- Hubei Key Laboratory of Genetic Regulation and Integrative Biology, School of Life Sciences, Central China Normal University, Wuhan, China
Small open reading frame encoded peptides (SEPs), also called microproteins, play a vital role in biological processes. Plenty of their open reading frames are located within the non-coding RNA (ncRNA) range. Recent research has demonstrated that ncRNA-encoded polypeptides have essential functions and exist ubiquitously in various tissues. To better understand the role of microproteins, especially ncRNA-encoded proteins, expressed in different tissues, we profiled the proteomic characterization of five mouse tissues by mass spectrometry, including bottom-up, top-down, and de novo sequencing strategies. Bottom-up and top-down with database-dependent searches identified 811 microproteins in the OpenProt database. De novo sequencing identified 290 microproteins, including 12 ncRNA-encoded microproteins that were not found in current databases. In this study, we discovered 1,074 microproteins in total, including 270 ncRNA-encoded microproteins. From the annotation of these microproteins, we found that the brain contains the largest number of neuropeptides, while the spleen contains the most immunoassociated microproteins. This suggests that microproteins in different tissues have tissue-specific functions. These unannotated ncRNA-coded microproteins have predicted domains, such as the macrophage migration inhibitory factor domain and the Prefoldin domain. These results expand the mouse proteome and provide insight into the molecular biology of mouse tissues.
Introduction
Microproteins are short peptides translated by mRNAs or non-coding RNAs (ncRNAs) with small open reading frames (sORFs, shorter than 100–150 codons; Ingolia, 2014; Ma et al., 2016). sORFs are widely distributed throughout the genomes of all species, such as human, mouse, and fruitfly (Frith et al., 2006). With the development of ribosome profiling, mass spectrometry (MS), and bioinformatics, increasing number of sORF-encoded microproteins have been discovered. It has been reported that ncRNAs tend to encode low molecular weight proteins (Lu et al., 2020). Furthermore, they could play essential roles in development, muscle function, and metabolism (Kondo et al., 2007; Magny et al., 2013; Lee et al., 2015). Together with microproteins encoded by mRNA, the polypeptides encoded by ncRNAs are particularly important (Jackson et al., 2018). Several polypeptides encoded by ncRNAs had fundamental functions in muscle regeneration and tumor development (Nelson et al., 2016; Huang et al., 2017). For example, the 34 amino acid (AA) peptide DWORF promotes muscle formation (Nelson et al., 2016); a 59 AA peptide SMIM30 induces cell proliferation and migration of liver cancer (Pang et al., 2020); and the 60 AA peptide SPRS inhibits angiogenesis in breast cancer (Wang et al., 2020).
ncRNAs have been found to exhibit tissue-specific expression (Landgraf et al., 2007; Liang et al., 2007; Cabili et al., 2011), suggesting that they can carry out tissue-specific functions. For example, a liver-enriched long non-coding RNA, lncLSTR, regulates systemic lipid metabolism in mice (Li et al., 2015). In the past year, researchers have provided some tissue-specific uncharacterized ncRNAs in various tissues that may be involved in health and disease (Isakova et al., 2020). Meanwhile, more and more ncRNAs are proved to be capable of coding (Ruiz-Orera et al., 2014). For example, peptide MLN encoded by a putative long non-coding RNA regulates muscle performance (Anderson et al., 2015). The study of ncRNA-encoded polypeptides in various tissues may significantly help us understand their functions. To study functional microproteins in different tissues, these microproteins must first be identified. However, large-scale microprotein identification has only been applied to a few tissues, such as the brain (Li et al., 2017; Budamgunta et al., 2018) and heart (van Heesch et al., 2019). This inspired us to systematically study the distribution of microproteins and ncRNA-encoded polypeptides among tissues.
Mass spectrometry was used to identify microproteins because of the direct detection of translated products. Bottom-up proteomic strategy involving specific sample preparation was the primary method used to search for microproteins. For example, Slavoff et al. (2013) detect sORF-encoded polypeptides (SEPs) in the human K526 cell line and identified 90 microproteins, 86 of which are previously uncharacterized. Using a similar method, 117 microproteins were identified in Saccharomyces cerevisiae (He et al., 2018). Our group identified 271 microproteins in the Hep3B cell line (Wang et al., 2021a). Researches have also used a digestion-free top-down strategy and database-independent de novo sequencing to identify microproteins (Hughes et al., 2010; Li et al., 2017; Wang et al., 2021b). Here, we used a combination of bottom-up, top-down, and de novo sequencing methods to identify microproteins in five mouse tissues. As a result, we found 1,074 microproteins, 270 of which were ncRNA-encoded, and 556 of which were tissue-specific. Nearly half of these microproteins have no MS or translation evidence according to the OpenProt database.
Materials and Methods
Tissue Preparation
BALB/c mice were obtained from the Hubei Center for Disease Control. The 11-week-old mice were sacrificed by cervical dislocation. Tissues were removed from the mice, washed with cold phosphate-buffered saline (PBS) to remove residual blood, and stored in a freezer at −80°C until further use. All animal experiments were conducted following the guidelines provided by the Institutional Animal Care and Use Committee of Central China Normal University.
Protein Extraction
From each sample, 100 mg of tissue were used. Microproteins were extracted using HCl and RIPA buffers separately, as described in the following steps:
Boiling water (200 μL) was added to the samples and left to boil for 10 min. Then, we took the aqueous components into a new centrifuge tube and added 500 μL of HCl buffer [50 mM HCl, 0.5% dithiothreitol (DTT)] to each sample. The samples were homogenized in a Dounce homogenizer (Kimble, Manzanillo, Mexicos) and centrifuged at 12,000 × g and 4°C for 30 min. The supernatant was collected and mixed with water-containing parts. Then, 125 μL of chloroform and 500 μL of ddH2O were added to each sample, mixed vigorously, centrifuged at 12,000 × g for 10 min at room temperature, and the supernatant was transferred to a new centrifuge tube. The supernatant was then dried under vacuum at a low temperature in a SpeedVac (Labconco, KS, United States).
On the other way, 1 mL RIPA buffer [150 mM NaCl, 50 mM Tris–HCl, 5 mM sodium fluoride, 1 mM sodium orthovanadate, 0.1% SDS, 1% NP40, 1 EDTA-free protease inhibitor tablet (Roche, Mannheim, Germany) per 10 mL of lysis buffer] was added to each tissue sample, homogenized in a Dounce homogenizer on ice, and centrifuged at 12,000 × g for 30 min at 4°C. The supernatant was collected, 250 μL chloroform and 1 mL ddH2O were added. The mixture was mixed vigorously and centrifuged at 12,000 × g for 10 min at room temperature. The supernatant was transferred to a new centrifuge tube. The BCA assay was used to quantify the protein amount of each sample.
SDS-PAGE
The samples extracted with HCl buffer were resuspended with 50 mM NH4HCO3, separated by 16% tricine sodium dodecyl sulfate-polyacrylamide gel electrophoresis (SDS-PAGE) and stained with Coomassie blue.
The samples extracted with RIPA buffer were resuspended in 50 mM NH4HCO3 and separated by 12% Glycine SDS-PAGE and stained with Coomassie blue. Each lane was sectioned below the 25 kDa marker into six sections followed by in-gel trypsin digestion.
Trypsin Digestion
The dried protein mixtures (100 μg each) were resuspended in 50 mM NH4HCO3, then incubated with DTT at a final concentration of 10 mM at 37°C for 1 h, and alkylated with 15 mM iodoacetamide (IAM) in the dark at room temperature for 30 min. Each sample was then incubated with 2 μg trypsin at 37°C for 16 h. The enzymatic digestion was stopped with formic acid at final concentration of 5%.
Each gel slice was washed with 500 mL of 50% acetonitrile (ACN)/50 mM NH4HCO3 (pH 8.0) for 10 min. This process was repeated three times. Gel slices were dried under vacuum at low temperature in a SpeedVac for 5 min, incubated in 10 mM DTT/50 mM NH4HCO3 at 56°C for 1 h, and then incubated in 50 mM IAM/50 NH4HCO3 at 37°C for 45 min in the dark. Then, the gel slices were washed with ACN and vacuum-dried for 5 min. Digestion was then performed at 37°C for 16 h with 0.02 μg/μL trypsin in 50 mM NH4HCO3. Peptides were extracted with 60% ACN/5% formic acid for 10 min, and the supernatant was collected into a new tube and vacuum-dried. All digested peptide mixtures were desalted on a C18 StageTips (3M EmporeTM, St.Paul, MN, United States).
