- 1Department of Stomatology and Dental Hygiene, The Fourth Affiliated Hospital, Harbin Medical University, Harbin, China
- 2General Hospital of Heilongjiang Province Land Reclamation Bureau, Harbin, China
- 3Department of Endocrinology, The First Affiliated Hospital of Harbin Medical University, Harbin, China
- 4School of Life Science and Technology, Harbin Institute of Technology, Harbin, China
Periodontal diseases are among the most frequent inflammatory diseases affecting children and adolescents, which affect the supporting structures of the teeth and lead to tooth loss and contribute to systemic inflammation. Gingivitis is the most common periodontal infection. Gingivitis, which is mainly caused by a substance produced by microbial plaque, systemic disorders, and genetic abnormalities in the host. Identifying gingivitis-related genes across human tissues is not only significant for understanding disease mechanisms but also disease development and clinical diagnosis. The Genome-wide association study (GWAS) a commonly used method to mine disease-related genetic variants. However, due to some factors such as linkage disequilibrium, it is difficult for GWAS to identify genes directly related to the disease. Hence, we constructed a data integration method that uses the Summary Mendelian randomization (SMR) to combine the GWAS with expression quantitative trait locus (eQTL) data to identify gingivitis-related genes. Five eQTL studies from different human tissues and one GWAS studies were referenced in this paper. This study identified several candidates SNPs and genes relate to gingivitis in tissue-specific or cross-tissue. Further, we also analyzed and explained the functions of these genes. The R program for the SMR method has been uploaded to GitHub(https://github.com/hxdde/SMR).
Introduction
Gingivitis is the most prevalent disease of the periodontium (Oliver et al., 1998) and is commonly known to be a site-specific inflammatory disease caused by the accumulation of dental biofilm (Loe et al., 1965; Theilade et al., 1966; Trombelli et al., 2004). Bacteria in the mouth from gingivitis can easily get into the bloodstream and cause damage to organs. The list of potential problems this bacteria can cause is long. Periodontitis may lead to an increased risk of stroke, heart attack, heart disease, dementia, rheumatoid arthritis, and lung disease (Humphrey et al., 2008; Jamieson et al., 2015). Studies have shown that the prevalence and severity of gingivitis are high (Oh et al., 2002). More than 70% of children older than 7 years old are affected by gingivitis (Stamm, 1986). The clinical symptoms of gingivitis are redness and edema of the gingival tissue, bleeding on provocation, changes in contour and consistency, occurrence of calculus and/or plaque, and lead to tooth loss and contribute to systemic inflammation (No authors listed, 2000; Kinane et al., 2017). Although The pathogenic factors of gingivitis are mainly related to the microbial biofilm of the dental plaque, hormonal fluctuations, drugs, malnutrition, and system disease, the genetic variation, and epigenetic program determine the susceptibility and the regulatory capacity for plaque pathogens (Kinane et al., 2017; Murakami et al., 2018; Zhang S. et al., 2020). Therefore, identify gingivitis-related genes and loci can elucidate disease mechanisms and guide clinical diagnosis and treatment.
Over the first decade of the twenty first century, with the maturity of high-throughput sequencing technology, a large amount of genomic data provides an important platform for researchers to discover abnormal genes related to diseases, understand disease mechanisms, and develop treatment methods (Zhao and Grant, 2011; Zou and Ma, 2019; Wang L. et al., 2019; Cheng et al., 2020). In recent years, several institutions and companies have discovered gingivitis-related pathways and susceptibility genes. For example, polymorphisms in the interleukin-1 gene cluster can influence the severity of gingivitis (Parkhill et al., 2000; Papapanou et al., 2001). Through transcriptome analysis of patients with gingivitis and healthy non-smokers, Demmer et al. (2008) identified 61 differentially expressed genes and function enrichment analysis show these are significantly related to apoptosis, antimicrobial humoral response, antigen presentation, regulation of metabolic processes, signal transduction, and angiogenesis. Kim et al. (2016) also identified 400 up-regulated genes and 62 down-regulated genes which mainly related to defense/immunity protein, receptor, protease, signaling molecules, cytoskeletal, and structural proteins by transcriptome sequencing of gingival biopsies. However, the current research is mainly to identify gingivitis-related genes through biological experiments or simple difference analysis. The major weakness with these study is that it does not incorporate more biological information and describe the disease in a single tissue, which makes it difficult to identify key disease-causing genes from thousands of genes. How to integrate more biological information across multiple tissues has become a research hotspot and challenge (Lewin et al., 2016).
The Genome-wide association study (GWAS) is an observational study that detects the single nucleotide polymorphisms (SNP) of multiple individuals of a specific species to find genetic variations associated with a particular trait (Li et al., 2015; Liu and Jiang, 2016; Jiang et al., 2017; Bush, 2019; Cheng et al., 2019b; Sun et al., 2019). In recent years, there have been many studies that have identified several risk genes associated with gingivitis through the GWAS analysis. The GWAS study of 4,910 European-American adults shows that high IL-1β and IL-6 expression is associated with IL37 locus variant, which induces more severe periodontal disease (Offenbacher et al., 2018). The genetic variation of ASIC2 (acid-sensing ionic channel 2) locus, which locates chromosome 17, is significantly associated with severe gingivitis (Zhang et al., 2016). While GSWA can effectively identify disease-related gene loci, there are still many limitations and problems. The GWAS can determine the locus related to the trait or disease instead of directly determining the gene itself. Due to the hypothesis of “disease-common variations,” it is difficult for GWAS to identify rare variants. And these rare variants may be an important role in the disease process (Liu et al., 2016, 2018; Hu et al., 2018). Besides, GWAS only gives statistical conclusions on genetic variants and phenotype, and there is no information on gene function studies. Therefore, GWAS cannot fully reveal the abnormal genetic loci of complex disease. How to accurately identify the genetic variant directly related to the disease and obtain these changed biological functions is a major challenge.
