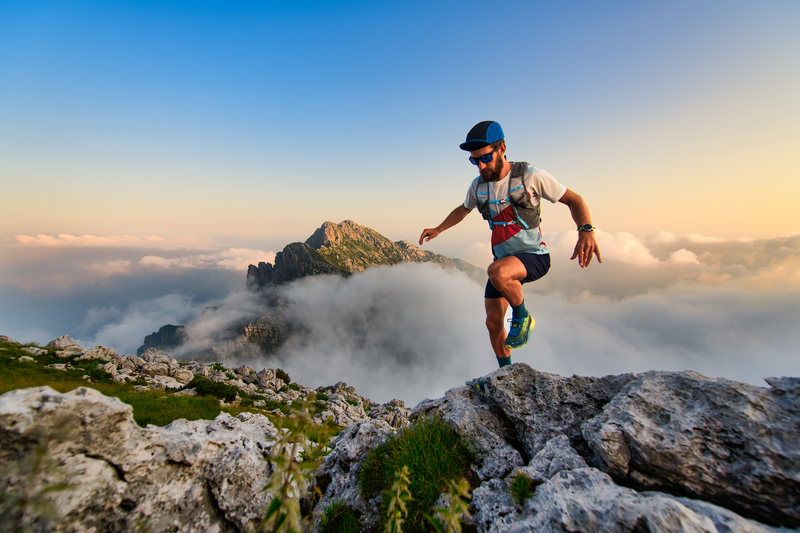
95% of researchers rate our articles as excellent or good
Learn more about the work of our research integrity team to safeguard the quality of each article we publish.
Find out more
ORIGINAL RESEARCH article
Front. Cell Dev. Biol. , 30 June 2021
Sec. Molecular and Cellular Pathology
Volume 9 - 2021 | https://doi.org/10.3389/fcell.2021.681745
This article is part of the Research Topic Tumor Microenvironment in Cancer Hallmarks and Therapeutics View all 57 articles
Recently, N6-methyladenosine (m6A) RNA methylation in eukaryotic mRNA has become increasingly obvious in the pathogenesis and prognosis of cancer. Moreover, tumor microenvironment is involved in the regulation of tumorigenesis. In our research, the clinical data, including 374 tumor and 50 normal patients, were obtained from The Cancer Genome Atlas (TCGA). Then 19 m6A regulators were selected from other studies. Hepatocellular carcinoma (HCC) patients were clustered in cluster1/2, according to the consensus clustering for the m6A RNA regulators. We found that m6A regulators were upregulated in cluster1. The cluster1 was associated with higher programmed death ligand 1 (PD-L1) expression level, higher immunoscore, worse prognosis, and distinct immune cell infiltration compared with cluster2. Five risk signatures were identified, including YTH N6-methyladenosine RNA-binding protein 1, YTHDF2, heterogeneous nuclear ribonucleoprotein C, WT1-associated protein, and methyltransferase-like 3, based on univariate Cox and least absolute shrinkage and selection operator regression analysis. High-risk group and low-risk group HCC patients were selected based on the risk score. Similarly, the high-risk group was extremely associated with higher PD-L1 expression level, higher grade, and worse overall survival (OS). Also, cluster1 was mainly enriched in high-risk group. Receiver operating characteristic (ROC) and a nomogram were used to predict the ability and the probability of 3- and 5-year OS of HCC patients. The time-dependent ROC curve (AUC) reached 0.77, 0.67, and 0.68 at 1, 3, and 5 years in the training dataset. Also, AUC areas of 1, 3, and 5 years were 0.7, 0.63, and 0.55 in the validation dataset. The gene set enrichment analysis showed that MTOR signaling pathway and WNT signaling pathway were correlated with cluster1 and high-risk group. Collectively, the research showed that the m6A regulators were significantly associated with tumor immune microenvironment in HCC. Risk characteristics based on m6A regulators may predict prognosis in patients with HCC and provide a new therapeutic target for improving the efficacy of immunotherapy.
Hepatocellular carcinoma (HCC) is one of the most prevalent cancers in the world and the fourth most deadly cancer (Forner et al., 2012; Bray et al., 2018). In China alone, more than 466,100 people are diagnosed with HCC, and approximately 422,100 individuals succumb to HCC (Chen et al., 2016). Behind the high incidence of HCC, there are several modifiable factors, including hepatitis virus, alcohol abuse, smoking, and metabolic syndrome. Especially the hepatitis virus, in most of Africa and Asia, such as China, hepatitis B virus is the single leading risk factor for HCC, whereas in northern Europe and the United States, hepatitis C virus is the major risk factor (El-Serag, 2012). According to the location and clinical stage of HCC, the main treatment methods include surgery, chemotherapy, and radiotherapy, but the prognosis of advanced HCC is poor and treatment methods are limited. Interestingly, immunotherapy has developed rapidly in the past few years, improving survival of patients with HCC (Zhong et al., 2021). However, only a few patients with HCC could benefit from this treatment, and most patients still respond negatively to immune therapies. Immune system imbalance is involved in the development of immune-characterized HCC. For example, activation and ability of NK cells are reduced in HCC patients. Tumor-associated neutrophils are significantly associated with the development of HCC. Postoperative HCC patients with high-level lymphocyte infiltration, especially T cells, are closely related to better prognosis (Unitt et al., 2006; Margetts et al., 2018; Fu et al., 2019). Therefore, to identify more biomarkers for accurate prediction of prognosis and to optimize individualized immunotherapy management to a large extent, the mechanism of tumor immune microenvironment (TIME) needs to be further explored.
