- 1Department of Oncology, The Affiliated Yantai Yuhuangding Hospital of Qingdao University, Yantai, China
- 2The 4th Department of Internal Medical Oncology, Harbin Medical University Cancer Hospital, Harbin, China
- 3CAS Center for Excellence in Brain Science and Intelligence Technology, Shanghai, China
N6-methyladenosine (m6A) is the most prevalent type of RNA modification, and we hypothesized that patterns of m6A-related genes may be useful for estimating risk of lung adenocarcinoma (LUAD). An m6A-related gene set variation score (m6A-GSVS) was generated using RNA-sequencing data from LUAD patients in The Cancer Genome Atlas (TCGA). We investigated the association of m6A-GSVS with stemness, tumor mutational burden (TMB), expression of three immune checkpoints, levels of tumor-infiltrating lymphocytes (TILs), and patient prognosis. We found that m6A-GSVS was higher in LUAD than in healthy lung tissue, and it strongly correlated with stemness and TMB. Activated CD4 + T cells were more numerous in LUAD samples that had higher m6A-GSVS than in those with lower scores. Biological processes and pathways, including “Cell cycle,” “DNA replication,” and “RNA degradation,” were significantly enriched in samples with high scores. Furthermore, m6A-GSVS was an independent prognostic indicator in LUAD. In conclusion, we proposed an m6A-GSVS in LUAD. It is a putative indicator for evaluating the ability to RNA m6A, an independent prognostic indicator and associated with tumor stemness.
Introduction
There are more than 100 posttranscriptional modifications known in eukaryotic RNAs (Roundtree et al., 2017), of which N6-methyladenosine (m6A) is the most prevalent type (Desrosiers et al., 1974; Lee et al., 2014). First discovered in the 1970s (Desrosiers et al., 1974), m6A and its functions did not begin to be studied in depth until recent years when transcriptome-wide profiling of m6A became possible. Increasing evidence suggests that m6A plays crucial roles in the regulation of gene expression by affecting RNA stability, mRNA degradation, translation, and microRNA maturation (Fu et al., 2014; Alarcon et al., 2015; Meyer et al., 2015). Aberrant m6A modification is associated with a variety of cancers (Panneerdoss et al., 2018), including acute myeloid leukemia (Vu et al., 2017), hepatocellular carcinoma (Ma et al., 2017), glioblastoma (Cui et al., 2017), and lung cancer (Choe et al., 2018). Both elevated and decreased levels of m6A are associated with cancer (Liu et al., 2019).
The m6A modification is catalyzed by the methyltransferase complex, also termed a “writer” (Bokar et al., 1994), while demethylase, also known as the “eraser,” removes m6A (Jia et al., 2011; Mauer et al., 2017). The RNA “reader” protein recognizes m6A and is then activated to perform downstream functions (Wang and He, 2014). Levels of m6A are determined by expression of genes encoding writers, erasers, and readers (He et al., 2019), so we hypothesized that the expression patterns of these three types of m6A-related genes may be useful for assessing risk of diseases related to m6A dysregulation.
Aberrant m6A modification has been associated with lung cancer, the most commonly diagnosed cancer and the leading cause of cancer-related deaths (Torre et al., 2015; Choe et al., 2018). Non-small cell lung cancer (NSCLC) represents 85% of all lung cancers, approximately half of which are lung adenocarcinoma (LUAD). To investigate our hypothesis, single-sample gene set enrichment analysis (ssGSEA) was used to calculate an m6A-related gene set variation score (m6A-GSVS) for LUAD patients in The Cancer Genome Atlas (TCGA). We found that LUAD patients with different m6A-GSVS varied in multiple clinical characteristics and prognosis.
Materials and Methods
Data Processing
RNA-sequencing data (displayed as raw counts), mutation annotations (aligned against the GRCh38 reference genome), and clinical data from LUAD patients were downloaded from TCGA1 using the “TCGAbiolinks” package (Colaprico et al., 2016) in R version 3.62. Gene IDs were converted based on the annotation file (gencode.v33.annotation.gff3), downloaded from GENCODE3. Profiles of mRNA expression from the three datasets GSE3141 (Bild et al., 2006), GSE30219 (Rousseaux et al., 2013), and GSE37745 (Botling et al., 2013), all based on the GPL570 platform, were downloaded from the Gene Expression Omnibus (GEO) database using the “GEOquery” package in R4. Batch effects were removed from the three datasets using the ComBat function in the “sva” package (Leek et al., 2012). If a gene has multiple probes, the average value of the probes was considered the expression of the corresponding gene. The LUAD samples were extracted from the three datasets to validate the prognostic value of m6A-GSVS. Three m6A regulator gene sets were obtained from a previous study (He et al., 2019), comprising seven writer genes (METTL3, METTL14, METTL16, RBM15, VIRMA, WTAP, and ZC3H13), two eraser genes (ALKBH5 and FTO), and 11 reader genes (EIF3A, HNRNPA2B1, HNRNPC, IGF2BP1, IGF2BP2, IGF2BP3, YTHDC1, YTHDC2, YTHDF1, YTHDF2, and YTHDF3).
Aberrant Expression and Mutation of m6A-Related Genes in LUAD
To identify differentially expressed m6A-related genes, the expression profiles of genes were compared between LUAD tissues and healthy lung tissues in the LUAD dataset from TCGA using the “DESeq2” package (Love et al., 2014). Results were displayed as an expression heatmap, with significance defined as a p-value (adjusted by the false discovery rate) < 0.05. The unregulated m6A-related genes were explored in The Human Protein Atlas (HPA5). In addition, mutations in those genes were extracted from mutation annotation format (MAF) files using the GDCquery_Maf function in the “TCGAbiolinks” package. Mutation frequencies were visualized as a waterfall plot using the oncoplot function in the “TCGAbiolinks” package.
Gene Set Variation Analysis
According to the currently known m6A theory, we conceptualized an “m6A pathway” that is activated by writers and readers and inhibited by erasers: the writers install m6A, erasers delete it, and readers recognize it. Aberrant expression patterns of genes in the pathway are likely to cause abnormal pathway activity. This is analogous to a previous study (Xiao et al., 2019) in which enrichment scores were separately calculated for the activated gene set and repressed gene set in an oncogenic pathway using the “GSVA” package based on ssGSEA. In that study, the enrichment score of the oncogenic pathway was defined as the enrichment score for the activated gene set minus the score for the repressed gene set. Thus, in the present study, the enrichment score of the “m6A pathway” for a given individual was defined as:
m6A-GSVS = “Writer” enrichment score − “Eraser” enrichment score + “Reader” enrichment score.
Gene set variation analysis (GSVA) (Hanzelmann et al., 2013) was used to generate an enrichment score of gene sets based on high-quality studies (Xiao et al., 2019; Zeng et al., 2020). Enrichment scores for the three types of m6A-related gene sets were estimated for each individual using the gsva function in the “GSVA” package (Hanzelmann et al., 2013), based on ssGSEA (Barbie et al., 2009; Abazeed et al., 2013). The parameter “method” was set to “ssgsea,” while the parameter “kcdf” was set to “Poisson.” The latter setting indicates that input data are raw counts. Although differences in the expression of some m6A-related genes were not significant, we retained them in the analysis because previous studies showed them to be important regulators of m6A, in accordance with the principle of the GSEA algorithm (Mootha et al., 2003; Subramanian et al., 2005). The median m6A-GSVS was used as the cutoff to stratify all individuals into groups with low or high m6A-GSVS.
Association of m6A-GSVS With Clinicopathological Features, Tumor Mutation Burden, Stemness, and Immune Checkpoints
The m6A-GSVSs of individuals with different clinicopathological features were compared and visualized using the “ggpubr” package in R6. Several studies have linked m6A-related genes with immune responses to immunotherapy and cancer stem cell in various types of cancers (Zhang et al., 2016, 2020; Yang et al., 2019; Wang et al., 2020). Tumor mutation burden (TMB) and the expression levels of immune checkpoints can predict efficacy of treatment with immune checkpoint inhibitors (Kazandjian et al., 2016; Carbone et al., 2017; Hellmann et al., 2018). Thus, we explored the relationships of m6A-GSVS with TMB, stemness, and expression levels of immune checkpoints.
The TMB of each sample was calculated as in a previous study (Lv et al., 2019). We compared the m6A-GSVSs between individuals with high or low TMB and calculated the Pearson correlation between the two variables. In addition, stemness (Malta et al., 2018) was calculated for each individual using the TCGAanalyze_Stemness function in the “TCGAbiolinks” package, then compared across healthy lung tissues and tissues from patients with low or high m6A-GSVS. Pearson correlations were explored between m6A-GSVS and the expression levels of three immune checkpoints: PDCD1 (PD1), CD274 (PDL1), and CTLA4.
