- Pure and Applied Sciences, University of Tsukuba, Tsukuba, Japan
The solution properties of amino acids determine the folding, aggregation, and liquid–liquid phase separation (LLPS) behaviors of proteins. Various indices of amino acids, such as solubility, hydropathy, and conformational parameter, describe the behaviors of protein folding and solubility both in vitro and in vivo. However, understanding the propensity of LLPS and aggregation is difficult due to the multiple interactions among different amino acids. Here, the solubilities of aromatic amino acids (SAs) were investigated in solution containing 20 types of amino acids as amino acid solvents. The parameters of SAs in amino acid solvents (PSASs) were varied and dependent on the type of the solvent. Specifically, Tyr and Trp had the highest positive values while Glu and Asp had the lowest. The PSAS values represent soluble and insoluble interactions, which collectively are the driving force underlying the formation of droplets and aggregates. Interestingly, the PSAS of a soluble solvent reflected the affinity between amino acids and aromatic rings, while that of an insoluble solvent reflected the affinity between amino acids and water. These findings suggest that the PSAS can distinguish amino acids that contribute to droplet and aggregate formation, and provide a deeper understanding of LLPS and aggregation of proteins.
Introduction
Phase Separation of Proteins and Amino Acid Characteristics
Liquid–liquid phase separation (LLPS) of proteins is a phenomenon is characterized by the formation of condensates consisting of homogeneously dispersed proteins with a composition different from that of the bulk material due to a stimulus or environmental change (Uversky, 2017; Boeynaems et al., 2018). Previous studies have reported that various biological reactions occur via phase separation of proteins (Yoshizawa et al., 2020). For example, droplets formed by LLPS are involved in the stress response and the regulation of transcription and translation (Hnisz et al., 2017; Riback et al., 2017; Nosella and Forman-Kay, 2021). Moreover, amyloids formed by liquid–solid phase separation are known to be involved in various diseases (Elbaum-Garfinkle, 2019; Wang and Zhang, 2019). The phase separation of proteins is also valuable for industrial applications, such as the concentration and stabilization of antibodies and enzymes for use as drugs (Kurinomaru et al., 2014; Izaki et al., 2015; Maruyama et al., 2015; Mimura et al., 2019). Furthermore, the phase separation of proteins from the dispersed and redispersed states can be controlled to some extent by small molecular additives (Kurinomaru and Shiraki, 2017; Shiraki et al., 2020).
Recently, a database was constructed to organize the relationships of LLPS with biological reactions (Li et al., 2020; Mészáros et al., 2020; Ning et al., 2020). Proteins that are prone to LLPS are characterized by intrinsically disordered regions (IDR) (Uversky, 2017; Kuechler et al., 2020). The propensity of proteins to form droplets and aggregates, which is defined as the “propensity of phase separation (PPS)” in this paper, can be predicted to some extent by the length and types of amino acids in the IDR (Liu et al., 2019; Schuster et al., 2020). In particular, charged or hydrophilic amino acids with low complexity sequences are involved in the LLPS of proteins (Chong et al., 2018; Das et al., 2020; Kuechler et al., 2020). Therefore, understanding the PPS at the amino acid level is important to elucidate the molecular mechanisms underlying the biological reactions of proteins.
Amino Acid Index
Various properties of amino acids have been investigated to reveal the nature of protein folding and aggregation (Table 1). The solubility of an amino acid (SA) is the simplest index of the affinity between an amino acid and a solvent (Fleck and Petrosyan, 2014). A study conducted in the 1930s by Dalton and McMeekin on SA in water is probably the first to propose indexing of the properties of amino acids (Amend and Helgeson, 1997). Thereafter, the concepts of hydrophobic, chaotropic, and kosmotropic were established (Relating et al., 1964; Bigelow, 1967; Collins, 1995, 1997). In the 1970s, Nozaki and Tanford (1971) measured the SA in the solution of protein denaturants, which is among the first studies of protein stability in solvents. In the solubility experiments conducted by Fauchere, the transfer free energy of each amino acid between octanol and water was measured and the hydrophobicity of the side chain of each amino acid was indexed by subtracting the transfer free energy of Gly from that of each amino acid (Fauchere et al., 1988). Since the affinity of an amino acid for a solvent is dependent on whether the amino acid is buried inside or exposed on the surface of the protein, Miller indexed the propensity of the internal or external presence of amino acid with the use of crystals structures of proteins (Miller et al., 1987). After the structure of proteins was elucidated, Chou and Fasman indexed the ability of each amino acid to form secondary structures by investigating the amino acids contained in the secondary structures of the proteins (Chou and Fasman, 1974). According to Kyte and Doolittle (1982), hydropathy is the most important index of amino acids. Hydropathy, which has been cited more than 20,000 times over the past 30 years, is a practical index calculated from SA and the position of the amino acid in the protein structure. Recently, Hirano and Kameda (2021) defined aromaphilicity as a new index to evaluate the binding affinity of amino acids to the aromatic rings of carbon materials. This index is applicable to assess the affinity of an amino acid to a particular substance.