Post-transcriptional modification is also involved in the progression of various diseases and has attracted significant attention in the biomedicine (He et al., 2019). N6-methyladenosine (m6A) is the methylated modification of the sixth N atom of adenine and the most abundant mRNA modification among numerous RNA modifications. The average 1,000 nt contain one or two m6A residues (Krug et al., 1976; Du et al., 2019). There are three types in the m6A regulators, including writers, erasers, and readers. The m6A is catalyzed by the methyltransferase complex (MTC), also known as the “writer,” which included methyltransferase-like 3 (METTL3), METTL14, METTL16, WT1-associated protein (WTAP), zinc finger CCCH domain-containing protein 13 (ZC3H13), ZCCHC4, KIAA1429, zinc finger protein (ZFP217), RNA-binding motif protein 15 (RBM15), and RBM15B (Ping et al., 2014; Patil et al., 2016; Wang et al., 2016, 2017; Warda et al., 2017; Knuckles et al., 2018; Yue et al., 2018; Song et al., 2019; Pinto et al., 2020). Demethylase, also termed as “eraser,” comprising fat mass- and obesity-associated protein (FTO) and α-ketoglutarate-dependent dioxygenase alkB homolog 5 (ALKBH5), removes m6A methylation groups from RNA (Zhao et al., 2014; Zhang et al., 2017). The “readers” bind to the m6A methylation site, which include YTH domain-containing 1 (YTHDC1), YTHDC2, heterogeneous nuclear ribonucleoprotein C (HNRNPC), HNRNPA2B1 YTH N6-methyladenosine RNA-binding protein 1 (YTHDF1), YTHDF2, and YTHDF3 (Wang et al., 2014, 2015; Alarcón et al., 2015; Liu et al., 2015; Hsu et al., 2017; Shi et al., 2017; Kasowitz et al., 2018). In HCC, patients with higher levels of YTHDF1 and METTL3 are associated with worse overall survival (OS). YTHDF1 can mediate the m6A to enhance Snail expression. METTL3 deficiency leads to a decrease in m6A, which blocks the EMT of cancer cells (Lin et al., 2019; Zhou et al., 2019). However, the mechanisms of other m6A methylation regulators in liver cancer remain unclear. Moreover, the correlation between m6A methylation modulator and programmed death ligand 1 (PD-L1) remains to be fully explored.
In this research, the relationship of m6A RNA methylation regulators with PD-L1, prognosis, and TIME in HCC was analyzed. In addition, we established a cluster subtype and risk model for m6A regulators to identify novel HCC markers and novel therapeutic strategies (Supplementary Figure 1).
The HCC clinical data and the mRNA expression data of patients were obtained from The Cancer Genome Atlas (TCGA) data portal.1 The research included 374 tumor and 50 normal samples. The TCGA data were downloaded by using the R package “TCGAbiolinks” (Colaprico et al., 2016).
In the research, 19 m6A regulators were selected. HCC patients were clustered into cluster1 (n = 166) and cluster2 (n = 169) by using R package “ConsensusClusterPlus”.2 Furthermore, we used univariate Cox analysis and least absolute shrinkage and selection operator (LASSO) regression to identify five risk signatures, including YTHDF1, YTHDF2, HNRNPC, WTAP, and METTL3, and a risk score was generated for each HCC patient. Kaplan–Meier curves and receiver operating characteristic (ROC) curves were used to assess the prognostic capacity of the risk scores.
The R package “estimate” was used to calculate the immunoscore for each patient with the ESTIMATE algorithm. The fraction of 22 immune cell types between cluster1 and cluster2 and the gene set enrichment analysis (GSEA) were analyzed through Sangerbox website.3 Also, the effect of somatic copy number change (CNA) based on m6A modulator signal on immune cell infiltration was explored based on CIBERSORT.4
A nomogram was built to investigate the probability of prognosis in patients (Iasonos et al., 2008). Then the discrimination and accuracy of the nomogram were assessed by the concordance index (C-index) and a calibration.
Statistical tests were carried out using GraphPad Prism 8.0 (GraphPad Software, San Diego, CA, United States) and R version 4.0.2 (version 4.0.25). “limma,” “ConsensusClusterPlus,” “survival,” “glmnet,” “edgeR,” and “timeROC” R package were used.
By comparing 50 normal and 374 tumor tissues, METTL14, YTHDC1, ZC3H13, ALKBH5, YTHDF2, and RBM15 had extremely lower expression in tumor tissues (p < 0.001, Figure 1). Also, the expression levels of YTHDC2, WTAP, and FTO were markedly lower in tumor tissues (p < 0.05). On the contrary, METTL3, KIAA1429, RBM15B, HNRNPA2B1, and HNRNPC had significantly higher expression in tumor tissues (p < 0.001). The results showed that m6A regulators could be involved in biological development of HCC.
Figure 1. Expression levels of m6A RNA methylation regulators in normal and tumor samples. *p < 0.05 and ***p < 0.001.
It is determined that k = 2 has the best clustering stability from k = 2 to 9, based on the similarity between the expression level of m6A regulators and the proportion of fuzzy clustering measures (Supplementary Figure 2). According to the expression levels of the m6A regulators, 335 HCC patients were clustered into cluster1 and cluster2 (n1 = 166, n2 = 169, Figure 2A). The findings indicated that the expression level of individual m6A methylation regulators in cluster1 was higher than in cluster2 (Figure 2B). Moreover, the clinical futures were compared between cluster1 and cluster2. Female and low immunoscore HCC patients were significantly enriched in cluster1 (p < 0.05, Figure 2B). The OS (OS, p = 0.0022) and progression-free survival (PFS, p = 0.0013) were worse in cluster1 (Figures 2C,D).