At present, a large number of GWAS studies have found that 80% of genetic variation sites are located in non-coding regions of the genome. At present, a large number of GWAS studies have found that 80% of genetic susceptibility sites are located in non-coding regions of the genome, which indicates that some pathogenic genetic sites may have the ability to regulate gene expression (Hu et al., 2018; Cheng et al., 2019a). The expression quantitative trait loci (eQTL) mapping analysis takes the expression level of genes as quantitative traits and uses traditional OTL methods to identify genetic sites that can regulate gene expression. Traditional QTL methods need to measure the genotype and gene expression level of each individual studied, and then use association analysis (outbred population) or linkage analysis (family or experimental hybrid population) to assess the association between genotype and gene expression level (Rockman and Kruglyak, 2006; Skelly et al., 2009; Albert and Kruglyak, 2015).
The Summary Mendelian Randomization (SMR) is a transcriptome-wide association analysis method that integrates summary-level data from independent GWAS with data from eQTL studies to identify genes whose expression levels are associated with a complex trait (Zhu et al., 2016). The statistical performance of the SMR method will increase with a higher research sample size and it can provide a test to distinguish the causal relationship between the genetic variant and gene expression (Pavlides et al., 2016). Since Zhu et al. (2016) first proposed the SMR method, considerable literature has grown up around the SMR method to predicts complex trait gene targets. Hu et al. (2018) used the SMR method to integrate 2 GWAS datasets and 5 eQTL datasets to identify27 SNPs related to Alzheimer’s disease. Meng et al. (2018) collected the largest GWAS and eQTL meta-analysis data and tested 5,967 genes through the SMR method, which identified two potentially causal genes (ASB16-AS1 and SYN2) associated with bone mineral density. In the study of complex collisions, Porcu et al. (2019) found 36% of genes have no genome-wide significant SNP nearby in previous GWAS by applying the SMR method for 43 human phenotypes and they think that the majority of these loci were missed by GWAS due to power issues. Veturi’s research also believes that the SMR method has excellent capabilities under the assumption of causality (Veturi and Ritchie, 2018; Cheng, 2019). Hence to obtain more accurate results, we used the SMR method to integrate GWAS and eQTL studies to identify genes related to gingivitis.
Materials and Methods
Data Collection
Reliable data is the key to further analysis (Liang et al., 2017; Zhang et al., 2017). We downloaded one GWAS data related to gingivitis from the GWAS Catalog database (The NHGRI-EBI Catalog of human genome-wide association studies1). Five eQTL studies data, which are five different tissues of patients with gingivitis, are downloaded from the GTEx database (Genotype-Tissue Expression2). The detailed data information has been shown in Table 1.
The Summary Mendelian Randomization Method
Some biological experiments have found that if a genetic variant affects the expression level of a gene, then the gene will have different expression levels among samples who carry different genotypes of the genetic variant (Weiss et al., 2008; McCarthy et al., 2009). In addition, if the gene can also affect the phenotype, the phenotype will be different in different genotypes (Golzio et al., 2012). This process is very similar to the theory of Mendelian randomization (MR) (Katan, 1986; Smith and Ebrahim, 2003). However, the current sample size of phenotype, SNP, and gene expression data cannot meet the needs of MR analysis. For this, we use the SMR method which can integrate summary-level data from independent GWAS with data from eQTL studies to identify genes whose expression levels are associated with a disease phenotype because of pleiotropy (Zhu et al., 2016).
Herein, we let Y be a disease phenotype (outcome), X be gene expression level (exposure), and Z be a genetic variant (instrumental variable). Then, the effect of gene expression on disease phenotype bXY is bXY = bZY/bZX, where bZY is the effect of the SNP effect on disease phenotype, bZX is the effect of the SNP effect on gene expression. The workflow is shown in Figure 1.
Results
To fully elucidate the gene abnormality across the tissues of patients with gingivitis, we downloaded 5 eQTL data from five different organizations (artery, blood, fibroblast, nerve, and skin) and 1 GWAS data (Table 1). The SMR method was used to integrate these data sets to obtain 5 experimental results. For these 5 experiments, we identified a total of 26 SNP-Gene pairs that are significantly associated with gingivitis (Table 2). In the eQTL data, multiple probes can label one SNP, which leads to one SNP that can be repeatedly selected to be associated with gingivitis in 5 experiments. Therefore, we counted the number of times a significant SNP was screened. Looking at Figure 2, in 5 experiments, most SNPs were selected more than four times and only two SNPs were selected once. This result indicates that SNP can be accurately selected in our method.
Functional Analysis of Gingivitis-Related Genes
For the gingivitis-related genes identified by the SMR method, the GO database was used for functional annotation. As shown in Figure 3, these genes are significantly annotated into 12 biological processes and 8 molecular functions.
The top two biological functions are all related to formaldehyde metabolism. A considerable amount of literature has been published on formaldehyde may be a causative factor of gingivitis. The study by Wantke et al. (2000) found that exposure to formaldehyde can induce the production of specific IgE and the research subjects have symptoms such as gingival bleeding, oral or pharyngeal itch. Tokar et al. (2020) test on the oral condition of woodworkers who have long-term contact with formaldehyde showed that exposure to formaldehyde has significant side effects on periodontal diseases such as gingivitis. In addition, there are six biological processes related to the metabolism of ethanol and aldehyde. Several lines of evidence suggest that ethanol and aldehyde both ethanol and aldehyde can damage the oral cavity and induce gingivitis and periodontal disease (Barczynski et al., 1987; Dong et al., 1996; Wyganowska-Świątkowska et al., 2018) and in severe cases, ethanol can even cause oral cancer (Calderón-Montaño et al., 2018). Both alcohols and aldehydes belong to the oxygen-containing derivatives of terpenoids. In our results, the two biological processes related to the terpenoids are also abnormal. Therefore, we infer that the metabolic disorders of alcohol and aldehydes in patients with gingivitis may be related to the occurrence and severity of gingivitis.
