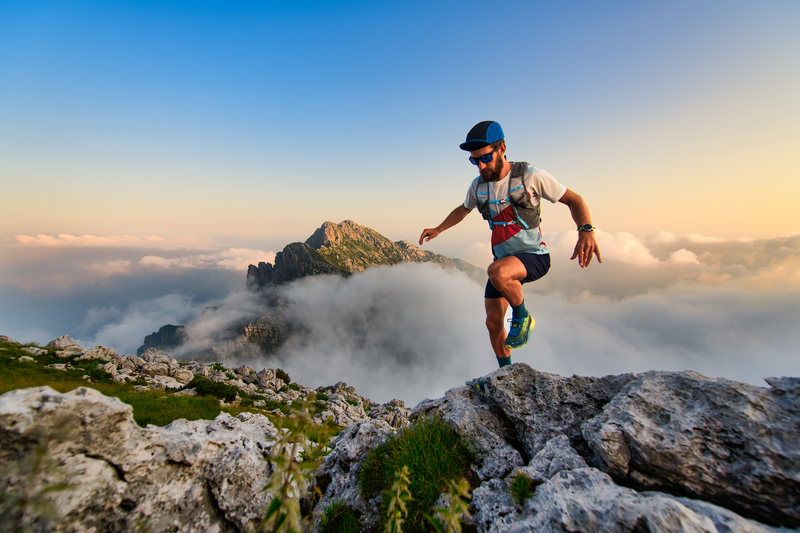
95% of researchers rate our articles as excellent or good
Learn more about the work of our research integrity team to safeguard the quality of each article we publish.
Find out more
ORIGINAL RESEARCH article
Front. Cell Dev. Biol. , 12 August 2021
Sec. Cell Death and Survival
Volume 9 - 2021 | https://doi.org/10.3389/fcell.2021.690882
This article is part of the Research Topic The Regulation of Autophagy Activity in Pathogenic Microorganism Infection View all 8 articles
Hepatitis is an inflammatory condition of the liver, which is frequently caused by the infection of hepatitis B virus (HBV) or hepatitis C virus (HCV). Hepatitis can lead to the development of chronic complications including cancer, making it a major public health burden. Co-infection of HBV and HCV can result in faster disease progression. Therefore, it is important to identify shared genetic susceptibility loci for HBV and HCV infection to further understand the underlying mechanism. Through a meta-analysis based on genome-wide association summary statistics of HBV and HCV infection, we found one novel locus in the Asian population and two novel loci in the European population. By functional annotation based on multi-omics data, we identified the likely target genes at each novel locus, such as HMGB1 and ATF3, which play a critical role in autophagy and immune response to virus. By re-analyzing a microarray dataset from Hmgb1–/– mice and RNA-seq data from mouse liver tissue overexpressing ATF3, we found that differential expression of autophagy and immune and metabolic gene pathways underlie these conditions. Our study reveals novel common susceptibility loci to HBV and HCV infection, supporting their role in linking autophagy signaling and immune response.
Hepatitis is an inflammatory condition of the liver, which can be an acute or a chronic infection. The most common types of hepatitis are caused by hepatitis B virus (HBV) or hepatitis C virus (HCV), both of which are major types of infectious disease affecting millions of patients and can lead to the development chronic complications such as cirrhosis or liver cancer (Schweitzer et al., 2015; Manns et al., 2017; Spearman et al., 2019). Therefore, it is important to understand the genetic and molecular mechanism underlying hepatitis viral infection for disease prevention and control. The contribution of host genetic factors is an important perspective of susceptibility and persistence of hepatitis infection (Ganem and Prince, 2004; Rehermann and Bertoletti, 2015). Genetic studies, especially genome-wide association studies (GWAS), in the last decade have identified a number of genetic susceptibility loci for HBV (Zhang et al., 2019b) and HCV (Yan and Wang, 2017). Most of the GWASes for HBV were conducted in Asian populations and those for HCV were conducted in European populations.
Though HBV and HCV are due to infection by different types of viruses, and they have differences in transmission and symptoms, they share certain common underlying molecular mechanisms (D’souza et al., 2020). The two types of virus can be co-transmitted, and some patients have co-infection of HBV and HCV simultaneously, which leads to acute fulminant hepatitis and chronic hepatitis. The worldwide prevalence rate for HBV and HCV co-infection is estimated to be 1–15% (Mavilia and Wu, 2018).
It is important to understand the shared host genetic and molecular factors for HBV and HCV within different populations for the purpose of disease prevention and control. To address these questions, we carried out a comparative study based on the GWAS data reported in the Pan UK Biobank, Japanese Biobank, and those in Gene Expression Omnibus (GEO). We found genes functioning in the autophagy pathway are among target genes of the shared genetic loci of HBV and HCV infection. A re-analysis of microarray data in a GEO dataset showed that deletion of target gene Hmgb1 in mouse hepatic tissue resulted in differential expression of genes associated with autophagosome–lysosome fusion and perturbation of immune response to viruses. Re-analyzing another RNA-seq dataset from mice hepatic tissue overexpressing ATF3 gene similarly demonstrated the significant change in the expression level of autophagy and metabolic genes. Our results suggest the role of autophagy-related genes in the common pathogenesis mechanism of HBV and HCV infection.
The GWAS summary statistics were downloaded from the website of the Japanese ENcyclopedia of GEnetic associations by Riken,1 including the datasets of chronic hepatitis B and chronic hepatitis C. The GWAS summary statistics of the Pan UK Biobank were downloaded from https://pan.ukbb.broadinstitute.org/, including two datasets: viral hepatitis B and viral hepatitis C (Supplementary Table 1).
Quality control (QC) filtering was performed on the summary statistics data of each cohort. Any variant with low confidence or minor allele frequency (MAF) < 0.01 was removed from further analysis. The number of variants before and after QC is shown in Supplementary Table 1.
After data QC, meta-analyses based on the above GWAS summary statistics of hepatitis infection were performed for each population. The Z-score-based method implemented in the software METAL (Willer et al., 2010) was adopted with sample overlap correction. This approach computed a weighted sum of Z-score for each effect allele of each single-nucleotide polymorphism (SNP) across cohorts, with weights defined by the square root of the effective sample size for each cohort. It also takes the direction of effect into account (Willer et al., 2010).
