- 1Department of Anesthesiology, The Second Affiliated Hospital of Nanchang University, Nanchang, China
- 2Department of Surgical Oncology, Zhejiang University Cancer Center, Hangzhou, China
- 3Queen Mary College, Nanchang University, Nanchang, China
Previous studies have revealed the relationship between toll-like receptor 4 (TLR4) polymorphisms and cancer susceptibility. However, the relationship between TLR4 and prognosis and immune cell infiltration in pan-cancer patients is still unclear. Through the Genotype-Tissue Expression (GTEx) and The Cancer Genome Atlas (TCGA) databases, the distinct expression of the TLR4 gene in 24 tumors and normal tissues was analyzed. Univariate Cox proportional hazards regression analysis was used to identify the cancer types whose TLR4 gene expression was related to prognosis. The relationship between TLR4 and tumor cell immune invasion was studied. Spearman’s rank correlation coefficient was used to analyze the relationship among TLR4 and immune neoantigens, tumor mutation burden (TMB), microsatellite instability (MSI), DNA repair genes, and DNA methylation. Gene Set Enrichment Analysis (GSEA) was used to identify the tumor-related pathways that the TLR4 gene was highly expressed in; the expression of the TLR4 gene was verified with the Human Protein Atlas (HPA) database. Low expression of TLR4 was associated with an inferior prognosis in kidney renal clear cell carcinoma (KIRC), skin cutaneous melanoma (SKCM), and uterine corpus endometrial carcinoma (UCEC), while high expression was related to a poor prognosis in head and neck squamous cell carcinoma (HNSC), prostate adenocarcinoma (PRAD), stomach adenocarcinoma (STAD), and testicular germ cell tumor (TGCT). The expression of TLR4 was negatively correlated with the expression of B cells in STAD. The expression of TLR4 was positively correlated with the infiltration of B cells, CD4 and CD8 T cells, neutrophils, macrophages, and dendritic cells in STAD, KIRC, UCEC, TGCT, and SKCM. The expression of the TLR4 gene in KIRC, SKCM, STAD, TGCT, and UCEC was highly correlated with inducible T-cell costimulator (ICOS), cytotoxic T lymphocyte-associated molecule 4 (CTLA4), and CD28 immune checkpoints. Spearman’s rank correlation coefficient showed that the expression of TLR4 gene was significantly correlated with TMB in STAD and UCEC and was prominently correlated with MSI in TGCT, STAD, and SKCM. The expression of the TLR4 gene was highly correlated with MLH1, MSH2, and MSH6 in KIRC, SKCM, and STAD. The expression of the TLR4 gene was remarkably correlated with the methyltransferases DNA methyltransferase 2 (DNMT2) and DNA methyltransferase 3-beta (DNMT3B) in SKCM and STAD. Enrichment analysis showed that TLR4 was highly expressed in the chemokine signaling pathway and the cell adhesion molecule and cytokine receptor interaction pathway. In summary, the expression of TLR4 is linked to the prognosis of KIRC, SKCM, STAD, TGCT, and UCEC patients and the level of immune infiltration of CD4, CD8 T cells, macrophages, neutrophils, and dendritic cells.
Introduction
Cancer is the second leading cause of death in the world. The rising cancer incidence and death rate and their impact on public health have attracted wide notice, and a great deal of research has been done on the occurrence, development, and metastasis mechanisms of cancer (Torre et al., 2015). Although targeted therapies and extensive treatments for certain cancers have made great progress, a large number of cancer patients still have a poor prognosis. Therefore, there is an urgent need for a useful biomarker that can forecast the prognosis of cancer. Chronic inflammation is closely linked to tumors (Balkwill et al., 2005). It has been discovered that under chronic inflammatory conditions, various mechanisms can promote the occurrence and development of cancer, including activating angiogenesis, inhibiting apoptosis, stimulating cell proliferation, and destroying antitumor immune responses (Baniyash, 2006; Mantovani et al., 2008), and inducing epigenetic changes. It is closely related to the development of cancer.
Previous studies have shown that inflammation-induced Toll-like receptors (TLRs) play a critical role in tumorigenesis and development (El-Omar et al., 2008; Grimm et al., 2010). TLRs play a vital role in innate immunity and activate and mediate the inflammatory response by identifying invading pathogens. Since TLR plays a pivotal role in innate immunity and chronic inflammation is identified as one of the important events in the carcinogenic process, the expression profile of TLR genes may also be a useful marker of early cancer susceptibility, development, and progression. To date, 11 TLR genes (TLR1–11) have been discovered in humans (Takeda and Akira, 2015). Toll-like receptor 4 (TLR4) is mainly expressed on immune cells, namely, T cells, natural killer cells, dendritic cells, macrophages, and neutrophils (McGettrick and O’Neill, 2007). A previous study also showed that TLR4 was expressed in the tumor microenvironment, especially in cancer cells (Cammarota et al., 2010; Li et al., 2017). In view of the limited reports of TLR4 expression in different cancer types, it is challenging to reach any conclusion at present. Comprehensive analysis of TLR4 expression is necessary; moreover, exploring effective prognostic biomarkers can help optimize the prognostic evaluation system of cancer.
Here, we used specific data to show that the TLR4 gene is a biomarker for a poor prognosis of kidney renal clear cell carcinoma (KIRC), skin cutaneous melanoma (SKCM), stomach adenocarcinoma (STAD), testicular germ cell tumors (TGCTs), and uterine corpus endometrial carcinoma (UCEC) and to explore the potential signaling pathways that the TLR4 gene plays in tumorigenesis and development. Compared with the existing TLR4 gene-related experiments, our work comprehensively and systematically studied the expression of the TLR4 gene in pan-cancer and screened out cancer types with a poor prognosis, which will provide reference data for follow-up studies.
Materials and Methods
Data Acquisition
First, we used the Genotype-Tissue Expression (GTEx) data set [https://commonfund.nih.gov/GTEx/. This database collects data from normal human tissues for sequencing and can be used to study the differential gene expression between different tissues and between normal and diseased tissues. In addition, since The Cancer Genome Atlas (TCGA) mainly collects data from cancer tissues, it can be used in conjunction with TCGA database to ensure more reliable results]. We analyzed the expression level of the TLR4 gene in 21 normal tissues, in accordance with the tissue source. Second, the data of tumor cell lines were downloaded from the Cancer Cell Line Encyclopedia (CCLE) database (https://portals.broadinstitute.org/ccle, a database of cancer cell lines that currently contains more than 1,000 cell lines), and we analyzed the expression of the TLR4 gene in each tumor cell line. Then, data were downloaded from TCGA database (https://cancergenome.nih.gov/. TCGA mainly contains data on cancer tissues, including data on 33 types of tumors. The data are comprehensive, covering miRNA, methylation, mutation, and other data). Threshold values were determined according to the following values to analyze the expression differences of the TLR4 gene in 33 kinds of tumors: ∗p < 0.05, ∗∗p < 0.01, and ∗∗∗p < 0.001. Because of the lack of normal tissue data in TCGA database, we compared the normal tissue data from the GTEx database with tumor data from TCGA database to analyze the differential expression of the TLR4 gene in 27 types of tumors and adjacent normal tissues.
TCGA Database Analysis
The prognostic difference of the TLR4 gene expression group in 33 kinds of tumors was analyzed with the dichotomy method. To explore the types of cancer that are significantly associated with TLR4 gene expression, we used univariate regression analysis to analyze the prognosis of 33 kinds of tumors, including process-free intervention (PFI), overall survival (OS) rate, disease-specific survival (DSS), and disease-free intervention (DFI). A survival curve was drawn, and the selected cancer types were used for further study and analysis.
TIMER Database Analysis
We analyzed the correlation between TLR4 gene expression and six kinds of immune cell scores in 33 kinds of cancer: six kinds of infiltrating immune cells (B cells, CD4+ T cells, CD8+ T cells, neutrophils, macrophages, and dendritic cells) through the tumor immune assessment resource (TIMER) algorithm database1. RNA-Seq expression profile data were used to detect the infiltration of immune cells into tumor tissues and provide the infiltration of six types of immune cells (B cells, CD4+ T cells, CD8+ T cells, neutrophils, macrophages, and dendritic cells).
Relationship Between Toll-Like Receptor 4 Gene Expression and Immunity
To study the relationship between TLR4 gene expression in KIRC, SKCM, STAD, TGCT, and UCEC and immune checkpoints, we extracted more than 40 immune checkpoints and used Spearman’s rank correlation coefficient to calculate and analyze the correlation between the two (∗indicates a mild correlation, p < 0.05; ∗∗indicates a moderate correlation, p < 0.01; ∗∗∗indicates a high correlation, p < 0.001).
In addition, Spearman’s rank correlation coefficient was applied to analyze the relationship of TLR4 gene expression and immune neoantigens, tumor mutation burden (TMB), microsatellite instability (MSI), DNA repair genes and DNA methylation, and Gene Set Enrichment Analysis (GSEA) (http://software.broadinstitute.org/gsea/msigdb/index.jsp, an enrichment method proposed by the Broad Institute that categorizes human genes in terms of location, function, etc., to construct many gene sets for scientific research) of the TLR4 gene in tumors with high and low expression. The Kyoto Encyclopedia of Genes and Genomes (KEGG) database (https://www.kegg.jp. It is a database that integrates genome, chemistry, and system function information and aims to reveal the genetic and chemical blueprints of life phenomena. It can use graphics to show numerous metabolic pathways and the relationships among each pathway) was used to analyze the enrichment of TLR4 gene expression in the signaling pathways. Finally, to further compare the expression of the TLR4 gene in the cancer tissues of 14 tumors and the corresponding normal tissues, the Human Protein Atlas (HPA) database2 was used. Transcriptomics and proteomics were used as techniques to study protein expression in different human tissues or organs at the RNA and protein levels and to explore the expression of protein-coding genes in normal and tumor tissues or organs. This database was used to verify the expression of the TLR4 gene in various tumors.
Immunohistochemical Staining
To further compare the expression of the TLR4 gene in the cancer tissues of 14 tumors and the corresponding normal tissues, the HPA database was used to verify the expression of the TLR4 gene in various tumors. To further verify the reliability of the above results, we performed immunohistochemical (IHC) staining. Samples from patients with seven kinds of cancer who were being treated in our hospital were collected [liver hepatocellular carcinoma (LIHC), colon adenocarcinoma (COAD), ovarian serous cystadenocarcinoma (OV), prostate adenocarcinoma (PRAD), breast invasive carcinoma (BRCA), pancreatic adenocarcinoma (PAAD), and UCEC]; three pairs of samples (cancer and normal tissues) were collected for each type of cancer. The samples were embedded in paraffin and incubated with anti-TLR4 antibody (1:100, sc-293072, Santa Cruz Biotechnology) at 4°C overnight. Then, a secondary antibody coupled to horseradish peroxidase (HRP) (1:500, ab150113; Abcam) was incubated with the sections for 60 min at room temperature, followed by 3,3’-diaminobenzidine (DAB substrate system; Dako) and hematoxylin staining. Images were taken with an Olympus cx-21 (Japan) magnified ×200. Using the analysis software Image-Pro Plus 6.0 (Media Cybernetics, Inc., Rockville, MD, United States), the same intensity of brown color was selected as the unified standard to judge the positivity of all photos, and each photo was analyzed to obtain the positive cumulative optical density (IOD) and tissue pixel area (AREA). The average optical density IOD/AREA (mean density) was calculated. SPSS 19.0 software was used for independent-sample t-tests of the average optical density values obtained from the cancer and normal tissues. p < 0.05 was defined as statistically significant.
