- 1Department of Thyroid Surgery, Guangzhou First People’s Hospital, School of Medicine, South China University of Technology, Guangzhou, China
- 2Department of Thyroid Surgery, Guangzhou First People’s Hospital, Guangzhou Medical University, Guangzhou, China
- 3Department of Hepatobiliary, Pancreatic and Splenic Surgery, Guangzhou First People’s Hospital, School of Medicine, South China University of Technology, Guangzhou, China
Thyroid carcinoma (TC) is the most common endocrine malignancy. The incidence rate of thyroid cancer has increased rapidly in recent years. The occurrence and development of thyroid cancers are highly related to the massive genetic and epigenetic changes. Therefore, it is essential to explore the mechanism of thyroid cancer pathogenesis. Genome-Wide Association Studies (GWAS) have been widely used in various diseases. Researchers have found multiple single nucleotide polymorphisms (SNPs) are significantly related to TC. However, the biological mechanism of these SNPs is still unknown. In this paper, we used one GWAS dataset and two eQTL datasets, and integrated GWAS with expression quantitative trait loci (eQTL) in both thyroid and blood to explore the mechanism of mutations and causal genes of thyroid cancer. Finally, we found rs1912998 regulates the expression of IGFALS (P = 1.70E-06) and HAGH (P = 5.08E-07) in thyroid, which is significantly related to thyroid cancer. In addition, KEGG shows that these genes participate in multiple thyroid cancer-related pathways.
Introduction
Thyroid carcinoma (TC) is one of the most common endocrine tumors (Cabanillas et al., 2016). The incidence rate has increased year by year in recent 30 years, driven largely by increases in Differentiated TC. At present, the research and treatment of TC are still unclear. Ionizing radiation is the only known carcinogenic mechanism, while other causes are still unclear (Albi et al., 2017). Differentiated TC is the most frequent subtype, and the main treatment methods of differentiated TC include surgical treatment (Asimakopoulos and Nixon, 2018), radioiodine therapy (Klain et al., 2002) and thyroid-stimulating hormone (TSH) suppression treatment (Haugen et al., 2016), while the treatment of poorly differentiated thyroid cancer (PDTC) is still very limited (Ibrahimpasic et al., 2019). With the development of sequencing technology and the decrease of cost, research on TC is progressing at the genetic level, it has been found that many genes are related to the occurrence, development, and prognosis of TC, which provides a new direction for early diagnosis, prognosis judgment and targeted treatment of TC.
BRAF gene plays an important role in the development of TC, especially in papillary thyroid cancer (PTC) (Pusztaszeri et al., 2015). BRAF gene mutations are most common in PTC, and less in other pathological types (Chen et al., 2018). Multiple biological experiments in mice and humans have found that BRAF mutation is significantly associated with the prognosis of PTC (Nakamura et al., 2005; Zou et al., 2009; Ohori et al., 2013). RET mutations are closely associated with hereditary myeloid carcinoma-related diseases, such as familial thyroid myeloid carcinoma, multiple endocrine adenomatosis type 2A, multiple endocrine adenomatosis type 2B, etc. (Gandhi et al., 2010; Accardo et al., 2017). It has also been suggested that RET gene plays an important role in evaluating the need for preventive thyroidectomy and the selection of surgical operation (Cohen and Moley, 2003; Hirsch et al., 2018). However, studies have found that the rate of RET gene mutations in Chinese PTC patients is very low, which means the relationship between RET and TC still needs further study (Wang et al., 2008). RAS mutations also occur in thyroid cancers, which frequently occur in follicular thyroid cancer and follicular variant papillary thyroid cancer (Garcia-Rendueles et al., 2015; Cabanillas et al., 2016). Other wide known TC-related genes, such as TP53 (Wang et al., 2014), PAX8-PPAR (Boos et al., 2013), TERT (Liu and Xing, 2016), PTEN (Puxeddu et al., 2005), and KRAS (Al-Salam et al., 2020), etc., are all found by complicated biological experiments. This leads to a high cost in time and money.
Using the computational method to handle complex networks provides a new way to study disease-related genes (Zhao et al., 2020a,b). The most commonly used network is the gene regulatory network which is a directed graph. Genes are the nodes of the network, and the regulatory relationship between genes is used to connect nodes and form directed edges (Zhao et al., 2020c). A large number of computational methods based on complex networks have been studied. Most of these methods identify important genes by measuring the centrality of network nodes, and the degree centrality method is representative of this kind of methods. The degree of a node is the number of nodes directly connected with it. It is generally believed that the degree of gene is directly proportional to the importance of gene. According to the importance of genes, we can sort the relationship between genes and diseases in the network.
Genome-Wide Association Studies (GWAS) have been widely used in various diseases. Rogounovitch et al. (2015) conducted a GWAS study using 507 sporadic PTC patients and 2,766 control subjects in the Japanese population. Jones et al. (2012) compared 781 PTC patients with 6,122 health controls in England population to find TC-related SNPs. Penna-Martinez et al. (2014) categorized rs944289 in 243 patients with differentiated TC and 270 healthy individuals in the German population, and the results indicated that rs944289 may play a minor role in the occurrence of differentiated thyroid cancer in the German population. Wang et al. (2013) selected 845 patients with PTC, 503 patients with thyroid benign Node (TBN), and 1,005 healthy controls in east China to conduct GWAS, and found that four candidate loci, rs965513 (9q22.33), rs944289 (14q13.3), rs966423 (2q35), and rs2439302 (8p12), identified for PTC risk in a Chinese population. Wei et al. (2015) collected anticoagulant blood samples from 1,237 thyroid tumor patients and 760 healthy people of Chinese Han nationality, and collected and compared clinic-pathological data. Although these GWAS studies revealed multiple TC-related SNPs, the biological mechanism between TC and these SNPs is still unknown.