Indices to Understand the PPS
The amino acid indices listed in Table 1 provide basic information to predict the folding and solubility of proteins (Bigelow, 1967; Dubchak et al., 1995; Bhandari et al., 2020). In addition, the degree of the IDR predicted from the amino acid sequence of a protein is also an important parameter to evaluate the PPS (Obradovic et al., 2003; Dosztányi et al., 2005; Xue et al., 2010). However, it is difficult to predict the PPS with combinations of these parameters because the most important feature of phase separation of proteins is caused by multivalent and dynamic interactions among the side chains of amino acids in an aqueous solution (Boeynaems et al., 2018; Murthy et al., 2019; Sahli et al., 2019). In order to gain a deeper understanding of the PPS, this paper proposes a new index of amino acids that reflects the interactions among amino acids from solubility experiments. For example, it is known that when Arg is present in a solvent, the solubility of solute molecules with aromatic rings increases because of the cation-π interaction between Arg in the solvent and the aromatic molecules of the solute (Arakawa et al., 2008; Hirano et al., 2010a, b; Ariki et al., 2011). In other words, it is the SA as a solute in a solvent containing Arg. Thus, the SA in an amino acid solvent is an index to predict the PPS because the interactions among amino acids influence solubility.
Subsections Relevant for the Subject
Materials and Methods
All amino acids, NaH2PO4, and Na2HPO4 were obtained from Wako Pure Chemical Industries Ltd. (Osaka, Japan). All chemicals were of reagent grade and used as received. The following procedure was used to measure solubility. To investigate the interaction between two amino acids, aromatic amino acids (AAAs) were prepared in powdered form and were respectively dissolved in 50 mM Na-phosphate buffer (pH 7.0) containing 20 different amino acids as “amino acid solvents”. Here, the maximum concentration of each amino acid solvents varied heavily because the solubility of each amino acid in aqueous solution varied. The suspension was heated at 50°C for 1 h with frequent vortexing to dissolve completely powdered AAAs and then incubated at 25°C for 14 h with frequent vortexing. Subsequently, the suspension was centrifuged at 25°C and 18,800 × g for 20 min to obtain a supernatant saturated with AAAs. After appropriate dilution of the supernatant with 50 mM Na-phosphate buffer (pH 7.0), the concentrations of the AAAs were determined by measuring the absorbance at 260 to 280 nm along with an appropriate blank using an ND-1000 spectrophotometer (NanoDrop Technologies, Wilmington, DE, United States). The solubilities were then calculated from the standard curves determined for each AAA. This method has the advantage of allowing accurate quantification of the concentrations of amino acids in mixtures (Nishinami et al., 2018; Hirano et al., 2020).
Solubility of an Amino Acid in 20 Kinds of Amino Acid Solvents
The solubilities of AAAs in 20 kinds of amino acid solvents are shown in Supplementary Figure 1A. Based on these results, the parameters of SAs in the amino acid solvents (PSASs) were calculated from a simple Equation (1),
where Δs is the change in the solubility ratio of AAA in the amino acid solvents when the solubility in water is 1 and Δc is the change in the concentration of the amino acid solvent. Briefly, the Equation (1) shows the slope of fitting line in Supplementary Figure 1A. Thus, PSAS indicates the degree to which an amino acid at a concentration of 1 M is present in the solvent solubilizes or insolubilizes the AAA. Some of the amino acids changed the solubility of AAAs at low or high concentrations. Since the concentration at which the effect of solubility changes varied among amino acids, we established the PSAS that did not consider the concentration range of amino acid solvents. A list of PSASs is provided in Figure 1A with solubilized AAAs presented as positive values (red) and insolubilized AAAs as negative values (green). Arg, Pro, and AAA solvents had positive PSAS values, indicating that the interactions between AAAs and these amino acids are favorable to soluble AAAs. However, anionic amino acid solvents had negative PSAS values, indicating that the interactions between AAAs and negatively charged amino acids are unfavorable to soluble AAAs. Unexpectedly, the solvents of Gln, Asn, and hydrophobic amino acid insolubilized AAAs, indicating that interactions between AAAs and these amino acids are favorable, but do not contribute to solubilization of AAAs. When the PSAS value was close to zero, neither soluble nor insoluble effects were at work, suggesting that the interactions among the amino acids were as weak as those with water.