Calculation of Tumor-Infiltrating Lymphocytes
Malignant solid tumor tissues comprise not only tumor cells but also tumor-associated normal cells, such as epithelial, stromal, immune, and vascular cells. These cells are thought to have important roles in tumor growth, invasion, and drug resistance (Pages et al., 2005; Kalluri and Zeisberg, 2006; Hanahan and Weinberg, 2011; Straussman et al., 2012). Based on a previous study (Xiao et al., 2019) that generated cell marker gene sets using CIBERSORT (Newman et al., 2015) and MCPcounter (Becht et al., 2016), we estimated the abundances of 24 types of stromal or immune cells for each LUAD sample using ssGSEA in the “GSVA” package (Hanzelmann et al., 2013). The relative abundances of the 24 types of tumor-infiltrating lymphocytes (TILs) were compared between samples with high or low m6A-GSVS.
Gene Set Enrichment Analysis
In order to explore the biological characteristics of the high m6A-GSVS group, we performed gene set enrichment analysis (GSEA) (Mootha et al., 2003; Subramanian et al., 2005) using the GSEA JAVA program7. Three reference gene sets were used from the Molecular Signatures Database v7.1 (Subramanian et al., 2005; Liberzon et al., 2011, 2015): the Kyoto Encyclopedia of Genes and Genomes (KEGG) gene set (c2.cp.kegg.v7.1.symbols.gmt), biological process gene set (c5.bp.v7.1.symbols.gmt), and hallmark gene set (h.all.v7.1.symbols.gmt).
Survival Analysis
Kaplan–Meier survival curves were compared between individuals with low or high m6A-GSVS using the log-rank method in the “survival” package8 and “survminer” package9. We carried out uni- and multivariate Cox proportional hazards analyses of the training set in order to compare the prognostic value of the m6A-GSVS with that of routine clinicopathologic features.
Statistical Analysis
All analyses were performed using R version 3.6 (see text footnote 2). Differentially expressed genes were identified using the unpaired t-test. The Shapiro–Wilk test was used to assess whether the m6A-GSVA score was normally distributed. Intergroup differences in continuous variables were assessed for significance using Wilcoxon, Kruskal–Wallis, or unpaired t-tests. All tests were two-sided and considered significant if p < 0.05, unless otherwise stated.
Results
Multiple m6A-Related Genes Aberrantly Expressed While Few Mutated in LUAD
In the TCGA-LUAD dataset, we identified multiple m6A-related genes that were aberrantly expressed in LUAD compared with healthy lung tissue (Figure 1A). The writer genes METTL3, RBM15, and VIRMA were upregulated in LUAD, while METTL14, METTL16, WTAP, and ZC3H13 were downregulated. Most reader genes were upregulated in LUAD (HNRNPA2B1, HNRNPC, IGF2BP1, IGF2BP2, IGF2BP3, YTHDF1, and YTHDF2). The two eraser genes, ALKBH5 and FTO, were downregulated in LUAD.
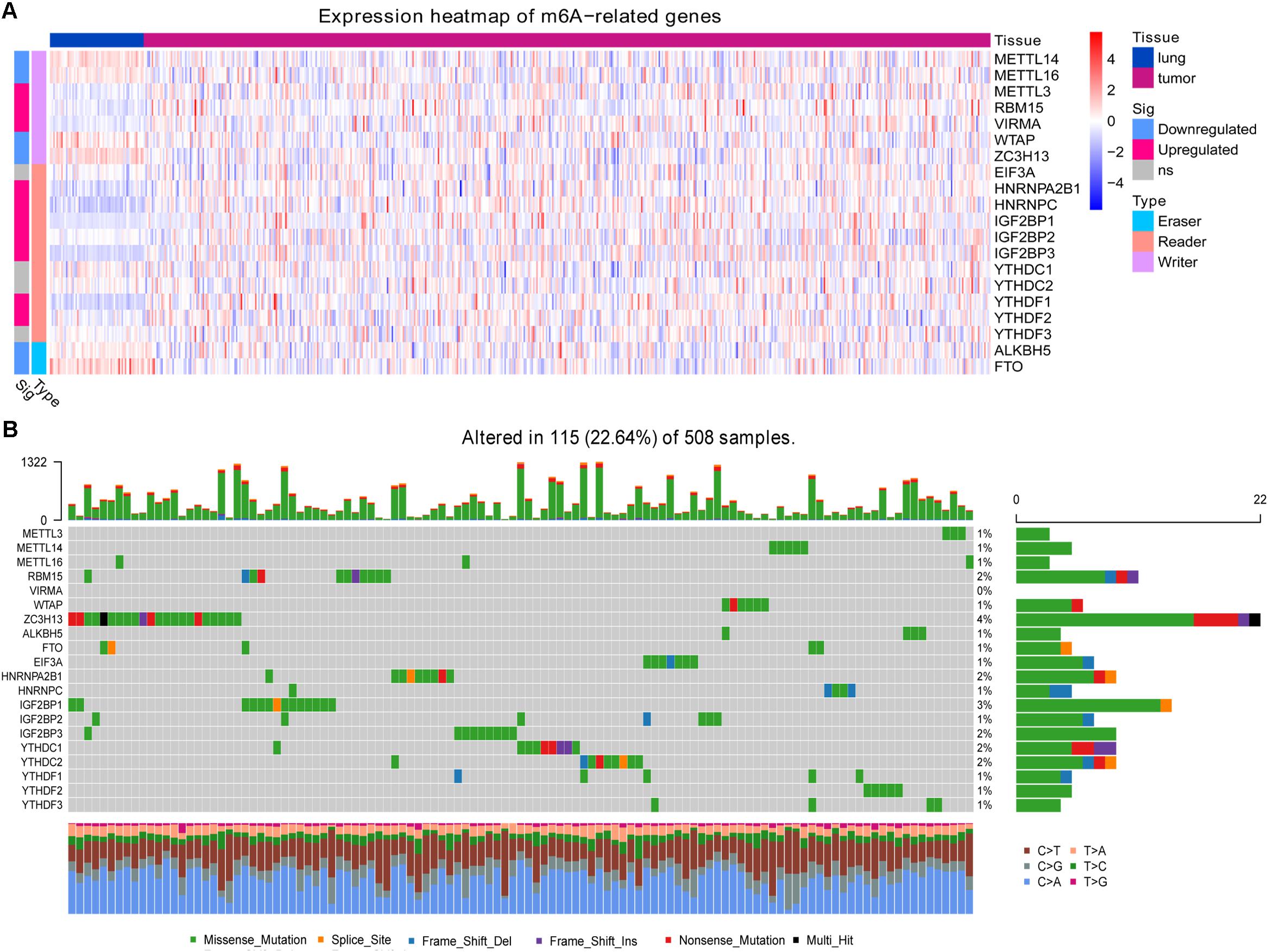
Figure 1. Expression and mutation status of 20 m6A-related genes. (A) Differential gene expression between lung adenocarcinoma and healthy lung tissue. (B) Mutation status of the genes in lung adenocarcinoma.
At least one of these 20 m6A-related genes was mutated in 22.64% of patients with LUAD (Figure 1B). However, each gene was mutated in fewer than 5% of patients. Most of the genes upregulated in LUAD were present in the HPA: RBM15 (Figure 2A), VIRMA (Figure 2B), HNRNPA2B1 (Figure 2C), HNRNPC (Figure 2D), IGF2BP2 (Figure 2F), IGF2BP3 (Figure 2G), and YTHDF2 (Figure 2H). In contrast, the upregulated genes METTL3 and YTHDF1 were not included in HPA, while IGF2BP1 was not detected in LUAD patients (Figure 2E). These results suggest that abnormal m6A levels in LUAD may be driven by aberrant expression, rather than mutation, of m6A-related genes.