The associated SNPs were clumped into linkage disequilibrium (LD)-independent genomic regions using the software PLINK (Purcell et al., 2007) with the following parameter settings: –clump-r2 = 0.4, –clump-kb = 500, –clump-p1 = 5e-08, and –clump-p2 = 5e-02. LD information from the 1,000 Genomes phase 3 data (Auton et al., 2015) of European and East Asian ancestry was used in the analysis. The MHC region (chr6: 25–35 MB) was considered as one locus. The resulting independent genomic loci were compared to those reported in the GWAS catalog for hepatitis C and hepatitis B diseases. Any locus within 1 MB of a reported locus was regarded as a known locus. By excluding known loci, the remaining genome-wide significant (GWS) loci were considered as novel association loci.
Fine mapping via the software FINEMAP (Benner et al., 2016) was conducted to identify variants in the 95% credible set of each novel GWS locus, with LD score calculation based on the EUR and EAS samples of the 1,000 Genome phase3 panel. The maximum number of allowed causal SNPs is set as 10 in the analysis. Causal variants were defined as those with r2 > 0.6 with the index SNP and the association P-value < 1 × 10–4 in the meta-analysis.
Functional annotation of each GWS locus was performed with FUMA GWAS (Watanabe et al., 2017). Annotation of the expression quantitative trait loci (eQTLs) was based on scRNA eQTLs (van der Wijst et al., 2018), DICE (Schmiedel et al., 2018), eQTLGen, blood eQTLs (Westra et al., 2013), and GTEx v8 (Battle et al., 2017). The threshold for significant eQTL SNP–gene pairs was defined as a false discovery rate (FDR) < 0.05. To annotate chromatin interactions, the liver Hi-C datasets were analyzed by using the 3D Genome Browser and 3D-genome Interaction Viewer (Lieberman-Aiden et al., 2009; Wang et al., 2018; Yang et al., 2018).
The microarray dataset GSE49824 was downloaded from the GEO database (Huebener et al., 2014). The dataset contains gene expression profiling data from mouse hepatic stellate cells from four mice with hepatocyte-specific deletion of Hmgb1 and four control mice. The R package GEOquery was used for data download (Davis and Meltzer, 2007). Calibration, quantile normalization, and differential expression analysis were conducted with the R package limma (Ritchie et al., 2015). The significant genes were defined as log2| fold change (FC)| > 0.5 and P-value < 0.05.
Count data were downloaded from the GEO dataset GSE148301 (Xu et al., 2021). Then, the variance-stabilizing transformation (vst) normalization and differential expression were performed via the R package DESeq2 (Love et al., 2014). The significant genes were defined as log2| fold change (FC)| > 1 and Padj < 0.05.
The online web portal DAVID (Huang et al., 2007; Huang da et al., 2009b) was used for pathway enrichment analysis with differentially expressed genes as input. The collection of GO and KEGG pathway datasets was used to find significantly enriched pathways (Huang da et al., 2009a).
The protein–protein interaction (PPI) network was predicted using the Search Tool for the Retrieval of Interacting Genes (STRING)2 online database (Franceschini et al., 2013), removing interactions with a combined score < 0.4. Then, the PPI network was drawn using Cytoscape (version 3.7.2). The significant interactions were identified using Degree (Smoot et al., 2011). Finally, DAVID was used to conduct pathway enrichment analysis on the 19 genes that were most closely related in the network (Huang da et al., 2009a).
Data for the replication cohort were extracted from the biobank of the Center for Applied Genomics (CAG), at the Children’s Hospital of Philadelphia (CHOP). Patients with a diagnosis of “Hepatitis C virus infection,” “Chronic hepatitis C,” “Hepatitis B virus infection,” or “Chronic hepatitis B” were selected for analyses. Age, sex, and ancestry-matched subjects without diagnosis of hepatitis were selected as controls. The ICD9 codes and the diagnosis name of the selected cases are listed in Supplementary Table 2.
The study was approved by the Institutional Review Board at CHOP, and written informed consent was obtained from all participating subjects and/or their parents. The study was carried out in accordance with the nationally approved guidelines.
Genomic DNA extracted from whole blood samples of the replication cohort was genotyped on Illumina HumanHap550-V1/V3, HumanHap610-Quad, or Infinium Global Screening Array-24 (GSA) DNA BeadChips at CAG.
Samples with call rate < 98%, with ambiguous sex detected by PLINK (Purcell et al., 2007), or heterozygosity outliers were excluded. Duplicated or cryptically related samples were defined as PI_HAT ≥ 0.1875 in identity-by-state analysis and one from each pair was excluded. The SNPs to replicate were checked for genotype rate, minor allele frequency, and Hardy–Weinberg equilibrium test. All the QC steps were performed with PLINK.
Principal component analysis (PCA) was performed with PLINK to confirm the self-reported European ancestry and to derive the principal components, which were then applied to association analysis as covariates to control for population stratification.
The unphased SNP-array genotype data of all the samples in the replication cohort were converted to VCF files and uploaded to Trans-Omics for Precision Medicine (TOPMed) imputation server3 for phasing using Eagle2 (Loh et al., 2016) and imputation using Minimac44 (Fuchsberger et al., 2015) with the TOPMed r2 as the reference panel. After imputation, BCFtools v1.6 (Li et al., 2009) was used to remove SNPs with imputation score R2 < 0.3.
Logistic regression was performed via PLINK to assess the association between SNP genotype and HBV/HCV status, including sex and the first 10 principal components as covariates.