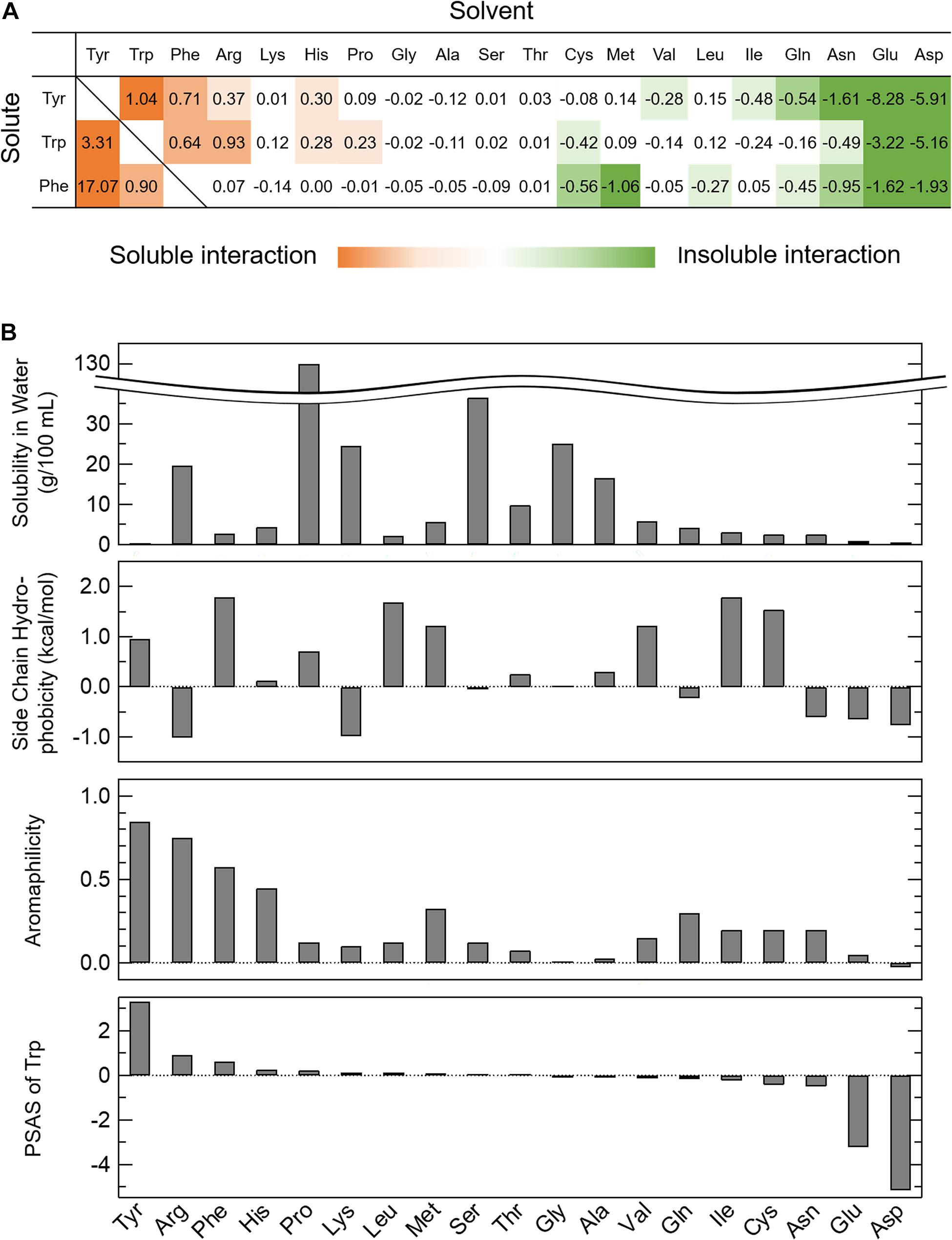
Figure 1. Parameter of solubility of AAAs in amino acid solvents (PSAS). (A) The PSAS of AAAs in 20 kinds of amino acid solvents. The PSAS was calculated from Equation (1). The PSAS is shown as a positive value when the solubility of the AAAs increases and as a negative value when it decreases. (B) Relationships of the PSAS with the various indices characterizing amino acids. The PSAS of Trp is compared to the solubility in water, side chain hydrophobicity, and aromaphilicity of 20 kinds of amino acids.
Comparison of PSAS With Existing Amino Acid Indices
To clarify the relationship between the PSAS proposed in this study and the amino acid indices proposed in previous studies, the PSAS was determined as the balance of the interactions among the amino acids and those of the amino acids and water, as determined by solubility experiments. Therefore, the PSAS was compared with some of the indices listed in Table 1 that reflect affinity for water and the interactions among amino acids and aromatic rings (solubility in water, side chain hydrophobicity, and aromaphilicity). When the PSAS was compared with these indices by row, the order of the PSAS of Trp did not match the solubility in water and side chain hydrophobicity, but it was partially consistent with the aromaphilicity (Figure 1B). The PSAS of Tyr and Phe showed a similar propensity. This result suggested that PSAS reflects the binding affinity of the side chains for aromatic rings rather than the interaction between amino acids and water. However, as the order of PSAS did not completely match the aromaphilicity, the PSAS could not be explained by a simple affinity between side chains and aromatic rings. Next, the PSAS was compared with these indices by column, which showed that the magnitude of the PSAS of the soluble solvents (Trp, Arg, His, and Pro) was in the order of Trp > Tyr > Phe, consistent with the magnitude of aromaphilicity (Figure 1A and Table 1). On the other hand, the magnitude of the PSAS of the insoluble solvents (Gln, Asn, Glu, Asp, Val, and Ile) was in the order of Phe > Trp > Tyr or Trp > Phe > Tyr, which was consistent with the magnitude of solubility in water or side chain hydrophobicity (Figure 1A and Table 1). These results suggest that the soluble interactions reflect the affinities among the amino acids and aromatic rings, while the insoluble interactions reflect the affinities of the amino acids and water. Hence, the PSAS mainly reflects the interactions among the amino acids as a soluble property and those of the amino acids and water as an insoluble property.