Statistical Analysis
Single-factor regression analysis was used to detect the expression and prognosis of the TLR4 gene in different tumors and tissues. Spearman’s correlation coefficients were used to assess the correlation between TLR4 gene expression and immune infiltration level, immune checkpoint, TMB, MSI, DNA repair genes, and DNA methylation in different types of cancer. A p-value < 0.05 was regarded as statistically significant.
Results
The Expression Levels of Toll-Like Receptor 4 Gene
The analysis of the GTEx data set showed that the TLR4 gene was basically not expressed in bone marrow or 31 other tissues and was most highly expressed in the spleen (Figure 1A). The CCLE database (n = 1,019) analysis showed that among 21 tumor cell lines, TLR4 gene expression was lowest in salivary glands and highest in the central nervous system (Figure 1B). Furthermore, because there are few normal tissue data in TCGA database (Figure 1C), we then compared the normal tissue data in the GTEx database (n = 6,678) to the tumor data in TCGA database (n = 9,498) (Figure 1D). Compared with normal tissues, TLR4 gene is low expression in adrenocortical carcinoma (ACC), bladder urothelial carcinoma (BLCA), BRCA, cervical squamous cell carcinoma and endocervical adenocarcinoma (CESC), cholangiocarcinoma (CHOL), head and neck squamous cell carcinoma (HNSC), kidney renal papillary cell carcinoma (KIRP), lung adenocarcinoma (LUAD), rectal adenocarcinoma (READ), thyroid cancer (THCA), UCEC, and uterine carcinoma (UCS). In some data sets, COAD, esophageal carcinoma (ESCA), glioblastoma multiforme (GBM), kidney chromophobe (KICH), brain lower grade glioma (LGG), LIHC, PAAD, SKCM, STAD, and TGCT have high TLR4 gene expression.
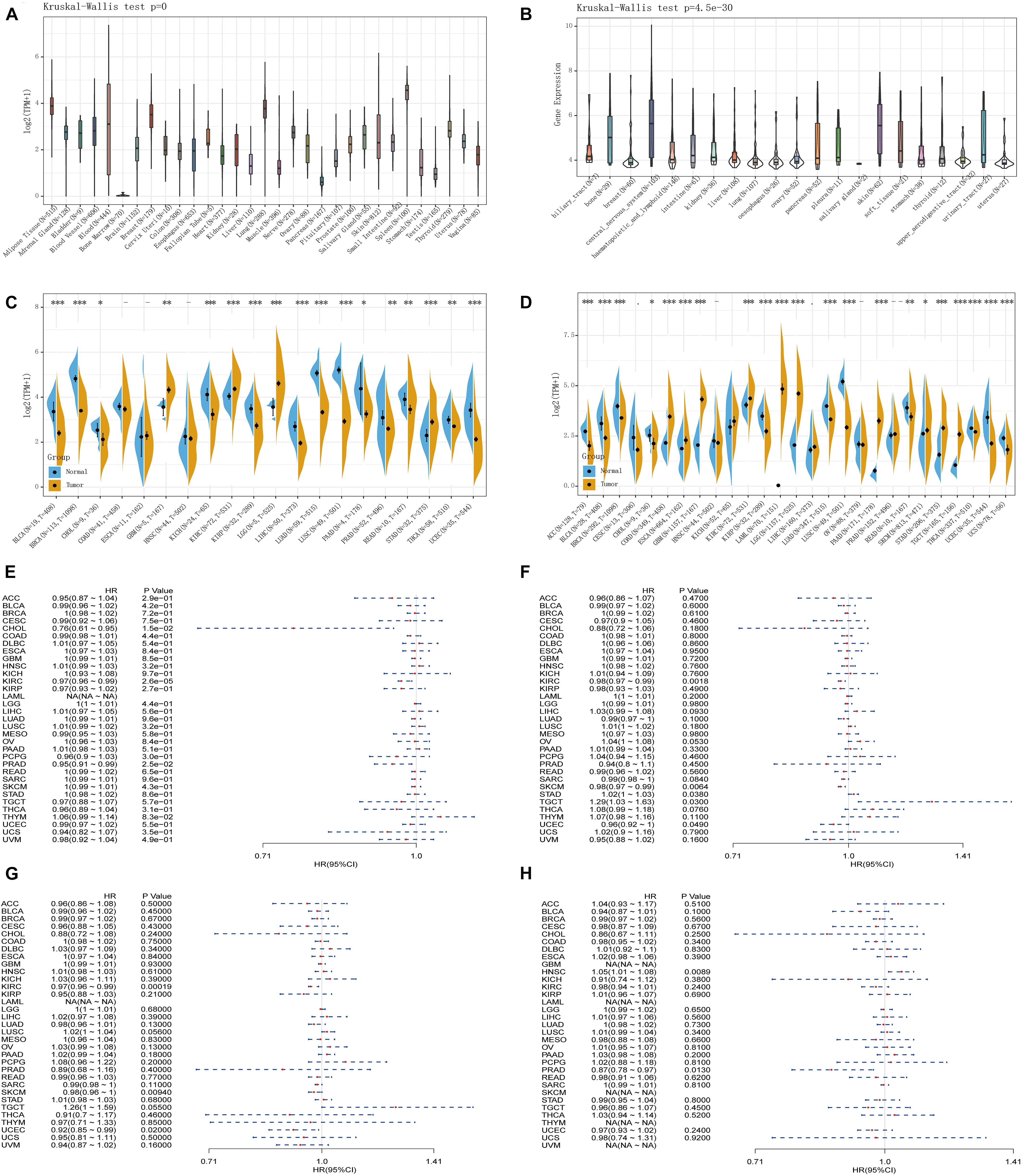
Figure 1. Comprehensive analysis of the expression of Toll-like receptor 4 (TLR4) gene in various databases. The Genotype-Tissue Expression (GTEx) data set showed that the TLR4 gene was basically not expressed in bone marrow or 31 other tissues and was most highly expressed in the spleen (A). The CCLE database (n = 1,019) showed that the TLR4 gene expression was lowest in salivary glands and highest in the central nervous system (B). There are few normal tissue data in The Cancer Genome Atlas (TCGA) database (C). The GTEx database (n = 6,678) and the tumor data in TCGA database (n = 9,498) are compared and displayed (D). Compared with normal tissues, TLR4 gene expression is low in ACC, BLCA, BRCA, CESC, CHOL, HNSC, KIRP, LUAD, READ, HCA, UCEC, and UCS. In some data sets, COAD, ESCA, GBM, KICH, LGG, LIHC, PAAD, SKCM, STAD, and TGCT have high expressions. PFI showed that TLR4 was notably related to the prognosis of CHOL (p = 0.015), KIRC (p = 2.6e-5), and PRAD (p = 0.025) (E). OS displayed that TLR4 was markedly correlated with the prognosis of KIRC (p = 0.0018), SKCM (p = 0.0064), STAD (p = 0.038), TGCT (p = 0.03), and UCEC (p = 0.049) (F). DSS reflected that TLR4 was observably correlated with the prognosis of KIRC (p = 0.00019), SKCM (p = 0.0094), and UCEC (p = 0.02) (G). The prognosis of DFI showed that TLR4 was dramatically correlated with the prognosis of HNSC (p = 0.0089) and PRAD (p = 0.013) (H). A p-value <0.05 was considered statistically significant. The symbols “∗,” “∗∗,” and “∗∗∗” refer to p-values <0.05, <0.01, and <0.001, respectively.
The Prognosis and Toll-Like Receptor 4 Gene Expression in 33 Tumors
To determine the correlation of TLR4 gene expression with the patient prognosis in 33 tumors, we used gene expression profile data and single-factor regression analysis to draw forest plots (p < 0.05). PFI showed that TLR4 was notably related to the prognosis of CHOL (p = 0.015), KIRC (p = 2.6e-5), and PRAD (p = 0.025) (Figure 1E). OS displayed that TLR4 was markedly correlated with the prognosis of KIRC (p = 0.0018), SKCM (p = 0.0064), STAD (p = 0.038), TGCT (p = 0.03), and UCEC (p = 0.049) (Figure 1F). DSS reflected that TLR4 was correlated with the prognosis of KIRC (p = 0.00019), SKCM (p = 0.0094), and UCEC (p = 0.02) (Figure 1G). DFI showed that TLR4 was dramatically correlated with the prognosis of HNSC (p = 0.0089) and PRAD (p = 0.013) (Figure 1H). These outcomes showed that low expression of TLR4 is related to the low survival rate of KIRC (p < 0.0001) (Figures 2C,G,I), SKCM (p = 0.00023) (Figures 2D,J), UCEC (p = 0.00079) (Figures 2E,M), and CHOL (p = 0.0048) (Figure 2F), while high TLR4 expression is related to low survival rates of HNSC (p = 0.001) (Figure 2A), PRAD (p = 0.0024) (Figures 2B,H), STAD (p = 0.0095) (Figure 2K), and TGCT (p = 0.00076) (Figure 2L). Finally, TLR4 gene expression and OS-related cancer types were screened for further study. Therefore, the low TLR4 expression may be a risk factor for an inferior prognosis in patients with KIRC, SKCM, UCEC, and CHOL, and similarly, high TLR4 expression may be a risk factor for an inferior prognosis in patients with HNSC, PRAD, STAD, and TGCT.
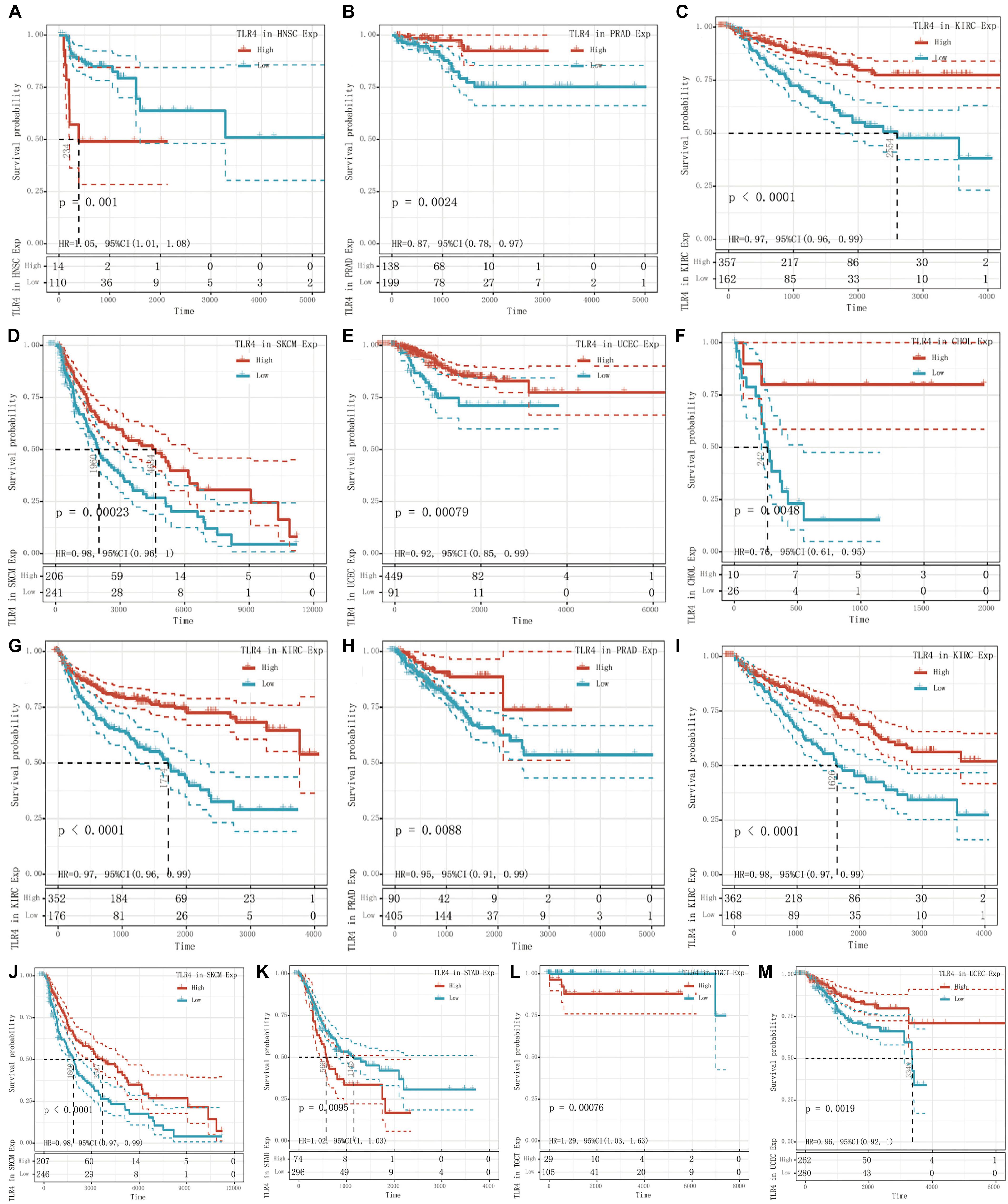
Figure 2. Survival curve of cancer types with significant correlation between TLR4 gene expression and prognosis. The low expression of TLR4 was related to the low survival rate of KIRC (p < 0.0001) (C,G,I), SKCM (p = 0.00023) (D,J), UCEC (p = 0.00079) (E,M), and CHOL (p = 0.0048) (F). High TLR4 expression is related to low survival rates of HNSC (p = 0.001) (A), PRAD (p = 0.0024) (B,H), STAD (p = 0.0095) (K), and TGCT (p = 0.00076) (L).
