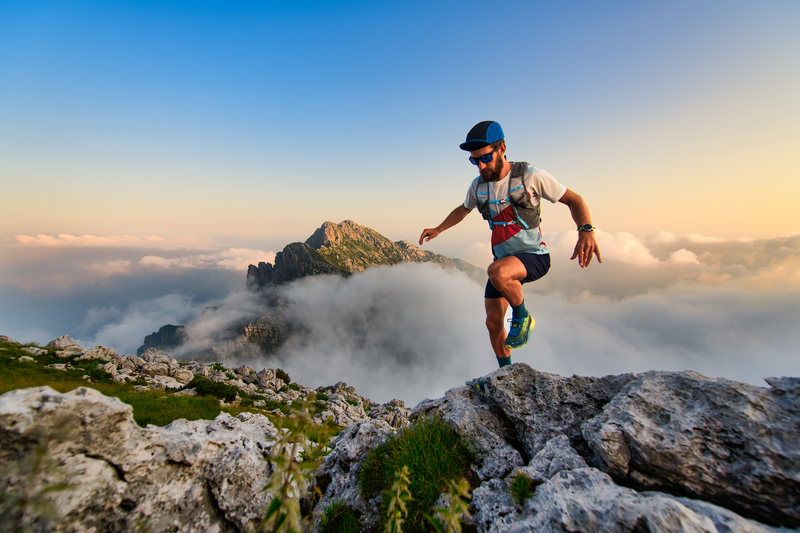
94% of researchers rate our articles as excellent or good
Learn more about the work of our research integrity team to safeguard the quality of each article we publish.
Find out more
ORIGINAL RESEARCH article
Front. Cell Dev. Biol. , 18 August 2021
Sec. Molecular and Cellular Oncology
Volume 9 - 2021 | https://doi.org/10.3389/fcell.2021.607782
This article is part of the Research Topic Advances in the Molecular Mechanisms in Gastrointestinal Tumorigenesis and Treatment View all 39 articles
Background: To identify the hub genes associated with chemoradiotherapy resistance in rectal cancer and explore the potential mechanism.
Methods: Weighted gene co-expression network analysis (WGCNA) was performed to identify the gene modules correlated with the chemoradiotherapy resistance of rectal cancer.
Results: The mRNA expression of 31 rectal cancer patients receiving preoperative chemoradiotherapy was described in our previous study. Through WGCNA, we demonstrated that the chemoradiotherapy resistance modules were enriched for translation, DNA replication, and the androgen receptor signaling pathway. Additionally, we identified and validated UTP6 as a new effective predictor for chemoradiotherapy sensitivity and a prognostic factor for the survival of colorectal cancer patients using our data and the GSE35452 dataset. Low UTP6 expression was correlated with significantly worse disease-free survival (DFS), overall survival (OS), and event- and relapse-free survival both in our data and the R2 Platform. Moreover, we verified the UTP6 expression in 125 locally advanced rectal cancer (LARC) patients samples by immunohistochemical analysis. The results demonstrated that low UTP6 expression was associated with worse DFS and OS by Kaplan-Meier and COX regression model analyses. Gene set enrichment and co-expression analyses showed that the mechanism of the UTP6-mediated chemoradiotherapy resistance may involve the regulation of FOXK2 expression by transcription factor pathways.
Conclusion: Low expression of the UTP6 was found to be associated with chemoradiotherapy resistance and the prognosis of colorectal cancer possibly via regulating FOXK2 expression by transcription factor pathways.
Preoperative chemoradiotherapy (CRT) and radical surgery have become the standard of care for stage II/III rectal cancer patients (Heald et al., 1982). The benefits of this multimodality therapy have been well documented, including tumor downsizing and downstaging, increased radical resection, and reduced local recurrence (Sauer et al., 2004, 2012; van Gijn et al., 2011). However, the treatment may increase the perioperative mortality rates by 48% (Rahbari et al., 2013). Moreover, 15% to 45% of the rectal cancers would are estimated to develop resistance to CRT, and might be exposed to CRT-related toxicitiestoxicity without oncological benefit (Ha et al., 2017). Therefore, the identification of valid biomarkers for colorectal cancer (CRC) with resistance to CRT has become imperative.
Currently, high-throughput sequencing is commonly used to screen and identify differentially expressed genes (DEGs). Conventional molecular biology methodology evaluates the variations in and functions of genes independently. Weighted gene co-expression network analysis (WGCNA) is an unbiased systematic biological approach, which clarifies the transcriptome function at a system-level, determines gene-gene correlations, and identifies gene modules with a high correlation across microarray data. It also bridges the gap between individual genes and tumorigenesis and progression (Zhang and Horvath, 2005; Langfelder and Horvath, 2008; Tian et al., 2017). WGCNA can facilitate the network-based gene screening approaches that screen key biomarkers associated with clinical traits in various cancers. However, this efficient bioinformatics approach has not yet been adopted to explore network-centric genes associated with CRT resistance in rectal cancer patients.
In this context, this study aimed to screen the relevant hub genes for CRT in our expression profile using WGCNA. Then, the hub genes were verified using testing data sets and patient tissue samples.
In total, 31 locally advanced rectal cancer (LARC) patients receiving preoperative CRT between March 2016 to December 2016 in Fujian Medical University Union Hospital, China were enrolled as the internal dataset in this study, which was used for the gene-chip analysis. The CRT, treatment, and follow-up protocol were described in a previous study (Zhang et al., 2017). All patients received preoperative CRT and underwent R0 resection of primary colorectal tumors after neoadjuvant CRT. And the samples were collected at diagnosis and without any treatment before biopsy from colonoscopy. The chemoradiotherapy protocol was as follows: preoperative radiotherapy consisted of 45 Gy to the pelvis for 5 weeks (180 cGy/25 fractions) and a tumor boost of 5.4 Gy. Concomitant chemotherapy was administered with oral capecitabine (825 mg/m2 twice daily from day 1 to day 14 per cycle).
Moreover, to further validate the UTP6 expression in the LARC patients’ colonoscopic samples before CRT. Consecutive LARC patients who underwent CRT and radical resection between 2011 and 2014 were identified. A total of 125 patients were enrolled in the present study. This study was approved by the Institutional Review Board of Fujian Medical University Union Hospital (2013051).