Protein Fractionation
Non-enzymatic samples extracted with HCl buffer were resuspended in 200 μL NH4FA. Proteins were separated by 5–70% B (mobile phase A: 25 mM NH4FA water; mobile phase B: 25 mM NH4FA acetonitrile), with a homemade C18 column (3M EmporeTM). Eight fractions were collected per sample and directly analyzed using MS.
Synthesized Peptides
Peptides were synthesized using standard Fmoc chemistry in the Guo Tai bio-company (www.bankpeptide.com, Hefei, China). The sequences of peptides are LPLPLGR, LLEPSLR, FNPDVSWDR, NVLEEEGR, FVSEAELDER, GLFLLDDK, LAVAAQNCYK, and NDVFVLEEWGR. The peptides were resuspended in 5% ACN. The peptide loading amount was 1 pmol. The mass spectrum parameters and database search were consistent with those of the digestion sample.
LC/MS/MS Analysis
The peptides were resuspended in 0.1% formic acid water and analyzed using the Q ExactivTMPlus Orbitrap high-resolution mass spectrometer and the EASY nLCTM1200 system (Thermo Fisher Scientific, Bremen, Gemany). The samples were separated on a homemade C18 column (15 cm, 75 μm, 3 μm, and 100 Å) at a flow rate of 0.5 μL/min. The peptides were separated by 120 min 5–80% B (mobile phase A: 0.1% formic acid water; mobile phase B: 0.1% formic acid ACN). MS analysis use data-dependent acquisition mode with parameters settings: full MS [automatic gain control (AGC), 3 × 106; resolution, 7 × 104; m/z range, 350–20,000; and maximum ion time, 20 ms]; MS/MS (AGC, 5 × 104; maximum ion time, 50 ms; minimum signal threshold, 4 × 103; dynamic exclusion time setting, 40 s; and unassigned and singly charged ions were excluded).
The MS parameters for non-enzymatic samples were the same as those mentioned above, except for the following settings: full MS (AGC, 5 × 106; maximum ion time, 200 ms); MS/MS (AGC, 1 × 106; maximum ion time, 200 ms; dynamic exclusion time setting, 45 s; unassigned, singly, and double charge ions were excluded).
All LC/MS/MS raw data related to this work will be uploaded to iProX1 and available for download with access ID IPX0002949000.
Data Analysis
Raw files obtained by the bottom-up method were analyzed using Proteome Discoverer v2.1 (Thermo Fisher Scientific, Rockford, United States) with the following parameters: enzyme, trypsin; missed cleavage, 2; precursor mass tolerance, 10 ppm; fragment mass tolerance, 0.02 Da; methionine oxidation and N-terminal acetylation as dynamic modification, carbamidomethylation as a static modification. The false discovery rate (FDR) was set to 1%. The protein fasta file used mouse protein data from OpenProt2, which contains RefProts, Isoforms, and AltProts.
Data analysis for de novo sequencing was performed as follows. Raw files were converted to mgf files using MSConvertGUI and analyzed by pNovo v3.13 with the following parameters: enzyme, trypsin; precursor mass tolerance, 10 ppm; fragment mass tolerance, 0.02 Da; methionine oxidation and N-terminal acetyl as dynamic modifications; carbamidomethylation as a static modification; open search, true; keep results, top-1. For one spectrum, if de novo sequencing yielded the same results as the data-dependent search, we considered it a positive result. However, if de novo sequencing differed from the data-dependent search, we considered the de novo sequencing result as a false positive. If the spectrum was not assigned to any peptide in the data-dependent search but assigned to a peptide in de novo sequencing, we considered it a new spectral peptide segment. Based on the precision-recall curves (Supplementary Figure 1), the optimized cutoff score was 72. Peptides with scores > 72 are remained as confident de novo sequencing results. The software ACTG (Choi et al., 2017) was used to map peptide sequences onto genome sequences. The Proteogenomic mapping tool (Sanders et al., 2011) was used to lookup these peptide segments’ open reading frames on the genome.
Raw data of non-enzymatic samples were analyzed using pFind v3.14 with the following parameters: enzyme, no enzyme; precursor tolerance, 10 ppm; fragment tolerance, 0.02 Da; FDR set to 1%. The protein database used mouse protein data from OpenProt.
The length of microprotein was defined as less than 50, 100, or 150 amino acid in different researches (Slavoff et al., 2013; Storz et al., 2014; Ma et al., 2016). In this work, we use 150 AA as a cutoff.
Bioinformatic Analysis of Identified Microproteins
Gene ontology (GO) enrichment analysis of microproteins was processed using DAVID online bioinformatics tools5. Microprotein domain analysis was performed using the Pfam search tool6. Protein-protein interactions were obtained from the STRING database7.
Results and Discussion
Workflow of Microproteins Identification in Mouse Tissue
We selected five mouse tissues (brain, heart, liver, spleen, and kidney) to investigate tissue-specific microproteins (<150 AA). The complexity of a complete proteome makes it challenging to detect all expressed microproteins because of their short lengths and low abundance (Khatun et al., 2013). To boost the identification of microproteins, we combined three MS methods, bottom-up, top-down, and de novo sequencing (Figure 1). Because de novo sequencing uses the dataset from the bottom-up method, the bottom-up method in this work refers to the method with trypsin digestion sample preparation and database-dependent searching. For the bottom-up strategy, RIPA lysis buffer and HCl lysis buffer (defined as RIPA and HCl, respectively) were used for protein extraction. Protein mixtures were separated by glycine or tricine SDS-PAGE to reduce the sample complexity (Figure 2A). Using this bottom-up and database dependent search approach, we identified 7,120 proteins in total from all samples (Supplementary Table 1).
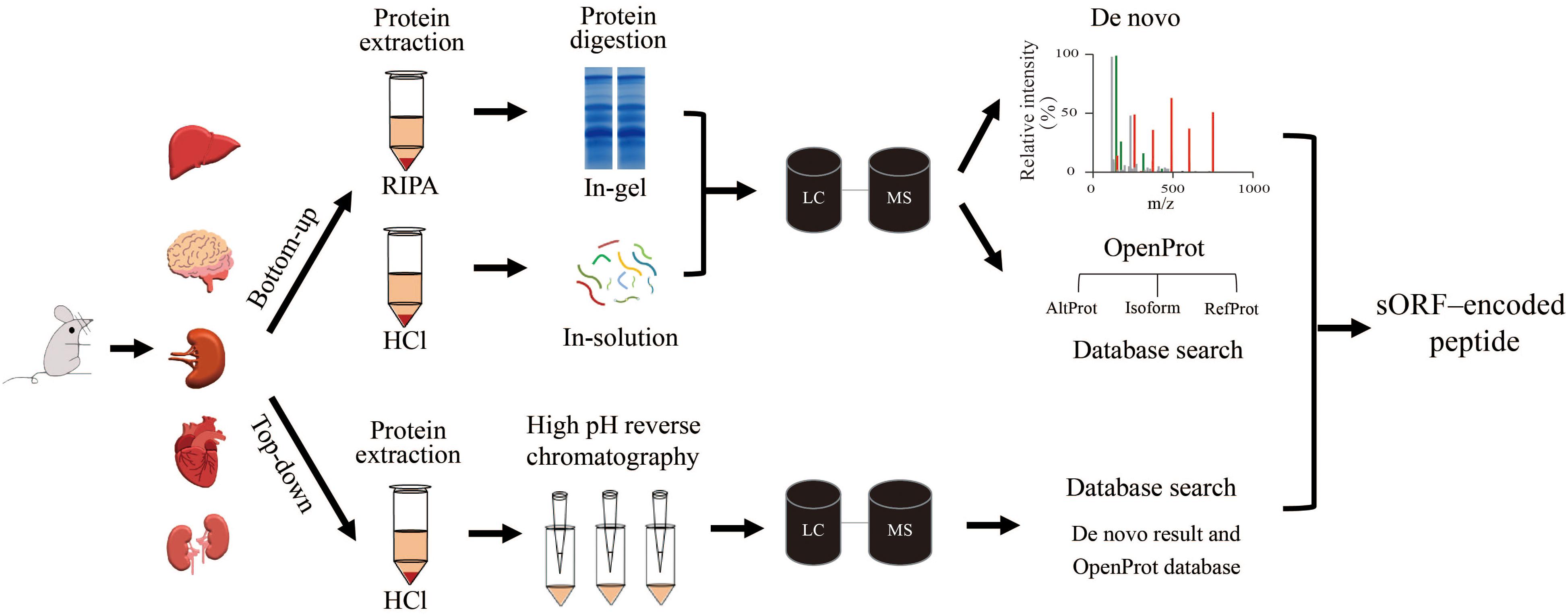
Figure 1. Workflows tested in the discovery of microproteins in different tissues. Sample preparation for the bottom-up method used two different lysis buffers: RIPA and HCl. The protein mixtures were then digested and subjected to LC/MS/MS. After that, the mass spectra were searched against the fasta file that was downloaded from the OpenProt database. Meanwhile, de novo sequencing directly determained the peptide sequence according to the spectrum without a fasta file. The top-down protein samples were fractionated with high pH reverse chromatography before LC/MS/MS analysis. The mass spectra were searched against a fasta file containing proteins from the OpenProt database and polypeptides from de novo results.
