- 1Key Laboratory of Chicken Genetics and Breeding, Ministry of Agriculture and Rural Affairs, Harbin, China
- 2Key Laboratory of Animal Genetics, Breeding and Reproduction, Education Department of Heilongjiang Province, Harbin, China
- 3College of Animal Science and Technology, Northeast Agricultural University, Harbin, China
One of the main objectives of broiler breeding is to prevent excessive abdominal adipose deposition. The role of RNA modification in adipose deposition is not clear. This study was aimed to map m6A modification landscape in chicken adipose tissue. MeRIP-seq was performed to compare the differences in m6A methylation pattern between fat and lean broilers. We found that start codons, stop codons, coding regions, and 3′-untranslated regions were generally enriched for m6A peaks. The high m6A methylated genes (fat birds vs. lean birds) were primarily associated with fatty acid biosynthesis and fatty acid metabolism, while the low m6A methylated genes were mainly involved in processes associated with development. Furthermore, we found that the mRNA levels of many genes may be regulated by m6A modification. This is the first comprehensive characterization of m6A patterns in the chicken adipose transcriptome, and provides a basis for studying the role of m6A modification in fat deposition.
Introduction
As a result of long-term breeding efforts, the growth rate and meat yield of broilers have significantly improved; however, this has led to excessive body fat (especially abdominal fat) deposition. The accumulation of excess fat in broilers has many undesirable consequences, such as decreased reproductive performance and reduced feed-conversion efficiencies (Zhou et al., 2006; Zhang et al., 2018). Adipose tissue is an important energy storage and endocrine organ and is the cornerstone of energy metabolism homeostasis (McGown et al., 2014; Choe et al., 2016). Adipose tissue development is controlled by a complex network of transcription factors (Farmer, 2006). In addition to transcriptional regulation, evidence suggests that adipose development and fat deposition can be regulated by the epigenetic mechanisms, such as DNA methylation (Zhu et al., 2012), histone modification (Wang et al., 2010), and chromatin remodeling (Siersbaek et al., 2011).
In addition to the chemical modification of DNA and proteins, RNA modification has become a research hotspot in the field of epigenetics in recent years. So far, more than 100 types of chemical modifications of RNA have been identified, with N6-methyladenosine (m6A) methylation being the most pervasive modification in eukaryotes (Yue et al., 2015). M6A is installed by a multicomponent methyltransferase complex consisting of Methyltransferase Like 3 (METTL3), METTL14 and Wilms Tumor 1 Associated Protein (WTAP), and erased by m6A demethylase fat mass and obesityassociated protein (FTO) and α-ketoglutarate-dependent dioxygenase alkB homolog 5 (ALKBH5) (Yang et al., 2018). M6A is involved in many important biological processes through the post-transcriptional regulation of gene expression, including mRNA export, the processing of pri-miRNA, alternative splicing, mRNA degradation and translation (Yang et al., 2018).
In mammals, emerging evidence shows that m6A modification plays a critical role in adipose development and hepatic lipid metabolism (Tao et al., 2017; Lu et al., 2018; Wang et al., 2018). Knockdown of METTL3, METTL14, WTAP or FTO inhibited the differentiation of mouse 3T3-L1 preadipocytes (Zhao et al., 2014; Kobayashi et al., 2018). However, whether m6A modification is involved in poultry adipose deposition is still largely unknown. Here, we used Northeast Agricultural University broiler lines divergently selected for abdominal fat content (NEAUHLF) as fat and lean animal models to compare the differences in m6A topological patterns and functions. We collected abdominal adipose tissue from the two broiler lines for m6A methylation profiling with methylated RNA immunoprecipitation (IP) sequencing (MeRIP-seq). Our data showed that the adipose tissue mRNA was extensively methylated with m6A to fine-tuning the expression of genes responsible for lipid metabolism and adipogenesis.
Materials and Methods
Experimental Birds and Management
Animal studies were conducted according to the guidelines for the care and use of experimental animals established by the Ministry of Science and Technology of the People's Republic of China (approval number: 2006-398) and were approved by the Laboratory Animal Management Committee and the Institutional Biosafety Committee of Northeast Agricultural University (Harbin, China). In total, six male birds (lean line, n = 3, and fat line, n = 3) from the 23rd generation (G23) of NEAUHLF were used for MeRIP-seq analysis. NEAUHLF has been selected since 1996 using plasma very-low-density lipoprotein concentration and abdominal fat percentage (AFP; abdominal fat weight [AFW]/body weight at 7 weeks [wk] of age [BW7]) as selection criteria. Details of the breeding procedure have been described previously (Guo et al., 2011; Zhang et al., 2017). All birds used in this study were kept in similar environmental conditions and had free access to feed and water. From hatching to 3 wk of age, all birds received the starter feed (3,100 kcal of ME/kg and 210 g/kg of crude protein [CP]).Then, from 4 to 7 wk of age, all birds were fed a grower diet (3,000 kcal of ME/kg and 190 g/kg of CP).
Tissue Collection
Six male birds (three birds of each broiler line at 7 wk of age) from G23 were slaughtered after fasting for 10 h, and the BW7 and AFW were measured and used to calculate AFP (Supplementary Figure 1). Abdominal fat tissues were collected, washed with 0.75% NaCl solution, snap-frozen in liquid nitrogen, and stored at −80°C until RNA extraction.
RNA Isolation and Fragmentation
The total RNA from the abdominal adipose tissue was extracted using TRIzol reagent (Invitrogen Co., CA, USA) according to the manufacturer's instructions. The ribosomal-RNA content of the total RNA was reduced using the Ribo-Zero rRNA Removal Kit (Illumina Inc., CA, USA). Then, the RNA was chemically fragmented into fragments of ~100 nucleotides in length using fragmentation buffer (Illumina Inc.).
Methylated RNA IP Library Construction and Sequencing
The MeRIP-seq service was provided by Cloudseq Biotech Inc. (Shanghai, China). Briefly, IP of the m6A RNA was performed with the GenSeqTM m6A RNA IP Kit (GenSeq Inc., China) following the manufacturer's instructions. Both the m6A IP samples and the input samples without IP were used for library generation with NEBNext® Ultra II Directional RNA Library Prep Kit (New England Biolabs Inc., USA). The quality of the libraries was evaluated with the BioAnalyzer 2100 system (Agilent Technologies Inc., USA). Library sequencing was performed on an Illumina Hiseq instrument with 150 bp paired-end reads.
MeRIP-seq Analysis
Briefly, paired-end reads were harvested from the Illumina HiSeq 4000 sequencer, and Q30 scoring was used for quality control. Cutadapt software (v1.9.3) was employed for 3′-adaptor-trimming and removal of low-quality reads. The clean reads were aligned to the reference chicken genome sequences (galGal6) using Hisat2 software (v2.0.4), and only the uniquely mapped and non-duplicated alignments were further analyzed. The m6A-modification peaks were called by MACS software (Zhang et al., 2008), the “effective genome size” parameter was adjusted to the calculated transcriptome size (1.77 × 108) (Dominissini et al., 2012); meanwhile, the input RNA sequencing (RNA-seq) data were used as the background when calling peaks. Peaks that shared at least 50% overlapping lengths were defined as recurrent peaks.
To examine the distribution pattern of the m6A peaks throughout different regions of the transcripts, the mRNA transcripts were divided into five non-overlapping segments: the 5′UTR, start codon (100 nucleotides centered on the start codon), CDS, stop codon (100 nucleotides centered on the stop codon), and 3′UTR. Each area was separated into 20 bins (Luo et al., 2014). Bedtools (v2.26.0) was used to count the peak number of each bin, and the counts were employed to plot the patterns by R (v3.4.4).
In the fat and lean groups, the top 1,000 significantly enriched peaks (MACS-assigned fold change > 2 and P < 0.00001) within the mRNAs from three biological replicates were combined (Dominissini et al., 2012), and 101 nucleotides centered on the collected peaks of each group were subjected to de novo motif analysis using DREME software (Bailey, 2011). The DREME tool in the MEME suite (http://meme-suite.org/tools/dreme) was used to discover relatively short (up to 8 bp), ungapped motifs that are enriched within a set of target sequences (m6A peak sequences) relative to a set of control sequences (shuffled m6A peak sequences) (Bailey, 2011).
We obtained the common and unique peaks using bedtools intersect (v2.26.0). For a peak to be classified as line-unique, it was assumed not to overlap (<50% overlapping length) any peak of the other line; meanwhile, we defined a peak that appeared in both chicken lines as a common peak (≥50% overlapping length). Line-dynamic methylated peaks (fold change ≥2 and P < 0.05), which showing a change of intensity in some of the common peaks, were identified by diffReps software.