We aimed to understand the common pathogenesis mechanism of HBV and HCV infection, to aid in protection from harmful coinfection of these viruses. We carried out a meta-analysis between the GWAS results of HBV and HCV within populations of European ancestry and Asian ancestry, respectively (Supplementary Table 1). In the Asian population, the HLA locus on chromosome 6 showed strong association and another previously reported locus on chromosome 19 at the 5’ of IFNL4 also reached GWS (Figure 1A). In addition, we identified a novel GWS locus at 13q12.3, located in 5’ of gene MEDAG (Figures 1A, 2A). From the meta-analysis of the GWAS summary statistics of HBV and HCV in the Pan UK Biobank, we found two novel GWS loci at 1q32.3 and 5p13.3 (P-value < 5 × 10–8). These loci are in the intron of gene INTS7 and 3’ of gene CDH6, respectively (Table 1 and Figures 1B, 2B,C). INTS7 encodes integrator complex subunit 7, and CDH6 is a type II cadherin. Looking into the association of the top SNP at the novel GWS loci with HBV and HCV infections separately, we found that the top SNP at each novel locus was associated at nominal significance level (P-value < 0.05) in the original GWAS statistics and showed consistent direction of effects (Table 1). They reached GWS level in the meta-analysis due to the synergistic contribution from both disease cohorts instead of being driven by HBV or HCV specifically.
Figure 1. Manhattan plots showing the association statistics for hepatitis B virus (HBV)/hepatitis C virus (HCV) meta-analysis results in populations of Asian and European ancestries, respectively. (A) A meta-analysis on genome-wide association study (GWAS) summary statistics in the database of the Japanese ENcyclopedia of GEnetic associations by Riken. (B) A meta-analysis on GWAS summary statistics in the Pan UK Biobank. The genomic coordination of single-nucleotide polymorphisms (SNPs) is indicated on the X-axis, and the -log10 of P-value per SNP in meta-analysis is indicated on the Y-axis. The horizontal red line represents the genome-wide significance threshold P-value = 5 × 10–8. The closest genes to the previously reported loci are shown in black, and those closest to the novel loci are shown in red.
Figure 2. The regional association plots for the novel genome-wide significant loci associated with HBV/HCV infection. The genomic coordination is indicated on the X-axis, and the -log10 P-value of meta-analysis is shown on the left Y-axis. The most significantly associated SNP is shown in purple, and the different colors represent the linkage disequilibrium between the surrounding SNPs and the lead SNP. (A) The locus 13q12.3; (B) the locus 1q32.3; and (C) the locus 5p13.3.
Next, we tried to understand how the novel loci in each population contribute to the development of HBV/HCV infection by performing functional annotation of SNPs at the novel loci and examining potential target genes. It is known that the causal variant at each locus may not be the lead SNP, but a SNP in LD. Therefore, we performed fine mapping to identify likely causal variants satisfying the criteria of LD, association in meta-analysis, and Bayesian statistics posterior probability (Table 2). The results show that lead SNPs rs142568263 at 1q32.3 and rs76561592 at 5p13.3 are in the credible set of potential causal variants. Additionally, four SNPs and two SNPs are in the credible set of these two loci, respectively, each with a posterior probability of 1. For locus 13q12.3, there are only three SNPs with a posterior probability > 0.2. Nine of these SNPs overlap with histone modification marks, such as H3K4me1, H3K4me3, H3K27ac, and H3K9ac in liver tissue, suggesting their potential role as cis-regulatory elements regulating target gene expression (Supplementary Table 3).
Table 2. The credible sets of potential causal variants at each novel genome-wide significant locus.
The SNPs at each GWAS locus could regulate the expression of distant genes through chromatin interaction, in addition to the regulation of its closest gene (Smemo et al., 2014; Claussnitzer et al., 2015; Xia et al., 2016). To identify target genes of each novel association locus, we examined eQTL genes in liver or immune cell types. Gene LPGAT1 is an eQTL gene for SNP rs150857619 at locus 1q32.3 with FDR < 0.05 (Supplementary Table 4). LPGAT1 encodes lysophosphatidylglycerol acyltransferase 1, which may be involved in triacylglycerol synthesis and secretion in the liver. Mouse Lpgat1 knockout resulted in phosphatidylglycerol remodeling defects and led to oxidative stress, mitochondrial DNA depletion, and mitochondrial dysfunction. The Lpgat1–/– mice developed diet-induced obesity and hepatopathy phenotypes (Zhang et al., 2019a). These results indicate the role of LPGAT1 in mitophagy, which is an important type of selective autophagy to maintain cellular homeostasis upon virus invasion. The expression level of additional genes was found to have nominal significant association with the top associated SNP or causal variants at each novel GWS locus (P-value < 0.05) (Supplementary Table 4).
We also examined genes having chromatin interactions with these loci in Hi-C datasets (Supplementary Table 5). The Hi-C data suggest that there is a chromatin interaction between the HBV/HCV susceptibility loci and the promoter region of multiple genes, which have been reported to be involved in autophagy and immune signaling pathways. For example, the target genes of locus 5p13.3: CDH6 inhibits autophagy and promotes re-organization of the mitochondrial network (Gugnoni et al., 2017); Drosha is involved in regulating hepatocyte growth (Fabris et al., 2019) and HBV replication control (Yang et al., 2017). GOLPH3 is another targeted gene of locus 5p13.3 functioning in selective autophagy (Lu et al., 2020) and hepatitis C virus secretion (Bishe et al., 2012). Similarly, the target genes at locus 1q32.3: TRAF3IP3 enhances autophagy through interaction with an ATG16L1-binding motif (Peng et al., 2015), and ATF3 is a negative regulator of autophagy (Sood et al., 2017). Another gene of particular interest is HMGB1, which is in the same topologically associated domain (TAD) as SNP rs34780238 at 13q12.3 (Figure 3 and Supplementary Figure 1). Twenty three out of 54 Hi-C genes at these three loci exhibited nominally significant eQTL association with the three GWS loci, including the above autophagy-related genes CDH6, ATF3, and HMGB1 (Supplementary Table 5).
Figure 3. The genome-wide significant SNPs at the novel locus of 13q12.3 and its target genes are located in the same topologically associated domains (TAD).