Expression quantitative trait loci (eQTL) refers to regions on chromosomes that can specifically regulate the expression level of mRNA/protein, and the expression level of mRNA/protein is proportional to the quantitative traits (Doss et al., 2005). eQTL can be divided into cis-eQTL and trans-eQTL. Cis-eQTL is that the eQTL of a gene is located in the genomic region of the gene, which indicates that the change of mRNA level may be caused by the difference of the gene itself. Overall, eQTL describes the ability of SNP to regulate gene expression.
At present, a considerable number of studies have taken eQTL as a very effective tool for in-depth interpretation of GWAS results (Yin et al., 2014; Zhao et al., 2020d, 2021). With the increase of sample size, the problem of low statistical efficiency caused by the small sample size in the past has gradually improved. Recently, studies on eQTL have gradually begun to be carried out in several major tissues of human body, and it has been found that many eQTLs are tissue-specific, that is, some SNPs only work in specific tissues or cell types (Van Nas et al., 2010; Simon et al., 2016; Battle et al., 2017). Therefore, the thyroid and peripheral blood of eQTL are needed to study TC-related genes.
In this study, a mendelian randomization-based method was used to integrate GWAS and eQTL data, which reveals the biological mechanism of significant SNPs and causal genes of TC.
Materials and Methods
Dataset
In this study, we used one GWAS dataset and two eQTL datasets. Rashkin et al. (2020) undertook GWAS and conducted a comprehensive assessment of the heritability and pleiotropy of 18 cancer types in two large, population-based cohorts: the United Kingdom Biobank (408,786 European ancestry individuals; 48,961 cancer cases) and the Kaiser Permanente Genetic Epidemiology Research on Adult Health and Aging cohorts (66,526 European ancestry individuals; 16,001 cancer cases).
The “Beta” and “P value” of all SNPs in the GWAS dataset are shown in Figures 1A,B.
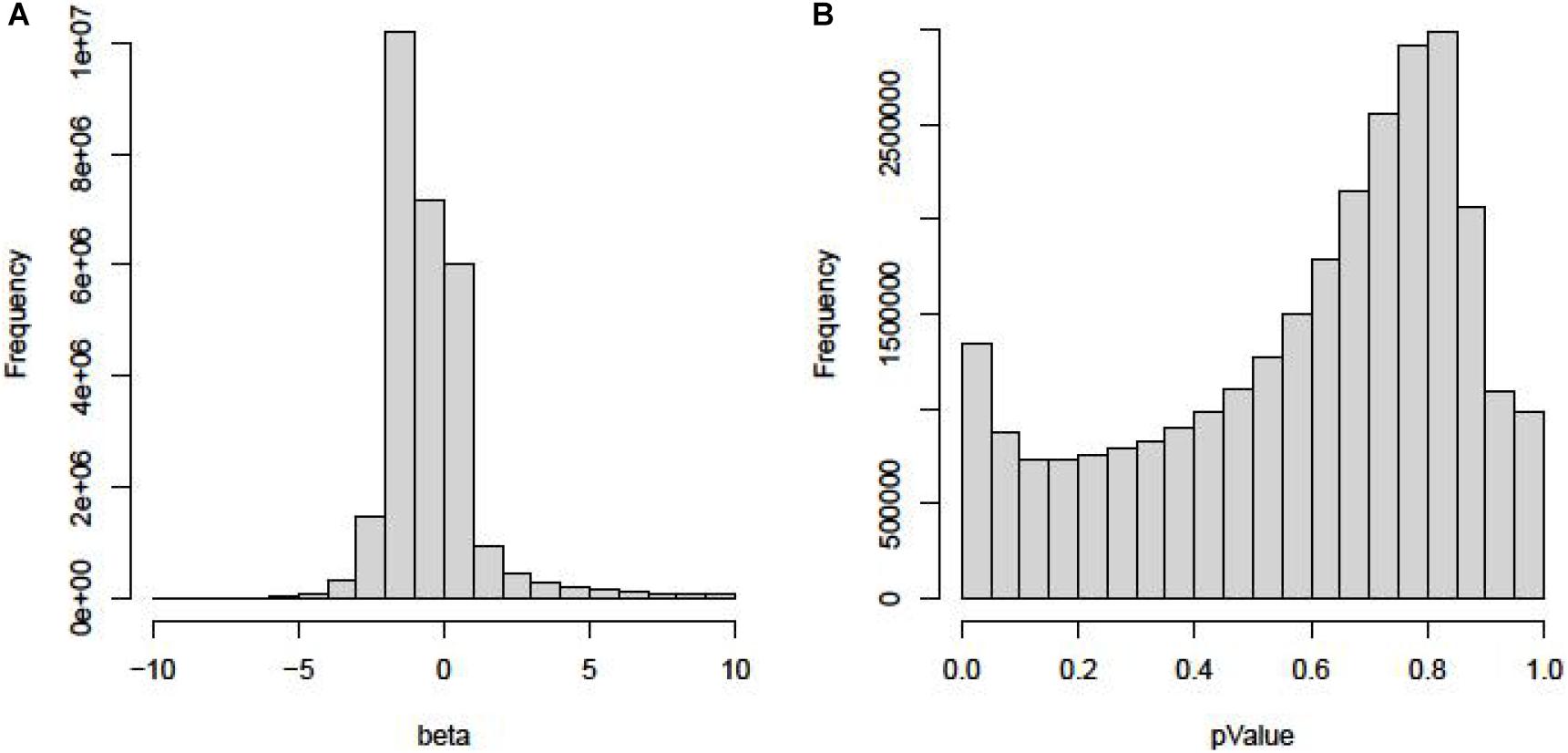
Figure 1. The “Beta” and “P-value” of all SNPs in the GWAS dataset. (A) Histogram of Beta in GWAS. (B) Histogram of P value in GWAS.