Relationship Between Toll-Like Receptor 4 Expression and Six Types of Infiltrating Immune Cells
Tumor-infiltrating lymphocytes are self-governed predictors of tumor sentinel lymph node status and survival rate (Dunn et al., 2007; Azimi et al., 2012). We analyzed the expression of TLR4 and six types of infiltrating immune cells (B cells, CD4+ T cells, CD8+ T cells, neutral granulocytes, macrophages, and dendritic cells). The outcomes indicated that the expression of TLR4 was negatively correlated with the expression of B cells in STAD, KIRC, UCEC, TGCT, and SKCM. The expression of TLR4 was positively correlated with the infiltration of B cells, CD4+ T cells, CD8+ T cells, neutrophils, macrophages, and dendritic cells in STAD, KIRC, UCEC, TGCT, and SKCM (Figures 3A–E). To make the results more convincing, we conducted more in-depth research on tumor samples. The results revealed that the expression of TLR4 gene in KIRC, TGCT, UCEC, SKCM and STAD has different degrees of correlation with tumor infiltrating immune cell subsets (Supplementary Figures 1–3). These findings strongly indicated that the TLR4 gene plays a role in the immune infiltration of KIRC, SKCM, STAD, TGCT, and UCEC.
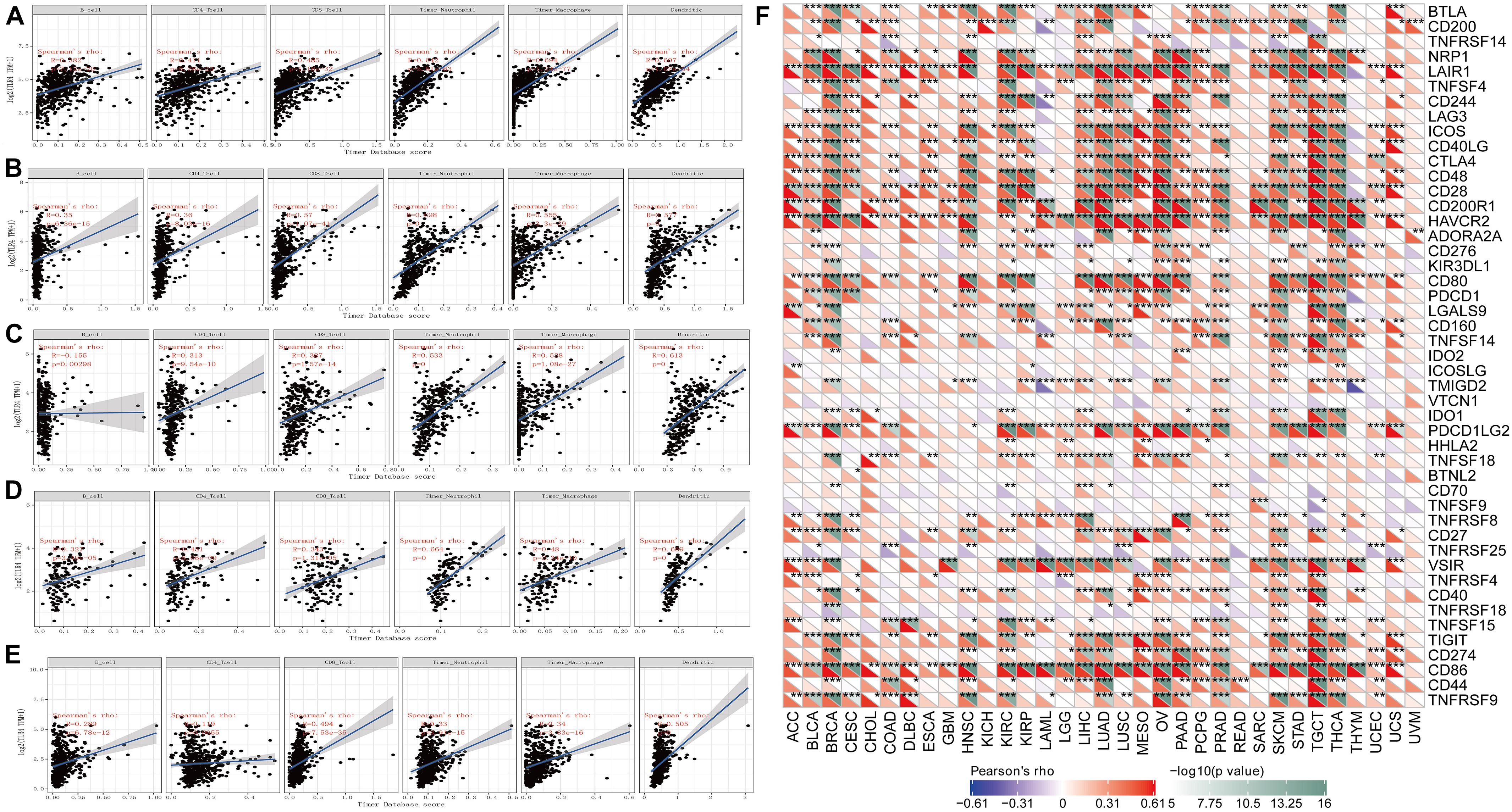
Figure 3. Correlation analysis of TLR4 gene expression in immune infiltration, immune checkpoint, and in 33 tumors. (A–E) The expression of TLR4 was negatively correlated with the expression of B cells in STAD, KIRC, UCEC, TGCT, and SKCM. While the expression of TLR4 was positively correlated with the infiltration of B cells, CD4+ T cells, CD8+ T cells, neutrophils, macrophages, and dendritic cells in STAD, KIRC, UCEC, TGCT, and SKCM. (F) The results showed that the five prognostic-related cancers of KIRC, SKCM, STAD, TGCT, and UCEC are highly positively correlated with the six immune checkpoints of ICOS, CTLA4, CD28, CD80, PDCD1LG2, and CD86 (r > 0, ***p < 0.001).
An increasing number of reports have proven that the tumor immune microenvironment plays a part in the occurrence and development of tumors. To further evaluate the role of TLR4 in the tumor immune microenvironment, our results showed that the expression of the TLR4 gene in the ESTIMATE immune score was associated with CESC (p = 0), ESCA (p = 0), and HNSC (p = 0) (Figure 4A). These results showed that the five prognosis-related cancers—KIRC, SKCM, STAD, TGCT, and UCEC—were highly positively correlated with the six immune checkpoints—inducible T-cell costimulator (ICOS), cytotoxic T lymphocyte-associated molecule 4 (CTLA4), CD28, CD80, PDCD1LG2, and CD86 (r > 0, ∗∗∗p < 0.001) (Figure 3F and Table 1).
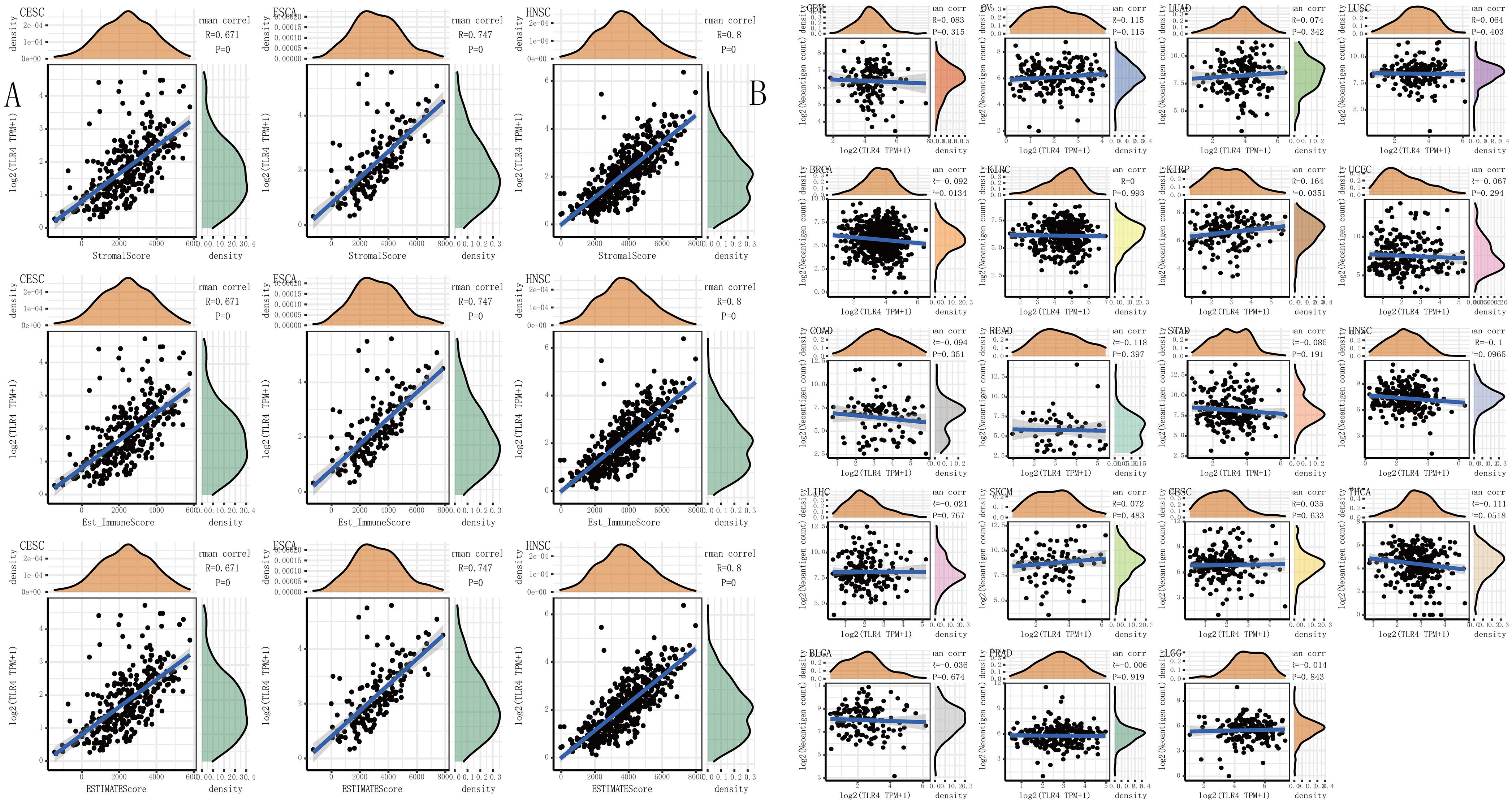
Figure 4. The relationship between the TLR4 gene expression and immune score, neoantigens. (A) The expression of TLR4 gene in the ESTIMATE immune score was concerned with CESC (p = 0), ESCA (p = 0), HNSC (p = 0) are prominently correlated. (B) KIRC, SKCM, STAD, TGCT, and UCEC were not notably related to neoantigens. A p-value <0.05 was considered statistically significant. The symbols “*,” “**,” and “***” refer to p-values <0.05, <0.01, and <0.001, respectively.