The gene expression levels were determined using the input data, and the number of sequenced fragments of each transcript was normalized using the algorithm of Fragments Per Kilobase of Transcript Per Million Fragments Mapped (FPKM) by Cufflinks software (v2.2.1). Differentially expressed transcripts (fold change ≥2 and P < 0.05) between fat and lean groups were identified with the Cuffdiff program (v2.2.1). The FPKM of input and IP samples were calculated by Cufflinks software (v2.2.1). NNFPKM (NNFPKM = FPKM_IP/FPKM_INPUT) were used to analyze the m6A enrichment level in fat and lean groups. Then FPKM_INPUT and NNFPKM were log2 transformed and Pearson correlation analysis of mRNA expression levels and m6A methylation levels was carried out. Gene Ontology (GO) and pathway analyses were performed using GO (www.geneontology.org) and the Kyoto Encyclopedia of Genes and Genomes (KEGG) database (www.genome.jp/kegg). The thresholds for significant enrichment for GO and KEGG analysis were set at P < 0.05.
Results
Transcriptome-Wide Mapping of the M6A Methylation Landscape in Chicken Adipose Tissues
MeRIP-seq produced 80,966,354–102,649,284 raw reads from input or IP abdominal fat tissues from lean line (L-AF) and fat line (F-AF) chickens. After filtering out low quality data, more than 71,600,000 high-quality reads from each sample were mapped to the galGal6 genome. More than 86% of the clean reads from all the samples were uniquely mapped to chicken reference genome (Table 1). In the input samples, we detected 9,041 and 9,452 expressed mRNA transcripts in lean line and fat line, respectively. After the methylated-RNA fragments were mapped to the transcriptome, 4,615 m6A transcripts (common m6A transcripts from three biologic replicates) were identified among the 5,965 coding transcripts (common mRNA transcripts from three biologic replicates) in the L-AF samples and 4,438 m6A transcripts among the 6,654 coding transcripts in the F-AF samples (Table 2). The proportion of methylated transcripts were 77 and 67% in L-AF and F-AF, respectively. In addition, we detected 7,097 recurrent m6A peaks within 5,965 coding transcripts in L-AF and 6,966 recurrent m6A peaks among 6,654 coding transcripts in F-AF (Table 2; Supplementary Data 1). Based on this information, we estimated that the chicken abdominal adipose transcriptome contained 1.54–1.57 m6A peaks per methylated transcript and 1.05–1.19 m6A peaks per transcript. These data are similar to those of the chicken ovary and pig longissimus dorsi muscle, which have ~1.5 m6A peaks per methylated transcript (Fan et al., 2019; Jiang et al., 2019); and mouse liver and pig subcutaneous fatty tissue transcriptomes, which possess 1.3 m6A peaks per transcript (Dominissini et al., 2012; Tao et al., 2017). However, our results were lower than that of the mouse L cells, which presenting about 3 m6A residues per mRNA transcript (Perry et al., 1975).
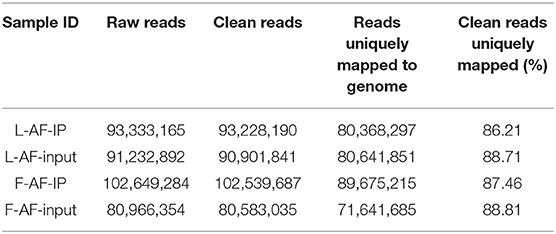
Table 1. Summary of sequencing data and read-alignment statistics from MeRIP-seq in abdominal adipose in fat and lean broiler lines.
To determine how the m6A modification was distributed throughout the chicken transcriptome. We classified the methylated transcripts based on the number of m6A peaks contained in each transcript, and found that nearly 85% of the methylated transcripts contained one or two m6A peaks, and about 5% of the methylated transcripts contained four or more peaks (Figure 1A); this ratio is similar to that previously reported in humans (5.5%) (Dominissini et al., 2012) but is lower than that in pigs (10%) (Wang et al., 2018) and Arabidopsis thaliana (17%) (Wan et al., 2015). We then investigated whether the m6A peaks we identified share a conservative RRACH motif (where R stands for purine, A represents m6A, and H represents a non-guanine base) (Dominissini et al., 2012; Meyer et al., 2012; Luo et al., 2014), we conducted a search for the motifs enriched in the regions around the m6A peaks. The results showed that GGACU was significantly enriched and consistently considered to be the best motif in both broiler lines (Figure 1B). To confirm the preferential localization of m6A in the transcripts, m6A peaks were categorized into five non-overlapping segments: 5′UTR, the start codon segment, coding sequence (CDS), the stop codon segment and 3′UTR. Our results show that m6A was most often located in the CDS, and sometimes near the start and stop codons (Figure 1C), which is consistent with the patterns identified in the mouse and pig (Tao et al., 2017; Luo et al., 2019). Metagene profiling of the m6A peaks showed that they were primarily enriched in CDSs, near the start and stop codons, and close to the beginning of 3′UTRs (Figure 1D), which differs from the pattern identified in mammals (Meyer et al., 2012; Tao et al., 2017).
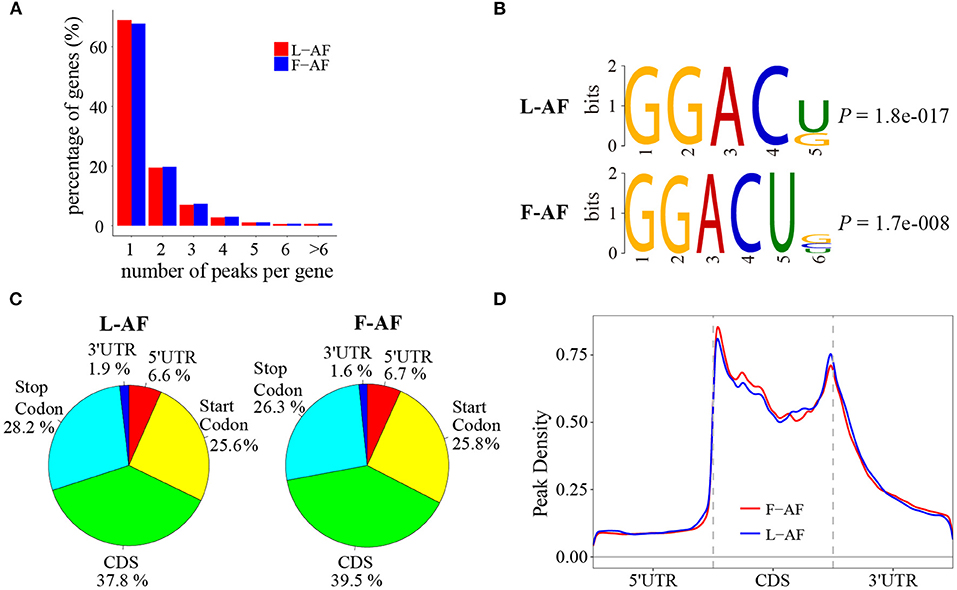
Figure 1. Overview of N6-methyladenosine methylation within mRNAs in fat- and lean-line broiler chickens. (A) Sequence motif of m6A-containing peak regions. (B) Percentage of m6A-methylated transcripts with different number of m6A peaks. (C) Pie charts showing the percentage of m6A peaks in five non-overlapping segments of mRNA transcripts. (D) Enrichment of m6A peaks along mRNA transcripts. L-AF and F-AF represent abdominal fat tissue from lean- and fat-line chickens, respectively.
Biological Pathways Associated With Common and Line-Unique M6A Genes
To discover the differencesin m6A modification between the two chicken lines, we first identified the line-unique m6A peaks and genes. We found 4,318 peaks (representing 3,325 genes) that were common methylated in L-AF and F-AF, along with 2,783 and 2,656 peaks (representing 1,290 and 1,113 genes, respectively) that were specifically methylated in L-AF and F-AF, respectively (Figure 2A; Supplementary Data 2). To predict the functions associated with the m6A-modified genes, we conducted the gene ontology (GO) biological process (BP) and KEGG pathway analysis. The m6A genes common to both lines were predominantly assigned to lipid metabolism, transcription, protein modification, the Wnt-signaling pathway, and the cytoskeleton (P < 0.05, Figures 2B,C, Supplementary Data 3, 4). In addition, the L-AF-unique m6A genes (L-AF UMGs) were significantly involved in development-associated processes, cell junction assembly, ribosome biogenesis, and others (P < 0.05, Figures 3A,B, Supplementary Data 3, 4). However, the F-AF-unique m6A genes (F-AF UMGs) were generally involved in the cellular responses to transforming growth factor-beta, mRNA processing, protein localization, and ubiquitin-mediated proteolysis (P < 0.05, Figures 4A,B, Supplementary Data 3, 4).