The remaining two biological processes are fatty acid omega-oxidation and retinoid metabolic process. Abnormal function of fatty acid omega-oxidation will hinder the metabolism and absorption of fatty acids. However, studies have found that some fatty acids have anti-inflammatory and antimicrobial effects to treat gingivitis (Peedikayil et al., 2015; Woelber et al., 2019). Vitamin A and its analogs have many physiological functions such as promoting growth and reproduction, maintaining bones, epithelial tissue, vision, and normal secretion of the mucosal epithelium (Chapman, 2012). When vitamin A is deficient, the mucosal barriers caused by infection cannot be repaired and the innate immunity is destroyed. At the same time, vitamin A deficiency can also reduce the adaptive immune response mediated by Th2 cells (Stephensen, 2001). Studies have found that the damage to mucosal epithelial regeneration and changes in immune function caused by vitamin A deficiency is important to the occurrence and recovery of periodontal diseases (Cutress et al., 1976; Dommisch et al., 2018). Currently, retinoid medication has been used for the treatment of gingivitis and periodontal disease (Lundgren et al., 1996; Epstein and Gorsky, 1999).
Similar to the enrichment results of biological processes, KEGG pathway enrichment results also indicate that gingivitis-related genes are mainly involved in tyrosine metabolism, fatty acid degradation, retinol metabolism and Glycolysis/Gluconeogenesis, etc. (Figure 4). The molecular functions of gingivitis-related genes are mainly related to the activity of S-(hydroxymethyl)glutathione dehydrogenase, alcohol dehydrogenase, retinol dehydrogenase, and oxidoreductase (Figure 3). This indicates that these biological enzymes play an important role in gingivitis and may be potential therapeutic targets.
Tissue Related Genes in Gingivitis