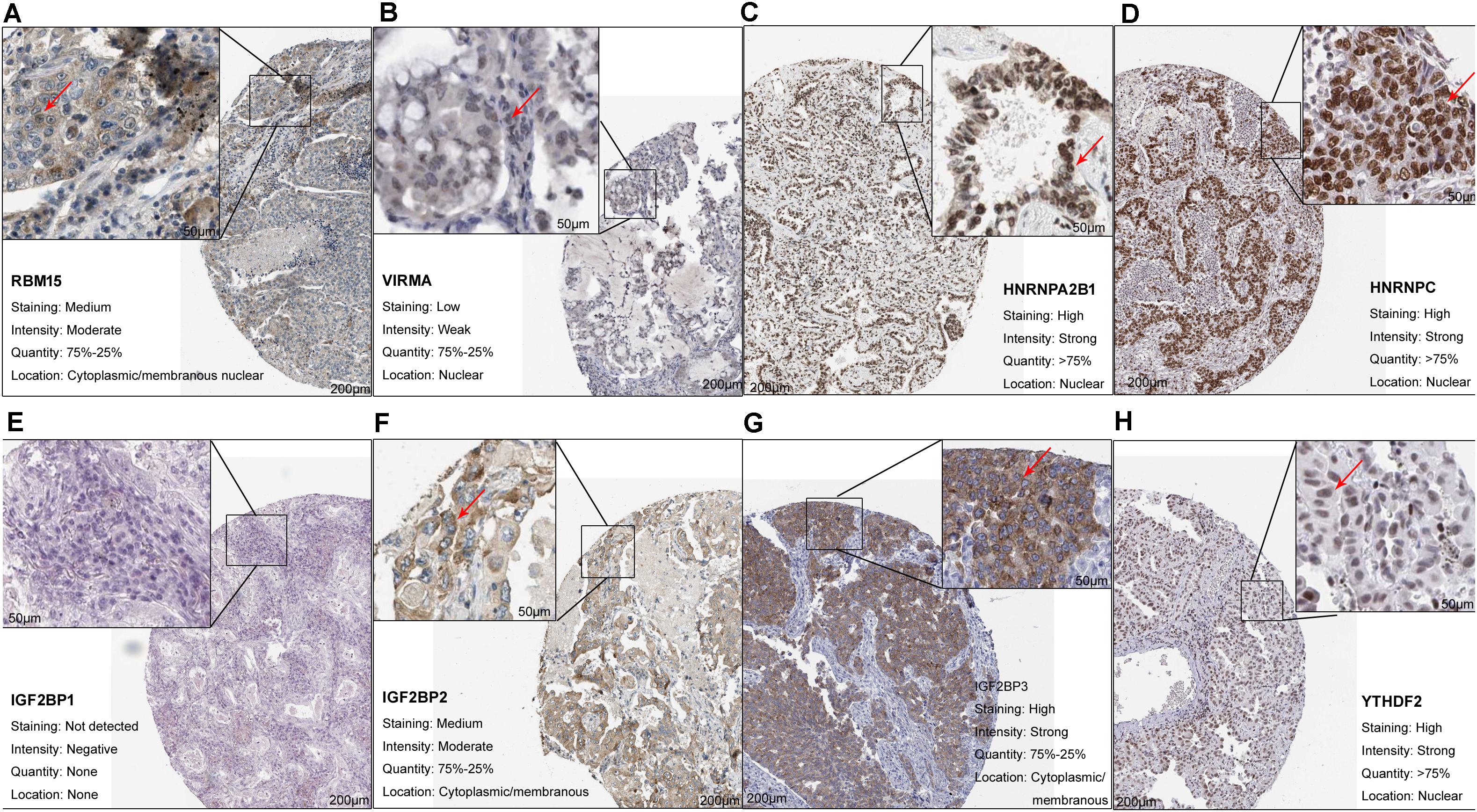
Figure 2. Upregulation of m6A-related genes in lung adenocarcinoma. (A) RBM15 (antibody CAB015201, patient 3003), (B) VIRMA (antibody HPA031530, patient 1847), (C) HNRNPA2B1 (antibody CAB012403, patient 2222), (D) HNRNPC (antibody CAB075755, patient 1932), (E) IGF2BP1 (antibody HPA062273, patient 3144), (F) IGF2BP2 (antibody CAB017126, patient 1394), (G) IGF2BP3 (antibody HPA076951, patient 4208), and (H) YTHDF2 (antibody HPA059621, patient 1303).
The m6A-GSVS Is Higher in LUAD Tissue Than in Healthy Lung
The m6A-GSVSs of LUAD tissues were significantly higher than those of healthy lung (Figure 3A). This suggests that elevated m6A level may be associated with a higher risk of LUAD. However, m6A-GSVS did not vary significantly with sex, age, or pathological stage among LUAD patients (Figure 3B).
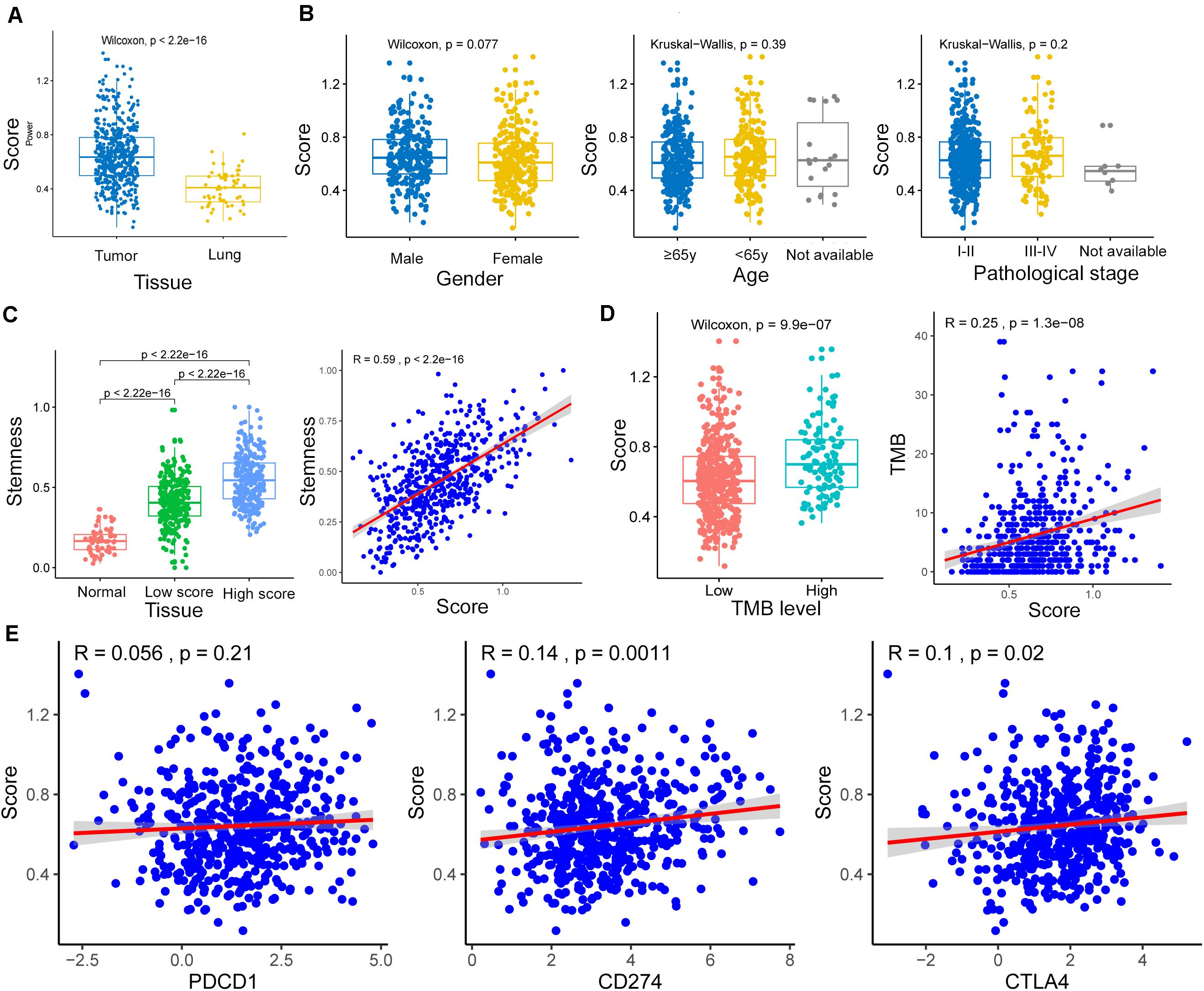
Figure 3. Associations of the m6A-related gene set variation score (m6A-GSVS) with clinicopathological features, stemness, tumor mutational burden (TMB), and expression of three immune checkpoints. (A) The m6A-GSVS was higher in lung adenocarcinoma than in healthy lung tissues. (B) The m6A-GSVS did not differ significantly with sex, age, or pathological stage. (C) The m6A-GSVS correlated strongly with stemness. (D) The m6A-GSVS correlated with TMB. (E) The m6A-GSVS was associated with the expression of three immune checkpoints.
The m6A-GSVS Correlates Strongly With Stemness and TMB but Weakly With Expression of CD274 and CTLA4
As expected, stemness was significantly higher in LUAD tissue than in healthy lung. We also found that stemness in LUAD patients correlated directly with m6A-GSVS (Pearson R = 0.59, p < 0.01) (Figure 3C). This suggests that the dedifferentiation of cells into tumors is accompanied by changes in m6A levels. The m6A-GSVS also correlated directly with TMB (Pearson R = 0.25, p < 0.01; Figure 3D). In contrast, m6A-GSVS showed a weak correlation with the expression of CD274 (Pearson R = 0.14, p < 0.01) and CTLA4 (Pearson R = 0.10, p = 0.02) and no correlation with PDCD1 expression (Figure 3E).
The m6A-GSVS and TILs in the Tumor Immune Microenvironment
The 24 types of TILs in each individual were displayed as a heatmap (Figure 4A). The abundances of nearly all TILs were lower in samples with high m6A-GSVS, including dendritic cells, endothelial cells, eosinophils, fibroblasts, macrophages (M1), resting mast cells, monocytes, neutrophils, plasma cells, and regulatory T cells (Tregs) (Figure 4B). Only activated CD4 T cells were more abundant in samples with high m6A-GSVS than in those with low m6A-GSVS.