To further understand the function and role of HMGB1 in the HBV/HCV infection, we analyzed a microarray dataset from the liver of Hmgb1 knockout mice. Our results yielded 194 differentially expressed genes, including 82 upregulated genes and 112 downregulated genes(log2| FC| > 0.5, P-value < 0.05) (Figure 4A and Supplementary Table 6). Consistent with the original report, we did not observe significant changes in expression levels of genes involved in autophagosome formation. However, the key gene Atp6v0d2 functioning at the step of fusion between autophagosome and lysosome showed increased expression in Hmgb1 KO mice (Figure 4B) (FC = 1.58, P-value = 9.11E-04). We further conducted pathway enrichment analysis to examine the signaling pathways affected by Hmgb1 deletion. Five pathways related to virus infection and immune response were significantly enriched among the 194 differentially expressed genes (DEGs), including “innate immune response” and “response to virus” pathways (Figure 4C and Supplementary Table 7). We also constructed a PPI network analysis among the DEGs, revealing 19 significantly associated genes (Figure 4D). Pathway analysis of the significantly associated genes demonstrated significant enrichment of “immune system process”, “defense response to virus”, “negative regulation of viral genome replication” and other immune function-related gene sets (Supplementary Table 8). These findings further suggest that Hmgb1 may regulate hepatitis virus infection.
Figure 4. Genes and pathways showed significant changes in Hmgb1–/– mice compared to controls. (A) Our in silico re-analysis of microarray data from Hmgb1–/– and controls showed 82 upregulated genes and 112 downregulated genes including Atp6v0d2. (B) A heatmap showing the comparison of the differentially expressed genes (DEGs) among the Hmgb1–/– and control mice. (C) Multiple immune pathways in response to viral infection are enriched in DEGs. (D) The protein–protein interaction (PPI) network formed by DEGs.
We also looked into the function of a potential target gene ATF3 at locus 1q32.3, which exhibited chromatin interaction with GWS SNPs at this locus and showed nominally significant association with these SNPs (Supplementary Tables 4, 5). ATF3 is a negative regulator of autophagy and cellular antiviral signaling by interacting with the promoter of Atg5, Stat1, Irf9, and Isg15 (Sood et al., 2017). A study also showed that ATF3 promotes hepatitis B virus x mRNA degradation by stimulating Ski2 expression (Shiromoto et al., 2018). We examined a GEO dataset from C57BL/6J mice injected with adeno-associated virus (AAV) expressing human ATF3 (Xu et al., 2021). We observed the significant upregulation of 389 genes and downregulation of 315 genes in mouse liver tissue (Figure 5A and Supplementary Table 9). Interestingly, the autophagy gene Atg16l2 showed significant upregulation (logFC = 2.89, adj. P-value = 8.07E-75). Atg16l2 is an isoform of Atg16l and can interact with Atg12-conjugated Atg5 to form a complex in vivo. Atg16l2 can function as an E3-like enzyme to catalyze the conjugation of LC3 to phosphatidylethanolamine (Ishibashi et al., 2011). The DEGs were enriched in metabolic signaling pathways including mitophagy-related pathways, such as oxidoreductase activity, mitochondrion morphogenesis, and endoplasmic reticulum, and they interconnect with each other (Supplementary Table 10 and Figure 5B). Consistently, we also found enrichment of the organelle membrane pathway, which is involved in diverse biological functions including autophagy (Lin et al., 2021). It is known that viruses may trigger and modulate mitophagy to affect the innate immune response of the host. ATF3 ChIP-seq data from the ENCyclopedia of DNA Elements (ENCODE) showed a strong signal at the genomic region including gene ATG16L2, suggesting the likely regulation of ATG16L2 by ATF3 (Figure 5C).
Figure 5. Genes showed significant changes in mouse liver tissue overexpressing ATF3 compared to controls. We did a differential expression analysis of RNA-seq data from mouse liver tissue overexpressing ATF3 and controls. (A) The results showed 389 significantly upregulated genes and 315 downregulated genes including Atg16l2. (B) PPI network of genes functioning in immune, endoplasmic reticulum, and mitochondria-related signaling pathways. (C) The peaks in the genomic region of human gene ATG16L2 from ATF3 ChIP-seq data in the ENCyclopedia of DNA Elements.
To validate the GWS loci identified in our study, we conducted a replication study with data extracted from the biobank of CAG, CHOP. The replication cohort of European ancestry after QC filtering includes 67 cases with the diagnosis of HBV or HCV and 649 controls (Supplementary Tables 2, 11). Both the lead SNP rs142568263 at locus 1q32.3 and the top SNP rs76561592 at locus 5p13.3 are associated with HBV/HCV infection in the replication cohort (rs142568263 P-value = 0.00874, rs76561592 P-value = 0.0139) (Supplementary Table 12); thus, the two loci were replicated with a P-value < 0.025. The number of genotyped HBV/HCV cases of East Asian ancestry that we could find is too small for a replication study; however, the peak SNP rs34780238 at locus 13q12.3 is associated with phenotype HBc antigen for hepatitis B virus (phenotype code 23016, P-value = 7.757E-5) in UKBB reported in Global Biobank Engine (Stanford, CA)5 (McInnes et al., 2019). Variants mapped to HMGB1 are associated with multiple blood traits, like mean corpuscular volume and eosinophil counts at GWS level, as reported in literature (Astle et al., 2016; Chen et al., 2020). The results from the replication cohort, the supporting evidence from literature, and the known biological function of the candidate genes at each locus suggest that these loci are likely to have bona fide HBV/HCV associations.
The HBV–HCV co-infection confers faster disease progression and higher risk of hepatocellular carcinoma development (Mavilia and Wu, 2018). To help to identify patients with high susceptibility to HBV–HCV co-infection, we first conducted a meta-analysis among the Asian population and the European population, respectively, then investigated the potential target genes of each novel GWS locus by functional annotation, and finally we focused on potential target genes HMGB1 and ATF3. We found three loci associated with both HBV and HCV infection. Our results demonstrate perturbation of the Atp6v0d2 gene and the virus immune response pathway upon Hmgb1 deletion. Also, in mouse liver tissue overexpressing ATF3, the influence on Atg16l2 expression and genes in metabolic signaling pathways was demonstrated. Our results suggest the role of target genes at novel GWS loci in connecting autophagy signaling to host immune response from virus invasion.