Properties of Gln, Asn, and Pro
Gln, Asn, and Pro are abundant in low complexity regions of proteins that undergo phase separation (Budini et al., 2012; Riback et al., 2017), although the functions of these amino acids are unclear. Gln and Asn insolubilized the AAAs, while Pro solubilized the AAAs (Figure 1A). Based on the PSAS, Gln and Asn can cause phase separation by interacting with AAAs. Molecular dynamics simulation may be able to reveal interactions among amino acids that have not received much attention so far by investigating the PSAS other than AAAs.
On the other hand, it is difficult to understand the PSAS of Pro because the Pro solvent in this experiment system may change the properties of the solution, such as viscosity and polarity, due to the extremely high solubility of Pro (Wu et al., 2001; Zhao, 2006; Ferreira et al., 2019). Therefore, the PSAS of Pro may not be simply comparable to the PSAS of other amino acids. When we investigate the solubility of 20 solute amino acids in 20 kinds of amino acid solvents, it is worthwhile to examine the difference between the solubility change due to the interaction between amino acids and that due to solvent effect.
Discussion
Phase Separation of Proteins Based on PSAS
The novel index PSAS categorizes amino acids into soluble and insoluble interactions (Figure 1A). A soluble interaction represented by the PSAS (red area in Figure 1A) indicates that an amino acid is highly soluble in an amino acid solvent. At the microscopic level, there is an attractive interaction of the solute amino acid with the solvent amino acid, meaning that the water molecules are involved in the interaction. In other words, the soluble interaction of the PSAS is considered similar to the intermolecular interactions within a droplet formed by LLPS. Actually, the cation-π and π–π interactions, which are known to be the driving forces for droplet formation (e.g., Ddx4 and hnRNPA2) (Nott et al., 2015; Vernon et al., 2018; Dignon et al., 2020), are represented by the PSAS as soluble interactions. On the other hand, the insoluble interaction represented by the PSAS (green area in Figure 1A) indicates that the solute amino acid is insoluble in the amino acid solvent. There are two possible mechanisms underlying the insoluble effect expressed by the PSAS: (i) the repulsive interactions between the solute and solvent amino acids result in precipitation of the solute amino acids because the solute and solvent amino acids are negatively charged at pH 7.0 (e.g., Glu and Asp) and (ii) water molecules are not involved in the attractive interaction between the solute and solvent amino acids, resulting in coprecipitation of the two amino acids (e.g., Gln, Asn, and hydrophobic amino acids). In particular, the insoluble interaction represented by (ii) is the same as the intermolecular interaction of aggregates formed by liquid–solid phase separation (e.g., ovalbumin, lysozyme, and α-synuclein) (Iwashita et al., 2018; Stephens and Kaminski Schierle, 2019). Actually, the hydrophobic interactions, which are known to be the driving force of aggregate formation, were represented by the PSAS as insoluble interactions. Therefore, the soluble interaction expressed by the PSAS may represent droplet formation ability and the insoluble interaction may represent aggregate formation ability.
The PPS of a protein must be considered in the interactions of many kinds of amino acids. However, the PSAS represents only the interaction between the two types of amino acids. Actually, it has been reported that hydrophobic interactions, which are considered the driving force of aggregation, stabilize droplets, as well as electrostatic and π–π interactions, which are considered the driving forces of droplet formation, stabilize aggregates (e.g., tau and IgG) (Ma et al., 2010; Matsuda et al., 2018; Krainer et al., 2021; Lin et al., 2021). Therefore, it will be difficult to completely distinguish between aggregates and droplets by only focusing on the main interactions. When distinguishing between droplet and aggregate formation abilities based on the PSAS, the properties of the assemblies must be considered from only the interaction between the two types of amino acids.
Finally, for future applications, the PSAS at the amino acid level did not fully match that at the protein level. To more clearly illustrate this finding, the PSAS of Trp was relatively large, but is rarely present in the IDR of proteins that are prone to LLPS (Wang et al., 2014; Martin and Mittag, 2018; Martin et al., 2020), while Gly and Ser had PSAS values close to zero, but are known to be abundant in the IDR of proteins that are prone to LLPS (Schuster et al., 2018; Hardenberg et al., 2021). These conflicts are thought to be due to differences in the properties of free amino acids as solutes and amino acids during peptide formation. These differences indicate the importance of the liquid–liquid or liquid–solid phase separations of proteins. In addition, several methods have been developed to predict the PPS of proteins (Vernon and Forman-Kay, 2019; Hardenberg et al., 2021; van Mierlo et al., 2021). These prediction methods can identify proteins with a high PPS from the proteome, such as the distribution of the amino acid residues and the complexity of the sequence. The PSAS is expected to be a prominent parameter for prediction methods, typically machine learning, due to the experimental interactions between the solute and solvent amino acids, including water.