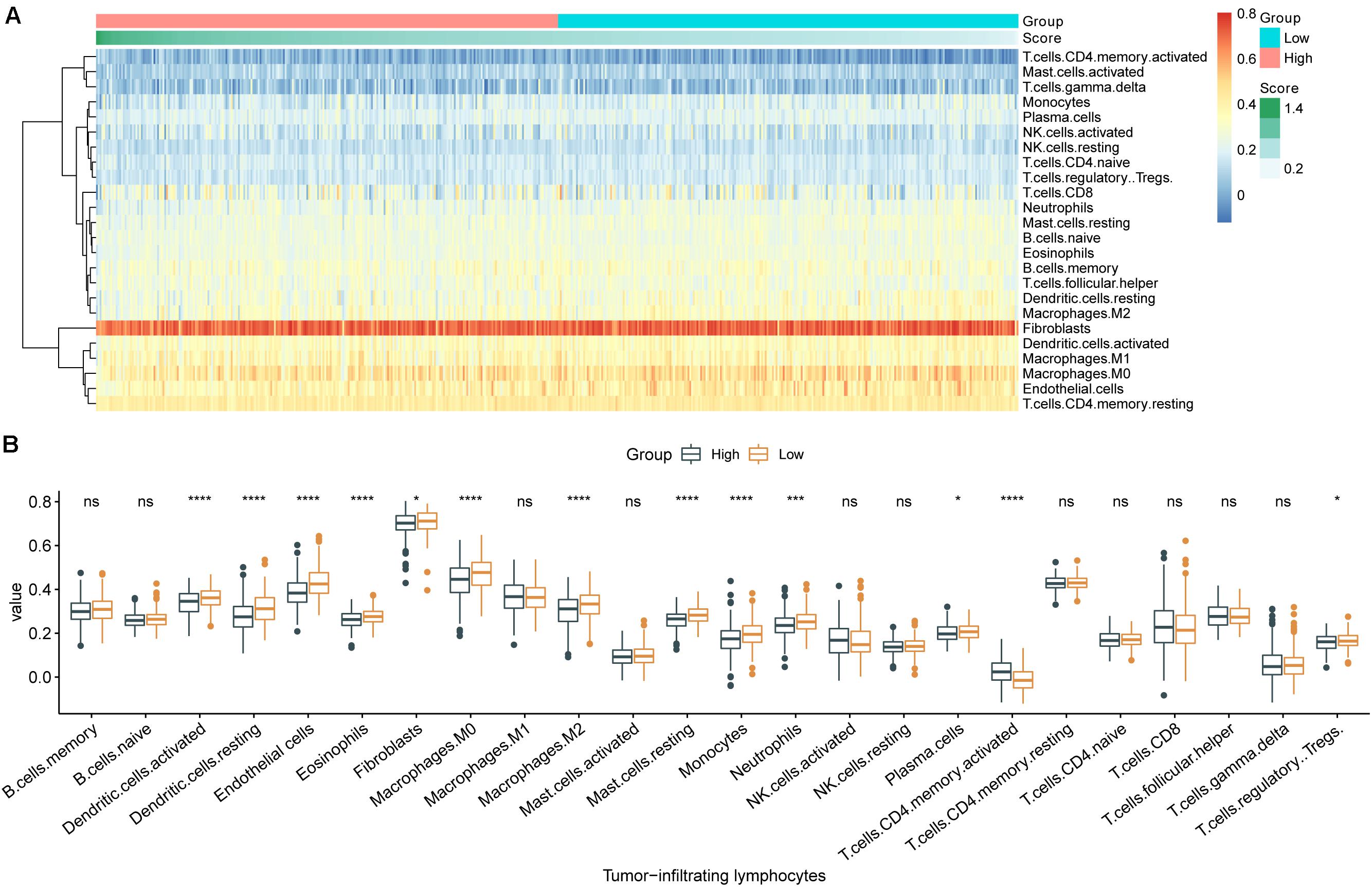
Figure 4. The m6A-related gene set variation score (m6A-GSVS) and the levels of tumor-infiltrating lymphocytes (TILs). (A) Heatmap of 24 types of TILs and the m6A-GSVS in each individual. (B) Comparison of relative abundances of 24 types of TILs between samples with high or low m6A-GSVS. *p < 0.05, **p < 0.01, ***p < 0.001, and ****p < 0.0001.
Different m6A-GSVSs in LUAD Samples Are Associated With Differences in Multiple Biological Processes and Pathways
Based on GSEA, the top five (ranked by false discovery rate) biological processes significantly enriched in samples with high m6A-GSVS were “RNA export from nucleus,” “RNA 3 end processing,” “mRNA 3 end processing,” “mRNA cis splicing via spliceosome,” and “mRNA export from nucleus” (Figure 5A). The top five KEGG pathways significantly enriched in samples with high m6A-GSVS were “Basal transcription factors,” “Cell cycle,” “DNA replication,” “RNA degradation,” and “Spliceosome” (Figure 5B). The top five hallmark gene sets (Figure 5C) significantly enriched in the high m6A-GSVS samples were “E2F targets,” “G2M checkpoint,” “Mitotic spindle,” “MYC targets v1,” and “MYC targets v2.”
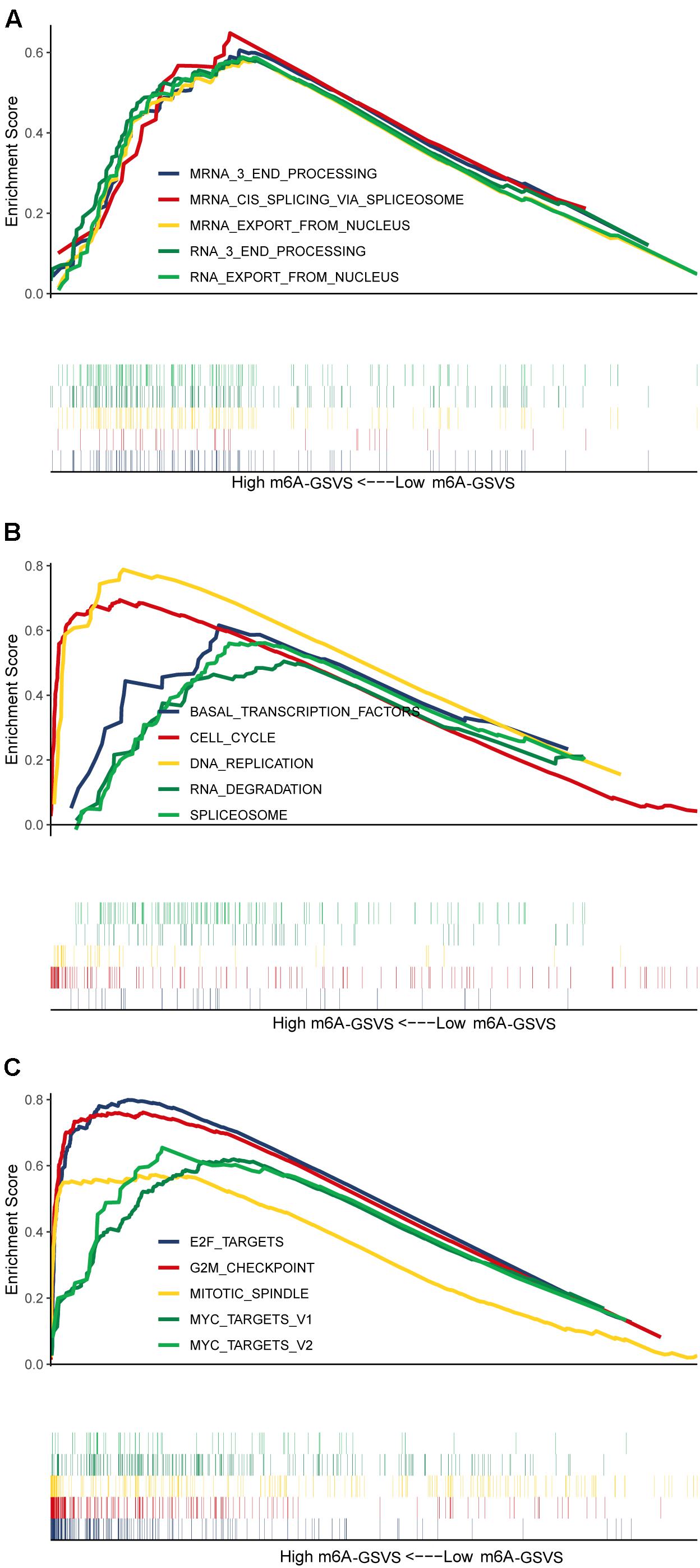
Figure 5. Gene set enrichment analysis. The top five (ranked by false discovery rate) (A) biological processes, (B) KEGG pathways, and (C) hallmark gene sets significantly enriched in samples with high m6A-related gene set variation score (m6A-GSVS).