The HMGB1 gene encodes a protein high-mobility group box 1, which is highly expressed in different organs, tissues, and cell types including the liver and immune cells. HMGB1 is a chromatin-binding protein that can regulate gene transcription. HMGB1 can also function as a class of damage-associated molecular pattern (DAMP) molecules in response to danger signals such as virus invasion. HMGB1 initiates an inflammatory reaction by interacting with RAGE and TLR4 on neighboring cells and activates the signaling pathway p38/p42/44 MAPK; pJNK; and cJun or p38 MAPK, pERK, and NFκB in different liver cell types (Yang et al., 2020). HMGB1 is involved in the pathogenesis of acute liver injury and chronic liver disease (Gaskell et al., 2018). It was reported that HMGB1 gene 1176G/G genotype is associated with an increased risk of progressive status in HBV infection (Deng et al., 2013). Experimental studies indicated that HCV infection led to the translocation of HMGB1 from the nucleus to the cytoplasm. HMGB1 protein interacts with the HCV genome and increases the replication but not translation of HCV (Yu et al., 2015).
In addition to its function as a DAMP, HMGB1 plays a key role in the regulation of autophagy. HMGB1 can compete with Bcl-2 for binding with Beclin 1 by directly interacting with Beclin 1 and inhibiting phosphorylation of Bcl-2 (Sun and Tang, 2014). Furthermore, oxidation and then cytosolic translocation of HMGB1 increase autophagic flux (Tang et al., 2010). It has been shown that HMGB1 is necessary for hepatic stellate cell activation and participates in HBV-related liver fibrosis progression through its interaction with RAGE and autophagy-inducing effects (Li et al., 2018). HMGB1 is also involved in the pathogenesis of hepatic carcinoma (Chen et al., 2018; Khambu et al., 2018, 2020).
Discrepancy exists regarding the role of HMGB1 in autophagy (Huebener et al., 2014). Here we conducted a re-analysis of microarray data from Hmgb1 KO mice, finding upregulation of Atp6v0d2 and significant expression change of immune response to virus signaling pathway genes. Atp6v0d2 gene encodes ATPase H + transporting V0 subunit D2, which is a subunit of the V0 complex of vacuolar ATPase. Atp6v0d2 is located at lysosomes and endosomes, in addition to vacuoles. Atp6v0d2 interacts with STX17 and VAMP8, which stimulate autophagosome–lysosome fusion, the key final autophagy process (Xia et al., 2019).
Thus, the above evidence suggests that HMGB1 senses HBV/HCV virus infection and transduces that signal to the autophagy pathway and inflammation. The detailed interaction between subsequent pathways downstream of HMGB1 remains to be investigated. Polymorphisms in the genomic locus targeting HMGB1 gene could confer susceptibility to HBV/HCV infection.
With overexpression of ATF3, the potential target gene at locus 1q32.3, an autophagy gene Atg16l2, displayed significant differential expression. Though not essential for canonical autophagy, Atg16l2 is involved in the autophagy process in various cell types. It was shown that knocking down Atg16l2 in human neuroblastoma H4 cells resulted in increased autophagy flux, suggesting the negative regulation of autophagy by Atg16l2 (Lipinski et al., 2010). Another study showed that in the pancreas, where Atg16l2 is highly expressed, knocking down of Atg16l2 led to the accumulation of LC3 and p62, decreased autophagic proteolysis, and slower LC3-II turnover, suggesting the role of ATG16L2 as an adaptor protein in autophagy completion (Li et al., 2013). In addition, with the overexpression of ATF3, genes in a number of metabolic pathways were affected. It is known that metabolism is closely related to both autophagy and immunity (Li et al., 2021). The metabolic status of a cell can have a profound influence on the nature and extent of autophagic induction (Rabinowitz and White, 2010).
In addition to HMGB1 and ATF3, other target genes at the HBV/HCV shared GWS loci are involved in both autophagy and immune signaling pathway to virus infection. Medications targeting these genes are worthy of being investigated for their potential therapeutic role in HBV and HCV infection or co-infection.
The datasets presented in this study can be found in online repositories. The names of the repository/repositories and accession number(s) can be found in the article/Supplementary Material.
The studies involving human participants were reviewed and approved by the Institutional Review Board at the Children’s Hospital of Philadelphia. Written informed consent to participate in this study was provided by the participants’ legal guardian/next of kin.
JL, QX, and HH were responsible for the conception and design of study. XH, JH, HW, and DZ conducted the data analysis and summarization. JG and MM conducted the replication study, data analysis, and interpretation. XH and JG drafted the manuscript. JG, QX, JL, and HH revised the manuscript. All authors have read and approved the manuscript.
This work was supported by the National Natural Science Foundation of China (81771769), the Natural Science Foundation of Guangdong Province (2021A1515012392), the State Key Laboratory of Respiratory Disease (SKLRD) Open Project 202008, and the Guangzhou Key Medical Discipline Construction Project Fund.
The authors declare that the research was conducted in the absence of any commercial or financial relationships that could be construed as a potential conflict of interest.
All claims expressed in this article are solely those of the authors and do not necessarily represent those of their affiliated organizations, or those of the publisher, the editors and the reviewers. Any product that may be evaluated in this article, or claim that may be made by its manufacturer, is not guaranteed or endorsed by the publisher.
The Supplementary Material for this article can be found online at: https://www.frontiersin.org/articles/10.3389/fcell.2021.690882/full#supplementary-material
Astle, W. J., Elding, H., Jiang, T., Allen, D., Ruklisa, D., Mann, A. L., et al. (2016). The allelic landscape of human blood cell trait variation and links to common complex disease. Cell 167, 1415–1429.
