- 1Department of Pulmonary and Critical Care Medicine, Qilu Hospital, Cheeloo College of Medicine, Shandong University, Jinan, China
- 2School of Physical Science, University of California, Irvine, Irvine, CA, United States
- 3Department of Biology Engineering, Shandong Jianzhu University, Jinan, China
- 4School of Statistics and Management, Shanghai University of Finance and Economics, Shanghai, China
Long non-coding RNA (lncRNA) plays a crucial role in modulating genome instability, immune characteristics, and cancer progression, within which genome instability was identified as a critical regulator in tumorigenesis and tumor progression. However, the existing accounts fail to detail the regulatory role of genome instability in lung adenocarcinoma (LUAD). We explored the clinical value of genome instability-related lncRNA in LUAD with multi-omics bioinformatics analysis. We extracted the key genome instability-related and LUAD-related gene modules using weighted gene co-expression network analysis (WGCNA) and established a competing endogenous RNA (ceRNA) network using four lncRNAs (LINC01224, LINC00346, TRPM2-AS, and CASC9) and seven target mRNAs (CCNF, PKMYT1, GCH1, TK1, PSAT1, ADAM33, and DDX11). We found that LINC01224 is primarily located in the cytoplasm and that LINC00346 and TRPM2-AS are primarily located in the nucleus (CASC9 unknown). We found that all 11 genes were positively related to tumor mutational burden and involve drug resistance, cancer stemness, and tumor microenvironment infiltration. Additionally, an eight-lncRNA genome instability-related lncRNA signature was established and validated, predicting the overall survival and immunotherapy outcomes in LUAD. To conclude, we discovered that sponging microRNA, genome instability-related lncRNA functions as ceRNA, modulating genomic integrity. This research provides clinical references for LUAD immunotherapy and prognosis and interprets a potential genome instability-related ceRNA regulatory network in which LINC01224-miR-485-5p/miR-29c-3p-CCNF-RRM2 and LINC01224-miR485-5p-PKMYT1-CDK1 axes were the most promising pathways. However, the potential mechanisms underlying our findings still need biological validation through in vitro and in vivo experiments.
Introduction
At present, the incidence and mortality of lung cancer rank first among malignant tumors worldwide. Non-small-cell lung cancer (NSCLC) is the most common pathological type of lung cancer, accounting for 85% of all tumors, among which lung adenocarcinoma (LUAD) is the primary subtype, accounting for up to 40% (Zhong et al., 2019; Li et al., 2020; Niu et al., 2020). Unlike lung squamous cell carcinoma (LUSC), women and non-smokers comprise most LUAD patients (Pros et al., 2020). Besides, blood metastasis can occur in the early stage of LUAD without clinical symptoms, leading to a poor prognosis (Wang et al., 2019). Even though traditional therapeutic strategies, including surgery, chemoradiotherapy, and targeted molecular therapy, have rapidly developed in recent years, LUAD patients’ overall survival (OS) does not improve considerably (Lee et al., 2019). Current clinical practice has demonstrated the prominent effect of immunotherapy, especially after the discovery of immune checkpoint, primarily including programmed cell death 1 (PD-1), programmed cell death-ligand 1 (PD-L1), and cytotoxic T lymphocyte antigen 4 (CTLA-4). A recent prospective randomized clinical trial, KEYNOTE-042, demonstrated that pembrolizumab achieved a significantly longer OS in advanced LUAD patients even with low PD-L1 expression (Mok et al., 2019). Nevertheless, immune checkpoint inhibitor (ICI) expense significantly burdens patients and government health insurance (Carbone et al., 2017; Niu et al., 2020), and the median objective response rate is only 48.5% in PD-L1-overexpressing (≥50%) NSCLC (Frost et al., 2021). In addition to PD-L1, microsatellite instability (MSI) has been proved to be a novel ICI biomarker for colorectal cancer (Overman et al., 2017), but due to its rarity in LUAD, MSI cannot become promising ICI biomarkers for LUAD (Takamochi et al., 2017). Therefore, there is still an urgent need to explore more effective immunotherapy biomarkers.
It has previously been observed that tumor mutational burden (TMB) is closely related to an increased tumor immunotherapy response rate in LUAD and other cancer types (Rizvi et al., 2018; Liu et al., 2019; Yu et al., 2019). Genome instability, demonstrated as the endogenous source of mutation and tumor heterogeneity, modulates the alterations of epigenomic features (Tubbs and Nussenzweig, 2017; Peyraud and Italiano, 2020). Recent evidence suggests that long non-coding RNA (lncRNA) plays a critical role in regulating genome instability (Lee et al., 2016; Ventura, 2016; Hu et al., 2018; Munschauer et al., 2018). NORAD is the first discovered lncRNA, which assembles a critical topoisomerase complex maintaining genomic integrity. Hu et al. (2018) found that lncRNA GUARDIN functions as a scaffold RNA to sustain breast cancer 1 (BRCA1) stability. Moreover, GUARDIN could maintain chromosome end-to-end fusion through the GUARDIN-miR-23/TRF2 pathway. However, the clinical value and potential regulatory mechanism of genome instability-related lncRNA in LUAD remain elusive.
Here, we performed an integrative multi-omics analysis to explore the mechanisms and clinical value of genome instability-related lncRNA (GlncR) in LUAD. We adopted a weighted gene co-expression network analysis (WGCNA) to extract the key genome instability-related and LUAD-related modules and then screened four lncRNAs (LINC01224, LINC00346, TRPM2-AS, and CASC9) that were most related to genome instability. We established a competing endogenous RNA (ceRNA) network and used subcellular localization analysis to investigate the potential regulatory role of the four lncRNAs. We applied TMB, MSI, drug sensitivity, immune analyses on the four lncRNAs and seven target messenger RNAs (mRNAs) (CCNF, PKMYT1, GCH1, TK1, PSAT1, ADAM33, and DDX11). To elucidate the clinical value of the GlncRs, we constructed an eight-lncRNA prognostic signature predicting the OS. Surprisingly, our signature could predict the response rate to PD-1/PD-L1 inhibitors, and several existing biomarkers (TMB, PD-L1 expression, POLE mutation rate, and CD8+ cell infiltration) proved this point. To conclude, we established a novel genome instability-related ceRNA network and proposed an eight-lncRNA gene signature predicting OS and immunotherapy outcomes in LUAD.
Materials and Methods
Data Collection
The graphical presentation and online resources of our study design are shown in the graphical abstract. Clinical features, transcriptome profiling (gene expression quantification (RNA-seq, which was preprocessed by fragments per kilobase of an exon model per million mapped fragments (FPKM)) and microRNA (miRNA) expression quantification (miRNA-seq)), and simple nucleotide variation (SNV, Masked Somatic Mutation detected by VarScan 2) from the LUAD project of The Cancer Genome Atlas (TCGA) database were download through the Genomic Data Commons Data Portal website (https://portal.gdc.cancer.gov/, 2020.12.25). RNA-seq included 535 tumor samples and 59 paired normal samples. Corresponding clinical features included 500 patients (13 tumor samples were excluded due to lack of OS, and 22 tumor samples were duplicate samples (the average expression data of duplicate samples from one patient were utilized)). We used the “limma” package to normalize the transcriptome profiling (log2(FPKM + 1)). To identify the lncRNA and mRNA from total RNA-seq, the genome annotation file Genome Reference Consortium Human Build 38 patch release 13 (GRCh38.p13, downloaded from the National Center for Biotechnology Information)1 was used to reannotate the RNA-seq. We randomly divided the 500 samples into a training cohort (250 samples) and a validation cohort (250 samples) to build and validate the lncRNA prognostic signature. A chi-square test was utilized to detect the selection bias. The miRNA-seq was normalized by the “edgR” package, including 521 tumor samples and 46 paired normal samples. Extraction of the mutation status of each patient from SNV data was fulfilled by Perl language. Each patient’s TMB/MSI status was retrieved from the open-access bioinformatics website cBioPortal (the MSIsensor score was used, https://www.cbioportal.org/, 2020.12.26) (Cerami et al., 2012). Since all the data are open access, no ethics approval was acquired. The policies and publication guidelines of the TCGA database were strictly followed.
WGCNA
We performed WGCNA by R package “WGCNA” to identify the gene co-expression modules that were most relevant to LUAD development and genome instability. The total RNA-seq, including 535 tumor samples and 59 normal samples, was included in the WGCNA of LUAD development. We first used R package “limma” to extract differentially expressed genes (DEGs) between the tumor sample and normal sample (adjusted P-value (adj.P) < 0.05; | log2 fold change (FC)| > 0.5) for the co-expression module construction. Six was set as the soft power using the pickSoftThreshold function. As for the WGCNA of LUAD genome instability, we first sorted all patients according to somatic mutation counts (SMC) from largest to smallest. The first quarter of patients was classified into the genome-unstable group (GU group). The last quarter of patients was classified into the genome-stable group (GS group). Only the RNA-seq of the GS and GU groups was included in the WGCNA of LUAD genome instability. Then, we extracted the DEGs between the GS and GU groups (adj.P < 0.05; | log2 FC| > 0.5) for the co-expression module construction. Five was set as soft power. The detailed steps of the WGCNA technology followed the “WGCNA” package instruction. The code we used could be acquired by contacting the corresponding author.
Identification of Genome Instability-Related lncRNA (GlncRs), mRNA (GmRs), and miRNA (GmiRs)
We used R package “limma” to extract DEGs between the GU and GS groups. Differentially expressed lncRNAs were named GlncRs (adj.P < 0.05; | log2 FC| > 1), mRNAs were named GmRs (adj.P < 0.05; | log2 FC| > 1), and miRNAs were named GmiRs (adj.P < 0.05; | log2 FC| > 0.5).
ceRNA Network Construction
The overlapping lncRNAs in the top three LUAD development-related modules, genome instability-related module, and GlncRs were included in the ceRNA network construction. We searched an online ceRNA interaction network predictive database, ENCORI (The Encyclopedia of RNA Interactomes, ceRNA interaction network, http://starbase.sysu.edu.cn/ceRNA.php?source=lncRNA, 2021.1.12), for each included lncRNA to explore the possible target mRNAs (Li et al., 2013). As described on the website, the presented ceRNA interactive network from thousands of interactions of miRNA–targets was supported by crosslinking immunoprecipitation (CLIP)-seq data. We then excluded the GlncR target mRNAs that are neither in the module most related to genome instability nor in the GmRs. Furthermore, only the lncRNA–mRNA pairs that are statistically significant (P < 0.05) in LUAD based on the ENCORI database (through a pan-cancer analysis of miRNA–targets and RBP (RNA-binding protein)-RNAs in 32 types of cancers) remained. Then, the predicted mediating miRNAs between the lncRNA–mRNA pairs were retrieved. The overlapping miRNAs in GmiRs and predicted miRNAs were included in the ceRNA networks. The graphical representation of the screening process is presented in Figures 2A–C. Then, to explore the potential function of the included lncRNAs, an online lncRNA subcellular localization database, lncATLAS,2 was utilized. Cytoscape, a software for processing complicated networks, was used to modify the ceRNA network (Shannon et al., 2003).
