lack of RNA-seq data corresponding to the 5′ end of the longer ZFAS1 isoforms (ENST00000450535, ENST00000441722, ENST00000417721, and ENST00000371743). To explore whether this is potentially due to mapping artifacts, we enabled the track “Multi-read mappability with 24mers.” The Umap track represents the probability that a randomly selected read of k-length (24 base pairs is the default) that overlaps a given position in the unconverted genome is uniquely mappable (Karimzadeh et al., 2018). According to the track the mappability is high in this region. We also noted high footprint peaks of initiating ribosomes at the first two exons of the shorter isoforms (ENST00000428008 and ENST00000326677). The 5′ parts of these transcripts are visualized at a closer zoom in Figure 6B. The first high footprint peak of initiating ribosomes occurs on the first exon of the shorter isoforms but does not appear to match any ATG sites. The second peak of initiating ribosomes matches the sixth ATG which is located on SNORD12C (ENST00000386307). There also appears to be high peak of elongating ribosomes at this locus. As expected for a snoRNA SNORD12C sequence is highly conserved as can be seen in the PhyloP track.
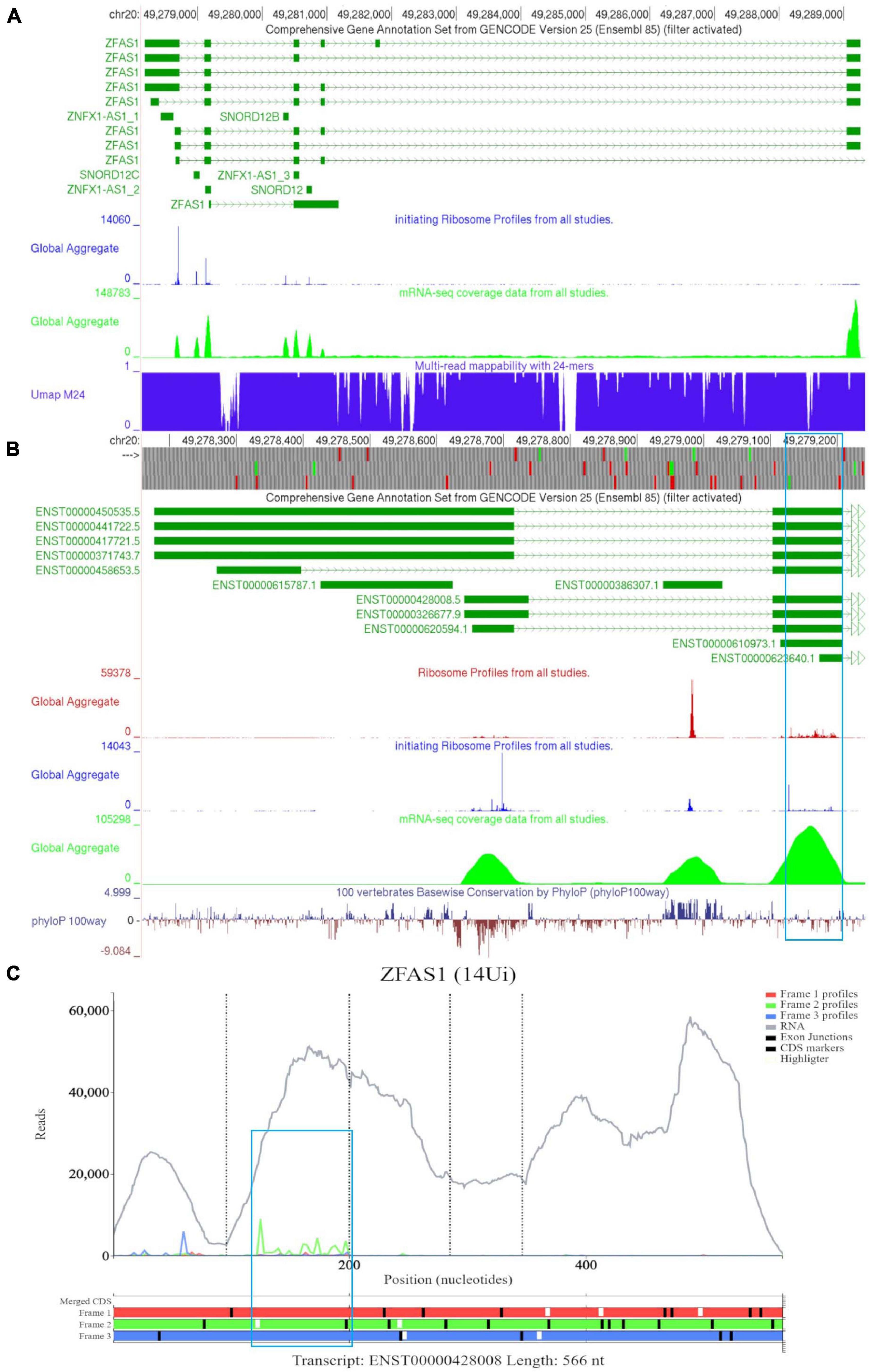
Figure 6. Visualization of ZFAS1 in GWIPS-viz and Trips-Viz. (A) Whole transcript view of ZFAS1 in GWIPS-viz with the ‘Comprehensive Annotation Set from Gencode Version 25,’ ‘initiating Ribosome Profiles from all studies,’ ‘mRNA-seq coverage data from all studies’ and ‘Multi-read mappability with 24mers’ tracks enabled. (B) Visualization of the 5′ end of ZFAS1 in GWIPS-viz with the tracks described in Section Setup and Configurations enabled. An ORF on the second exon of multiple ZFAS1 isoforms is outlined in blue. (C) Visualization of ZFAS1 isoform ENST00000428008 in Trips-viz. The ORF previously outlined in blue in GWIPS-viz is similarly outlined again.
The third high footprint peak of initiating ribosomes matches the eighth ATG site. Multiple transcript isoforms appear to contain this ORF, which is outlined in a blue rectangle (Figure 6B). Poor nucleotide conservation is seen for this sequence on the PhyloP track. RNAseq data support existence of ENST00000428008 and ENST00000326677 transcript isoforms. This ATG is the first ATG from the 5′ end in these transcripts. We elected to examine transcript ENST00000428008 in Trips-Viz (Figure 6C). There is a good support for translation of the ORF that starts with this ATG in the corresponding reading frame (green). The corresponding region is shown at a closer zoom in Figure 7A (RNA-seq data disabled and only reading frame two enabled). However, codon substitution patterns do not support selection typical for protein coding evolution for this ORF (Figure 7B).