Data Availability Statement
The original contributions presented in the study are included in the article/Supplementary Material, further inquiries can be directed to the corresponding author.
Author Contributions
AN performed all the experiments and wrote the manuscript. SN supervised the experiments. KS revised and edited the manuscript. All authors have read and approved the final manuscript.
Funding
This work was partly supported by the JSPS KAKENHI (grant numbers 18H02383 and 19K22377).
Conflict of Interest
The authors declare that the research was conducted in the absence of any commercial or financial relationships that could be construed as a potential conflict of interest.
Supplementary Material
The Supplementary Material for this article can be found online at: https://www.frontiersin.org/articles/10.3389/fcell.2021.691052/full#supplementary-material
References
Amend, J. P., and Helgeson, H. C. (1997). Solubilities of the common L-α-amino acids as a function of temperature and solution pH. Pure Appl. Chem. 69, 935–942. doi: 10.1351/pac199769050935
Arakawa, T., Kita, Y., and Koyama, A. H. (2008). Solubility enhancement of gluten and organic compounds by arginine. Int. J. Pharm. 355, 220–223. doi: 10.1016/j.ijpharm.2007.12.009
Ariki, R., Hirano, A., Arakawa, T., and Shiraki, K. (2011). Arginine increases the solubility of alkyl gallates through interaction with the aromatic ring. J. Biochem. 149, 389–394. doi: 10.1093/jb/mvr004
Bhandari, B. K., Gardner, P. P., and Lim, C. S. (2020). Solubility-weighted index: fast and accurate prediction of protein solubility. Bioinformatics 36, 4691–4698. doi: 10.1093/bioinformatics/btaa578
Bigelow, C. C. (1967). On the average hydrophobicity of proteins and the relation between it and protein structure. J. Theor. Biol. 16, 187–211. doi: 10.1016/0022-5193(67)90004-5
Boeynaems, S., Alberti, S., Fawzi, N. L., Mittag, T., Polymenidou, M., Rousseau, F., et al. (2018). Protein phase separation: a new phase in cell biology. Trends Cell Biol. 28, 420–435. doi: 10.1016/j.tcb.2018.02.004
Budini, M., Buratti, E., Stuani, C., Guarnaccia, C., Romano, V., De Conti, L., et al. (2012). Cellular model of TAR DNA-binding Protein 43 (TDP-43) aggregation based on its C-terminal Gln/Asn-rich region. J. Biol. Chem. 287, 7512–7525. doi: 10.1074/jbc.M111.288720
Chong, P. A., Vernon, R. M., and Forman-Kay, J. D. (2018). RGG/RG motif regions in RNA binding and phase separation. J. Mol. Biol. 430, 4650–4665. doi: 10.1016/j.jmb.2018.06.014
Chou, P. Y., and Fasman, G. D. (1974). Conformational parameters for amino acids in helical, β-Sheet, and random coil regions calculated from proteins. Biochemistry 13, 211–222. doi: 10.1021/bi00699a001
Collins, K. D. (1995). Sticky ions in biological systems. Proc. Natl. Acad. Sci. U.S.A. 92, 5553–5557. doi: 10.1073/pnas.92.12.5553
Collins, K. D. (1997). Charge density-dependent strength of hydration and biological structure. Biophys. J. 72, 65–76. doi: 10.1016/S0006-3495(97)78647-8
Das, S., Lin, Y. H., Vernon, R. M., Forman-Kay, J. D., and Chan, H. S. (2020). Comparative roles of charge, π, and hydrophobic interactions in sequence-dependent phase separation of intrinsically disordered proteins. Proc. Natl. Acad. Sci. U.S.A. 117, 28795–28805. doi: 10.1073/pnas.2008122117
Dignon, G. L., Best, R. B., and Mittal, J. (2020). Biomolecular phase separation: from molecular driving forces to macroscopic properties. Annu. Rev. Phys. Chem. 71, 53–75. doi: 10.1146/annurev-physchem-071819-113553
Dosztányi, Z., Csizmok, V., Tompa, P., and Simon, I. (2005). IUPred: web server for the prediction of intrinsically unstructured regions of proteins based on estimated energy content. Bioinformatics 21, 3433–3434. doi: 10.1093/bioinformatics/bti541
Dubchak, I., Muchnik, I., Holbrook, S. R., and Kim, S. H. (1995). Prediction of protein folding class using global description of amino acid sequence. Proc. Natl. Acad. Sci. U.S.A. 92, 8700–8704. doi: 10.1073/pnas.92.19.8700