Total RNA was extracted from the tissue mentioned previously using Trizol reagent (Invitrogen), according to the manufacturer’s protocol. RNA quantity and quality were measured by a NanoDrop ND-1000 and RNA integrity was assessed by standard denatured agarose gel electrophoresis. Sample labeling and array hybridization were performed according to the human GeneChip Analysis protocol (Affymetrix, Santa Clara, CA). The scanned data obtained from each microarray were normalized to correct for small differences in the amounts of each of the cRNA probes applied to the microarray and were processed for signal values using Affymetrix software (LIMS 5.0). The differentially expressed mRNAs were identified through fold-change filtering. Hierarchical clustering was performed using Agilent Gene Spring GX software (version 11.5.1). The upregulated and downregulated mRNA groups were defined as the mRNA expression in the CRT-responsive group compared with the CRT-non-responsive group. The Gene Ontology (GO) functional analysis and Kyoto Encyclopedia of Genes and Genomes (KEGG) pathway analysis were performed using standard enrichment computation method.
Datasets were downloaded from the Gene Expression Omnibus (GEO) database1 on December 19, 2019. The GSE35452 dataset included mRNA expression gene microarray of 46 rectal cancer patients receiving preoperative CRT and biopsy specimens were collected before preoperative CRT, which was used as the external validation dataset. Cancer stem cells are regarded as having self-renewal and differentiation properties and are one of the integral factors mediating the response to CRT (Baumann et al., 2008; Wahab et al., 2017). GSE14773 and GSE24747 were used to determine the hub gene expression in CRC stem cells. The GSE14773 dataset included one cell line with markers of CRC stem cells, high CD44, and CD166 expression, and the other one was a parental control CRC cell. The GSE24747 dataset included two groups, CD133 + and CD133-, CACO-2 cells. DNA methylation is considered an important component in gene expression regulation, and high levels of DNA promoter region methylation result in transcriptional silencing. Additionally, promoter regions methylation is reported to be associated with the CRT-resistance and considered as a predictor for CRT (Wilting and Dannenberg, 2012; Ivanova et al., 2013). The GSE104271 dataset was utilized to explore whether the hub genes were regulated by DNA methylation. As described by Huang et al. (2018) previously, FIBP knockdown cell lines were defined as cancer cells compared to cancer stem cells. The methylation data of the CRT-resistant genes CRC and normal colon tissues were obtained from The Cancer Genome Atlas (TCGA) in the UALCAN database2 (Chandrashekar et al., 2017). A flow diagram of the present study is shown in Figure 1.
Tumor response to CRT was graded according to the American Joint Committee on Cancer pathological tumor regression grade (AJCC TRG) (Amin et al., 2017; Benson et al., 2018); that is, TRG 0, no residual tumor cells; TRG 1, single cells or small groups of cells; TRG 2, residual cancer with the desmoplastic response; and TRG 3, minimal evidence of tumor response. Pathological complete response (pCR) was defined as the absence of viable tumor cells in the resected specimen, either at the primary site or in the lymph nodes. Venous blood samples were obtained within 1 week before CRT.
The WGCNA algorithm was previously described in detail (Zhang and Horvath, 2005). Briefly, first, we identified the qualification of the profile data. The co-expression network was constructed using the “WGCNA” package in R software (Horvath and Dong, 2008; Mason et al., 2009). Next, the correlation matrix was established and the soft threshold power was determined by analysis of the network topology. Then, the topological overlap matrix (TOM) was established (Yip and Horvath, 2007; Ivanova et al., 2013; Botía et al., 2017). Based on the phenotypic data of the groups, we calculated each module p-value by the t-test gene significance.
To explore the relevant modules, we examined the association between module eigengenes (MEs) and CRT-resistance using Pearson’s correlation analysis. To identify the hub genes, the CRT-resistance model with the highest correlation coefficient (P < 0.05) in the data set was chosen, and the module also had the highest specific weight of all of the modules. The hub genes in the module were defined by module connectivity as measured by thean absolute value of the >0.3 in Pearson’s correlation >0.3 analysis. The protein–protein interaction (PPI) network was constructed by all genes in the CRT-resistance module, nd PPI network analysis was performed to screen the hub genes by Cytoscape. And the top 10 values were included for further analysis.
Statistical analysis was performed using SPSS software (version. 23 SPSS Inc., Chicago, IL, United States) and R software (version. 3.4.1). The continuous variables were reported as means and standard deviation from the analysis of variance test. The survival outcomes were assessed using Kaplan-Meier and log-rank analyses. Receiver operating characteristic (ROC) curve analysis was performed. Finally, the prognostic significance of the hub genes in CRC patients was analyzed using the R2: Genomics Analysis and Visualization Platform,3 on January, 03, 2020. The optimal cutoff points for the expression of UTP6 and FTSJ3 were calculated and determined using Cut-off Finder4 Visit and download data, 2019.12.26). Budczies et al. (2012), a new bio-informatics tool for biomarker assessment and outcome-based cut-point optimization, which identified the cut-off with the minimum p-values from log-rank χ2 statistics in terms of disease-free survival (DFS). A P-value of <0.05 was considered statistically significant.
To explore the potential function of UTP6 in LARC patients, gene set enrichment analysis (GSEA) was performed in the patients in our previous datasets. A P-value of <0.05 and an enrichment score (ES) of >0.3 were set as the cutoff criteria. To further explore the correlation between UTP6 and relevant genes in the GSEA results, we analyzed the co-expressed genes in the TCGA data in the UALCAN dataset (Visit and download data, 2020.02.01).
The protein expression of UTP6 in specimens obtained before and after CRT in 125 LARC patients was assessed using the immunohistochemical streptavidin-biotin complex method (Zhang et al., 2018). Phosphate-buffered saline (PBS) was used as the negative control and the image of the positive control from GE Healthcare Life Sciences. Immunoreactivity was scored by semi-quantitative analysis, and the fields were randomly selected in five directions (up, center, down, left, and right) under high magnification (∗400). The color was determined based on the intensity score as follows: 0 (no staining), 1 (light yellow), 2 (brown), and 3 (deep brown). The percentage of positive cells was scored as 0 (<5%), 1 (5–25%), 2 (25–50%), 3 (50–75%), and 4 (>75%). The mean value was calculated for each case with the aforementioned scoring methods and the final score was obtained by multiplying these two scores. The score between 0 and 4 was defined as the low expression and >4 was defined as high expression. All analyses were performed in a double-blind manner.
A gene chip array was used to examine the gene expression profiles in primary tumor cells. A supervised hierarchical cluster analysis of the gene expression profiling data showed that the two groups had a clustering trend (Figures 2A,B). A total of 18419 genes were detected in the gene chip array. The SAM for the DEGs revealed that the two groups significantly differed in genes related to tumor cell biology, including 798 upregulated genes and 450 downregulated genes.