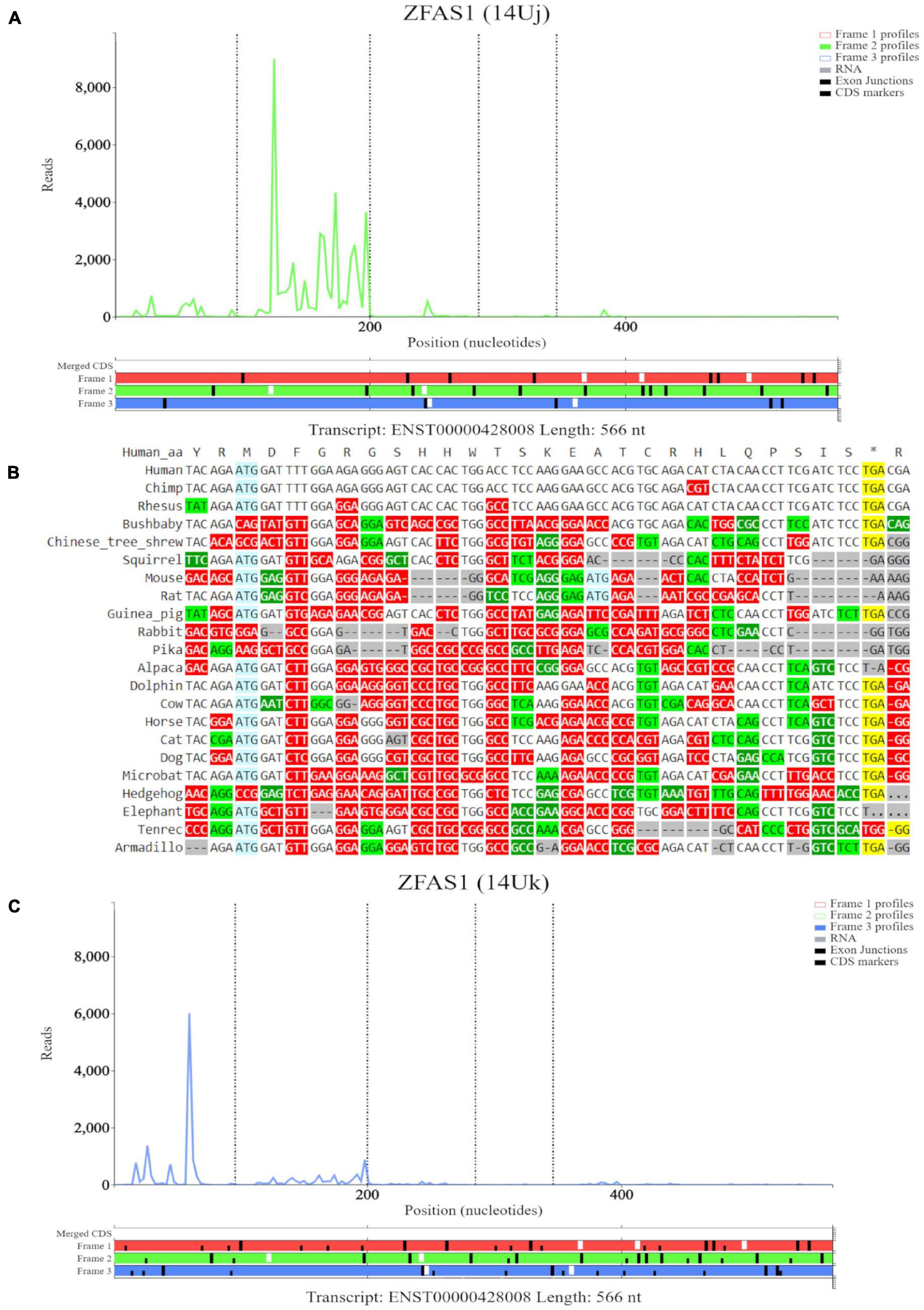
Figure 7. Visualization of ZFAS1 in Trips-Viz and CodAlignView. (A) Visualization of transcript ENST00000428008 without RNA-seq data enabled and only reading frame 2 enabled. (B) CodAlignView for the ORF on ZFAS1 showing a high ratio of radical non-synonymous codon substitutions. (C) Visualization of transcript ENST00000428008 without RNA-seq data enabled and only reading frame 3 enabled. Alternative start sequences of CTG and GTG were enabled and are represented by the black dashes.
Additionally, there were footprints upstream of the first ATG that were biased to reading frame three (blue) on Trips-viz that match the first high footprint peak of initiating ribosomes seen in GWIPS-viz (Figure 6B). However, when looking specifically only at footprints supporting frame three (Figure 7C, short black dashes show positions of near cognate start codons CTG and GTG). It is clear that these footprints are not contained within a single ORF and span the area containing a stop codon in this reading frame. Thus, these protected fragments are unlikely to derive from actively translated ribosomes. We further visualized sequencing reads aligned to SNORD12C using Trips-Viz (Supplementary Figure 3B). The distribution of sequencing reads does not exhibit good triplet periodicity and their positions do not match a particular ORF. Like with other snoRNAs, these fragments are unlikely to be genuine ribosomal footprints. It is more likely that they originate from ribonucleprotein or ribosomal complexes according to snoRNAs role in ribosomal RNA processing (Sloan et al., 2017).
Lastly, we examined X Inactive Specific Transcript (XIST), a nuclear lncRNA with over 19,000 nucleotides (19,296) in humans and located on the q arm of the X chromosome. Previous work proposed that XIST evolved in eutherians from the pseudogenization of a protein coding gene (Duret et al., 2006). Following this, another study suggested XIST had dual origins, namely pseudogenization of a protein coding gene and a set of transposable elements. Specifically, the XIST promoter region and four exons in eutherians retained homology to exons of the protein coding LNX3 gene, while the other six exons were similar to different transposable elements (Elisaphenko et al., 2008). The authors further suggest that the XIST gene lost the coding functions of LNX3 gene, but due to transposon insertions and subsequent partial amplification, formed new functional domains. These new domains are now believed to be necessary for its role in the silencing of X-chromosome genes (Elisaphenko et al., 2008; Romito and Rougeulle, 2011).