The m6A-GSVS Is an Independent Prognostic Factor in LUAD
In the training set (N = 513), univariable Cox analysis showed that higher m6A-GSVS was associated with poor overall survival (OS) [hazard ratio (HR) 2.270, 95% confidence interval (CI) 1.192–4.324, p = 0.013]. These results were validated using data from 249 patients with LUAD from the GSE3141, GSE30219, and GSE37745 datasets (HR 5.764, 95% CI 1.549–21.451, p = 0.009). Since the m6A-GSVS score showed a skewed distribution, patients were divided into groups with high or low score based on median m6A-GSVS. In the TCGA-LUAD dataset, patients with high m6A-GSVS had worse OS (Figure 6A) and disease-free survival (DFS) (Figure 6B) than those with low m6A-GSVS. Similar results were found for LUAD patients in the GSE3141, GSE30219, and GSE37745 datasets (Figures 6C,D). Multivariable Cox analysis confirmed m6A-GSVS as a prognostic indicator independent of routine clinicopathological characteristics (Table 1).
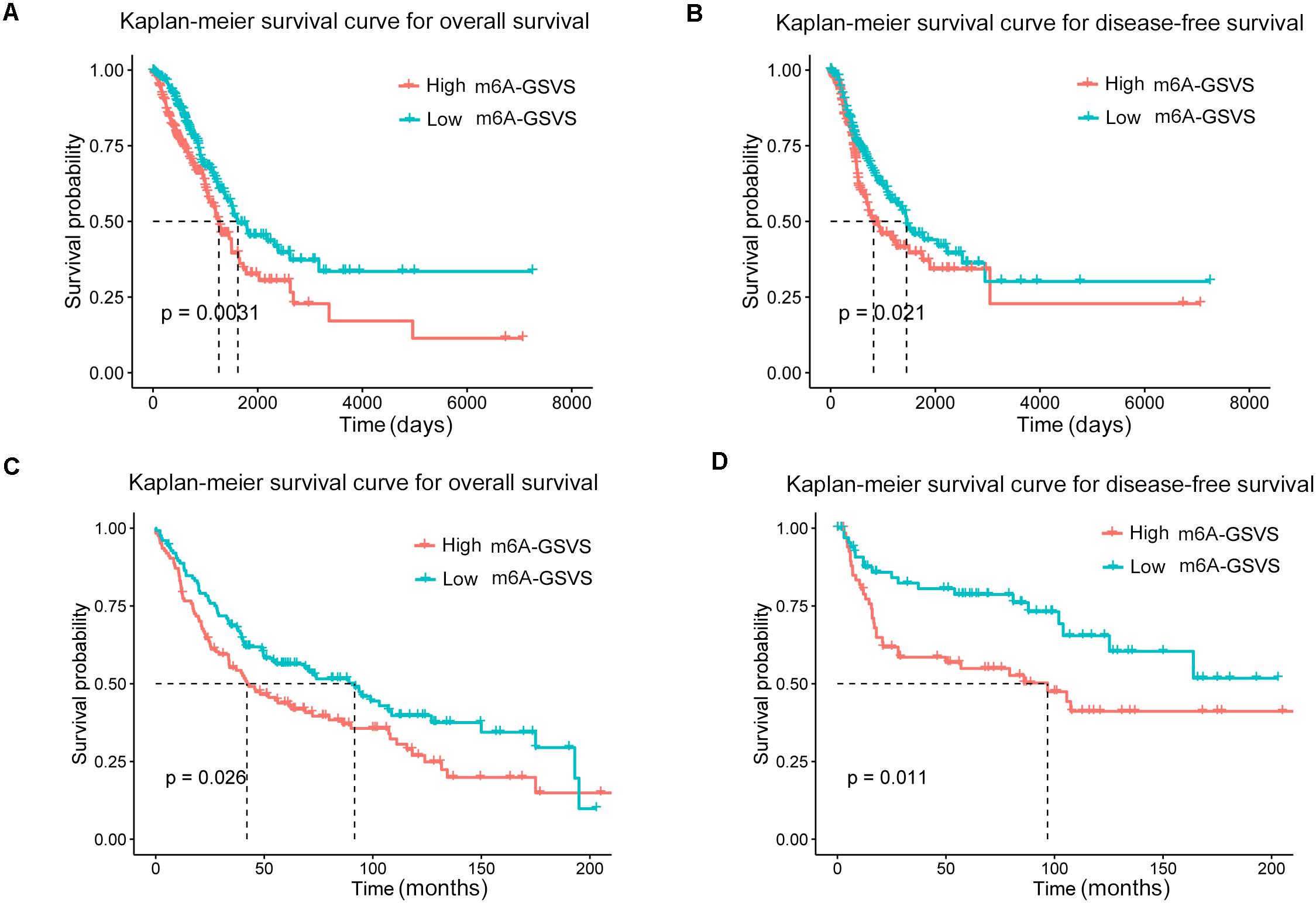
Figure 6. Kaplan–Meier curves for overall survival and disease-free survival of lung adenocarcinoma patients stratified by m6A-related gene set variation score (m6A-GSVS). (A,B) Analysis of patient data in the lung adenocarcinoma dataset in The Cancer Genome Atlas. (C,D) Analysis of patient data in the GSE3141, GSE30219, and GSE37745 datasets.
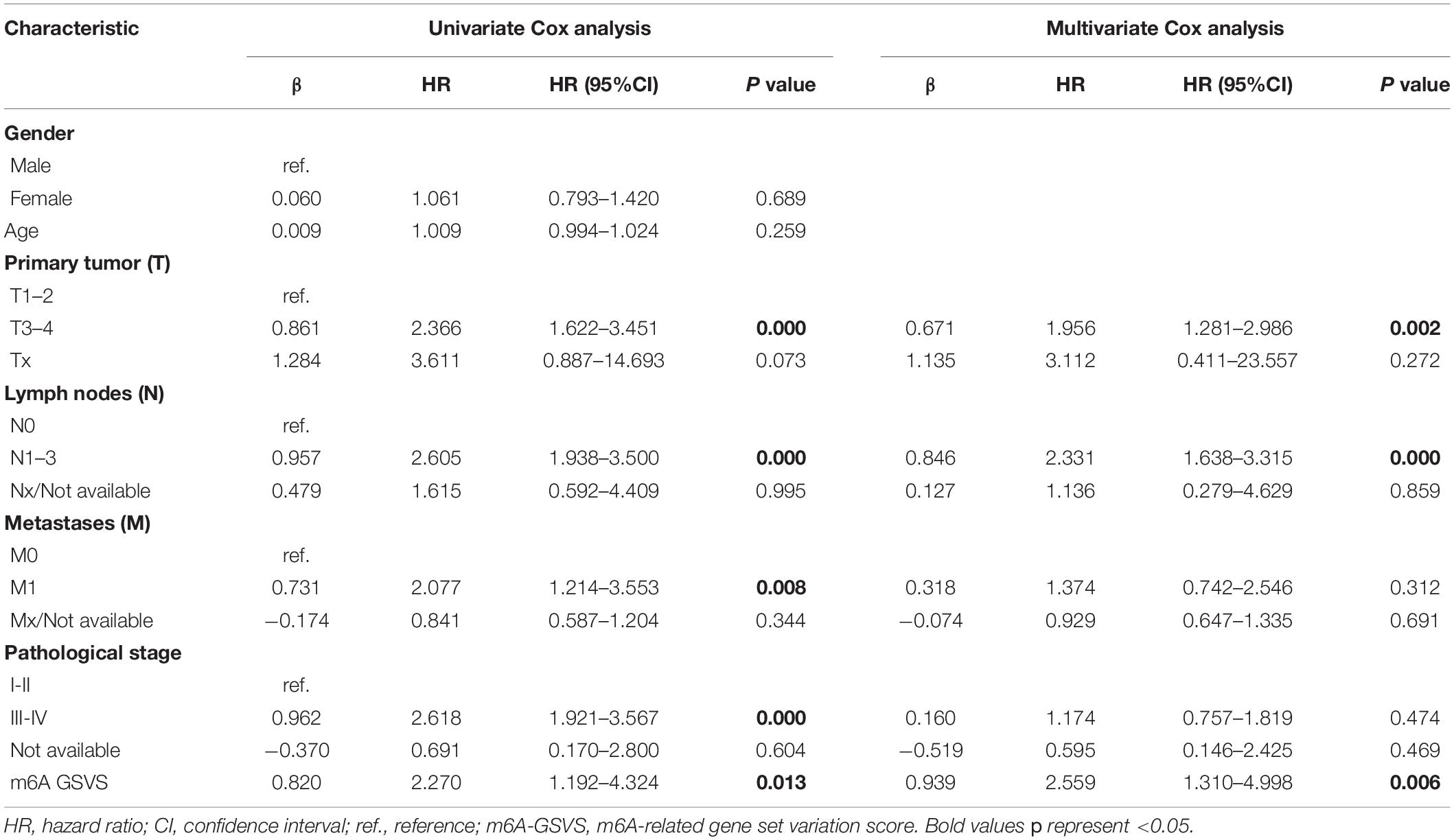
Table 1. Univariate and multivariate Cox analyses of the m6A-GSVS and routine clinicopathologic characteristics in patients with lung adenocarcinomas.
