- 1Department of Biochemistry and Molecular Biology, School of Basic Medical Sciences, Fujian Medical University, Fuzhou, China
- 2Department of Clinical Laboratory, Jinjiang Municipal Hospital, Jinjiang, China
- 3Microbiological Laboratory Sanming Center for Disease Control and Prevention, Sanming, China
- 4Department of Clinical Laboratory, The Second Affiliated Hospital of Fujian Medical University, Quanzhou, China
- 5Department of Clinical Laboratory, Quanzhou Women’s and Children’s Hospital, Quanzhou, China
To distinguish Methicillin-Resistant Staphylococcus aureus (MRSA) from Methicillin-Sensitive Staphylococcus aureus (MSSA) in the protein sequences level, test the susceptibility to antibiotic of all Staphylococcus aureus isolates from Quanzhou hospitals, define the virulence factor and molecular characteristics of the MRSA isolates. MRSA and MSSA Pfam protein sequences were used to extract feature vectors of 188D, n-gram and 400D. Weka software was applied to classify the two Staphylococcus aureus and performance effect was evaluated. Antibiotic susceptibility testing of the 81 Staphylococcus aureus was performed by the Mérieux Microbial Analysis Instrument. The 65 MRSA isolates were characterized by Panton-Valentine leukocidin (PVL), X polymorphic region of Protein A (spa), multilocus sequence typing test (MLST), staphylococcus chromosomal cassette mec (SCCmec) typing. After comparing the results of Weka six classifiers, the highest correctly classified rates were 91.94, 70.16, and 62.90% from 188D, n-gram and 400D, respectively. Antimicrobial susceptibility test of the 81 Staphylococcus aureus: Penicillin-resistant rate was 100%. No resistance to teicoplanin, linezolid, and vancomycin. The resistance rate of the MRSA isolates to clindamycin, erythromycin and tetracycline was higher than that of the MSSAs. Among the 65 MRSA isolates, the positive rate of PVL gene was 47.7% (31/65). Seventeen sequence types (STs) were identified among the 65 isolates, and ST59 was the most prevalent. SCCmec type III and IV were observed at 24.6 and 72.3%, respectively. Two isolates did not be typed. Twenty-one spa types were identified, spa t437 (34/65, 52.3%) was the most predominant type. MRSA major clone type of molecular typing was CC59-ST59-spa t437-IV (28/65, 43.1%). Overall, 188D feature vectors can be applied to successfully distinguish MRSA from MSSA. In Quanzhou, the detection rate of PVL virulence factor was high, suggesting a high pathogenic risk of MRSA infection. The cross-infection of CA-MRSA and HA-MRSA was presented, the molecular characteristics were increasingly blurred, HA-MRSA with typical CA-MRSA molecular characteristics has become an important cause of healthcare-related infections. CC59-ST59-spa t437-IV was the main clone type in Quanzhou, which was rare in other parts of mainland China.
Introduction
Staphylococcus aureus has been considered the mainly pathogen that cause skin and soft-tissue infections, central nervous system infections, necrotizing pneumonia and infections associated with intravascular devices (Conceicao et al., 2007; Nadig et al., 2010; Rosa et al., 2016). Staphylococcus aureus is categorized into two groups, methicillin-sensitive Staphylococcus aureus (MSSA) and methicillin-resistant Staphylococcus aureus (MRSA) (Ahmed and Mukherjee, 2018). Which is based on the well-known differences of the mecA gene conferred to the pathogen, and the significant difference of the biofilm formation between MRSA and MSSA strains (Gidari et al., 2020). MRSA is responsible for most global Staphylococcus aureus bacteremia cases, and MRSA infection is related to poorer clinical outcomes than MSSA (Hassoun et al., 2017). Thus, MRSA is an important nosocomial pathogen that is being observed with increasing frequency in community settings. However, some studies have shown that tst-positive MSSA strains belonging to ST1, ST8, and ST30 are a potential source of tst-positive community-acquired MRSA and speculated that the tst-positive MRSA clones may have emerged from their respective MSSA counterparts. Therefore, MRSA and MSSA may owe the tst gene as an aid to targeted infection control (Schlebusch et al., 2009). Machine learning methods have a broad application in the bioinformatics, especially in the biological classification fields (Jiang et al., 2013; Liao et al., 2017, 2018a; Xu et al., 2018, 2019; Yu et al., 2018, 2020a,b; Ding et al., 2019; Liu G. et al., 2019; Liu B. et al., 2020; Shen et al., 2019a,b; Li et al., 2020; Shao et al., 2020; Wang H. et al., 2020; Zhao et al., 2020a,b). Here, Machine learning algorithm was performed to accomplish the classification of MRSA and MSSA based on their protein sequences (Liao et al., 2018b).
Since the first methicillin-resistant staphylococcus aureus (MRSA) reported in 1961 (Jevons, 1961), MRSA isolates were soon recovered from other European countries, and later from the United States, Japan, and Australia. At the same time, the resistance rate of MRSA was so high that it gave rise to significant morbidity and mortality. Currently, MRSA is also resistant to various non-β-lactam antibiotics, such as erythromycin, clindamycin, gentamicin, ciprofloxacin, and levofloxacin (Jiun-Ling et al., 2010). MRSA has caused an increasing public and occupational health concern.
In the early 1990s, community-associated MRSA (CA-MRSA) first broke out sporadically in several parts of Western Australia and the United States (Udo et al., 1993; Diekema et al., 2014). In 1999, The Centers for Disease Control and Prevention (CDC) reported that four children from Minnesotans and North Dakota died of sepsis in CA-MRSA infection, causing widespread concern (CDC, 1999). CA-MRSA is different from hospital-acquired MRSA (HA-MRSA) and has its own unique characteristics in virulence factors, genetic characteristics, epidemiology, and clinical manifestations. CA-MRSA infection most commonly affects skin and soft tissues, it is also associated with severe invasive diseases such as necrotizing pneumonia and sepsis, which often infect healthy young people such as students, athletes, and military personnel. The outbreak of CA-MRSA is associated with several common features, including close contact, poor sanitation, sharing among public goods or public facilities, skin surface abrasions, and lack of medical care to treat infections. Generally, CA-MRSA carries PVL virulence factors belonging to type IV SCCmec, and its SCCmec elements are relatively small, which is conducive to widespread transmission. Therefore, it is easy to form a wide range of epidemics. Usually without carrying other antibiotic resistance genes and therefore non β-lactam antibiotics are sensitive. While HA-MRSA usually contains large SCCmec such as type I, type II or type III, and contains a variety of anti-drug genes, the resistance of HA-MRSA isolates is not limited to β-lactam antibiotics. Pathogens are often resistant to multiple antibiotics.
The prevalence and resistance phenotypes of MRSA in different countries and regions are different and always changing over time. After reviewing the literature of nearly a decade, almost no paper reported on the molecular epidemiological investigation of MRSA in Quanzhou. This study analyzed the antimicrobial resistance of the Staphylococcus aureus isolated from several Three-A hospitals in Quanzhou, and tested the PVL virulence factor, spa typing, MLST typing and SCCmec typing of MRSA in this region, which provided a reference for clinical treatment of MRSA infection and response to explosive epidemics.
Materials and Methods
Data Retrieval and Treatment
All the primary sequences of both MRSA and MSSA Pfam proteins (in FASTA files) were retrieved from the UniProt database1, the raw data are preprocessed by cd-hit program2 to merge the sequence similarities and reduce the complexity. To avoid bias in the classifier, we set the identity at rigorous 30% similarity and remove the intersecting sequences, finally we obtained the results of 439 MRSA sequences as positive dataset and 62 MSSA entries as negative dataset. Since the MRSA sequences are seven times that of the MSSA sequences, the MRSA sequence is divided into seven, and the positive sequence and the negative sequence 1:1 constitute seven sets of data.
Construction of Feature Vectors for Positive and Negative Sequences
Feature selection (Wang G. et al., 2008; Zhao et al., 2015; Cheng and Hu, 2018; Du et al., 2018; Su et al., 2018; Tang et al., 2018; Wei et al., 2018a,b; Cheng et al., 2020) is the important process to select the extracted features that give the best classification results. To predict the potential MRSA from MSSA at the amino acid sequence level, firstly, we extracted the feature vectors from positive versus negative protein sequence dataset by using three novel machine-learning-based methods developed by our group, that are 188D, n-gram and 400D feature vectors (Wang G. et al., 2010; Liao et al., 2016; Liu and Jiang, 2016; Xinrui et al., 2018; Leyi et al., 2019; Liu B. et al., 2019; Yu et al., 2019; Zhang and Liu, 2019; Ao et al., 2020b).
Construction of Classifier With Weka and Classification Evaluation
Weka3 is a machine learning software for many applications that is widely used for teaching and research (Ye et al., 2019), and the Classify module contains several kinds of classifiers such as bayes, functions, lazy, meta, misc, rules, and trees in Weka Explorer. We use all the classifiers to train and select the best 6 performed ones to compare each other: AdaBoostM1, RandomSubSpace, DecisionTable, OneR, RandomForest, and REPTree. All the classifiers were set the parameters as default and test mode set as 10-fold cross validation. The identification process was showed in Figure 1.