As we can see in Figure 1A, most of the beta are between −1 and −2. Few SNPs have high beta values, which mean that these mutations pose a high risk of TC. In Figure 1B, the P value of 99% SNPs is higher than 0.05.
The eQTL data of blood tissue is downloaded from Genotype-Tissue Expression (GTEx) (GTEx Consortium, 2013). Using 12,360 gene expression probes, this dataset includes 1,272,372 eQTL loci. eQTL data of thyroid tissue is downloaded from GTEx too. Using 17,664 gene expression probes, this dataset includes 1,757,598 eQTL loci. The distributions of these two datasets are shown in Figure 2.
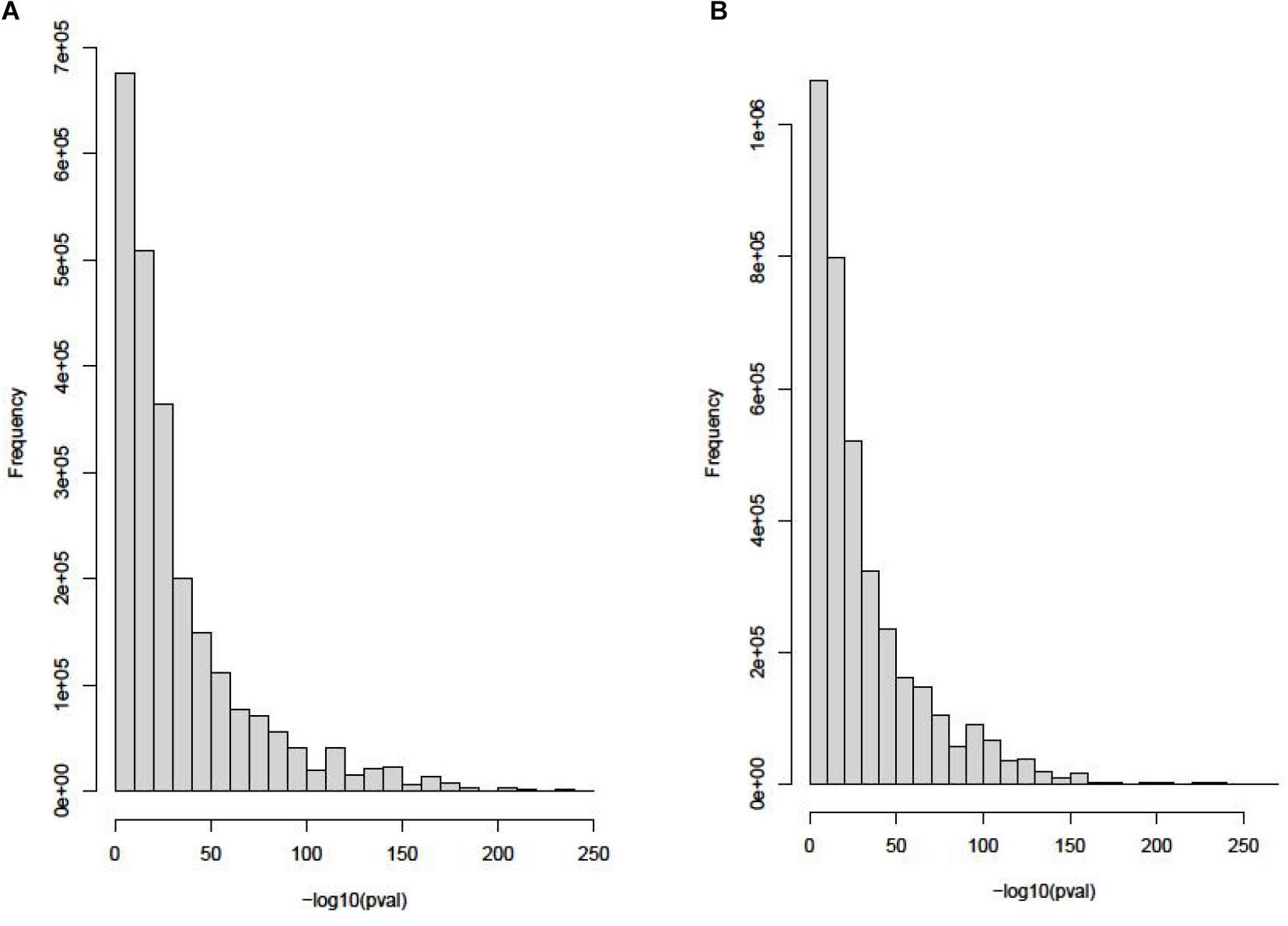
Figure 2. The distributions of blood tissue and thyroid tissue in the eQTL data. (A) Histogram of P value in eQTL of blood tissue. (B) Histogram of P value in eQTL of thyroid tissue.