Discussion
Previous studies investigating the aberrant expression of one or two m6A-related genes in cancer (Zhang et al., 2016; Liu et al., 2017; Vu et al., 2017; Hua et al., 2018) have suggested that m6A can regulate the expression of tumor-promoting or tumor-suppressor genes in ways that can promote or inhibit cancer progression (Lin et al., 2016; Choe et al., 2018; He et al., 2018; Wang et al., 2018; Cayir et al., 2019; Zhong et al., 2019). Thus, alterations in the expression of certain m6A-related genes may influence the net effect of m6A alterations on cancer. To explore this idea, the present study looked at multiple validated m6A-related genes as part of an “m6A pathway.” On this basis, we calculated an m6A-GSVS for each sample. We found that most patients with LUAD showed aberrantly expressed m6A-related genes, but only a few patients contained mutations in those genes. This finding is consistent with a previous work (He et al., 2019).
We found that m6A-GSVS was strongly associated with stemness, consistent with a previous report linking m6A level with stem cell fate (Geula et al., 2015). In breast cancer, downregulation of ALKBH5 and m6A may drive cancer stem cell formation (Jaffrey and Kharas, 2017). These observations suggest that m6A may regulate cancer stem cell in certain types of cancer. Further study is needed to clarify the mechanisms linking m6A level and cancer stem cells.
Our analyses showed that m6A-GSVS positively correlated with TMB, and a previous work linked higher TMB with more new transcripts whose protein products act as new antigens (Schumacher and Schreiber, 2015). We infer that tumor cells may increase m6A levels in order to degrade the additional RNAs and thereby avoid attack by immune cells (Fu et al., 2014; Alarcon et al., 2015; Meyer et al., 2015). Consistent with a relationship between m6A level and tumor immunity, m6A modification of mRNA and expression of YTHDF1 in dendritic cells have been associated with antitumor immunity (Han et al., 2019).
As higher TMB is associated with better efficacy of anti-immune checkpoint treatment (Carbone et al., 2017; Hellmann et al., 2018), high m6A level may be associated with response to such treatment in LUAD. For example, m6A level appears to regulate the response to anti-PD1 treatment in melanoma (Yang et al., 2019). However, m6A-GSVS in our study showed weak association with the expression of CD274 and CTLA4, even though the expression of both proteins predicts the efficacy of anti-immune checkpoint treatment (Kazandjian et al., 2016); whether anti-immune checkpoint treatment is more effective for LUAD patients with high m6A-GSVS requires further exploration. Among all the TILs that we analyzed, only activated CD4 + T cells were more numerous in LUAD samples with high m6A-GSVS than in those with low scores. This suggests that higher m6A-GSVS may be associated with greater recruitment of CD4 + T cells, which should be explored in future work.
Consistent with the idea that m6A affects RNA stability and translation (Fu et al., 2014; Alarcon et al., 2015; Meyer et al., 2015), our GSEA indicated that high m6A-GSVS in LUAD was associated with more active nucleic acid metabolism, including “RNA export from nucleus,” “mRNA 3 end processing,” and “mRNA cis splicing via spliceosome.” High m6A-GSVS was also associated with enrichment in pathways involving “basal transcription factors,” “cell cycle,” “DNA replication,” “RNA degradation,” and the “spliceosome.” These results suggest that the m6A-GSVS may accurately predict m6A activity. In addition, we found that the targets of the transcription factors MYC and E2F were upregulated in LUAD involving high m6A-GSVS, so these two transcription factors may play a crucial role in m6A progression.
We found that the m6A-GSVS was an independent prognostic factor for patients with LUAD: those who had higher m6A-GSVS had worse OS and DFS than those with lower scores. This finding extends studies linking single m6A-related genes to prognosis in various types of cancer (Kwok et al., 2017; Chen et al., 2018; Niu et al., 2019; Liu et al., 2020). Rather than focusing on a single gene, our score evaluates the activity of the “m6A pathway” as a whole.
Our understanding of the mechanism of m6A is still far from complete. The techniques of MeRIP-seq and m6A sequencing have commonly been used to map m6A sites in transcriptomes (Dominissini et al., 2012), but they cannot always determine the number or positions of such sites because a given peak may contain multiple m6A sites (Grozhik and Jaffrey, 2018). Although our present study provided a new insight on m6A RNA modification, it has several limitations. First, the included m6A-related genes are based on previous studies. More such genes may be discovered in future studies, so the m6A-GSVS should be validated and improved accordingly. Second, the m6A-GSVS was designed to estimate the activity of m6A in an individual with LUAD, not in a single RNA molecule. Whether the m6A-GSVS can represent the real level of m6A modifications in LUAD samples requires further study. Third, it is not clear whether m6A-GSVS contributes to prognosis in LUAD or it serves simply as a prognostic marker. Fourth, the lack of data on microsatellite instability in LUAD in TCGA prevented us from exploring its correlation with the m6A-GSVA score, which should be examined given that such instability effectively predicts response to treatment with immune checkpoint inhibitors (Le et al., 2017). Fifth, whether anti-immune checkpoint treatment is more effective for LUAD patients with high m6A-GSVS still requires exploration.
Conclusion
We propose the m6A-GSVS as an index of m6A activity in LUAD. This score appears to be associated with tumor stemness and may be useful for predicting prognosis.
Data Availability Statement
Publicly available datasets were analyzed in this study. This data can be found here: https://portal.gdc.cancer.gov/; https://www.ncbi.nlm.nih.gov/geo/.
Author Contributions
PS and SM designed the study. HZ, JH, AL, and HQ performed the experiment. HZ and JH collected the data and prepared the manuscript draft. HJ and HQ performed the statistical analysis. All of the authors approved the final manuscript.
Funding
Plan of the Affiliated Yantai Yuhuangding Hospital of Qingdao University, Grant/Award Number: 201906; the National Natural Science Fund of China grant (81972162 to JH); the Natural Science Fund for Outstanding Youth of Heilongjiang Province grant (No. YQ2019H026 to JH); the Postdoctoral Scientific Research Staring Fund of Heilongjiang Province grant (No. LBH-Q19042 to JH); and the Major Program of Haiyan Fund of Harbin Medical University Cancer Hospital grant (No. JJZD2019-04 to JH).
Conflict of Interest
The authors declare that the research was conducted in the absence of any commercial or financial relationships that could be construed as a potential conflict of interest.
Acknowledgments
We would like to thank the patients and investigators who participated in the TCGA and the GEO for providing data.
Footnotes
- ^ https://portal.gdc.cancer.gov/
- ^ https://www.r-project.org
- ^ https://www.gencodegenes.org/human/
- ^ https://bioconductor.org/packages/GEOquery/
- ^ https://www.proteinatlas.org/
- ^ https://CRAN.R-project.org/package=ggpubr
- ^ http://www.broadinstitute.org/gsea
- ^ https://CRAN.R-project.org/package=survival
- ^ https://CRAN.R-project.org/package=survminer
References
Abazeed, M. E., Adams, D. J., Hurov, K. E., Tamayo, P., Creighton, C. J., Sonkin, D., et al. (2013). Integrative radiogenomic profiling of squamous cell lung cancer. Cancer Res. 73, 6289–6298. doi: 10.1158/0008-5472.CAN-13-1616
Alarcon, C. R., Lee, H., Goodarzi, H., Halberg, N., and Tavazoie, S. F. (2015). N6-methyladenosine marks primary microRNAs for processing. Nature 519, 482–485. doi: 10.1038/nature14281
Barbie, D. A., Tamayo, P., Boehm, J. S., Kim, S. Y., Moody, S. E., Dunn, I. F., et al. (2009). Systematic RNA interference reveals that oncogenic KRAS-driven cancers require TBK1. Nature 462, 108–112. doi: 10.1038/nature08460
Becht, E., Giraldo, N. A., Lacroix, L., Buttard, B., Elarouci, N., Petitprez, F., et al. (2016). Estimating the population abundance of tissue-infiltrating immune and stromal cell populations using gene expression. Genome Biol. 17:218. doi: 10.1186/s13059-016-1070-5
Bild, A. H., Yao, G., Chang, J. T., Wang, Q., Potti, A., Chasse, D., et al. (2006). Oncogenic pathway signatures in human cancers as a guide to targeted therapies. Nature 439, 353–357. doi: 10.1038/nature04296
Bokar, J. A., Rath-Shambaugh, M. E., Ludwiczak, R., Narayan, P., and Rottman, F. (1994). Characterization and partial purification of mRNA N6-adenosine methyltransferase from HeLa cell nuclei. internal mRNA methylation requires a multisubunit complex. J. Biol. Chem. 269, 17697–17704.