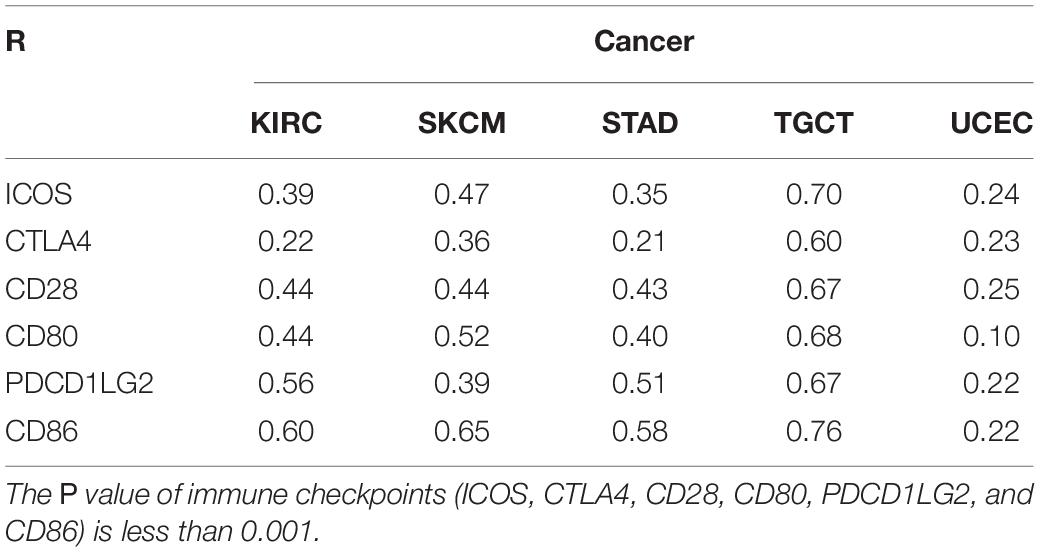
Table 1. The relationship between TLR4 gene expression in KIRC, SKCM, STAD, TGCT, UCEC and immune checkpoint.
The Relationship Between Toll-Like Receptor 4 Gene Expression in Immunotherapy-Related Markers and Clinicopathological Characteristics
We separately counted the number of neoantigens in each tumor sample, and the results showed that KIRC, SKCM, STAD, TGCT, and UCEC were not notably related to neoantigens (Figure 4B). TMB is a quantitative biomarker that can reflect the number of mutations in tumor cells. We separately counted the TMB of KIRC, SKCM, STAD, TGCT, and UCEC samples, and the results showed that the expression of the TLR4 gene was noticeably related to TMB in STAD (p = 0.046) and UCEC (p = 0.0044) (Figure 5A). Our research results displayed that the expression of the TLR4 gene in TGCTs (p = 0.0071), STAD (p = 1.6e-05), and SKCM (p = 4.6e-05) was significantly correlated with MSI (Figure 5B). The analysis outcomes revealed that the expression of the TLR4 gene was conspicuously linked to the methyltransferases DNA methyltransferase 2 (DNMT2) and DNA methyltransferase 3B (DNMT3B) in SKCM and STAD (p < 0.05) and was markedly correlated with the methyltransferase DNMT2 in UCEC and KIRC (p < 0.05) (Figure 5C). The above results suggest that TLR4 gene expression has a certain effect on the prognosis of patients with KIRC, SKCM, STAD, TGCT, and UCEC. Moreover, we also analyzed the mutation patterns of the TLR4 gene in tumor samples. TCGA analysis revealed that the mutation rates of the TLR4 gene in KIRC (Figure 5D), SKCM (Figure 5E), STAD (Figure 5F), and UCEC (Figure 5G) were 0.6, 11.35, 5.95, and 7.74%, respectively. In addition, we further explored the somatic mutations of TLR4 gene in KIRC, SKCM, STAD, TGCT, and UCEC and the relationship between TLR4 gene expression and age, gender, race and stage. The results showed that the TLR4 gene somatic mutations in KIRC, SKCM, STAD, TGCT and UCEC are mainly diploid/normal, arm-level deletion, arm-level gain, and high amplification rarely (Supplementary Figure 4). Moreover, the expression of TLR4 gene is correlated with gender and race of TGCT, KIRC, SKCM and UCEC, and correlated with the age of KIRC. In addition, the expression of TLR4 gene is correlated with the tumor stages of KIRC and UCEC (Supplementary Figure 5). Except for TGCT and UCEC, the expression of TLR4 gene in KIRC, SKCM and STAD tumor stages is consistent with the results of previous studies (Supplementary Figure 6).
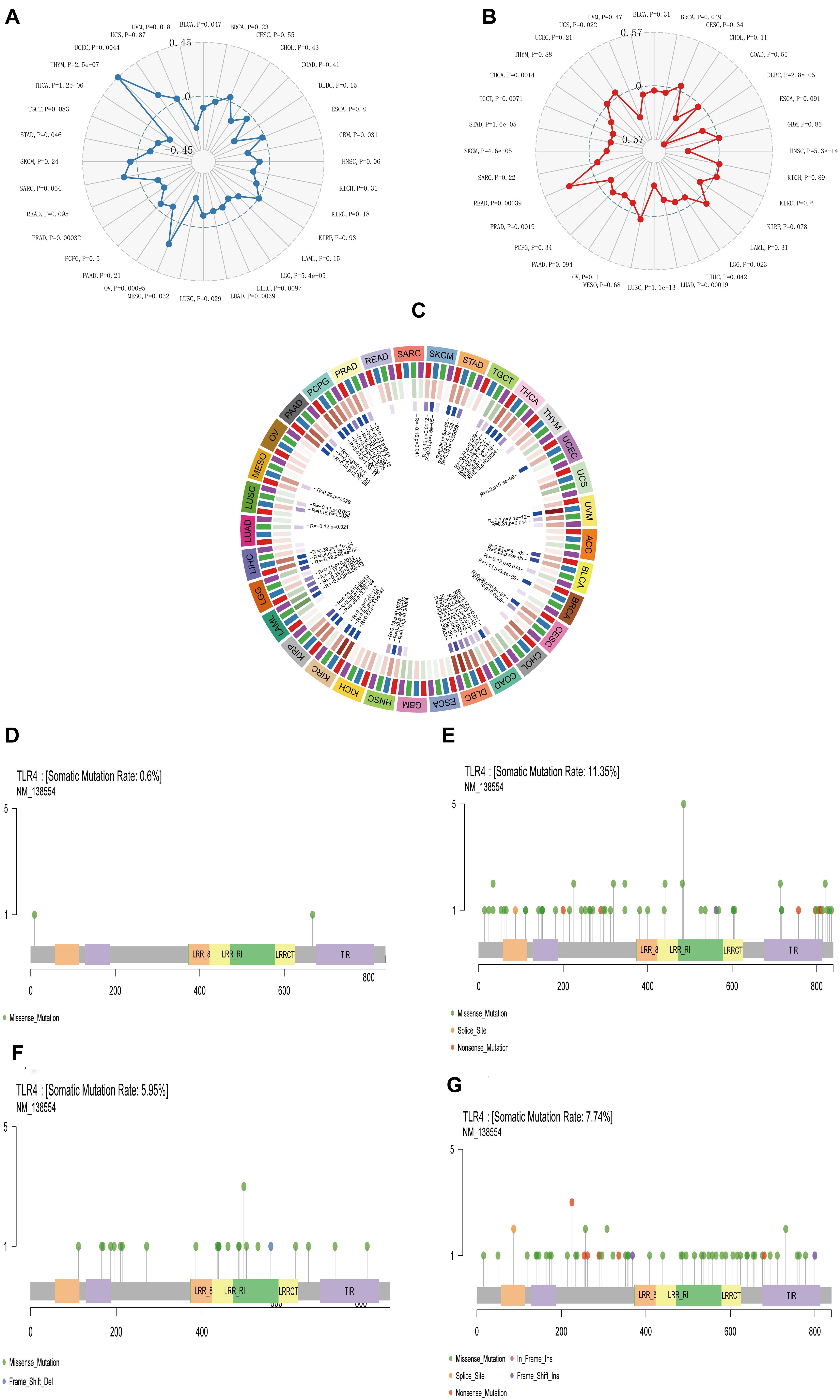
Figure 5. The relationship between TLR4 gene expression and TMB, MSI, methyltransferase, and mutation frequency. (A) The expression of TLR4 gene was noticeably relevant to TMB in STAD (p = 0.046) and UCEC (p = 0.0044). (B) The expression of TLR4 gene in TGCT (p = 0.0071), STAD (p = 1.6e-05), and SKCM (p = 4.6e-05) was significantly correlated with MSI. (C) The analysis outcomes revealed that the expression of TLR4 gene was conspicuously linked to methyltransferase DNMT2 and DNMT3B in SKCM and STAD (p < 0.05) and was markedly correlated with methyltransferase DNMT2 in UCEC and KIRC (p < 0.05). TCGA analysis displayed that the mutation rates of TLR4 gene in KIRC (D), SKCM (E), STAD (F), and UCEC (G) were 0.6, 11.35, 5.95, and 7.74%, respectively.
For the purpose of better understanding the correlation and latent mechanism of TLR4 expression in cancer, TCGA expression profile data were used to assess the relationship between five DNA repair genes (MLH1, MSH2, MSH6, PMS2, EPCAM mutations) and gene expression. Our results showed that TLR4 gene expression was highly linked to the DNA repair genes MLH1, MSH2, MSH6, and PMS2 in KIRC and STAD and MLH1, MSH2, and MSH6 in SKCM (∗∗p < 0.001); mildly correlated with MLH1, MSH2, and PMS2 in UCEC (∗p < 0.001) 05); and moderately correlated with EPCAM (∗∗p < 0.01) (Figure 6B).