Summary Data-Based Mendelian Randomization
Potential and unmeasurable confounding factors, such as growth environment, economic factors, behavior, and so on, lead to great challenges in inferring the causal relationship between genes and TC. The allele Randomization rate in Mendelian genetic law is an important theoretical support of the Mendelian Randomization (MR) method. If the phenotype is determined by genotype, a genotype is related to the disease by controlling phenotype. Therefore, the genotype can be used as an instrumental variable to infer the causal relationship between phenotype and disease.
Zhu et al. (2016) developed a summary data-based Mendelian Randomization (SMR) method to test the associations between gene expression and five complex traits. Then, SMR has been widely used in various diseases, such as Alzheimer’s disease (AD) (Zhao et al., 2019, 2020e), Bone Mineral Density (BMD) (Meng et al., 2018), Amyotrophic Lateral Sclerosis (ALS) (Du et al., 2018), etc.
Let x be exposure factors (Gene expression), y be outcome variables (phenotype), z be instrumental variable (SNP). bxy is defined as the effect of x on y.
The variance of the association between gene and TC should be:
is the estimated value of bxy. is the variance that gene expression regresses to the TC. is the variance that SNP regresses to the gene expression. n is the sample size.
Therefore, we can define TSMR to test the significance of bxy.
where , , p is the Allele frequency.
Since TSMR obeys the chi-square distribution with degree of freedom of 1, we can obtain a P value for each SNP.
The workflow of our work is as following:
As shown in Figure 3, we can obtain the statistic TSMR from GWAS and eQTL. Since we used eQTL datasets from two tissues, we totally obtained two TSMR. We test TSMR by chi-square test to find casual genes of TC.
Results
Results of SMR Test
Since 12,360 probes in the peripheral blood eQTL data set labeled all SNPs, and each probe represents a gene, we conducted 12,360 hypothesis tests. According to the Bonferroni correction method, we set the threshold as 0.05/12360 = 4.04 e-06.
The result of SMR by using peripheral blood eQTL and GWAS is shown in Figure 4.
As shown in Figure 4, none of SNPs passed the test and reach the significance in this test. Then we tested by thyroid eQTL dataset.
There are 17,664 probes in the thyroid eQTL dataset, so the threshold is 0.05/17664 = 2.83e-6.
As we can see in Figure 5 and Table 1, rs1912998 passed the test and regulated two gene expressions, IGFALS (P = 1.70E-06) and HAGH (P = 5.08E-07).
Case Study
We explore the pathway of both IGFALS and HAGH in KEGG (Kanehisa and Goto, 2000).
IGFALS (hsa3483) is described as insulin-like growth factor binding protein acid-labile subunit, which participates in a pathway named growth hormone synthesis, secretion, and action (hsa04935) (Högler et al., 2014). The mutations caused by IGFALS are always associated with the endocrine system. According to the classification of the KEGG disease database, thyroid cancer (H00032) is a kind of malignant neoplasms of endocrine glands. In the pathway of transcriptional misregulation in cancer, which is related to the disease thyroid cancer, IGF system members are participating in the process of tumor growth and survival.
In the research of Vella et al. (2001), they found that the activation of the insulin-like growth factor (IGF) system in cancer has emerged as a key factor for tumor progression and resistance to apoptosis, and experiments validated that IGF system members were found to be locally produced in thyroid cancer.
HAGH (hsa3029) is described as hydroxyacylglutathione hydrolase, which participates in the pathways Pyruvate metabolism (hsa00620) and Metabolic pathways (hsa01100). This indicates that HAGH is associated with pyruvate kinase. Pyruvate kinase is an enzyme that catalyzes the conversion of phosphoenolpyruvate and ADP to pyruvate and ATP in glycolysis and displays a role in regulating cell metabolism (Goswami et al., 2015). TC is the most frequent endocrine tumor with a growing incidence worldwide. Recently, new emphasis has been given to the altered cellular metabolism of proliferating cancer cells which require a high amount of glucose for energy production and macromolecules biosynthesis. Also, TC displays alteration of energy metabolism orchestrated by oncogenes activation and tumor suppressors inactivation leading to abnormal proliferation. Furthermore, TC shows significant metabolic heterogeneity within the tumor microenvironment and metabolic coupling between cancer and stromal cells (Ciavardelli et al., 2017).
In the study of Liu et al. (2020), overexpression of PPAT (Phosphoribosyl pyrophosphate amidotransferase) significantly promotes tumor cell proliferation and invasion via activating pyruvate kinase (PK, which related to HAGH). And they identified that PPAT plays a crucial role in regulating proliferation, migration, and invasion of thyroid cancer.
Gene Regulatory Network
We explored the interactions between IGFALS/HAGH and other genes in the String database.
Figure 6 only shows the interaction score above 0.7. Among these genes, several studies have reported the association between IGF1, IGFBP3, IGF2, and TC. Polat et al. (2016) enrolled seventy TC patients and 84 age-matched controls and found that IGFBP3 levels in serum were significantly higher in TC patients compared to the control group.