Genome Instability, Immune, Drug Sensitivity, and Cancer Stemness Analyses
We performed a pan-cancer analysis to explore the potential biological function of the lncRNAs and their target mRNAs in the ceRNA network based on TCGA pan-cancer data downloaded from the Xena platform,3 including RNA-seq, clinical data, and cancer stemness scores based on mRNA expression (RNA stemness score, RNAss) and DNA methylation pattern (DNA stemness score, DNAss) (Malta et al., 2018). Thirty-three cancer types (ACC, BLCA, BRCA, CESC, CHOL, COAD, DLBC, ESCA, GBM, HNSC, KICH, KIRC, KIRP, LAML, LGG, LIHC, LUAD, LUSC, MESO, OV, PAAD, PCPG, PRAD, READ, SARC, SKCM, STAD, TGCT, THCA, THYM, UCEC, UCS, and UVM) were included. We calculated the correlation coefficient between each gene and the TMB/MSI status in 33 cancer types by Spearman correlation. We then calculated the correlation coefficient between every two genes by Spearman correlation to detect these genes’ interactions. Genome instability fosters tumor heterogeneity, contributing to tumor progression, drug resistance, tumor microenvironment (TME) alterations, and cancer stemness (Morel et al., 2017; Dagogo-Jack and Shaw, 2018; Sansregret et al., 2018). Therefore, the prognosis, drug sensitivity, TME infiltration, and cancer stemness analysis of these genes were conducted. GEPIA,4 an online website bioinformatics tool, was utilized to perform survival analysis based on overall analysis and disease-free survival (DFS). We utilized the National Cancer Institute (NCI)-605 database to perform the drug sensitivity analysis of the lncRNAs and their target mRNAs. NCI-60 is an open-access database based on nine cancer types and 60 cancer cell lines, consisting of mRNA expression level and corresponding z scores of cell sensitivity data (GI50) after drug treatment. We calculated the Pearson correlation between each gene expression and the GI50 to explore the association between these genes and drug sensitivity. We selected 262 FDA-approved drugs or drugs that are currently in clinical trials in this drug sensitivity analysis (Zhang et al., 2020). TME infiltration analysis was performed by the Estimation of STromal and Immune cells in MAlignant Tumor tissues using Expression data (ESTIMATE) immune and stromal score downloaded from ESTIMATE6 (Yoshihara et al., 2013). Furthermore, to validate these genes’ immune function, the six immune subtypes obtained from TCGA pan-cancer data were used to test the association between each gene and immune infiltrate types by analysis of variance (Thorsson et al., 2018). The cancer stemness features obtained from TCGA pan-cancer data were used to test the association between each gene and stem-cell-like features of tumor cells by Spearman correlation test. To further explore these genes’ potential mechanisms in modulating genomic integrity and TME, we detected the correlation between each gene and four MMR genes (MLH1, MSH2, MSH6, and PMS2), six immune-checkpoint-related genes (PD-L1 (CD274), PDCD1, PDCD1LG2, CTLA4, CD80, and CD86), and two previously discovered genome instability regulatory lncRNAs (NORAD (Munschauer et al., 2018) and LNCTAM34A (GUARDIN) (Hu et al., 2018)). The normality test of the indexes (TMB/MSI score, drug sensitivity index, ESTIMATE score, and RNAss/DNAss) was performed by the Kolmogorov–Smirnov test (Supplementary Table 1). The drug sensitivity indexes were normally distributed. Therefore, the method of Pearson correlation between gene expression and drug sensitivity was reasonable.
Construction and Validation of the Genome Instability-Related lncRNA Prognostic Signature (GIRlncPS)
We used the uni- and multi-variate Cox regression to construct the GIRlncPS based on OS in the training cohort using GlncRs. The risk score was calculated as follows: risk score = sum(coefficient (multivariate Cox regression) × corresponding lncRNA expression). Hazard ratio (HR) was calculated as exp(coefficient). Dividing the training cohort based on the median risk score, we classified all 500 patients into high- and low-risk groups. Survival analysis by the Kaplan–Meier (KM) curve and the log-rank test was conducted to evaluate the prognostic value of the GIRlncPS. The receiver operating characteristic (ROC) curve and area under the curve (AUC) were utilized to evaluate the reliability of the GIRlncPS. The TMB status, MSI status, expression of four MMR genes (MLH1, MSH6, PMS2, and MSH2), two immune-checkpoint-related genes (CD274 (PD-L1) and CTLA4), and the mutation rate of POLE (a novel discovered biomarker for ICI therapy outcomes (Wang F. et al., 2019)) of the two groups were compared by the Mann–Whitney U test. The R package “gsva” was utilized to perform the single-sample gene set enrichment analysis (ssGSEA) to compare the two groups’ immune cell infiltration and immune functions. Furthermore, we compared the mutation rate of TP53 between the two groups in the TCGA cohort. TP53 is one of the most mutated tumor suppressor genes acting as a genomic integrity guard. We classified the patients into four groups, TP53 mutated/high-risk, TP53 mutated/low-risk, TP53 wild/high-risk, TP53 wild/low-risk groups, to detect if the GIRlncPS has better predictive ability than TP53. Survival analysis by the KM curve between the four groups was performed. Then, we searched Google Scholar for previously published prognostic lncRNA signatures for LUAD. ROC curve and AUC were used to compare these signatures’ predictive ability with the GIRlncPS based on OS at 1, 2, and 3 years in the overlapping patients (500 patients). The included signatures were as follows: Li’s signature, 2020, and Sui’s signature, 2020. Independent analysis with clinical prognostic factors was performed by uni- and multi-variate Cox regression in the TCGA cohort. The included clinical features were as follows: age (>65 vs. ≤65), gender (male vs. female), stage (III/IV vs. I/II), T (III/IV vs. I/II), M (I vs. 0), N (I/II vs. 0), EGFR (mutation vs. wild), and TP53 (mutation vs. wild). We plotted the nomogram in the TCGA cohort for clinical reference. The calibration curve was plotted to evaluate the reliability of the nomogram.
Statistics
The descriptive analysis and normality test were conducted by IBM SPSS Statistics 26.0 (International Business Machines Corporation, Armonk, NY, United States). Other statistics were performed by R language (version 4.0.3) (R Core Team, 2013). The adj.P (q-value and false discovery rate (FDR)) was adjusted by Benjamini and Hochberg. Adj.P < 0.05 was considered statistically significant in DEG extraction, and P-value < 0.05 was significant in other conditions.
Results
WGCNA
We utilized the “WGCNA” to explore the potential gene modules with the closest relation to LUAD development and genome instability. The results of the WGCNA are presented in Figure 1. As for the WGCNA of LUAD development, 5,326 genes were included in the co-expression module construction, and a total of 10 gene co-expression modules were acquired. The top three LUAD development-related modules were turquoise (r = 0.8, P = 7e−136), yellow (r = 0.64, P = 9e−69), and blue modules (r = 0.59, P = 2e−57), indicating that the genes cooperate to promote tumorigenesis in these three modules, respectively. Similarly, a total of 3,489 genes were included in the WGCNA of LUAD genome instability. As shown in Figures 1B,D, eight modules were acquired, and the turquoise module was most relevant to genome instability (r = 0.64, P = 4e−33). The detailed results, including the gene significance (GS) and module membership (MM) of each gene, are provided in Supplementary Tables 2 and 3.
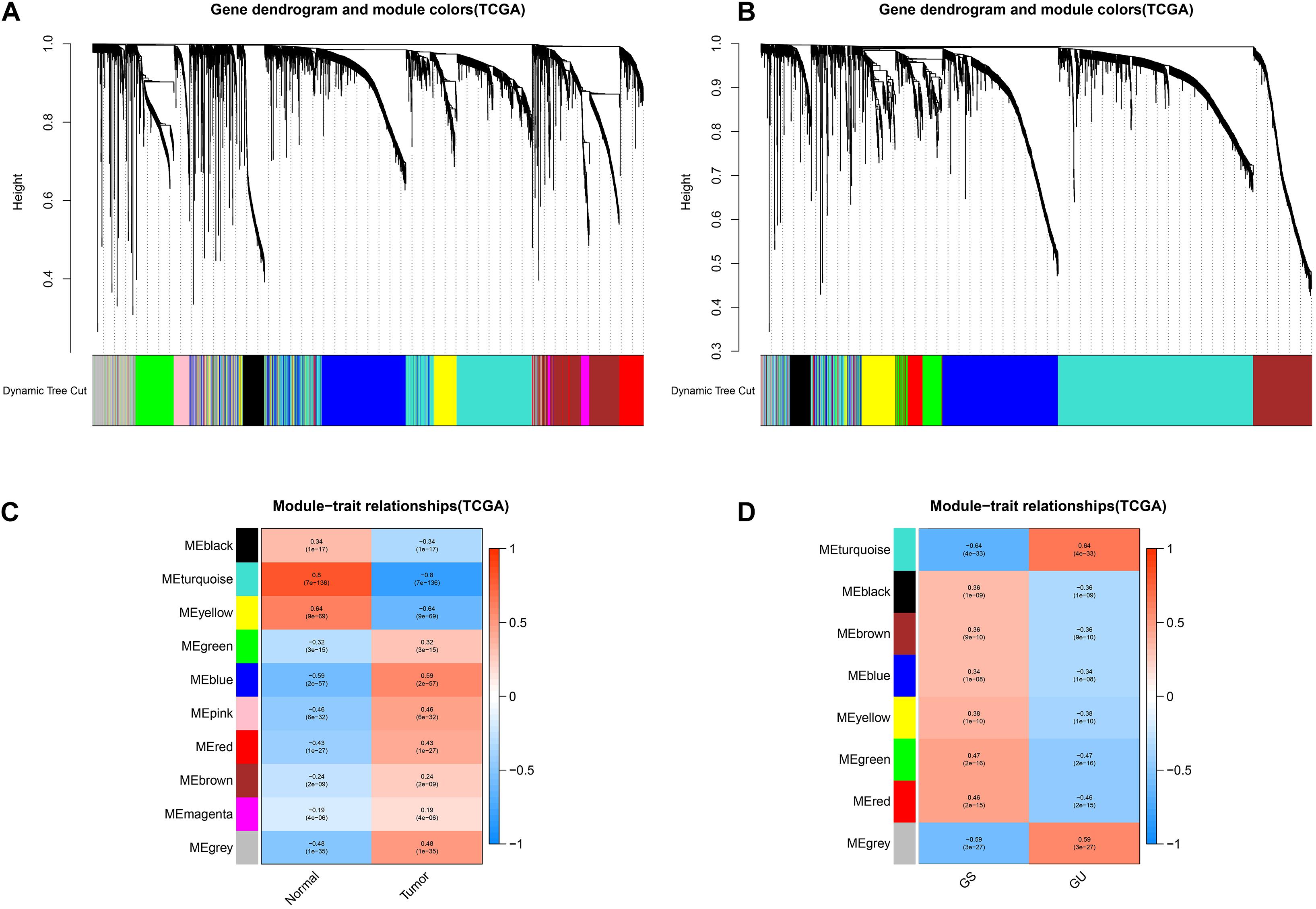
Figure 1. Identification of key tumor- and genome instability-related modules via the WGCNA. The cluster dendrogram of genes in the key tumor-related modules (A) and genome instability-related modules (B). The module–trait relationship of key tumor-related modules (C) and genome instability-related modules (D). The coefficient varies from –1 to 1 as color changes as blue–white–red. GS, genome stable; GU, genome unstable.