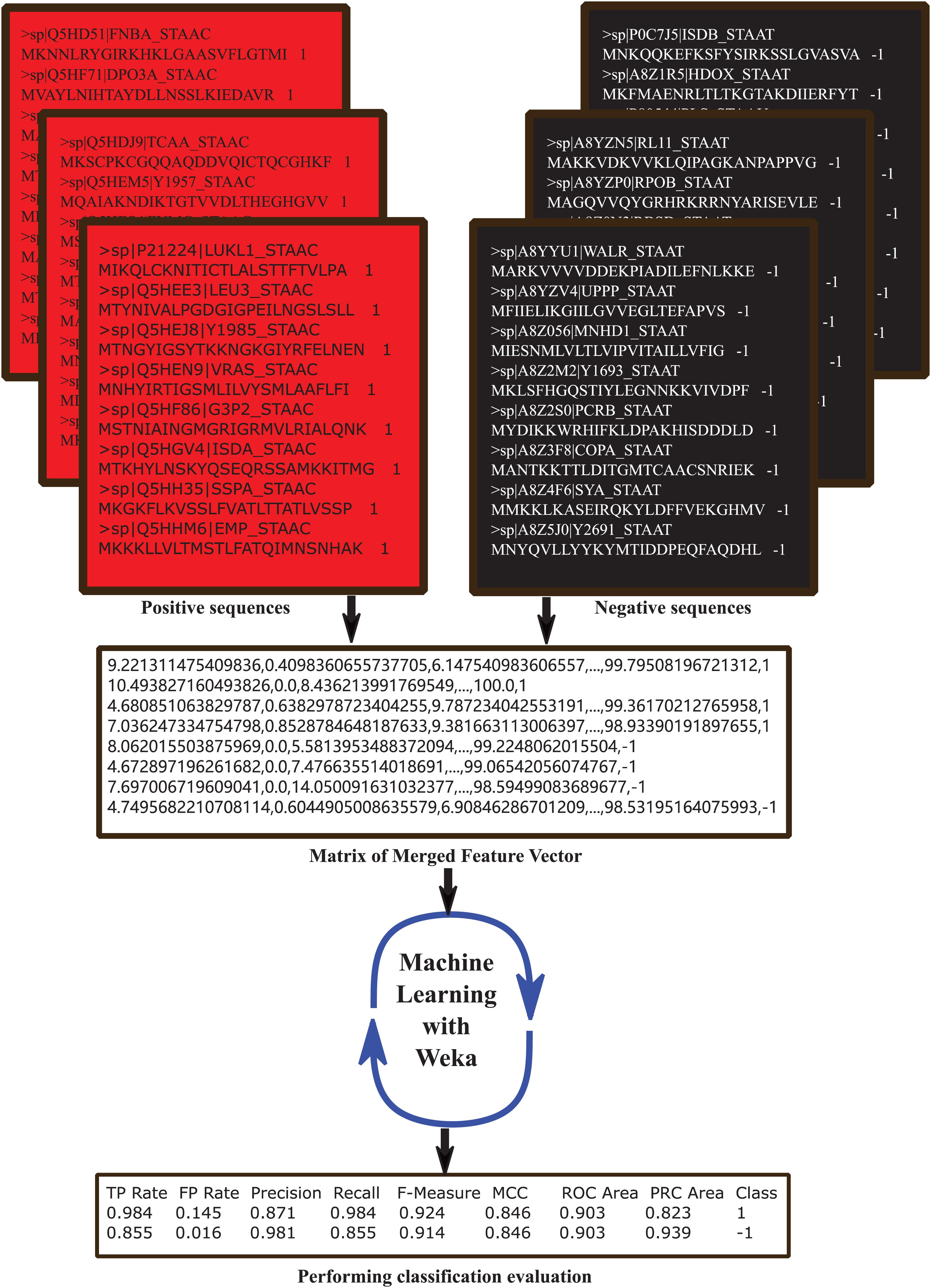
Figure 1. The computational framework of classification prediction for distinguishing MRSA from MSSA based on amino acid sequences. Firstly, both MRSA (positive) and MSSA (negative) protein sequences were retrieved from UniProt and pretreated by CD-HIT (30% similarity) and obtained dataset containing 439 MRSA and 62 MSSA entries. Secondly, MRSA were randomly divided into 7 groups with 62 entries in each group, each group MRSA and MSSA were extracted the feature vectors including 188D, n-gram and 400D methods. Thirdly, positive and negative feature vectors matrix were imported into Weka (10-fold cross-validation) and the six best performing classifiers were selected for further analysis. Finally, the 4 common measurements (Sn, Sp, Acc, and MCC) were used to evaluate classification performance.
We use four common measurements to illuminate the performance quality, that is Sensitivity (Sn), Specificity (Sp), Accuracy (Acc) and Matthew’s correlation coefficient (MCC)were adopted to evaluate the above three methods and four classifiers. These methods are formulated as follows (Wei et al., 2014, 2017a,b, 2018c; Zhao et al., 2017; Wang G. et al., 2018; Cheng, 2019; Cheng et al., 2019; Yang et al., 2019; Ao et al., 2020a; Hasan et al., 2020; Qiang et al., 2020; Tang et al., 2020):
Where TP, TN, FP, and FN stand for the numbers of true positive, true negative, false positive, and false negative, respectively.
Clinical Strains
A total of 81 non-repetitive Staphylococcus aureus strains were isolated from the outpatients and inpatients in three tertiary hospitals, which were Second Affiliated Hospital of Fujian Medical University, Quanzhou Children’s Hospital and Jinjiang Municipal Hospital, between October 2018 and July 2019. Staphylococcus aureus was identified by the Mérieux automated bacterial tester. According to the Clinical and Laboratory Standards Institute (CLSI) Antibiotic Sensitivity Test Execution Standard, Methicillin-Resistant Staphylococcus aureus (MRSA) was the MIC value of oxacillin ≥4 μg/ml and the plasma coagulase was also positive. At the same time, the mecA gene was detected by PCR to confirm. All the isolates were stored at −80°C for further experiments.
Ethics Statement
After inquiring the hospital, this study didn’t require any ethics statement because no work was developed with human samples. Strains were isolated directly from the patients to plates. Strains were collected not only for this study, but also for diagnosing of infection. Patient identifying information was collected by medical doctors as part of the routine hospital patient care procedure, and a number was assigned to each patient. Information arrived at the laboratory with this number after isolating and identifying all strains. Patient consents for collecting their clinical signs, medical histories, and characteristics were obtained during the admission of the hospital as a part of the routine hospital patient care procedure.
Antimicrobial Susceptibility Testing of the 81 Staphylococcus Aureus Strains
The antimicrobial susceptibility testing was conducted on all Staphylococcus aureus strains by the Mérieux Microbial Analysis Instrument according to the guidelines of CLSI M100-S29. The antibiotics tested were penicillin, linezolid, teicoplanin, vancomycin, ciprofloxacin, gentamicin, levofloxacin, clindamycin, Sulfamethoxazole/trimethoprim, erythromycin, rifampicin, and tetracycline. Staphylococcus aureus ATCC25923 and ATCC29213 were used for quality control.
PVL Gene and Molecular Typing of the 65 MRSA Isolates
Extraction of Genomic DNA
Sixty-five MRSA clinical isolates and standard strains were inoculated on blood agar culture plates overnight for 16–18 h, and DNA was extracted according to the bacterial genomic DNA rapid extraction kit. The obtained DNA was dissolved in 50 μl of TE Buffer and placed in an autoclaved eppendorf tube, and stored at −20°C.
Detection of PVL Gene
The PVL gene was amplified by PCR as described previously (Mcclure et al., 2006). The amplified product was performed to agarose gel electrophoresis. One amplified band appeared at 433 bp as the PVL gene, and 146 bp was the mecA gene. The identity of the PCR product was confirmed by sequencing. PVL quality control strain was CCUG46923.
Spa Typing
Spa typing was performed as described previously (Harmsen et al., 2003). Purified spa PCR products were sequenced, and short sequence repeats were assigned by using the spa database website4. The spa complex was defined by visual analysis, whereby spa types with similar short sequence repeats were clustered into the complexes previously described by Ruppitsch et al. (2006).
SCCmec Typing
The SCCmec types were determined by a multiplex PCR developed by Oliveira et al. (2006). Non-types (NT) were defined as isolates showing unexpected fragments or lacking some fragments as determined by multiplex PCR. The quality controls were MRSA NCTC10442 (SCCmec I), MRSA N315 (SCCmec II), MRSA 85/2082 (SCCmec III), MRSA JCSC 4744 (SCCmec IV).
MLST and Data Analysis
Multilocus sequence typing test was carried out as described previously (Enright et al., 2000). The sequences of the PCR products were compared with the existing sequences available on the MLST website5 for Staphylococcus aureus, and the allelic number was determined for each sequence. The clustering of related STs, which were defined as clonal complexes (CCs), was determined by using the program eBURST (based upon related sequence types) (Feil et al., 2004).
Results
Classification of Positive and Negative Proteins
We obtained the 188D, n-gram and 400D feature vector datasets from both positive and negative groups and used them as input to the Weka explorer. The results showed that the highest correctly classified rates were 91.94, 70.16, and 62.90%, respectively. The four common classification measurement values from 188D, n-gram and 400D feature vectors are illustrated in Figures 2–4.