Auton, A., Brooks, L. D., Durbin, R. M., Garrison, E. P., Kang, H. M., Korbel, J. O., et al. (2015). A global reference for human genetic variation. Nature 526, 68–74. doi: 10.1038/nature15393
Battle, A., Brown, C. D., Engelhardt, B. E., and Montgomery, S. B. (2017). Genetic effects on gene expression across human tissues. Nature 550, 204–213. doi: 10.1038/nature24277
Benner, C., Spencer, C. C., Havulinna, A. S., Salomaa, V., Ripatti, S., and Pirinen, M. (2016). FINEMAP: efficient variable selection using summary data from genome-wide association studies. Bioinformatics 32, 1493–1501. doi: 10.1093/bioinformatics/btw018
Bishe, B., Syed, G. H., Field, S. J., and Siddiqui, A. (2012). Role of phosphatidylinositol 4-phosphate (PI4P) and its binding protein GOLPH3 in hepatitis C virus secretion. J. Biol. Chem. 287, 27637–27647. doi: 10.1074/jbc.m112.346569
Chen, M. H., Raffield, L. M., Mousas, A., Sakaue, S., Huffman, J. E., Moscati, A., et al. (2020). Trans-ethnic and ancestry-specific blood-cell genetics in 746,667 individuals from 5 global populations. Cell 182, 1198–1213.
Chen, R., Zhu, S., Fan, X. G., Wang, H., Lotze, M. T., Zeh, H. J., et al. (2018). High mobility group protein B1 controls liver cancer initiation through yes-associated protein -dependent aerobic glycolysis. Hepatology 67, 1823–1841. doi: 10.1002/hep.29663
Claussnitzer, M., Dankel, S. N., Kim, K. H., Quon, G., Meuleman, W., Haugen, C., et al. (2015). FTO obesity variant circuitry and adipocyte browning in humans. N. Engl. J. Med. 373, 895–907. doi: 10.1056/nejmoa1502214
D’souza, S., Lau, K. C., Coffin, C. S., and Patel, T. R. (2020). Molecular mechanisms of viral hepatitis induced hepatocellular carcinoma. World J. Gastroenterol. 26, 5759–5783. doi: 10.3748/wjg.v26.i38.5759
Davis, S., and Meltzer, P. S. (2007). GEOquery: a bridge between the gene expression omnibus (GEO) and bioconductor. Bioinformatics 23, 1846–1847. doi: 10.1093/bioinformatics/btm254
Deng, C. Q., Deng, G. H., and Wang, Y. M. (2013). HMGB1 gene polymorphisms in patients with chronic hepatitis B virus infection. World J. Gastroenterol. 19, 5144–5149. doi: 10.3748/wjg.v19.i31.5144
Fabris, G., Dumortier, O., Pisani, D. F., Gautier, N., and Van Obberghen, E. (2019). Amino acid-induced regulation of hepatocyte growth: possible role of Drosha. Cell Death Dis. 10:566.
Franceschini, A., Szklarczyk, D., Frankild, S., Kuhn, M., Simonovic, M., Roth, A., et al. (2013). STRING v9.1: protein-protein interaction networks, with increased coverage and integration. Nucleic Acids Res. 41, D808–D815.
Fuchsberger, C., Abecasis, G. R., and Hinds, D. A. (2015). Minimac2: faster genotype imputation. Bioinformatics 31, 782–784. doi: 10.1093/bioinformatics/btu704
Ganem, D., and Prince, A. M. (2004). Hepatitis B virus infection–natural history and clinical consequences. N. Engl. J. Med. 350, 1118–1129. doi: 10.1056/nejmra031087
Gaskell, H., Ge, X., and Nieto, N. (2018). High-mobility group box-1 and liver disease. Hepatol. Commun. 2, 1005–1020. doi: 10.1002/hep4.1223
Gugnoni, M., Sancisi, V., Gandolfi, G., Manzotti, G., Ragazzi, M., Giordano, D., et al. (2017). Cadherin-6 promotes EMT and cancer metastasis by restraining autophagy. Oncogene 36, 667–677. doi: 10.1038/onc.2016.237
Huang da, W., Sherman, B. T., and Lempicki, R. A. (2009a). Bioinformatics enrichment tools: paths toward the comprehensive functional analysis of large gene lists. Nucleic Acids Res. 37, 1–13. doi: 10.1093/nar/gkn923
Huang da, W., Sherman, B. T., and Lempicki, R. A. (2009b). Systematic and integrative analysis of large gene lists using DAVID bioinformatics resources. Nat. Protoc. 4, 44–57. doi: 10.1038/nprot.2008.211
Huang, D. W., Sherman, B. T., Tan, Q., Kir, J., Liu, D., Bryant, D., et al. (2007). DAVID bioinformatics resources: expanded annotation database and novel algorithms to better extract biology from large gene lists. Nucleic Acids Res. 35, W169–W175. doi: 10.1093/nar/gkm415
Huebener, P., Gwak, G. Y., Pradere, J. P., Quinzii, C. M., Friedman, R., Lin, C. S., et al. (2014). High-mobility group box 1 is dispensable for autophagy, mitochondrial quality control, and organ function in vivo. Cell Metab. 19, 539–547. doi: 10.1016/j.cmet.2014.01.014
Ishibashi, K., Fujita, N., Kanno, E., Omori, H., Yoshimori, T., Itoh, T., et al. (2011). Atg16L2, a novel isoform of mammalian Atg16L that is not essential for canonical autophagy despite forming an Atg12-5-16L2 complex. Autophagy 7, 1500–1513. doi: 10.4161/auto.7.12.18025
Khambu, B., Hong, H., Liu, S., Liu, G., Chen, X., Dong, Z., et al. (2020). The HMGB1-RAGE axis modulates the growth of autophagy-deficient hepatic tumors. Cell Death Dis. 11:333.