Botling, J., Edlund, K., Lohr, M., Hellwig, B., Holmberg, L., Lambe, M., et al. (2013). Biomarker discovery in non-small cell lung cancer: integrating gene expression profiling, meta-analysis, and tissue microarray validation. Clin. Cancer Res. 19, 194–204. doi: 10.1158/1078-0432.CCR-12-1139
Carbone, D. P., Reck, M., Paz-Ares, L., Creelan, B., Horn, L., Steins, M., et al. (2017). First-line nivolumab in stage IV or recurrent non-small-cell lung cancer. N. Engl. J. Med. 376, 2415–2426. doi: 10.1056/NEJMoa1613493
Cayir, A., Barrow, T. M., Guo, L., and Byun, H. M. (2019). Exposure to environmental toxicants reduces global N6-methyladenosine RNA methylation and alters expression of RNA methylation modulator genes. Environ. Res. 175, 228–234. doi: 10.1016/j.envres.2019.05.011
Chen, M., Wei, L., Law, C. T., Tsang, F. H., Shen, J., Cheng, C. L., et al. (2018). RNA N6-methyladenosine methyltransferase-like 3 promotes liver cancer progression through YTHDF2-dependent posttranscriptional silencing of SOCS2. Hepatology 67, 2254–2270. doi: 10.1002/hep.29683
Choe, J., Lin, S., Zhang, W., Liu, Q., Wang, L., Ramirez-Moya, J., et al. (2018). mRNA circularization by METTL3-eIF3h enhances translation and promotes oncogenesis. Nature 561, 556–560. doi: 10.1038/s41586-018-0538-8
Colaprico, A., Silva, T. C., Olsen, C., Garofano, L., Cava, C., Garolini, D., et al. (2016). TCGAbiolinks: an R/Bioconductor package for integrative analysis of TCGA data. Nucleic Acids Res. 44:e71. doi: 10.1093/nar/gkv1507
Cui, Q., Shi, H., Ye, P., Li, L., Qu, Q., Sun, G., et al. (2017). m(6)A RNA methylation regulates the self-renewal and tumorigenesis of glioblastoma stem cells. Cell Rep. 18, 2622–2634. doi: 10.1016/j.celrep.2017.02.059
Desrosiers, R., Friderici, K., and Rottman, F. (1974). Identification of methylated nucleosides in messenger RNA from Novikoff hepatoma cells. Proc. Natl. Acad. Sci. U.S.A. 71, 3971–3975. doi: 10.1073/pnas.71.10.3971
Dominissini, D., Moshitch-Moshkovitz, S., Schwartz, S., Salmon-Divon, M., Ungar, L., Osenberg, S., et al. (2012). Topology of the human and mouse m6A RNA methylomes revealed by m6A-seq. Nature 485, 201–206. doi: 10.1038/nature11112
Fu, Y., Dominissini, D., Rechavi, G., and He, C. (2014). Gene expression regulation mediated through reversible m(6)A RNA methylation. Nat. Rev. Genet. 15, 293–306. doi: 10.1038/nrg3724
Geula, S., Moshitch-Moshkovitz, S., Dominissini, D., Mansour, A. A., Kol, N., Salmon-Divon, M., et al. (2015). Stem cells. m6A mRNA methylation facilitates resolution of naive pluripotency toward differentiation. Science 347, 1002–1006. doi: 10.1126/science.1261417
Grozhik, A. V., and Jaffrey, S. R. (2018). Distinguishing RNA modifications from noise in epitranscriptome maps. Nat. Chem. Biol. 14, 215–225. doi: 10.1038/nchembio.2546
Han, D., Liu, J., Chen, C., Dong, L., Liu, Y., Chang, R., et al. (2019). Anti-tumour immunity controlled through mRNA m(6)A methylation and YTHDF1 in dendritic cells. Nature 566, 270–274. doi: 10.1038/s41586-019-0916-x
Hanahan, D., and Weinberg, R. A. (2011). Hallmarks of cancer: the next generation. Cell 144, 646–674. doi: 10.1016/j.cell.2011.02.013
Hanzelmann, S., Castelo, R., and Guinney, J. (2013). GSVA: gene set variation analysis for microarray and RNA-seq data. BMC Bioinformatics 14:7. doi: 10.1186/1471-2105-14-7
He, L., Li, H., Wu, A., Peng, Y., Shu, G., and Yin, G. (2019). Functions of N6-methyladenosine and its role in cancer. Mol. Cancer 18:176. doi: 10.1186/s12943-019-1109-9
He, L., Li, J., Wang, X., Ying, Y., Xie, H., Yan, H., et al. (2018). The dual role of N6-methyladenosine modification of RNAs is involved in human cancers. J. Cell. Mol. Med. 22, 4630–4639. doi: 10.1111/jcmm.13804
Hellmann, M. D., Ciuleanu, T. E., Pluzanski, A., Lee, J. S., Otterson, G. A., Audigier-Valette, C., et al. (2018). Nivolumab plus ipilimumab in lung cancer with a high tumor mutational burden. N. Engl. J. Med. 378, 2093–2104. doi: 10.1056/NEJMoa1801946
Hua, W., Zhao, Y., Jin, X., Yu, D., He, J., Xie, D., et al. (2018). METTL3 promotes ovarian carcinoma growth and invasion through the regulation of AXL translation and epithelial to mesenchymal transition. Gynecol. Oncol. 151, 356–365. doi: 10.1016/j.ygyno.2018.09.015
Jaffrey, S. R., and Kharas, M. G. (2017). Emerging links between m(6)A and misregulated mRNA methylation in cancer. Genome Med. 9:2. doi: 10.1186/s13073-016-0395-8
Jia, G., Fu, Y., Zhao, X., Dai, Q., Zheng, G., Yang, Y., et al. (2011). N6-methyladenosine in nuclear RNA is a major substrate of the obesity-associated FTO. Nat. Chem. Biol. 7, 885–887. doi: 10.1038/nchembio.687
Kalluri, R., and Zeisberg, M. (2006). Fibroblasts in cancer. Nat. Rev. Cancer 6, 392–401. doi: 10.1038/nrc1877
Kazandjian, D., Suzman, D. L., Blumenthal, G., Mushti, S., He, K., Libeg, M., et al. (2016). FDA approval summary: nivolumab for the treatment of metastatic non-small cell lung cancer with progression on or after platinum-based chemotherapy. Oncologist 21, 634–642. doi: 10.1634/theoncologist.2015-0507
Kwok, C. T., Marshall, A. D., Rasko, J. E., and Wong, J. J. (2017). Genetic alterations of m(6)A regulators predict poorer survival in acute myeloid leukemia. J. Hematol. Oncol. 10:39. doi: 10.1186/s13045-017-0410-6
Le, D. T., Durham, J. N., Smith, K. N., Wang, H., Bartlett, B. R., Aulakh, L. K., et al. (2017). Mismatch repair deficiency predicts response of solid tumors to PD-1 blockade. Science 357, 409–413. doi: 10.1126/science.aan6733
Lee, M., Kim, B., and Kim, V. N. (2014). Emerging roles of RNA modification: m(6)A and U-tail. Cell 158, 980–987. doi: 10.1016/j.cell.2014.08.005
Leek, J. T., Johnson, W. E., Parker, H. S., Jaffe, A. E., and Storey, J. D. (2012). The sva package for removing batch effects and other unwanted variation in high-throughput experiments. Bioinformatics 28, 882–883. doi: 10.1093/bioinformatics/bts034
Liberzon, A., Birger, C., Thorvaldsdottir, H., Ghandi, M., Mesirov, J. P., and Tamayo, P. (2015). The molecular signatures database (MSigDB) hallmark gene set collection. Cell Syst. 1, 417–425. doi: 10.1016/j.cels.2015.12.004
Liberzon, A., Subramanian, A., Pinchback, R., Thorvaldsdottir, H., Tamayo, P., and Mesirov, J. P. (2011). Molecular signatures database (MSigDB) 3.0. Bioinformatics 27, 1739–1740. doi: 10.1093/bioinformatics/btr260
Lin, S., Choe, J., Du, P., Triboulet, R., and Gregory, R. I. (2016). The m(6)A methyltransferase METTL3 promotes translation in human cancer cells. Mol. Cell 62, 335–345. doi: 10.1016/j.molcel.2016.03.021
Liu, J., Harada, B. T., and He, C. (2019). Regulation of gene expression by N(6)-methyladenosine in cancer. Trends Cell Biol. 29, 487–499. doi: 10.1016/j.tcb.2019.02.008
Liu, N., Zhou, K. I., Parisien, M., Dai, Q., Diatchenko, L., and Pan, T. (2017). N6-methyladenosine alters RNA structure to regulate binding of a low-complexity protein. Nucleic Acids Res. 45, 6051–6063. doi: 10.1093/nar/gkx141
Liu, T., Wei, Q., Jin, J., Luo, Q., Liu, Y., Yang, Y., et al. (2020). The m6A reader YTHDF1 promotes ovarian cancer progression via augmenting EIF3C translation. Nucleic Acids Res. 48, 3816–3831. doi: 10.1093/nar/gkaa048