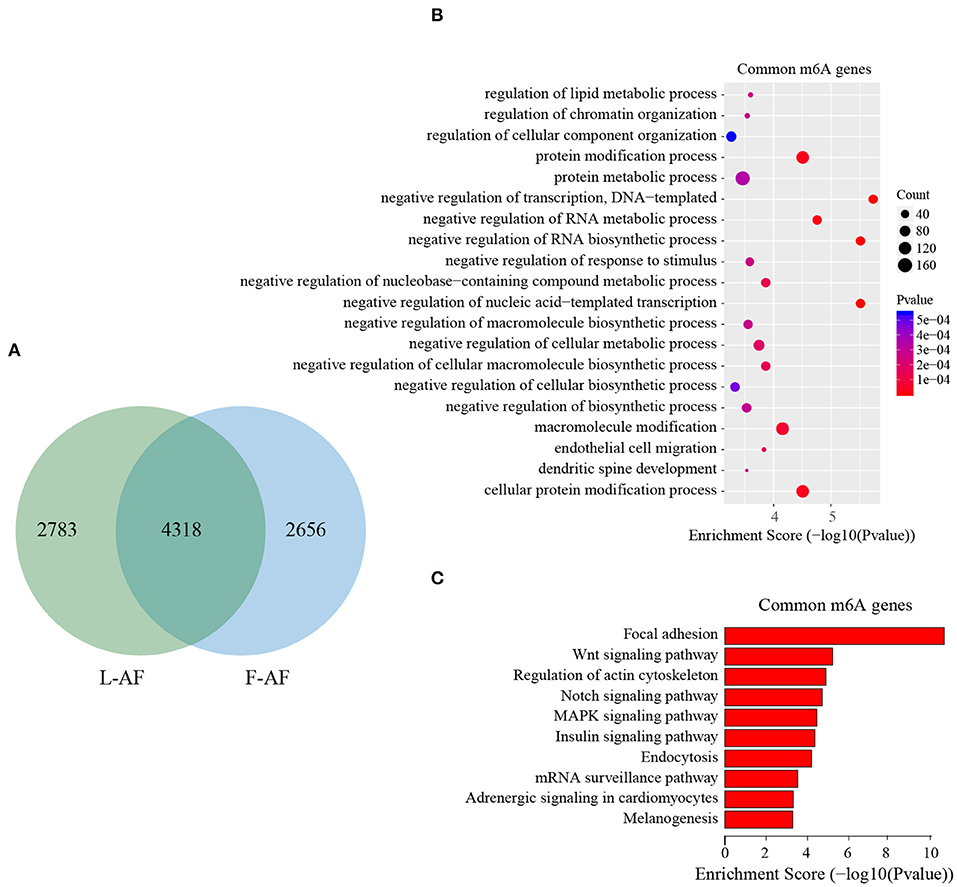
Figure 2. GO biological process and KEGG pathway analyses of common m6A genes in broiler chickens. (A) Venn diagram showing overlap of the m6A peaks from L-AF and F-AF samples. (B) GO enrichment analysis of common m6A genes (P < 0.05). (C) Pathway analysis of common m6A genes (P < 0.05).
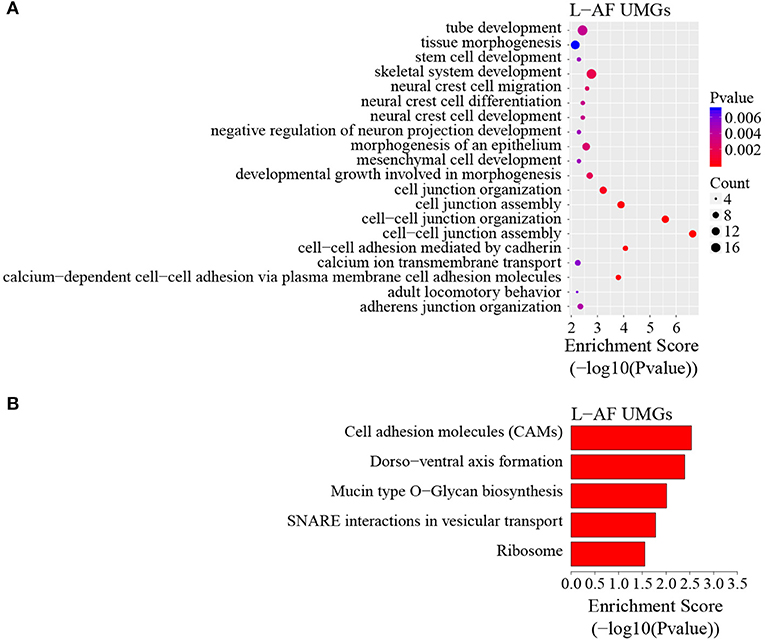
Figure 3. GO biological process and KEGG pathway analyses of unique m6A genes in lean-line broiler chickens. (A) GO analysis of unique m6A genes in the lean line (P < 0.05). (B) Pathway analysis of unique m6A genes in the lean line (P < 0.05).
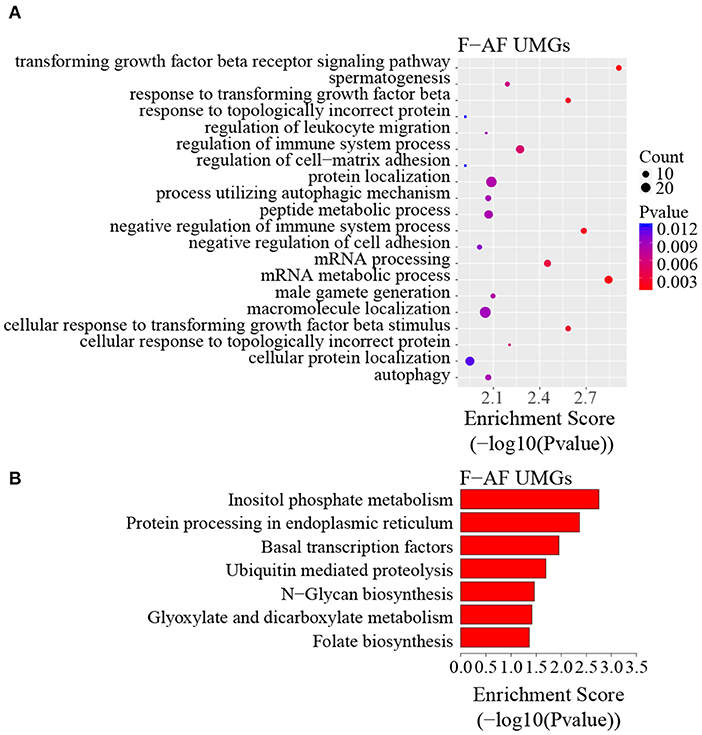
Figure 4. GO biological process and KEGG pathway analyses of unique m6A genes in fat-line broiler chickens. (A) GO analysis of unique m6A genes in the fat line (P < 0.05). (B) Pathway analysis of unique m6A genes in the fat line (P < 0.05).
Involvement of Line-Dynamic M6A Genes in Lipogenesis-Related Pathways
In addition to the line-unique m6A genes, the line-dynamic m6A genes (common to both chicken lines but with different m6A peak intensities) were also selected for GO biological process and KEGG pathway analyses. We found 1,504 common m6A peaks with remarkably different abundances between the two chicken lines, which represented 1,172 coding genes, of which 71.1% (1,069/1,504) were lower in the fat line compared with the lean line (Table 3, Supplementary Data 5). Tables 4, 5 show the top 15 high and low m6A peaks of mRNAs (fat line vs. lean line) with the highest fold-change values. We discovered that the genes with high m6A peaks were mainly involved in the cellular responses to peptide hormone stimuli and lipogenesis-related pathways, including fatty acid biosynthesis and fatty acid metabolism (P < 0.05, Figures 5A,B, Supplementary Data 6, 7), while those with low m6A peaks were involved with development-associated processes, calcium-signaling pathway, steroid hormone biosynthesis, and others (P < 0.05, Figures 5C,D, Supplementary Data 6, 7).