Elbaum-Garfinkle, S. (2019). Matter over mind: liquid phase separation and neurodegeneration. J. Biol. Chem. 294, 7160–7168. doi: 10.1074/jbc.REV118.001188
Fauchere, J. -L., Charton, M., Kier, L. B., Verloop, A., and Pliska, V. (1988). Amino acid side chain parameters for correlation studies in biology and pharmacology. Int. J. Pept. Protein Res 32, 269–278. doi: 10.1111/j.1399-3011.1988.tb01261.x
Ferreira, L. A., Uversky, V. N., and Zaslavsky, B. Y. (2019). Effects of amino acids on solvent properties of water. J. Mol. Liq. 277, 123–131. doi: 10.1016/j.molliq.2018.12.071
Fleck, M., and Petrosyan, A. M. (2014). Salts of Amino Acids: Crystallization, Structure and Properties. Cham: Springer, doi: 10.1007/978-3-319-06299-0
Hardenberg, M., Horvath, A., Ambrus, V., Fuxreiter, M., and Vendruscolo, M. (2021). Widespread occurrence of the droplet state of proteins in the human proteome. Proc. Natl. Acad. Sci. U.S.A. 117, 33254–33262. doi: 10.1073/PNAS.2007670117
Hirano, A., and Kameda, T. (2021). Aromaphilicity index of amino acids: molecular dynamics simulations of the protein binding affinity for carbon nanomaterials. ACS Appl. Nano Mater. 4, 2486–2495. doi: 10.1021/acsanm.0c03047
Hirano, A., Kameda, T., Arakawa, T., and Shiraki, K. (2010a). Arginine-assisted solubilization system for drug substances: solubility experiment and simulation. J. Phys. Chem. B 114, 13455–13462. doi: 10.1021/jp101909a
Hirano, A., Nagatoishi, S., Wada, M., Tsumoto, K., Maluf, K. N., and Arakawa, T. (2020). Technical capabilities and limitations of optical spectroscopy and calorimetry using water-miscible solvents: the case of dimethyl sulfoxide, acetonitrile, and 1,4-dioxane. J. Pharm. Sci. 109, 524–531. doi: 10.1016/j.xphs.2019.10.056
Hirano, A., Tokunaga, H., Tokunaga, M., Arakawa, T., and Shiraki, K. (2010b). The solubility of nucleobases in aqueous arginine solutions. Arch. Biochem. Biophys. 497, 90–96. doi: 10.1016/j.abb.2010.03.009
Hnisz, D., Shrinivas, K., Young, R. A., Chakraborty, A. K., and Sharp, P. A. (2017). A phase separation model for transcriptional control. Cell 169, 13–23. doi: 10.1016/j.cell.2017.02.007
Iwashita, K., Handa, A., and Shiraki, K. (2018). Coacervates and coaggregates: liquid–liquid and liquid–solid phase transitions by native and unfolded protein complexes. Int. J. Biol. Macromol. 120, 10–18. doi: 10.1016/j.ijbiomac.2018.08.063
Izaki, S., Kurinomaru, T., Handa, K., Kimoto, T., and Shiraki, K. (2015). Stress tolerance of antibody-poly(amino acid) complexes for improving the stability of high concentration antibody formulations. J. Pharm. Sci. 104, 2457–2463. doi: 10.1002/jps.24515
Krainer, G., Welsh, T. J., Joseph, J. A., St George-Hyslop, P., Hyman, A. A., Collepardo-Guevara, R., et al. (2021). Reentrant liquid condensate phase of proteins is stabilized by hydrophobic and non-ionic interactions. Biophys. J. 120, 28a. doi: 10.1016/j.bpj.2020.11.426
Kuechler, E. R., Budzyñska, P. M., Bernardini, J. P., Gsponer, J., and Mayor, T. (2020). distinct features of stress granule proteins predict localization in membraneless organelles. J. Mol. Biol. 432, 2349–2368. doi: 10.1016/j.jmb.2020.02.020
Kurinomaru, T., Maruyama, T., Izaki, S., Handa, K., Kimoto, T., and Shiraki, K. (2014). Protein-poly(amino acid) complex precipitation for high-concentration protein formulation. J. Pharm. Sci. 103, 2248–2254. doi: 10.1002/jps.24025
Kurinomaru, T., and Shiraki, K. (2017). Aggregative protein–polyelectrolyte complex for high-concentration formulation of protein drugs. Int. J. Biol. Macromol. 100, 11–17. doi: 10.1016/j.ijbiomac.2016.06.016
Kyte, J., and Doolittle, R. F. (1982). A simple method for displaying the hydropathic character of a protein. J. Mol. Biol. 157, 105–132. doi: 10.1016/0022-2836(82)90515-0
Li, Q., Peng, X., Li, Y., Tang, W., Zhu, J., Huang, J., et al. (2020). LLPSDB: a database of proteins undergoing liquid-liquid phase separation in vitro. Nucleic Acids Res. 48, D320–D327. doi: 10.1093/nar/gkz778