Love, M. I., Huber, W., and Anders, S. (2014). Moderated estimation of fold change and dispersion for RNA-seq data with DESeq2. Genome Biol. 15:550. doi: 10.1186/s13059-014-0550-8
Lv, Y., Huang, Z., Lin, Y., Fang, Y., Chen, Z., Pan, L., et al. (2019). MiRNA expression patterns are associated with tumor mutational burden in lung adenocarcinoma. Oncoimmunology 8:e1629260. doi: 10.1080/2162402X.2019.1629260
Ma, J. Z., Yang, F., Zhou, C. C., Liu, F., Yuan, J. H., Wang, F., et al. (2017). METTL14 suppresses the metastatic potential of hepatocellular carcinoma by modulating N(6) -methyladenosine-dependent primary MicroRNA processing. Hepatology 65, 529–543. doi: 10.1002/hep.28885
Malta, T. M., Sokolov, A., Gentles, A. J., Burzykowski, T., Poisson, L., Weinstein, J. N., et al. (2018). Machine learning identifies stemness features associated with oncogenic dedifferentiation. Cell 173, 338–354.e15. doi: 10.1016/j.cell.2018.03.034
Mauer, J., Luo, X., Blanjoie, A., Jiao, X., Grozhik, A. V., Patil, D. P., et al. (2017). Reversible methylation of m(6)Am in the 5’ cap controls mRNA stability. Nature 541, 371–375. doi: 10.1038/nature21022
Meyer, K. D., Patil, D. P., Zhou, J., Zinoviev, A., Skabkin, M. A., Elemento, O., et al. (2015). 5’ UTR m(6)A promotes cap-independent translation. Cell 163, 999–1010. doi: 10.1016/j.cell.2015.10.012
Mootha, V. K., Lindgren, C. M., Eriksson, K. F., Subramanian, A., Sihag, S., Lehar, J., et al. (2003). PGC-1alpha-responsive genes involved in oxidative phosphorylation are coordinately downregulated in human diabetes. Nat. Genet. 34, 267–273. doi: 10.1038/ng1180
Newman, A. M., Liu, C. L., Green, M. R., Gentles, A. J., Feng, W., Xu, Y., et al. (2015). Robust enumeration of cell subsets from tissue expression profiles. Nat. Methods 12, 453–457. doi: 10.1038/nmeth.3337
Niu, Y., Lin, Z., Wan, A., Chen, H., Liang, H., Sun, L., et al. (2019). RNA N6-methyladenosine demethylase FTO promotes breast tumor progression through inhibiting BNIP3. Mol. Cancer 18:46. doi: 10.1186/s12943-019-1004-4
Pages, F., Berger, A., Camus, M., Sanchez-Cabo, F., Costes, A., Molidor, R., et al. (2005). Effector memory T cells, early metastasis, and survival in colorectal cancer. N. Engl. J. Med. 353, 2654–2666. doi: 10.1056/NEJMoa051424
Panneerdoss, S., Eedunuri, V. K., Yadav, P., Timilsina, S., Rajamanickam, S., Viswanadhapalli, S., et al. (2018). Cross-talk among writers, readers, and erasers of m(6)A regulates cancer growth and progression. Sci. Adv. 4:eaar8263. doi: 10.1126/sciadv.aar8263
Roundtree, I. A., Evans, M. E., Pan, T., and He, C. (2017). Dynamic RNA modifications in gene expression regulation. Cell 169, 1187–1200. doi: 10.1016/j.cell.2017.05.045
Rousseaux, S., Debernardi, A., Jacquiau, B., Vitte, A. L., Vesin, A., Nagy-Mignotte, H., et al. (2013). Ectopic activation of germline and placental genes identifies aggressive metastasis-prone lung cancers. Sci. Transl. Med. 5:186ra166. doi: 10.1126/scitranslmed.3005723
Schumacher, T. N., and Schreiber, R. D. (2015). Neoantigens in cancer immunotherapy. Science 348, 69–74. doi: 10.1126/science.aaa4971
Straussman, R., Morikawa, T., Shee, K., Barzily-Rokni, M., Qian, Z. R., Du, J., et al. (2012). Tumour micro-environment elicits innate resistance to RAF inhibitors through HGF secretion. Nature 487, 500–504. doi: 10.1038/nature11183
Subramanian, A., Tamayo, P., Mootha, V. K., Mukherjee, S., Ebert, B. L., Gillette, M. A., et al. (2005). Gene set enrichment analysis: a knowledge-based approach for interpreting genome-wide expression profiles. Proc. Natl. Acad. Sci. U.S.A. 102, 15545–15550. doi: 10.1073/pnas.0506580102
Torre, L. A., Bray, F., Siegel, R. L., Ferlay, J., Lortet-Tieulent, J., and Jemal, A. (2015). Global cancer statistics, 2012. CA Cancer J. Clin. 65, 87–108. doi: 10.3322/caac.21262
Vu, L. P., Pickering, B. F., Cheng, Y., Zaccara, S., Nguyen, D., Minuesa, G., et al. (2017). The N(6)-methyladenosine (m(6)A)-forming enzyme METTL3 controls myeloid differentiation of normal hematopoietic and leukemia cells. Nat. Med. 23, 1369–1376. doi: 10.1038/nm.4416
Wang, L., Hui, H., Agrawal, K., Kang, Y., Li, N., Tang, R., et al. (2020). m(6) A RNA methyltransferases METTL3/14 regulate immune responses to anti-PD-1 therapy. EMBO J. 39:e104514. doi: 10.15252/embj.2020104514
Wang, S., Chai, P., Jia, R., and Jia, R. (2018). Novel insights on m(6)A RNA methylation in tumorigenesis: a double-edged sword. Mol. Cancer 17:101. doi: 10.1186/s12943-018-0847-4
Wang, X., and He, C. (2014). Reading RNA methylation codes through methyl-specific binding proteins. RNA Biol. 11, 669–672. doi: 10.4161/rna.28829
Xiao, Y., Ma, D., Zhao, S., Suo, C., Shi, J., Xue, M. Z., et al. (2019). Multi-omics profiling reveals distinct microenvironment characterization and suggests immune escape mechanisms of triple-negative breast cancer. Clin. Cancer Res. 25, 5002–5014. doi: 10.1158/1078-0432.CCR-18-3524
Yang, S., Wei, J., Cui, Y. H., Park, G., Shah, P., Deng, Y., et al. (2019). m(6)A mRNA demethylase FTO regulates melanoma tumorigenicity and response to anti-PD-1 blockade. Nat. Commun. 10:2782. doi: 10.1038/s41467-019-10669-0
Zeng, D., Ye, Z., Wu, J., Zhou, R., Fan, X., Wang, G., et al. (2020). Macrophage correlates with immunophenotype and predicts anti-PD-L1 response of urothelial cancer. Theranostics 10, 7002–7014. doi: 10.7150/thno.46176
Zhang, C., Huang, S., Zhuang, H., Ruan, S., Zhou, Z., Huang, K., et al. (2020). YTHDF2 promotes the liver cancer stem cell phenotype and cancer metastasis by regulating OCT4 expression via m6A RNA methylation. Oncogene 39, 4507–4518. doi: 10.1038/s41388-020-1303-7
Zhang, C., Samanta, D., Lu, H., Bullen, J. W., Zhang, H., Chen, I., et al. (2016). Hypoxia induces the breast cancer stem cell phenotype by HIF-dependent and ALKBH5-mediated m(6)A-demethylation of NANOG mRNA. Proc. Natl. Acad. Sci. U.S.A. 113, E2047–E2056. doi: 10.1073/pnas.1602883113
Keywords: lung adenocarcinoma, N6-methyladenosine, tumor mutational burden, tumor-infiltrating lymphocytes, prognostic biomarker
Citation: Zhang H, Hu J, Liu A, Qu H, Jiang F, Wang C, Mo S and Sun P (2021) An N6-Methyladenosine-Related Gene Set Variation Score as a Prognostic Tool for Lung Adenocarcinoma. Front. Cell Dev. Biol. 9:651575. doi: 10.3389/fcell.2021.651575
Received: 10 January 2021; Accepted: 04 June 2021;
Published: 08 July 2021.
Edited by:
Rui Henrique, Portuguese Oncology Institute, PortugalReviewed by:
Sarah Ashley, Royal Children’s Hospital, AustraliaHernando Lopez Bertoni, Johns Hopkins Medicine, United States
Copyright © 2021 Zhang, Hu, Liu, Qu, Jiang, Wang, Mo and Sun. This is an open-access article distributed under the terms of the Creative Commons Attribution License (CC BY). The use, distribution or reproduction in other forums is permitted, provided the original author(s) and the copyright owner(s) are credited and that the original publication in this journal is cited, in accordance with accepted academic practice. No use, distribution or reproduction is permitted which does not comply with these terms.
*Correspondence: Steven Mo, c3dtb0Bpb24uYWMuY24=; Ping Sun, c3VucGluZzIwMDM5QGhvdG1haWwuY29t
†These authors have contributed equally to this work and share first authorship