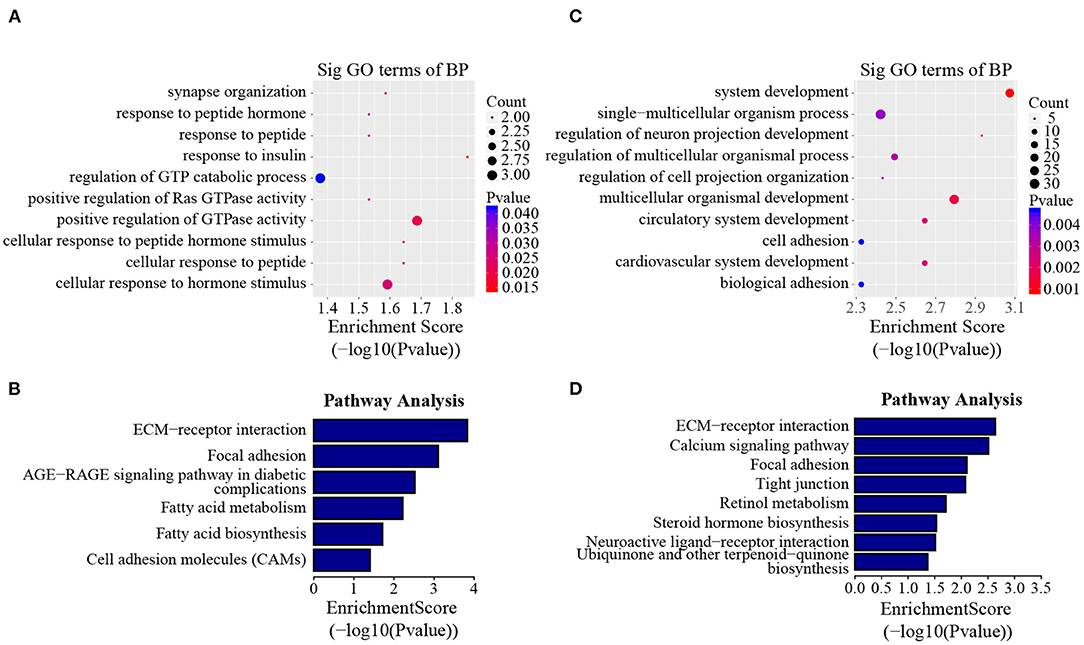
Figure 5. GO biological process and KEGG pathway analyses of line-dynamic m6A genes. (A) GO analysis of high m6A methylated genes (fat line vs. lean line; P < 0.05). (B) Pathway analysis of high m6A methylated genes (fat line vs. lean line; P < 0.05). (C) GO analysis of low m6A methylated genes (fat line vs. lean line; P < 0.05). (D) Pathway analysis of low m6A methylated genes (fat line vs. lean line; P < 0.05). BP, biological process; Sig, significant.
Gene mRNA-Level Regulation by m6A Modification
To understand whether m6A modification can affect gene expression, we used the input RNA-seq data to investigate the differential expression of genes between the two chicken lines. In total, 352 high expression genes and 424 low expression genes in the fat line compared with the lean line were identified (Supplementary Figure 2, Supplementary Data 8). Of the 1,172 line-dynamic m6A genes in total, 146 (12.5%) showed mRNA-expression differences (Supplementary Data 9), indicating that the mRNA levels of these genes may be regulated by m6A modification. Among the 146 genes, it should be noted that the mRNA levels of 95% (52/55) of the high m6A methylated genes were high and were named “hyper-up” genes. Similarly, the mRNA levels of 92% (84/91) of the low m6A methylated genes were low and were named “hypo-down” genes. Only 10 of the 146 genes (7%) showed opposing mRNA expression and m6A-methylation trends, and these genes were termed hyper-down or hypo-up genes (Figure 6A). Interestingly, several lipogenesis-related genes showed differences in both m6A methylation and mRNA expression. For instance, acyl-CoA synthetase long-chain family member 1 (ACSL1), which is associated with fatty acid transport, showed significantly higher m6A methylation and mRNA levels in the fat birds than in the lean birds (Figure 6B); while lipin1 (LPIN1), which is associated with adipocyte differentiation, exhibited lower m6A methylation and mRNA expression levels in the fat birds than in the lean birds (Figure 6C). Finally, we further examined whether gene regulation in the chicken adipose tissue is correlated with the m6A modification by plotting the abundance of m6A peaks with the mRNA expression levels. As shown in Figure 7, the plot of m6A peak enrichment level vs. mRNA abundance revealed a negative correlation between global RNA methylation and gene expression in both chicken lines (L-AF: Pearson's r = −0.9966, P < 0.0001; F-AF: Pearson's r =-0.9966, P < 0.0001).
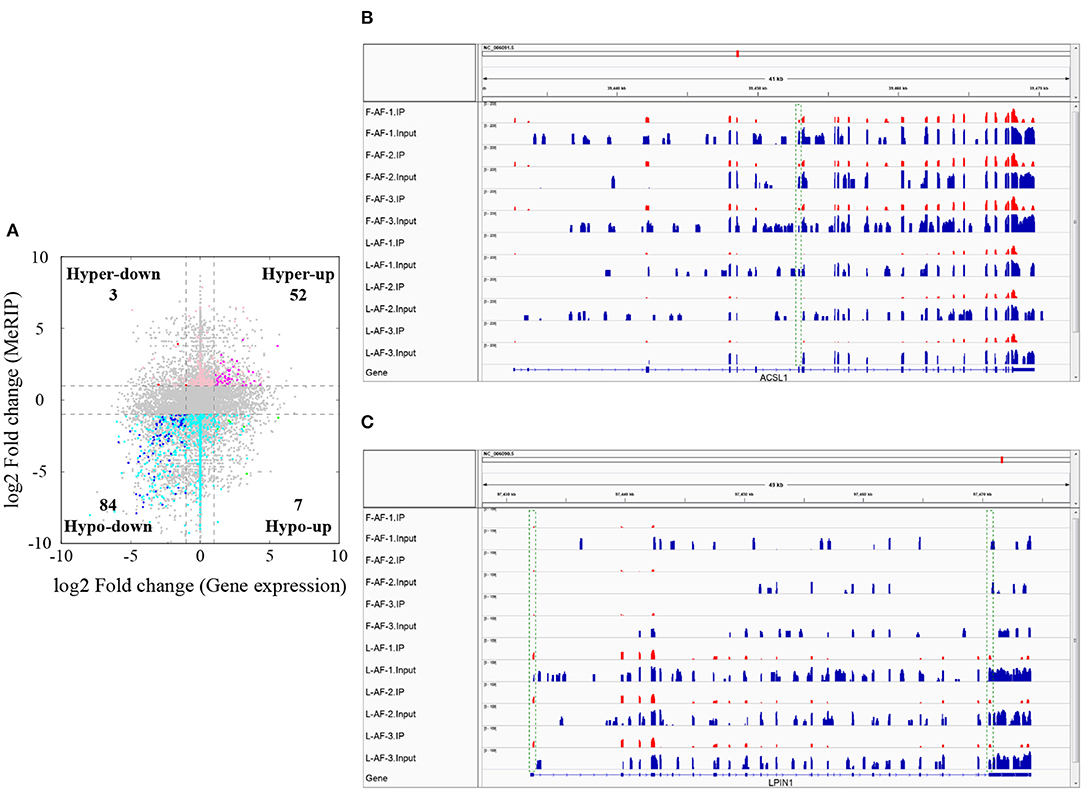
Figure 6. Relationship between m6A content and mRNA levels in adipose tissue of broiler chickens. (A) Distribution of genes with a marked change in both mRNA expression and m6A methylation in fat-line compared with lean-line chickens. (B) The abundance of m6A in the ACSL1 mRNA transcripts of fat (F-AF-1, F-AF-2, and F-AF-3) and lean (L-AF-1, L-AF-2, and L-AF-3) birds, as detected by MeRIP-seq. The intensity of the m6A peak shown in the green rectangle is markedly higher in fat birds compared with lean birds. (C) The abundance of m6A in the LPIN1 mRNA transcript in fat and lean birds, as detected by MeRIP-seq. The intensities of the m6A peaks shown in the green rectangles are markedly lower in fat birds compared with lean birds.
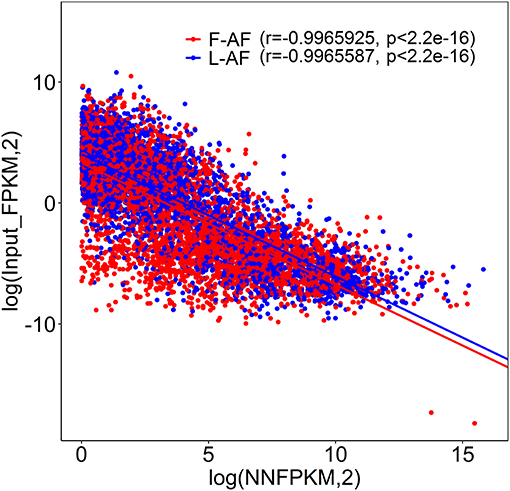
Figure 7. Plot of m6A peak enrichment and mRNA abundance in fat and lean chicken lines. Obvious negative correlation between m6A peak enrichment and modified mRNA abundance was found.