Figure 2. Correlation of consensus clustering for m6A RNA methylation regulators with the characteristics and survival of patients with HCC. (A) Consensus clustering matrix for k = 2. (B) Heatmap of correlation of m6A RNA methylation regulators with characteristics of HCC patients. (C,D) OS and PFS of HCC patients in cluster1 and cluster2. *p < 0.05.
It showed that the expression of PD-L1 was dramatically higher in cluster1 (Figure 3A). Furthermore, PD-L1 was positively correlated with ZCCHC4, WTAP, YTHDF2, RBM15, METTL3, YTHDF1, RBM15B, YTHDC1, HNRNPA2B1, HNRNPC, KIAA1429, ALKBH5, and METTL16 (p < 0.001, Figure 3C).
Figure 3. Association of PD-L1 and immune cell infiltration with m6A RNA methylation regulators. (A) Expression level of PD-L1 and immunoscore in cluster1 and cluster2. (B) GSEA showed that mTOR and Wnt signaling pathways were enriched in cluster1. (C) Correction between PD-L1 and m6A RNA regulators. (D) Expression levels of 22 immune cell infiltration in cluster1 and cluster2. *p < 0.05, **p < 0.01, and ***p < 0.001.
To analyze the correction between m6A regulators and TIME in HCC, we analyzed the immunoscore and immune infiltrate level of two subgroups (Figure 3A). The immunoscore was higher in cluster2 with a longer prognosis (p = 0.0265). Then we analyzed infiltration levels of 22 immune cell types between cluster1 and cluster2 (Figure 3D). The finding indicated that the infiltration levels of naïve B cells, T follicular helper cells, and macrophages M0 were higher in cluster1 (p < 0.05), whereas cluster2 showed higher infiltration levels of macrophages M2 (p < 0.05). To elucidate the underlying regulatory mechanisms that lead to temporal differences between cluster1 and cluster2, GSEA was used. Finally, the results indicated that mTOR and Wnt signaling pathways were correlated with cluster1 (Figure 3B).
First, 340 HCC patients were randomly divided into validation dataset (170 patients) and training dataset (170 patients). Second, in the training dataset, 13 m6A regulators were selected by using univariate regression analysis. Then five m6A regulators were identified based on the LASSO regression analysis, including YTHDF1, YTHDF2, HNRNPC, WTAP, and METTL3 (Figure 4). Subsequently, these candidate m6A regulators integrated into a predictive signature based on their risk coefficients. The formula went as follows: Risk Score = (0.4111 × YTHDF1 Expression) + (0.1969 × YTHDF2 Expression) + (0.0930 × HNRNPC Expression) + (0.2004 × WTAP Expression) + (0.3277 × METTL3 Expression). Afterward, according to the median risk score, patients were divided into high- and low-risk groups. The distributions of five m6A regulators’ expression profiles are shown in Figure 5A. The heatmap revealed higher expression levels of these m6A regulators in the high-risk group compared with the low-risk group (Figure 5A). The high-risk group had worse prognosis, compared with the low-risk group, based on Kaplan–Meier curve analysis (Figure 5C). The results were validated in the validation dataset (Supplementary Figure 3C). Moreover, we constructed a time-dependent ROC curve (AUC). As shown in Figure 5B, the AUC of five risk signatures was 0.77, 0.67, and 0.68 at 1, 3, and 5 years. Regarding the validation dataset, the 1-, 3-, and 5-year AUC values were 0.7, 0.63, and 0.55 (Supplementary Figure 3B). The results revealed that five risk signatures had a strong predictive ability in the prognosis of HCC.
Figure 4. Identification of five m6A regulator-based risk signatures. (A,B) LASSO analysis of m6A regulator-based risk signatures. (C) Multivariate Cox analysis of the five m6A regulator-based risk signatures. #The five regulators have strong predictive ability and the results has statistical significance.
Figure 5. Construction of prognostic signatures for m6A regulator-based risk signatures. (A) Association of status and five m6A RNA regulators with risk score. (B) The area under AUC of five risk signatures in training dataset. (C) OS of high-risk group and low-risk group in training dataset.
Furthermore, we aimed to explore the correction between risk score and clinical characteristics in the training dataset (Figure 6A). The heatmap showed that the high-risk group mainly contained cluster1 (p < 0.001), alive status (p < 0.001), and high-grade patients (p < 0.05). Then we found that YTHDF1, YTHDF2, HNRNPC, WTAP, and METTL3 had higher expression in high-risk group. Also, PD-L1 was expressed higher in high-risk group with worse OS, which was validated in the validation dataset (Figure 6C). Similarly, mTOR and Wnt signaling pathways were enriched in the high-risk group (Figure 6D). Then we built a nomogram for HCC patients to investigate the probability of 3- and 5-year OS. The results demonstrated the risk score could be a prognostic biomarker for HCC patients (Figure 6B). Finally, we found that the C-index was 0.738 and the calibration curve was close to the ideal curve, which indicated that the nomogram has good predictive effects (Supplementary Figure 4).
Figure 6. Association of clinical features and functional features with m6A regulator-based risk signatures. (A) Correction between clinical features and five m6A regulator-based risk signatures. (B) A nomogram for HCC patients. (C) Expression level of PD-L1 in high-risk and low-risk groups. (D) GSEA showed that mTOR and Wnt signaling pathways were enriched in high-risk group. *p < 0.05, **p < 0.01, and ***p < 0.001.