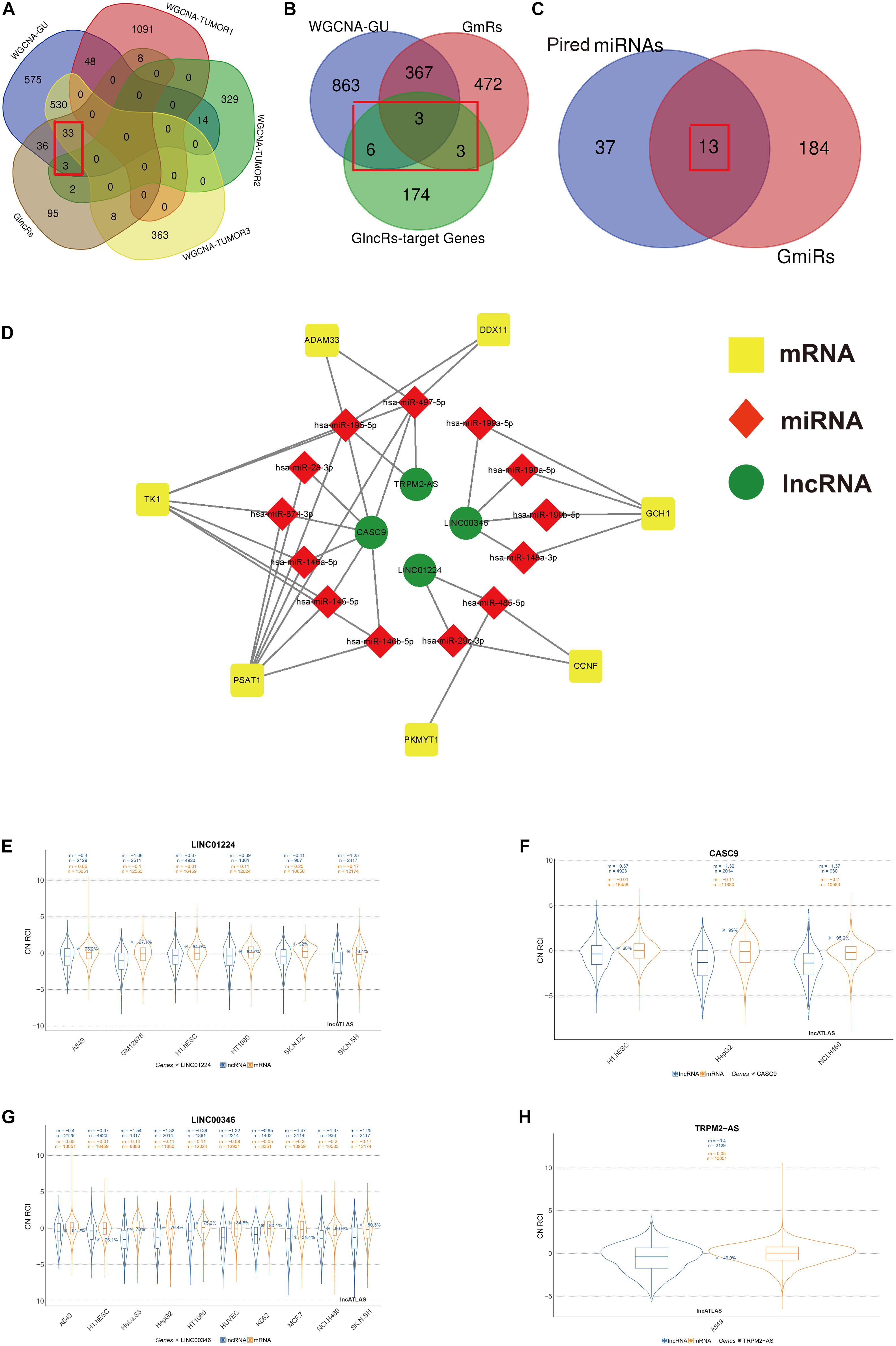
Figure 2. Screening process of the included lncRNA, mRNA, and miRNA and construction of genome instability-related ceRNA network. The Venn diagrams present the screening process of included lncRNA (A), mRNA (B), and miRNA (C) by WGCNA gene modules that are the most relevant to both genome instability and tumorigenesis and differential expression analysis between GS samples and GU samples. (D) The ceRNA network based on screened lncRNA, mRNA, and miRNA using an online database ENCORI. (E–H) The subcellular localization of LINC01224, CASC9, LINC00346, and TRPM2-AS based on the lncATLAS database. “n”, the number of total genes; “m”, the mean value of total gene expressions. Blue, mRNA. Orange, lncRNA. GU, genome unstable. GlncRs, genome instability-related lncRNAs; GmRs, genome instability-related mRNAs; GmiRs, genome instability-related miRNAs; RCI, relative concentration index; CN, cytoplasmic/nuclear.
Identification of GlncRs, GmRs, and GmiRs and ceRNA Network Construction
We used R package “limma” to extract genome instability-related genes between the GU and GS groups. In total, 185 GlncRs, 845 GmRs, and 197 GmiRs were obtained. ceRNA is a common regulatory mechanism of lncRNA. Salmena et al. (2011) first proposed the ceRNA mechanism, describing that RNA transcripts containing numerous miRNA-binding sites could competitively sponge miRNA, altering the miRNA target genes’ function (Tay et al., 2014). Because we hope to extract the genome instability-related lncRNA–miRNA–mRNA pairs precisely, a rigorous screening process is presented in Figures 2A–C. Given that WGCNA was used to extract key gene modules related to genome instability and tumorigenesis, we first intersected the module that was most related to genome instability, the top three modules that were most related to tumorigenesis, and the GlncRs to screen the crucial lncRNAs (we found 36 lncRNAs) that are related to genome instability and LUAD tumorigenesis. Then, we utilized an online database (ENCORI) to explore the potential mRNAs (GlncR target mRNAs) of these 36 lncRNAs. We excluded the GlncR target mRNAs that are neither in the module most related to genome instability nor in the GmRs. Further, only the lncRNA–mRNA pairs that are statistically significant (P < 0.05) in LUAD based on the ENCORI database (through a pan-cancer analysis of miRNA–targets and RBP (RNA-binding protein)-RNAs in 32 types of cancers) remained. The remaining 12 mRNAs were considered the crucial mRNAs that were most related to genome instability. Last, we intersected the predicted miRNAs (based on ENCORI) and the GmiRs (we found 13 miRNAs). Meanwhile, some mRNAs in the 12 mRNAs were excluded because their paired miRNAs were not included in the 13 miRNAs. Finally, we only got four lncRNAs, seven mRNAs, and 13 miRNAs. The detailed retrieved information about the 36 lncRNAs from the ENCORI database and lncRNA–miRNA–mRNA pairs before being screened by crucial mRNA and miRNA is provided in Supplementary Tables 4 and 5. Table 1 displays the ceRNA network. The modified graphical ceRNA network is presented in Figure 2D.
It is reported that lncRNAs’ unique subcellular localization was closely associated with their functions and that cytoplasmic lncRNAs could serve as ceRNA (Chen, 2016). We utilized the lncATLAS database to investigate the subcellular localization of the four lncRNAs. A549 is the specific cell line for NSCLC. Among the four included lncRNA, LINC01224 was mainly located in the cytoplasm in A549, and LINC00346 and TRPM2-AS were mainly located in the nucleus in A549. The subcellular localization of CASC9 in A549 was unclear, but mainly in the cytoplasm in other cell lines (Figures 2E–H). These results indicated that LINC01224 and CASC9 might primarily modulate genomic integrity via the ceRNA mechanism and that LINC00346 and TRPM2-AS might primarily modulate genomic integrity via direct regulation.
Genome Instability, Immune, Cancer Stemness, and Drug Sensitivity Analyses
To comprehensively understand these four lncRNAs (LINC01224, CASC9, LINC00346, and TRPM2-AS) and seven target mRNAs (CCNF, PKMYT1, GCH1, TK1, PSAT1, ADAM33, and DDX11), we performed genome instability, immune, cancer stemness, and drug sensitivity analysis.