Discussion
Adipose tissue is important for energy storage, endocrine functions, and the control of energy metabolism (McGown et al., 2014; Choe et al., 2016). Over the past few decades, the regulatory mechanisms of adipose tissue development and fat deposition, such as transcription factors, DNA methylation and histone modification, have been extensively studied, and a series of important progressions have been made (Farmer, 2006; Wang et al., 2010; Zhu et al., 2012). Recently, various chemical modifications of RNA, such as m6A, N1-methyladenosine (m1A), and 5-methylcytosine (m5C), have been reported to play important roles in many physiological and pathological processes, including embryonic development, spermatogenesis, and the occurrence and development of a variety of diseases (Lin et al., 2017; Yang et al., 2019; Zhao et al., 2019). However, the role and underlying mechanism of RNA modification in adipose deposition are still uncharted territory. To this end, we conducted m6A methylome profiling of chicken adipose tissue using MeRIP-seq. To our knowledge, this is the first comprehensive high-throughput study to explore RNA modification in poultry adipose tissue. Our findings show the differences in m6A-modification patterns between the adipose tissue of fat and lean broilers. Further analysis suggested that m6A methylation may be an important factor in chicken adipose deposition via the regulation of gene expression.
Nearly 77 and 67% of mRNA transcripts underwent m6A methylation in L-AF and F-AF on average, respectively, suggesting that m6A plays a major role in adipose tissue development and fat deposition. In addition, the m6A peaks were primarily found in the highly conserved sequence motif GGACU (Figure 1B). M6A is generated by the binding of m6A methyltransferase to a highly conserved consensus sequence, GGACU (Shen et al., 2016). The RNA binding motif of METTL3, METTL14 and WTAP are GGAC, GGAC, and GACU, respectively (Liu et al., 2014). When the highly conserved GAC was mutated to GAU, m6A was no longer methylated in Rous sarcoma virus mRNA transcript (Kane and Beemon, 1987). A recent study showed that single nucleotide polymorphisms located at the GGAC positions could affect m6A methylation status (Zhang et al., 2020). Despite GGACU motif is important for the recognition by m6A methyltransferase, only a portion of GGACU sites are methylated in vivo (Gilbert et al., 2016), suggesting that the molecular mechanism regulating m6A modification needs to be further explored. In this study, interestingly, the m6A peaks were abundant not only in the CDS, stop codons, and 3′UTRs but also near the start codons (Figure 1D). This m6A-enrichment pattern is inconsistent with that of mammalian species (Meyer et al., 2012; Tao et al., 2017) but is similar to that of Xenopus laevis and Arabidopsis thaliana (Luo et al., 2014; Sai et al., 2020). This phenomenon may be attributed to differences in lipogenesis patterns between mammalian and birds (Gondret et al., 2001). It also seems to reflect the unique position of birds in the long evolutionary history of m6A modification in animals. In general, the predominance of m6A near stop codons and 3′UTRs has been found in most of the mRNAs of mammals, birds, amphibians, and plants (Luo et al., 2014; Tao et al., 2017; Fan et al., 2019; Sai et al., 2020), and this m6A-enrichment pattern may be representative of the typical mRNA m6A topology of eukaryotes. The high levels of m6A methylation in the 3′UTRs or near stop codons may be responsible for mRNA stability and alternative polyadenylation (Shen et al., 2016; Yue et al., 2018). Previous studies showed that m6A methylation in the CDS is likely to be associated with alternative splicing and translation efficiency (Zhao et al., 2014; Lin et al., 2019). Furthermore, the high m6A levels near the start codon may prevent mRNA degradation (Luo et al., 2014). In the present study, a negative relationship was observed between the global mRNA expression level and m6A methylation extent in chicken, which indicates m6A might affect chicken fat deposition at least in part through the regulation of mRNA stability.
The results of the GO and KEGG analyses in this study showed that m6A genes common to both broiler lines were significantly enriched in the processes and pathways associated with adipose development and fat deposition, such as lipid metabolism and the Wnt-signaling pathway (Figures 2B,C), which is consistent with a previous study that showed that the genes commonly methylated in the backfat of both fat (Jinhua) and lean (Landrace) pigs were mainly involved in cellular lipid metabolic processes (Wang et al., 2018). This result supports the findings of the previous study, in which mRNA m6A modifications were relevant to tissue-specific functions (Li et al., 2014). In addition, the L-AF-unique m6A genes were primarily enriched in developmental-associated processes and, intriguingly, in “ribosome” (Figures 3A,B), which includes genes such as ribosomal protein S10 (RPS10), ribosomal protein L10a (RPL10A), and mitochondrial ribosomal protein L16 (MRPL16). This result is different from the previous study, which showed that the unique m6A genes in the backfat of fat Jinhua pigs were significantly involved in translational initiation and ribosomal large-subunit biogenesis (Wang et al., 2018). This phenomenon may be attributed to differences in lipogenesis patterns between mammalian and avian species (Gondret et al., 2001). However, F-AF-unique m6A genes were significantly enriched in “mRNA processing” (Figure 4A), which includes genes such as pre-mRNA-processing factor 19 (PRPF19), cleavage- and polyadenylation-specific factor 6 (CPSF6), and CWC22 spliceosome-associated protein homolog (CWC22). The regulation of RNA metabolism by m6A modification depends on the recognition and binding of the specific m6A-reader proteins to the m6A sites (Yang et al., 2018). The m6A-reader protein YTH-domain-containing 1 (YTHDC1) has been shown to mediate alternative splicing, and YTH N6-methyladenosine RNA-binding protein 1 (YTHDF1) is responsible for enhancing translation efficiency (Yang et al., 2018). From our results, we speculated that the methylation of the mRNAs associated with mRNA processing and ribosome function might affect the expression of these same mRNAs, resulting in subsequent changes to global pre-mRNA splicing and protein synthesis, which might be another level of regulation involving alternative splicing and translation.
In mammals, an abundance of evidence has shown that m6A modification is involved in the regulation of adipose development and fat deposition (Zhang et al., 2015; Wu et al., 2017; Zong et al., 2019). Intriguingly, the results of the GO and KEGG analyses of the genes harboring dynamic methylated peaks showed that the high m6A methylated genes (fat line vs. lean line) were mainly involved in processes and pathways associated with lipid metabolism, such as fatty acid metabolism and fatty acid biosynthesis (Figure 5B), which further supports the importance of m6A in obesity. For example, stearoyl coenzyme A desaturase (SCD) is related to fatty acid metabolism and was up-methylated approximately 52-fold in the fat birds compared with the lean birds. SCD is a rate-limiting enzyme that catalyzes the formation of unsaturated fatty acids (Ntambi, 1999). A genome-wide association study in pigs identified SCD as a major gene affecting fatty acid composition and intramuscular fat content (Ros-Freixedes et al., 2016), and a recent study showed that SCD may be important for chicken adipose deposition and metabolism (Mihelic et al., 2020). It worth mentioning that insulin-like growth factor binding protein 2 (IGFBP2) was up-methylated about 20-fold in the fat birds compared with the lean birds (Table 4), although IGFBP2 is not involved with the pathways associated with lipid metabolism. IGFBP2 is a cytokine secreted by differentiating white adipocytes and is regulated by DNA methylation in human abdominal obesity (Zhang et al., 2019). In a previous study, we found that IGFBP2 polymorphism (1196C>A) is significantly associated with AFW and AFP in the NEAUHLF population (Leng et al., 2009). In addition, we also found that the SNP 1196C>A within IGFBP2 3′UTR could influence its expression by affecting the regulation of gga-miR-456-3p (Yu et al., 2014). In this study, the m6A peak was found to be located in the CDS of IGFBP2, not 3′UTR. So we considered that the SNP 1196C>A may not affect the m6A methylation of IGFBP2. In contrast to the high m6A methylated genes, the genes with low m6A peaks (fat line vs. lean line) were primarily related to developmental-associated processes, such as cardiovascular system development (Figure 5C), further reinforcing the theory that there is a close relationship between abdominal obesity and cardiovascular disease (Sahakyan et al., 2015). Therefore, we postulated that the different m6A-methylation patterns might reflect significant phenotypic changes between the fat and lean broiler lines.
The mRNA m6A modifications are recognized and bound by m6A-reader proteins, which include YTH-domain family member 2 (YTHDF2) and insulin-like growth factor 2 mRNA-binding proteins (IGF2BPs) and are involved in the regulation of mRNA stability. YTHDF2 was shown to mediate mRNA decay (Zhu et al., 2014), and IGF2BPs (including IGF2BP1/2/3) are responsible for enhancing mRNA stability (Huang et al., 2018). In the present study, the mRNA expression levels of many genes were found to be affected by their m6A levels (Figures 6A, 7). Based on this result, we speculated that the m6A sites in the mRNA transcripts in chicken adipose tissue might be recognized and bound by YTHDF2 or IGF2BPs, thus changing the mRNA stabilities. However, further research is required to validate this hypothesis.