Figure 2. mRNAs expression profile comparison between chemotherapy-resistance and chemotherapy-sensitivity groups. Gene Ontology (GO) functional and Kyoto Encyclopedia of Genes and Genomes (KEGG) pathway analysis of the differentially expressed genes. (A) The hierarchical clustering of all targets values of mRNA expression profiling among samples. (B) Between the chemotherapy-resistance and chemotherapy-sensitivity group. The purple dots indicated the up-regulated genes of mRNAs and the green dots indicated the down-regulated genes of mRNAs. (C) GO functional analysis of the top ten functional classifications of the upregulated genes. (D) GO functional analysis of the top ten functional classifications of the downregulated genes. (E) KEGG pathway analysis of the top ten significant pathways of upregulated genes. (F) KEGG pathway analysis of the top ten pathways of downregulated genes.
GO enrichment analysis was performed to investigate the molecular mechanism of the differently expressed genes involved in the resistance to CRT in LARC patients. We detected the top significant GO-enriched terms and KEGG terms in both the significantly upregulated and downregulated genes in rectal cancer patients (Figures 2C–F). The results showed that the top three significant GO terms for the upregulated genes were related to pancreatic A cell differentiation, muscle contraction, and type B pancreatic cell differentiation involved in the immune response. Among the downregulated genes, the top three significant GO terms were related to the ER-associated ubiquitin-dependent protein catabolic process, mRNA processing, and RNA splicing. Moreover, the top three significant KEGG terms in the upregulated genes were related to neuroactive ligand-receptor interaction, lysine degradation, and olfactory transduction involved in the immune response. In the downregulated genes, the top three significant GO terms were related to protein processing in the endoplasmic reticulum, amino sugar and nucleotide sugar metabolism, and the proteasome.
To identify the hub genes, a weighted co-expression network was utilized to analyze our data (Figure 3A). A total of 31 modules were identified, as shown in Figure 3C. We further analyzed the relationship between CRT-resistance and the modules (Figure 3C). Among these modules, the module shown in dark turquoise (Figure 3C) had the strongest negative association with CRT-resistance (r = −0.45, P = 0.01), while the light cyan module showed the highest positive association with CRT-resistance (r = 0.25, P = 0.18). Then, we selected the dark turquoise module as the hub module. GO and KEGG enrichment analyses were conducted to explore the functions of the dark turquoise module. The results demonstrated that the dark turquoise module was mainly enriched for translation, DNA replication, and the androgen receptor signaling pathway (Figure 3B).
Figure 3. Weighted gene co-expression network analysis and hub gene screened. (A) Dendrogram of all expressed genes in the top 25% of variance clustered based on a dissimilarity measure (1-TOM). (B) KEGG pathway and GO functional analysis of the pathways of genes in darkturquoise modules. (C) Heatmap of the correlation between module eigengenes and CRT-resistance. (D) PPI network of genes that had the highest score in the PPI degree in the darkturquoise module. The color intensity in each node was proportional to the degree of connectivity in the weighted gene co-expression network.
Through co-expression analysis, 92 genes in the dark turquoise module were considered genes with high module connectivity. Then, the genes were analyzed by the PPI network and 59 genes in the dark turquoise module were identified as hub genes in the co-expression network. Ten hub genes were analyzed by the degree of PPI and the correlation between clinical traits and module connectivity was analyzed? (Figure 3D and Table 1). Finally, we chose the most associated genes, UTP6 and FTSJ3, as the actual hub genes.
To validate the hub genes, we examined the expression of UTP6 and FTSJ3 in rectal cancer tissues of CRT-resistant and CRT-sensitive cases in our datasets. In the internal testing data sets, the relative expression of UTP6 (6.46 ± 0.40 vs. 6.07 ± 0.40, P = 0.02; Figure 4A) and FTSJ3 (6.21 ± 0.32 vs. 5.75 ± 0.47, P < 0.01, Supplementary Figure 1A) was significantly increased in the CRT-sensitive tissues. The ROC curve demonstrated that both UTP6 (P = 0.02, AUC = 0.76, Figure 4D) and FTSJ3 (P < 0.01, AUC = 0.79, Supplementary Figure 1D) could efficiently discriminate CRT-resistant from CRT-sensitive rectal cancer cases. Moreover, Pearson’s analysis was conducted to determine whether the hub gene expression was associated with the TRG grade. The results demonstrated that UTP6 (r = −0.35, P = 0.02, Figure 4C) and FTSJ3 (r = −0.37, P = 0.04, Supplementary Figure 1C) had significant associations with the TRG grade.
Figure 4. Validation of UTP6. (A) In our data (6.46 ± 0.40 vs. 6.07 ± 0.40, P = 0.02) and (B) GSE35452 (0.45 ± 1.51 vs. –0.47 ± 1.45, P = 0.042). (C) Pearson’s correlation analysis between the AJCC TRG grade and UTP6 expression. ROC curves and AUC statistics to evaluate the predictive efficiency of the UTP6 in our data and external data to distinguish CRT-resistance from CRT-sensitive CRC cases from (D) our data and (E) GSE35452. The disease-free survival (F) and overall survival (G) between low and high expression of UTP6. (H) UTP6 expression in the pCR and non-pCR group in the LARC patients’ sample. (I) ROC curves and AUC statistics to evaluate the predictive efficiency of the UTP6 in LARC patients’ samples to distinguish pCR from non-pCR LARC cases. The overall survival (J) and disease-free survival (K) between low and high expression of UTP6 in LARC patients’ sample.
We further explored the prognostic impact of UTP6 and FTSJ3 on the survival of LARC patients. Cut-off Finder was used to identify the cutoff values for UTP6 and FTSJ3. Cut-off Finder identified 6.18 and 5.97 as the cutoff values for UTP6 and FTSJ3 expression, respectively (Supplementary Figure 2). After a median follow-up period of 42 months (range, 4 – 47 months), low expressions of UTP6 and FTSJ3 were associated with significantly worse disease-free survival (DFS) compared to high expression (UTP6 low expression VS. high expression: 50.0% vs. 100.0%, P < 0.001; FTSJ3 low expression VS. high expression: 54.5% vs. 100.0%, P < 0.001), as shown in Figure 4F and Supplementary Figure 1F. Similarly, a high expression of UTP6 and FTSJ3 were correlated with better overall survival (OS) compared to low expression (UTP6 high expression VS. low expression: 100.0%, vs. 50.0%, P < 0.001; FTSJ3 high expression VS. low expression: 100.0% vs. 57.7%, P < 0.001), as demonstrated in Figure 4G and Supplementary Figure 1G.