Examining XIST on GWIPS-viz revealed a long transcript on the reverse strand (Figure 8A). Dense ribosomal peaks and footprints are noted at the 5′ end of the long isoform ENST00000429829. Available RNA-seq data further supports that the long isoform is transcribed. Zooming in to the area of dense footprints (Figure 8B), showed a high footprint peak of initiating ribosomes that matches the first ATG. The ORF at this locus, outlined in blue, is very short (30nt), the distribution of footprints is consistent with its translation The second ATG also matches a footprint peak of initiating ribosomes. The ORF at this locus is outlined in orange and shows elongating ribosomes mapped to it.
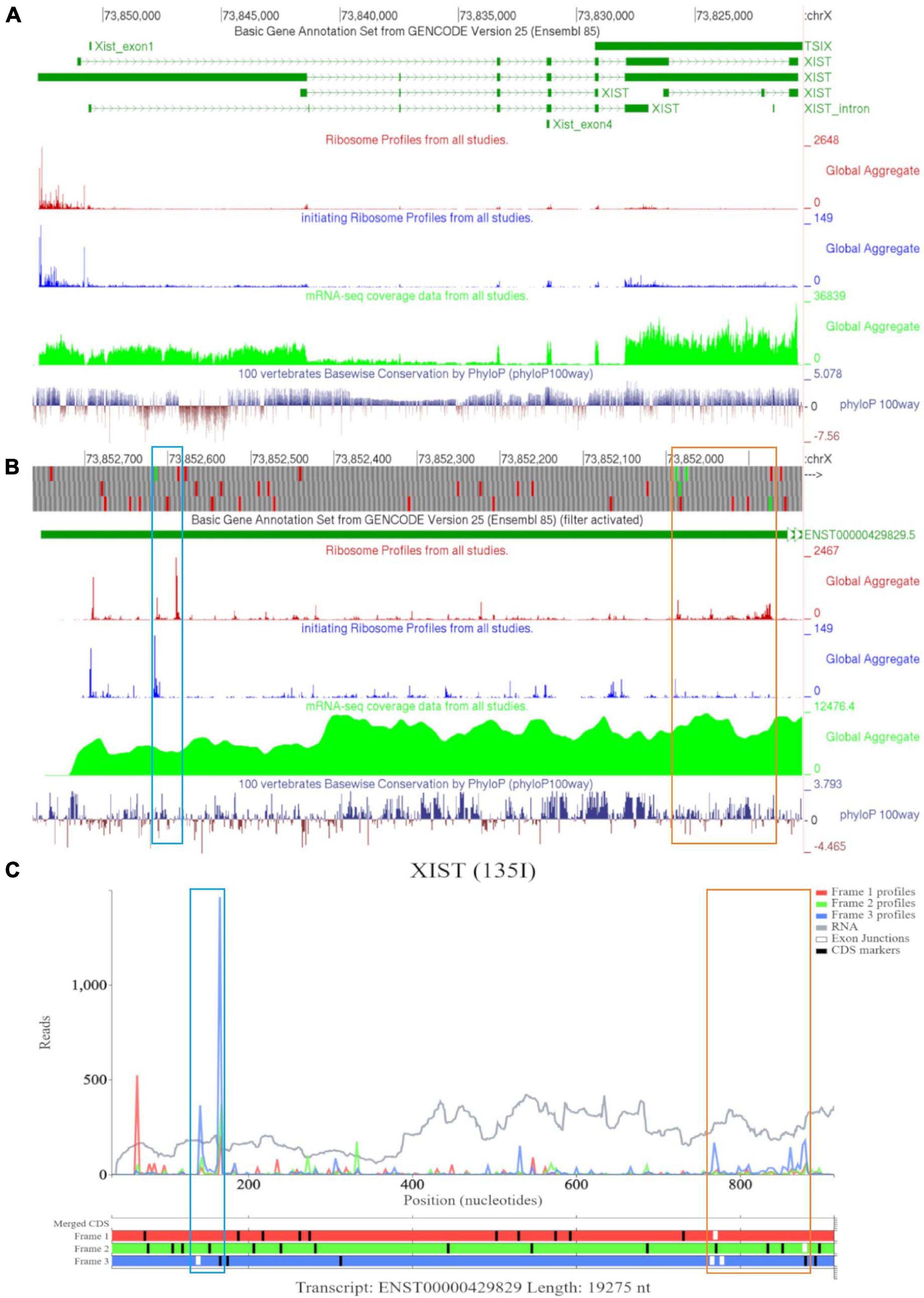
Figure 8. Visualization of XIST in GWIPS-viz and Trips-Viz. (A) Whole transcript view of XIST in GWIPS-viz with the tracks described in Section Setup and Configurations enabled. (B) Visualization of the 5′ end of XIST isoform ENST00000429829 in GWIPS-viz with the tracks described in Section Setup and Configurations enabled. Two translated ORFs are outlined in blue and orange, respectively. (C) Visualization of the 5′ end of transcript ENST00000429829 in Trips-Viz. The two translated ORFs previously outlined in blue and orange on GWIPS-viz are similarly outlined again.
Trips-Viz visualization of the data for this region of transcript ENST00000429829 is shown in Figure 8C. Translation of ORFs initiated at the first and second ATGs is supported with good triplet periodicity matching expected reading frame three (blue) in both cases. Figure 9A shows distribution of footprints that support only these reading frames. We could see an increase of footprint densities in these ORF that exceeds background. However, the codon substitution patterns do not support protein coding evolution for both ORFs (Figures 9B, C, respectively).