Lin, Y., Fichou, Y., Longhini, A. P., Llanes, L. C., Yin, P., Bazan, G. C., et al. (2021). Liquid-Liquid phase separation of tau driven by hydrophobic interaction facilitates fibrillization of Tau. J. Mol. Biol. 433:166731. doi: 10.1016/j.jmb.2020.166731
Liu, Y., Wang, X., and Liu, B. (2019). A comprehensive review and comparison of existing computational methods for intrinsically disordered protein and region prediction. Brief. Bioinform. 20, 1–17. doi: 10.1093/bib/bbx126
Ma, M., Kuang, Y., Gao, Y., Zhang, Y., Gao, P., and Xu, B. (2010). Aromatic-aromatic interactions induce the self-assembly of pentapeptidic derivatives in water to form nanofibers and supramolecular hydrogels. J. Am. Chem. Soc. 132, 2719–2728. doi: 10.1021/ja9088764
Martin, E. W., Holehouse, A. S., Peran, I., Farag, M., Incicco, J. J., Bremer, A., et al. (2020). Valence and patterning of aromatic residues determine the phase behavior of prion-like domains. Science 367, 694–699. doi: 10.1126/science.aaw8653
Martin, E. W., and Mittag, T. (2018). Relationship of sequence and phase separation in protein low-complexity regions. Biochemistry 57, 2478–2487. doi: 10.1021/acs.biochem.8b00008
Maruyama, T., Izaki, S., Kurinomaru, T., Handa, K., Kimoto, T., and Shiraki, K. (2015). Protein-poly(amino acid) precipitation stabilizes a therapeutic protein L-asparaginase against physicochemical stress. J. Biosci. Bioeng. 120, 720–724. doi: 10.1016/j.jbiosc.2015.04.010
Matsuda, A., Mimura, M., Maruyama, T., Kurinomaru, T., Shiuhei, M., and Shiraki, K. (2018). Liquid droplet of protein-polyelectrolyte complex for high-concentration formulations. J. Pharm. Sci. 107, 2713–2719. doi: 10.1016/j.xphs.2018.06.021
Mészáros, B., Erdos, G., Szabó, B., Schád, É, Tantos, Á, Abukhairan, R., et al. (2020). PhaSePro: the database of proteins driving liquid-liquid phase separation. Nucleic Acids Res. 48, D360–D367. doi: 10.1093/nar/gkz848
Miller, S., Janin, J., Lesk, A. M., and Chothia, C. (1987). Interior and surface of monomeric proteins. J. Mol. Biol. 196, 641–656. doi: 10.1016/0022-2836(87)90038-6
Mimura, M., Tsumura, K., Matsuda, A., Akatsuka, N., and Shiraki, K. (2019). Effect of additives on liquid droplet of protein-polyelectrolyte complex for high-concentration formulations. J. Chem. Phys. 150, 1–11. doi: 10.1063/1.5063378
Murthy, A. C., Dignon, G. L., Kan, Y., Zerze, G. H., Parekh, S. H., Mittal, J., et al. (2019). Molecular interactions underlying liquid-liquid phase separation of the FUS low-complexity domain. Nat. Struct. Mol. Biol. 26, 637–648. doi: 10.1038/s41594-019-0250-x
Ning, W., Guo, Y., Lin, S., Mei, B., Wu, Y., Jiang, P., et al. (2020). DrLLPS: a data resource of liquid-liquid phase separation in eukaryotes. Nucleic Acids Res. 48, D288–D295. doi: 10.1093/nar/gkz1027
Nishinami, S., Yoshizawa, S., Arakawa, T., and Shiraki, K. (2018). Allantoin and hydantoin as new protein aggregation suppressors. Int. J. Biol. Macromol. 114, 497–503. doi: 10.1016/j.ijbiomac.2018.03.011
Nosella, M. L., and Forman-Kay, J. D. (2021). Phosphorylation-dependent regulation of messenger RNA transcription, processing and translation within biomolecular condensates. Curr. Opin. Cell Biol. 69, 30–40. doi: 10.1016/j.ceb.2020.12.007
Nott, T. J., Petsalaki, E., Farber, P., Jervis, D., Fussner, E., Plochowietz, A., et al. (2015). Phase transition of a disordered nuage protein generates environmentally responsive membraneless organelles. Mol. Cell 57, 936–947. doi: 10.1016/j.molcel.2015.01.013
Nozaki, Y., and Tanford, C. (1971). The solubility of amino acids and two glycine peptides in aqueous ethanol and dioxane solutions. Establishment of a hydrophobicity scale. J. Biol. Chem. 246, 2211–2217. doi: 10.1016/S0021-9258(19)77210-X
Obradovic, Z., Peng, K., Vucetic, S., Radivojac, P., Brown, C. J., and Dunker, A. K. (2003). Predicting intrinsic disorder from amino acid sequence. Proteins Struct. Funct. Genet. 53, 566–572. doi: 10.1002/prot.10532
Relating, A. L. L. A. W., Protein, O. F., To, M., and Composition, T. (1964). A limiting law relating the size and shape of protein molecules to their composition. Biochemistry 51, 1285–1291.