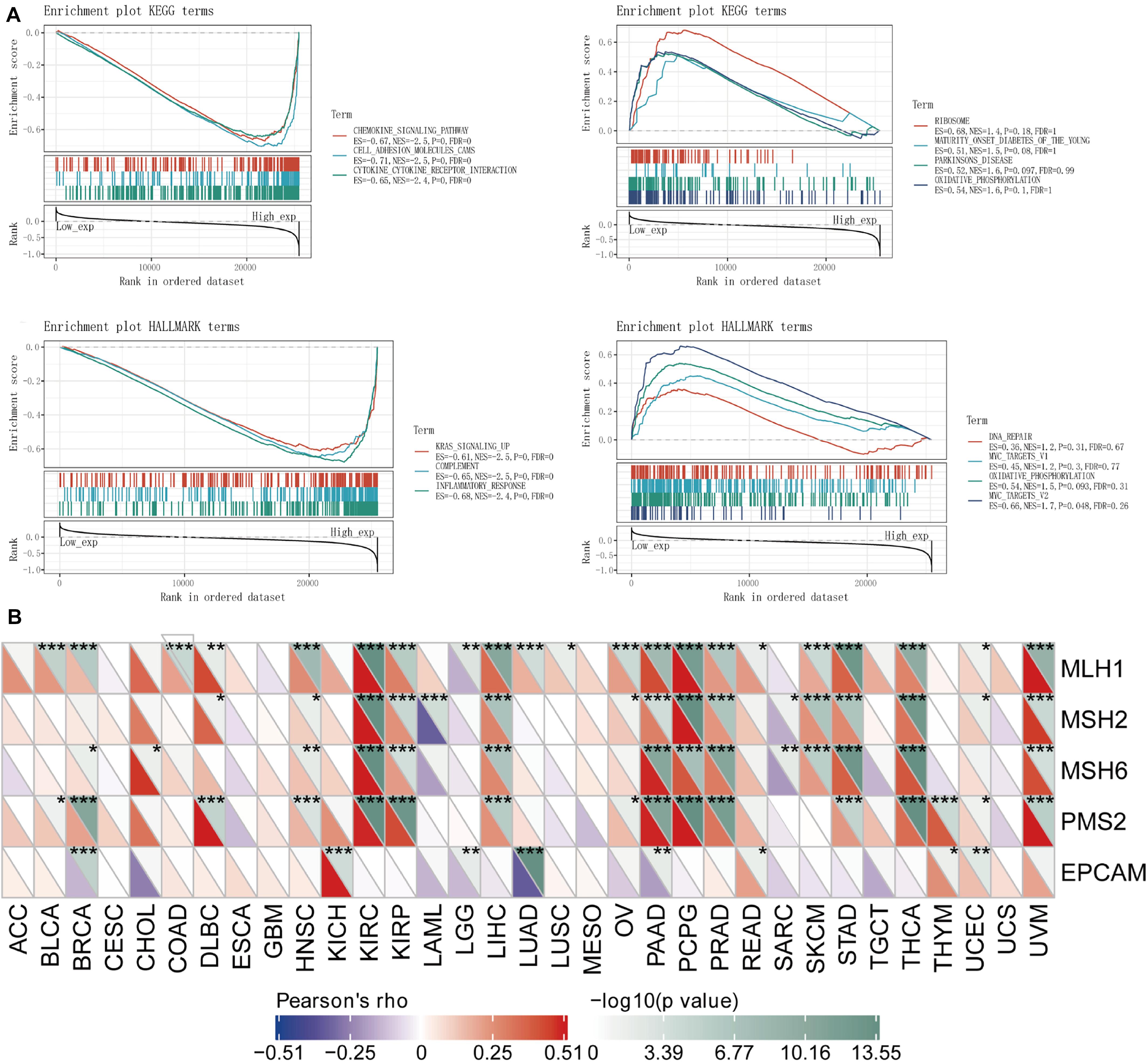
Figure 6. Analysis of the TLR4 gene enrichment and DNA repair genes. (A) KEGG analysis displayed that TLR4 was highly expressed in chemokine signaling pathway (p = 0), cell adhesion molecule (p = 0), and interaction of cytokine receptor (p = 0). HALLMARK analysis showed that TLR4 is lowly expressed in MYC_TARGETS_V2 (p = 0.048) and highly expressed in KRAS signaling pathway (p = 0) and inflammatory response (p = 0) pathway. (B) The TLR4 gene expression was highly linked to DNA repair genes MLH1, MSH2, MSH6, and PMS2 in KIRC and STAD and MLH1, MSH2, and MSH6 in SKCM (**p < 0.001); mildly correlated with MLH1, MSH2, and PMS2 in UCEC (*p < 0.001) 05); and showed a moderate correlation with EPCAM (**p < 0.01). A p-value <0.05 was considered statistically significant.
To observe the effect of gene expression on tumors, we used GSEA to analyze the enrichment of the KEGG and HALLMARK pathways in the high-expression group and the low-expression group. KEGG analysis showed that TLR4 was highly expressed in the chemokine signaling pathway (p = 0), cell adhesion molecule (p = 0), and cytokine receptor interaction (p = 0). HALLMARK analysis showed that TLR4 was expressed at low levels MYC_TARGETS_V2 cells (p = 0.048) and highly expressed in the KRAS signaling pathway (p = 0) and inflammatory response (p = 0) pathway (Figure 6A and Table 2).
Finally, to further compare whether the expression of the TLR4 gene is different in the cancer tissues of 14 types of tumors and the corresponding normal tissues, both the HPA database and IHC staining were verified, and the results confirmed that the TLR4 gene was significantly highly expressed in COAD, LIHC, OV, PRAD, BRCA, PAAD, and UCEC (Figures 7, 8 and Table 3).
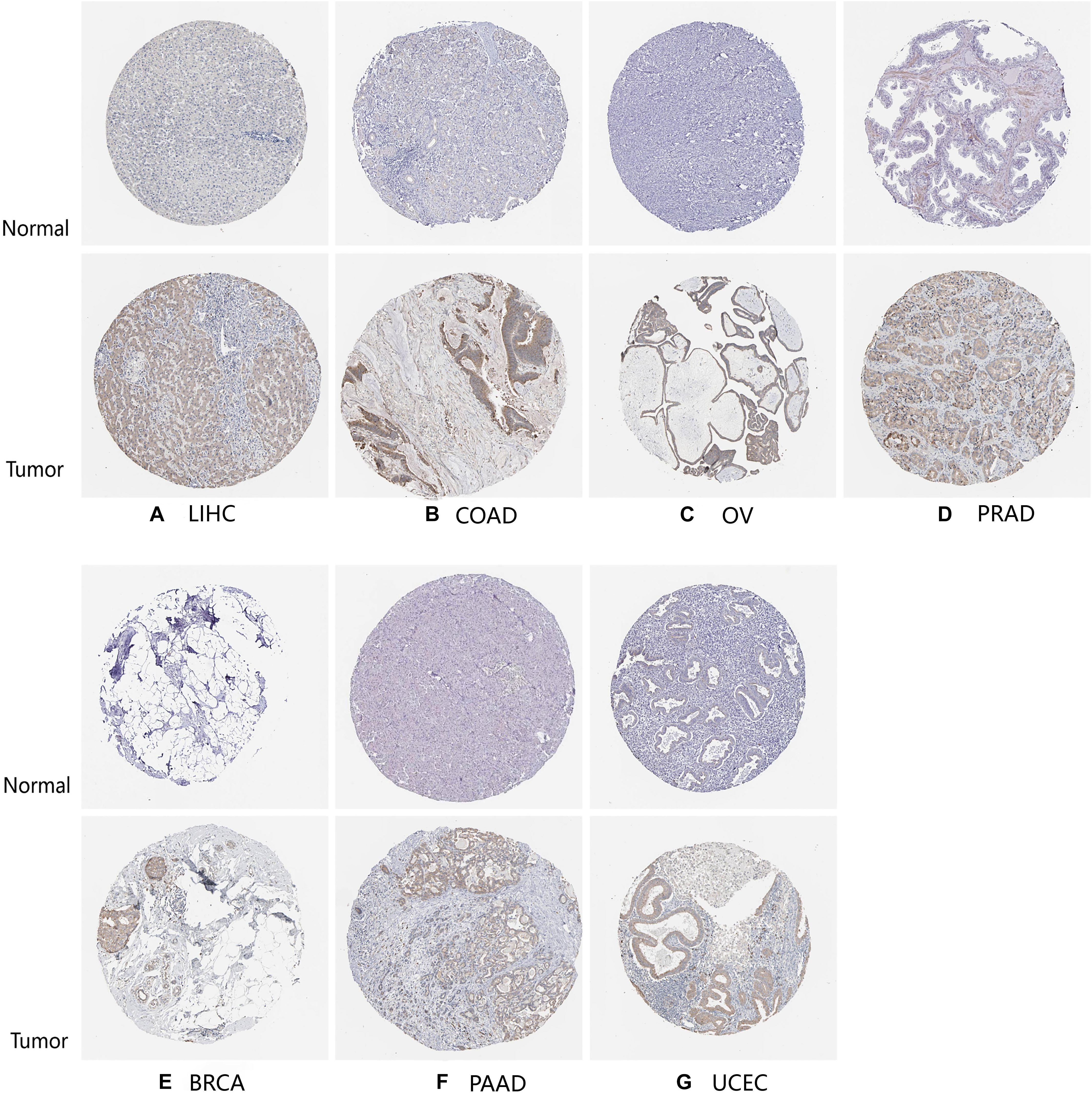
Figure 7. HPA database verifies the expression of TLR4 gene in seven tumors. The expression of TLR4 gene in LIHC (A), COAD (B), OV (C), PRAD (D), BRCA (E), PAAD (F), and UCEC (G) is significantly higher than that in the corresponding normal tissues.
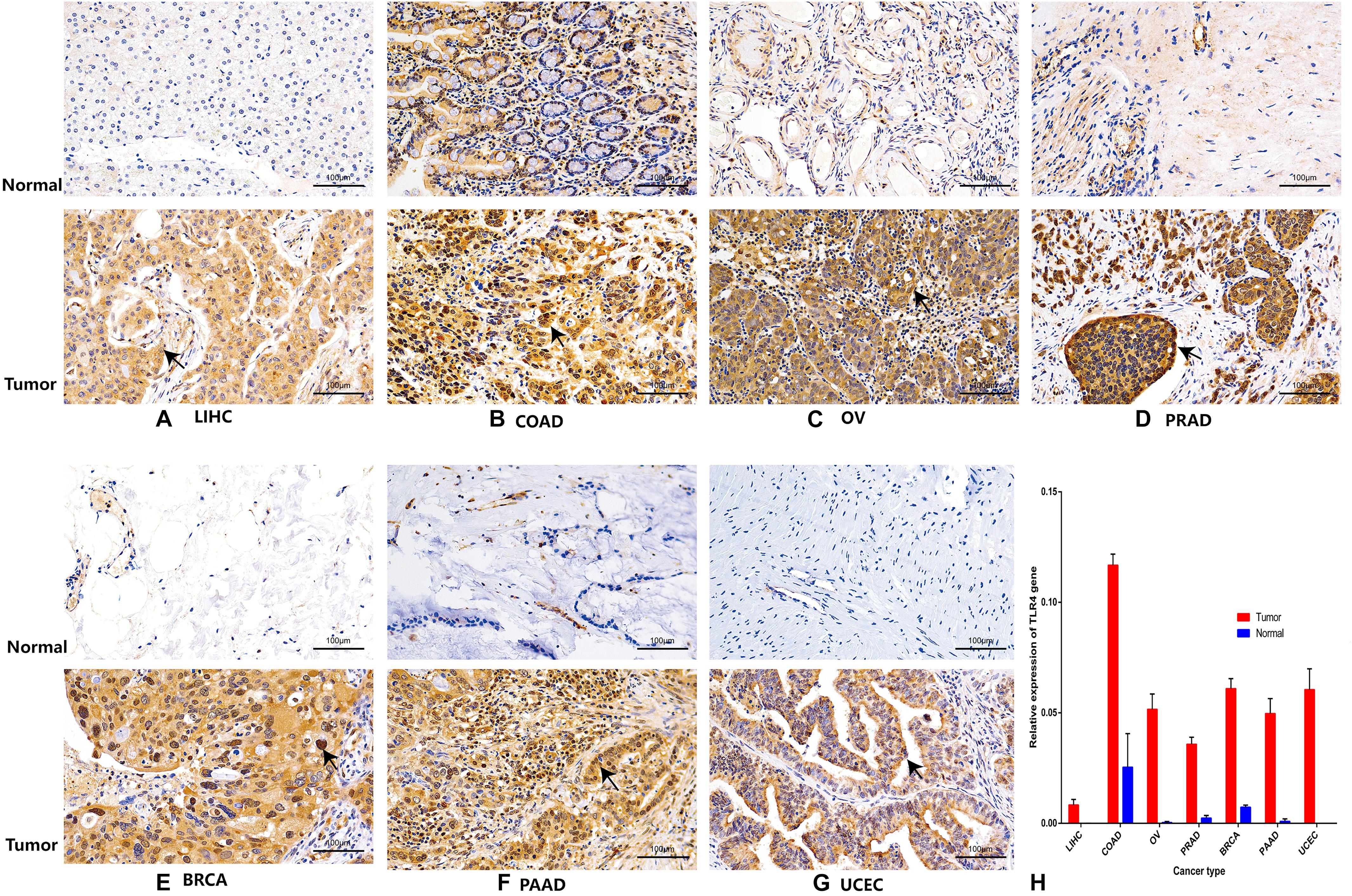
Figure 8. Immunohistochemistry verifies the expression of TLR4 gene in seven tumors. The expression of TLR4 gene in LIHC (A), COAD (B), OV (C), PRAD (D), BRCA (E), PAAD (F), and UCEC (G) is significantly higher than that in the corresponding normal tissues.