Our results show that several important lipogenic genes, including ACSL1, fatty acid synthase (FASN), LPIN1, and LDL receptor related protein 4 (LRP4), showed variations in both m6A methylation and mRNA expression. The expansion of adipose tissue mass is the result of an increase in the number of adipocytes and an increase in the size of individual fat cells. The number of adipocytes is determined by adipocyte differentiation (adipogenesis), while the size of the adipocytes is related to triglyceride (TG) accumulation in lipid droplets (Rosen and Spiegelman, 2006). ACSL1 is an acyl-CoA synthetaseand a long-chain fatty acid transport protein that can promote fatty acid uptake by adipocytes (Schaffer and Lodish, 1994; Tong et al., 2006). FASN is a key rate-limiting enzyme in de novo synthesis of fatty acids (Song et al., 2018). When there is excess energy in the body, most newly synthesized fatty acids are esterified into TGs for storage (Song et al., 2018). Our findings showed that the m6A methylation and mRNA expression of ACSL1 and FASN were higher in the fat birds compared with the lean birds, indicating that hypermethylation of ACSL1 and FASN mRNA in the fat line might promote the formation of TGs by enhancing mRNA stability and, thus, increasing gene-expression levels. Several lines of evidence have shown that adipocytes are integral to energy metabolism regulation (Rondinone, 2006). Preadipocyte differentiation is controlled by a complex network of multiple transcriptional regulators, of which peroxisome proliferator-activated receptor gamma (PPARγ) is the most important (Farmer, 2006). Research has shown that LPIN1 interacts with and enhances the transcriptional activity of PPARγ and promotes the differentiation of 3T3-L1 preadipocytes (Kim et al., 2016). In the current study, the m6A methylation and mRNA expression of LPIN1 were higher in the lean birds compared with the fat birds, which is consistent with a previous study that found LPIN1 expression levels were increased in the adipose tissue of lean subjects compared with the fat subjects (van Harmelen et al., 2007) This phenomenon might be due to the compensatory increase in LPIN1 expression to maintain the balance of energy metabolism in the lean birds. We also found 10 genes showing opposing mRNA expression and m6A methylation patterns (Figure 6A). Interestingly, one of these, LRP4, is related to lipid metabolism; LRP4 mRNA was low methylated and its expression level was high in the fat line compared with the lean line. LRP4 is a transmembrane protein of the low-density lipoprotein receptor family (Alrayes et al., 2020). A recent study showed that the mice knockout of LRP4 gene in adipocytes exhibit a reduction in adipocyte size and improved lipid and glucose homeostasis (Kim et al., 2019), suggesting that LRP4 is a positive regulator of adipocyte size. Therefore, it is conceivable that the hypomethylation of LRP4 in the fat line might increase adipocyte size by promoting LRP4 mRNA levels through a reduction in YTHDF2-mediated mRNA decay, although, further exploration is needed to shed light on this aspect. We propose that the m6A modifications within the mRNAs of ACSL1, FASN, LPIN1,and LRP4 may be closely involved in adipose deposition and energy homeostasis in chickens. It is notable that, of the 1,172 line-dynamic m6A genes, most (87.5%) did not show mRNA-level variations between the fat and lean broiler lines. This phenomenon may be due to two reasons: (1) m6A may affect chicken abdominal fat deposition via other mechanisms, such as translation regulation, in addition to the regulation of mRNA stability; (2) Gene expression regulation is complex. Besides m6A mehthylation, the mRNA level of gene is influenced by various transcription and posttranscriptional regulatory factors, such as transcription factors (Farmer, 2006), transcription cofactors (Fabre et al., 2012), DNA methylation (Zhu et al., 2012), histone modification (Wang et al., 2010), chromatin remodeling (Siersbaek et al., 2011), and non-coding RNAs (Li et al., 2016; Losko et al., 2018).
Conclusion
In summary, we analyzed the m6A methylomes of chicken abdominal adipose tissues and proposed that m6A modification may play a key role in regulating the expression of genes contributing to lipid metabolism and adipogenesis. This comprehensive m6A map not only provides a basis for studying the roles of m6A methylation in chicken fat deposition but also opens a new avenue in the study of RNA epigenetics in adipobiology.
Data Availability Statement
The datasets presented in this study can be found in online repositories. The names of the repository/repositories and accession number(s) can be found below: [NCBI SRA AND PRJNA657377].
Ethics Statement
The animal study was reviewed and approved by The Ministry of Science and Technology of the People's Republic of China (Approval Number: 2006-398) and were approved by the Laboratory Animal Management Committee and the Institutional Biosafety Committee of Northeast Agricultural University (Harbin, China).
Author Contributions
BC contributed to the design of the experiments, carried out the experiments, performed the statistical analyses, and prepared the manuscript. LL contributed to the design of the experiments and helped with managing the birds. ZL, WW, YL, YJ, NW, and HL contributed to writing the manuscript. SW conceived and designed the study, participated in data interpretation, and contributed to writing the manuscript. BC and LL contribute equally to this study. All authors gave final approval for publication.
Funding
This work was supported by the National Natural Science Foundation (No. 31902142), the National Natural Science Foundation (No. 31572394), and the China Agriculture Research System (No. CARS-41).
Conflict of Interest
The authors declare that the research was conducted in the absence of any commercial or financial relationships that could be construed as a potential conflict of interest.
Acknowledgments
The authors would like to thank the members of the poultry breeding group at Northeast Agricultural University for help in managing the birds and collecting the data.
Supplementary Material
The Supplementary Material for this article can be found online at: https://www.frontiersin.org/articles/10.3389/fcell.2021.590468/full#supplementary-material
References
Alrayes, N., Aziz, A., Ullah, F., Ishfaq, M., Jelani, M., and Wali, A. (2020). Novel missense alteration in LRP4 gene underlies Cenani-Lenz syndactyly syndrome in a consanguineous family. J. Gene Med. 22:e3143. doi: 10.1002/jgm.3143
Bailey, T. L. (2011). DREME: motif discovery in transcription factor ChIP-seq data. Bioinformatics 27, 1653–1659. doi: 10.1093/bioinformatics/btr261
Choe, S. S., Huh, J. Y., Hwang, I. J., Kim, J. I., and Kim, J. B. (2016). Adipose tissue remodeling: its role in energy metabolism and metabolic disorders. Front. Endocrinol. 7:30. doi: 10.3389/fendo.2016.00030
Dominissini, D., Moshitch-Moshkovitz, S., Schwartz, S., Salmon-Divon, M., Ungar, L., Osenberg, S., et al. (2012). Topology of the human and mouse m6A RNA methylomes revealed by m6A-seq. Nature 485, 201–206. doi: 10.1038/nature11112
Fabre, O., Salehzada, T., Lambert, K., Seok, Y. B., Zhou, A., Mercier, J., et al. (2012). RNase L controls terminal adipocyte differentiation, lipids storage and insulin sensitivity via CHOP10 mRNA regulation. Cell Death Differ. 19, 1470–1481. doi: 10.1038/cdd.2012.23