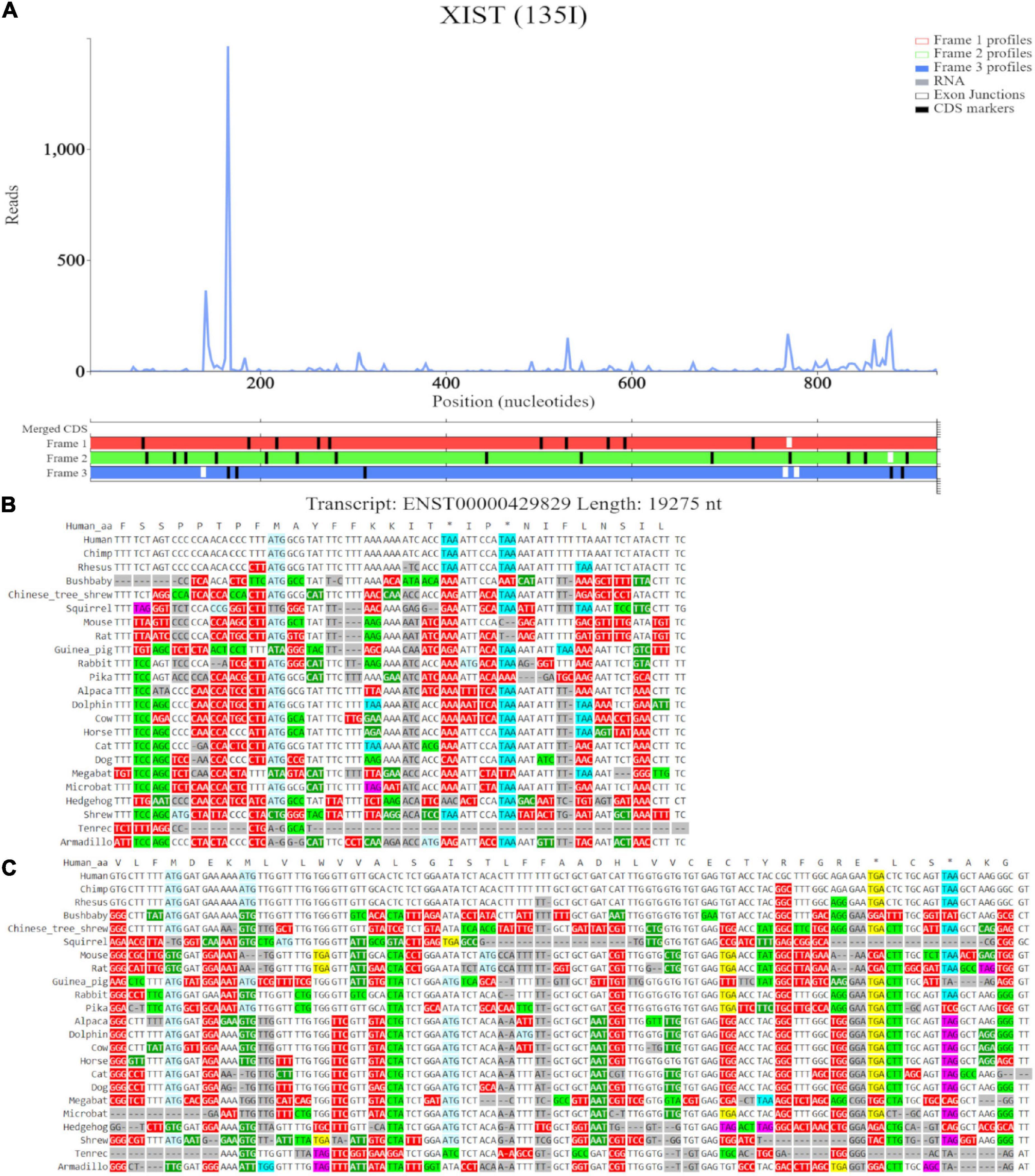
Figure 9. Visualization of XIST in Trips-Viz and CodAlignView. (A) Visualization of transcript ENST00000429829 without RNA-seq data enabled and only reading frame 3 enabled. (B) CodAlignView for the ORF previously outlined in blue showing predominantly absolute codon conservation. (C) CodAlignView for the ORF previously outlined in orange showing mostly absolute codon conservation, there are also more radical non-synonymous codon substitutions than synonymous substitutions.
Final Thoughts
Here we used examples of lncRNAs with reported translated ORFs to guide in the manual examination of publicly available ribosome profiling data using Trips-viz and GWIPS-viz. CodAlignView was then used for detailed examination of codon substitution patterns as evidence for evolutionary selection acting on potential protein coding sequences. We used MTLN as an example of genuine protein coding RNA and illustrated typical features of ribosome profiling data and codon substitution patterns associated with genuine ORF translation and protein coding evolution. Expression of lncRNAs is highly specific (Hon et al., 2017; Douka et al., 2021), therefore a long RNA isoform of MTLN (ENST00000414416) may be expressed in some cells, however, translation of such mRNA is unlikely to produce MTLN proteoforms since its start codon cannot be reached by scanning preinitiation complex.
RNA component of RNase P encoded by RPPH1 was used as a negative example to demonstrate the patterns that are inconsistent with translation and protein evolution. Finally, we examined the data available for other lncRNAs with reported translated ORFs, i.e., SNHG8 and ZFAS1 and XIST and concluded that they contain multiple short ORFs that are likely translated even though they do not exhibit signatures of protein coding evolution. We can only speculate on the biological significance of translation of these short ORFs. We do not know if they code any stable and biologically active peptides, as there is no support for their evolutionary selection. Yet it is possible that they could be used by the immune system as antigens for self-recognition. Additionally the translation of these ORFs may influence processing, stability, localization and structural folding of the corresponding lncRNAs irrespective of biological significance of the products of this translation.
Because of the complexity of translation and of ribosome profiling data, it is very difficult to design automatic tools for translation detections that are highly accurate. Thus, we hope that manual examination of individual cases using the tools described here, will benefit researchers in examining translation status of individual ORFs in non-coding RNAs.
Data Availability Statement
Publicly available datasets were analyzed in this study. This data can be found in Trips-viz which is freely available at https://trips.ucc.ie. Source code for Trips-viz is available at https://github.com/skiniry/Trips-viz. Gwips-viz is freely available at https://gwips.ucc.ie/. CodAlignView is freely available at https://data.broadinstitute.org/compbio1/cav.php.
Author Contributions
OZ: data curation, visualization, and writing. SK: software, data curation, and writing. PB: conceptualization, supervision, writing, project administration, and funding acquisition. KD: supervision, writing, project administration, and funding acquisition. All authors contributed to the article and approved the submitted version.
Funding
This work was supported by: SFI Centre for Research Training in Genomics Data Science 18/CRT/6214 to OZ, Irish Research Council, Government of Ireland Postgraduate Scholarship Programme to SK, and Russian Science Foundation 20-14-00121 to PB.
Conflict of Interest
PB is a founder of Ribomaps Ltd., a company that provides ribosome profiling as a service.