Riback, J. A., Katanski, C. D., Kear-Scott, J. L., Pilipenko, E. V., Rojek, A. E., Sosnick, T. R., et al. (2017). Stress-triggered phase separation is an adaptive, evolutionarily tuned response. Cell 168, 1028–1040. doi: 10.1016/j.cell.2017.02.027
Sahli, L., Renard, D., Solé-Jamault, V., Giuliani, A., and Boire, A. (2019). Role of protein conformation and weak interactions on γ-gliadin liquid-liquid phase separation. Sci. Rep. 9, 1–13. doi: 10.1038/s41598-019-49745-2
Schuster, B. S., Dignon, G. L., Tang, W. S., Kelley, F. M., Ranganath, A. K., Jahnke, C. N., et al. (2020). Identifying sequence perturbations to an intrinsically disordered protein that determine its phase-separation behavior. Proc. Natl. Acad. Sci. U.S.A. 117, 1–11. doi: 10.1073/pnas.2000223117
Schuster, B. S., Reed, E. H., Parthasarathy, R., Jahnke, C. N., Caldwell, R. M., Bermudez, J. G., et al. (2018). Controllable protein phase separation and modular recruitment to form responsive membraneless organelles. Nat. Commun. 9, 2985. doi: 10.1038/s41467-018-05403-1
Shiraki, K., Mimura, M., Nishinami, S., and Ura, T. (2020). Effect of additives on liquid droplets and aggregates of proteins. Biophys. Rev. 12, 587–592. doi: 10.1007/s12551-020-00682-9
Stephens, A. D., and Kaminski Schierle, G. S. (2019). The role of water in amyloid aggregation kinetics. Curr. Opin. Struct. Biol. 58, 115–123. doi: 10.1016/j.sbi.2019.06.001
Uversky, V. N. (2017). Intrinsically disordered proteins in overcrowded milieu: membrane-less organelles, phase separation, and intrinsic disorder. Curr. Opin. Struct. Biol. 44, 18–30. doi: 10.1016/j.sbi.2016.10.015
van Mierlo, G., Jansen, J. R. G., Wang, J., Poser, I., van Heeringen, S. J., and Vermeulen, M. (2021). Predicting protein condensate formation using machine learning. Cell Rep. 34:108705. doi: 10.1016/j.celrep.2021.108705
Vernon, R. M., and Forman-Kay, J. D. (2019). First-generation predictors of biological protein phase separation. Curr. Opin. Struct. Biol. 58, 88–96. doi: 10.1016/j.sbi.2019.05.016
Vernon, R. M. C., Chong, P. A., Tsang, B., Kim, T. H., Bah, A., Farber, P., et al. (2018). Pi-Pi contacts are an overlooked protein feature relevant to phase separation. Elife 7, 1–48. doi: 10.7554/eLife.31486
Wang, J. T., Smith, J., Chen, B. C., Schmidt, H., Rasoloson, D., Paix, A., et al. (2014). Regulation of RNA granule dynamics by phosphorylation of serine-rich, intrinsically disordered proteins in C. Elegans. Elife 3, 1–23. doi: 10.7554/eLife.04591
Wang, Z., and Zhang, H. (2019). Phase separation, transition, and autophagic degradation of proteins in development and pathogenesis. Trends Cell Biol. 29, 417–427. doi: 10.1016/j.tcb.2019.01.008
Wu, Y., Ma, P., Liu, Y., and Li, S. (2001). Diffusion coefficients of L-proline, L-threonine and L-arginine in aqueous solutions at 25°C. Fluid Phase Equilib. 186, 27–38. doi: 10.1016/S0378-3812(01)00355-7
Xue, B., Dunbrack, R. L., Williams, R. W., Dunker, A. K., and Uversky, V. N. (2010). PONDR-FIT: a meta-predictor of intrinsically disordered amino acids. Biochim. Biophys. Acta Proteins Proteomics 1804, 996–1010. doi: 10.1016/j.bbapap.2010.01.011
Yoshizawa, T., Nozawa, R. S., Jia, T. Z., Saio, T., and Mori, E. (2020). Biological phase separation: cell biology meets biophysics. Biophys. Rev. 12, 519–539. doi: 10.1007/s12551-020-00680-x
Keywords: phase separation, amino acid, solubility, soluble interaction, insoluble interaction
Citation: Nomoto A, Nishinami S and Shiraki K (2021) Solubility Parameters of Amino Acids on Liquid–Liquid Phase Separation and Aggregation of Proteins. Front. Cell Dev. Biol. 9:691052. doi: 10.3389/fcell.2021.691052
Received: 05 April 2021; Accepted: 20 May 2021;
Published: 16 June 2021.
Edited by:
Eiichiro Mori, Nara Medical University, JapanReviewed by:
Takuya Yoshizawa, Ritsumeikan University, JapanSachiko Toma-Fukai, Nara Institute of Science and Technology, Japan
Copyright © 2021 Nomoto, Nishinami and Shiraki. This is an open-access article distributed under the terms of the Creative Commons Attribution License (CC BY). The use, distribution or reproduction in other forums is permitted, provided the original author(s) and the copyright owner(s) are credited and that the original publication in this journal is cited, in accordance with accepted academic practice. No use, distribution or reproduction is permitted which does not comply with these terms.
*Correspondence: Kentaro Shiraki, shiraki@bk.tsukuba.ac.jp