We calculated the Spearman correlation between each of the 11 gene expressions and the TMB and MSI score in pan-cancer. Figure 3 presents the results of genome-instability analysis of included lncRNAs and target mRNAs. Surprisingly, we found that all the 11 genes’ expression was significantly associated with TMB in LUAD (P < 0.001). Most (10/11, 90.9%) genes were positively related to the TMB, and only ADAM33 was negatively related to the TMB (r = −0.18), indicating that ADAM33 plays a crucial role in maintaining genomic integrity and that other genes contribute to genome instability in LUAD. Notably, among the four lncRNAs, LINC00346 had the closest relation to TMB with a Spearman correlation exceeding 0.25. Among the seven target genes, the Spearman correlation between CCNF, PKMYT1, and TK1 reached 0.35. Besides, we found that TRPM2-AS, CCNF, PKMYT1, and DDX11 (4/11, 36.4%) were positively related to MSI score, indicating that these four genes function as key regulators in MMR mechanisms. We then calculated the correlation coefficient between every two genes by Spearman correlation to detect these genes’ interactions. The results were presented in Figure 4A. The correlation between PKMYT1 and DDX11 reached 0.61, that between PKMYT1 and CCNF reached 0.86, that between PKMYT1 and TK1 reached 0.81, and that between CCNF and TK1 reached 0.72, indicating these genes have a synergistic effect. Extensive research has shown that genome instability is one of the major causes of intratumoral heterogeneity, leading to drug resistance. The top 16 correlations between genes and drug sensitivity are shown in Figure 4B. It is shown that sensitivity of drugs that affect DNA replication and synthesis, such as 5-fluorodeoxyuridine 10mer, pyrazoloacridine, and palbociclib, was positively related to GlncRs and paired mRNA expression. These results further indicate the clinical usage of GlncRs. Furthermore, as for some protein kinase-targeted drugs, such as dasatinib and cobimetinib, genome instability-related genes were negatively related to the sensitivity. We then performed the immune analysis of these 11 genes. The results of immune subtype analysis in LUAD are shown in Figure 4C, which evaluates various immune functions of each gene related to lung cancer: wound healing (C1), IFN-γ dominant (C2), inflammatory (C3), lymphocyte depleted (C4), immunologically quiet (C5), and TGF-β dominant (C6) (Thorsson et al., 2018). PKMYT1 in C1 had the highest expression among the five subtypes, corresponding to wound healing, and this may account for the increase of expression of angiogenic genes and the high proliferation rate. All genes were significantly related to immune subtypes, indicating that genome instability-related genes play a crucial role in tumor immunity. We performed survival analysis of the 11 genes based on OS and DFS using an online bioinformatics website tool GEPIA. The results were displayed in Supplementary Figure 1. The high expression of PKMYT1, TK1, and LINC00346 was related to lower OS and DFS, indicating that these three genes could promote LUAD progression and serve as novel prognostic biomarkers. Besides, the high expression of ADAM33 was related to lower OS. To explore potential mechanisms of genome instability-related genes in modulating genome integrity and tumor immune features, we calculated the Spearman correlation between the expression of the 11 genes and genome instability and immune-related genes (PDCD1, CD274, PDCD1LG2, CTLA4, CD80, CD86, MSH2, MLH1, PMS2, MSH6, NORAD, and GUARDIN) (Figure 4E). We found that most genes (10/11, 90.9%) were positively related to MSH2 and MSH6 (P < 0.01), indicating that high expression of MMR proteins was not consistent with high genomic integrity in LUAD. Most genes were positively related to PD-L1 (CD274) (6/11, 54.5%) and PD-1 (PDCD1) (7/11, 63.6%) (P < 0.01). Furthermore, five genes (CCNF, PKMYT1, LINC00346, GCH1, and TK1) were negatively related to GUARDIN (LNCTAM334A). These findings suggested that these 11 genes modulate the GUARDIN pathway and contribute to a predicted good ICI outcome. Furthermore, we utilized the ESTIMATE and CIBERSORT databases to explore LUAD TME infiltration. As shown in Figure 4D, most genes were negatively related to stromal cell infiltration (8/11, 72.7%) and immune cell infiltration (9/11, 81.8%) and positively related to tumor purity (9/11, 81.8%). Besides, as shown in Supplementary Figure 2, some critical immune cell infiltrations that affect immunotherapy, such as CD8+ T cells and active NK cells, were positively related to these genes’ expression (10/11, 90.9% for CD8+ cells; 9/11, 81.8% for active NK cells). Moreover, M2-type macrophage infiltration, which mainly exerts protumor effects, was negatively related to most genes’ expression (10/11, 90.9%), while M1-type macrophage infiltration, which mainly exerts antitumor and proinflammatory effects, was positively related to most genes’ expression (10/11, 90.9%). These findings indicated that the 11 genes we proposed contribute to an antitumor TME infiltration and are beneficial for immunotherapy. Given that genome instability is a crucial regulator for cancer stemness, we explored the association between gene expression and cancer stemness features (including RNAss and DNAss). The results showed that most genes were positively related to cancer stemness (10/11, 90.9%) (Figure 4D), among which the correlation between CCNF, PKMYT1, TK1, and RNAss reached 0.5 (P < 0.001). Noticeably, one mRNA, ADAM33, was different from the other 10 genes in most conditions, indicating that ADAM33 involves other complex biological processes.
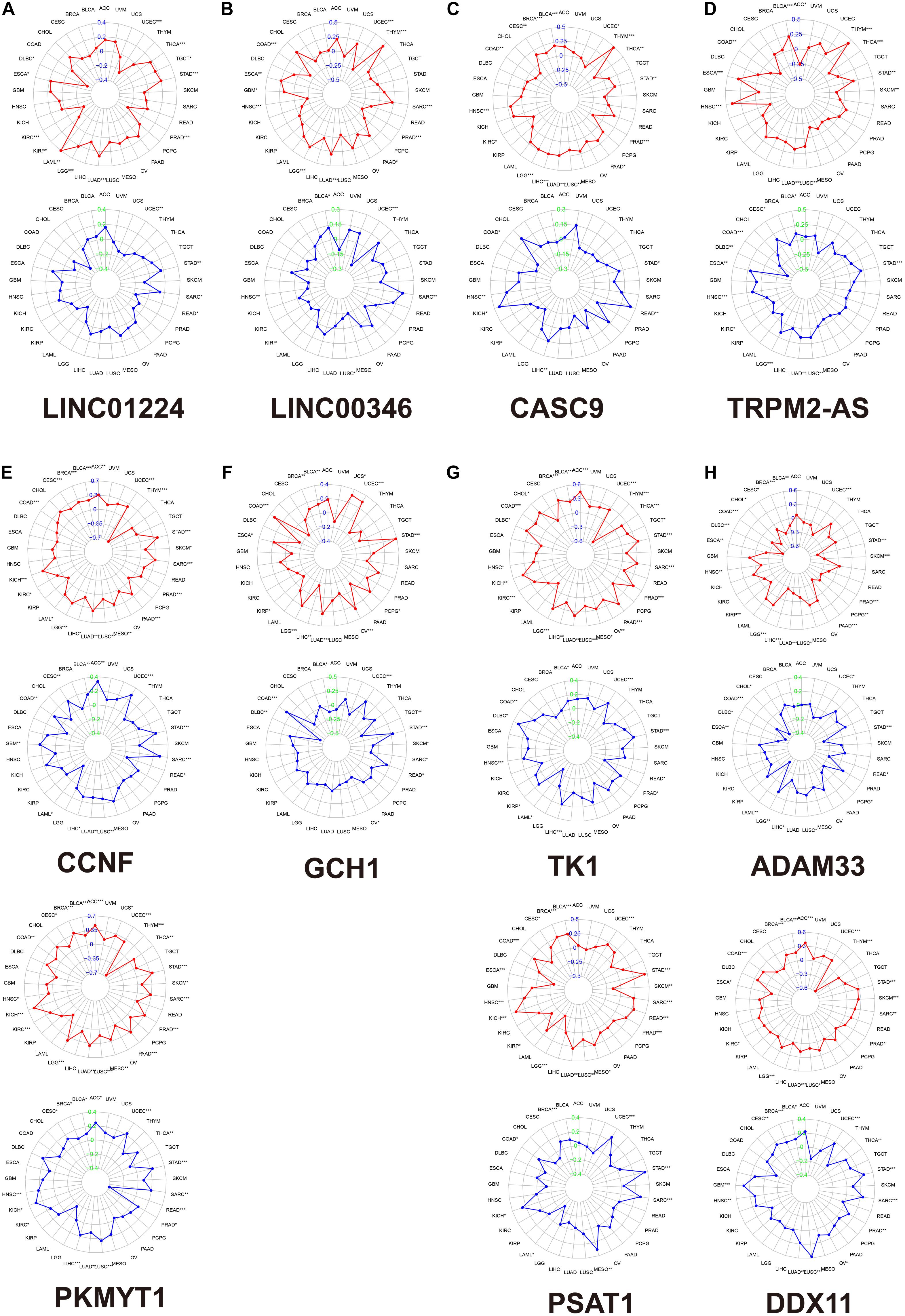
Figure 3. The association between each gene expression and TMB/MSI score based on the TCGA database by Spearman correlation. The red plot indicates TMB. The blue plot indicates MSI. (A) LINC01224. (B) LINC00346. (C) CASC9. (D) TRPM2-AS. (E) LINC01224 target mRNAs (CCNF and PKMYT1). (F) LINC00346 target mRNA (GCH1). (G) CASC9 target mRNAs (TK1 and PSAT1). (H) TRPM2-AS target mRNAs (ADAM33 and DDX11). *, P < 0.05; **, P < 0.01; ***, P < 0.001. TMB, tumor mutation burden; MSI, microsatellite instability.