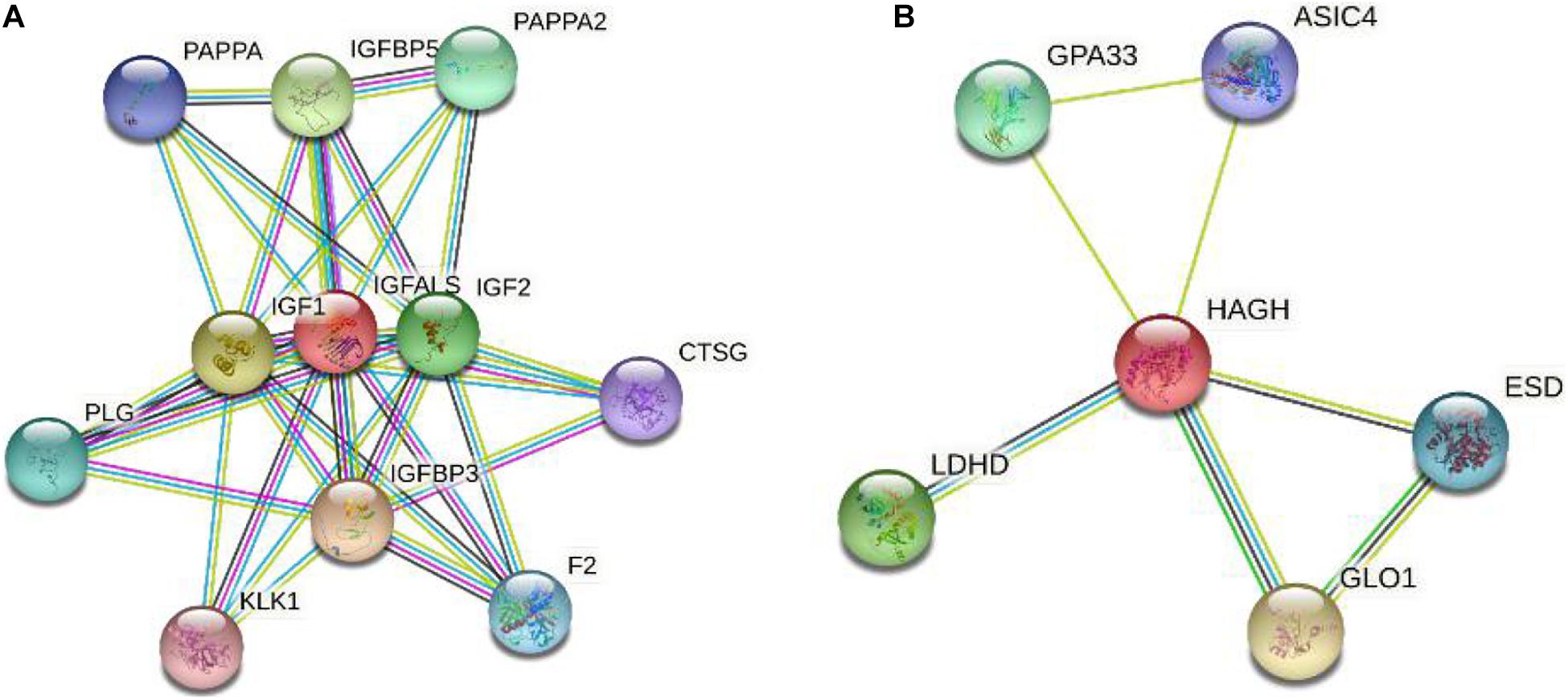
Figure 6. Gene interaction network of IGFALS and HAGH. (A) Gene interaction network of IGFALS. (B) Gene interaction network of HAGH.
Conclusion
The etiology and pathogenesis of TC are still unclear. Studies have shown that high iodine, radiation exposure, chronic lymphocytic thyroiditis, oral contraceptives, and other factors have a certain role in contributing to the incidence of TC, but not all the people exposed to these pathogenic factors will lead to the occurrence of TC. This individual difference is determined by the genetic susceptibility of the tumor. A number of research teams have used GWAS to find the genetic susceptibility locus of TC. However, the biological mechanism of these SNPs is still unknown.
In this paper, we integrated GWAS with eQTL in thyroid and blood to explore the pathogenic mechanism of susceptibility locus and find casual genes of TC. Although none of SNPs passed the SMR test in the blood eQTL dataset, the expression of the IGFALS and HAGH mediated by rs1912998 was significantly correlated with TC by using thyroid eQTL. By searching their pathway in KEGG and exploring their regulatory network, we found the functions of both IGFALS and HAGH show potential correlation with TC.
In this study, we reveal effector genes via GWAS with eQTL in both thyroid and blood, and provide evidence for further studies about the potential effect of these genes within TC. Although we discussed our results in multiple aspects, these conclusions still need to be further verified by biological experiments.
Data Availability Statement
Publicly available datasets were analyzed in this study. This data can be found here. GWAS: http://ftp.ebi.ac.uk/pub/databases/gwas/summary_statistics/RashkinSR_32887889_GCST90011813 and eQTL: https://storage.googleapis.com/gtex_analysis_v8/single_tissue_qtl_data/GTEx_Analysis_v8_eQTL.tar.
Ethics Statement
Ethical review and approval was not required for the study on human participants in accordance with the local legislation and institutional requirements. Written informed consent for participation was not required for this study in accordance with the national legislation and the institutional requirements.
Author Contributions
FS, XG, RZ, WC, and BX carried out the GWAS with expression quantitative trait loci (eQTL) method to explore the mechanism of mutations and causal genes of thyroid cancer and participated in its design. JF, ZC, MG, YL, and ZW analyzed the data. FS, XG, and RZ wrote the manuscript. All authors read and approved the final manuscript.
Funding
Financial support comes from the Guangzhou Medicine and Health Care Technology Projects (20191A010012).