Fan, Y., Zhang, C. S., and Zhu, G. Y. (2019). Profiling of RNA N6-methyladenosine methylation during follicle selection in chicken ovary. Poult. Sci. 98, 6117–6124. doi: 10.3382/ps/pez277
Farmer, S. R. (2006). Transcriptional control of adipocyte formation. Cell Metab. 4, 263–273. doi: 10.1016/j.cmet.2006.07.001
Gilbert, W. V., Bell, T. A., and Schaening, C. (2016). Messenger RNA modifications: form, distribution, and function. Science 352, 1408–1412. doi: 10.1126/science.aad8711
Gondret, F., Ferré, P., and Dugail, I. (2001). ADD-1/SREBP-1 is a major determinant of tissue differential lipogenic capacity in mammalian and avian species. J. Lipid Res. 42, 106–113. doi: 10.1016/S0022-2275(20)32341-5
Guo, L., Sun, B., Shang, Z., Leng, L., Wang, Y., Wang, N., et al. (2011). Comparison of adipose tissue cellularity in chicken lines divergently selected for fatness. Poult. Sci. 90, 2024–2034. doi: 10.3382/ps.2010-00863
Huang, H., Weng, H., Sun, W., Qin, X., Shi, H., Wu, H., et al. (2018). Recognition of RNA N(6)-methyladenosine by IGF2BP proteins enhances mRNA stability and translation. Nat. Cell Biol. 20, 285–295. doi: 10.1038/s41556-018-0045-z
Jiang, Q., Sun, B., Liu, Q., Cai, M., Wu, R., Wang, F., et al. (2019). MTCH2 promotes adipogenesis in intramuscular preadipocytes via an m(6)A-YTHDF1-dependent mechanism. FASEB J. 33, 2971–2981. doi: 10.1096/fj.201801393RRR
Kane, S., and Beemon, K. (1987). Inhibition of methylation at two internal N6-methyladenosine sites caused by GAC to GAU mutations. J. Biol. Chem. 262, 3422–3427. doi: 10.1016/S0021-9258(18)61520-0
Kim, J., Lee, Y. J., Kim, J. M., Lee, S. Y., Bae, M. A., Ahn, J. H., et al. (2016). PPARgamma agonists induce adipocyte differentiation by modulating the expression of Lipin-1, which acts as a PPARgamma phosphatase. Int. J. Biochem. Cell. Biol. 81, 57–66. doi: 10.1016/j.biocel.2016.10.018
Kim, S. P., Da, H., Li, Z., Kushwaha, P., Beil, C., Mei, L., et al. (2019). Lrp4 expression by adipocytes and osteoblasts differentially impacts sclerostin's endocrine effects on body composition and glucose metabolism. J. Biol. Chem. 294, 6899–6911. doi: 10.1074/jbc.RA118.006769
Kobayashi, M., Ohsugi, M., Sasako, T., Awazawa, M., Umehara, T., Iwane, A., et al. (2018). The RNA methyltransferase complex of WTAP, METTL3, and METTL14 regulates mitotic clonal expansion in adipogenesis. Mol. Cell. Biol. 38, e00116–e00118. doi: 10.1128/MCB.00116-18
Leng, L., Wang, S., Li, Z., Wang, Q., and Li, H. (2009). A polymorphism in the 3'-flanking region of insulin-like growth factor binding protein 2 gene associated with abdominal fat in chickens. Poult. Sci. 88, 938–942. doi: 10.3382/ps.2008-00453
Li, M., Sun, X., Cai, H., Sun, Y., Plath, M., Li, C., et al. (2016). Long non-coding RNA ADNCR suppresses adipogenic differentiation by targeting miR-204. Biochim. Biophys. Acta. Gene Regul. Mech. 1859, 871–882. doi: 10.1016/j.bbagrm.2016.05.003
Li, Y. L., Wang, X. L., Li, C. P., Hu, S. N., Yu, J., and Song, S. H. (2014). Transcriptome-wide N-6-methyladenosine profiling of rice callus and leaf reveals the presence of tissue-specific competitors involved in selective mRNA modification. RNA Biol. 11, 1180–1188. doi: 10.4161/rna.36281
Lin, X. Y., Chai, G. S., Wu, Y. M., Li, J. X., Chen, F., Liu, J. Z., et al. (2019). RNA m(6)A methylation regulates the epithelial mesenchymal transition of cancer cells and translation of Snail. Nat. Commun. 10:2065. doi: 10.1038/s41467-019-09865-9
Lin, Z., Hsu, P. J., Xing, X. D., Fang, J. H., Lu, Z. K., Zou, Q., et al. (2017). Mettl3-/Mettl14-mediated mRNA N-6-methyladenosine modulates murine spermatogenesis. Cell Res. 27, 1216–1230. doi: 10.1038/cr.2017.117
Liu, J. Z., Yue, Y. N., Han, D. L., Wang, X., Fu, Y., Zhang, L., et al. (2014). A METTL3-METTL14 complex mediates mammalian nuclear RNA N6-adenosine methylation. Nat. Chem. Biol. 10, 93–95. doi: 10.1038/nchembio.1432
Losko, M., Lichawska-Cieslar, A., Kulecka, M., Paziewska, A., Rumienczyk, I., Mikula, M., et al. (2018). Ectopic overexpression of MCPIP1 impairs adipogenesis by modulating microRNAs. Biochim. Biophys. Acta. Mol. Cell Res. 1865, 186–195. doi: 10.1016/j.bbamcr.2017.09.010
Lu, N., Li, X. M., Yu, J. Y., Li, Y., Wang, C., Zhang, L. L., et al. (2018). Curcumin attenuates lipopolysaccharide-induced hepatic lipid metabolism disorder by modification of m(6)A RNA methylation in piglets. Lipids 53, 53–63. doi: 10.1002/lipd.12023
Luo, G. Z., MacQueen, A., Zheng, G., Duan, H., Dore, L. C., Lu, Z., et al. (2014). Unique features of the m6A methylome in Arabidopsis thaliana. Nat. Commun. 5:5630. doi: 10.1038/ncomms6630
Luo, Z. P., Zhang, Z. W., Tai, L. N., Zhang, L. F., Sun, Z., and Zhou, L. (2019). Comprehensive analysis of differences of N-6-methyladenosine RNA methylomes between high-fat-fed and normal mouse livers. Epigenomics 11, 1267–1282. doi: 10.2217/epi-2019-0009
McGown, C., Birerdinc, A., and Younossi, Z. M. (2014). Adipose tissue as an endocrine organ. Clin. Liver Dis. 18, 41–58. doi: 10.1016/j.cld.2013.09.012
Meyer, K. D., Saletore, Y., Zumbo, P., Elemento, O., Mason, C. E., and Jaffrey, S. R. (2012). Comprehensive analysis of mRNA methylation reveals enrichment in 3'UTRs and near stop codons. Cell 149, 1635–1646. doi: 10.1016/j.cell.2012.05.003
Mihelic, R., Winter, H., Powers, J. B., Das, S., Lamour, K., Campagna, S. R., et al. (2020). Genes controlling polyunsaturated fatty acid synthesis are developmentally regulated in broiler chicks. Br. Poult. Sci. 61, 508–517. doi: 10.1080/00071668.2020.1759788
Ntambi, J. M. (1999). Regulation of stearoyl-CoA desaturase by polyunsaturated fatty acids and cholesterol. J. Lipid Res. 40, 1549–1558. doi: 10.1016/S0022-2275(20)33401-5
Perry, R. P., Kelley, D. E., Friderici, K., and Rottman, F. (1975). The methylated constituents of L cell messenger RNA: evidence for an unusual cluster at the 5' terminus. Cell 4, 387–394. doi: 10.1016/0092-8674(75)90159-2
Rondinone, C. M. (2006). Adipocyte-derived hormones, cytokines, and mediators. Endocrine 29, 81–90. doi: 10.1385/ENDO:29:1:81
Rosen, E. D., and Spiegelman, B. M. (2006). Adipocytes as regulators of energy balance and glucose homeostasis. Nature 444, 847–853. doi: 10.1038/nature05483
Ros-Freixedes, R., Gol, S., Pena, R. N., Tor, M., Ibanez-Escriche, N., Dekkers, J. C. M., et al. (2016). Genome-wide association study singles out SCD and LEPR as the two main loci influencing intramuscular fat content and fatty acid composition in duroc pigs. PLoS ONE 11:e0152496. doi: 10.1371/journal.pone.0152496
Sahakyan, K. R., Somers, V. K., Rodriguez-Escudero, J. P., Hodge, D. O., Carter, R. E., Sochor, O., et al. (2015). Normal-weight central obesity: implications for total and cardiovascular mortality. Ann. Intern. Med. 163, 827–835. doi: 10.7326/M14-2525
Sai, L. L., Li, Y., Zhang, Y. C., Zhang, J., Qu, B. P., Guo, Q. M., et al. (2020). Distinct m(6)A methylome profiles in poly(A) RNA from Xenopus laevis testis and that treated with atrazine. Chemosphere 245:125631. doi: 10.1016/j.chemosphere.2019.125631
Schaffer, J. E., and Lodish, H. F. (1994). Expression cloning and characterization of a novel adipocyte long chain fatty acid transport protein. Cell 79, 427–436. doi: 10.1016/0092-8674(94)90252-6
Shen, L., Liang, Z., Gu, X., Chen, Y., Teo, Z. W. N., Hou, X., et al. (2016). N6-methyladenosine RNA modification regulates shoot stem cell fate in Arabidopsis. Dev. Cell 38, 186–200. doi: 10.1016/j.devcel.2016.06.008