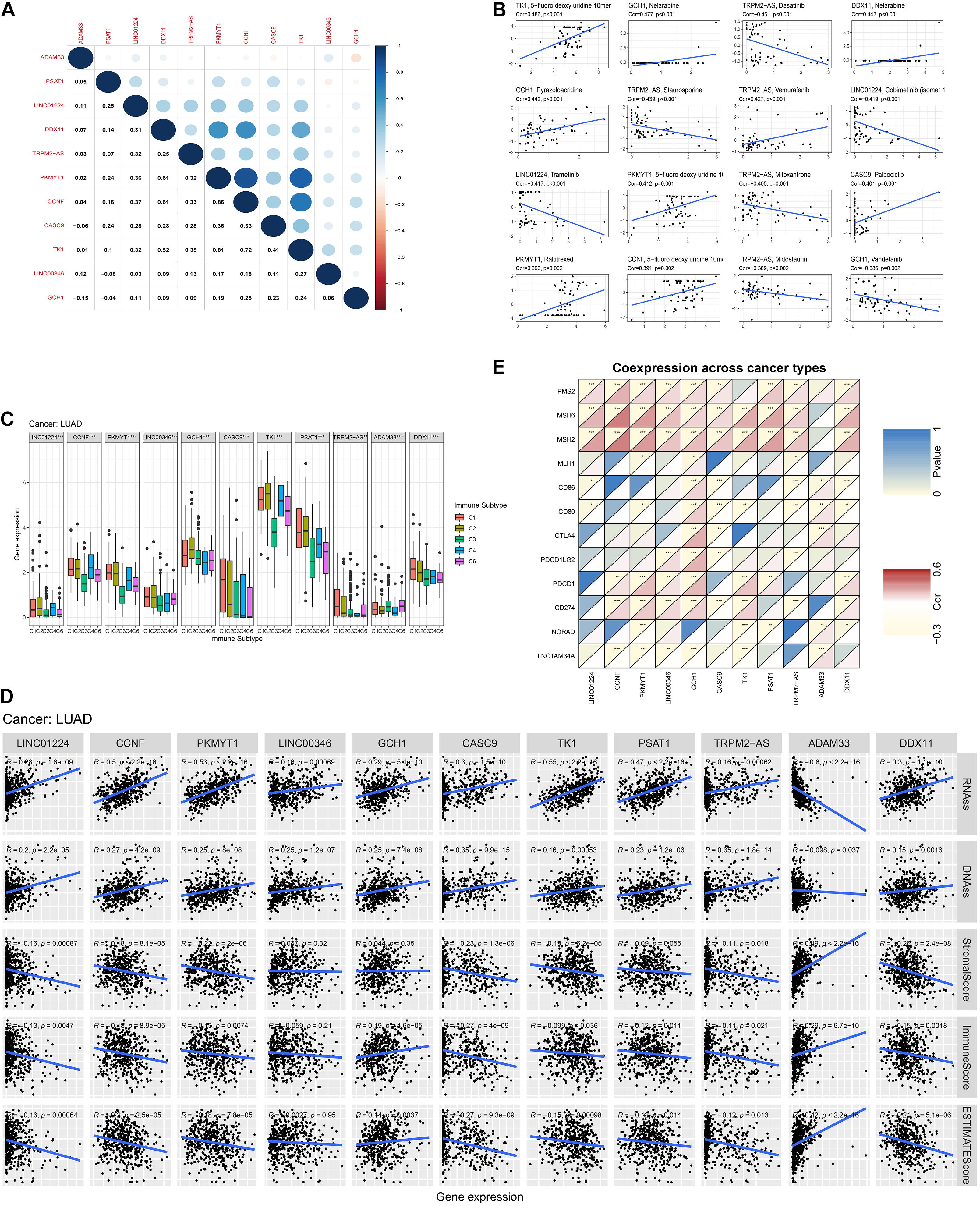
Figure 4. Pan-cancer analysis of the four lncRNAs and their seven target mRNAs. (A) The co-expression analysis between every two genes by Spearman correlation based on pan-cancer data. (B) The drug sensitivity analysis based on the NCI-60 database by Spearman correlation. (C) The relationship between each gene and immune subtype by the Kruskal–Wallis test in LUAD. (D) The co-expression analysis between each gene and four MMR genes (MLH1, MSH2, MSH6, and PMS2), six immune-checkpoint-related genes (PD-L1 (CD274), PDCD1, PDCD1LG2, CTLA4, CD80, and CD86), and two previously discovered genome instability regulatory lncRNAs (NORAD and LNCTAM34A (GUARDIN)) by Spearman analysis in LUAD. (E) The relationship between each gene and tumor stemness indexes (RNA stemness score, RNAss; DNA stemness score, DNAss) and immune cell, stromal cell, and tumor purity (ESTIMATE score) in LUAD.
Construction and Validation of the GIRlncPS
To explore the prognostic value of GlncRs in LUAD, we established a prognostic signature based on the 185 GlncRs. We randomly divided the 500 samples into a training cohort (250 patients) and a validation cohort (250 patients). The basic characteristics of the TCGA-LUAD cohort are presented in Supplementary Table 6. According to the chi-square test, there was no selection bias between the training and validation cohorts. We then performed uni- and multi-variate Cox regression in the training cohort, and the results are displayed in Table 2. The risk score was calculated as follows: risk score = sum(coefficient × corresponding lncRNA expression (log2(normalized gene expression + 1))). Hazard ratio (HR) was calculated as exp(coefficient). An eight-lncRNA GIRlncPS was acquired: 0.03 × FAM83A-AS1 + 0.05 × LINC02587 − 0.69 × MIR99AHG − 0.154 × AL07 8645.1 + 0.05 × LINC01671 + 0.03 × PLAC4 + 0.06 × LINC0 1511 + 0.09 × LINC01116. The predictive ability of our GIRlncPS in the training, validation, and whole TCGA cohorts was acceptable for lncRNA prognostic signature. Survival analysis indicated the OS was significantly longer in the low-risk group in the three cohorts (Figures 5A–C), and all the AUC reached 0.65 (Figures 5D–F). Compared with two previously published lncRNA prognostic signatures based on OS at 1, 2, and 3 years in LUAD, the GIRlncPS had the best prognostic ability (AUC = 0.726, 0.672, and 0.677, respectively) (Figures 5G–I). To explore whether the GIRlncPS had better prognostic ability than he TP53 mutation, we analyzed the TP53 mutation rate between the two groups. The TP53 mutation rate in the high-risk group was 58%, significantly higher than that in the low-risk group (41%). We classified the patients into four groups: TP53 mutated/high-risk, TP53 mutated/low-risk, TP53 wild/high-risk, TP53 wild/low-risk groups. According to the survival analysis between the four groups, GIRlncPS had better prognostic ability than TP53 (Figure 5K). Regardless of whether the TP53 was mutated, the high-risk groups had a significantly lower OS. Independent analysis with clinical features by uni- and multi-variate Cox regression indicated older age, higher stage, T, and N, which were significant risk factors based on OS in the TCGA cohort, and the low-risk group was a significant protective factor (Supplementary Figures 3A,B). To provide a clinical reference for clinicians, a nomogram based on OS in the TCGA cohort was presented in Supplementary Figure 3C. The calibration curve indicated that the nomogram could perform well in predicting LUAD patients’ OS, especially at 2 and 3 years (Supplementary Figure 3D).
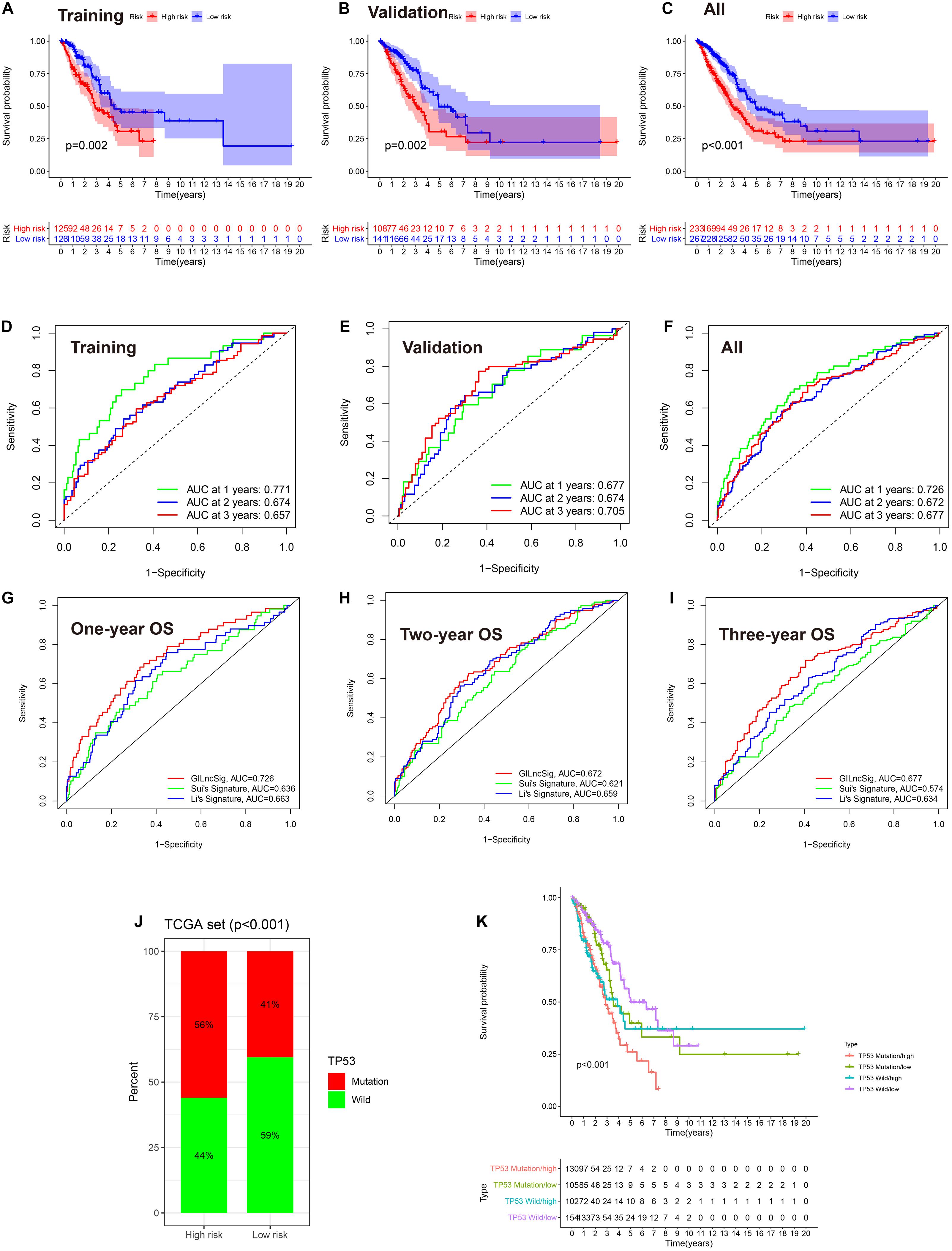
Figure 5. The eight-lncRNA prognostic signature based on the TCGA-LUAD cohort. (A–C) Survival analysis between the high- and low-risk groups based on OS. (D–F) ROC curve of the signature model based on OS at 1, 2, and 3 years. AUC, area under the curve. (G–I) Model comparison with two previously published lncRNA prognostic signatures by the ROC curve based on OS in the TCGA cohort at 1, 2, and 3 years. (J) The comparison of the mutation status of TP53 between the high- and low-risk groups. (K) Survival analysis between TP53 mutated/high-risk, TP53 mutated/low-risk, TP53 wild/high-risk, and TP53 wild/low-risk groups by the Kaplan–Meier curve. (A,D) training cohort; (B,E) validation cohort; (C,F), the entire TCGA cohort.