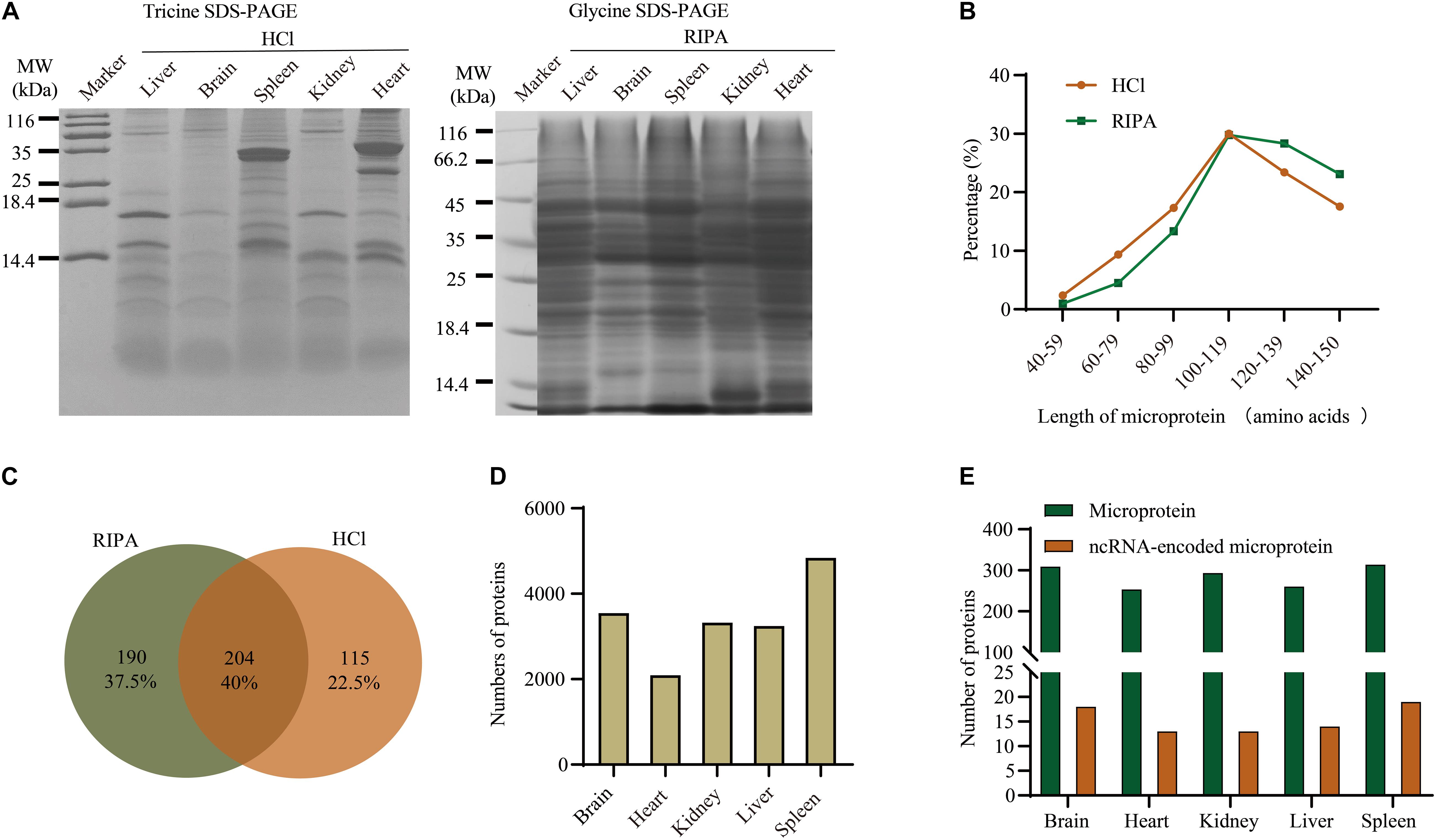
Figure 2. Overview of proteins identified in different tissues using the bottom-up method and database search. (A) Separation of total proteins from five tissues using glycine SDS-PAGE and tricine SDS-PAGE. (B) Comparison of microprotein lengths using different buffers. (C) Venn diagram of microproteins identified using RIPA and HCl buffers. (D) Numbers of detected proteins in different tissues. (E) Distribution of microproteins and ncRNA-encoded microproteins among five tissues.
The length distribution of microproteins (Figure 2B) showed that the RIPA extraction method favored proteins with a higher molecular weight than HCl extraction. This is because the HCl buffer was supposed to precipitate larger proteins in order to extract low molecular weight microproteins (Ma et al., 2016). Finally, 319 and 394 microproteins were identified from HCI and RIPA, respectively, and 204 microproteins were identified by the two methods (Figure 2C). These microproteins were unequally distributed among the five tissue types. Spleen sample with the most proteome identified also contained more microproteins and ncRNA-encoded microproteins, while heart tissue had less proteome and microproteins (Figures 2D,E).
De novo Sequencing Provides Information Regarding New Microproteins and Related sORFs
De novo sequencing obtains peptide sequences directly from the LC/MS/MS spectra, which are not limited by sequences in any database (Hughes et al., 2010). There may still be plenty of unknown gene products, especially ncRNA-encoded proteins, not in the database. So we searched our LC/MS/MS raw files with the de novo sequencing technique. In total, we found about 400 k spectra from MS, among which 152 k were interpreted by database dependent search and 305 k by de novo sequencing (Figure 3A). These spectra correspond to 200 k new peptides (Figure 3B). To obtain high confidence peptides, we filtered the de novo results with a score > 72 (Supplementary Figure 1). After that, we got 11,097 peptides from 15,158 spectra, including 550 peptides that corresponded to open reading frames in the mouse genome. These peptides belong to 526 proteins (Supplementary Table 2), including 290 microproteins (Figure 3C and Supplementary Figure 2). Among these microproteins, 121 are novel gene coding products named Denovo001 to Denovo121 (Supplementary Table 2). A total of 104 de novo microproteins were tissue-specific.