The remaining authors declare that the research was conducted in the absence of any commercial or financial relationships that could be construed as a potential conflict of interest.
Publisher’s Note
All claims expressed in this article are solely those of the authors and do not necessarily represent those of their affiliated organizations, or those of the publisher, the editors and the reviewers. Any product that may be evaluated in this article, or claim that may be made by its manufacturer, is not guaranteed or endorsed by the publisher.
Acknowledgments
We would like to thank all users of Trips-Viz and GWIPS-viz for their feedback and suggestions for improvements. We would also like to thank the SFI Centre for Research Training in Genomics Data Science for their support of Ph.D. candidate OZ. We would also like to thank Paul Young, Darren Fenton and Alla Fedorova for helpful discussions in the writing of this manuscript.
Supplementary Material
The Supplementary Material for this article can be found online at: https://www.frontiersin.org/articles/10.3389/fcell.2021.703374/full#supplementary-material
Footnotes
References
Anderson, D. M., Anderson, K. M., Chang, C. L., Makarewich, C. A., Nelson, B. R., McAnally, J. R., et al. (2015). A micropeptide encoded by a putative long noncoding RNA regulates muscle performance. Cell 160, 595–606. doi: 10.1016/j.cell.2015.01.009
Andreev, D. E., O’Connor, P. B. F., Loughran, G., Dmitriev, S. E., Baranov, P. V., and Shatsky, I. N. (2017). Insights into the mechanisms of eukaryotic translation gained with ribosome profiling. Nucleic Acids Res. 45, 513–526. doi: 10.1093/nar/gkw1190
Askarian-Amiri, M. E., Crawford, J., French, J. D., Smart, C. E., Smith, M. A., Clark, M. B., et al. (2011). SNORD-host RNA Zfas1 is a regulator of mammary development and a potential marker for breast cancer. RNA 17, 878–891. doi: 10.1261/rna.2528811
Baranov, P. V., and Michel, A. M. (2016). Illuminating translation with ribosome profiling spectra. Nat. Methods 13, 123–124. doi: 10.1038/nmeth.3738
Benitez-Cantos, M. S., Yordanova, M. M., O’Connor, P. B. F., Zhdanov, A. V., Kovalchuk, S. I., Papkovsky, D. B., et al. (2020). Translation initiation downstream from annotated start codons in human mRNAs coevolves with the Kozak context. Genome Res. 30, 974–984. doi: 10.1101/gr.257352.119
Birney, E., Stamatoyannopoulos, J. A., Dutta, A., Guigó, R., Gingeras, T. R., Margulies, E. H., et al. (2007). Identification and analysis of functional elements in 1% of the human genome by the ENCODE pilot project. Nature 447, 799–816. doi: 10.1038/nature05874
Brar, G. A., and Weissman, J. S. (2015). Ribosome profiling reveals the what, when, where and how of protein synthesis. Nat. Rev. Mol. Cell Biol. 16, 651–664. doi: 10.1038/nrm4069
Brunet, M. A., Brunelle, M., Lucier, J. F., Delcourt, V., Levesque, M., Grenier, F., et al. (2019). OpenProt: a more comprehensive guide to explore eukaryotic coding potential and proteomes. Nucleic Acids Res. 47, D403–D410. doi: 10.1093/nar/gky936
Calviello, L., Mukherjee, N., Wyler, E., Zauber, H., Hirsekorn, A., Selbach, M., et al. (2016). Detecting actively translated open reading frames in ribosome profiling data. Nat. Methods 13, 165–170. doi: 10.1038/nmeth.3688
Catherman, A. D., Li, M., Tran, J. C., Durbin, K. R., Compton, P. D., Early, B. P., et al. (2013). Top down proteomics of human membrane proteins from enriched mitochondrial fractions. Anal. Chem. 85, 1880–1888. doi: 10.1021/ac3031527
Chen, J., Brunner, A. D., Cogan, J. Z., Nuñez, J. K., Fields, A. P., Adamson, B., et al. (2020). Pervasive functional translation of noncanonical human open reading frames. Science 367, 140–146. doi: 10.1126/science.aav5912
Cheng, J., Kapranov, P., Drenkow, J., Dike, S., Brubaker, S., Patel, S., et al. (2005). Transcriptional maps of 10 human chromosomes at 5-nucleotide resolution. Science 308, 1149–1154. doi: 10.1126/science.1108625
Chew, G. L., Pauli, A., Rinn, J. L., Regev, A., Schier, A. F., and Valen, E. (2013). Ribosome profiling reveals resemblance between long non-coding RNAs and 5′ leaders of coding RNAs. Development 140, 2828–2834. doi: 10.1242/dev.098343
Chugunova, A., Loseva, E., Mazin, P., Mitina, A., Navalayeu, T., Bilan, D., et al. (2019). LINC00116 codes for a mitochondrial peptide linking respiration and lipid metabolism. Proc. Natl. Acad. Sci. U.S.A. 116, 4940–4945. doi: 10.1073/pnas.1809105116
Crappé, J., Ndah, E., Koch, A., Steyaert, S., Gawron, D., De Keulenaer, S., et al. (2015). PROTEOFORMER: deep proteome coverage through ribosome profiling and MS integration. Nucleic Acids Res. 43:e29. doi: 10.1093/nar/gku1283
da Veiga Leprevost, F., Haynes, S. E., Avtonomov, D. M., Chang, H. Y., Shanmugam, A. K., Mellacheruvu, D., et al. (2020). Philosopher: a versatile toolkit for shotgun proteomics data analysis. Nat. Methods 17, 869–870. doi: 10.1038/s41592-020-0912-y
D’Lima, N. G., Ma, J., Winkler, L., Chu, Q., Loh, K. H., Corpuz, E. O., et al. (2017). A human microprotein that interacts with the mRNA decapping complex. Nat. Chem. Biol. 13, 174–180. doi: 10.1038/nchembio.2249
Douka, K., Birds, I., Wang, D., Kosteletos, A., Clayton, S., Byford, A., et al. (2021). Cytoplasmic long non-coding RNAs are differentially regulated and translated during human neuronal differentiation. RNA [Epub ahead of print]. doi: 10.1261/rna.078782.121
Duret, L., Chureau, C., Samain, S., Weissanbach, J., and Avner, P. (2006). The Xist RNA gene evolved in eutherians by pseudogenization of a protein-coding gene. Science 312, 1653–1655. doi: 10.1126/science.1126316