We also performed the genome instability and immune analyses in the high- and low-risk groups. As shown in Figures 6A–C, both SMC and TMB were significantly higher in the high-risk group, while there was no difference between the MSI score of the two groups. The patients in the high-risk group had a higher PD-L1 (CD274) expression and POLE mutation rate but not CTLA4 expression (Figures 6D–F). Immune cell infiltration and immune function analysis indicated that most immune cell types, such as T helper cells, mast cells, and B cells, were significantly higher in the low-risk group. But some essential immune cells participating in immune checkpoint mechanisms, such as CD8+ cells and Th cells, were even higher in high-risk groups. Similarly, in immune function analysis, although some innate immune processes, such as type II IFN response, were lower in the high-risk group, cytolytic activity and antigen presentation process such as MHC class I were higher in the high-risk groups (Figures 6G,H). These results indicated that the high-risk group probably had a higher response rate to PD-1/PD-L1 inhibitors, and several existing biomarkers (TMB, PD-L1 expression, POLE mutation rate, and CD8+ cell infiltration) proved this point.
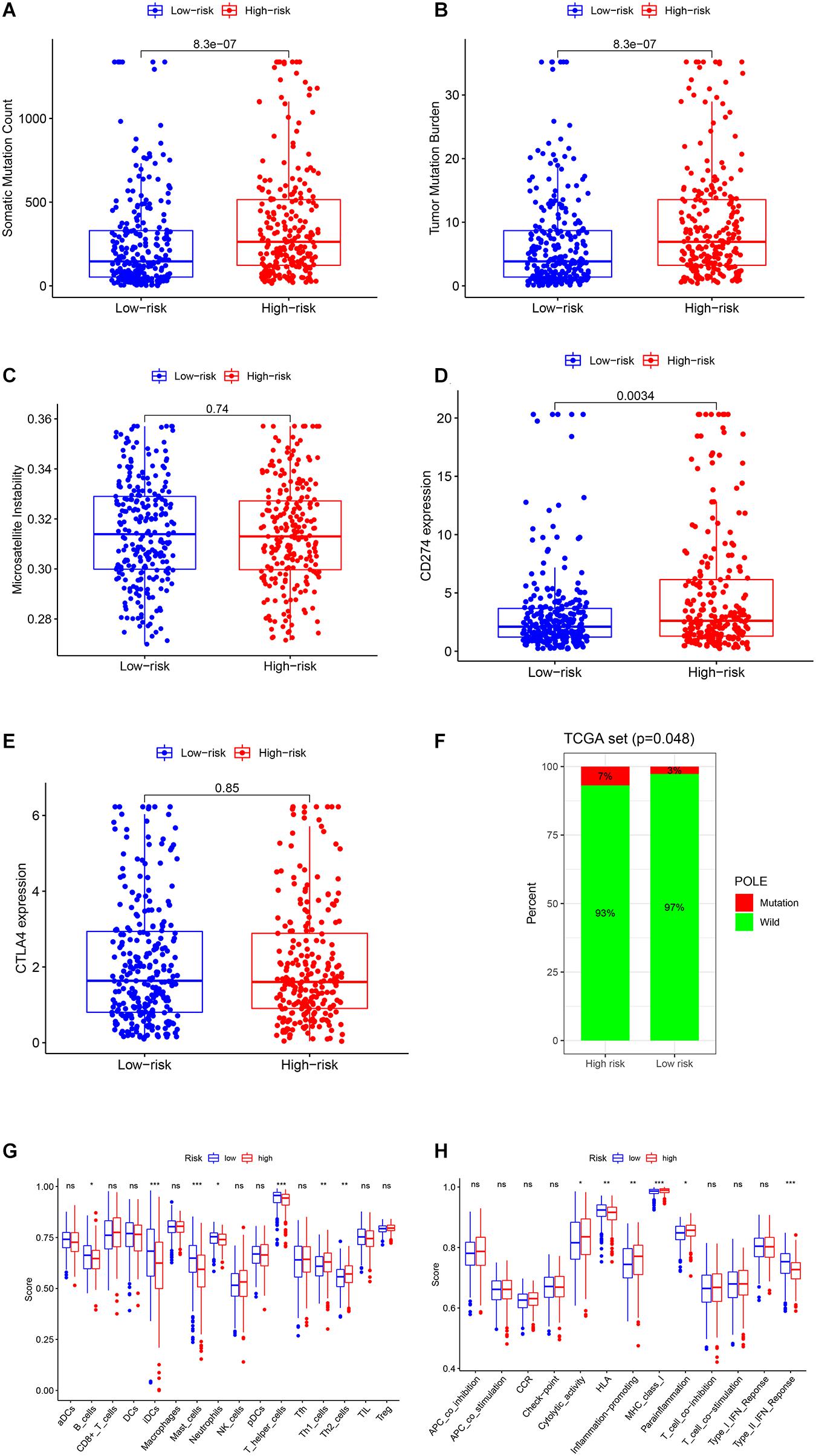
Figure 6. Genome instability and immune analysis of high- and low-risk groups. The comparison of the SMC (A), TMB (B), MSI (C), the expression and mutation status of CD274 (PD-L1) (D), the expression of CTLA4 (E), and the mutation status of POLE (F) between the high- and low-risk groups. The comparison of the immune cell infiltration (G) and immune functions (H) of the two groups by ssGSEA. *, P < 0.05; **, P < 0.01; ***, P < 0.001.
Discussion
Immunotherapy has achieved a gratifying breakthrough in lung cancer, especially NSCLC (Sun et al., 2020). Predominantly, ICIs aroused interest and led to a remarkable improvement of DFS and OS. Monoclonal antibodies targeting the CTLA-4 pathway (ipilimumab) (Rittmeyer et al., 2017), PD-1 (nivolumab and pembrolizumab) (Tanvetyanon et al., 2016), and PD-L1 (durvalumab, atezolizumab, and avelumab) (Zhou et al., 2016) have achieved promising improvements in second-line therapy for advanced lung cancers. ICIs enhance the intrinsic immune response against tumor antigens by eliminating the brake on T-cell activation by antigen-presenting cells. Despite substantive progress in lung carcinoma immunotherapy, the objective response rate in LUAD patients remains unsatisfying. The combination of standard immunotherapy and neoadjuvant therapy promoting immune system antitumor effects is an alluring strategy (Li F. et al., 2020). Tumor initiation and progression depend on the genomic alterations, involving the generation of new peptide sequences and taking the shape of neoantigens (Mardis, 2019). Genome instability explains tumor heterogeneity, offering critical regulation of cancer pathways, driving phenotypic variation, and impacting epigenetics modification (Burrell et al., 2013). Hence, we look forward to selecting genome instability-related biomarkers and treatment targets to shed light on LUAD immunotherapy.
lncRNA has been reported to play a direct role in regulating genome instability. NORAD was the first reported lncRNA maintaining genomic integrity via sequestering PUMILIO proteins (Lee et al., 2016). Munschauer et al. (2018) proved that NORAD controls RNA-binding motif protein X-linked (RBMX) to assemble a ribonucleoprotein complex (NORAD-activated ribonucleoprotein complex 1), mainly including suppressors of genome instability topoisomerase 1, necessary for the assembly of topoisomerase complex NARC1 (Munschauer et al., 2018; Elguindy et al., 2019). Hu et al. found that a p53-responsive lncRNA GUARDIN could maintain genomic integrity via the ceRNA mechanism. Therefore, we suppose a ceRNA network regulating genome instability in LUAD and GlncRs are promising immunotherapy biomarkers and treatment targets. We extracted hub-lncRNAs through WGCNA and differential expression analysis. Among the four lncRNAs (LINC01224, LINC00346, TRPM2-AS, and CASC9), LINC01224 is primarily located in the cytoplasm, and LINC00346 and TRPM2-AS are primarily located in the nucleus (the localization of CASC9 in a lung cancer cell line is unknown). lncRNA in the cytoplasm functions as ceRNA sponging miRNA and upregulates the target mRNA (Tay et al., 2014). The target mRNAs of LINC01224 were CCNF and PKMYT1. CCNF belongs to the F-box protein family, which participates in the Skp1-Cul1-F-box protein (SCF) ubiquitin ligase complexes, serving as substrate recognition subunits. D’Angiolella et al. reported that CCNF-mediated degradation of ribonucleotide reductase family member 2 (RRM2) plays a crucial role in maintaining the balance of dNTP (which is essential for DNA synthesis and repair) levels. The alteration of dNTP levels leads to genome instability and a hypermutator phenotype (D’Angiolella et al., 2013). Besides, CCNF regulates the CP110 level, a centrosomal protein promoting centrosome duplication localized in the cytoplasm. Another target gene of LINC01224, PKMYT1, a “forgotten” member of the WEE kinase family, has similar functions to WEE1, regulating the G2–M checkpoint via cyclin-dependent kinase 1 (CDK1) phosphorylation. Notably, unlike WEE1, PKMYT1 is predominantly localized in the cytoplasm and associates with the Golgi apparatus and endoplasmic reticulum through a membrane tether, where PKMYT1 modulates CDK1 by sequestering it in the cytoplasm (Asquith et al., 2020). Therefore, it is possible to hypothesize that LINC01224 regulates genome instability through the CCNF/RRM2 axis and PKMYT1/CDK1 axis via the ceRNA mechanism. Further studies are needed to prove this point. In the nucleus, lncRNA participates in the constituents of complexes and has to do with epigenetic modulation. Although LINC00346 and TRPM2-AS are predominantly localized in the nucleus, there is still a part of them that is localized in the cytoplasm, modulating genome instability via the ceRNA mechanism. In this study, we did not explore how lncRNA directly affects genome instability. In summary, we constructed a ceRNA regulatory network for genome instability in LUAD and proposed four hub-lncRNAs and seven target genes (CCNF, PKMYT1, GCH1, TK1, PSAT1, ADAM33, and DDX11) as potential immunotherapy biomarkers and neoadjuvant therapy treatment targets, among which LINC01224-CCNF and LINC01224-PKMYT1 were the most promising axes.