The correlation between risk score and immune cell infiltration was further analyzed (Figure 7). The risk score had a negative correction with infiltration levels of macrophages M2 and resting memory CD4 T cells (p < 0.05). Then the risk score was significantly corrected with B-cell memory, naïve B cells, T follicular helper cells, and eosinophils (p < 0.05). The results confirmed that the risk signatures based on m6A regulators were related to the HCC immune microenvironment. Moreover, to clarify the potential mechanism of risk score and different immune cell infiltration, the effect of somatic CNA based on m6A modulator signal on immune cell infiltration was analyzed (Figure 8). The CNAs of m6A regulatory factor signaling, mainly including deep deletion and arm-level deletion, could affect the infiltration levels of B cells, CD8+ T cells, CD4+ T cells, neutrophils, dendritic cells, and especially macrophages (p < 0.05). It revealed that the five m6A regulators play an important role in TIME of HCC patients.
The m6A regulates gene expression, which regulates cellular processes such as cell self-proliferation, differentiation, invasion, and apoptosis (He et al., 2019). The m6A is immobilized by m6A methyltransferase, removed by m6A demethylases, and recognized by the reader proteins to regulate RNA metabolism and progression of various tumors (Li et al., 2017; Ma et al., 2017; Roignant and Soller, 2017). However, the role of some m6A regulators in tumor is unclear. For example, METTL14 plays different roles in different types of tumors. Yang et al. (2020) showed that METTL14 inhibits the proliferation and metastasis of colorectal cancer by downregulating the oncogenic long non-coding RNA XIST. Wang M. et al. (2020) reported that upregulation of METTL14 promotes the growth and metastasis of pancreatic cancer by mediating the increase of PERP mRNA N6-adenosine methylation. Therefore, the expression levels and functions of m6A regulators are complex in different tumors. The mechanism of RNA methylation in tumors needs to be further investigated. Currently, the effect of m6A RNA methylation in the TIME of HCC needs to be analyzed further.
In the research, we demonstrated the expression of m6A regulators in HCC, its prognostic value, and the effect of TIME, YTHDF1, YTHDF2, HNRNPC, WTAP, and METTL3. METTL3 and HNRNPC dramatically decreased in HCC compared with normal tissues (p < 0.001). YTHDF2 (p < 0.001) and WTAP (p < 0.05) were significantly upregulated in HCC tissues. However, the expression level of YTHDF1 was down-regulated in HCC tissues. Then, two subtypes of HCC, cluster1 and cluster2, were identified. We found that all m6A regulators upregulated in cluster1. Also, OS and PFS of patients in cluster1 were worse compared with cluster2. Compared with cluster2, cluster1 was closely associated with lower immune score and higher PD-L1 expression level. The results were confirmed by a previous report, which showed that patients with high PD-L1 expression had a distinct poorer prognosis than those with low PD-L1 expression (Gao et al., 2009). Moreover, the expression levels of immune cells were significantly deferent in the two subtypes. The analysis showed that the infiltration levels of naïve B cells, T follicular helper cells, and macrophages M0 were higher in cluster1, whereas the level of macrophages M2 was lower. The results showed that the m6A regulators were closely associated with prognosis and TIME in HCC patients. The GSEA reveled that the functional characteristics of HCC, especially Wnt and mTOR signaling pathways, were mainly enriched in cluster1. Tang et al. (2020) reported that m6A demethylase inhibits tumor by mediating Wnt signaling. Zhao et al. (2020) reported that m6A RNA modification regulates mTOR signal pathway in gastrointestinal cancer. Also, Zhang H. et al. (2020) found that the m6A regulator METTL3 promotes the progression of retinoblastoma through mTOR signal pathway. The findings revealed that m6A regulators could affect the progression of HCC by targeting Wnt and mTOR pathways, which could provide a new therapeutic strategy for the treatment of HCC.
Furthermore, high- and low-risk group patients were identified based on the five m6A regulator-based risk signatures. Interestingly, we found that cluster1 was distinctly enriched in the high-risk group. Similarly, high-risk group with high PD-L1 expression level had worse prognosis than low-risk group with low PD-L1 expression level in the training dataset and the validation dataset. The nomogram indicated that the risk score could effectively predict the prognosis of patients with HCC. Regarding the five m6A regulator-based risk signatures, four of five m6A regulators, including YTHDF1, YTHDF2, WTAP, and METTL3, could facilitate the progression of HCC, and the results were confirmed by previous reports (Chen et al., 2018, 2019; Liu et al., 2020; Zhang C. et al., 2020). However, Zhong et al. (2019) showed that YTHDF2 suppresses cell proliferation and growth in HCC. Moreover, five m6A regulators are also associated with different cancers. For example, YTHDF1, YTHDF2, and especially METTL3 are associated with gastric cancer. YTHDF1 and its m6A-mediated regulation of Wnt/β-catenin signaling promote gastric cancer progression. METTL3-mediated m6A modification facilitates gastric cancer progression and has poor prognosis (Pi et al., 2020; Shen et al., 2020; Wang Q. et al., 2020). Also, HNRNPC and YTHDF1 have an effect on prognosis in breast cancer patients (Wu et al., 2018; Anita et al., 2020). Also, the results were confirmed by the data obtained from Kaplan–Meier Plotter (Supplementary Figure 5). The results showed that the dysregulation of specific m6A regulators plays a key role in the progression of various kinds of tumors.