Khambu, B., Huda, N., Chen, X., Antoine, D. J., Li, Y., Dai, G., et al. (2018). HMGB1 promotes ductular reaction and tumorigenesis in autophagy-deficient livers. J. Clin. Invest. 128, 2419–2435. doi: 10.1172/jci91814
Li, H., Handsaker, B., Wysoker, A., Fennell, T., Ruan, J., Homer, N., et al. (2009). The sequence alignment/map format and SAMtools. Bioinformatics 25, 2078–2079. doi: 10.1093/bioinformatics/btp352
Li, J., Zeng, C., Zheng, B., Liu, C., Tang, M., Jiang, Y., et al. (2018). HMGB1-induced autophagy facilitates hepatic stellate cells activation: a new pathway in liver fibrosis. Clin. Sci. 132, 1645–1667. doi: 10.1042/cs20180177
Li, N., Wu, X., Holzer, R. G., Lee, J. H., Todoric, J., Park, E. J., et al. (2013). Loss of acinar cell IKKalpha triggers spontaneous pancreatitis in mice. J. Clin. Invest. 123, 2231–2243. doi: 10.1172/jci64498
Li, W., He, P., Huang, Y., Li, Y. F., Lu, J., Li, M., et al. (2021). Selective autophagy of intracellular organelles: recent research advances. Theranostics 11, 222–256. doi: 10.7150/thno.49860
Lieberman-Aiden, E., Van Berkum, N. L., Williams, L., Imakaev, M., Ragoczy, T., Telling, A., et al. (2009). Comprehensive mapping of long-range interactions reveals folding principles of the human genome. Science 326, 289–293. doi: 10.1126/science.1181369
Lin, S., Meng, T., Huang, H., Zhuang, H., He, Z., Yang, H., et al. (2021). Molecular machineries and physiological relevance of ER-mediated membrane contacts. Theranostics 11, 974–995. doi: 10.7150/thno.51871
Lipinski, M. M., Hoffman, G., Ng, A., Zhou, W., Py, B. F., Hsu, E., et al. (2010). A genome-wide siRNA screen reveals multiple mTORC1 independent signaling pathways regulating autophagy under normal nutritional conditions. Dev. Cell 18, 1041–1052. doi: 10.1016/j.devcel.2010.05.005
Loh, P. R., Danecek, P., Palamara, P. F., Fuchsberger, C., Reshef, Y. A., Finucane, H. K., et al. (2016). Reference-based phasing using the haplotype reference consortium panel. Nat. Genet. 48, 1443–1448. doi: 10.1038/ng.3679
Love, M. I., Huber, W., and Anders, S. (2014). Moderated estimation of fold change and dispersion for RNA-seq data with DESeq2. Genome Biol. 15:550.
Lu, L. Q., Tang, M. Z., Qi, Z. H., Huang, S. F., He, Y. Q., Li, D. K., et al. (2020). Regulation of the Golgi apparatus via GOLPH3-mediated new selective autophagy. Life Sci. 253:117700. doi: 10.1016/j.lfs.2020.117700
Manns, M. P., Buti, M., Gane, E., Pawlotsky, J. M., Razavi, H., Terrault, N., et al. (2017). Hepatitis C virus infection. Nat. Rev. Dis. Primers 3:17006.
Mavilia, M. G., and Wu, G. Y. (2018). HBV-HCV coinfection: viral interactions, management, and viral reactivation. J. Clin. Transl. Hepatol. 6, 296–305.
McInnes, G., Tanigawa, Y., Deboever, C., Lavertu, A., Olivieri, J. E., Aguirre, M., et al. (2019). Global biobank engine: enabling genotype-phenotype browsing for biobank summary statistics. Bioinformatics 35, 2495–2497. doi: 10.1093/bioinformatics/bty999
Peng, S., Wang, K., Gu, Y., Chen, Y., Nan, X., Xing, J., et al. (2015). TRAF3IP3, a novel autophagy up-regulated gene, is involved in marginal zone B lymphocyte development and survival. Clin. Exp. Immunol. 182, 57–68. doi: 10.1111/cei.12658
Purcell, S., Neale, B., Todd-Brown, K., Thomas, L., Ferreira, M. A., Bender, D., et al. (2007). PLINK: a tool set for whole-genome association and population-based linkage analyses. Am. J. Hum. Genet. 81, 559–575. doi: 10.1086/519795
Rehermann, B., and Bertoletti, A. (2015). Immunological aspects of antiviral therapy of chronic hepatitis B virus and hepatitis C virus infections. Hepatology 61, 712–721. doi: 10.1002/hep.27323
Ritchie, M. E., Phipson, B., Wu, D., Hu, Y., Law, C. W., Shi, W., et al. (2015). Limma powers differential expression analyses for RNA-sequencing and microarray studies. Nucleic Acids Res. 43:e47. doi: 10.1093/nar/gkv007
Schmiedel, B. J., Singh, D., Madrigal, A., Valdovino-Gonzalez, A. G., White, B. M., Zapardiel-Gonzalo, J., et al. (2018). Impact of genetic polymorphisms on human immune cell gene expression. Cell 175, 1701–1715. doi: 10.1016/j.cell.2018.10.022
Schweitzer, A., Horn, J., Mikolajczyk, R. T., Krause, G., and Ott, J. J. (2015). Estimations of worldwide prevalence of chronic hepatitis B virus infection: a systematic review of data published between 1965 and 2013. Lancet 386, 1546–1555. doi: 10.1016/s0140-6736(15)61412-x
Shiromoto, F., Aly, H. H., Kudo, H., Watashi, K., Murayama, A., Watanabe, N., et al. (2018). IL-1beta/ATF3-mediated induction of Ski2 expression enhances hepatitis B virus x mRNA degradation. Biochem. Biophys. Res. Commun. 503, 1854–1860. doi: 10.1016/j.bbrc.2018.07.126
Smemo, S., Tena, J. J., Kim, K. H., Gamazon, E. R., Sakabe, N. J., Gomez-Marin, C., et al. (2014). Obesity-associated variants within FTO form long-range functional connections with IRX3. Nature 507, 371–375. doi: 10.1038/nature13138
Smoot, M. E., Ono, K., Ruscheinski, J., Wang, P. L., and Ideker, T. (2011). Cytoscape 2.8: new features for data integration and network visualization. Bioinformatics 27, 431–432. doi: 10.1093/bioinformatics/btq675
Sood, V., Sharma, K. B., Gupta, V., Saha, D., Dhapola, P., Sharma, M., et al. (2017). ATF3 negatively regulates cellular antiviral signaling and autophagy in the absence of type I interferons. Sci. Rep. 7:8789.
Spearman, C. W., Dusheiko, G. M., Hellard, M., and Sonderup, M. (2019). Hepatitis C. Lancet 394, 1451–1466.