We proved that the hub-lncRNAs and target mRNAs were involved in TME modulation. Immune subtypes C1–C6 mark diverse immune functions: wound healing (C1), IFN-γ dominant (C2), inflammatory (C3), lymphocyte depleted (C4), immunologically quiet (C5), and TGF-β dominant (C6) (Venteicher et al., 2017; Thorsson et al., 2018). CCNF had the highest expression in C4 within all six subtypes, suggesting that it was sensitive to a temporary shutdown of the naïve lymphocyte recirculation process. CCNF remains for further research to indicate the increase of lymphocyte numbers in responding to lymphoid organ locally or immunosuppression due to the depletion of recirculation lymphocytes systemically (Shiow et al., 2006). PKMYT1, GCH1, and TK1 presented the highest expression in C2—IFN-γ dominant. They were biomarkers measuring inflammation. Notably, GCH1 and TK1 had relatively high immunophenotype expressions among all the hub-lncRNAs and target mRNAs, although they seem less valuable in other analyses. The results suggested that GCH1 and TK1 expressions can be used as specific indicators of impaired immune functions. Furthermore, we explored LUAD TME infiltration. Although most genes were negatively associated with immune cell infiltration, some critical immune cells that affect immunotherapy, such as CD8+ T cells and active NK cells, were positively related to these genes’ expression. Besides, most genes (6/11, 54.5%) were positively associated with PD-L1. These findings indicated that the 11 genes we proposed contribute to an antitumor TME infiltration, leading to tumor immune escape. To conclude, these 11 genes could be novel immunotherapy biomarkers on the aspects of LUAD genome instability and immune features.
Genome instability is associated with DNA replication, the most vulnerable cellular process, and is often accompanied by increased tumor heterogeneity. Severe DNA damage leads to replication stress, which is a feature of pre-cancer and cancer cells and provides a source of genome instability. Tumors are a patchwork of cells with diverse capacities of self-renewal, tumorigenicity, and differentiation potential with hierarchical organization, and intratumor heterogeneity offers the fuel. Cancer stem cell (CSC) plays a driver role in intratumor heterogeneity (Kreso and Dick, 2014). High cancer stemness is usually associated with an increased mutation load and provides a strong hint of a “cold” immune microenvironment, resulting in immune suppression (Miranda et al., 2019). The clinical value of CSC is intriguing, while the sensitivity of current biomarkers used for monitoring CSCs, including CD133, CD44, and aldehyde dehydrogenase (ALDH), is not guaranteed in LUAD (Skvortsov et al., 2018). In this study, we found that most genes were positively related to cancer stemness (10/11, 90.9%), among which the correlation between CCNF, PKMYT1, TK1, and RNAss reached 0.5 (P < 0.001), which is consistent with previous literature, and these genes could be cancer stemness and tumor heterogeneity biomarkers. Besides, only ADAM33 was negatively related to cancer stemness, still needing further research. Tumor heterogeneity is a significant cause of drug resistance, caused by the expansion of specific drug-tolerant subclonal populations or the evolution of novel drug-tolerant cells under selective therapeutic pressure (Dagogo-Jack and Shaw, 2018). Our drug sensitivity analysis is consistent with this hypothesis. We found that some protein kinase-targeted drugs, such as dasatinib and cobimetinib, and genome instability-related genes were negatively related to sensitivity, which the high tumor heterogeneity could explain. In contrast, the sensitivity of drugs that affect DNA replication and synthesis, such as 5-fluorodeoxyuridine 10mer, pyrazoloacridine, and palbociclib, was positively related to these 11 genes. These findings provide a new perspective for genomic instability and emphasize the clinical significance of genomic instability in tumor treatment.
There are still some limitations in this study. First, we did not explore how lncRNA directly affects genome instability. Second, the potential mechanisms underlying our findings still need biological validation through in vitro and in vivo experiments. Third, due to the diversity of lncRNA sequencing techniques, we did not find an appropriate testing cohort independent of the TCGA cohort to validate our clustering and signature further. Nevertheless, we tried our best to minimize the selection bias, including random division and the chi-square test.
To conclude, we performed an integrative multi-omics analysis to explore the mechanisms and clinical value of genome instability-related lncRNA in LUAD. We discovered that sponging miRNA, genome instability-related lncRNA functions as ceRNA, modulating genomic integrity. This research provides clinical references for LUAD immunotherapy and prognosis and interprets a potential genome instability-related ceRNA regulatory network in which LINC01224-miR-485-5p/miR-29c-3p-CCNF-RRM2 and LINC01224-miR485-5p-PKMYT1-CDK1 axes were the most promising pathways.
Data Availability Statement
The datasets presented in this study can be found in online repositories. The names of the repository/repositories and accession number(s) can be found in the article/Supplementary Material.
Author Contributions
ZW and ZR performed the data analysis and wrote the first draft. RL, JG, and YX revised the manuscript and carried out data collection. JG and GZ revised the manuscript and drafted the tables. YQ designed and supervised the study. All authors contributed to the article and approved the submitted version.
Funding
This work was supported by grants from the Major Scientific and Technological Innovation Project of Shandong Province (Grant No. 2018CXGC1212), the CSCO-Qilu Cancer Research Fund (Grant No. Y-Q201802-014), the Medical and Health Technology Innovation Plan of Jinan City (Grant No. 201805002), and the National Natural Science Foundation of China (Grant No. 81372333).
Conflict of Interest
The authors declare that the research was conducted in the absence of any commercial or financial relationships that could be construed as a potential conflict of interest.
Supplementary Material
The Supplementary Material for this article can be found online at: https://www.frontiersin.org/articles/10.3389/fcell.2021.691540/full#supplementary-material
Footnotes
- ^ https://www.ncbi.nlm.nih.gov/assembly/GCF_000001405.39/
- ^ http://lncatlas.crg.eu/
- ^ https://xenabrowser.net/datapages/
- ^ http://gepia.cancer-pku.cn/about.html
- ^ https://discover.nci.nih.gov/cellminer/
- ^ http://bioinformatics.mdanderson.org/estimate/
References
Asquith, C. R. M., Laitinen, T., and East, M. P. (2020). PKMYT1: a forgotten member of the WEE1 family. Nat. Rev. Drug Discov. 19:157. doi: 10.1038/d41573-019-00202-9
Burrell, R. A., McGranahan, N., Bartek, J., and Swanton, C. (2013). The causes and consequences of genetic heterogeneity in cancer evolution. Nature 501, 338–345. doi: 10.1038/nature12625
Carbone, D. P., Reck, M., Paz-Ares, L., Creelan, B., Horn, L., Steins, M., et al. (2017). First-line nivolumab in stage IV or recurrent non-small-cell lung cancer. N. Engl. J. Med. 376, 2415–2426. doi: 10.1056/NEJMoa1613493
Cerami, E., Gao, J., Dogrusoz, U., Gross, B. E., Sumer, S. O., Aksoy, B. A., et al. (2012). The cBio cancer genomics portal: an open platform for exploring multidimensional cancer genomics data. Cancer Discov. 2, 401–404. doi: 10.1158/2159-8290.Cd-12-0095
Chen, L.-L. (2016). Linking long noncoding RNA localization and function. Trends Biochem. Sci. 41, 761–772. doi: 10.1016/j.tibs.2016.07.003
D’Angiolella, V., Esencay, M., and Pagano, M. (2013). A cyclin without cyclin-dependent kinases: cyclin F controls genome stability through ubiquitin-mediated proteolysis. Trends Cell Biol. 23, 135–140. doi: 10.1016/j.tcb.2012.10.011
Dagogo-Jack, I., and Shaw, A. T. (2018). Tumour heterogeneity and resistance to cancer therapies. Nat. Rev. Clin. Oncol. 15, 81–94. doi: 10.1038/nrclinonc.2017.166
Elguindy, M. M., Kopp, F., Goodarzi, M., Rehfeld, F., Thomas, A., Chang, T. C., et al. (2019). PUMILIO, but not RBMX, binding is required for regulation of genomic stability by noncoding RNA NORAD. Elife 8:e48625. doi: 10.7554/eLife.48625
Frost, N., Kollmeier, J., Misch, D., Vollbrecht, C., Grah, C., Matthes, B., et al. (2021). Pembrolizumab as first-line palliative therapy in PD-L1 overexpressing (= 50%) NSCLC: real-world results with special focus on PS = 2, brain metastases, and steroids. Clin. Lung Cancer. doi: 10.1016/j.cllc.2021.02.001 [Epub ahead of print].
Hu, W. L., Jin, L., Xu, A., Wang, Y. F., Thorne, R. F., Zhang, X. D., et al. (2018). GUARDIN is a p53-responsive long non-coding RNA that is essential for genomic stability. Nat. Cell Biol. 20, 492–502. doi: 10.1038/s41556-018-0066-7
Kreso, A., and Dick, J. E. (2014). Evolution of the cancer stem cell model. Cell Stem Cell 14, 275–291. doi: 10.1016/j.stem.2014.02.006
Lee, J. J., Park, S., Park, H., Kim, S., Lee, J., Lee, J., et al. (2019). Tracing oncogene rearrangements in the mutational history of lung adenocarcinoma. Cell 177, 1842–1857.e21. doi: 10.1016/j.cell.2019.05.013
Lee, S., Kopp, F., Chang, T. C., Sataluri, A., Chen, B., Sivakumar, S., et al. (2016). Noncoding RNA NORAD regulates genomic stability by sequestering PUMILIO proteins. Cell 164, 69–80. doi: 10.1016/j.cell.2015.12.017
Li, F., Huang, Q., Luster, T. A., Hu, H., Zhang, H., Ng, W. L., et al. (2020). In vivo epigenetic CRISPR screen identifies Asf1a as an immunotherapeutic target in Kras-mutant lung adenocarcinoma. Cancer Discov. 10, 270–287. doi: 10.1158/2159-8290.Cd-19-0780
Li, J., Ge, J., Yang, Y., Liu, B., Zheng, M., and Shi, R. (2020). Long noncoding RNA ZFPM2-AS1 is involved in lung adenocarcinoma via miR-511-3p/AFF4 pathway. J. Cell. Biochem. 121, 2534–2542. doi: 10.1002/jcb.29476
Li, J.-H., Liu, S., Zhou, H., Qu, L.-H., and Yang, J.-H. (2013). starBase v2.0: decoding miRNA-ceRNA, miRNA-ncRNA and protein–RNA interaction networks from large-scale CLIP-Seq data. Nucleic Acids Res. 42, D92–D97. doi: 10.1093/nar/gkt1248
Liu, L., Bai, X., Wang, J., Tang, X. R., Wu, D. H., Du, S. S., et al. (2019). Combination of TMB and CNA stratifies prognostic and predictive responses to immunotherapy across metastatic cancer. Clin. Cancer Res. 25, 7413–7423. doi: 10.1158/1078-0432.Ccr-19-0558
Malta, T. M., Sokolov, A., Gentles, A. J., Burzykowski, T., Poisson, L., Weinstein, J. N., et al. (2018). Machine learning identifies stemness features associated with oncogenic dedifferentiation. Cell 173, 338–354.e15.