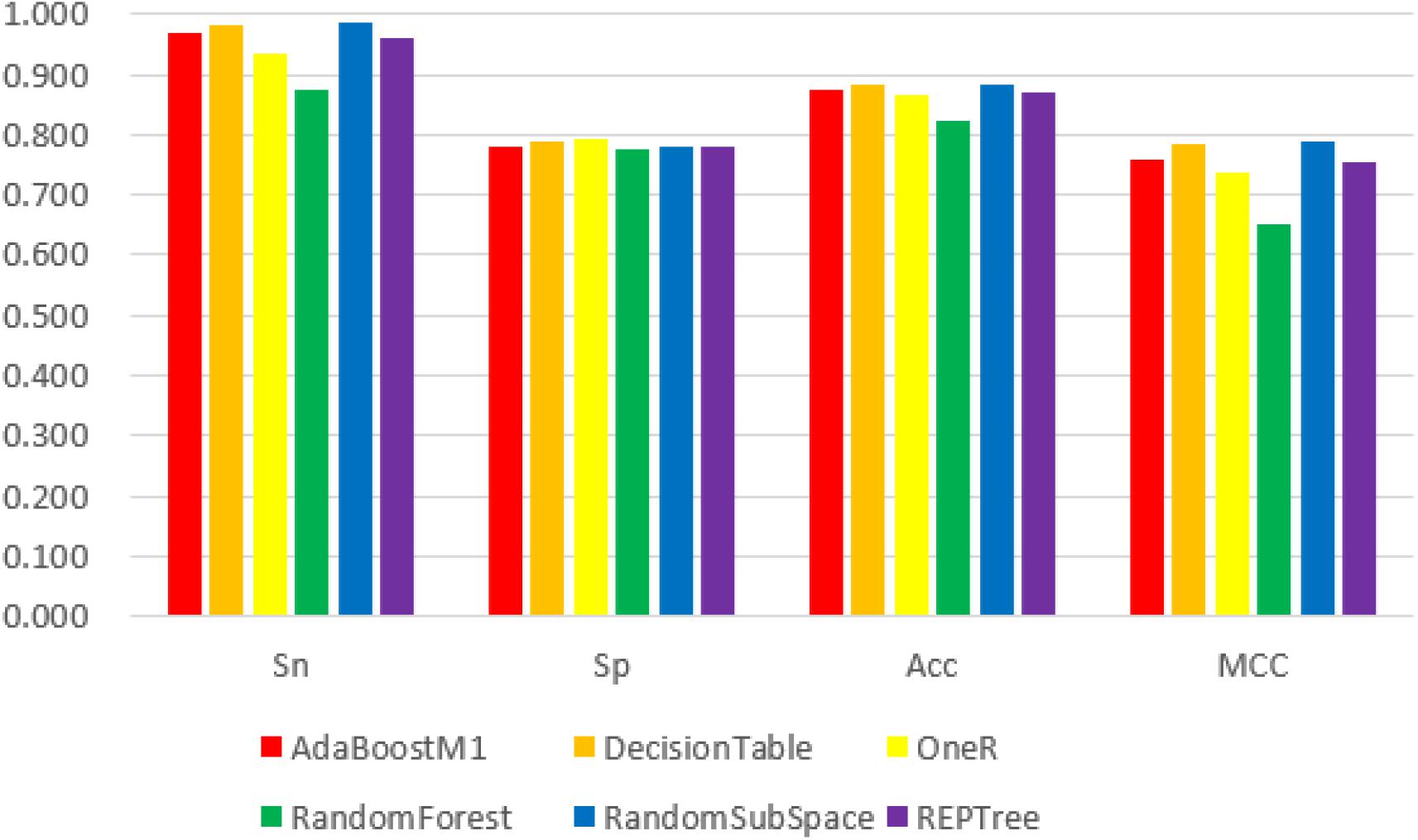
Figure 2. The 6 classifiers performance comparison using Sensitivity (Sn), Specificity (Sp), Accuracy (Acc) and Matthew’s Correlation Coefficient (MCC) values for 188D features. Among them, the RandomSubSpace classifier performs best in the four evaluation indexes with its values 98.8, 78.2, 88.5, and 78.8%, respectively.
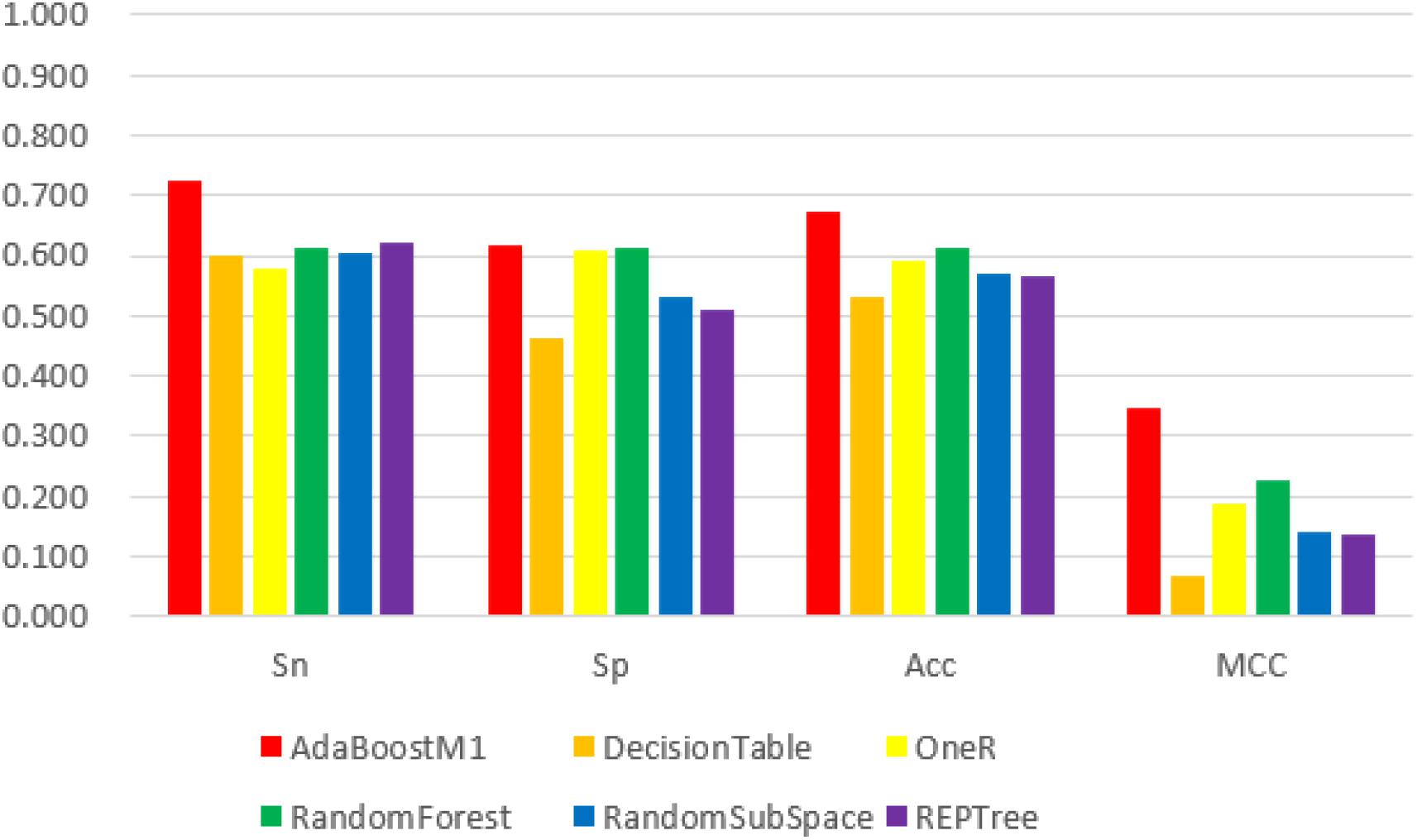
Figure 3. The 6 classifiers performance comparison using Sensitivity (Sn), Specificity (Sp), Accuracy (Acc) and Matthew’s Correlation Coefficient (MCC) values for n-gram features. Among them, the AdaBoostM1 classifier performs best in the four evaluation indexes with its values 72.6, 61.7, 67.1, and 34.5%, respectively. However, all evaluation index values of this feature are lower than 188D.
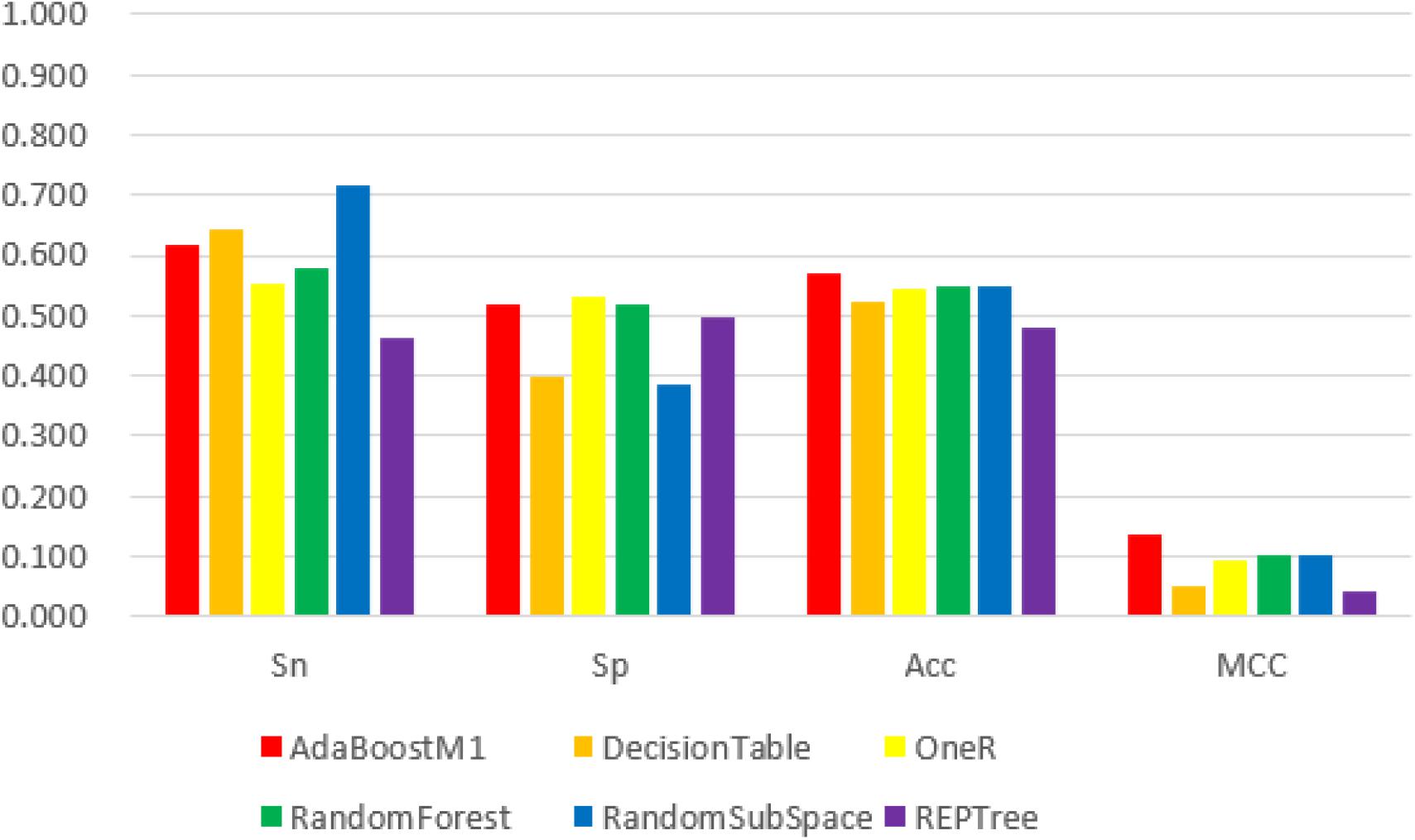
Figure 4. The 6 classifiers performance comparison using Sensitivity (Sn), Specificity (Sp), Accuracy (Acc) and Matthew’s Correlation Coefficient (MCC) values for 400D features. For the Sn index, RandomSupSpace classifier performs best with a value 71.8%, and OneR classifier performs best with a value 53.2% for Sp index, but for the Acc and MCC indexes the AdaBoostM1 classifier performs best with its value 56.9%, 13.8%, respectively. Among the above three features, 400D get the worst performance.