As shown in Figure 5A, only two genes FAM86C1 and FYCO1 occur simultaneously in 4 tissues. They are related to protein methylation, metabolism of proteins and inflammation, the microtubule transport of autophagosomes, respectively (Cloutier et al., 2013; Cheng et al., 2016). The Venn diagram of the distribution of gingivitis-related genes in five tissues shows that the expression of gingivitis-related genes has obvious tissue specificity (Figure 5B). The genes MYT1L and ADH6 are related to arterial tissue; The genes ZNF705E and MRPL48 are related to skin tissues; The genes CCR1, ADH4, and CITF22-92A6.2 are related to fibroblast; The genes NUMA1, RP11-493L12.6, and EVI2A are related to blood. The ADH4 as a member of the alcohol dehydrogenase family metabolizes a wide variety of substrates, including ethanol, retinol, other aliphatic alcohols, hydroxysteroids, and lipid peroxidation products (Tokar et al., 2020). It is worth noting that studies have found that alcohol has an irreversible effect on human gingival fibroblasts (Wyganowska-Świątkowska et al., 2018). In addition, some studies have found that the expression level of CCR1 tends to change in the gingival fibroblast (Candotto et al., 2019; Lauritano et al., 2019).
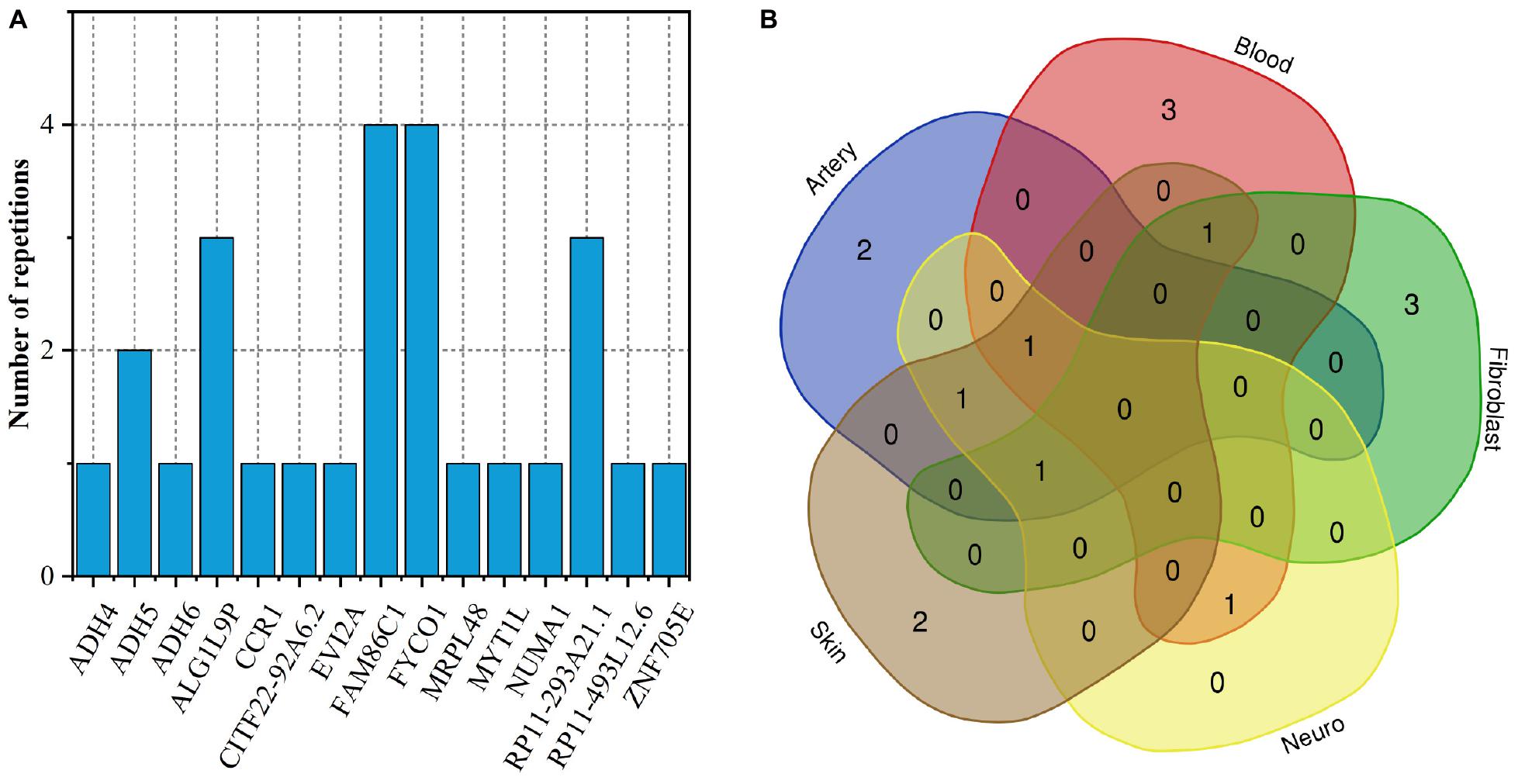
Figure 5. (A) The number of duplicate genes in five tissues; (B) Venn diagram of gene distribution in tissues.
We use the Database for Annotation, Visualization and Integrated Discovery (DAVID) v6.8 tool3 to enrich gingivitis-related genes into the functional set. As shown in Figure 6, apart from the alcohol dehydrogenase family (ADH4, ADH5, and ADH6), other genes have smaller functional overlaps.
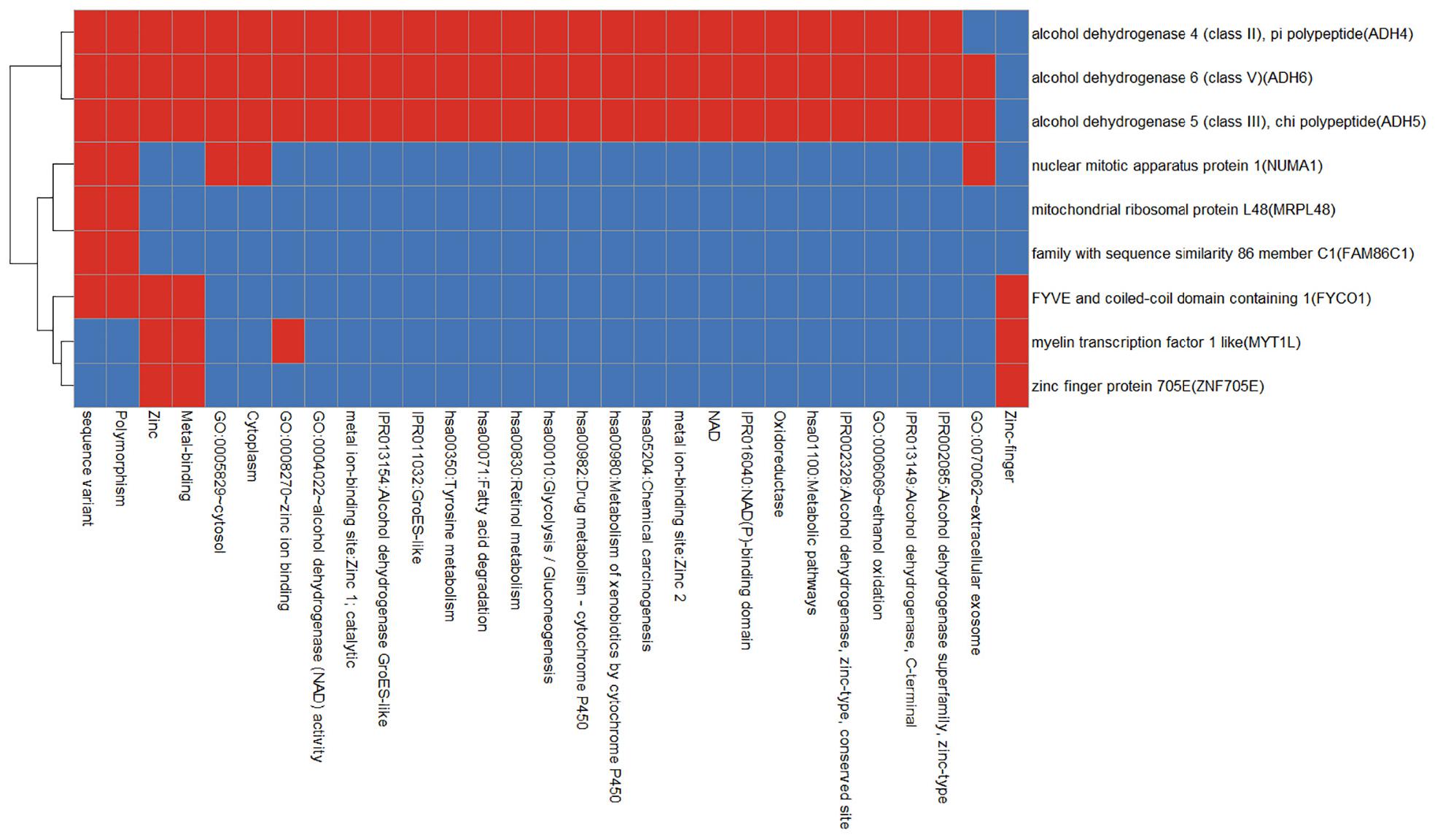
Figure 6. Functional annotation heat map of gingivitis related genes. Red means corresponding gene-term association positively reported and blue means corresponding gene-term association not reported yet.