Conflict of Interest
The authors declare that the research was conducted in the absence of any commercial or financial relationships that could be construed as a potential conflict of interest.
References
Accardo, G., Conzo, G., Esposito, D., Gambardella, C., Mazzella, M., Castaldo, F., et al. (2017). Genetics of medullary thyroid cancer: an overview. Int. J. Surg. 41, S2–S6. doi: 10.1016/j.ijsu.2017.02.064
Albi, E., Cataldi, S., Lazzarini, A., Codini, M., Beccari, T., Ambesi-Impiombato, F. S., et al. (2017). Radiation and thyroid cancer. Int. J. Mol. Sci. 18:911. doi: 10.3390/ijms18050911
Al-Salam, S., Sharma, C., Afandi, B., Al Dahmani, K., Al-Zahrani, A. S., Al Shamsi, A., et al. (2020). BRAF and KRAS mutations in papillary thyroid carcinoma in the United Arab Emirates. PLoS One 15:e0231341. doi: 10.1371/journal.pone.0231341
Asimakopoulos, P., and Nixon, I. J. (2018). Surgical management of primary thyroid tumours. Eur. J. Surg. Oncol. 44, 321–326. doi: 10.1016/j.ejso.2016.12.015
Battle, A., Brown, C. D., Engelhardt, B. E., and Montgomery, S. B. (2017). Genetic effects on gene expression across human tissues. Nature 550, 204–213. doi: 10.1038/nature24277
Boos, L. A., Dettmer, M., Schmitt, A., Rudolph, T., Steinert, H., Moch, H., et al. (2013). Diagnostic and prognostic implications of the PAX8-PPARγ translocation in thyroid carcinomas-a TMA-based study of 226 cases. Histopathology 63, 234–241. doi: 10.1111/his.12150
Cabanillas, M. E., McFadden, D. G., and Durante, C. (2016). Thyroid cancer. Lancet 388, 2783–2795. doi: 10.1016/s0140-6736(16)30172-6
Chen, D., Qi, W., Zhang, P., Zhang, Y., Liu, Y., Guan, H., et al. (2018). Investigation of BRAF V600E detection approaches in papillary thyroid carcinoma. Pathol. Res. Pract. 214, 303–307. doi: 10.1016/j.prp.2017.09.001
Ciavardelli, D., Bellomo, M., Consalvo, A., Crescimanno, C., and Vella, V. (2017). Metabolic alterations of thyroid cancer as potential therapeutic targets. Biomed. Res. Int. 2017:2545031. doi: 10.1155/2017/2545031
Cohen, M. S., and Moley, J. F. (2003). Surgical treatment of medullary thyroid carcinoma. J. Intern. Med. 253, 616–626. doi: 10.1046/j.1365-2796.2003.01166.x
Doss, S., Schadt, E. E., Drake, T. A., and Lusis, A. J. (2005). Cis-acting expression quantitative trait loci in mice. Genome Res. 15, 681–691. doi: 10.1101/gr.3216905
Du, Y., Wen, Y., Guo, X., Hao, J., Wang, W., He, A., et al. (2018). A genome-wide expression association analysis identifies genes and pathways associated with amyotrophic lateral sclerosis. Cell Mol. Neurobiol. 38, 635–639. doi: 10.1007/s10571-017-0512-2
Gandhi, M., Dillon, L. W., Pramanik, S., Nikiforov, Y. E., and Wang, Y. H. (2010). DNA breaks at fragile sites generate oncogenic RET/PTC rearrangements in human thyroid cells. Oncogene 29, 2272–2280. doi: 10.1038/onc.2009.502
Garcia-Rendueles, M. E., Ricarte-Filho, J. C., Untch, B. R., Landa, I., Knauf, J. A., Voza, F., et al. (2015). NF2 Loss promotes oncogenic RAS-induced thyroid cancers via YAP-dependent transactivation of RAS proteins and sensitizes them to MEK inhibition. Cancer Discov. 5, 1178–1193. doi: 10.1158/2159-8290.cd-15-0330
Goswami, M. T., Chen, G., Chakravarthi, B. V., Pathi, S. S., Anand, S. K., Carskadon, S. L., et al. (2015). Role and regulation of coordinately expressed de novo purine biosynthetic enzymes PPAT and PAICS in lung cancer. Oncotarget 6, 23445–23461. doi: 10.18632/oncotarget.4352
GTEx Consortium (2013). The genotype-tissue expression (GTEx) project. Nat. Genet. 45, 580–585. doi: 10.1038/ng.2653
Haugen, B. R., Alexander, E. K., Bible, K. C., Doherty, G. M., Mandel, S. J., Nikiforov, Y. E., et al. (2016). 2015 American thyroid association management guidelines for adult patients with thyroid nodules and differentiated thyroid cancer: the american thyroid association guidelines task force on thyroid nodules and differentiated thyroid cancer. Thyroid 26, 1–133. doi: 10.1089/thy.2015.0020
Hirsch, D., Twito, O., Levy, S., Bachar, G., Robenshtok, E., Gross, D. J., et al. (2018). Temporal trends in the presentation, treatment, and outcome of medullary thyroid carcinoma: an israeli multicenter study. Thyroid 28, 369–376. doi: 10.1089/thy.2017.0371