Identification of MRSA/MSSA and Antimicrobial Resistances
Of the 81 strains of Staphylococcus aureus, 65 were MRSA, 16 strains were MSSA strains. According to the definition of HA-MRSA and CA-MRSA, 65 strains of Staphylococcus aureus were divided into 22 HA-MRSAs and 43 CA-MRSAs.
Penicillin-resistant rate was 100%. No resistance to teicoplanin, linezolid, and vancomycin. The antimicrobial resistance rates to ciprofloxacin, sulfamethoxazole/trimethoprim, gentamicin, levofloxacin, clindamycin, erythromycin, rifampin, and tetracycline were 14.8, 11.9, 12.5, 13.2, 76.6, 77.7, 5.9, and 32.7%, respectively. Statistical analysis show that the MRSA isolates had significantly higher resistance rates to clindamycin than the MSSA isolates (87.7% vs. 31.8%, p < 0.001), erythromycin (86.2% vs. 43.1%, p < 0.001), tetracycline (36.9% vs. 15.6%, p = 0.03). The multi-resistance rate of MRSA was 93.8% (61/65) (Figure 5).
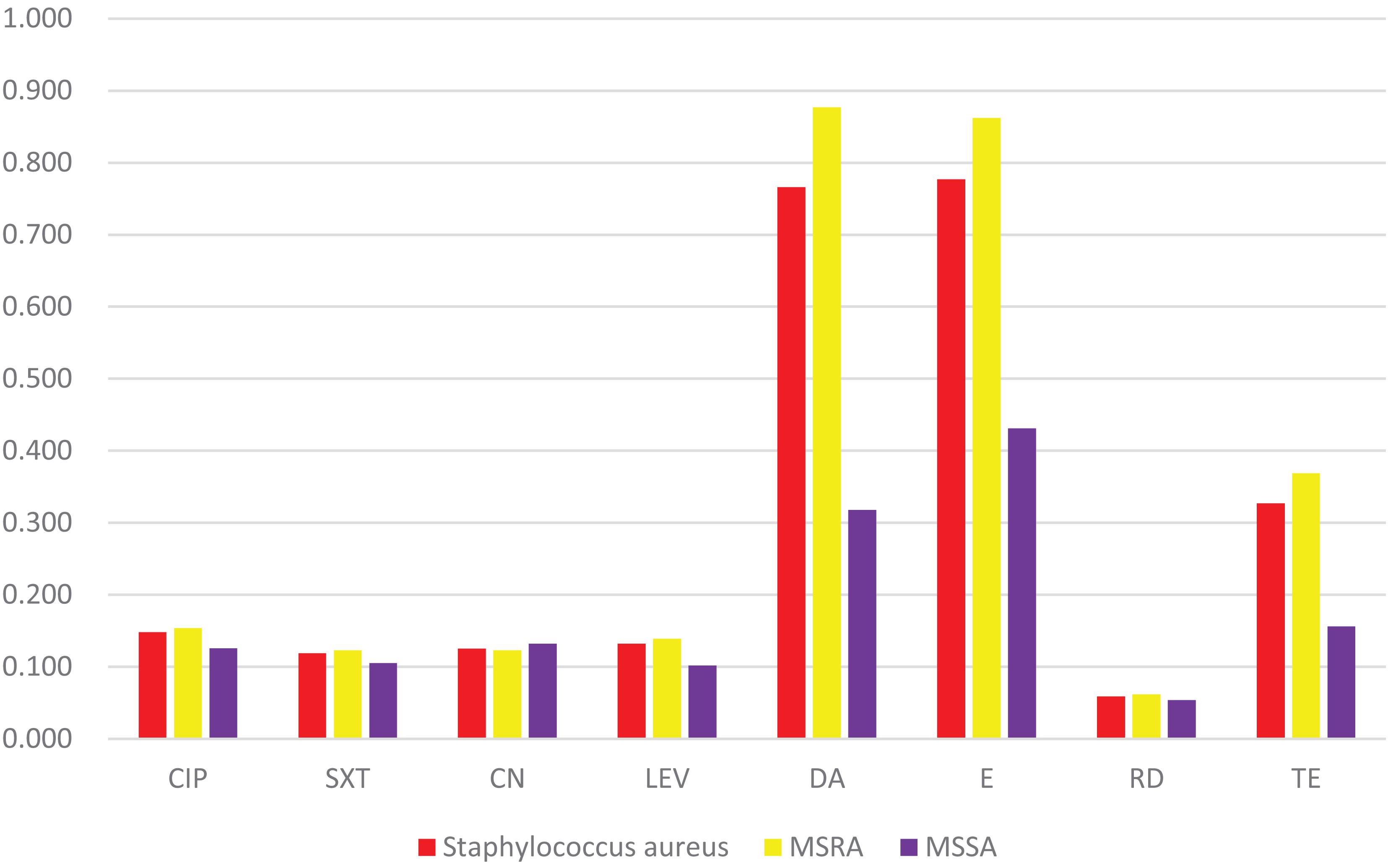
Figure 5. Resistance rates of eight antibiotics. It shows that the MRSA isolates possess significantly higher resistance rates to DA, E, and TE than the MSSA isolates. CIP (ciprofloxacin), SXT (Sulfamethoxazole/trimethoprim), CN (gentamicin), LEV (levofloxacin), DA (clindamycin), E (erythromycin), RD (rifampicin), TE (tetracycline).
PVL Gene Screening
Of the 65 MRSA isolates, thirty-one were PVL positive (31/65, 47.7%), among which nine were detected by HA-MRSA (9/22, 40.9%), and twenty-two were detected by CA-MRSA (22/43, 51.2%) (p > 0.5). The mecA gene was detected in all MRSA (Figure 6).
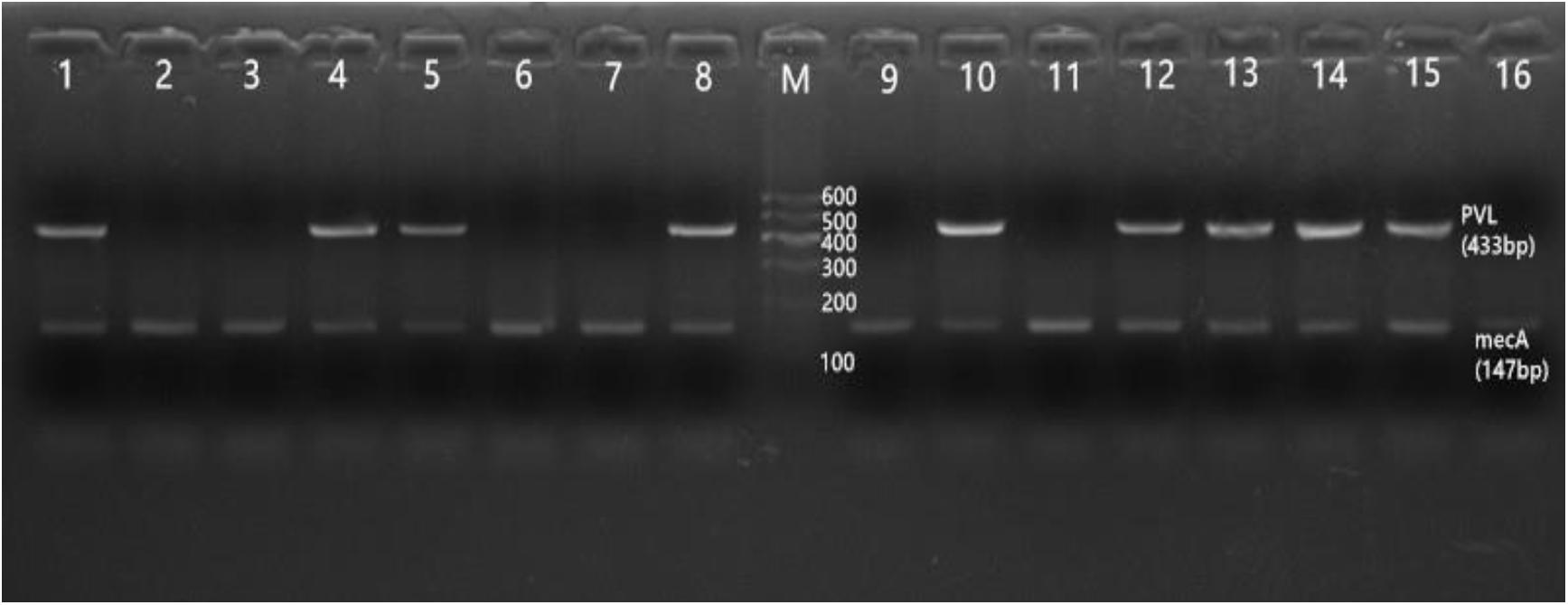
Figure 6. Results of agarose gel electrophoresis. This figure shows that all MRSA isolates can be detected with mecA gene, as well as the positive and negative test results of the PVL gene. M: 600 bp DNA Ladder; 1: positive PVL control; 2: PVL negative control. 4, 5, 8, 10, 12–15: PVL positive strains; 3, 6, 7, 9, 11, 16: PVL negative strains. 1–16: mecA gene.