Genes Associated With Gingivitis and Other Diseases
Gingivitis is common chronic inflammation and recent research has suggested it play an important role in the occurrence and development of some systemic diseases (Humphrey et al., 2008; Shah et al., 2010; Hou et al., 2019; Lombardi et al., 2019; Goodson, 2020). We found that some gingivitis-related genes can mediate multiple diseases at the same time.
MYT1L (myelin transcription factor 1 like) encodes a member of the zinc finger superfamily of transcription factors. MYT1L mutation can lead to intellectual disability and obesity (Blanchet et al., 2017; Loid et al., 2018). Research finds gingivitis and obesity exhibit disease reciprocity and gingivitis is more prevalent in obesity (Dursun et al., 2016; Goodson, 2020). CCR1 can regulate the transduction of immune signals and affect the recruitment of effector immune cells to the site of inflammation (Foroughi et al., 2016). CCR1 has an important role in the occurrence of chronic inflammation of gingivitis (Silva et al., 2005). At the same time, it is a target for multiple myeloma and kidney diseases (Ninichuk and Anders, 2005; Vallet and Anderson, 2011). In addition, early diagnosis and treatment of gingivitis can effectively improve the survival expectations of primary liver cancer (Hou et al., 2019). And among genes related to gingivitis, ADH4 and FAM86C1 may be potential prognostic and diagnostic markers of liver cancer (Wei et al., 2012; Wang X. et al., 2019).
These abnormal genes in multiple diseases indicate the connection between gingivitis and other systemic diseases, but also predict the mechanism of gingivitis inducing other diseases.
Conclusion
Gingivitis is a common periodontal disease and inflammation. Gingivitis is mainly caused by a substance produced by microbial plaque, systemic disorders, and genetic abnormalities in the host. Bacteria that infect the human oral can easily get into the bloodstream and cause damage to organs and may lead to systemic disorders and an increased risk of stroke, heart attack, heart disease, dementia, rheumatoid arthritis, and lung disease. Discovering abnormal genes related to gingivitis is important for understanding the disease mechanism, early diagnosis, and treatment of the disease.
Herein, we used the SMR method to integrates summary-level data from independent GWAS with data from eQTL studies to identify gingivitis-related genes. One GWAS dataset and 5 different eQTL datasets from organizations are combined into 5 experiments. In total, we identified 26 SNP-Gene pairs that are related to gingivitis in different tissues. Through GO function enrichment analysis, gingivitis-related genes were enriched into 12 biological processes and 8 molecular functions. A number of studies have confirmed that the functions and genes we discovered are indeed related to the occurrence, development, and treatment of gingivitis and periodontal diseases. These prove the reliability of our results and the accuracy of the method. Besides, we also present gingivitis-related biological enzymes that can be used as potential therapeutic targets and tissue-specific gingivitis-related genes which guide further research on gingivitis on systemic disorders. Machine learning (Zou et al., 2018; Qu et al., 2019; Zou, 2019; Dao et al., 2020; Zhang Z.M. et al., 2020; Zhao et al., 2020) and big data mining will also help in-depth mining biological knowledge.
Data Availability Statement
The datasets presented in this study can be found in online repositories. The names of the repository/repositories and accession number(s) can be found in the article/supplementary material.
Author Contributions
JZ and MS wrote the manuscript and did the experiments. YH provided ideas of this work. GG and YZ revised this manuscript and guided how to do experiments. YH supervised this work. All authors contributed to the article and approved the submitted version.
Funding
This work was supported by the National Key R&D Program of China (2017YFC1201201, 2018YFC0910504, and 2017YFC0907503), the Natural Science Foundation of China (61801147 and 82003553), and Heilongjiang Postdoctoral Science Foundation (LBH-Z6064).
Conflict of Interest
The authors declare that the research was conducted in the absence of any commercial or financial relationships that could be construed as a potential conflict of interest.
Footnotes
- ^ https://www.ebi.ac.uk/gwas/
- ^ http://commonfund.nih.gov/GTEx/
- ^ https://david.ncifcrf.gov/home.jsp
References
Albert, F. W., and Kruglyak, L. (2015). The role of regulatory variation in complex traits and disease. Nat. Rev. Genet. 16, 197–212. doi: 10.1038/nrg3891
Barczynski, J. L., Fletcher, R. D., Segal, A. H., and Conway, J. C. (1987). Viadent, ethanol, and pH effects upon gingival epithelial-like cells, in vitro. J. Periodontol. 58, 622–627. doi: 10.1902/jop.1987.58.9.622
Blanchet, P., Bebin, M., Bruet, S., Cooper, G. M., Thompson, M. L., Duban-Bedu, B., et al. (2017). MYT1L mutations cause intellectual disability and variable obesity by dysregulating gene expression and development of the neuroendocrine hypothalamus. PLoS Genet. 13:e1006957. doi: 10.1371/journal.pgen.1006957
Bush, W. S. (2019). “Genome-wide association studies,” in Encyclopedia of Bioinformatics and Computational Biology, eds S. Ranganathan, M. Gribskov, K. Nakai, and C. Schönbach (Oxford: Academic Press), 235–241.