To validate the hub genes in our data set, we examined the expression level of UTP6 and FTSJ3 by comparing the rectal cancer tissues of CRT-resistant and-sensitive cases using an external dataset. In the external GSE35452 database, UTP6 expression was significantly higher in the CRT-sensitive tissues compared to the CRT-resistant tissues (0.45 ± 1.51 vs. −0.47 ± 1.45, P = 0.042; Figure 4B), but the expression of FTSJ3 was not statistically different between the two groups (0.11 ± 0.40 vs. −0.05 ± 0.53, P = 0.246; Supplementary Figure 1B). Thus, we chose UTP6 as the “real” hub gene for further analysis. ROC analysis revealed that UTP6 could efficiently discriminate CRT-resistant from CRT-sensitive cases (P = 0.045, AUC = 0.672; Figure 3E). However, FTSJ3 could not efficiently distinguish CRT-resistant from CRT-sensitive cases (P = 0.12, AUC = 0.63; Supplementary Figure 1D).
The R2 were utilized to plot Kaplan-Meier curves by using datasets “Tumor Colon-Sieber-290- MAS5.0-u133p2”, “Tumor Colon MVRM -SieberSmith-345- fRMA (bc) - u133p2”, “Tumor Colon-Smith-232-MAS5.0-u133p2”, “Tumor Colon (KRAS mut)-Hase-59- MAS5.0-u133p2”, “Tumor Colon CIT (Combat)-Marisa-566- rma-u133p2”, “Tumor Colon MSI-status (Core Exon)-Sveen-95-rma-sketch- huex10p”, “Mixed Colon Adenocarcinoma-TCGA-174 custom-agg4502a073”, “Tumor Colon (Core-Transcript)-Sveen-333-rma-sketch-huex10p” and “Tumor Colon (Core-Exon)-Sveen-333-rma- sketch-huex10p”. Low UTP6 expression was correlated with significantly worse event and relapse-free survival (all P < 0.05; Figures 5A–H).
Figure 5. High UTP6 expression was associated with a better event-, disease-, and relapse-free survival. (A–H) High UTP6 expression was associated with a significantly better event-, disease- and relapse-free survival (both P < 0.05).
To further verify UTP6 expression in the tumor samples, colonoscopy samples were collected from LARC patients before CRT. The immunohistochemical analysis demonstrated that higher UTP6 scores were associated with better prognosis in LARC patients following CRT. The 3-year OS rate of the low-UTP6 group was significantly lower than that in the high-UTP6 group (60.5% vs. 89.5%; P < 0.01, Figure 4J). Lower UTP6 scores were correlated with better DFS (Figure 4K). The 3-year DFS rate for the low-UTP6 group was significantly higher than that in the high-UTP6 group (42.4% vs. 87.1%; P < 0.01). Moreover, we compared the UTP6 expression in the pCR and non-pCR group. The results revealed that the relative expression of UTP6 was significantly increased in the pCR group (P < 0.01; Figure 4H). The ROC curve demonstrated that UTP6 could efficiently discriminate pCR from non-pCR LARC cases (P < 0.001, AUC = 0.77, Figure 4I). In addition, we detected the UTP6 expression in the rectal cancer tissues and adjuvant cancer tissues, the result demonstrated that UTP6 expression value was similar in rectal cancer and adjacent cancer tissues (P = 0.571, Supplementary Figure 3).
To explore the prognostic impact of UTP6 on the OS and DFS of LARC patients, a Cox regression analysis was performed. Univariate analysis revealed that tumor size (P = 0.004), ypTNM stage (P < 0.001), AJCC TRG grade (P = 0.013), UTP6 expression (P < 0.001), CD133 expression (P < 0.001), and the pre-CRT-CA19-9 level (P = 0.012) were independently associated with DFS in LARC patients following CRT and TME (Table 2). Multivariate Cox regression analysis demonstrated that UTP6 expression (HR = 0.398, 95%CI: 0.280 – 0.567, P < 0.001) and CD133 expression (HR = 1.205, 95%CI: 1.077 - 1.348, P = 0.001) were the independent predictors of DFS in LARC patients following CRT, as shown in Table 2.
Table 2. Cox regression analysis of predictive factors for disease-free survival in the training dataset patients with LARC following CRT (n = 125).
Upon the univariate analysis of the predictors of OS, tumor size (P = 0.006), ypTNM stage (P < 0.001), AJCC grade (P = 0.003), UTP6 expression (P < 0.001), the pre-CRT-CA19-9 level (P = 0.012), CD133 expression (P < 0.001), and tumor differentiation (P = 0.013) were independently associated with OS in LARC patients following CRT and TME (Table 3). The results from the multivariate Cox regression model demonstrated that UTP6 expression (HR = 0.398, 95%CI: 0.280 – 0.567, P < 0.001) and CD133 expression (HR = 1.185, 95%CI: 1.026 - 1.367, P = 0.021) were the independent predictors of OS in LARC patients following CRT, as shown in Table 3.
Table 3. Cox regression analysis of predictive factors for overall survival in the training dataset patients with LARC following CRT (n = 125).
No significant differences were observed between UTP6 low expression group and UTP6 high expression group in terms of gender, age, American Society of Anesthesiologists (ASA) grade, interval time between CRT and surgery, distance from the anal verge, clinical T stage, clinical N stage, pre-CRT CEA level, and pre-CRT CA199 level, as shown in Table 4.
Table 4. Baseline characteristics in patients with LARC following CRT stratified by UTP6 expression (n = 125).
No significant differences were observed between UTP6 low expression group and UTP6 high expression group in terms of pathological type, postoperative complication, circumferential resection margin (CRM) involvement, tumor differentiation, perineural invasion, vascular invasion, and organ preservation procedure (Table 5). Compared to the UTP6 low expression group, the UTP6 high expression group was associated with an increased metastasis to the lymph nodes (0.45 ± 1.4 vs. 3.1 ± 7.1, P < 0.001), pathological T stage, pathological N stage (all P < 0.01), and poorer TRG grade (P < 0.01). In the training dataset, in the high-risk score group a larger tumor size was seen (2.7 ± 1.2 vs. 3.5 ± 1.6, P = 0.002), more lymph nodes were retrieved (11.2 ± 8.7 vs. 15.7 ± 13.8, P = 0.028), and poorer histopathology was observed (P = 0.026) compared with UTP6 low expression group.
Table 5. Operative and postoperative outcomes in patients with LARC following CRT stratified by UTP6 expression (n = 125).