Molecular Typing
Sixteen isolates belonged to SCCmec type III and forty-seven belonged to SCCmec type IV. Two MRSA isolates could not be SCCmec typed. Twenty-one spa types were identified. T437 (34/65, 52.3%) was the most common, followed by t030 (6/65, 9.2%), t062 (3/65, 4.6%), t309 (3/65, 4.6%), and t13774 (2/65, 3.1%).
Among all MRSA isolates, sixteen sequence types (STs) were identified by MLST. The most common ST was ST59 (36/65, 55.4%), followed by ST239 (6/65, 9.2%) and ST5 (4/65, 6.2%). Ten clonal complexes (CCs) were identified by eBURST. CC59 (37/65, 56.9%) was the most common clone, followed by CC8 (6/65, 9.2%) and CC5 (4/65, 6.1%) (Table 1). MRSA major clone types of molecular typing were CC59-ST59-spa t437-IV (28/65, 43.1%), followed by CC59-ST59-spa t437-III (6/65, 9.2%), CC8-ST239-spa t030-III/IV (6/65, 9.2%), and CC5-ST5-spa t062-IV (4/65, 6.1%), respectively (Table 1).
Comparison of Antimicrobial Resistance Rates Between CC59-ST59-spa t437-IV and Other Types of MRSA
Comparing the antibiotic resistance rate between CC59-ST59-spa t437-IV clone and other types, this study found that the resistance rate of CC59-ST59-spa t437-IV clone to CIP (ciprofloxacin), CN (gentamicin) and RD (rifampicin) was lower than other clone types (p < 0.05) (Table 2).
Discussion
Machine-learning techniques can be applied to extract features from bacterial protein sequences (Patel et al., 2017; Huang and Li, 2018; Shao and Liu, 2020; Zhao et al., 2020c). In this study, we successfully use them to distinguish MRSA from MSSA despite their similar sequences. It is reported that MRSA has reached over 60% of all isolated Staphylococcus aureus and the incidence of MRAS has increased to 49% in the United States (Jiang et al., 2020). So, it is very important to identify MRAS from MSSA rapidly. Because traditional assay methods are often time-consuming and with poor sensitivity, our classified recognition method shows its obvious advantages. Here, we have successfully established a machine learning method that based on our develop 188D feature vectors (Li Y. et al., 2019) being able to distinguish MRSA from MSSA. This method shows high specificity and sensitivity, the average discrimination ability reaches more than 90%. Thus, the 188D feature extraction method in this paper could be used as valuable tool for rapid, simple, sensitive and reliable identification of MRSA.
Panton-Valentine leukocidin (PVL) is an exotoxin produced by a variety of Staphylococcus aureus isolates that has a strong killing effect on white blood cells (Gauduchon et al., 2001). PVL–positive Staphylococcus aureus is highly toxic and is often associated with mild or moderate skin and soft tissue infections (SSTI) and can cause severe invasive infections, including necrotizing pneumonia or invasive bone joint infections (Vandenesch et al., 2012). The prevalence of PVL gene in different regions is diverse. In Europe, Glasner et al. (2015) tested 147 spa 437-MRSA strains in 11 European countries for PVL gene, and the positive rate was as high as 82%. In Asia, the prevalence of PVL in CA-MRSA and HA-MRSA was 14.3 and 5.7%, respectively (Song et al., 2012). In Taiwan, the data were also different. The percentage of PVL-positive isolates in the study of 253 MRSA strains from blood infection was 11.1% (Wang L. et al., 2010). The detection rate of PVL was 45.2% in Sun Yat-sen Memorial hospital of Guangzhou (Xie et al., 2016), and was 47.6% in Hainan’s hospitals in China (Li X. et al., 2019). Consistently in this study, the detection rate of PVL was as high as 47.7%. There was no significant difference between CA-MRSA (51.2%) and HA-MRSA (40.9%) groups. The positive rate of PVL in this area is relatively high, which suggests that the strong toxicity of MRSA in Quanzhou and the serious invasive infection may result from it. That should be paid more attention by clinicians.
Although PVL is usually considered to be a common pathogenic factor for CA-MRSA, some studies have shown that HA-MRSA isolates have a relatively high PVL positive rate in some areas (De-Zhi et al., 2011). Zetola et al. (2005) found that the prevalence of PVL tends to increase in nosocomial infections. These results suggest that these CA-MRSA may be cloned in hospital environment. Therefore, PVL may no longer be a reliable marker for CA-MRSA isolates, but all MRSA may be an important repository of PVL virulence factors. This suggests that HA-MRSA with typical CA-MRSA molecular characteristics (SCCmec IV and PVL positive) has become an important cause of health care related infections.
We found that CC59-ST59-spa t437-IV was the predominant clone in Quanzhou. This clone was also one of the most common CA-MRSA strains in East Asia (Song et al., 2012; Chuang and Huang, 2013; Liu G. et al., 2016). In 2007, Tristan and his colleagues reported for the first time the isolates of Staphylococcus aureus ST59 associated with spa-t437 (Anne et al., 2007). Subsequently, a large community and hospital study in Asia described CC59 as the most popular Complex clone (CC). In addition, ST59-MRSA-t437 was identified as the most prevalent clone between 2004 and 2006 (Song et al., 2012).
In China, ST59-MRSA-t437-IV is prevalent among children and adolescents (Li et al., 2013; Ning et al., 2015; Zhen et al., 2017). According to the report by Wang X. et al. (2016), the detection rate of this clone in Shanghai Children’s Medical Center between 2012 and 2013 was 21.3%, while the proportion of ST59-MRSA-t437-IV clones detected by Beijing Children’s Hospital in 2016 was as high as 61.7% (Yang et al., 2017). Interestingly, our study found that the proportion of adolescents and children carrying the clone was 36.4% (8/22) in Quanzhou, but the positive rate of the clone was 69.8% (30/43) in adults and significantly higher than that in minors (p < 0.05). This clone seems to be more popular in adults. The possible reason is that Quanzhou is located on the southeast coast of China, and the close interaction between local residents and Taiwan, Hong Kong, and Southeast Asian countries have enabled ST59-MRSA-t437 cloning to have a wide cross-infection among the populations in these areas. Song et al. (2012) also confirmed that community and hospital related MRSA CC59 strains collected from 8 countries and regions in Asia spread rapidly across national boundaries in both directions. In our study, the clone belonged to CA-MRSA accounted for 67.6% (23/34), HA-MRSA accounted for 32.4% (11/34), and both SCCmec type III and IV were present in both community and hospital MRSA infections. This indicated that CC59-MRSA-t437-IV can’t be used as a molecular marker for community infection. The difference between CA-MRSA and HA-MRSA has become blurred. More and more CA-MRSA-based clones have successfully invaded into hospital institutions, which has become an important pathogen of infection in hospitals. In many medical centers, they have become a common cause of medical-associated bacteremia (Uhlemann et al., 2014; Chen et al., 2015; Miura et al., 2018).
CC8-ST239-MRSA-III is the major HA-MRSA clone in China and some Asian countries, and the corresponding spa typing are mostly t030, t037, and t002 (Chen et al., 2014). The main HA-MRSA clones in Quanzhou were ST239-MRSA-III/IV-spa t030. ST239-MRSA-III-spa t037 was the most important MRSA clone in Beijing before 2000. Since 2000, ST239-MRSA-III-spa t030 has replaced t037 as the main clone (Chen et al., 2010). The most common clones in Shanghai were ST5-MRSA-II-spa t002, followed by ST239-MRSA-III-spa t037 and ST239-MRSA-III-spa t030 (Song et al., 2013). This inconsistent distribution means that the prevalence of MRSA isolates varies considerably even within the same country.
In 2014, China CHINET bacterial resistance surveillance data showed that the resistance rate of vancomycin, teicoplanin and linezolid was zero, ciprofloxacin, sulfamethoxazole/trimethoprim, gentamicin, levofloxacin, clindamycin Erythromycin, and rifampicin were 68.3, 7.0, 62.3, 71.7, 52.9, 77.1, and 47.2%, respectively (Hu et al., 2016). The resistance rate in Quanzhou is generally lower than the national average. CC59-spat437 is the main MRSA clone in the region, and its resistance rates of ciprofloxacin, levofloxacin, gentamicin and rifampicin are less than 6%, which can be used as the main drug for the treatment of this type of MRSA.
Data Availability Statement
The original contributions presented in the study are included in the article/supplementary material, further inquiries can be directed to the corresponding author.