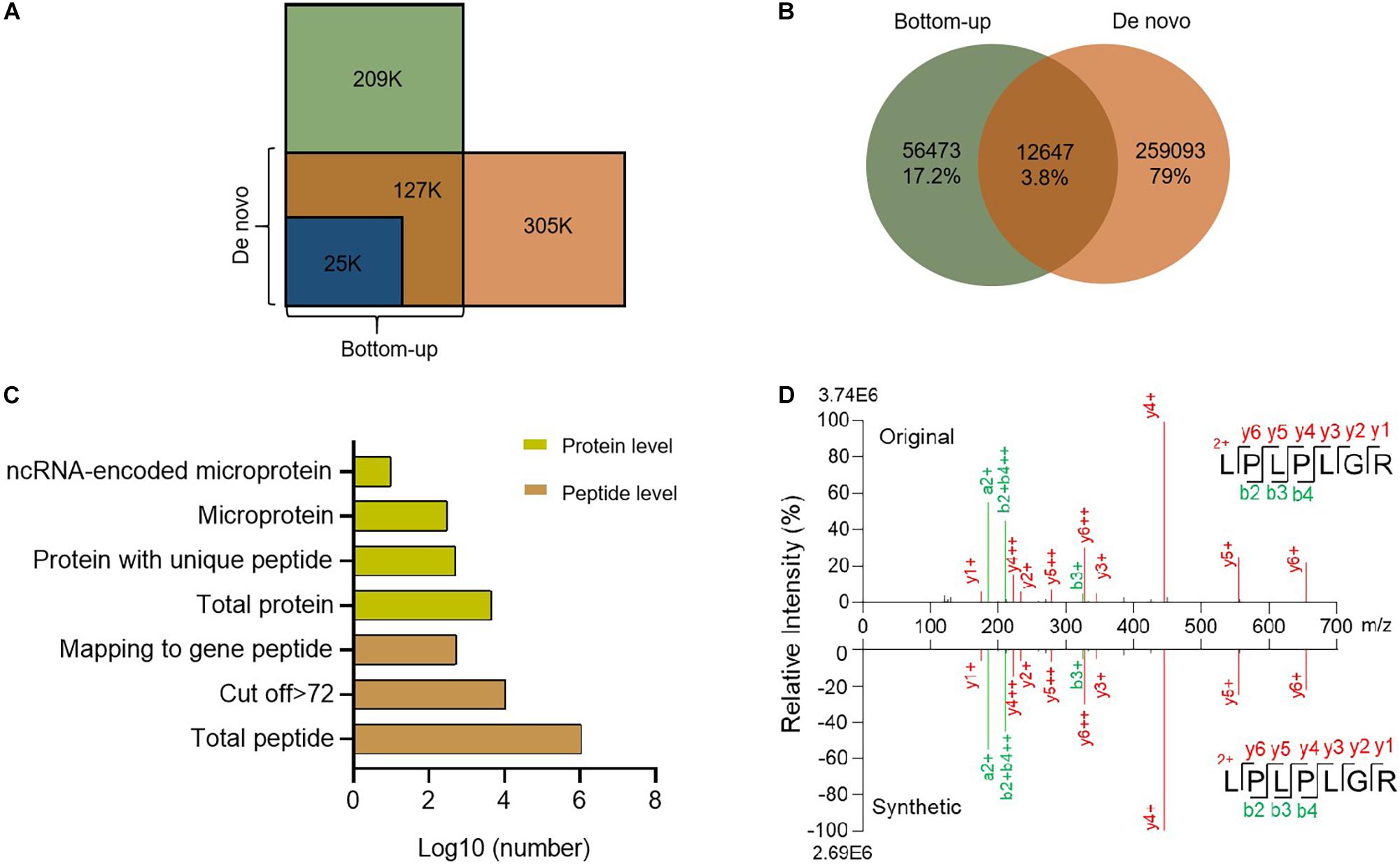
Figure 3. Summary of de novo sequencing results. (A) Comparison of spectrum counts between de novo sequencing and bottom-up database search. (B) Venn diagram of peptides between de novo sequencing and bottom-up. (C) Identification of peptides and proteins from de novo sequencing. (D) Comparison of the spectra for the de novo search and synthetic peptide.
To further confirm the quality of the de novo sequencing data, we randomly selected certain peptides for synthesis. We compared the spectra of the synthesized peptides with the de novo results and found them to be highly consistent (Figure 3D and Supplementary Figure 3), which suggested that our results were reliable. De novo sequencing identified new peptides or proteins that were not annotated in any current database (Yang et al., 2019). These novel peptides might have important functions.
High Sequence Coverage of Microproteins Identified by Top-Down Approach
Top-down is another helpful tool for identifying microproteins (Breuker et al., 2008; Ahlf et al., 2012) because it is superior to complete sequence analysis of intact protein (Cupp-Sutton and Wu, 2020). To improve the sequence coverage of microproteins, we adopted a top-down method. Without trypsin digestion, longer peptides could be identified, for example, a peptide of O08997 (Figure 4A). This microprotein contains 68 AA, and we identified its peptide with 36 AA, which provides 52.9% sequence coverage. This microprotein was also identified via the bottom-up strategy, but the coverage was only 11.8%. It was shown that the top-down strategy could greatly improve the coverage of peptide sequences. Using a top-down strategy, we detected a total of 1,238 proteins distributed among different tissues (Supplementary Table 3 and Supplementary Figure 4), including 483 microproteins and 166 encoded by ncRNA (Figure 4B). We also discovered a number of novel tissue-specific microproteins, such as IP_2438407 in the spleen and IP_1072519 in the heart, which have not been detected before. By comparing the top-down results with bottom-up and de novo sequencing results, we found that the length and the sequence coverage of the identified polypeptides were higher in the top-down approach (Figure 4C and Supplementary Figure 5). These results confirmed that the top-down strategy was more effective for longer peptide identification, thus increasing sequence coverage.
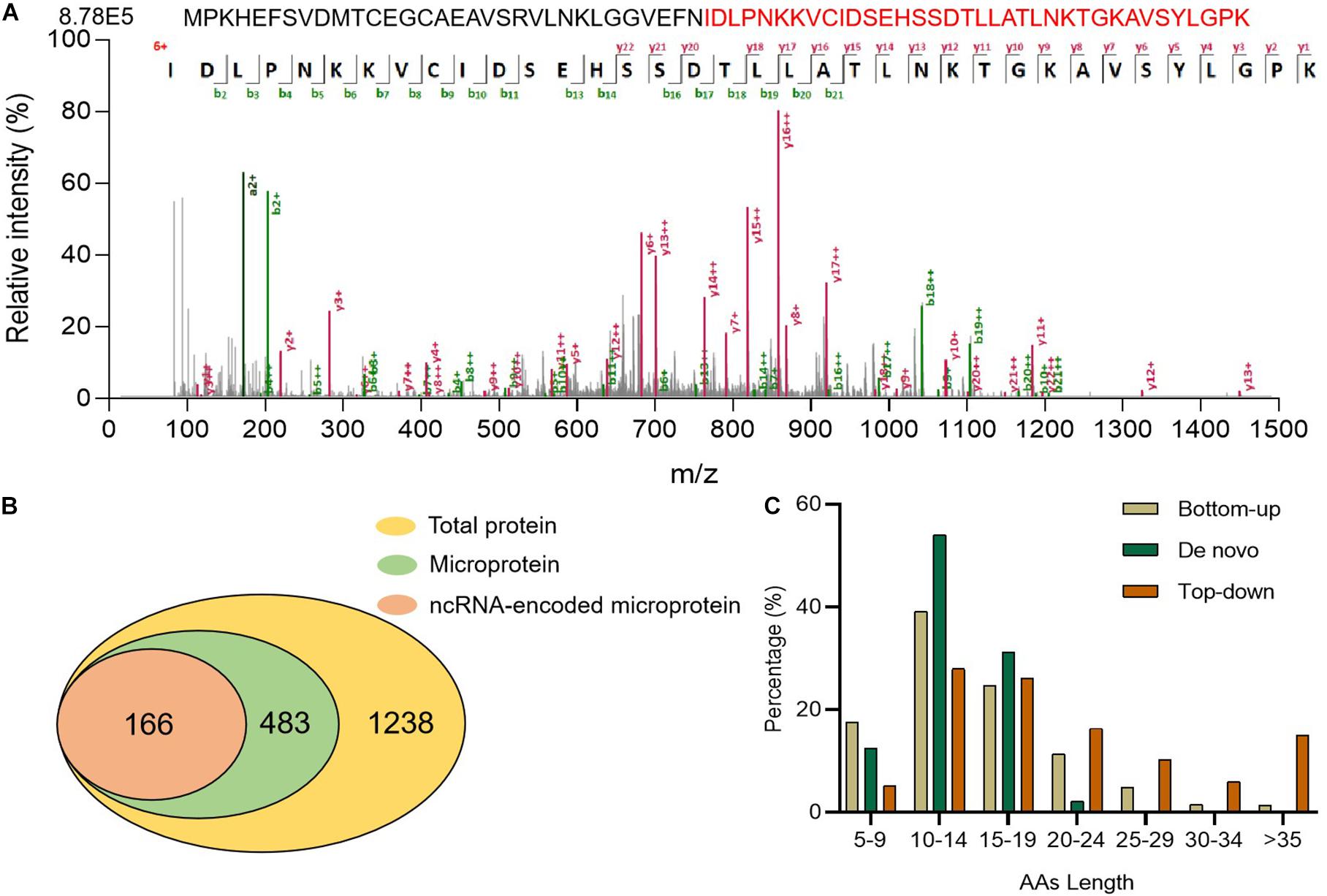
Figure 4. Proteins detected using the top-down strategy. (A) Full length O08997 and its MS/MS spectrum (B) Numbers of identified total proteins, microproteins, and ncRNA-encoded microproteins with unique peptides. (C) Comparison of microprotein AA lengths identified using three strategies.