Tumor microenvironment is involved in the regulation of patient prognosis and response to treatment (Fridman et al., 2012; Galon et al., 2014; Hui and Chen, 2015). A previous study showed that tumor-infiltrating lymphocytes could serve as prognostic biomarkers and targets for immunotherapy in HCC (Ding et al., 2018). However, the mechanism of immune infiltration in response to HCC is largely unclear. In this study, we analyzed the correction between immune cell infiltration and risk score. We found that the risk score was positively associated with the infiltration levels of memory B cells, naïve B cells, T follicular helper cells, and eosinophils, and negatively associated with the infiltration levels of macrophages M2 and resting memory CD4 T cells. Zheng et al. (2020) reported that the RNA m6A methylation and its reader proteins play a key regulatory role in early B-cell development. These findings reveled that the m6A regulators are associated with TIME in HCC to some extent. Furthermore, we found that the CNAs of regulators were closely related to the immune infiltration levels, including B cells, CD8+ T cells, CD4+ T cells, neutrophils, dendritic cells, and especially macrophages. It is further confirmed that the m6A regulators could affect TIME regulation in HCC.
However, there are some limitations in this research. First, the data of research are only obtained from the TCGA datasets. We lack our own independent clinical sample data to verify our findings. Then the results of our research need further experimental verification. In the future, we will further research the correction between m6A regulators and TIME in HCC.
In summary, we systematically assessed the relationship of TIME, PD-L1, and m6A regulators in HCC. According to the expression levels of the m6A regulators, HCC patients were divided into cluster1 and cluster2. The two clusters were significantly different in PD-L1 expression level, immunoscore, prognosis, and TIME in HCC. HCC patients with higher PD-L1 expression or immunoscore were associated with worse prognosis. Then high-risk group and low-risk group patients were identified based on the five risk signatures. The high-risk group was significantly associated with higher PD-L1 expression levels, higher grades, and worse OS. The GSEA results revealed that the m6A regulators were associated with the malignant functional features of HCC, including Wnt and mTOR signaling pathways. Therefore, the m6A regulators are associated with TIME in HCC, which could provide a new treatment strategy for HCC patients.
The datasets presented in this study can be found in online repositories. The names of the repository/repositories and accession number(s) can be found in the article/Supplementary Material.
XX: conceptualization, methodology, writing-review, and editing. YX: methodology, resources, software, formal analysis, and writing-original draft. XH: methodology, resources, formal analysis, and writing-original draft. JD: methodology, resources, software, and writing-original draft. LX: validation and data curation. YL and XZ: visualization and supervision. WC and XL: visualization. All authors contributed to the article and approved the submitted version.
This work was supported by funding from the National Natural Science Foundation of China (No. 31971166) and the Natural Science Foundation of Hubei Province (No. 2019CFB157).
The authors declare that the research was conducted in the absence of any commercial or financial relationships that could be construed as a potential conflict of interest.
The Supplementary Material for this article can be found online at: https://www.frontiersin.org/articles/10.3389/fcell.2021.681745/full#supplementary-material
Supplementary Figure 1 | Flowchart of the article.
Supplementary Figure 2 | Correlation of consensus clustering for m6A RNA methylation regulators. Consensus clustering matrix from k = 2–9.
Supplementary Figure 3 | Construction of prognostic signatures for m6A regulator-based risk signatures in the validation dataset. (A) Association of status and five m6A RNA regulators with risk score. (B) The area under AUC of five risk signatures. (C) OS of high-risk group and low-risk group.
Supplementary Figure 4 | The calibration curve of 3 and 5 years.
Supplementary Figure 5 | OS of YTHTF1, METTL3, and HNRNPC in gastric carcinoma.
Alarcón, C. R., Goodarzi, H., Lee, H., Liu, X., Tavazoie, S., and Tavazoie, S. F. (2015). HNRNPA2B1 is a mediator of m(6)A-dependent nuclear RNA processing events. Cell 162, 1299–1308. doi: 10.1016/j.cell.2015.08.011
Anita, R., Paramasivam, A., Priyadharsini, J. V., and Chitra, S. (2020). The m6A readers YTHDF1 and YTHDF3 aberrations associated with metastasis and predict poor prognosis in breast cancer patients. Am. J. Cancer Res. 10, 2546–2554.