Elisaphenko, E. A., Kolesnikov, N. N., Shevchenko, A. I., Rogozin, I. B., Nesterova, T. B., Brockdorff, N., et al. (2008). A dual origin of the Xist gene from a protein-coding gene and a set of transposable elements. PLoS One 3:e2521. doi: 10.1371/journal.pone.0002521
Erhard, F., Halenius, A., Zimmermann, C., L’Hernault, A., Kowalewski, D. J., Weekes, M. P., et al. (2018). Improved Ribo-seq enables identification of cryptic translation events. Nat. Methods 15, 363–366. doi: 10.1038/nmeth.4631
Evans, D., Marquez, S. M., and Pace, N. R. (2006). RNase P: interface of the RNA and protein worlds. Trends Biochem. Sci. 31, 333–341. doi: 10.1016/j.tibs.2006.04.007
Fang, Y., and Fullwood, M. J. (2016). Roles, functions, and mechanisms of long non-coding RNAs in cancer. Genomics Proteomics Bioinform. 14, 42–54. doi: 10.1016/j.gpb.2015.09.006
Fields, A. P., Rodriguez, E. H., Jovanovic, M., Stern-Ginossar, N., Haas, B. J., Mertins, P., et al. (2015). A regression-based analysis of ribosome-profiling data reveals a conserved complexity to mammalian translation. Mol. Cell 60, 816–827. doi: 10.1016/j.molcel.2015.11.013
Fija-Lkowska, D., Verbruggen, S., Ndah, E., Jonckheere, V., Menschaert, G., and Van Damme, P. (2017). EIF1 modulates the recognition of suboptimal translation initiation sites and steers gene expression via uORFs. Nucleic Acids Res. 45, 7997–8013. doi: 10.1093/nar/gkx469
Friesen, M., Warren, C. R., Yu, H., Toyohara, T., Ding, Q., Florido, M. H. C., et al. (2020). Mitoregulin controls β-oxidation in human and mouse adipocytes. Stem Cell Rep. 14, 590–602. doi: 10.1016/j.stemcr.2020.03.002
Gaertner, B., van Heesch, S., Schneider-Lunitz, V., Schulz, J. F., Witte, F., Blachut, S., et al. (2020). A human esc-based screen identifies a role for the translated lncrna linc00261 in pancreatic endocrine differentiation. eLife 9:58659. doi: 10.7554/ELIFE.58659
Gameiro, P. A., and Struhl, K. (2018). Nutrient deprivation elicits a transcriptional and translational inflammatory response coupled to decreased protein synthesis. Cell Rep. 24, 1415–1424. doi: 10.1016/j.celrep.2018.07.021
Goodarzi, H., Nguyen, H. C. B., Zhang, S., Dill, B. D., Molina, H., and Tavazoie, S. F. (2016). Modulated expression of specific tRNAs drives gene expression and cancer progression. Cell 165, 1416–1427. doi: 10.1016/j.cell.2016.05.046
Großhans, H., and Filipowicz, W. (2008). Molecular biology: the expanding world of small RNAs. Nature 451, 414–416. doi: 10.1038/451414a
Guo, B., Wu, S., Zhu, X., Zhang, L., Deng, J., Li, F., et al. (2020). Micropeptide CIP 2A- BP encoded by LINC 00665 inhibits triple-negative breast cancer progression. EMBO J. 39:e102190. doi: 10.15252/embj.2019102190
Guo, J. U., Agarwal, V., Guo, H., and Bartel, D. P. (2014). Expanded identification and characterization of mammalian circular RNAs. Genome Biol. 15:409. doi: 10.1186/s13059-014-0409-z
Guttman, M., Russell, P., Ingolia, N. T., Weissman, J. S., and Lander, E. S. (2013). Ribosome profiling provides evidence that large noncoding RNAs do not encode proteins. Cell 154, 240–251. doi: 10.1016/j.cell.2013.06.009
Hon, C. C., Ramilowski, J. A., Harshbarger, J., Bertin, N., Rackham, O. J. L., Gough, J., et al. (2017). An atlas of human long non-coding RNAs with accurate 5′ ends. Nature 543, 199–204. doi: 10.1038/nature21374
Ingolia, N. T. (2014). Ribosome profiling: new views of translation, from single codons to genome scale. Nat. Rev. Genet. 15, 205–213. doi: 10.1038/nrg3645
Ingolia, N. T., Brar, G. A., Stern-Ginossar, N., Harris, M. S., Talhouarne, G. J. S., Jackson, S. E., et al. (2014). Ribosome profiling reveals pervasive translation outside of annotated protein-coding genes. Cell Rep. 8, 1365–1379. doi: 10.1016/j.celrep.2014.07.045
Ingolia, N. T., Ghaemmaghami, S., Newman, J. R. S., and Weissman, J. S. (2009). Genome-wide analysis in vivo of translation with nucleotide resolution using ribosome profiling. Science 324, 218–223. doi: 10.1126/science.1168978
Ingolia, N. T., Hussmann, J. A., and Weissman, J. S. (2019). Ribosome profiling: global views of translation. Cold Spring Harb. Perspect. Biol. 11:a032698. doi: 10.1101/cshperspect.a032698
Ingolia, N. T., Lareau, L. F., and Weissman, J. S. (2011). Ribosome profiling of mouse embryonic stem cells reveals the complexity and dynamics of mammalian proteomes. Cell 147, 789–802. doi: 10.1016/j.cell.2011.10.002
Iwasaki, S., Floor, S. N., and Ingolia, N. T. (2016). Rocaglates convert DEAD-box protein eIF4A into a sequence-selective translational repressor. Nature 534, 558–561. doi: 10.1038/nature17978
Ji, Z. (2018). RibORF: identifying genome-wide translated open reading frames using ribosome profiling. Curr. Protoc. Mol. Biol. 124:67. doi: 10.1002/cpmb.67
Ji, Z., Song, R., Huang, H., Regev, A., and Struhl, K. (2016). Transcriptome-scale RNase-footprinting of RNA-protein complexes. Nat. Biotechnol. 34, 410–413. doi: 10.1038/nbt.3441
Ji, Z., Song, R., Regev, A., and Struhl, K. (2015). Many lncRNAs, 5′UTRs, and pseudogenes are translated and some are likely to express functional proteins. eLife 4:e08890. doi: 10.7554/eLife.08890
Jungreis, I., Lin, M., and Kellis, M. (2021). CodAlignView: A Tool For Visualizing Protein-Coding Constraint. Available online at: https://data.broadinstitute.org/compbio1/CodAlignViewUsersGuide.html">https://data.broadinstitute.org/compbio1/CodAlignViewUsersGuide.html (accessed January 14, 2021).