To explore whether UTP6 was modulated by methylation, we evaluated data on the methylation of the UTP6 promoter region in CRC and normal colon tissues. Based on the ULACAN database, methylation of the UTP6 promoter was decreased in normal colon tissue compared to CRC tissue (0.0409 ± 0.0013 vs. 0.0441 ± 0.0005, P = 0.017, Figure 6A). Further, the expression and promoter methylation levels of UTP6 were analyzed in CRC stem and non-stem cells. The relative UTP6 expression was significantly reduced in CRC stem cells compared to CRC non-stem cells (GSE14773: 10.33 ± 0.13 vs. 10.80 ± 0.14; P = 0.04; GSE24747: 3.44 ± 0.09 and 3.81 ± 0.00, P = 0.03; Figures 6C,D). We analyzed promoter methylation in CRC stem cells in the GSE104271 dataset. The results demonstrated that the UTP6 promoter was hypermethylated at cg10893370 (stem cell vs. non-stem cell: 0.06 ± 0.00 vs. 0.09 ± 0.00, P = 0.008, Figure 6B) and cg13453082 (0.05 ± 0.01 vs. 0.08 ± 0.00, P = 0.03, Figure 6B) sites in CRC stem cells.
Figure 6. UTP6 had increased promoter hypermethylation in colon tumors, lower expression in CRC stem cells. (A) From the TCGA database. UTP6 had increased promoter hypermethylation in colon cancer. (B) UTP6 had higher promoter methylation levels in cg10893370 and cg13453082 sites in CRC stem cells. Heat map and bar graph showed that CRC non-stem cells had higher UTP6 expression compared with CRC stem cells in GSE24747 (C) and GSE14773 (D).
GSEA was conducted to investigate the potential mechanism of the UTP6-mediated CRT-resistance in CRC. Our data demonstrated that the negatively correlated KEGG pathways were enriched for the ABC transporter signaling pathway (Figures 7A–C). The positively correlated pathways included transcription factor pathways and cell cycle signaling pathways. To further explore the mechanism of the UTP6-mediated CRT response, the genes co-expressed with UTP6 in the TCGA colon tumor data from ULACAN were analyzed. The results demonstrated that FOXK2 was significantly correlated with UTP6 (r = 0.55, P < 0.01; Figure 7D).
Figure 7. GSEA using our data and co-expression analysis between UTP6 and FOXK2 in the TCGA colon tumor dataset. (A) Transcription factors pathway. (B) Cell cycle. (C) ABC transportanters. (D) Co-expression analysis between UTP6 and FOXK2 in the TCGA colon tumor dataset.
To date, reliable molecular markers for CRT-resistance in LARC patients are still unavailable. In this study, a gene chip array was performed to detect the gene expression in LARC patients who received CRT. Then WGCNA, an advanced methodology for multigene analysis, was conducted to identify the gene co-expression modules associated with CRT resistance. UTP6 was identified and validated as a hub gene correlated with CRT resistance. High UTP6 expression was correlated with better survival in CRC patients. UTP6 was hypermethylated in CRC tissues, especially in the CRC stem cell subpopulation. The underlying mechanism of UTP6 in maintaining CRC stemness might involve transcription factor pathways, especially FOXK2.
Currently, microarray expression profiling has been utilized to screen biomarkers in patients with CRT-resistant rectal cancer. Conventional molecular biology methodology identifies DEGs, but it is difficult to correlate biological information provided by gene names with biological functions independently. WGCNA has emerged as an effective method to discover the relationship between networks, genes, phenotypes, and biological sample information, thus avoiding the shortcomings of the traditional methods (Bakhtiarizadeh et al., 2018; Gao et al., 2016; Magani et al., 2018).
Herein, we performed WGCNA to identify the actual hub gene in our patient cohort. The results demonstrated that the dark turquoise module was chosen as the hub module and enriched for translation, DNA replication, and androgen receptor signaling pathways. Those pathways are involved in multi-drug resistant CRT tumors, indicating that the dark turquoise module was significantly associated with CRT-resistance. As described previously (Liu et al., 2017; Wang et al., 2018), PPI network analysis and clinical trait correlation were performed to screen for the actual hub gene. The results suggested that the UTP6 gene had the highest correlation coefficient. We further analyzed the expression of UTP6 in our previous data and testing data set (GSE35452), which showed that UTP6 was the relevant gene for CRT-sensitivity. ROC analysis showed that UTP6 could effectively discriminate between CRT-resistant and -sensitive cases. To our knowledge, this was the first study to identify and verify UTP6 as an effective new marker for the prediction of CRT response.
Having shown that UTP6 was associated with CRT-resistance and survival in CRC patients, the underlying mechanism remained unclear. Cancer stem cells are widely known as tumor-initiating cells due to stem cell properties. Increasing evidence has shown that cancer stem cells are involved in the resistance to conventional cytotoxic therapies, radio- and chemotherapy (Baumann et al., 2008; Wahab et al., 2017). Herein, we evaluated the relative UTP6 expression in two independent data sets including CRC stem cells and parental cells (GSE14773 and GSE24747). The results demonstrated that the expression of UTP6 was significantly decreased in CRC stem cells compared to CRC non-stem cells.
DNA methylation, a main epigenetic modification in the mammalian genome (Baylin et al., 2001; Herman and Baylin, 2003), may inactivate genes and affect the CRT response (Wilting and Dannenberg, 2012; Ivanova et al., 2013). Several studies have reported that DNA methylation could promote drug or radiation resistance (Su et al., 2011), and thus, could be used to predict treatment response. In the present study, we found hypermethylation in colon cancer tissue and the cg10893370 and cg13453082 sites located in the UTP6 promoter in CRC stem cells. These results indicated that the hypermethylation of UTP6 may result in a decreased expression of UTP6 in CRC stem cells and that the downregulation UTP6 expression in cancer stem cells may be due to hypermethylation of the promoter sites. Several studies have reported that DNA methylation could affect gene expression in the transcriptome, which is involved in CRT-resistance in various cancer stem cells (Kurscheid et al., 2015; Kostopoulou et al., 2018). Importantly, DNA methylation can be reversed by anti-tumor drugs, such as decitabine (Ariazi et al., 2017; Flotho et al., 2018). The findings suggest that the combination of demethylation therapy targeting UTP6 and cancer stem cells during CRT might be beneficial to rectal cancer patients.