Bray, F., Ferlay, J., Soerjomataram, I., Siegel, R., Torre, L. A., and Jemal, A. (2018). Global cancer statistics 2018: GLOBOCAN estimates of incidence and mortality worldwide for 36 cancers in 185 countries. CA A Cancer J. Clin. 68, 394–424. doi: 10.3322/caac.21492
Chen, M., Wei, L., Law, C. T., Tsang, F. H., Shen, J., Cheng, C., et al. (2018). RNA N6-methyladenosine methyltransferase-like 3 promotes liver cancer progression through YTHDF2-dependent posttranscriptional silencing of SOCS2. Hepatology (Baltimore, MD) 67, 2254–2270. doi: 10.1002/hep.29683
Chen, W., Zheng, R., Baade, P. D., Zhang, S., Zeng, H., Bray, F., et al. (2016). Cancer statistics in China, 2015. CA A Cancer J. Clin. 66, 115–132. doi: 10.3322/caac.21338
Chen, Y., Peng, C., Chen, J., Chen, D., Yang, B., He, B., et al. (2019). WTAP facilitates progression of hepatocellular carcinoma via m6A-HuR-dependent epigenetic silencing of ETS1. Mol. Cancer 18:127. doi: 10.1186/s12943-019-1053-8
Colaprico, A., Silva, T. C., Olsen, C., Garofano, L., Cava, C., Garolini, D., et al. (2016). TCGAbiolinks: an R/Bioconductor package for integrative analysis of TCGA data. Nucleic Acids Res. 44:e71. doi: 10.1093/nar/gkv1507
Ding, W., Xu, X., Qian, Y., Xue, W., Wang, Y., Du, J., et al. (2018). Prognostic value of tumor-infiltrating lymphocytes in hepatocellular carcinoma: a meta-analysis. Medicine (Baltimore) 97:e13301. doi: 10.1097/md.0000000000013301
Du, K., Zhang, L., Lee, T., and Sun, T. (2019). m(6)A RNA methylation controls neural development and is involved in human diseases. Mol. Neurobiol. 56, 1596–1606. doi: 10.1007/s12035-018-1138-1
El-Serag, H. B. (2012). Epidemiology of viral hepatitis and hepatocellular carcinoma. Gastroenterology 142, 1264–1273.e1261. doi: 10.1053/j.gastro.2011.12.061
Forner, A., Llovet, J. M., and Bruix, J. (2012). Hepatocellular carcinoma. Lancet 379, 1245–1255. doi: 10.1016/s0140-6736(11)61347-0
Fridman, W. H., Pagès, F., Sautès-Fridman, C., and Galon, J. (2012). The immune contexture in human tumours: impact on clinical outcome. Nat. Rev. Cancer 12, 298–306. doi: 10.1038/nrc3245
Fu, Y., Liu, S., Zeng, S., and Shen, H. (2019). From bench to bed: the tumor immune microenvironment and current immunotherapeutic strategies for hepatocellular carcinoma. J. Exp. Clin. Cancer Res. CR 38:396. doi: 10.1186/s13046-019-1396-4
Galon, J., Mlecnik, B., Bindea, G., Angell, H., Berger, A., Lagorce, C., et al. (2014). Towards the introduction of the ‘Immunoscore’ in the classification of malignant tumours. J. Pathol. 232, 199–209. doi: 10.1002/path.4287
Gao, Q., Wang, X. Y., Qiu, S. J., Yamato, I., Sho, M., Nakajima, Y., et al. (2009). Overexpression of PD-L1 significantly associates with tumor aggressiveness and postoperative recurrence in human hepatocellular carcinoma. Clin. Cancer Res. 15, 971–979. doi: 10.1158/1078-0432.Ccr-08-1608
He, L., Li, H., Wu, A., Peng, Y., Shu, G., and Yin, G. (2019). Functions of N6-methyladenosine and its role in cancer. Mol. Cancer 18:176. doi: 10.1186/s12943-019-1109-9
Hsu, P. J., Zhu, Y., Ma, H., Guo, Y., Shi, X., Liu, Y., et al. (2017). Ythdc2 is an N(6)-methyladenosine binding protein that regulates mammalian spermatogenesis. Cell Res. 27, 1115–1127. doi: 10.1038/cr.2017.99
Hui, L., and Chen, Y. (2015). Tumor microenvironment: sanctuary of the devil. Cancer Lett. 368, 7–13. doi: 10.1016/j.canlet.2015.07.039
Iasonos, A., Schrag, D., Raj, G. V., and Panageas, K. (2008). How to build and interpret a nomogram for cancer prognosis. J. Clin. Oncol. 26, 1364–1370. doi: 10.1200/jco.2007.12.9791
Kasowitz, S. D., Ma, J., Anderson, S. J., Leu, N. A., Xu, Y., Gregory, B., et al. (2018). Nuclear m6A reader YTHDC1 regulates alternative polyadenylation and splicing during mouse oocyte development. PLoS Genet. 14:e1007412. doi: 10.1371/journal.pgen.1007412
Knuckles, P., Lence, T., Haussmann, I. U., Jacob, D., Kreim, N., Carl, S., et al. (2018). Zc3h13/Flacc is required for adenosine methylation by bridging the mRNA-binding factor Rbm15/Spenito to the m(6)A machinery component Wtap/Fl(2)d. Genes Dev. 32, 415–429. doi: 10.1101/gad.309146.117
Krug, R. M., Morgan, M. A., and Shatkin, A. J. (1976). Influenza viral mRNA contains internal N6-methyladenosine and 5′-terminal 7-methylguanosine in cap structures. J. Virol. 20, 45–53. doi: 10.1128/jvi.20.1.45-53.1976
Li, Z., Weng, H., Su, R., Weng, X., Zuo, Z., Li, C., et al. (2017). FTO plays an oncogenic role in acute myeloid leukemia as a N(6)-Methyladenosine RNA demethylase. Cancer Cell 31, 127–141. doi: 10.1016/j.ccell.2016.11.017