Discussion
Malignant tumors are a complex disease that is related to the expression and functional changes of a variety of immune system-related molecules (Gu et al., 2016). On the one hand, the immune system plays a strong antitumor role in; on the other hand, it also contributes to the occurrence and development of tumors, so it is thought to be a double-edged sword (Hagemann et al., 2007). Previous studies have indicated that tumor progression, drug resistance, and metastasis are caused by cytokines and chemokines secreted by tumor cells and their adjacent stromal cells. In previous experiments, it has been shown that cancer cells are usually highly infiltrated by innate immune cells, and they have co-opted some immune-related pathways during the development of the disease (He et al., 2007). Recently, the important role of the TLR4 pathway in drug resistance and metastasis has drawn the attention of scientists (González-Reyes et al., 2010).
TLR4 belongs to the pathogen recognition receptor (PRR) family, and it plays an important role in activating/inhibiting immune and non-immune cells by recognizing pathogen-related molecular patterns (PAMPs) and damage-related molecular patterns (DAMPs; Bagheri et al., 2014). Extracellular TLR4 contains a leucine repeat sequence (LRR) domain that binds to a ligand, and it can recognize a variety of ligands such as lipopolysaccharide (LPS) and heat shock proteins 60 and 70 (Evans et al., 2003). It interacts with MYD88 and TRIF through the cytoplasmic Toll/IL-1 receptor (TIR) domain (Yamamoto et al., 2003). In the MYD88-dependent pathway, TLR4 and myeloid differentiation 2 produce dimers and then recruit the adaptor protein [toll-interleukin 1 receptor domain-containing adaptor protein (TIRAP)] containing the TIRAP domain and the two adaptor proteins of MYD88. The activation of MYD88 in turn leads to the phosphorylation of interleukin-1 receptor-associated kinase-4 (IRAK-4) and IRAK-1, and tumor necrosis factor (TNF) receptor-associated factor-6 (TRAF-6) is the main transcription factor molecule that activates the nuclear factor-κB (NF-κB) chain enhancer of B cells. After activation, both of these proteins stimulate the κB-inhibitor (IκB) complex (IKK) and degrade IκBα. This leads to NF-κB activation and nuclear translocation, thereby altering the cell function (Yamamoto et al., 2003).
In the process of tumor initiation or progression, different tumors may have different degrees of the TLR4 involvement. Based on these facts, we decided to study the expression of TLR4 gene across cancers and its relationship with the prognosis of different cancer patients and tumor-infiltrating lymphocytes. To the best of our knowledge, this is the first comprehensive and systematic study of the differential expression and related mechanisms of TLR4 across cancers.
Here, we analyzed the expression and prognosis of the TLR4 gene in 33 tumors in TCGA database using gene expression profile data. Differential expression of TLR4 between cancer cells and normal tissues existed in many types of cancer. We found that compared with normal tissues, TLR4 gene expression was lower in ACC, BLCA, BRCA, CESC, CHOL, HNSC, KIRP, LUAD, READ, THCA, UCEC, and UCS. In some data sets, high expression was observed in COAD, ESCA, GBM, KICH, LGG, LIHC, PAAD, SKCM, STAD, and TGCT. In previous experiments, it was proven that the genes contained in this feature are all related to cancer (Schmausser et al., 2004; Hassan et al., 2006; He et al., 2007; Ikebe et al., 2009; Szczepanski et al., 2009; Tang and Zhu, 2012; Tewari et al., 2012; Wang et al., 2014; Jain et al., 2015; Olbert et al., 2015; Lin et al., 2016; Jiang et al., 2017). However, in these databases, we also found that low TLR4 expression was associated with a poor prognosis in KIRC, SKCM, and UCEC. Interestingly, high TLR4 expression was associated with an inferior prognosis of HNSC, PRAD, STAD, and TGCT. The expression level of the TLR4 gene was also related to the OS of KIRC, SKCM, STAD, TGCT, and UCEC, which indicated that TLR4 expression could be used as a predictor of tumor prognosis.
Another vital aspect of this study is that the TLR4 gene is associated with different levels of immune infiltration in cancers (KIRC, SKCM, STAD, TGCT, and UCEC), and this is further associated with OS. Through time analysis of public databases, our results revealed that TLR4 gene expression was markedly negatively correlated with B cells in STAD and positively mutually related to the infiltration levels of CD4+ T cells, CD8+ T cells, neutrophils, macrophages, and dendritic cells. The expression of the TLR4 gene was related to B cells, CD4+ T cells, CD8+ T cells, and neutrophils in KIRC, UCEC, TGCT, and SKCM. The infiltration levels of the cells, macrophages and dendritic cells, were significantly positively correlated. These results suggested that TLR4 gene can regulate tumor immunity in KIRC, SKCM, STAD, TGCT, and UCEC. In this era of stratified medicine, the identification of immune biomarkers is increasingly vital (Chen et al., 2019). A number of studies have found that tumor-infiltrating lymphocytes, namely, tumor-associated macrophages (TAMs) and tumor-infiltrating neutrophils (TINs), influence the prognosis and the efficacy of chemotherapy and immunotherapy (Waniczek et al., 2017; Zhang et al., 2018). Therefore, our findings may play a crucial role in identifying new immune-related therapeutic targets.
Furthermore, immune checkpoints are molecules that have the ability to regulate T cells. They are a set of inhibition and stimulation pathways that directly affect the function of immune cells (Feng et al., 2019). When cancer occurs, the immune checkpoint (IC) signal becomes a way for the tumor to engage in immune escape, leading to tumor aggressiveness. To date, the most effective immune checkpoint inhibitor is a monoclonal antibody that binds to programmed death-1 (PD-1) or its ligand PD-L1 (Traini et al., 2019). However, only a small number of patients receiving immune-checkpoint inhibitors (ICIs) targeting PD-L1 or CTLA-4 have good clinical treatment effects (Sun et al., 2018). Therefore, it is necessary to study new suppression checkpoints and their target molecules to expand the use and efficacy of existing immune checkpoint suppression therapies (Granier et al., 2017). In this study, we conducted a systematic analysis of more than 40 common immune checkpoint genes. The results showed that the five TLR4 prognosis-related cancers KIRC, SKCM, STAD, TGCT, and UCEC were related to ICOS, CTLA4, CD28, and CD80. The six immune checkpoints, PDCD1LG2, and CD86 are highly correlated. To our knowledge, previous studies have not reported a comprehensive checkpoint analysis that is highly relevant in KIRC, SKCM, STAD, TGCT, and UCEC. Immune checkpoints have triggered a wave of research on immune checkpoint therapy, that is, CD8 tumor-infiltrating T lymphocytes (TILs) are reactivated, which is a promising anticancer treatment. Hauzenberger et al. (1999) proved that TNF regulates the expression of CXCL12 through TLR4, thereby impairing the function of CD8 TILs. By regulating CD8 TILs, it helps to evade antitumor immunity. This mechanism seems to be related to human breast cancer (Hauzenberger et al., 1999). Similarly, a study conducted by Beswick et al. (2014) discussed the immune tolerance of colonic mucosa induced by TLR4/PD-L1. In addition, Wölfle et al. (2011) described a similar induction effect of TLR4 activation on PD-L1 expression. Given that the expression of TLR4 is significantly related to the prognosis of KIRC, SKCM, STAD, TGCT, and UCEC, and previous studies have paved the way for the discovery of new checkpoints and follow-up studies, our research can provide a foundation for the development of more immunotherapies and model animals in the future and could provide potential therapeutic targets.
According to most reports, the efficacy of immune checkpoint blockade is related to the immunogenicity of the tumors (Snyder et al., 2014). Because the number of T cells infiltrated by tumors is small, tumors with low immunogenicity tend to respond weakly to immune checkpoint-blocking therapy (Tumeh et al., 2014). Under normal circumstances, immune checkpoint molecules downregulate activation signals from costimulatory molecules to maintain self-tolerance and prevent autoimmunity. However, tumor cells can use this mechanism to inhibit the activation and functional status of T cells, leading to T-cell exhaustion and tumor immune escape. Previous studies have shown that dual CD40–TLR4 activation in a tumor is one way to overcome resistance to PD-1 blockade. The unique feature of this method is that it can lead to the loss of depleted T cells in the tumor (Khalil et al., 2019). Recent studies have shown that silica nanoparticles promote the tumor infiltration of CD8+ cytotoxic T lymphocytes (CTLs) by targeting the TLR4/NF-κB pathway. Through PD-1 blockade, the depletion of CTLs is activated and reversed, thereby generating durable antitumor immunity (Sun et al., 2021). Recent findings in chronic inflammatory diseases and cancer indicate that T-cell failure and lymphopenia may be related to changes in the expression level of TLR4 (Wild et al., 2012). T-cell depletion is a key obstacle to effective immunotherapy (Pauken and Wherry, 2015). Due to the TLR4 signaling pathway and its extensive interactions, TLR4 is considered to be a potential target of immune agents. In view of the differences in immune efficacy caused by individual differences, it is very important to choose the most suitable therapy for each patient. Therefore, there is still much work to be done in the field of effective immunotherapy. Future research will need to fully clarify how TLR agonists affect the depletion of T cells, and the therapeutic effect on distant tumors is a very interesting issue.
In view of the correlation between TLR4 expression and the immune invasion level and immune checkpoints in different types of cancer, we determined the relationship between TLR4 and the prognosis of different types of cancer immune neoantigen, TMB, MSI, DNA repair genes, and DNA methylation. As expected, our results demonstrated that there was no obvious correlation with the new tumor antigen. The expression of TLR4 gene was correlated with the target cancers. These findings may help to understand the special role of TLR4 in genetic expression and gene repair.