UTP6 (also known as HCA66) is an essential component of the UTPB, a large complex composed of six proteins Utp1/Pwp2, Utp6, Utp12/Dip2, Utp13, Utp18, and Utp21 (Grandi et al., 2002; Krogan et al., 2004). Ferraro et al. (2011) reported that Apaf1 could bind with UTP6 and participate in the regulation of centrosomal microtubule nucleation, spindle assembly, cell migration, and mitochondrial network organization. Meanwhile, UTP6 has been proposed as a promising prognostic indicator in bladder cancer (Shivakumar et al., 2017). A previous study reported that UTPB was the consist of the UTP6, which is an important complex in regulating ribosome synthesis (Hunziker et al., 2016; Zhang et al., 2016). Herein, we found that UTP6 was not only related to the ABC transporter signal pathway, but also transcription factor pathways, which are associated with CRT resistance (Shinto et al., 2014; McCoy et al., 2015). Pearson’s correlation analysis showed that the expression of UTP6 was correlated with that of FOXK2, which is a transcription factor known as an anti-oncogene (Shan et al., 2016; de Moraes et al., 2019). The results indicated that the anti-chemoradiotherapy resistance effect of UTP6 may be related to FOXK2 expression. Unfortunately, the precise mechanism of UTP6 involved in CRT sensitivity in rectal cancer patients is still unclear.
There were several limitations to our study. Although the underlying mechanism of UTP6 was explored by bioinformatics analysis, the results warrant further confirmation by in vitro or in vivo experiments. To our knowledge, this was the first study to explore CRT-resistance-related genes through WGCNA using a patient cohort with complete clinicopathological and follow-up data. The present study provided insight into the role of UTP6 in CRT-sensitive in rectal cancer patients.
Through WGCNA, UTP6 was identified and validated as a hub gene and a valid novel predictor for CRT-sensitivity. High expression of UTP6 associated with better survival in LARC patients. UTP6 was hypermethylated in CRC tissues, especially in CRC stem cells subpopulation. GSEA demonstrated that the mechanism underlying UTP6 maintains CRC stemness might be involved in the transcription factors pathway especially FOXK2. Further research is needed to confirm the above findings.
The datasets presented in this study can be found in online repositories. The names of the repository/repositories and accession number(s) can be found below: https://www.ncbi.nlm.nih.gov/geo/, GSE145037.
Studies relative to humans in this article were approved by the Ethics Committee of The Fujian Medical University Union Hospital. The patients/participants provided their written informed consent to participate in this study. Written informed consent was obtained from the individual(s) for the publication of any potentially identifiable images or data included in this article.
YZ, QG, YW, GG, and XL designed and performed the experiments, analyzed the data, and wrote the manuscript. YP, JZ, and YY performed the experiments. All authors read and approved the final manuscript.
This study was supported by the Science Foundation of the Fujian Province (Nos. 2016J01602 and 2019J0105), Special Financial Foundation of Fujian Provincial (Nos. 2015-1297 and 2020B1050), Young and Middle-aged Backbone Training Project in the Health System of Fujian Province (2016-ZQN-26), the Startup Fund for Scientific Research, Fujian Medical University (2017XQ1029 and 2018QH2027), and the Professor Development Foundation of Fujian Medical University (No. JS11006). Talent programs granted from The First Affiliated Hospital of Fujian Medical University (YJRC3600).
The authors declare that the research was conducted in the absence of any commercial or financial relationships that could be construed as a potential conflict of interest.
All claims expressed in this article are solely those of the authors and do not necessarily represent those of their affiliated organizations, or those of the publisher, the editors and the reviewers. Any product that may be evaluated in this article, or claim that may be made by its manufacturer, is not guaranteed or endorsed by the publisher.
The Supplementary Material for this article can be found online at: https://www.frontiersin.org/articles/10.3389/fcell.2021.607782/full#supplementary-material
Supplementary Figure 1 | Validation of FTSJ3. (A) In our data (6.21 ± 0.32 vs. 5.75 ± 0.47, P < 0.01) and (B) GSE35452 (0.11 ± 0.40 vs. −0.05 ± 0.53, P = 0.246). (C) Person analysis between the AJCC TRG grade and FTSJ3 expression. ROC curves and AUC statistics to evaluate the predictive efficiency of the FTSJ3 in our data and external data to distinguish CRT-resistance from CRT−sensitive CRC cases from (D) our data and (E) GSE35452. The disease-free survival (F) and overall survival (G) between low and high expression of FTSJ3.
Supplementary Figure 2 | The result of Cut-off Finder. Plot of the OR of the UTP6 (A) and FTSJ3 (D) expression and the optimal cutoff value. And the optimal cutoff value is compared to the gene expression. ROC curves and AUC analysis to evaluate the predictive efficiency of the optimal cutoff of the UTP6 (B) and FTSJ3 (E) expression. The classification using UTP6 (C) and FTSJ3 (F) expression and patients’ DFS status. And the optimal cutoff value is compared to the gene expression. OR, odds ratio; DFS, disease-free survival.
WGCNA, weighted gene co-expression network analysis; CRT, chemoradiotherapy; CRC, colorectal cancer; LARC, local advanced rectal cancer; GEO, Gene Expression Omnibus; TCGA, the cancer genome atlas; PPI, protein–protein interaction; GSEA, Gene set enrichment analysis; ROC, receiver operating characteristic curve.
Amin, M. B., Edge, S., Greene, F., Byrd, D. R., Brookland, R. K., Washington, M. K., et al. (2017). AJCC Cancer Staging Manual, 8th Edn. Chicago, IL: Springer.
Ariazi, E. A., Taylor, J. C., Black, M. A., Nicolas, E., Slifker, M. J., Azzam, D. J., et al. (2017). A new role for ERα: silencing via dna methylation of Basal, stem cell, and EMT genes. Mol. Cancer Res. 15, 152–164. doi: 10.1158/1541-7786.mcr-16-0283
Bakhtiarizadeh, M. R., Hosseinpour, B., Shahhoseini, M., Korte, A., and Gifani, P. (2018). Weighted gene co-expression network analysis of endometriosis and identification of functional modules associated with its main hallmarks. Front. Genet. 9:453. doi: 10.3389/fgene.2018.00453
Baumann, M., Krause, M., and Hill, R. (2008). Exploring the role of cancer stem cells in radioresistance. Nat. Rev. Cancer 8, 545–554. doi: 10.1038/nrc2419
Baylin, S. B., Esteller, M., Rountree, M. R., Bachman, K. E., Schuebel, K., and Herman, J. G. (2001). Aberrant patterns of DNA methylation, chromatin formation and gene expression in cancer. Hum. Mol. Genet. 10, 687–692. doi: 10.1093/hmg/10.7.687
Benson, A. B., Venook, A. P., Al-Hawary, M. M., Cederquist, L., Chen, Y. J., Ciombor, K. K., et al. (2018). Rectal cancer, version 2.2018, NCCN clinical practice guidelines in oncology. J. Natl. Compr. Cancer Netw. 16, 874–901. doi: 10.6004/jnccn.2018.0061
Botía, J. A., Vandrovcova, J., Forabosco, P., Guelfi, S., D’Sa, K., Hardy, J., et al. (2017). An additional k-means clustering step improves the biological features of WGCNA gene co-expression networks. BMC Syst. Biol. 11:47. doi: 10.1186/s12918-017-0420-6
Budczies, J., Klauschen, F., Sinn, B. V., Gyõrffy, B., Schmitt, W. D., Darb-Esfahani, S., et al. (2012). Cutoff Finder: a comprehensive and straightforward Web application enabling rapid biomarker cutoff optimization. PLoS One 7:e51862. doi: 10.1371/journal.pone.0051862
Chandrashekar, D. S., Bashel, B., Sah, B., Creighton, C. J., Ponce-Rodriguez, I., Bvsk, C., et al. (2017). UALCAN: a portal for facilitating tumor subgroup gene expression and survival analyses. Neoplasia 19, 649–658.