Lin, X., Chai, G., Wu, Y., Li, J., Chen, F., Liu, J., et al. (2019). RNA m(6)A methylation regulates the epithelial mesenchymal transition of cancer cells and translation of Snail. Nat. Commun. 10:2065. doi: 10.1038/s41467-019-09865-9
Liu, N., Dai, Q., Zheng, G., He, C., Parisien, M., and Pan, T. (2015). N(6)-methyladenosine-dependent RNA structural switches regulate RNA-protein interactions. Nature 518, 560–564. doi: 10.1038/nature14234
Liu, X., Qin, J., Gao, T., Li, C., He, B., Pan, B., et al. (2020). YTHDF1 facilitates the progression of hepatocellular carcinoma by promoting FZD5 mRNA translation in an m6A-Dependent manner. Mol. Ther. Nucleic Acids 22, 750–765. doi: 10.1016/j.omtn.2020.09.036
Ma, J. Z., Yang, F., Zhou, C. C., Liu, F., Yuan, J., Wang, F., et al. (2017). METTL14 suppresses the metastatic potential of hepatocellular carcinoma by modulating N(6) -methyladenosine-dependent primary MicroRNA processing. Hepatology (Baltimore, MD) 65, 529–543. doi: 10.1002/hep.28885
Margetts, J., Ogle, L. F., Chan, S. L., Chan, A. W. H., Chan, K. C. A., Jamieson, D., et al. (2018). Neutrophils: driving progression and poor prognosis in hepatocellular carcinoma? Br. J. Cancer 118, 248–257. doi: 10.1038/bjc.2017.386
Patil, D. P., Chen, C. K., Pickering, B. F., Chow, A., Jackson, C., Guttman, M., et al. (2016). m(6)A RNA methylation promotes XIST-mediated transcriptional repression. Nature 537, 369–373. doi: 10.1038/nature19342
Pi, J., Wang, W., Ji, M., Wang, X., Wei, X., Jin, J., et al. (2020). YTHDF1 promotes gastric carcinogenesis by controlling translation of FZD7. Cancer Res. 81, 2651–2665. doi: 10.1158/0008-5472.Can-20-0066
Ping, X. L., Sun, B. F., Wang, L., Xiao, W., Yang, X., Wang, W., et al. (2014). Mammalian WTAP is a regulatory subunit of the RNA N6-methyladenosine methyltransferase. Cell Res. 24, 177–189. doi: 10.1038/cr.2014.3
Pinto, R., Vågbø, C. B., Jakobsson, M. E., Kim, Y., Baltissen, M., O’Donohue, M., et al. (2020). The human methyltransferase ZCCHC4 catalyses N6-methyladenosine modification of 28S ribosomal RNA. Nucleic Acids Res. 48, 830–846. doi: 10.1093/nar/gkz1147
Roignant, J. Y., and Soller, M. (2017). m(6)A in mRNA: an ancient mechanism for fine-tuning gene expression. Trends Genet. 33, 380–390. doi: 10.1016/j.tig.2017.04.003
Shen, X., Zhao, K., Xu, L., Cheng, G., Zhu, J., Gan, L., et al. (2020). YTHDF2 inhibits gastric cancer cell growth by regulating FOXC2 signaling pathway. Front. Genet. 11:592042. doi: 10.3389/fgene.2020.592042
Shi, H., Wang, X., Lu, Z., Zhao, B. S., Ma, H., Hsu, P. J., et al. (2017). YTHDF3 facilitates translation and decay of N(6)-methyladenosine-modified RNA. Cell Res. 27, 315–328. doi: 10.1038/cr.2017.15
Song, T., Yang, Y., Wei, H., Xie, X., Lu, J., Zeng, Q., et al. (2019). Zfp217 mediates m6A mRNA methylation to orchestrate transcriptional and post-transcriptional regulation to promote adipogenic differentiation. Nucleic Acids Res. 47, 6130–6144. doi: 10.1093/nar/gkz312
Tang, B., Yang, Y., Kang, M., Wang, Y., Wang, Y., Bi, Y., et al. (2020). m(6)A demethylase ALKBH5 inhibits pancreatic cancer tumorigenesis by decreasing WIF-1 RNA methylation and mediating Wnt signaling. Mol. Cancer 19:3. doi: 10.1186/s12943-019-1128-6
Unitt, E., Marshall, A., Gelson, W., Rushbrook, S., Davies, S., Vowler, S., et al. (2006). Tumour lymphocytic infiltrate and recurrence of hepatocellular carcinoma following liver transplantation. J. Hepatol. 45, 246–253. doi: 10.1016/j.jhep.2005.12.027
Wang, M., Liu, J., Zhao, Y., He, R., Xu, X., Guo, X., et al. (2020). Upregulation of METTL14 mediates the elevation of PERP mRNA N(6) adenosine methylation promoting the growth and metastasis of pancreatic cancer. Mol. Cancer 19:130. doi: 10.1186/s12943-020-01249-8
Wang, P., Doxtader, K. A., and Nam, Y. (2016). Structural basis for cooperative function of Mettl3 and Mettl14 methyltransferases. Mol. Cell 63, 306–317. doi: 10.1016/j.molcel.2016.05.041
Wang, Q., Chen, C., Ding, Q., Zhao, Y., Wang, Z., Chen, J., et al. (2020). METTL3-mediated m(6)A modification of HDGF mRNA promotes gastric cancer progression and has prognostic significance. Gut 69, 1193–1205. doi: 10.1136/gutjnl-2019-319639
Wang, X., Feng, J., Xue, Y., Guan, Z., Zhang, D., Liu, Z., et al. (2017). Corrigendum: structural basis of N(6)-adenosine methylation by the METTL3-METTL14 complex. Nature 542:260. doi: 10.1038/nature21073
Wang, X., Lu, Z., Gomez, A., Hon, G., Yue, Y., Han, D., et al. (2014). N6-methyladenosine-dependent regulation of messenger RNA stability. Nature 505, 117–120. doi: 10.1038/nature12730
Wang, X., Zhao, B. S., Roundtree, I. A., Lu, Z., Han, D., Ma, H., et al. (2015). N(6)-methyladenosine modulates messenger RNA translation efficiency. Cell 161, 1388–1399. doi: 10.1016/j.cell.2015.05.014