Högler, W., Martin, D. D., Crabtree, N., Nightingale, P., Tomlinson, J., and Metherell, L. (2014). IGFALS gene dosage effects on serum IGF-I and glucose metabolism, body composition, bone growth in length and width, and the pharmacokinetics of recombinant human IGF-I administration. J. Clin. Endocrinol. Metab. 99, E703–E712. doi: 10.1210/jc.2013-3718
Ibrahimpasic, T., Ghossein, R., Shah, J. P., and Ganly, I. (2019). Poorly differentiated carcinoma of the thyroid gland: current status and future prospects. Thyroid 29, 311–321. doi: 10.1089/thy.2018.0509
Jones, A. M., Howarth, K. M., Martin, L., Gorman, M., Mihai, R., Moss, L., et al. (2012). Thyroid cancer susceptibility polymorphisms: confirmation of loci on chromosomes 9q22 and 14q13, validation of a recessive 8q24 locus and failure to replicate a locus on 5q24. J. Med. Genet. 49, 158–163. doi: 10.1136/jmedgenet-2011-100586
Kanehisa, M., and Goto, S. (2000). KEGG: kyoto encyclopedia of genes and genomes. Nucleic Acids Res. 28, 27–30. doi: 10.1093/nar/28.1.27
Klain, M., Ricard, M., Leboulleux, S., Baudin, E., and Schlumberger, M. (2002). Radioiodine therapy for papillary and follicular thyroid carcinoma. Eur. J. Nucl. Med. Mol. Imaging 29, S479–S485. doi: 10.1007/s00259-002-0810-9
Liu, B., Song, M., Qin, H., Zhang, B., Liu, Y., Sun, Y., et al. (2020). Phosphoribosyl pyrophosphate amidotransferase promotes the progression of thyroid cancer via regulating pyruvate kinase M2. Onco Targets Ther. 13, 7629–7639. doi: 10.2147/ott.s253137
Liu, R., and Xing, M. (2016). TERT promoter mutations in thyroid cancer. Endocr. Relat. Cancer 23, R143–R155. doi: 10.1530/erc-15-0533
Meng, X. H., Chen, X. D., Greenbaum, J., Zeng, Q., You, S. L., Xiao, H. M., et al. (2018). Integration of summary data from GWAS and eQTL studies identified novel causal BMD genes with functional predictions. Bone 113, 41–48. doi: 10.1016/j.bone.2018.05.012
Nakamura, N., Carney, J. A., Jin, L., Kajita, S., Pallares, J., Zhang, H., et al. (2005). RASSF1A and NORE1A methylation and BRAFV600E mutations in thyroid tumors. Lab. Invest. 85, 1065–1075. doi: 10.1038/labinvest.3700306
Ohori, N. P., Singhal, R., Nikiforova, M. N., Yip, L., Schoedel, K. E., Coyne, C., et al. (2013). BRAF mutation detection in indeterminate thyroid cytology specimens: underlying cytologic, molecular, and pathologic characteristics of papillary thyroid carcinoma. Cancer Cytopathol. 121, 197–205. doi: 10.1002/cncy.21229
Penna-Martinez, M., Epp, F., Kahles, H., Ramos-Lopez, E., Hinsch, N., Hansmann, M. L., et al. (2014). FOXE1 association with differentiated thyroid cancer and its progression. Thyroid 24, 845–851. doi: 10.1089/thy.2013.0274
Polat, S. B., Oğuz, O., Sacikara, M., Cuhaci, F. N., Evranos, B., Ersoy, R., et al. (2016). Thyroid disorders in young females with polycystic ovary syndrome and correlation of thyroid volume with certain hormonal parameters. J. Reprod. Med. 61, 27–32.
Pusztaszeri, M. P., Krane, J. F., and Faquin, W. C. (2015). BRAF testing and thyroid FNA. Cancer Cytopathol. 123, 689–695. doi: 10.1002/cncy.21614
Puxeddu, E., Zhao, G., Stringer, J. R., Medvedovic, M., Moretti, S., Fagin, J. A. et al. (2005). Characterization of novel non-clonal intrachromosomal rearrangements between the H4 and PTEN genes (H4/PTEN) in human thyroid cell lines and papillary thyroid cancer specimens. Mutat. Res. 570, 17–32. doi: 10.1016/j.mrfmmm.2004.09.010
Rashkin, S. R., Graff, R. E., Kachuri, L., Thai, K. K., Alexeeff, S. E., Blatchins, M. A., et al. (2020). Pan-cancer study detects genetic risk variants and shared genetic basis in two large cohorts. Nat. Commun. 11:4423. doi: 10.1038/s41467-020-18246-6
Rogounovitch, T. I., Bychkov, A., Takahashi, M., Mitsutake, N., Nakashima, M., Nikitski, A. V., et al. (2015). The common genetic variant rs944289 on chromosome 14q13.3 associates with risk of both malignant and benign thyroid tumors in the japanese population. Thyroid 25, 333–340. doi: 10.1089/thy.2014.0431
Simon, L. M., Chen, E. S., Edelstein, L. C., Kong, X., Bhatlekar, S., Rigoutsos, I., et al. (2016). Integrative multi-omic analysis of human platelet eQTLs reveals alternative start site in mitofusin 2. Am. J. Hum. Genet. 98, 883–897. doi: 10.1016/j.ajhg.2016.03.007
Van Nas, A., Ingram-Drake, L., Sinsheimer, J. S., Wang, S. S., Schadt, E. E., Drake, T., et al. (2010). Expression quantitative trait loci: replication, tissue- and sex-specificity in mice. Genetics 185, 1059–1068. doi: 10.1534/genetics.110.116087