Characteristics of Microproteins Identified in Five Mouse Tissues
Combining bottom-up, de novo sequencing, and top-down results, we identified 7,922 proteins in total, of which 1,074 were microproteins (Supplementary Table 4). These three methods collectively identified only 19 microproteins, which suggested excellent complementarity among them (Supplementary Figure 6).
A total of 207 microproteins were found in all five tissues, 518 in more than one tissue, and 556 in only one tissue (Figure 5A and Supplementary Figure 7). Brain tissue had the most tissue-specific microproteins, while the heart tissue had the least specific microproteins. 61% of the microproteins had MS evidence, and 54.2% had translation evidence according to the OpenProt database (Supplementary Figure 8). We found many novel microproteins in various tissues of mice, indicating that the data in our study can enrich the proteomic data of mice. Over half of these microproteins had predicted domains (Supplementary Figure 8), suggesting that they may have specific functions. However, half of them were non-annotated, of which 270 were encoded by ncRNAs (Figure 5B). Finally, 270 ncRNA-encoded microproteins were identified in the different tissues (Figure 5C and Supplementary Table 5). Kidney tissue had the highest number of ncRNA-encoded microproteins, and heart tissue contained minimal ncRNA-encoded microproteins. Although the focus was on microproteins, we also identified 116 ncRNA-encoded polypeptides larger than 150 AA (Supplementary Table 5). The length distribution of the ncRNA-encoded polypeptides showed that 79% of the proteins were greater than 50 AA in length (Supplementary Figure 9).
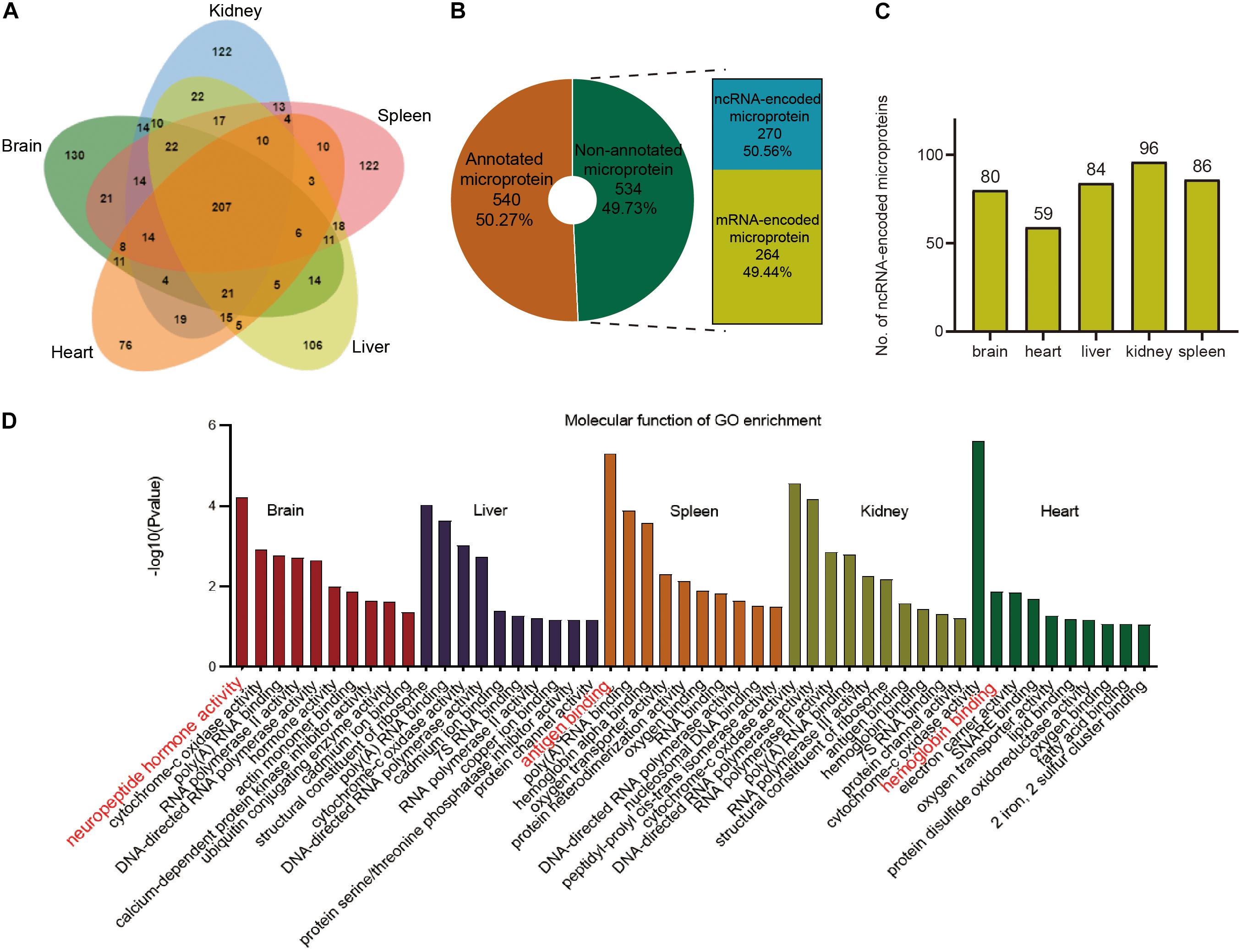
Figure 5. Overview of total microproteins and ncRNA-encoded microproteins. (A) Venn diagram of total microproteins identified in different tissues. (B) Distribution of annotated and non-annotated microproteins and the proportion of ncRNA-encoded non-annotated microproteins. (C) Distribution of ncRNA-encoded proteins across different tissues. (D) Gene ontology (GO) enrichment of the identified annotated microproteins.
To explore the functions of the annotated microproteins in various tissues, we performed the GO enrichment analysis. The results indicated that microproteins in different tissues were mostly related to tissue-specific functions (Figure 5D). For example, brain tissue has many microproteins related to nerves and hormones, which is consistent with previous research findings (Marcus et al., 2004; Davis et al., 2018). Splenic tissue was rich in immunity-related microproteins, which is consistent with previous research (Hu et al., 2018; Ma et al., 2019). Representative microproteins with tissue-specific functions and domains are presented in Table 1. From our data, we found a microprotein named Neuromedin-B specifically expressed in the brain tissue. This protein in UniProt was inferred by homology and has a Bombesin domain, which is very important in mouse brain development (Secher et al., 2016). Interestingly, a negative regulator of P-body association was identified in brain, spleen and kidney tissues. This protein has high sequence similarity with Nobody (>80%), which was originally thought to be encoded by ncRNA, and plays an essential role in regulating mRNA processing (D’Lima et al., 2017).