As a functional unit of the genome, cell signaling pathways play an important biological role in major cellular processes including cancer occurrence and development. Bioinformatics enrichment analysis showed that TLR4 was highly expressed in the interaction pathways of chemokine signaling pathways, cell adhesion molecules, and cytokine receptors. HALLMARK analysis showed that TLR4 was expressed at low levels in MYC_TARGETS_V2 and that TLR4 was expressed in the KRAS signaling pathway and highly expressed in the inflammatory response pathway. The MYC gene family consists of three members, C-MYC, L-MYC, and N-MYC. Among them, C-MYC is the most widely studied, and MYC is the most frequently amplified gene (Duffy and Crown, 2021). All MYC proteins mainly act as transcription regulators, regulating the expression of genes involved in a variety of cellular processes, proliferation, survival, metabolism, invasion, and metastasis (Duffy and Crown, 2021). According to previous reports, among 33 different cancers, MYC amplification was found in 21% of patients, especially BRCA, UCEC, and OV (McAnulty and DiFeo, 2020). High MYC has been reported to be associated with a poor prognosis of prostate cancer and breast cancer (Maroto et al., 2017). Our hallmark analysis showed that low expression of the TLR4 gene was enriched in the MYC pathway. In addition, TLR4 gene expression was related to the prognosis of the above five cancers. Combined with the above studies, we found that there may be an indispensable link between the expression of the TLR4 gene and MYC, and they may be potential therapeutic candidates for the development of new therapeutic strategies. Although it is full of challenges, there is no doubt that in-depth studies of various tumor immune states are needed to provide valuable insights for potentially effective treatment strategies. Recent research showed that chemokines and cytokines, namely, TNF-α, IL-6, and chemokine (C-C motif) ligand 2 (CCL2), have roles in the formation of the cancer microenvironment and are responsible for the migration of inflammatory cells and cancer cells (King, 2015). It is worth noting that our findings have similar results to all known studies. The TLR4 signaling pathway and its extensive interactions seem to be potential targets for immunotherapy.
Finally, to confirm the results of the above database analysis, we verified from the HPA database that the TLR4 gene was significantly highly expressed in COAD, LIHC, OV, PRAD, BRCA, PAAD, and UCEC. In the past few years, genetic markers have often been used to predict the outcome of various tumors (Karamichalis et al., 2016), and to a certain extent, they are even better than TNM staging and histopathological diagnosis (Chlis et al., 2018). In recent years, the expression of TLR in tumor tissues has been reported, which may provide a vital mechanism for alleviation of improper immune enhancement and immune dysfunction and lead to antitumor immune tolerance (Tang and Zhu, 2012; Fu et al., 2013; Mai et al., 2013; Wang et al., 2013). There is evidence that TLR4 has multiple associations with cancer. Different cell lines and tissue samples from patients with head and neck, esophageal, stomach, colon and rectum, liver, pancreatic, skin, breast, ovarian, and cervical cancer showed an increase in TLR4 expression (Mai et al., 2013).
In addition, we must admit that our analysis has potential limitations. The association between the expression of the TLR4 gene across cancers and prognosis is based on an analysis of public databases. Therefore, our work is retrospective rather than prospective. Moreover, follow-up animal experiment verification and further multicenter, large-sample, prospective studies are required to testify the relationship between TLR4 and patient prognosis and to seek more effective treatment strategies.
Data Availability Statement
The datasets presented in this study can be found in online repositories. The names of the repository/repositories and accession number(s) can be found in the article/Supplementary Material.
Ethics Statement
The studies involving human participants were reviewed and approved by Institutional Ethics Committee of the Second Affiliated Hospital of Nanchang University. The patients/participants provided their written informed consent to participate in this study.
Author Contributions
GX designed the research. JH, JX, XF, and YL performed the experiments and analyzed the data. JH wrote the manuscript. FH and GX revised the manuscript. All authors read and approved the final manuscript.
Funding
This work was supported by grants from the National Natural Science Foundation of China (Nos. 81760208 and 81760261).
Conflict of Interest
The authors declare that the research was conducted in the absence of any commercial or financial relationships that could be construed as a potential conflict of interest.
Publisher’s Note
All claims expressed in this article are solely those of the authors and do not necessarily represent those of their affiliated organizations, or those of the publisher, the editors and the reviewers. Any product that may be evaluated in this article, or claim that may be made by its manufacturer, is not guaranteed or endorsed by the publisher.
Supplementary Material
The Supplementary Material for this article can be found online at: https://www.frontiersin.org/articles/10.3389/fcell.2021.700661/full#supplementary-material
Abbreviations
TLRs, toll-like receptors; TLR4, toll-like receptor 4; KIRC, kidney renal clear cell carcinoma; SKCM, skin cutaneous melanoma; STAD, stomach adenocarcinoma; TGCT, testicular germ cell tumor; UCEC, uterine corpus endometrial carcinoma; GTEx, Genotype-Tissue Expression; CCLE, Cancer Cell Line Encyclopedia; TCGA, The Cancer Genome Atlas; PFI, process-free intervention; OS, overall survival; DSS, disease-specific survival; DFI, disease-free intervention; TIMER, Tumor Immune Assessment Resource; TMB, tumor mutation burden; MSI, microsatellite instability; GSEA, Gene Set Enrichment Analysis; HPA, Human Protein Atlas; ACC, adrenocortical carcinoma; BLCA, bladder urothelial carcinoma; BRCA, breast invasive carcinoma; CESC, cervical squamous cell carcinoma and adenocarcinoma; CHOL, cholangiocarcinoma; HNSC, head and neck squamous cell carcinoma; KIRP, kidney renal papillary cell carcinoma; LUAD, lung adenocarcinoma; READ, rectal adenocarcinoma; THCA, thyroid cancer; UCS, uterine carcinoma; COAD, colon adenocarcinoma; ESCA, esophageal carcinoma; GBM, glioblastoma multiforme; KICH, kidney chromophobe; LGG, brain lower grade glioma; LIHC, liver hepatocellular carcinoma; PAAD, pancreatic adenocarcinoma; PRAD, prostate adenocarcinoma; OV, ovarian serous cystadenocarcinoma; KEGG, Kyoto Encyclopedia of Genes and Genomes; TAM, tumor-associated macrophage; TIN, tumor-infiltrating neutrophil; PRR, pathogen recognition receptor; PAMPs, pathogen-related molecular patterns; DAMPs, damage-related molecular patterns; LRR, leucine-rich repeat; TIR, Toll/interleukin-1 receptor; IRAK-4, interleukin-1 receptor-associated kinase-4; TNF, tumor necrosis factor; TRAF-6, tumor necrosis factor receptor-associated factor-6; NF- κ B, nuclear factor- κ B; PD-1, programmed death-1; TIL, tumor-infiltrating T lymphocyte; CTLs, cytotoxic T lymphocytes; I κ B, κ B inhibitor.
Footnotes
References
Azimi, F., Scolyer, R. A., Rumcheva, P., Moncrieff, M., Murali, R., McCarthy, S. W., et al. (2012). Tumor-infiltrating lymphocyte grade is an independent predictor of sentinel lymph node status and survival in patients with cutaneous melanoma. J. Clin. Oncol. 30, 2678–2683. doi: 10.1200/jco.2011.37.8539
Bagheri, V., Askari, A., Arababadi, M. K., and Kennedy, D. (2014). Can Toll-Like receptor (TLR) 2 be considered as a new target for immunotherapy against hepatitis B infection? Hum. Immunol. 75, 549–554. doi: 10.1016/j.humimm.2014.02.018
Balkwill, F., Charles, K. A., and Mantovani, A. (2005). Smoldering and polarized inflammation in the initiation and promotion of malignant disease. Cancer Cell 7, 211–217. doi: 10.1016/j.ccr.2005.02.013
Baniyash, M. (2006). Chronic inflammation, immunosuppression and cancer: new insights and outlook. Semin. Cancer Biol. 16, 80–88. doi: 10.1016/j.semcancer.2005.12.002
Beswick, E. J., Johnson, J. R., Saada, J. I., Humen, M., House, J., Dann, S., et al. (2014). TLR4 activation enhances the PD-L1-mediated tolerogenic capacity of colonic CD90+ stromal cells. J. Immunol. 193, 2218–2229. doi: 10.4049/jimmunol.1203441
Cammarota, R., Bertolini, V., Pennesi, G., Bucci, E. O., Gottardi, O., Garlanda, C., et al. (2010). The tumor microenvironment of colorectal cancer: stromal TLR-4 expression as a potential prognostic marker. J. Transl. Med. 8:112. doi: 10.1186/1479-5876-8-112
Chen, X. Y., Yeong, J., Thike, A. A., Bay, B. H., and Tan, P. H. (2019). Prognostic role of immune infiltrates in breast ductal carcinoma in situ. Breast Cancer Res. Treat. 177, 17–27. doi: 10.1007/s10549-019-05272-2
Chlis, N. K., Bei, E. S., and Zervakis, M. (2018). Introducing a stable bootstrap validation framework for reliable genomic signature extraction. IEEE/ACM Trans. Comput. Biol. Bioinform. 15, 181–190. doi: 10.1109/tcbb.2016.2633267
Duffy, M. J., and Crown, J. (2021). Drugging “undruggable” genes for cancer treatment: are we making progress? Int. J. Cancer 148, 8–17. doi: 10.1002/ijc.33197
Dunn, G. P., Dunn, I. F., and Curry, W. T. (2007). Focus on TILs: prognostic significance of tumor infiltrating lymphocytes in human glioma. Cancer Immun. 7:12.
El-Omar, E. M., Ng, M. T., and Hold, G. L. (2008). Polymorphisms in toll-like receptor genes and risk of cancer. Oncogene 27, 244–252. doi: 10.1038/sj.onc.1210912
Evans, J. T., Cluff, C. W., Johnson, D. A., Lacy, M. J., Persing, D. H., and Baldridge, J. R. (2003). Enhancement of antigen-specific immunity via the TLR4 ligands MPL adjuvant and Ribi.529. Expert Rev. Vaccines. 2, 219–229. doi: 10.1586/14760584.2.2.219
Feng, M., Jiang, W., Kim, B. Y. S., Zhang, C. C., Fu, Y. X., and Weissman, I. L. (2019). Phagocytosis checkpoints as new targets for cancer immunotherapy. Nat. rev. Cancer 19, 568–586. doi: 10.1038/s41568-019-0183-z
Fu, H. Y., Li, C., Yang, W., Gai, X. D., Jia, T., Lei, Y. M., et al. (2013). FOXP3 and TLR4 protein expression are correlated in non-small cell lung cancer: implications for tumor progression and escape. Acta Histochem. 115, 151–157. doi: 10.1016/j.acthis.2012.06.002
González-Reyes, S., Marín, L., González, L., González, L. O., del Casar, J. M., Lamelas, M. L., et al. (2010). Study of TLR3, TLR4 and TLR9 in breast carcinomas and their association with metastasis. BMC Cancer 10:665. doi: 10.1186/1471-2407-10-665
Granier, C., De Guillebon, E., Blanc, C., Roussel, H., Badoual, C., Colin, E., et al. (2017). Mechanisms of action and rationale for the use of checkpoint inhibitors in cancer. ESMO Open 2:e000213. doi: 10.1136/esmoopen-2017-000213
Grimm, M., Kim, M., Rosenwald, A., Heemann, U., Germer, C. T., Waaga-Gasser, A. M., et al. (2010). Toll-like receptor (TLR) 7 and TLR8 expression on CD133+ cells in colorectal cancer points to a specific role for inflammation-induced TLRs in tumourigenesis and tumour progression. Eur. J. Cancer 46, 2849–2857. doi: 10.1016/j.ejca.2010.07.017
Gu, G., Dustin, D., and Fuqua, S. A. (2016). Targeted therapy for breast cancer and molecular mechanisms of resistance to treatment. Curr. Opin. Pharmacol. 31, 97–103. doi: 10.1016/j.coph.2016.11.005
Hagemann, T., Balkwill, F., and Lawrence, T. (2007). Inflammation and cancer: a double-edged sword. Cancer Cell 12, 300–301. doi: 10.1016/j.ccr.2007.10.005
Hassan, F., Islam, S., Tumurkhuu, G., Naiki, Y., Koide, N., Mori, I., et al. (2006). Intracellular expression of toll-like receptor 4 in neuroblastoma cells and their unresponsiveness to lipopolysaccharide. BMC Cancer 6:281. doi: 10.1186/1471-2407-6-281
Hauzenberger, D., Olivier, P., Gundersen, D., and Rüegg, C. (1999). Tenascin-C inhibits beta1 integrin-dependent T lymphocyte adhesion to fibronectin through the binding of its fnIII 1-5 repeats to fibronectin. Eur. J. Immunol. 29, 1435–1447. doi: 10.1002/(sici)1521-4141(199905)29:05<1435::aid-immu1435>3.0.co;2-n
He, W., Liu, Q., Wang, L., Chen, W., Li, N., and Cao, X. (2007). TLR4 signaling promotes immune escape of human lung cancer cells by inducing immunosuppressive cytokines and apoptosis resistance. Mol. Immunol. 44, 2850–2859. doi: 10.1016/j.molimm.2007.01.022
Ikebe, M., Kitaura, Y., Nakamura, M., Tanaka, H., Yamasaki, A., Nagai, S., et al. (2009). Lipopolysaccharide (LPS) increases the invasive ability of pancreatic cancer cells through the TLR4/MyD88 signaling pathway. J. Surg. Oncol. 100, 725–731. doi: 10.1002/jso.21392
Jain, S., Suklabaidya, S., Das, B., Raghav, S. K., Batra, S. K., and Senapati, S. (2015). TLR4 activation by lipopolysaccharide confers survival advantage to growth factor deprived prostate cancer cells. Prostate 75, 1020–1033. doi: 10.1002/pros.22983
Jiang, N., Xie, F., Guo, Q., Li, M. Q., Xiao, J., and Sui, L. (2017). Toll-like receptor 4 promotes proliferation and apoptosis resistance in human papillomavirus-related cervical cancer cells through the Toll-like receptor 4/nuclear factor-κB pathway. Tumour Biol. 39, 1010428317710586.