de Moraes, G. N., Ldt, C., Maia, R. C., Lam, E. W., and Sharrocks, A. D. (2019). FOXK2 transcription factor and its emerging roles in cancer. Cancers (Basel) 11:393. doi: 10.3390/cancers11030393
Ferraro, E., Pesaresi, M. G., De Zio, D., Cencioni, M. T., Gortat, A., Cozzolino, M., et al. (2011). Apaf1 plays a pro-survival role by regulating centrosome morphology and function. J. Cell Sci. 124(Pt 20), 3450–3463.
Flotho, C., Sommer, S., and Lübbert, M. (2018). DNA-hypomethylating agents as epigenetic therapy before and after allogeneic hematopoietic stem cell transplantation in myelodysplastic syndromes and juvenile myelomonocytic leukemia. Semin. Cancer Biol. 51, 68–79. doi: 10.1016/j.semcancer.2017.10.011
Gao, B., Shao, Q., Choudhry, H., Marcus, V., Dong, K., Ragoussis, J., et al. (2016). Weighted gene co-expression network analysis of colorectal cancer liver metastasis genome sequencing data and screening of anti-metastasis drugs. Int. J. Oncol. 49, 1108–1118. doi: 10.3892/ijo.2016.3591
Grandi, P., Rybin, V., Bassler, J., Petfalski, E., Strauss, D., Marzioch, M., et al. (2002). 90S pre-ribosomes include the 35S pre-rRNA, the U3 snoRNP, and 40S subunit processing factors but predominantly lack 60S synthesis factors. Mol. Cell 10, 105–115. doi: 10.1016/s1097-2765(02)00579-8
Ha, Y. J., Tak, K. H., Kim, C. W., Roh, S. A., Choi, E. K., Cho, D. H., et al. (2017). PSMB8 as a candidate marker of responsiveness to preoperative radiation therapy in rectal cancer patients. Int. J. Radiat. Oncol. Biol. Phys. 98, 1164–1173. doi: 10.1016/j.ijrobp.2017.03.023
Heald, R. J., Husband, E. M., and Ryall, R. D. (1982). The mesorectum in rectal cancer surgery–the clue to pelvic recurrence. Br. J. Surg. 69, 613–616. doi: 10.1002/bjs.1800691019
Herman, J. G., and Baylin, S. B. (2003). Gene silencing in cancer in association with promoter hypermethylation. N. Engl. J. Med. 349, 2042–2054. doi: 10.1056/nejmra023075
Horvath, S., and Dong, J. (2008). Geometric interpretation of gene coexpression network analysis. PLoS Comput. Biol. 4:e1000117. doi: 10.1371/journal.pcbi.1000117
Huang, Y. F., Niu, W. B., Hu, R., Wang, L. J., Huang, Z. Y., Ni, S. H., et al. (2018). FIBP knockdown attenuates growth and enhances chemotherapy in colorectal cancer via regulating GSK3β-related pathways. Oncogenesis 7:77.
Hunziker, M., Barandun, J., Petfalski, E., Tan, D., Delan-Forino, C., Molloy, K. R., et al. (2016). UtpA and UtpB chaperone nascent pre-ribosomal RNA and U3 snoRNA to initiate eukaryotic ribosome assembly. Nat. Commun. 7:12090.
Ivanova, T., Zouridis, H., Wu, Y., Cheng, L. L., Tan, I. B., Gopalakrishnan, V., et al. (2013). Integrated epigenomics identifies BMP4 as a modulator of cisplatin sensitivity in gastric cancer. Gut 62, 22–33. doi: 10.1136/gutjnl-2011-301113
Kostopoulou, O. N., Mohammad, A. A., Bartek, J., Winter, J., Jung, M., Stragliotto, G., et al. (2018). Glucocorticoids promote a glioma stem cell-like phenotype and resistance to chemotherapy in human glioblastoma primary cells: biological and prognostic significance. Int. J. Cancer 142, 1266–1276. doi: 10.1002/ijc.31132
Krogan, N. J., Peng, W. T., Cagney, G., Robinson, M. D., Haw, R., Zhong, G., et al. (2004). High-definition macromolecular composition of yeast RNA-processing complexes. Mol. Cell 13, 225–239. doi: 10.1016/s1097-2765(04)00003-6
Kurscheid, S., Bady, P., Sciuscio, D., Samarzija, I., Shay, T., Vassallo, I., et al. (2015). Chromosome 7 gain and DNA hypermethylation at the HOXA10 locus are associated with expression of a stem cell related HOX-signature in glioblastoma. Genome Biol. 16:16.
Langfelder, P., and Horvath, S. (2008). WGCNA: an R package for weighted correlation network analysis. BMC Bioinformatics 9:559. doi: 10.1186/1471-2105-9-559
Liu, J., Li, H., Sun, L., Wang, Z., Xing, C., and Yuan, Y. (2017). Aberrantly methylated-differentially expressed genes and pathways in colorectal cancer. Cancer Cell Int. 17:75. doi: 10.1186/s12935-017-0444-4
Magani, F., Bray, E. R., Martinez, M. J., Zhao, N., Copello, V. A., Heidman, L., et al. (2018). Identification of an oncogenic network with prognostic and therapeutic value in prostate cancer. Mol. Syst. Biol. 14:e8202. doi: 10.15252/msb.20188202
Mason, M. J., Fan, G., Plath, K., Zhou, Q., and Horvath, S. (2009). Signed weighted gene co-expression network analysis of transcriptional regulation in murine embryonic stem cells. BMC Genomics 10:327. doi: 10.1186/1471-2164-10-327
McCoy, M. J., Hemmings, C., Miller, T. J., Austin, S. J., Bulsara, M. K., Zeps, N., et al. (2015). Low stromal Foxp3+ regulatory T-cell density is associated with complete response to neoadjuvant chemoradiotherapy in rectal cancer. Br. J. Cancer 113, 1677–1686. doi: 10.1038/bjc.2015.427
Rahbari, N. N., Elbers, H., Askoxylakis, V., Motschall, E., Bork, U., Büchler, M. W., et al. (2013). Neoadjuvant radiotherapy for rectal cancer: meta-analysis of randomized controlled trials. Ann. Surg. Oncol. 20, 4169–4182. doi: 10.1245/s10434-013-3198-9
Sauer, R., Becker, H., Hohenberger, W., Rödel, C., Wittekind, C., Fietkau, R., et al. (2004). Preoperative versus postoperative chemoradiotherapy for rectal cancer. N. Engl. J. Med. 351, 1731–1740.