Sun, X., and Tang, D. (2014). HMGB1-dependent and -independent autophagy. Autophagy 10, 1873–1876. doi: 10.4161/auto.32184
Tang, D., Kang, R., Livesey, K. M., Cheh, C. W., Farkas, A., Loughran, P., et al. (2010). Endogenous HMGB1 regulates autophagy. J. Cell Biol. 190, 881–892. doi: 10.1083/jcb.200911078
van der Wijst, M. G. P., Brugge, H., De Vries, D. H., Deelen, P., Swertz, M. A., and Franke, L. (2018). Single-cell RNA sequencing identifies celltype-specific cis-eQTLs and co-expression QTLs. Nat. Genet. 50, 493–497. doi: 10.1038/s41588-018-0089-9
Wang, Y., Song, F., Zhang, B., Zhang, L., Xu, J., Kuang, D., et al. (2018). The 3D genome browser: a web-based browser for visualizing 3D genome organization and long-range chromatin interactions. Genome Biol. 19:151.
Watanabe, K., Taskesen, E., Van Bochoven, A., and Posthuma, D. (2017). Functional mapping and annotation of genetic associations with FUMA. Nat. Commun. 8:1826.
Westra, H. J., Peters, M. J., Esko, T., Yaghootkar, H., Schurmann, C., Kettunen, J., et al. (2013). Systematic identification of trans eQTLs as putative drivers of known disease associations. Nat. Genet. 45, 1238–1243.
Willer, C. J., Li, Y., and Abecasis, G. R. (2010). METAL: fast and efficient meta-analysis of genomewide association scans. Bioinformatics 26, 2190–2191. doi: 10.1093/bioinformatics/btq340
Xia, Q., Chesi, A., Manduchi, E., Johnston, B. T., Lu, S., Leonard, M. E., et al. (2016). The type 2 diabetes presumed causal variant within TCF7L2 resides in an element that controls the expression of ACSL5. Diabetologia 59, 2360–2368. doi: 10.1007/s00125-016-4077-2
Xia, Y., Liu, N., Xie, X., Bi, G., Ba, H., Li, L., et al. (2019). The macrophage-specific V-ATPase subunit ATP6V0D2 restricts inflammasome activation and bacterial infection by facilitating autophagosome-lysosome fusion. Autophagy 15, 960–975. doi: 10.1080/15548627.2019.1569916
Xu, Y., Li, Y., Jadhav, K., Pan, X., Zhu, Y., Hu, S., et al. (2021). Hepatocyte ATF3 protects against atherosclerosis by regulating HDL and bile acid metabolism. Nat. Metab. 3, 59–74. doi: 10.1038/s42255-020-00331-1
Yan, Z., and Wang, Y. (2017). Viral and host factors associated with outcomes of hepatitis C virus infection (Review). Mol. Med. Rep. 15, 2909–2924. doi: 10.3892/mmr.2017.6351
Yang, D., Jang, I., Choi, J., Kim, M. S., Lee, A. J., Kim, H., et al. (2018). 3DIV: A 3D-genome interaction viewer and database. Nucleic Acids Res. 46, D52–D57.
Yang, H., Wang, H., and Andersson, U. (2020). Targeting inflammation driven by HMGB1. Front. Immunol. 11:484. doi: 10.3389/fimmu.2020.00484
Yang, X., Li, H., Sun, H., Fan, H., Hu, Y., Liu, M., et al. (2017). Hepatitis B virus-encoded Microrna controls viral replication. J. Virol. 91:e01919.
Yu, R., Yang, D., Lei, S., Wang, X., Meng, X., Xue, B., et al. (2015). HMGB1 promotes hepatitis C virus replication by interaction with stem-loop 4 in the viral 5’ untranslated region. J. Virol. 90, 2332–2344. doi: 10.1128/jvi.02795-15
Zhang, X., Zhang, J., Sun, H., Liu, X., Zheng, Y., Xu, D., et al. (2019a). Defective phosphatidylglycerol remodeling causes hepatopathy, linking mitochondrial dysfunction to hepatosteatosis. Cell. Mol. Gastroenterol. Hepatol. 7, 763–781. doi: 10.1016/j.jcmgh.2019.02.002
Keywords: hepatitis infection, autophagy, immune response to virus, genome-wide association study, microarray
Citation: Huang X, Glessner JT, Huang J, Zhou D, March ME, Wang H, Xia Q, Hakonarson H and Li J (2021) Discovery of Novel Host Molecular Factors Underlying HBV/HCV Infection. Front. Cell Dev. Biol. 9:690882. doi: 10.3389/fcell.2021.690882
Received: 04 April 2021; Accepted: 06 July 2021;
Published: 12 August 2021.
Edited by:
Shou-Long Deng, Chinese Academy of Medical Sciences and Peking Union Medical College, ChinaReviewed by:
Shubham Shrivastava, Bharati Vidyapeeth Deemed University, IndiaCopyright © 2021 Huang, Glessner, Huang, Zhou, March, Wang, Xia, Hakonarson and Li. This is an open-access article distributed under the terms of the Creative Commons Attribution License (CC BY). The use, distribution or reproduction in other forums is permitted, provided the original author(s) and the copyright owner(s) are credited and that the original publication in this journal is cited, in accordance with accepted academic practice. No use, distribution or reproduction is permitted which does not comply with these terms.
*Correspondence: Qianghua Xia, cWh4aWFAbXNuLmNvbQ==; Hakon Hakonarson, aGFrb25hcnNvbkBlbWFpbC5jaG9wLmVkdQ==; Jin Li, amlubGlAZ3pobXUuZWR1LmNu
†These authors have contributed equally to this work
Disclaimer: All claims expressed in this article are solely those of the authors and do not necessarily represent those of their affiliated organizations, or those of the publisher, the editors and the reviewers. Any product that may be evaluated in this article or claim that may be made by its manufacturer is not guaranteed or endorsed by the publisher.
Research integrity at Frontiers
Learn more about the work of our research integrity team to safeguard the quality of each article we publish.