Author Contributions
ZB, MC, and ZL conceived the study and designed the experiments. ZB, KW, DH, and YL collected the strains. ZB, HF, and YY performed the experiments. ZB, MC, QL, KW, JZ, DH, WM, and YL analyzed the data. ZB and ZL wrote the manuscript. All authors reviewed the manuscript.
Funding
This work was supported by the Natural Science Foundation of Fujian Province of China (Nos. 2020J01610 and 2018J01839).
Conflict of Interest
The authors declare that the research was conducted in the absence of any commercial or financial relationships that could be construed as a potential conflict of interest.
Footnotes
- ^ http://www.uniprot.org/
- ^ http://cd-hit.org
- ^ http://www.cs.waikato.ac.nz/ml/weka/
- ^ https://spa.ridom.de
- ^ http://saureus.mlst.net
References
Ahmed, M. I., and Mukherjee, S. (2018). Treatment for chronic methicillin-sensitive Staphylococcus aureus pulmonary infection in people with cystic fibrosis. Cochrane Database System. Rev. 7:CD011581. doi: 10.1002/14651858.CD011581
Anne, T., Michele, B., Helene, M., Gerard, L., and Bülent, B. (2007). Global distribution of Panton-Valentine leukocidin–positive methicillin-resistant Staphylococcus aureus, 2006. Emerg. Infect. Dis. 13, 594–600.
Ao, C., Jin, S., Ding, H., Zou, Q., and Yu, L. (2020a). Application and development of artificial intelligence and intelligent disease diagnosis. Curr. Pharmaceut. Des. 26, 3069–3075.
Ao, C., Zhou, W., Gao, L., Dong, B., and Yu, L. (2020b). Prediction of antioxidant proteins using hybrid feature representation method and random forest. Genomics 112, 4666–4674. doi: 10.1016/j.ygeno.2020.08.016
CDC. (1999). Four pediatric deaths from community-acquired methicillin-resistant Staphylococcus aureus-Minnesota and North Dakota, 1997-1999. JAMA 282, 1123–1125. doi: 10.1001/jama.282.12.1123
Chen, H., Liu, Y., Jiang, X., Chen, M., and Wang, H. (2010). Rapid change of methicillin-resistant Staphylococcus aureus clones in a Chinese tertiary care hospital over a 15-year period. Antimicrobial Agents Chemotherapy. 54:1842. doi: 10.1128/aac.01563-09
Chen, Y., Liu, Z., Duo, L., Xiong, J., Gong, Y., Yang, J., et al. (2014). Characterization of Staphylococcus aureus from distinct geographic locations in China: an increasing prevalence of spa-t030 and SCCmec Type III. PLoS One 9:e96255. doi: 10.1371/journal.pone.0096255
Chen, Y. J., Liu, K. L., Chen, C. J., and Huang, Y. C. (2015). Comparative molecular characteristics of community-associated and healthcare-associated methicillin-resistant Staphylococcus aureus isolates from adult patients in Northern Taiwan. Medicine (Baltimore) 94:e1961. doi: 10.1097/md.0000000000001961
Cheng, L. (2019). Computational and biological methods for gene therapy. Curr. Gene Ther. 19:210. doi: 10.2174/156652321904191022113307
Cheng, L., and Hu, Y. (2018). Human disease system biology. Curr. Gene Ther. 18, 255–256. doi: 10.2174/1566523218666181010101114
Cheng, L., Qi, C., Zhuang, H., Fu, T., and Zhang, X. (2020). gutMDisorder: a comprehensive database for dysbiosis of the gut microbiota in disorders and interventions. Nucleic Acids Res. 48, D554–D560.
Cheng, L., Zhao, H., Wang, P., Zhou, W., Luo, M., Li, T., et al. (2019). Computational methods for identifying similar diseases. Mol. Ther. Nucleic Acids 18, 590–604. doi: 10.1016/j.omtn.2019.09.019
Chuang, Y. Y., and Huang, Y. C. (2013). Molecular epidemiology of community-associated meticillin-resistant Staphylococcus aureus in Asia. Lancet Infect. Dis. 13, 698–708. doi: 10.1016/s1473-3099(13)70136-1
Conceicao, T., Aires-De-Sousa, M., Füzi, M., Tóth, Á, Pászti, J., Ungvári, E., et al. (2007). Replacement of methicillin-resistant Staphylococcus aureus clones in hungary over time: a 10-year surveillance study. Clin. Microbiol. Infect. 13, 971–979. doi: 10.1111/j.1469-0691.2007.01794.x
De-Zhi, L. I., Yu-Sheng, C., Jing-Ping, Y., Wei, Z., Cheng-Ping, H. U., Jia-Shu, L. I., et al. (2011). Preliminary molecular epidemiology of the Staphylococcus aureus in lower respiratory tract infections: a multicenter study in China. Chinese Med. J. 124, 687–692.
Diekema, D. J., Richter, S. S., Heilmann, K. P., Dohrn, C. L., Riahi, F., Tendolkar, S., et al. (2014). Continued emergence of USA300 methicillin- resistant Staphylococcus aureus in the United States: results from a nationwide surveillance study. Infect. Cont. Hospital Epidemiol. 35, 285–292. doi: 10.1086/675283
Ding, Y., Tang, J., and Guo, F. (2019). Identification of drug-side effect association via multiple information integration with centered kernel alignment. Neurocomputing 325, 211–224. doi: 10.1016/j.neucom.2018.10.028
Du, X., Li, X., Li, W., Yan, Y., and Zhang, Y. (2018). Identification and analysis of cancer diagnosis using probabilistic classification vector machines with feature selection. Curr. Bioinform. 13, 625–632. doi: 10.2174/1574893612666170405125637
Enright, M. C., Day, N. P., Davies, C. E., Peacock, S. J., and Spratt, B. G. (2000). Multilocus sequence typing for characterization of methicillin-resistant and methicillin-susceptible clones of Staphylococcus aureus. J. Clin. Microbiol. 38, 1008–1015. doi: 10.1128/jcm.38.3.1008-1015.2000
Feil, E. J., Li, B. C., Aanensen, D. M., Hanage, W. P., and Spratt, B. G. (2004). eBURST: inferring patterns of evolutionary descent among clusters of related bacterial genotypes from multilocus sequence typing data. J. Bacteriol. 186, 1518–1530. doi: 10.1128/jb.186.5.1518-1530.2004
Gauduchon, V., Werner, S., Prevost, G., Monteil, H., and Colin, D. A. (2001). Flow cytometric determination of panton-valentine leucocidin S component binding. Infect. Immun. 69, 2390–2395. doi: 10.1128/iai.69.4.2390-2395.2001
Gidari, A., Sabbatini, S., Schiaroli, E., Perito, S., and Monari, C. (2020). Tedizolid-Rifampicin combination prevents rifampicin-resistance on in vitro model of Staphylococcus aureus mature biofilm. Front. Microbiol. 11:2085. doi: 10.3389/fmicb.2020.02085
Glasner, C., Pluister, G., Westh, H., Arends, J. P., Empel, J., and Giles, E. (2015). Staphylococcus aureus spa type t437: identification of the most dominant community-associated clone from Asia across Europe. Clin. Microbiol. Infect. 21, e1–e8.
Harmsen, D., Claus, H., Witte, W., Rothgänger, J., Claus, H., Turnwald, D., et al. (2003). Typing of methicillin-resistant Staphylococcus aureus in a university hospital setting by using novel software for spa repeat determination and database management. J. Clin. Microbiol. 41, 5442–5448. doi: 10.1128/jcm.41.12.5442-5448.2003
Hasan, M. A. M., Ben Islam, M. K., Rahman, J., and Ahmad, S. (2020). Citrullination site prediction by incorporating sequence coupled effects into PseAAC and resolving data imbalance issue. Curr. Bioinform. 15, 235–245. doi: 10.2174/1574893614666191202152328
Hassoun, A., Linden, P. K., and Friedman, B. (2017). Incidence, prevalence, and management of MRSA bacteremia across patient populations—a review of recent developments in MRSA management and treatment. Crit. Care 21:211.
Hu, F. P., Guo, Y., and Zhu, D. M. (2016). Resistance trends among clinical isolates in China reported from CHINET surveillance of bacterial resistance, 2005-2014. Clin. Microbiol. Infect. 22, S9–S14.
Huang, G., and Li, J. (2018). Feature extractions for computationally predicting protein post-translational modifications. Curr. Bioinform. 13, 387–395. doi: 10.2174/1574893612666170707094916
Jevons, M. (1961). “Celbenin”-resistant Staphylococci. BMJ 1, 124–125. doi: 10.1136/bmj.1.5219.124-a
Jiang, L., Li, X., Gu, R., and Mu, D. (2020). Nanoparticles-Based biosensor coupled with multiplex loop-mediated isothermal amplification for detection of Staphylococcus aureus and identification of methicillin-resistant S. aureus. Infect. Drug Resist. 13, 1251–1262. doi: 10.2147/idr.s243881
Jiang, Q., Wang, G., Jin, S., Li, Y., and Wang, Y. (2013). Predicting human microRNA-disease associations based on support vector machine. Int. J. Data Min. Bioinform. 8, 282–293. doi: 10.1504/ijdmb.2013.056078
Jiun-Ling, W., Jann-Tay, W., Shey-Ying, C., and Yee-Chun, C. (2010). Distribution of staphylococcal cassette chromosome mec types and correlation with comorbidity and infection type in patients with MRSA Bacteremia. PLoS One 5:e9489. doi: 10.1371/journal.pone.0009489
Leyi, W., Ran, S., Shasha, L., Zhijun, L., Balachandran, M., Quan, Z., et al. (2019). Iterative feature representations improve N4-methylcytosine site prediction. Bioinformatics 35, 4930–4937. doi: 10.1093/bioinformatics/btz408
Li, J., Pu, Y., Tang, J., Zou, Q., and Guo, F. (2020). DeepAVP: a dual-channel deep neural network for identifying variable-length antiviral peptides. IEEE J. Biomed. Health Inform. 24, 3012–3019. doi: 10.1109/JBHI.2020.2977091
Li, S., Sun, J., Zhang, J., Li, X., Tao, X., Wang, L., et al. (2013). Comparative analysis of the virulence characteristics of epidemic methicillin-resistantStaphylococcus aureus(MRSA) strains isolated from Chinese children: ST59 MRSA highly expresses core gene-encoded toxin. APMIS 122, 101–114. doi: 10.1111/apm.12105
Li, X., Huang, T., and Xu, K. (2019). Molecular characteristics and virulence gene profiles of Staphylococcus aureus isolates in Hainan. China. BMC Infect. Dis. 19:873. doi: 10.1186/s12879-019-4547-5
Li, Y., Niu, M., and Zou, Q. (2019). ELM-MHC: an improved mhc identification method with extreme learning machine algorithm. J. Proteome Res. 18, 1392–1401. doi: 10.1021/acs.jproteome.9b00012
Liao, Z., Huang, Y., Yue, X., Lu, H., and Ju, Y. (2016). In silico prediction of gamma-aminobutyric acid Type-A receptors using novel machine-learning-based SVM and GBDT approaches. BioMed. Res. Int. 2016:2375268.