Tissue-Specific Microproteins Coded by ncRNAs
To determine the distribution of microproteins in various tissues, we performed label-free quantification with spectra counts (Figure 6A). So far, studies regarding SEPs have mainly focused on the identification of these peptides, and very few reports provide quantitative data concerning changes in SEP expression under different biological conditions (Cao et al., 2020; Fabre et al., 2021). Microprotein identification often has a low number of identification and low reproducibility (Cardon et al., 2020), which restricts the progress of quantification. Therefore, in this work, we only presented rough quantification results using spectral counts. From the results, we found that several microproteins, such as IP_1053598, IP_828139, IP_879384, and IP_950324, exist in multiple tissues. These four microproteins are ncRNA-encoded and structural constituents of the cytoskeleton. Some ncRNA-encoded microproteins, which may have important functions, were only identified in one tissue. To identify potential functions, we analyzed the domains of microproteins with PFAM online software8. According to domain analysis, liver-specific microproteins, IP_856169, IP_875930, and IP_973081 have glyceraldehyde-3-phosphate dehydrogenase domains. IP_873734 is specifically expressed in the heart and has a mitochondrial ATP synthesis domain.
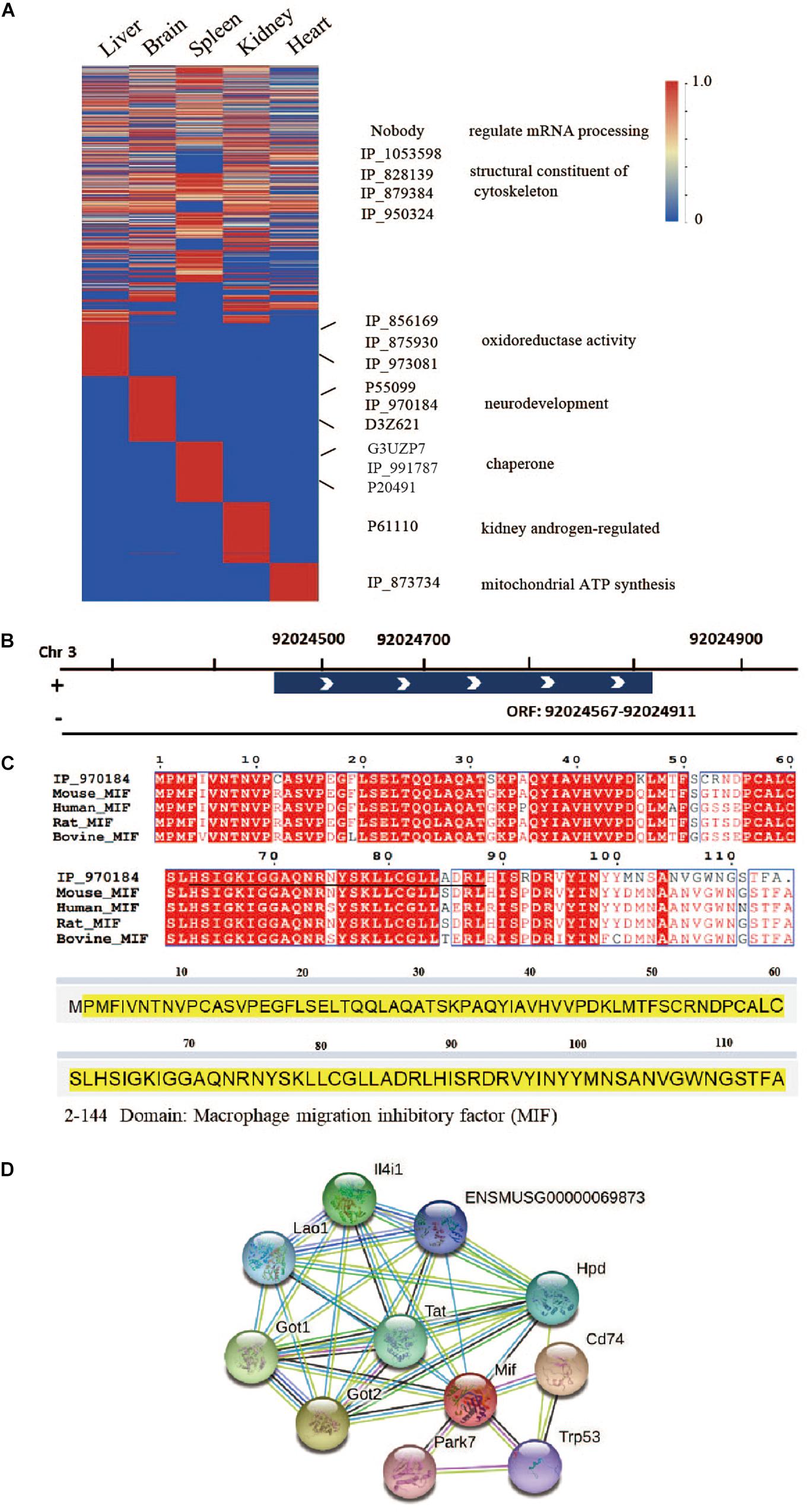
Figure 6. Tissue-specific microproteins. (A) Microprotein expression across different tissues (normalized spectral counts). (B) Location of IP_970184 on chromosome 3. (C) Sequence similarity between IP_970184 and macrophage migration inhibitory factor (MIF) across different species. The identified peptides of IP_970184 are labeled in black, and the domain sequence is highlighted in yellow. (D) Protein interactions of mouse macrophage migration inhibitory factor (MIF) from STRING database.
One interesting ncRNA-encoded microprotein IP_970184, is only found in brain tissue (Supplementary Figure 10). Its open reading frame is located on chromosome 3 (Figure 6B). This microprotein had a macrophage migration inhibitory factor (MIF) domain and quite high sequence similarity to protein MIF. Furthermore, the microprotein was also conservative across 4 species (Figure 6C). Several proteins would interacts with MIF (Figure 6D), such as aspartate aminotransferase, which is encoded by Got1 gene. Gotl is an important regulator of glutamate levels, acting as a glutamate scavenger in brain neuroprotection (Daikhin and Yudkoff, 2000). Previous studies have confirmed that MIF is critically involved in anxiety, depression, and memory-related behaviors. In addition to exerting a pro-inflammatory function, MIF expression is related to adult hippocampal neurogenesis (Conboy et al., 2011). Therefore, we believe that this ncRNA-encoded microprotein IP_970184 might have a regulatory function in the nervous system.
Another ncRNA-encoded microprotein, IP_991787, was only found in spleen tissue (Supplementary Figure 11). There is no mass spectrum or translation evidence for this microprotein in OpenProt database. Sequence alignment suggested that it has high sequence homology (>90%) to prefoldin subunit 6 with a prefoldin domain and conserved across four species (Supplementary Figure 12). The prefoldin complex is chaperone protein with multiple functions (Liang et al., 2020). A recent study proved that one novel prefolding-like microprotein, ASDURF, is a subunit of the PAQosome, which is a chaperone complex related to the biogenesis of plenty protein complexes (Cloutier et al., 2020). These results demonstrated that microprotein IP_991787 might have similar functions.
There are other interesting ncRNA-encoded polypeptides in our data, such as 88 AA IP_988951, which is expressed in all five tissues and contains NAD binding domain. Therefore, it may participate in the tricarboxylic acid cycle. Our dataset may provide useful information for future functional studies.
Conclusion
In total, we detected 1,074 microproteins in five mouse tissues. There were 556 tissue-specific microproteins in various tissues. Brain tissue had the highest number of microproteins related to nerves and hormones; spleen and kidney tissues contained more immune-related microproteins. At the same time, we have also found 386 ncRNA-encoded polypeptides, 270 of which are microproteins. Some ncRNA-encoded microproteins have functional domains or are conserved across species, indicating that these microproteins might have important functions. Our protein express dataset was only based on MS quantification. It will be better to validate the microprotein expression by western blot with specific antibodies. However, we have presented a large-scale survey of microproteins encoded by mRNA or ncRNA and mined these data to better understand the biochemical basis of tissue specificity. These results will hopefully stimulate future microprotein and ncRNA-encoded microprotein studies involving different tissues and organisms.