Warda, A. S., Kretschmer, J., Hackert, P., Lenz, C., Urlaub, H., Höbartner, C., et al. (2017). Human METTL16 is a N(6)-methyladenosine (m(6)A) methyltransferase that targets pre-mRNAs and various non-coding RNAs. EMBO Rep. 18, 2004–2014. doi: 10.15252/embr.201744940
Wu, Y., Zhao, W., Liu, Y., Tan, X., Li, X., Zou, Q., et al. (2018). Function of HNRNPC in breast cancer cells by controlling the dsRNA-induced interferon response. EMBO J. 37:e99017. doi: 10.15252/embj.201899017
Yang, X., Zhang, S., He, C., Xue, P., Zhang, L., He, Z., et al. (2020). METTL14 suppresses proliferation and metastasis of colorectal cancer by down-regulating oncogenic long non-coding RNA XIST. Mol. Cancer 19:46. doi: 10.1186/s12943-020-1146-4
Yue, Y., Liu, J., Cui, X., Cao, J., Luo, G., Zhang, Z., et al. (2018). VIRMA mediates preferential m(6)A mRNA methylation in 3′UTR and near stop codon and associates with alternative polyadenylation. Cell Discov. 4:10. doi: 10.1038/s41421-018-0019-0
Zhang, C., Huang, S., Zhuang, H., Ruan, S., Zhou, Z., Huang, K., et al. (2020). YTHDF2 promotes the liver cancer stem cell phenotype and cancer metastasis by regulating OCT4 expression via m6A RNA methylation. Oncogene 39, 4507–4518. doi: 10.1038/s41388-020-1303-7
Zhang, H., Zhang, P., Long, C., Ma, X., Huang, H., Kuang, X., et al. (2020). m(6)A methyltransferase METTL3 promotes retinoblastoma progression via PI3K/AKT/mTOR pathway. J. Cell. Mol. Med. 24, 12368–12378. doi: 10.1111/jcmm.15736
Zhang, S., Zhao, B. S., Zhou, A., Lin, K., Zheng, S., Lu, Z., et al. (2017). m(6)A demethylase ALKBH5 maintains tumorigenicity of glioblastoma stem-like cells by sustaining FOXM1 expression and cell proliferation program. Cancer Cell 31, 591–606.e596. doi: 10.1016/j.ccell.2017.02.013
Zhao, Q., Zhao, Y., Hu, W., Zhang, Y., Wu, X., Lu, J., et al. (2020). m(6)A RNA modification modulates PI3K/Akt/mTOR signal pathway in Gastrointestinal Cancer. Theranostics 10, 9528–9543. doi: 10.7150/thno.42971
Zhao, X., Yang, Y., Sun, B. F., Shi, Y., Yang, X., Xiao, W., et al. (2014). FTO-dependent demethylation of N6-methyladenosine regulates mRNA splicing and is required for adipogenesis. Cell Res. 24, 1403–1419. doi: 10.1038/cr.2014.151
Zheng, Z., Zhang, L., Cui, X. L., Yu, X., Hsu, P. J., Lyu, R., et al. (2020). Control of early B cell development by the RNA N(6)-Methyladenosine methylation. Cell Rep. 31:107819. doi: 10.1016/j.celrep.2020.107819
Zhong, C., Li, Y., Yang, J., Jin, S., Chen, G., Li, D., et al. (2021). Immunotherapy for hepatocellular carcinoma: current limits and prospects. Front. Oncol. 11:589680. doi: 10.3389/fonc.2021.589680
Zhong, L., Liao, D., Zhang, M., Zeng, C., Li, X., Zhang, R., et al. (2019). YTHDF2 suppresses cell proliferation and growth via destabilizing the EGFR mRNA in hepatocellular carcinoma. Cancer Lett. 442, 252–261. doi: 10.1016/j.canlet.2018.11.006
Keywords: hepatocellular carcinoma, m6A RNA methylation, PD-L1, tumor immune microenvironment, immune infiltrates, prognosis
Citation: Xu Y, He X, Deng J, Xiong L, Li Y, Zhang X, Chen W, Liu X and Xu X (2021) Comprehensive Analysis of the Immune Infiltrates and PD-L1 of m6A RNA Methylation Regulators in Hepatocellular Carcinoma. Front. Cell Dev. Biol. 9:681745. doi: 10.3389/fcell.2021.681745
Received: 17 March 2021; Accepted: 28 May 2021;
Published: 30 June 2021.
Edited by:
Hongming Miao, Army Medical University, ChinaCopyright © 2021 Xu, He, Deng, Xiong, Li, Zhang, Chen, Liu and Xu. This is an open-access article distributed under the terms of the Creative Commons Attribution License (CC BY). The use, distribution or reproduction in other forums is permitted, provided the original author(s) and the copyright owner(s) are credited and that the original publication in this journal is cited, in accordance with accepted academic practice. No use, distribution or reproduction is permitted which does not comply with these terms.
*Correspondence: Ximing Xu, ZG9jdG9yeHUxMjBAYWxpeXVuLmNvbQ==
†These authors have contributed equally to this work
Disclaimer: All claims expressed in this article are solely those of the authors and do not necessarily represent those of their affiliated organizations, or those of the publisher, the editors and the reviewers. Any product that may be evaluated in this article or claim that may be made by its manufacturer is not guaranteed or endorsed by the publisher.
Research integrity at Frontiers
Learn more about the work of our research integrity team to safeguard the quality of each article we publish.