Karamichalis, R., Kari, L., Konstantinidis, S., Kopecki, S., and Solis-Reyes, S. (2016). Additive methods for genomic signatures. BMC Bioinformatics 17:313. doi: 10.1186/s12859-016-1157-8
Khalil, D. N., Suek, N., Campesato, L. F., Budhu, S., Redmond, D., Samstein, R. M., et al. (2019). In situ vaccination with defined factors overcomes T cell exhaustion in distant tumors. J. Clin. Invest. 129, 3435–3447. doi: 10.1172/jci128562
King, P. T. (2015). Inflammation in chronic obstructive pulmonary disease and its role in cardiovascular disease and lung cancer. Clin. Transl. Med. 4:68.
Li, J., Yang, F., Wei, F., and Ren, X. (2017). The role of toll-like receptor 4 in tumor microenvironment. Oncotarget 8, 66656–66667. doi: 10.18632/oncotarget.19105
Lin, A., Wang, G., Zhao, H., Zhang, Y., Han, Q., Zhang, C., et al. (2016). TLR4 signaling promotes a COX-2/PGE(2)/STAT3 positive feedback loop in hepatocellular carcinoma (HCC) cells. Oncoimmunology 5:e1074376. doi: 10.1080/2162402x.2015.1074376
Mai, C. W., Kang, Y. B., and Pichika, M. R. (2013). Should a Toll-like receptor 4 (TLR-4) agonist or antagonist be designed to treat cancer? TLR-4: its expression and effects in the ten most common cancers. Onco Targets Ther. 6, 1573–1587. doi: 10.2147/ott.s50838
Mantovani, A., Allavena, P., Sica, A., and Balkwill, F. (2008). Cancer-related inflammation. Nature 454, 436–444.
Maroto, P., Esteban, E., Parra, E. F., Mendez-Vidal, M. J., Domenech, M., Pérez-Valderrama, B., et al. (2017). HIF pathway and c-Myc as biomarkers for response to sunitinib in metastatic clear-cell renal cell carcinoma. Onco Targets Ther. 10, 4635–4643. doi: 10.2147/ott.s137677
McAnulty, J., and DiFeo, A. (2020). The molecular ‘myc-anisms’ behind myc-driven tumorigenesis and the relevant myc-directed therapeutics. Int. J. Mol. Sci. 21:9486. doi: 10.3390/ijms21249486
McGettrick, A. F., and O’Neill, L. A. (2007). Toll-like receptors: key activators of leucocytes and regulator of haematopoiesis. Br. J. Haematol. 139, 185–193. doi: 10.1111/j.1365-2141.2007.06802.x
Olbert, P. J., Kesch, C., Henrici, M., Subtil, F. S., Honacker, A., Hegele, A., et al. (2015). TLR4- and TLR9-dependent effects on cytokines, cell viability, and invasion in human bladder cancer cells. Urol. Oncol. 33, 110.e19–e27.
Pauken, K. E., and Wherry, E. J. (2015). Overcoming T cell exhaustion in infection and cancer. Trends Immunol. 36, 265–276. doi: 10.1016/j.it.2015.02.008
Schmausser, B., Andrulis, M., Endrich, S., Lee, S. K., Josenhans, C., Müller-Hermelink, H. K., et al. (2004). Expression and subcellular distribution of toll-like receptors TLR4, TLR5 and TLR9 on the gastric epithelium in Helicobacter pylori infection. Clin. Exp. Immunol. 136, 521–526. doi: 10.1111/j.1365-2249.2004.02464.x
Snyder, A., Makarov, V., Merghoub, T., Yuan, J., Zaretsky, J. M., Desrichard, A., et al. (2014). Genetic basis for clinical response to CTLA-4 blockade in melanoma. N. Engl. J. Med. 371, 2189–2199. doi: 10.1056/nejmoa1406498
Sun, C., Mezzadra, R., and Schumacher, T. N. (2018). Regulation and function of the PD-L1 checkpoint. Immunity 48, 434–452. doi: 10.1016/j.immuni.2018.03.014
Sun, M., Gu, P., Yang, Y., Yu, L., Jiang, Z., Li, J., et al. (2021). Mesoporous silica nanoparticles inflame tumors to overcome anti-PD-1 resistance through TLR4-NFκB axis. J. Immunother. Cancer 9:e002508. doi: 10.1136/jitc-2021-002508
Szczepanski, M. J., Czystowska, M., Szajnik, M., Harasymczuk, M., Boyiadzis, M., Kruk-Zagajewska, A., et al. (2009). Triggering of Toll-like receptor 4 expressed on human head and neck squamous cell carcinoma promotes tumor development and protects the tumor from immune attack. Cancer Res. 69, 3105–3113. doi: 10.1158/0008-5472.can-08-3838
Takeda, K., and Akira, S. (2015). Toll-like receptors. Curr. Protoc. Immunol. 109, 14.12.1–14.12.10.
Tang, X., and Zhu, Y. (2012). TLR4 signaling promotes immune escape of human colon cancer cells by inducing immunosuppressive cytokines and apoptosis resistance. Oncol. Res. 20, 15–24. doi: 10.3727/096504012x13425470196092
Tewari, R., Choudhury, S. R., Ghosh, S., Mehta, V. S., and Sen, E. (2012). Involvement of TNFα-induced TLR4-NF-κB and TLR4-HIF-1α feed-forward loops in the regulation of inflammatory responses in glioma. J. Mol. Med. 90, 67–80. doi: 10.1007/s00109-011-0807-6
Torre, L. A., Bray, F., Siegel, R. L., Ferlay, J., Lortet-Tieulent, J., and Jemal, A. (2015). Global cancer statistics, 2012. CA Cancer J. Clin. 65, 87–108. doi: 10.3322/caac.21262
Traini, G., Ruiz-de-Angulo, A., Blanco-Canosa, J. B., Zamacola Bascarán, K., Molinaro, A., Silipo, A., et al. (2019). Cancer immunotherapy of TLR4 agonist-antigen constructs enhanced with pathogen-mimicking magnetite nanoparticles and checkpoint blockade of PD-L1. Small 15:e1803993.
Tumeh, P. C., Harview, C. L., Yearley, J. H., Shintaku, I. P., Taylor, E. J., Robert, L., et al. (2014). PD-1 blockade induces responses by inhibiting adaptive immune resistance. Nature 515, 568–571.
Wang, A. C., Ma, Y. B., Wu, F. X., Ma, Z. F., Liu, N. F., Gao, R., et al. (2014). TLR4 induces tumor growth and inhibits paclitaxel activity in MyD88-positive human ovarian carcinoma in vitro. Oncol. Lett. 7, 871–877. doi: 10.3892/ol.2013.1759
Wang, L., Zhao, Y., Qian, J., Sun, L., Lu, Y., Li, H., et al. (2013). Toll-like receptor-4 signaling in mantle cell lymphoma: effects on tumor growth and immune evasion. Cancer 119, 782–791. doi: 10.1002/cncr.27792
Waniczek, D., Lorenc, Z., Śnietura, M., Wesecki, M., Kopec, A., and Muc-Wierzgoń, M. (2017). Tumor-associated macrophages and regulatory T cells infiltration and the clinical outcome in colorectal cancer. Arch. Immunol. Ther. Exp. 65, 445–454. doi: 10.1007/s00005-017-0463-9
Wild, C. A., Bergmann, C., Fritz, G., Schuler, P., Hoffmann, T. K., Lotfi, R., et al. (2012). HMGB1 conveys immunosuppressive characteristics on regulatory and conventional T cells. Int. Immunol. 24, 485–494. doi: 10.1093/intimm/dxs051
Wölfle, S. J., Strebovsky, J., Bartz, H., Sähr, A., Arnold, C., Kaiser, C., et al. (2011). PD-L1 expression on tolerogenic APCs is controlled by STAT-3. Eur. J. Immunol. 41, 413–424. doi: 10.1002/eji.201040979
Yamamoto, M., Sato, S., Hemmi, H., Hoshino, K., Kaisho, T., Sanjo, H., et al. (2003). Role of adaptor TRIF in the MyD88-independent toll-like receptor signaling pathway. Science 301, 640–643. doi: 10.1126/science.1087262
Keywords: immune cell infiltration, toll-like receptor 4, pan-cancer, prognosis, bioinformatics
Citation: Hu J, Xu J, Feng X, Li Y, Hua F and Xu G (2021) Differential Expression of the TLR4 Gene in Pan-Cancer and Its Related Mechanism. Front. Cell Dev. Biol. 9:700661. doi: 10.3389/fcell.2021.700661
Received: 26 April 2021; Accepted: 27 July 2021;
Published: 23 September 2021.
Edited by:
Giuseppe Palma, Istituto Nazionale Tumori Fondazione G. Pascale (IRCCS), ItalyReviewed by:
Josephin Nerling Rashida Gnanaprakasam, Nationwide Children’s Hospital, United StatesHongyu Xu, School of Medicine, Virginia Commonwealth University, United States
Copyright © 2021 Hu, Xu, Feng, Li, Hua and Xu. This is an open-access article distributed under the terms of the Creative Commons Attribution License (CC BY). The use, distribution or reproduction in other forums is permitted, provided the original author(s) and the copyright owner(s) are credited and that the original publication in this journal is cited, in accordance with accepted academic practice. No use, distribution or reproduction is permitted which does not comply with these terms.
*Correspondence: Fuzhou Hua, huafuzhou@126.com; Guohai Xu, 852432755@qq.com
†These authors have contributed equally to this work and share first authorship