Data Availability Statement
The original contributions presented in the study are publicly available. This data can be found here: Integrated proteome resources accession, project ID IPX0002949000, ProteomeXchange ID PXD025158 https://www.iprox.org/page/project.html?id=IPX0002949000.
Ethics Statement
The animal study was reviewed and approved by Institutional Animal Care and Use Committee Central China Normal University.
Author Contributions
NP performed the experiments with the association of BW and JW, and wrote the manuscript. ZW did the de novo data analysis with NP. CW supervised the project, designed experiments, and wrote the manuscript. All authors read and approved the final manuscript.
Funding
This work was supported by the Natural Science Foundation of China (31800647) and self-determined research funds of CCNU from the colleges’ basic research and operation of MOE (CCNU19TD007).
Conflict of Interest
The authors declare that the research was conducted in the absence of any commercial or financial relationships that could be construed as a potential conflict of interest.
Publisher’s Note
All claims expressed in this article are solely those of the authors and do not necessarily represent those of their affiliated organizations, or those of the publisher, the editors and the reviewers. Any product that may be evaluated in this article, or claim that may be made by its manufacturer, is not guaranteed or endorsed by the publisher.
Acknowledgments
We thank Prof. Rui Li at CCNU for providing mouse samples.
Supplementary Material
The Supplementary Material for this article can be found online at: https://www.frontiersin.org/articles/10.3389/fcell.2021.687748/full#supplementary-material
Footnotes
- ^ http://www.iprox.org
- ^ http://openprot.org/
- ^ http://pfind.ict.ac.cn/software/pNovo/index.html
- ^ http://pfind.ict.ac.cn/software/pFind/index.html
- ^ https://david.ncifcrf.gov/tools.jsp
- ^ http://pfam.xfam.org/search
- ^ https://string-db.org/
- ^ http://pfam.xfam.org
References
Ahlf, D. R., Compton, P. D., Tran, J. C., Early, B. P., Thomas, P. M., and Kelleher, N. L. (2012). Evaluation of the compact high-field orbitrap for top-down proteomics of human cells. J. Proteome Res. 11, 4308–4314. doi: 10.1021/pr3004216
Anderson, D. M., Anderson, K. M., Chang, C. L., Makarewich, C. A., Nelson, B. R., McAnally, J. R., et al. (2015). A micropeptide encoded by a putative long noncoding RNA regulates muscle performance. Cell 160, 595–606. doi: 10.1016/j.cell.2015.01.009
Breuker, K., Jin, M., Han, X., Jiang, H., and McLafferty, F. W. (2008). Top-down identification and characterization of biomolecules by mass spectrometry. J. Am. Soc. Mass Spectrom. 19, 1045–1053. doi: 10.1016/j.jasms.2008.05.013
Budamgunta, H., Olexiouk, V., Luyten, W., Schildermans, K., Maes, E., Boonen, K., et al. (2018). Comprehensive peptide pnalysis of mouse brain striatum identifies novel sORF-encoded polypeptides. Proteomics 18:e1700218. doi: 10.1002/pmic.201700218
Cabili, M. N., Trapnell, C., Goff, L., Koziol, M., Tazon-Vega, B., Regev, A., et al. (2011). Integrative annotation of human large intergenic noncoding RNAs reveals global properties and specific subclasses. Genes Dev. 25, 1915–1927. doi: 10.1101/gad.17446611
Cao, X., Khitun, A., Na, Z., Dumitrescu, D. G., Kubica, M., Olatunji, E., et al. (2020). Comparative proteomic profiling of unannotated microproteins and alternative proteins in human cell lines. J. Proteome Res. 19, 3418–3426. doi: 10.1021/acs.jproteome.0c00254
Cardon, T., Hervé, F., Delcourt, V., Roucou, X., Salzet, M., Franck, J., et al. (2020). Optimized sample preparation workflow for improved identification of ghost proteins. Anal. Chem. 92, 1122–1129. doi: 10.1021/acs.analchem.9b04188
Choi, S., Kim, H., and Paek, E. (2017). ACTG: novel peptide mapping onto gene models. Bioinformatics 33, 1218–1220. doi: 10.1093/bioinformatics/btw787
Cloutier, P., Poitras, C., Faubert, D., Bouchard, A., Blanchette, M., Gauthier, M. S., et al. (2020). Upstream ORF-nncoded ASDURF is a novel prefoldin-like subunit of the PAQosome. J. Proteome Res. 19, 18–27. doi: 10.1021/acs.jproteome.9b00599
Conboy, L., Varea, E., Castro, J. E., Sakouhi-Ouertatani, H., Calandra, T., Lashuel, H. A., et al. (2011). Macrophage migration inhibitory factor is critically involved in basal and fluoxetine-stimulated adult hippocampal cell proliferation and in anxiety, depression, and memory-related behaviors. Mol. Psychiatry 16, 533–547. doi: 10.1038/mp.2010.15
Cupp-Sutton, K. A., and Wu, S. (2020). High-throughput quantitative top-down proteomics. Mol. Omics. 16, 91–99. doi: 10.1039/c9mo00154a
Daikhin, Y., and Yudkoff, M. (2000). Compartmentation of brain glutamate metabolism in neurons and glia. J. Nutr. 130, 1026S–1031S. doi: 10.1093/jn/130.4.1026S
Davis, R. G., Park, H. M., Kim, K., Greer, J. B., Fellers, R. T., Romanova, E. V., et al. (2018). Top-down proteomics enables comparative analysis of brain proteoforms between mouse Strains. Anal. Chem. 90, 3802–3810. doi: 10.1021/acs.analchem.7b04108
D’Lima, N. G., Ma, J., Winkler, L., Chu, Q., Loh, K. H., Corpuz, E. O., et al. (2017). A human microprotein that interacts with the mRNA decapping complex. Nat. Chem. Biol. 13, 174–180. doi: 10.1038/nchembio.2249
Fabre, B., Combier, J. P., and Plaza, S. (2021). Recent advances in mass spectrometry-based peptidomics workflows to identify short-open-reading-frame-encoded peptides and explore their functions. Curr. Opin. Chem. Biol. 60, 122–130. doi: 10.1016/j.cbpa.2020.12.002
Frith, M. C., Forrest, A. R., Nourbakhsh, E., Pang, K. C., Kai, C., Kawai, J., et al. (2006). The abundance of short proteins in the mammalian proteome. PLoS Genet. 2:e52. doi: 10.1371/journal.pgen.0020052
He, C. T., Jia, C. X., Zhang, Y., and Xu, P. (2018). Enrichment-based proteogenomics identifies microproteins, missing proteins, and novel smORFs in Saccharomyces cerevisiae. J. Proteome Res. 17, 2335–2344. doi: 10.1021/acs.jproteome.8b00032
Hu, Z., Gu, H., Hu, J., Hu, S., Wang, X., Liu, X., et al. (2018). Quantitative proteomics identify an association between extracellular matrix degradation and immunopathology of genotype VII Newcastle disease virus in the spleen in chickens. J. Proteomics. 181, 201–212. doi: 10.1016/j.jprot.2018.04.019
Huang, J., Chen, M., Chen, D., Gao, X., Zhu, S., Huang, H., et al. (2017). A peptide encoded by a putative lncRNA HOXB-AS3 suppresses colon cancer growth. Mol. Cell 68, 171–184.e6. doi: 10.1016/j.molcel.2017.09.015
Hughes, C., Ma, B., and Lajoie, G. A. (2010). De novo sequencing methods in proteomics. Methods Mol. Biol. 604, 105–121. doi: 10.1007/978-1-60761-444-9_8
Ingolia, N. (2014). Ribosome profiling: new views of translation, from single codons to genome scale. Nat. Rev. Genet. 15, 205–213. doi: 10.1038/nrg3645