Vella, V., Sciacca, L., Pandini, G., Mineo, R., Squatrito, S., Vigneri, R., et al. (2001). The IGF system in thyroid cancer: new concepts. Mol. Pathol. 54, 121–124. doi: 10.1136/mp.54.3.121
Wang, F., Wang, P., Wang, B., Fu, Z. J., Yuan, Y., Yan, S. L., et al. (2014). Association between TP53 Arg72Pro polymorphism and thyroid carcinoma risk. Tumour Biol. 35, 2723–2728. doi: 10.1007/s13277-013-1359-x
Wang, Y. L., Feng, S. H., Guo, S. C., Wei, W. J., Li, D. S., Wang, Y., et al. (2013). Confirmation of papillary thyroid cancer susceptibility loci identified by genome-wide association studies of chromosomes 14q13, 9q22, 2q35 and 8p12 in a Chinese population. J. Med. Genet. 50, 689–695. doi: 10.1136/jmedgenet-2013-101687
Wang, Y. L., Zhang, R. M., Luo, Z. W., Wu, Y., Du, X., Wang, Z. Y., et al. (2008). High frequency of level II-V lymph node involvement in RET/PTC positive papillary thyroid carcinoma. Eur. J. Surg. Oncol. 34, 77–81. doi: 10.1016/j.ejso.2007.08.012
Wei, W. J., Lu, Z. W., Wang, Y., Zhu, Y. X., Wang, Y. L., and Ji, Q. H. (2015). Clinical significance of papillary thyroid cancer risk loci identified by genome-wide association studies. Cancer Genet. 208, 68–75. doi: 10.1016/j.cancergen.2015.01.004
Yin, X., Cheng, H., Lin, Y., Fan, X., Cui, Y., Zhou, F., et al. (2014). Five regulatory genes detected by matching signatures of eQTL and GWAS in psoriasis. J. Dermatol. Sci. 76, 139–142. doi: 10.1016/j.jdermsci.2014.07.007
Zhao, T., Hu, Y., and Cheng, L. (2020a). Deep-DRM: a computational method for identifying disease-related metabolites based on graph deep learning approaches. Brief Bioinform. 2020:bbaa212. doi: 10.1093/bib/bbaa212
Zhao, T., Hu, Y., Peng, J., and Cheng, L. (2020b). DeepLGP: a novel deep learning method for prioritizing lncRNA target genes. Bioinformatics 36, 4466–4472. doi: 10.1093/bioinformatics/btaa428
Zhao, T., Hu, Y., Valsdottir, L. R., Zang, T., and Peng, J. (2020c). Identifying drug-target interactions based on graph convolutional network and deep neural network. Brief Bioinform. 2010:bbaa004. doi: 10.1093/bib/bbaa044
Zhao, T., Hu, Y., Zang, T., and Cheng, L. (2020d). MRTFB regulates the expression of NOMO1 in colon. Proc. Natl. Acad. Sci. U S A. 117, 7568–7569. doi: 10.1073/pnas.2000499117
Zhao, T., Hu, Y., Zang, T., and Wang, Y. (2019). Integrate GWAS, eQTL, and mQTL Data to identify Alzheimer’s Disease-related genes. Front. Genet. 10:1021. doi: 10.3389/fgene.2019.01021
Zhao, T., Hu, Y., Zang, T., and Wang, Y. (2020e). Identifying protein biomarkers in blood for Alzheimer’s Disease. Front. Cell Dev. Biol. 8:472. doi: 10.3389/fcell.2020.00472
Zhao, T., Lyu, S., Lu, G., Juan, L., Zeng, X., Wei, Z., et al. (2021). SC2disease: a manually curated database of single-cell transcriptome for human diseases. Nucleic Acids Res. 49, D1413–D1419. doi: 10.1093/nar/gkaa838
Zhu, Z., Zhang, F., Hu, H., Bakshi, A., Robinson, M. R., Powell, J. E., et al. (2016). Integration of summary data from GWAS and eQTL studies predicts complex trait gene targets. Nat. Genet. 48, 481–487. doi: 10.1038/ng.3538
Keywords: thyroid carcinoma, rs1912998, IGFALS, HAGH, GWAS, eQTL
Citation: Shen F, Gan X, Zhong R, Feng J, Chen Z, Guo M, Li Y, Wu Z, Cai W and Xu B (2021) Identifying Thyroid Carcinoma-Related Genes by Integrating GWAS and eQTL Data. Front. Cell Dev. Biol. 9:645275. doi: 10.3389/fcell.2021.645275
Received: 22 December 2020; Accepted: 15 January 2021;
Published: 04 February 2021.
Edited by:
Liang Cheng, Harbin Medical University, ChinaReviewed by:
Hui Ding, University of Electronic Science and Technology of China, ChinaHaixiu Yang, Harbin Medical University, China
Copyright © 2021 Shen, Gan, Zhong, Feng, Chen, Guo, Li, Wu, Cai and Xu. This is an open-access article distributed under the terms of the Creative Commons Attribution License (CC BY). The use, distribution or reproduction in other forums is permitted, provided the original author(s) and the copyright owner(s) are credited and that the original publication in this journal is cited, in accordance with accepted academic practice. No use, distribution or reproduction is permitted which does not comply with these terms.
*Correspondence: Wensong Cai, Y2Fpd2Vuc29uZ0AxNjMuY29t; Bo Xu, ZXlib3h1QHNjdXQuZWR1LmNu
†These authors share first authorship