Liao, Z., Li, D., Wang, X., Li, L., and Zou, Q. (2018a). Cancer diagnosis through isomir expression with machine learning method. Curr. Bioinform. 13, 57–63. doi: 10.2174/1574893611666160609081155
Liao, Z., Wan, S., He, Y., and Zou, Q. (2018b). Classification of small GTPases with hybrid protein features and advanced machine learning techniques. Curr. Bioinform. 12, 492–500. doi: 10.2174/1574893612666171121162552
Liao, Z., Wang, X., Chen, X., and Zou, Q. (2017). Prediction and identification of krüppel-like transcription factors by machine learning method. Comb. Chem. High Throughput Screen. 20, 594–602.
Liu, B., Gao, X., and Zhang, H. (2019). BioSeq-Analysis2.0: an updated platform for analyzing DNA, RNA, and protein sequences at sequence level and residue level based on machine learning approaches. Nucleic Acids Res. 47:e127. doi: 10.1093/nar/gkz740
Liu, G., Hu, Y., Han, Z., Jin, S., and Jiang, Q. (2019). Genetic variant rs17185536 regulates SIM1 gene expression in human brain hypothalamus. Proc. Natl. Acad. Sci. U S A. 116, 3347–3348. doi: 10.1073/pnas.1821550116
Liu, B., Li, C., and Yan, K. (2020). DeepSVM-fold: protein fold recognition by combining support vector machines and pairwise sequence similarity scores generated by deep learning networks. Brief. Bioinform. 21, 1733–1741. doi: 10.1093/bib/bbz098
Liu, G., and Jiang, Q. (2016). Alzheimer’s disease CD33 rs3865444 variant does not contribute to cognitive performance. Proc. Natl. Acad. Sci. U S A. 113, E1589–E1590.
Liu, G., Xu, Y., Jiang, Y., Zhang, L., Feng, R., and Jiang, Q. (2016). PICALM rs3851179 variant confers susceptibility to Alzheimer’s disease in chinese population. Mol. Neurobiol. 54, 3131–3136. doi: 10.1007/s12035-016-9886-9882
Mcclure, J. A., Conly, J. M., Lau, V., Elsayed, S., Louie, T., Hutchins, W., et al. (2006). Novel multiplex PCR assay for detection of the staphylococcal virulence marker Panton-Valentine leukocidin genes and simultaneous discrimination of methicillin-susceptible from -resistant staphylococci. J. Clin. Microbiol. 44, 1141–1144. doi: 10.1128/jcm.44.3.1141-1144.2006
Miura, Y., Yamaguchi, T., Nakamura, I., Koyama, S., Tamai, K., Okanda, T., et al. (2018). Epidemiological trends observed from molecular characterization of methicillin-resistant Staphylococcus aureus isolates from blood cultures at a Japanese University Hospital, 2012-2015. Microbial Drug Resist. Mdr Mechan. Epidemiol. Dis. 24, 70–75. doi: 10.1089/mdr.2017.0008
Nadig, S., Ramachandra Raju, S., and Arakere, G. (2010). Epidemic meticillin-resistant Staphylococcus aureus (EMRSA-15) variants detected in healthy and diseased individuals in India. J. Med. Microbiol. 59, 815–821. doi: 10.1099/jmm.0.017632-17630
Ning, X., Sun, M., Qiao, Y., Dong, F., and Song, W. (2015). Characterization of pediatric hospital-associated infection caused by methicillin-resistant Staphylococcus aureus in mainland China. Infect. Dis. 47, 410–417.
Oliveira, D. C., Milheirico, C., and De Lencastre, H. (2006). redefining a structural variant of staphylococcal cassette chromosome mec, SCCmec type VI. Antimicrob. Agents Chemotherapy 50, 3457–3459. doi: 10.1128/aac.00629-06
Patel, S., Tripathi, R., Kumari, V., and Varadwaj, P. (2017). DeepInteract: deep neural network based protein-protein interaction prediction tool. Curr. Bioinform. 12, 551–557.
Qiang, X., Zhou, C., Ye, X., Du, P., Su, R., and Wei, L. (2020). CPPred-FL: a sequence-based predictor for large-scale identification of cell-penetrating peptides by feature representation learning. Brief. Bioinform. 21, 11–23. doi: 10.1093/bib/bby091
Rosa, F. G. D., Corcione, S., Motta, I., and Petrolo, A. (2016). Risk factors for mortality in patients with Staphylococcus aureus bloodstream infection. J. Chemotherapy 28, 187–190. doi: 10.1179/1973947815Y.0000000076
Ruppitsch, W., Indra, A., Stoger, A., Mayer, B., Stadlbauer, S., Wewalka, G., et al. (2006). Classifying spa types in complexes improves interpretation of typing results for methicillin-resistant Staphylococcus aureus. Clin. Microbiol. 44, 2442–2448. doi: 10.1128/jcm.00113-06
Schlebusch, S., Schooneveldt, J. M., Huygens, F., and Nimmo, G. R. (2009). Prevalence of Staphylococcus aureus strains in an Australian cohort, 1989-2003: evidence for the low prevalence of the toxic shock toxin and Panton-Valentine leukocidin genes. Eur. J. Clin. Microbiol. Infect. Dis. 28, 1183–1189. doi: 10.1007/s10096-009-0761-1
Shao, J., and Liu, B. (2020). ProtFold-DFG: protein fold recognition by combining directed fusion graph and pagerank algorithm. Brief. Bioinform. 7:bbaa192. doi: 10.1093/bib/bbaa192
Shao, J., Yan, K., and Liu, B. (2020). FoldRec-C2C: protein fold recognition by combining cluster-to-cluster model and protein similarity network. Brief. Bioinform. 20:bbaa144. doi: 10.1093/bib/bbaa144
Shen, Y., Ding, Y., Tang, J., Zou, Q., and Guo, F. (2019a). Critical evaluation of web-based prediction tools for human protein subcellular localization. Brief. Bioinform. 21, 1628–1640. doi: 10.1093/bib/bbz106
Shen, Y., Tang, J., and Guo, F. (2019b). Identification of protein subcellular localization via integrating evolutionary and physicochemical information into Chou’s general PseAAC. J. Theoret. Biol. 462, 230–239. doi: 10.1016/j.jtbi.2018.11.012
Song, J. H., Hsueh, P. R., Chung, D. R., Ko, K. S., and Kang, C. I. (2012). Spread of methicillin-resistant Staphylococcus aureus between the community and the hospitals in Asian countries: an ANSORP study. J. Antimicrob. Chemother. 66, 1061–1069.
Song, Y., Du, X., Li, T., Zhu, Y., and Li, M. (2013). Phenotypic and molecular characterization of Staphylococcus aureus recovered from different clinical specimens of inpatients at a teaching hospital in Shanghai between 2005 and 2010. J. Med. Microbiol. 62, 274–282. doi: 10.1099/jmm.0.050971-0
Su, R., Wu, H., Xu, B., Liu, X., and Wei, L. (2018). Developing a Multi-Dose Computational Model For Drug-Induced Hepatotoxicity Prediction Based On Toxicogenomics Data. IEEE/ACM Trans. Comput. Biol. Bioinform. 16, 1231–1239. doi: 10.1109/TCBB.2018.2858756
Tang, H., Zhao, Y. W., Zou, P., Zhang, C. M., Chen, R., Huang, P., et al. (2018). HBPred: a tool to identify growth hormone-binding proteins. Int. J. Biol. Sci. 14, 957–964. doi: 10.7150/ijbs.24174
Tang, Y.-J., Pang, Y.-H., and Liu, B. (2020). IDP-Seq2Seq: identification of intrinsically disordered regions based on sequence to sequence learning. Bioinformaitcs 23:btaa667. doi: 10.1093/bioinformatics/btaa667
Udo, E. E., Pearman, J. W., and Grubb, W. B. (1993). Genetic analysis of community isolates of methicillin-resistant Staphylococcus aureus in Western Australia. J. Hospital Infect. 25, 97–108. doi: 10.1016/0195-6701(93)90100-e
Uhlemann, A. C., Otto, M., Lowy, F. D., and Deleo, F. R. (2014). Evolution of community- and healthcare-associated methicillin-resistant Staphylococcus aureus. Infect. Genet. Evol. 21, 563–574. doi: 10.1016/j.meegid.2013.04.030
Vandenesch, F., Lina, G., and Henry, T. (2012). Staphylococcus aureus hemolysins, bi-component leukocidins, and cytolytic peptides: a redundant arsenal of membrane-damaging virulence factors? Front. Cell. Infect. Microbiol. 2:12. doi: 10.3389/fcimb.2012.00012
Wang, G., Luo, X., Wang, J., Wan, J., Xia, S., Zhu, H., et al. (2018). MeDReaders: a database for transcription factors that bind to methylated DNA. Nucleic Acids Res. 46, D146–D151.