Mardis, E. R. (2019). Neoantigens and genome instability: impact on immunogenomic phenotypes and immunotherapy response. Genome Med. 11:71. doi: 10.1186/s13073-019-0684-0
Miranda, A., Hamilton, P. T., Zhang, A. W., Pattnaik, S., Becht, E., Mezheyeuski, A., et al. (2019). Cancer stemness, intratumoral heterogeneity, and immune response across cancers. Proc. Natl. Acad. Sci. U.S.A. 116, 9020–9029. doi: 10.1073/pnas.1818210116
Mok, T. S. K., Wu, Y. L., Kudaba, I., Kowalski, D. M., Cho, B. C., Turna, H. Z., et al. (2019). Pembrolizumab versus chemotherapy for previously untreated, PD-L1-expressing, locally advanced or metastatic non-small-cell lung cancer (KEYNOTE-042): a randomised, open-label, controlled, phase 3 trial. Lancet 393, 1819–1830. doi: 10.1016/s0140-6736(18)32409-7
Morel, A. P., Ginestier, C., Pommier, R. M., Cabaud, O., Ruiz, E., Wicinski, J., et al. (2017). A stemness-related ZEB1-MSRB3 axis governs cellular pliancy and breast cancer genome stability. Nat. Med. 23, 568–578. doi: 10.1038/nm.4323
Munschauer, M., Nguyen, C. T., Sirokman, K., Hartigan, C. R., Hogstrom, L., Engreitz, J. M., et al. (2018). The NORAD lncRNA assembles a topoisomerase complex critical for genome stability. Nature 561, 132–136. doi: 10.1038/s41586-018-0453-z
Niu, Y., Lin, A., Luo, P., Zhu, W., Wei, T., Tang, R., et al. (2020). Prognosis of lung adenocarcinoma patients with NTRK3 mutations to immune checkpoint inhibitors. Front. Pharmacol. 11:1213. doi: 10.3389/fphar.2020.01213
Overman, M. J., McDermott, R., Leach, J. L., Lonardi, S., Lenz, H. J., Morse, M. A., et al. (2017). Nivolumab in patients with metastatic DNA mismatch repair-deficient or microsatellite instability-high colorectal cancer (CheckMate 142): an open-label, multicentre, phase 2 study. Lancet Oncol. 18, 1182–1191. doi: 10.1016/s1470-2045(17)30422-9
Peyraud, F., and Italiano, A. (2020). Combined PARP inhibition and immune checkpoint therapy in solid tumors. Cancers 12:1502. doi: 10.3390/cancers12061502
Pros, E., Saigi, M., Alameda, D., Gomez-Mariano, G., Martinez-Delgado, B., Alburquerque-Bejar, J. J., et al. (2020). Genome-wide profiling of non-smoking-related lung cancer cells reveals common RB1 rearrangements associated with histopathologic transformation in EGFR-mutant tumors. Ann. Oncol. 31, 274–282. doi: 10.1016/j.annonc.2019.09.001
R Core Team (2013). R: A Language and Environment for Statistical Computing. Vienna: R Foundation for Statistical Computing.
Rittmeyer, A., Barlesi, F., Waterkamp, D., Park, K., Ciardiello, F., von Pawel, J., et al. (2017). Atezolizumab versus docetaxel in patients with previously treated non-small-cell lung cancer (OAK): a phase 3, open-label, multicentre randomised controlled trial. Lancet 389, 255–265. doi: 10.1016/s0140-6736(16)32517-x
Rizvi, H., Sanchez-Vega, F., La, K., Chatila, W., Jonsson, P., Halpenny, D., et al. (2018). Molecular determinants of response to anti-programmed cell death (PD)-1 and anti-programmed death-ligand 1 (PD-L1) blockade in patients with non-small-cell lung cancer profiled with targeted next-generation sequencing. J. Clin. Oncol. 36, 633–641. doi: 10.1200/jco.2017.75.3384
Salmena, L., Poliseno, L., Tay, Y., Kats, L., and Pandolfi, P. P. (2011). A ceRNA hypothesis: the Rosetta stone of a hidden RNA language? Cell 146, 353–358. doi: 10.1016/j.cell.2011.07.014
Sansregret, L., Vanhaesebroeck, B., and Swanton, C. (2018). Determinants and clinical implications of chromosomal instability in cancer. Nat. Rev. Clin. Oncol. 15, 139–150. doi: 10.1038/nrclinonc.2017.198
Shannon, P., Markiel, A., Ozier, O., Baliga, N. S., Wang, J. T., Ramage, D., et al. (2003). Cytoscape: a software environment for integrated models of biomolecular interaction networks. Genome Res. 13, 2498–2504. doi: 10.1101/gr.1239303
Shiow, L. R., Rosen, D. B., Brdicková, N., Xu, Y., An, J., Lanier, L. L., et al. (2006). CD69 acts downstream of interferon-alpha/beta to inhibit S1P1 and lymphocyte egress from lymphoid organs. Nature 440, 540–544. doi: 10.1038/nature04606
Skvortsov, S., Skvortsova, I. I., Tang, D. G., and Dubrovska, A. (2018). Concise review: prostate cancer stem cells: current understanding. Stem Cells 36, 1457–1474. doi: 10.1002/stem.2859
Sun, J., Zhang, Z., Bao, S., Yan, C., Hou, P., Wu, N., et al. (2020). Identification of tumor immune infiltration-associated lncRNAs for improving prognosis and immunotherapy response of patients with non-small cell lung cancer. J. Immunother. Cancer 8:e000110. doi: 10.1136/jitc-2019-000110
Takamochi, K., Takahashi, F., Suehara, Y., Sato, E., Kohsaka, S., Hayashi, T., et al. (2017). DNA mismatch repair deficiency in surgically resected lung adenocarcinoma: microsatellite instability analysis using the Promega panel. Lung Cancer 110, 26–31. doi: 10.1016/j.lungcan.2017.05.016
Tanvetyanon, T., Creelan, B. C., and Antonia, S. J. (2016). The safety and efficacy of nivolumab in advanced (metastatic) non-small cell lung cancer. Expert Rev. Anticancer Ther. 16, 903–910. doi: 10.1080/14737140.2016.1220836
Tay, Y., Rinn, J., and Pandolfi, P. P. (2014). The multilayered complexity of ceRNA crosstalk and competition. Nature 505, 344–352. doi: 10.1038/nature12986
Thorsson, V., Gibbs, D. L., Brown, S. D., Wolf, D., Bortone, D. S., Ou Yang, T. H., et al. (2018). The immune landscape of cancer. Immunity 48, 812–830.e14. doi: 10.1016/j.immuni.2018.03.023
Tubbs, A., and Nussenzweig, A. (2017). Endogenous DNA damage as a source of genomic instability in cancer. Cell 168, 644–656. doi: 10.1016/j.cell.2017.01.002
Venteicher, A. S., Tirosh, I., Hebert, C., Yizhak, K., Neftel, C., Filbin, M. G., et al. (2017). Decoupling genetics, lineages, and microenvironment in IDH-mutant gliomas by single-cell RNA-seq. Science 355:eaai8478. doi: 10.1126/science.aai8478
Ventura, A. (2016). NORAD: defender of the genome. Trends Genet. 32, 390–392. doi: 10.1016/j.tig.2016.04.002
Wang, F., Zhao, Q., Wang, Y. N., Jin, Y., He, M. M., Liu, Z. X., et al. (2019). Evaluation of POLE and POLD1 mutations as biomarkers for immunotherapy outcomes across multiple cancer types. JAMA Oncol. 5, 1504–1506. doi: 10.1001/jamaoncol.2019.2963
Wang, L., Liang, Y., Mao, Q., Xia, W., Chen, B., Shen, H., et al. (2019). Circular RNA circCRIM1 inhibits invasion and metastasis in lung adenocarcinoma through the microRNA (miR)-182/miR-93-leukemia inhibitory factor receptor pathway. Cancer Sci. 110, 2960–2972. doi: 10.1111/cas.14131
Yoshihara, K., Shahmoradgoli, M., Martínez, E., Vegesna, R., Kim, H., Torres-Garcia, W., et al. (2013). Inferring tumour purity and stromal and immune cell admixture from expression data. Nat. Commun. 4:2612. doi: 10.1038/ncomms3612
Yu, Y., Zeng, D., Ou, Q., Liu, S., Li, A., Chen, Y., et al. (2019). Association of survival and immune-related biomarkers with immunotherapy in patients with non-small cell lung cancer: a meta-analysis and individual patient-level analysis. JAMA Netw. Open 2:e196879. doi: 10.1001/jamanetworkopen.2019.6879
Zhang, X., Klamer, B., Li, J., Fernandez, S., and Li, L. (2020). A pan-cancer study of class-3 semaphorins as therapeutic targets in cancer. BMC Med. Genomics 13(Suppl. 5):45. doi: 10.1186/s12920-020-0682-5
Zhong, Y., Wang, J., Lv, W., Xu, J., Mei, S., and Shan, A. (2019). LncRNA TTN-AS1 drives invasion and migration of lung adenocarcinoma cells via modulation of miR-4677-3p/ZEB1 axis. J. Cell. Biochem. 120, 17131–17141. doi: 10.1002/jcb.28973
Keywords: genome instability, lung adenocarcinoma, lncRNA, bioinformatics, ceRNA, prognosis
Citation: Wang Z, Ren Z, Li R, Ge J, Zhang G, Xin Y and Qu Y (2021) Multi-Omics Integrative Bioinformatics Analyses Reveal Long Non-coding RNA Modulates Genomic Integrity via Competing Endogenous RNA Mechanism and Serves as Novel Biomarkers for Overall Survival in Lung Adenocarcinoma. Front. Cell Dev. Biol. 9:691540. doi: 10.3389/fcell.2021.691540
Received: 06 April 2021; Accepted: 28 June 2021;
Published: 22 July 2021.
Edited by:
Hernandes F. Carvalho, State University of Campinas, BrazilReviewed by:
Karine Damasceno, Gonçalo Moniz Institute (IGM), BrazilApollonia Tullo, National Research Council, Italy
Copyright © 2021 Wang, Ren, Li, Ge, Zhang, Xin and Qu. This is an open-access article distributed under the terms of the Creative Commons Attribution License (CC BY). The use, distribution or reproduction in other forums is permitted, provided the original author(s) and the copyright owner(s) are credited and that the original publication in this journal is cited, in accordance with accepted academic practice. No use, distribution or reproduction is permitted which does not comply with these terms.
*Correspondence: Yiqing Qu, cXV5aXFpbmdAc2R1LmVkdS5jbg==
†These authors have contributed equally to this work and share first authorship