Sauer, R., Liersch, T., Merkel, S., Fietkau, R., Hohenberger, W., Hess, C., et al. (2012). Preoperative versus postoperative chemoradiotherapy for locally advanced rectal cancer: results of the German CAO/ARO/AIO-94 randomized phase III trial after a median follow-up of 11 years. J. Clin. Oncol. 30, 1926–1933. doi: 10.1200/jco.2011.40.1836
Shan, L., Zhou, X., Liu, X., Wang, Y., Su, D., Hou, Y., et al. (2016). FOXK2 elicits massive transcription repression and suppresses the hypoxic response and breast cancer carcinogenesis. Cancer Cell 30, 708–722.
Shinto, E., Hase, K., Hashiguchi, Y., Sekizawa, A., Ueno, H., Shikina, A., et al. (2014). CD8+ and FOXP3+ tumor-infiltrating T cells before and after chemoradiotherapy for rectal cancer. Ann. Surg. Oncol. 21(Suppl. 3), S414–S421.
Shivakumar, M., Lee, Y., Bang, L., Garg, T., Sohn, K. A., and Kim, D. (2017). Identification of epigenetic interactions between miRNA and DNA methylation associated with gene expression as potential prognostic markers in bladder cancer. BMC Med. Genomics 10(Suppl. 1):30. doi: 10.1186/s12920-017-0269-y
Su, L. J., Mahabir, S., Ellison, G. L., McGuinn, L. A., and Reid, B. C. (2011). Epigenetic contributions to the relationship between cancer and dietary intake of nutrients, bioactive food components, and environmental toxicants. Front. Genet. 2:91. doi: 10.3389/fgene.2011.00091
Tian, F., Zhao, J., Fan, X., and Kang, Z. (2017). Weighted gene co-expression network analysis in identification of metastasis-related genes of lung squamous cell carcinoma based on the Cancer Genome Atlas database. J. Thorac. Dis. 9, 42–53. doi: 10.21037/jtd.2017.01.04
van Gijn, W., Marijnen, C. A., Nagtegaal, I. D., Kranenbarg, E. M., Putter, H., Wiggers, T., et al. (2011). Preoperative radiotherapy combined with total mesorectal excision for resectable rectal cancer: 12-year follow-up of the multicentre, randomised controlled TME trial. Lancet Oncol. 12, 575–582. doi: 10.1016/s1470-2045(11)70097-3
Wahab, S. M. R., Islam, F., Gopalan, V., and Lam, A. K. (2017). The identifications and clinical implications of cancer stem cells in colorectal cancer. Clin. Colorectal Cancer 16, 93–102. doi: 10.1016/j.clcc.2017.01.011
Wang, X., Ghareeb, W. M., Lu, X., Huang, Y., Huang, S., and Chi, P. (2018). Coexpression network analysis linked H2AFJ to chemoradiation resistance in colorectal cancer. J. Cell. Biochem. 120, 10351–10362. doi: 10.1002/jcb.28319
Wilting, R. H., and Dannenberg, J. H. (2012). Epigenetic mechanisms in tumorigenesis, tumor cell heterogeneity and drug resistance. Drug Resist. Updat. 15, 21–38. doi: 10.1016/j.drup.2012.01.008
Yip, A. M., and Horvath, S. (2007). Gene network interconnectedness and the generalized topological overlap measure. BMC Bioinformatics 8:22.
Zhang, B., and Horvath, S. (2005). A general framework for weighted gene co-expression network analysis. Stat. Appl. Genet. Mol. Biol. 4:Article17. doi: 10.2202/1544-6115.1128
Zhang, C., Sun, Q., Chen, R., Chen, X., Lin, J., and Ye, K. (2016). Integrative structural analysis of the UTPB complex, an early assembly factor for eukaryotic small ribosomal subunits. Nucleic Acids Res. 44, 7475–7486.
Zhang, Y., Sun, Y., Xu, Z., Chi, P., and Lu, X. (2017). Is neoadjuvant chemoradiotherapy always necessary for mid/high local advanced rectal cancer: a comparative analysis after propensity score matching. Eur. J. Surg. Oncol. 43, 1440–1446. doi: 10.1016/j.ejso.2017.04.007
Keywords: rectal cancer, chemoradiotherapy, weighted gene co-expression network analysis, UTP6, prognosis
Citation: Zhang Y, Gao Q, Wu Y, Peng Y, Zhuang J, Yang Y, Jiang W, Liu X and Guan G (2021) Hypermethylation and Downregulation of UTP6 Are Associated With Stemness Properties, Chemoradiotherapy Resistance, and Prognosis in Rectal Cancer: A Co-expression Network Analysis. Front. Cell Dev. Biol. 9:607782. doi: 10.3389/fcell.2021.607782
Received: 18 September 2020; Accepted: 12 July 2021;
Published: 18 August 2021.
Edited by:
Yu Zhang, Jinzhou Medical University, ChinaReviewed by:
Zhengjie Huang, First Affiliated Hospital of Xiamen University, ChinaCopyright © 2021 Zhang, Gao, Wu, Peng, Zhuang, Yang, Jiang, Liu and Guan. This is an open-access article distributed under the terms of the Creative Commons Attribution License (CC BY). The use, distribution or reproduction in other forums is permitted, provided the original author(s) and the copyright owner(s) are credited and that the original publication in this journal is cited, in accordance with accepted academic practice. No use, distribution or reproduction is permitted which does not comply with these terms.
*Correspondence: Guoxian Guan, Zmp4aGdneEAxNjMuY29t; Xing Liu, ZmptdWZ5bHhAMTYzLmNvbQ==
†These authors have contributed equally to this work
Disclaimer: All claims expressed in this article are solely those of the authors and do not necessarily represent those of their affiliated organizations, or those of the publisher, the editors and the reviewers. Any product that may be evaluated in this article or claim that may be made by its manufacturer is not guaranteed or endorsed by the publisher.
Research integrity at Frontiers
Learn more about the work of our research integrity team to safeguard the quality of each article we publish.