Wang, G., Wang, Y., Feng, W., Wang, X., Yang, J. Y., Zhao, Y., et al. (2008). Transcription factor and microRNA regulation in androgen-dependent and -independent prostate cancer cells. BMC Genom. 9(Suppl. 2):S22. doi: 10.1186/1471-2164-9-S2-S22
Wang, G., Wang, Y., Teng, M., Zhang, D., Li, L., and Liu, Y. (2010). Signal transducers and activators of transcription-1 (STAT1) regulates microRNA transcription in interferon gamma-stimulated HeLa cells. PLoS One 5:e11794. doi: 10.1371/journal.pone.0011794
Wang, L., Chie, W. C., Weng, T. C., Fang, C. T., Chang, C. T., Wang, J., et al. (2010). Risk factors for mortality of nosocomial methicillin-resistant Staphylococcus aureus (MRSA) bloodstream infection: With investigation of the potential role of community-associated MRSA strains. J. Infect. 61, 449–457. doi: 10.1016/j.jinf.2010.09.029
Wang, H., Ding, Y., Tang, J., and Guo, F. (2020). Identification of membrane protein types via multivariate information fusion with Hilbert-Schmidt independence criterion. Neurocomputing 383, 257–269. doi: 10.1016/j.neucom.2019.11.103
Wang, X., Li, X., Liu, W., Huang, W., Fu, Q., and Li, M. (2016). Molecular characteristic and virulence gene profiles of community-associated methicillin-resistant Staphylococcus aureus isolates from pediatric patients in Shanghai. China. Front. Microbiol. 7:1818. doi: 10.3389/fmicb.2016.01818
Wei, L., Chen, H., and Su, R. (2018a). M6APred-EL: a sequence-based predictor for identifying n6-methyladenosine sites using ensemble learning. Mol. Therapy-Nucleic Acids 12, 635–644. doi: 10.1016/j.omtn.2018.07.004
Wei, L., Ding, Y., Su, R., Tang, J., and Zou, Q. (2018b). Prediction of human protein subcellular localization using deep learning. J. Parallel Distrib. Comp. 117, 212–217.
Wei, L., Su, R., Wang, B., Li, X., and Zou, Q. (2018c). Integration of deep feature representations and handcrafted features to improve the prediction of N 6-methyladenosine sites. Neurocomputing 324, 3–9. doi: 10.1016/j.neucom.2018.04.082
Wei, L., Liao, M., Gao, Y., Ji, R., He, Z., and Zou, Q. (2014). Improved and promising identification of human MicroRNAs by incorporating a high-quality negative set. IEEE/ACM Trans. Comput. Biol. Bioinform. 11, 192–201. doi: 10.1109/tcbb.2013.146
Wei, L., Wan, S., Guo, J., and Wong, K. K. (2017a). A novel hierarchical selective ensemble classifier with bioinformatics application. Art. Intell. Med. 83, 82–90. doi: 10.1016/j.artmed.2017.02.005
Wei, L., Xing, P., Zeng, J., Chen, J., Su, R., and Guo, F. (2017b). Improved prediction of protein–protein interactions using novel negative samples, features, and an ensemble classifier. Art. Intell. Med. 83, 67–74. doi: 10.1016/j.artmed.2017.03.001
Xie, X., Bao, Y., Ouyang, N., Dai, X., Pan, K., Chen, B., et al. (2016). Molecular epidemiology and characteristic of virulence gene of community-acquired and hospital-acquired methicillin-resistant Staphylococcus aureus isolates in Sun Yat-sen Memorial hospital. Guangzhou, Southern China. BMC Infect. Dis. 16:339. doi: 10.1186/s12879-016-1684-y
Xinrui, W., Zhijun, L., Zhimin, B., Yan, H., Juan, D., and Leyi, W. (2018). MiR-93-5p promotes cell proliferation through down-regulating PPARGC1A in hepatocellular carcinoma cells by bioinformatics analysis and experimental verification. Genes 9:51. doi: 10.3390/genes9010051
Xu, L., Liang, G., Liao, C., Chen, G.-D., and Chang, C.-C. (2018). An efficient classifier for alzheimer’s disease genes identification. Molecules 23:3140. doi: 10.3390/molecules23123140
Xu, L., Liang, G., Liao, C., Chen, G.-D., and Chang, C.-C. (2019). k-Skip-n-Gram-RF: a random forest based method for Alzheimer’s disease protein identification. Front. Genet. 10:33. doi: 10.3389/fgene.2019.00033
Yang, H., Yang, W., Dao, F. Y., Lv, H., Ding, H., Chen, W., et al. (2019). A comparison and assessment of computational method for identifying recombination hotspots in Saccharomyces cerevisiae. Brief. Bioinform. 21, 1568–1580. doi: 10.1093/bib/bbz123
Yang, X., Qian, S., Yao, K., Wang, L., Liu, Y., Dong, F., et al. (2017). Multiresistant ST59-SCCmec IV-t437 clone with strong biofilm-forming capacity was identified predominantly in MRSA isolated from Chinese children. BMC Infect. Dis. 17:733. doi: 10.1186/s12879-017-2833-7
Ye, M., Wang, W., Yao, C., Fan, R., and Wang, P. (2019). Gene selection method for microarray data classification using particle swarm optimization and neighborhood rough set. Curr. Bioinform. 14, 422–431. doi: 10.2174/1574893614666190204150918
Yu, L., Shi, Y., Zou, Q., Wang, S., Zheng, L., and Gao, L. (2020a). Exploring drug treatment patterns based on the action of drug and multilayer network model. Int. J. Mol. Sci. 21:5014. doi: 10.3390/ijms21145014
Yu, L., Xu, F., and Gao, L. (2020b). Predict new therapeutic drugs for hepatocellular carcinoma based on gene mutation and expression. Front. Bioeng. Biotechnol. 8:8. doi: 10.3389/fbioe.2020.00008
Yu, L., Sun, X., Tian, S., Shi, X., and Yan, Y. (2018). Drug and nondrug classification based on deep learning with various feature selection strategies. Curr. Bioinform. 13, 253–259. doi: 10.2174/1574893612666170125124538
Yu, L., Yao, S. Y., Gao, L., and Zha, Y. H. (2019). Conserved disease modules extracted from multilayer heterogeneous disease and gene networks for understanding disease mechanisms and predicting disease treatments. Front. Genet. 9:745. doi: 10.3389/fgene.2018.00745
Zetola, N., Francis, J. S., Nuermberger, E. L., and Bishai, W. R. (2005). Community-acquired meticillin-resistant Staphylococcus aureus: an emerging threat. Lancet Infect. Dis. 5, 275–286. doi: 10.1016/s1473-3099(05)70112-2
Zhang, J., and Liu, B. (2019). A review on the recent developments of sequence-based protein feature extraction methods. Curr. Bioinform. 14, 190–199. doi: 10.2174/1574893614666181212102749
Zhao, T., Hu, Y., and Cheng, L. (2020a). Deep-DRM: a computational method for identifying disease-related metabolites based on graph deep learning approaches. Brief. Bioinform. 13:bbaa212. doi: 10.1093/bib/bbaa212
Zhao, T., Hu, Y., Peng, J., and Cheng, L. (2020b). DeepLGP: a novel deep learning method for prioritizing lncRNA target genes. Bioinformatics 36, 4466–4472. doi: 10.1093/bioinformatics/btaa428
Zhao, X., Jiao, Q., Li, H., Wu, Y., Wang, H., Huang, S., et al. (2020c). ECFS-DEA: an ensemble classifier-based feature selection for differential expression analysis on expression profiles. BMC Bioinform. 21:43. doi: 10.1186/s12859-020-3388-y
Zhao, Y., Wang, F., Chen, S., Wan, J., and Wang, G. (2017). Methods of MicroRNA promoter prediction and transcription factor mediated regulatory network. Biomed. Res. Int. 2017:7049406.
Zhao, Y., Wang, F., and Juan, L. (2015). MicroRNA promoter identification in Arabidopsis using multiple histone markers. Biomed. Res. Int. 2015:861402.
Keywords: Staphylococcus aureus, feature vector, virulence factor, molecular characterization, antibiotic resistance, machine learning
Citation: Bai Z, Chen M, Lin Q, Ye Y, Fan H, Wen K, Zeng J, Huang D, Mo W, Lei Y and Liao Z (2021) Identification of Methicillin-Resistant Staphylococcus Aureus From Methicillin-Sensitive Staphylococcus Aureus and Molecular Characterization in Quanzhou, China. Front. Cell Dev. Biol. 9:629681. doi: 10.3389/fcell.2021.629681
Received: 15 November 2020; Accepted: 04 January 2021;
Published: 21 January 2021.
Edited by:
Liang Cheng, Harbin Medical University, ChinaCopyright © 2021 Bai, Chen, Lin, Ye, Fan, Wen, Zeng, Huang, Mo, Lei and Liao. This is an open-access article distributed under the terms of the Creative Commons Attribution License (CC BY). The use, distribution or reproduction in other forums is permitted, provided the original author(s) and the copyright owner(s) are credited and that the original publication in this journal is cited, in accordance with accepted academic practice. No use, distribution or reproduction is permitted which does not comply with these terms.
*Correspondence: Zhijun Liao, bGlhb3pqMTAwQDE2My5jb20=
†These authors have contributed equally to this work