Calderón-Montaño, J. M., Jiménez-Alonso, J. J., Guillén-Mancina, E., Burgos-Morón, E., and López-Lázaro, M. (2018). A 30-s exposure to ethanol 20% is cytotoxic to human keratinocytes: possible mechanistic link between alcohol-containing mouthwashes and oral cancer. Clin. Oral Invest. 22, 2943–2946. doi: 10.1007/s00784-018-2602-z
Candotto, V., Pezzetti, F., Baj, A., Beltramini, G., Lauritano, D., Di Girolamo, M., et al. (2019). Phenytoin and gingival mucosa: a molecular investigation. Int. J. Immunopathol. Pharmacol. 33:2058738419828259.
Chapman, M. S. (2012). Vitamin a: history, current uses, and controversies. Semin. Cutan. Med. Surg. 31, 11–16. doi: 10.1016/j.sder.2011.11.009
Cheng, L. (2019). Computational and biological methods for gene therapy. Curr. Gene Ther. 19, 210–210. doi: 10.2174/156652321904191022113307
Cheng, L., Qi, C., Zhuang, H., Fu, T., and Zhang, X. (2020). gutMDisorder: a comprehensive database for dysbiosis of the gut microbiota in disorders and interventions. Nucleic Acids Res. 48, D554–D560.
Cheng, L., Zhao, H., Wang, P., Zhou, W., Luo, M., Li, T., et al. (2019a). Computational methods for identifying similar diseases. Mol. Ther. Nucleic Acids 18, 590–604. doi: 10.1016/j.omtn.2019.09.019
Cheng, L., Zhuang, H., Ju, H., Yang, S., Han, J., Tan, R., et al. (2019b). Exposing the causal effect of body mass index on the risk of type 2 diabetes mellitus: a mendelian randomization study. Front. Genet. 10:94. doi: 10.3389/fgene.2019.00094
Cheng, X., Wang, Y., Gong, Y., Li, F., Guo, Y., Hu, S., et al. (2016). Structural basis of FYCO1 and MAP1LC3A interaction reveals a novel binding mode for Atg8-family proteins. Autophagy 12, 1330–1339. doi: 10.1080/15548627.2016.1185590
Cloutier, P., Lavallée-Adam, M., Faubert, D., Blanchette, M., and Coulombe, B. (2013). A newly uncovered group of distantly related lysine methyltransferases preferentially interact with molecular chaperones to regulate their activity. PLoS Genet. 9:e1003210. doi: 10.1371/journal.pgen.1003210
Cutress, T. W., Mickleson, K. N., and Brown, R. H. (1976). Vitamin A absorption and periodontal disease in trisomy G. J. Ment. Defic. Res. 20, 17–23. doi: 10.1111/j.1365-2788.1976.tb00012.x
Dao, F. Y., Lv, H., Zulfiqar, H., Yang, H., Su, W., Gao, H., et al. (2020). A computational platform to identify origins of replication sites in eukaryotes. Brief. Bioinform. doi: 10.1093/bib/bbaa017
CrossRef Full Text Online ahead of print | PubMed Abstract | Google Scholar
Demmer, R. T., Behle, J. H., Wolf, D. L., Handfield, M., Kebschull, M., Celenti, R., et al. (2008). Transcriptomes in healthy and diseased gingival tissues. J. Periodontol. 79, 2112–2124. doi: 10.1902/jop.2008.080139
Dommisch, H., Kuzmanova, D., Jönsson, D., Grant, M., and Chapple, I. (2018). Effect of micronutrient malnutrition on periodontal disease and periodontal therapy. Periodontology 2000 78, 129–153. doi: 10.1111/prd.12233
Dong, Y. J., Peng, T. K., and Yin, S. J. (1996). Expression and activities of class IV alcohol dehydrogenase and class III aldehyde dehydrogenase in human mouth. Alcohol 13, 257–262. doi: 10.1016/0741-8329(95)02052-7
Dursun, E., Akalin, F. A., Genc, T., Cinar, N., Erel, O., and Yildiz, B. O. (2016). Oxidative stress and periodontal disease in obesity. Medicine 95:e3136. doi: 10.1097/md.0000000000003136
Epstein, J. B., and Gorsky, M. (1999). Topical application of vitamin A to oral leukoplakia: a clinical case series. Cancer 86, 921–927. doi: 10.1002/(sici)1097-0142(19990915)86:6<921::aid-cncr5>3.0.co;2-6
Foroughi, F., Amirzargar, A., Ahmadpoor, P., Noorbakhsh, F., Nafar, M., Yekaninejad, M. S., et al. (2016). Increased levels of CD4(+) and CD8(+) T cells expressing CCR1 in patients developing allograft dysfunction; a cohort study. Transpl. Immunol. 38, 67–74. doi: 10.1016/j.trim.2016.05.003
Golzio, C., Willer, J., Talkowski, M. E., Oh, E. C., Taniguchi, Y., Jacquemont, S., et al. (2012). KCTD13 is a major driver of mirrored neuroanatomical phenotypes of the 16p11.2 copy number variant. Nature 485, 363–367. doi: 10.1038/nature11091
Goodson, J. M. (2020). Disease reciprocity between gingivitis and obesity. J. Periodontol. 91(Suppl. 1), S26–S34.