Karimzadeh, M., Ernst, C., Kundaje, A., and Hoffman, M. M. (2018). Umap and Bismap: quantifying genome and methylome mappability. Nucleic Acids Res. 46:e120. doi: 10.1093/nar/gky677
Kiniry, S. J., Judge, C. E., Michel, A. M., and Baranov, P. V. (2021). Trips-Viz: an environment for the analysis of public and user-generated ribosome profiling data. Nucleic Acids Res. 49, W662–W670. doi: 10.1093/nar/gkab323
Kiniry, S. J., Michel, A. M., and Baranov, P. V. (2020). Computational methods for ribosome profiling data analysis. Wiley Interdiscip. Rev. RNA 11:1577. doi: 10.1002/wrna.1577
Kiniry, S. J., O’Connor, P. B. F., Michel, A. M., and Baranov, P. V. (2019). Trips-Viz: a transcriptome browser for exploring Ribo-Seq data. Nucleic Acids Res. 47, D847–D852. doi: 10.1093/nar/gky842
Kong, A. T., Leprevost, F. V., Avtonomov, D. M., Mellacheruvu, D., and Nesvizhskii, A. I. (2017). MSFragger: ultrafast and comprehensive peptide identification in mass spectrometry-based proteomics. Nat. Methods 14, 513–520. doi: 10.1038/nmeth.4256
Lee, S., Liu, B., Lee, S., Huang, S. X., Shen, B., and Qian, S. B. (2012). Global mapping of translation initiation sites in mammalian cells at single-nucleotide resolution. Proc. Natl. Acad. Sci. U.S.A. 109, E2424–E2432. doi: 10.1073/pnas.1207846109
Lin, M. F., Jungreis, I., and Kellis, M. (2011). PhyloCSF: a comparative genomics method to distinguish protein coding and non-coding regions. Bioinformatics 27, i275–i282. doi: 10.1093/bioinformatics/btr209
Lin, Y. F., Xiao, M. H., Chen, H. X., Meng, Y., Zhao, N., Yang, L., et al. (2019). A novel mitochondrial micropeptide MPM enhances mitochondrial respiratory activity and promotes myogenic differentiation. Cell Death Dis. 10, 1–11. doi: 10.1038/s41419-019-1767-y
Loughran, G., Chou, M. Y., Ivanov, I. P., Jungreis, I., Kellis, M., Kiran, A. M., et al. (2014). Evidence of efficient stop codon readthrough in four mammalian genes. Nucleic Acids Res. 42, 8928–8938. doi: 10.1093/nar/gku608
Martinez, T. F., Chu, Q., Donaldson, C., Tan, D., Shokhirev, M. N., and Saghatelian, A. (2020). Accurate annotation of human protein-coding small open reading frames. Nat. Chem. Biol. 16, 458–468. doi: 10.1038/s41589-019-0425-0
Michel, A. M., Andreev, D. E., and Baranov, P. V. (2014a). Computational approach for calculating the probability of eukaryotic translation initiation from ribo-seq data that takes into account leaky scanning. BMC Bioinformatics 15:380. doi: 10.1186/s12859-014-0380-4
Michel, A. M., Choudhury, K. R., Firth, A. E., Ingolia, N. T., Atkins, J. F., and Baranov, P. V. (2012). Observation of dually decoded regions of the human genome using ribosome profiling data. Genome Res. 22, 2219–2229. doi: 10.1101/gr.133249.111
Michel, A. M., Fox, G., Kiran, M., De Bo, C., O’Connor, P. B. F., Heaphy, S. M., et al. (2014b). GWIPS-viz: development of a ribo-seq genome browser. Nucleic Acids Res. 42, D859–D864. doi: 10.1093/nar/gkt1035
Munzarová, V., Pánek, J., Gunišová, S., Dányi, I., Szamecz, B., and Valášek, L. S. (2011). Translation reinitiation relies on the interaction between eIFa/TIF32 and progressively folded cis-acting mRNA elements preceding short uORFS. PLoS Genet. 7:e1002137. doi: 10.1371/journal.pgen.1002137
Navarro Gonzalez, J., Zweig, A. S., Speir, M. L., Schmelter, D., Rosenbloom, K. R., Raney, B. J., et al. (2021). The UCSC genome browser database: 2021 update. Nucleic Acids Res. 49, D1046–D1057. doi: 10.1093/nar/gkaa1070
O’Connor, P. B. F., Andreev, D. E., and Baranov, P. V. (2016). Comparative survey of the relative impact of mRNA features on local ribosome profiling read density. Nat. Commun. 7:12915. doi: 10.1038/ncomms12915
Pang, Y., Liu, Z., Han, H., Wang, B., Li, W., Mao, C., et al. (2020). Peptide SMIM30 promotes HCC development by inducing SRC/YES1 membrane anchoring and MAPK pathway activation. J. Hepatol. 73, 1155–1169. doi: 10.1016/j.jhep.2020.05.028
Park, J. E., Yi, H., Kim, Y., Chang, H., and Kim, V. N. (2016). Regulation of Poly(A) tail and translation during the somatic cell cycle. Mol. Cell 62, 462–471. doi: 10.1016/j.molcel.2016.04.007
Pollard, K. S., Hubisz, M. J., Rosenbloom, K. R., and Siepel, A. (2010). Detection of nonneutral substitution rates on mammalian phylogenies. Genome Res. 20, 110–121. doi: 10.1101/gr.097857.109
Raj, A., Wang, S. H., Shim, H., Harpak, A., Li, Y. I., Engelmann, B., et al. (2016). Thousands of novel translated open reading frames in humans inferred by ribosome footprint profiling. eLife 5:e13328. doi: 10.7554/eLife.13328
Reuter, K., Biehl, A., Koch, L., and Helms, V. (2016). PreTIS: a tool to predict non-canonical 5′ UTR translational initiation sites in human and mouse. PLoS Comput. Biol. 12:e1005170. doi: 10.1371/journal.pcbi.1005170
Romito, A., and Rougeulle, C. (2011). Origin and evolution of the long non-coding genes in the X-inactivation center. Biochimie 93, 1935–1942. doi: 10.1016/j.biochi.2011.07.009