Siersbaek, R., Nielsen, R., John, S., Sung, M. H., Baek, S., Loft, A., et al. (2011). Extensive chromatin remodelling and establishment of transcription factor 'hotspots' during early adipogenesis. Embo. J. 30, 1459–1472. doi: 10.1038/emboj.2011.65
Song, Z. Y., Xiaoli, A. M., and Yang, F. J. (2018). Regulation and metabolic significance of de novo lipogenesis in adipose tissues. Nutrients 10:1383. doi: 10.3390/nu10101383
Tao, X. L., Chen, J. N., Jiang, Y. Z., Wei, Y. Y., Chen, Y., Xu, H. M., et al. (2017). Transcriptome-wide N-6-methyladenosine methylome profiling of porcine muscle and adipose tissues reveals a potential mechanism for transcriptional regulation and differential methylation pattern. BMC Genomics 18:336. doi: 10.1186/s12864-017-3719-1
Tong, F. M., Black, P. N., Coleman, R. A., and DiRusso, C. C. (2006). Fatty acid transport by vectorial acylation in mammals: roles played by different isoforms of rat long-chain acyl-CoA synthetases. Arch. Biochem. Biophys. 447, 46–52. doi: 10.1016/j.abb.2006.01.005
van Harmelen, V., Ryden, M., Sjolin, E., and Hoffstedt, J. (2007). A role of lipin in human obesity and insulin resistance: relation to adipocyte glucose transport and GLUT4 expression. J. Lipid Res. 48, 201–206. doi: 10.1194/jlr.M600272-JLR200
Wan, Y. Z., Tang, K., Zhang, D. Y., Xie, S. J., Zhu, X. H., Wang, Z. G., et al. (2015). Transcriptome-wide high-throughput deep m(6)A-seq reveals unique differential m(6)A methylation patterns between three organs in Arabidopsis thaliana. Genome Biol. 16:272. doi: 10.1186/s13059-015-0839-2
Wang, L. F., Jin, Q. H., Lee, J. E., Su, I. H., and Ge, K. (2010). Histone H3K27 methyltransferase Ezh2 represses Wnt genes to facilitate adipogenesis. Proc. Natl. Acad. Sci. U. S. A. 107, 7317–7322. doi: 10.1073/pnas.1000031107
Wang, X., Sun, B., Jiang, Q., Wu, R., Cai, M., Yao, Y., et al. (2018). mRNA m(6)A plays opposite role in regulating UCP2 and PNPLA2 protein expression in adipocytes. Int. J. Obes. 42, 1912–1924. doi: 10.1038/s41366-018-0027-z
Wu, W. C., Feng, J. E., Jiang, D. H., Zhou, X. H., Jiang, Q., Cai, M., et al. (2017). AMPK regulates lipid accumulation in skeletal muscle cells through FTO-dependent demethylation of N-6-methyladenosine. Sci. Rep. 7:41606. doi: 10.1038/srep41606
Yang, Y., Hsu, P. J., Chen, Y. S., and Yang, Y. G. (2018). Dynamic transcriptomic m(6)A decoration: writers, erasers, readers and functions in RNA metabolism. Cell Res. 28, 616–624. doi: 10.1038/s41422-018-0040-8
Yang, Y., Wang, L., Han, X., Yang, W. L., Zhang, M. M., Ma, H. L., et al. (2019). RNA 5-methylcytosine facilitates the maternal-to-zygotic transition by preventing maternal mRNA decay. Mol. Cell 75:1188. doi: 10.1016/j.molcel.2019.06.033
Yu, Y. Y., Qiao, S. P., Sun, Y. N., Song, H., Zhang, X. F., Yan, X. H., et al. (2014). Identification and analysis of a functional SNP 1196C>A in 3′UTR of chicken IGFBP2 gene. Prog. Biochem. Biophys. 41, 1163–1172. doi: 10.3724/SP.J.1206.2014.00062
Yue, Y., Liu, J., and He, C. (2015). RNA N6-methyladenosine methylation in post-transcriptional gene expression regulation. Genes Dev. 29, 1343–1355. doi: 10.1101/gad.262766.115
Yue, Y. A., Liu, J., Cui, X. L., Cao, J., Luo, G. Z., Zhang, Z. Z., et al. (2018). VIRMA mediates preferential m(6)A mRNA methylation in 3'UTR and near stop codon and associates with alternative polyadenylation. Cell Discov. 4:10. doi: 10.1038/s41421-018-0019-0
Zhang, H., Shi, X. R., Huang, T., Zhao, X. N., Chen, W. Y., Gu, N. N., et al. (2020). Dynamic landscape and evolution of m6A methylation in human. Nucleic Acids Res. 48, 6251–6264. doi: 10.1093/nar/gkaa347
Zhang, K., Cheng, B. H., Yang, L. L., Wang, Z. P., Zhang, H. L., Xu, S. S., et al. (2017). Identification of a potential functional single nucleotide polymorphism for fatness and growth traits in the 3'-untranslated region of the PCSK1 gene in chickens. J. Anim. Sci. 95, 4776–4786. doi: 10.2527/jas2017.1706
Zhang, M. Z., Zhang, Y., Ma, J., Guo, F. M., Cao, Q., Zhang, Y., et al. (2015). The demethylase activity of FTO (Fat Mass and Obesity Associated Protein) is required for preadipocyte differentiation. PLoS ONE 10:e0133788. doi: 10.1371/journal.pone.0133788
Zhang, X. L., Gu, H. F., Frystyk, J., Efendic, S., Brismar, K., and Thorell, A. (2019). Analyses of IGFBP2 DNA methylation and mRNA expression in visceral and subcutaneous adipose tissues of obese subjects. Growth Horm. IGF Res. 45, 31–36. doi: 10.1016/j.ghir.2019.03.002
Zhang, X. Y., Wu, M. Q., Wang, S. Z., Zhang, H., Du, Z. Q., Li, Y. M., et al. (2018). Genetic selection on abdominal fat content alters the reproductive performance of broilers. Animal 12, 1232–1241. doi: 10.1017/S1751731117002658
Zhang, Y., Liu, T., Meyer, C. A., Eeckhoute, J., Johnson, D. S., Bernstein, B. E., et al. (2008). Model-based Analysis of ChIP-Seq (MACS). Genome Biol. 9:R137. doi: 10.1186/gb-2008-9-9-r137
Zhao, X., Yang, Y., Sun, B. F., Shi, Y., Yang, X., Xiao, W., et al. (2014). FTO-dependent demethylation of N6-methyladenosine regulates mRNA splicing and is required for adipogenesis. Cell Res. 24, 1403–1419. doi: 10.1038/cr.2014.151
Zhao, Y. S., Zhao, Q. J., Kaboli, P. J., Shen, J., Li, M. X., Wu, X., et al. (2019). m1A regulated genes modulate PI3K/AKT/mTOR and ErbB pathways in gastrointestinal cancer. Transl. Oncol. 12, 1323–1333. doi: 10.1016/j.tranon.2019.06.007
Zhou, H., Deeb, N., Evock-Clover, C. M., Ashwell, C. M., and Lamont, S. J. (2006). Genome-wide linkage analysis to identify chromosomal regions affecting phenotypic traits in the chicken. II. Body composition. Poult. Sci. 85, 1712–1721. doi: 10.1093/ps/85.10.1712
Zhu, J. G., Xia, L., Ji, C. B., Zhang, C. M., Zhu, G. Z., Shi, C. M., et al. (2012). Differential DNA methylation status between human preadipocytes and mature adipocytes. Cell Biochem. Biophys. 63, 1–15. doi: 10.1007/s12013-012-9336-3
Zhu, T. T., Roundtree, I. A., Wang, P., Wang, X., Wang, L., Sun, C., et al. (2014). Crystal structure of the YTH domain of YTHDF2 reveals mechanism for recognition of N6-methyladenosine. Cell Res. 24, 1493–1496. doi: 10.1038/cr.2014.152
Keywords: chicken, fat deposition, adipose tissue, N6-methyladenosine, MeRIP-seq
Citation: Cheng B, Leng L, Li Z, Wang W, Jing Y, Li Y, Wang N, Li H and Wang S (2021) Profiling of RNA N6-Methyladenosine Methylation Reveals the Critical Role of m6A in Chicken Adipose Deposition. Front. Cell Dev. Biol. 9:590468. doi: 10.3389/fcell.2021.590468
Received: 01 August 2020; Accepted: 18 January 2021;
Published: 05 February 2021.
Edited by:
Xiao Han, Chinese Academy of Agricultural Sciences, ChinaReviewed by:
Xuemei Deng, China Agricultural University, ChinaYing Wang, University of California, Davis, United States
Copyright © 2021 Cheng, Leng, Li, Wang, Jing, Li, Wang, Li and Wang. This is an open-access article distributed under the terms of the Creative Commons Attribution License (CC BY). The use, distribution or reproduction in other forums is permitted, provided the original author(s) and the copyright owner(s) are credited and that the original publication in this journal is cited, in accordance with accepted academic practice. No use, distribution or reproduction is permitted which does not comply with these terms.
*Correspondence: Shouzhi Wang, shouzhiwang@neau.edu.cn
†These authors have contributed equally to this work