Hou, Y., Deng, W., Deng, G., Hu, L., Liu, C., and Xu, L. (2019). Gingival metastasis from primary hepatocellular carcinoma: a case report and literature review of 30 cases. BMC Cancer 19:925. doi: 10.1186/s12885-019-6020-7
Hu, Y., Zhao, T., Zang, T., Zhang, Y., and Cheng, L. (2018). Identification of Alzheimer’s disease-related genes based on data integration method. Front. Genet. 9:703. doi: 10.3389/fgene.2018.00703
Humphrey, L. L., Fu, R., Buckley, D. I., Freeman, M., and Helfand, M. (2008). Periodontal disease and coronary heart disease incidence: a systematic review and meta-analysis. J. Gen. Intern. Med. 23, 2079–2086. doi: 10.1007/s11606-008-0787-6
Jamieson, L., Skilton, M., Maple-Brown, L., Kapellas, K., Askie, L., Hughes, J., et al. (2015). Periodontal disease and chronic kidney disease among Aboriginal adults; an RCT. BMC Nephrol. 16:181. doi: 10.1186/s12882-015-0169-3
Jiang, Q., Jin, S., Jiang, Y., Liao, M., Feng, R., Zhang, L., et al. (2017). Alzheimer’s disease variants with the genome-wide significance are significantly enriched in immune pathways and active in immune cells. Mol. Neurobiol. 54, 594–600. doi: 10.1007/s12035-015-9670-8
Katan, M. B. (1986). Apolipoprotein E isoforms, serum cholesterol, and cancer. Lancet 1, 507–508. doi: 10.1016/s0140-6736(86)92972-7
Kim, Y. G., Kim, M., Kang, J. H., Kim, H. J., Park, J. W., Lee, J. M., et al. (2016). Transcriptome sequencing of gingival biopsies from chronic periodontitis patients reveals novel gene expression and splicing patterns. Hum. Genomics 10:28.
Kinane, D. F., Stathopoulou, P. G., and Papapanou, P. N. (2017). Periodontal diseases. Nat. Rev. Dis. Primers 3:17038.
Lauritano, D., Martinelli, M., Baj, A., Beltramini, G., Candotto, V., Ruggiero, F., et al. (2019). Drug-induced gingival hyperplasia: an in vitro study using amlodipine and human gingival fibroblasts. Int. J. Immunopathol. Pharmacol. 33:2058738419827746.
Lewin, A., Saadi, H., Peters, J. E., Moreno-Moral, A., Lee, J. C., Smith, K. G., et al. (2016). MT-HESS: an efficient Bayesian approach for simultaneous association detection in OMICS datasets, with application to eQTL mapping in multiple tissues. Bioinformatics 32, 523–532. doi: 10.1093/bioinformatics/btv568
Li, P., Guo, M., Wang, C., Liu, X., and Zou, Q. (2015). An overview of SNP interactions in genome-wide association studies. Brief. Funct. Genomics 14, 143–155. doi: 10.1093/bfgp/elu036
Liang, Z. Y., Lai, H. Y., Yang, H., Zhang, C. J., Yang, H., Wei, H. H., et al. (2017). Pro54DB: a database for experimentally verified sigma-54 promoters. Bioinformatics 33, 467–469.
Liu, G., and Jiang, Q. (2016). Alzheimer’s disease CD33 rs3865444 variant does not contribute to cognitive performance. Proc. Natl. Acad. Sci. U.S.A. 113, E1589–E1590.
Liu, G., Wang, T., Tian, R., Hu, Y., Han, Z., Wang, P., et al. (2018). Alzheimer’s disease risk variant rs2373115 regulates GAB2 and NARS2 expression in human brain tissues. J. Mol. Neurosci. 66, 37–43. doi: 10.1007/s12031-018-1144-9
Liu, G., Xu, Y., Jiang, Y., Zhang, L., Feng, R., and Jiang, Q. (2016). PICALM rs3851179 variant confers susceptibility to Alzheimer’s disease in chinese population. Mol. Neurobiol. 54, 3131–3136. doi: 10.1007/s12035-016-9886-2
Loe, H., Theilade, E., and Jensen, S. B. (1965). Experimental gingivitis in man. J. Periodontol. 36, 177–187. doi: 10.1902/jop.1965.36.3.177
Loid, P., Mäkitie, R., Costantini, A., Viljakainen, H., Pekkinen, M., and Mäkitie, O. (2018). A novel MYT1L mutation in a patient with severe early-onset obesity and intellectual disability. Am. J. Med. Genet. A 176, 1972–1975. doi: 10.1002/ajmg.a.40370
Lombardi, N., Flora, A., Franchini, R., Sorrentino, D., Lodi, G., and Varoni, E. M. (2019). Gingival localisation of extramedullary multiple myeloma. Lancet Oncol. 20:e653. doi: 10.1016/s1470-2045(19)30650-3
Lundgren, T., Crossner, C. G., Twetman, S., and Ullbro, C. (1996). Systemic retinoid medication and periodontal health in patients with Papillon-Lefèvre syndrome. J. Clin. Periodontol. 23, 176–179. doi: 10.1111/j.1600-051x.1996.tb02073.x
McCarthy, S. E., Makarov, V., Kirov, G., Addington, A. M., McClellan, J., Yoon, S., et al. (2009). Microduplications of 16p11.2 are associated with schizophrenia. Nat. Genet. 41, 1223–1227.
Meng, X. H., Chen, X. D., Greenbaum, J., Zeng, Q., You, S. L., Xiao, H. M., et al. (2018). Integration of summary data from GWAS and eQTL studies identified novel causal BMD genes with functional predictions. Bone 113, 41–48. doi: 10.1016/j.bone.2018.05.012
Murakami, S., Mealey, B. L., Mariotti, A., and Chapple, I. L. C. (2018). Dental plaque-induced gingival conditions. J. Periodontol. 89(Suppl. 1), S17–S27.