Rosenbloom, K. R., Armstrong, J., Barber, G. P., Casper, J., Clawson, H., Diekhans, M., et al. (2015). The UCSC Genome Browser database: 2015 update. Nucleic Acids Res. 43, D670–D681. doi: 10.1093/nar/gku1177
Ruiz-Orera, J., and Albà, M. M. (2019). Conserved regions in long non-coding RNAs contain abundant translation and protein–RNA interaction signatures. NAR Gen. Bioinforma 1:e2. doi: 10.1093/nargab/lqz002
Schramm, L., and Hernandez, N. (2002). Recruitment of RNA polymerase III to its target promoters. Genes Dev. 16, 2593–2620. doi: 10.1101/gad.1018902
Shahrouki, P., and Larsson, E. (2012). The non-coding oncogene: a case of missing DNA evidence? Front. Genet. 3:170. doi: 10.3389/fgene.2012.00170
Sloan, K. E., Warda, A. S., Sharma, S., Entian, K. D., Lafontaine, D. L. J., and Bohnsack, M. T. (2017). Tuning the ribosome: the influence of rRNA modification on eukaryotic ribosome biogenesis and function. RNA Biol. 14, 1138–1152. doi: 10.1080/15476286.2016.1259781
Smith, C. M., and Steitz, J. A. (1998). Classification of gas5 as a multi-small-nucleolar-RNA (snoRNA) host gene and a member of the 5′-terminal oligopyrimidine gene family reveals common features of snoRNA host genes. Mol. Cell. Biol. 18, 6897–6909. doi: 10.1128/mcb.18.12.6897
Stein, C. S., Jadiya, P., Zhang, X., McLendon, J. M., Abouassaly, G. M., Witmer, N. H., et al. (2018). Mitoregulin: a lncRNA-encoded microprotein that supports mitochondrial supercomplexes and respiratory efficiency. Cell Rep. 23, 3710.e8–3720.e8. doi: 10.1016/j.celrep.2018.06.002
Storz, G. (2002). An expanding universe of noncoding RNAs. Science 296, 1260–1263. doi: 10.1126/science.1072249
Sun, Y. H., Zhu, J., Xie, L. H., Li, Z., Meduri, R., Zhu, X., et al. (2020). Ribosomes guide pachytene piRNA formation on long intergenic piRNA precursors. Nat. Cell Biol. 22, 200–212. doi: 10.1038/s41556-019-0457-4
Tycowski, K. T., Di Shu, M., and Steitz, J. A. (1996). A mammalian gene with introns instead of exons generating stable RNA products. Nature 379, 464–466. doi: 10.1038/379464a0
van Bakel, H., Nislow, C., Blencowe, B. J., and Hughes, T. R. (2010). Most “dark matter” transcripts are associated with known genes. PLoS Biol. 8:e1000371. doi: 10.1371/journal.pbio.1000371
van Heesch, S., Witte, F., Schneider-Lunitz, V., Schulz, J. F., Adami, E., Faber, A. B., et al. (2019). The translational landscape of the human heart. Cell 178, 242.e29–260.e29. doi: 10.1016/j.cell.2019.05.010
Washietl, S., Pedersen, J. S., Korbel, J. O., Stocsits, C., Gruber, A. R., Hackermüller, J., et al. (2007). Structured RNAs in the ENCODE selected regions of the human genome. Genome Res. 17, 852–864. doi: 10.1101/gr.5650707
Weinberg, D. E., Shah, P., Eichhorn, S. W., Hussmann, J. A., Plotkin, J. B., and Bartel, D. P. (2016). Improved ribosome-footprint and mRNA measurements provide insights into dynamics and regulation of yeast translation. Cell Rep. 14, 1787–1799. doi: 10.1016/j.celrep.2016.01.043
Werner, A., Iwasaki, S., McGourty, C. A., Medina-Ruiz, S., Teerikorpi, N., Fedrigo, I., et al. (2015). Cell-fate determination by ubiquitin-dependent regulation of translation. Nature 525, 523–527. doi: 10.1038/nature14978
Wolfe, A. L., Singh, K., Zhong, Y., Drewe, P., Rajasekhar, V. K., Sanghvi, V. R., et al. (2014). RNA G-quadruplexes cause eIF4A-dependent oncogene translation in cancer. Nature 513, 65–70. doi: 10.1038/nature13485
Xiao, Z., Huang, R., Xing, X., Chen, Y., Deng, H., and Yang, X. (2018). De novo annotation and characterization of the translatome with ribosome profiling data. Nucleic Acids Res. 46:e61. doi: 10.1093/nar/gky179
Xu, B., Gogol, M., Gaudenz, K., and Gerton, J. L. (2016). Improved transcription and translation with L-leucine stimulation of mTORC1 in Roberts syndrome. BMC Genomics 17:25. doi: 10.1186/s12864-015-2354-y
Keywords: translation, ribosome profiling, Ribo-seq, small open reading frames (smORFs), non-coding RNAs, LncRNA - long noncoding RNA, microprotein, RNA-Seq
Citation: Zaheed O, Kiniry SJ, Baranov PV and Dean K (2021) Exploring Evidence of Non-coding RNA Translation With Trips-Viz and GWIPS-Viz Browsers. Front. Cell Dev. Biol. 9:703374. doi: 10.3389/fcell.2021.703374
Received: 30 April 2021; Accepted: 12 July 2021;
Published: 12 August 2021.
Edited by:
Wanting Liu, Jinan University, ChinaReviewed by:
Jorge Ruiz, Helmholtz Association of German Research Centers (HZ), GermanyZhe Ji, Northwestern University, United States
Copyright © 2021 Zaheed, Kiniry, Baranov and Dean. This is an open-access article distributed under the terms of the Creative Commons Attribution License (CC BY). The use, distribution or reproduction in other forums is permitted, provided the original author(s) and the copyright owner(s) are credited and that the original publication in this journal is cited, in accordance with accepted academic practice. No use, distribution or reproduction is permitted which does not comply with these terms.
*Correspondence: Pavel V. Baranov, p.baranov@ucc.ie; Kellie Dean, k.dean@ucc.ie