Isakova, A., Fehlmann, T., Keller, A., and Quake, S. R. (2020). A mouse tissue atlas of small noncoding RNA. Proc. Natl. Acad. Sci. U.S.A. 117, 25634–25645. doi: 10.1073/pnas.2002277117
Jackson, R., Kroehling, L., Khitun, A., Bailis, W., Jarret, A., York, A. G., et al. (2018). The translation of non-canonical open reading frames controls mucosal immunity. Nature 564, 434–438. doi: 10.1038/s41586-018-0794-7
Khatun, J., Yu, Y., Wrobel, J. A., Risk, B. A., Gunawardena, H. P., Secrest, A., et al. (2013). Whole human genome proteogenomic mapping for ENCODE cell line data: identifying protein-coding regions. BMC Genomics 14:141. doi: 10.1186/1471-2164-14-141
Kondo, T., Hashimoto, Y., Kato, K., Inagaki, S., Hayashi, S., and Kageyama, Y. (2007). Small peptide regulators of actin-based cell morphogenesis encoded by a polycistronic mRNA. Nat. Cell Biol. 9, 660–665. doi: 10.1038/ncb1595
Landgraf, P., Rusu, M., Sheridan, R., Sewer, A., Iovino, N., Aravin, A., et al. (2007). A mammalian microRNA expression atlas based on small RNA library sequencing. Cell 129, 1401–1414. doi: 10.1016/j.cell.2007.04.040
Lee, C., Zeng, J., Drew, B. G., Sallam, T., Martin-Montalvo, A., Wan, J., et al. (2015). The mitochondrial-derived peptide MOTS-c promotes metabolic homeostasis and reduces obesity and insulin resistance. Cell Metab. 21, 443–454. doi: 10.1016/j.cmet.2015.02.009
Li, P., Ruan, X., Yang, L., Kiesewetter, K., Zhao, Y., Luo, H., et al. (2015). A liver-enriched long non-coding RNA, lncLSTR, regulates systemic lipid metabolism in mice. Cell Metab. 21, 455–467. doi: 10.1016/j.cmet.2015.02.004
Li, W., Petruzziello, F., Zhao, N., Zhao, H., Ye, X., Zhang, X., et al. (2017). Separation and identification of mouse brain tissue microproteins using top-down method with high resolution nanocapillary liquid chromatography mass spectrometry. Proteomics 17:1600419. doi: 10.1002/pmic.201600419
Liang, J., Xia, L., Oyang, L., Lin, J., Tan, S., Yi, P., et al. (2020). The functions and mechanisms of prefoldin complex and prefoldin-subunits. Cell Biosci. 10:87. doi: 10.1186/s13578-020-00446-8
Liang, Y., Ridzon, D., Wong, L., and Chen, C. (2007). Characterization of microRNA expression profiles in normal human tissues. BMC Genomics 8:166. doi: 10.1186/1471-2164-8-166
Lu, S., Wang, T., Zhang, G., and He, Q. (2020). Understanding the proteome encoded by “non-coding RNAs”: new insights into human genome. Sci. China Life Sci. 63, 986–995. doi: 10.1007/s11427-019-1677-8
Ma, D., Liu, Q., Zhang, M., Feng, J., Li, X., Zhou, Y., et al. (2019). iTRAQ-based quantitative proteomics analysis of the spleen reveals innate immunity and cell death pathways associated with heat stress in broilers (Gallus gallus). J. Proteomics 196, 11–21. doi: 10.1016/j.jprot.2019.01.012
Ma, J., Diedrich, J. K., Jungreis, I., Donaldson, C., Vaughan, J., Kellis, M., et al. (2016). Improved identification and analysis of small open reading frame encoded polypeptides. Anal. Chem. 88, 3967–3975. doi: 10.1021/acs.analchem.6b00191
Magny, E. G., Pueyo, J. I., Pearl, F. M., Cespedes, M. A., Niven, J. E., Bishop, S. A., et al. (2013). Conserved regulation of cardiac calcium uptake by peptides encoded in small open reading frames. Science 341, 1116–1120. doi: 10.1126/science.1238802
Marcus, K., Schmidt, O., Schaefer, H., Hamacher, M., van Hall, A., and Meyer, H. E. (2004). Proteomics–application to the brain. Int. Rev. Neurobiol. 61, 285–311. doi: 10.1016/S0074-7742(04)61011-7
Nelson, B. R., Makarewich, C. A., Anderson, D. M., Winders, B. R., Troupes, C. D., Wu, F., et al. (2016). A peptide encoded by a transcript annotated as long noncoding RNA enhances SERCA activity in muscle. Science 351, 271–275. doi: 10.1126/science.aad4076
Pang, Y., Liu, Z., Han, H., Wang, B., Li, W., Mao, C., et al. (2020). Peptide SMIM30 promotes HCC development by inducing SRC/YES1 membrane anchoring and MAPK pathway activation. J. Hepatol. 73, 1155–1169. doi: 10.1016/j.jhep.2020.05.028
Ruiz-Orera, J., Messeguer, X., Subirana, J. A., and Alba, M. M. (2014). Long non-coding RNAs as a source of new peptides. Elife 3:e03523. doi: 10.7554/eLife.03523
Sanders, W. S., Wang, N., Bridges, S. M., Malone, B. M., Dandass, Y. S., McCarthy, F. M., et al. (2011). The proteogenomic mapping tool. BMC Bioinformatics 12:115. doi: 10.1186/1471-2105-12-115
Secher, A., Kelstrup, C. D., Conde-Frieboes, K. W., Pyke, C., Raun, K., Wulff, B. S., et al. (2016). Analytic framework for peptidomics applied to large-scale neuropeptide identification. Nat. Commun. 7:11436. doi: 10.1038/ncomms11436
Slavoff, S. A., Mitchell, A. J., Schwaid, A. G., Cabili, M. N., Ma, J., Levin, J. Z., et al. (2013). Peptidomic discovery of short open reading frame-encoded peptides in human cells. Nat. Chem. Biol. 9, 59–64. doi: 10.1038/nchembio.1120
Storz, G., Wolf, Y. I., and Ramamurthi, K. S. (2014). Small proteins can no longer be ignored. Annu. Rev. Biochem. 83, 753–777. doi: 10.1146/annurev-biochem-070611-102400
van Heesch, S., Witte, F., Schneider-Lunitz, V., Schulz, J. F., Adami, E., Faber, A. B., et al. (2019). The translational landscape of the human heart. Cell 178, 242–260. doi: 10.1016/j.cell.2019.05.010
Wang, B., Hao, J., Pan, N., Wang, Z., Chen, Y., and Wan, C. (2021a). Identification and analysis of small proteins and short open reading frame encoded peptides in Hep3B cell. J. Proteomics. 230:103965. doi: 10.1016/j.jprot.2020.103965
Wang, B., Wang, Z., Pan, N., Huang, J., and Wan, C. (2021b). Improved identification of small open reading frames encoded peptides by top-down proteomic approaches and de novo sequencing. Int. J. Mol. Sci. 22:5476. doi: 10.3390/ijms22115476
Wang, Y., Wu, S., Zhu, X., Zhang, L., Deng, J., Li, F., et al. (2020). LncRNA-encoded polypeptide ASRPS inhibits triplenegative breast cancer angiogenesis. J. Exp. Med. 217:jem.20190950. doi: 10.1084/jem.20190950
Keywords: small open reading frame, non-coding RNAs, de novo sequencing, top-down, mouse tissue
Citation: Pan N, Wang Z, Wang B, Wan J and Wan C (2021) Mapping Microproteins and ncRNA-Encoded Polypeptides in Different Mouse Tissues. Front. Cell Dev. Biol. 9:687748. doi: 10.3389/fcell.2021.687748
Received: 30 March 2021; Accepted: 30 June 2021;
Published: 26 July 2021.
Edited by:
Marie A. Brunet, Université de Sherbrooke, CanadaReviewed by:
Xavier Roucou, Université de Sherbrooke, CanadaSarah Slavoff, Yale University, United States
Copyright © 2021 Pan, Wang, Wang, Wan and Wan. This is an open-access article distributed under the terms of the Creative Commons Attribution License (CC BY). The use, distribution or reproduction in other forums is permitted, provided the original author(s) and the copyright owner(s) are credited and that the original publication in this journal is cited, in accordance with accepted academic practice. No use, distribution or reproduction is permitted which does not comply with these terms.
*Correspondence: Cuihong Wan, Y2hfd2FuQG1haWwuY2NudS5lZHUuY24=