Ninichuk, V., and Anders, H. J. (2005). Chemokine receptor CCR1: a new target for progressive kidney disease. Am. J. Nephrol. 25, 365–372. doi: 10.1159/000087185
No authors listed. (2000). Parameter on plaque-induced gingivitis. american academy of periodontology. J. Periodontol. 71(Suppl. 5), 851–852. doi: 10.1902/jop.2000.71.5-s.851
Offenbacher, S., Jiao, Y., Kim, S. J., Marchesan, J., Moss, K. L., Jing, L., et al. (2018). GWAS for Interleukin-1β levels in gingival crevicular fluid identifies IL37 variants in periodontal inflammation. Nat. Commun. 9, 3686–3686.
Oh, T. J., Eber, R., and Wang, H. L. (2002). Periodontal diseases in the child and adolescent. J. Clin. Periodontol. 29, 400–410. doi: 10.1034/j.1600-051x.2002.290504.x
Oliver, R. C., Brown, L. J., and Löe, H. (1998). Periodontal diseases in the United States population. J. Periodontol. 69, 269–278. doi: 10.1902/jop.1998.69.2.269
Papapanou, P. N., Neiderud, A. M., Sandros, J., and Dahlén, G. (2001). Interleukin-1 gene polymorphism and periodontal status. A case-control study. J. Clin. Periodontol. 28, 389–396. doi: 10.1034/j.1600-051x.2001.028005389.x
Parkhill, J. M., Hennig, B. J., Chapple, I. L., Heasman, P. A., and Taylor, J. J. (2000). Association of interleukin-1 gene polymorphisms with early-onset periodontitis. J. Clin. Periodontol. 27, 682–689. doi: 10.1034/j.1600-051x.2000.027009682.x
Pavlides, J. M., Zhu, Z., Gratten, J., McRae, A. F., Wray, N. R., and Yang, J. (2016). Predicting gene targets from integrative analyses of summary data from GWAS and eQTL studies for 28 human complex traits. Genome Med. 8:84.
Peedikayil, F. C., Sreenivasan, P., and Narayanan, A. (2015). Effect of coconut oil in plaque related gingivitis - A preliminary report. Niger. Med. J. 56, 143–147. doi: 10.4103/0300-1652.153406
Porcu, E., Rüeger, S., Lepik, K., eQTLGen Consortium, Santoni, F. A., Reymond, A., et al. (2019). Mendelian randomization integrating GWAS and eQTL data reveals genetic determinants of complex and clinical traits. Nat. Commun. 10:3300.
Qu, K., Guo, F., Liu, X., Lin, Y., and Zou, Q. (2019). Application of machine learning in microbiology. Front. Microbiol. 10:827. doi: 10.3389/fmicb.2019.00827
Rockman, M. V., and Kruglyak, L. (2006). Genetics of global gene expression. Nat. Rev. Genet. 7, 862–872. doi: 10.1038/nrg1964
Shah, A., Ali, A., Latoo, S., and Ahmad, I. (2010). Multiple myeloma presenting as Gingival mass. J. Maxillofac. Oral Surg. 9, 209–212. doi: 10.1007/s12663-010-0050-7
Silva, T. A., Garlet, G. P., Lara, V. S., Martins, W. Jr., Silva, J. S., Cunha, F. Q., et al. (2005). Differential expression of chemokines and chemokine receptors in inflammatory periapical diseases. Oral Microbiol. Immunol. 20, 310–316. doi: 10.1111/j.1399-302x.2005.00232.x
Skelly, D. A., Ronald, J., and Akey, J. M. (2009). Inherited variation in gene expression. Annu. Rev. Genomics Hum. Genet. 10, 313–332. doi: 10.1146/annurev-genom-082908-150121
Smith, G. D., and Ebrahim, S. (2003). ‘Mendelian randomization’: can genetic epidemiology contribute to understanding environmental determinants of disease? Int. J. Epidemiol. 32, 1–22. doi: 10.1093/ije/dyg070
Stamm, J. W. (1986). Epidemiology of gingivitis. J. Clin. Periodontol. 13, 360–366. doi: 10.1111/j.1600-051x.1986.tb01473.x
Sun, L., Liu, G., Su, L., and Wang, R. (2019). HS-MMGKG: a fast multi-objective harmony search algorithm for two-locus model detection in GWAS. Curr. Bioinform. 14, 749–761. doi: 10.2174/1574893614666190409110843
Theilade, E., Wright, W. H., Jensen, S. B., and Löe, H. (1966). Experimental gingivitis in man. II. A longitudinal clinical and bacteriological investigation. J. Periodont. Res. 1, 1–13. doi: 10.1111/j.1600-0765.1966.tb01842.x
Tokar, O. M., Batig, V. M., Ostafiichuk, M. O., Ishkov, M. O., and Sheremet, M. I. (2020). Investigation of the effect of formaldehyde on the condition of periodontal tissues of woodworking industry workers. J. Med. Life 13, 225–228.
Trombelli, L., Tatakis, D. N., Scapoli, C., Bottega, S., Orlandini, E., and Tosi, M. (2004). Modulation of clinical expression of plaque-induced gingivitis. II. Identification of “high-responder” and “low-responder” subjects. J. Clin. Periodontol. 31, 239–252. doi: 10.1111/j.1600-051x.2004.00478.x
Vallet, S., and Anderson, K. C. (2011). CCR1 as a target for multiple myeloma. Expert Opin. Ther. Targets 15, 1037–1047. doi: 10.1517/14728222.2011.586634
Veturi, Y., and Ritchie, M. D. (2018). How powerful are summary-based methods for identifying expression-trait associations under different genetic architectures? Pac. Symp. Biocomput. 23, 228–239.
Wang, L., Xuan, Z., Zhou, S., Kuang, L., and Pei, T. (2019). A novel model for predicting LncRNA-disease associations based on the LncRNA-MiRNA-disease interactive network. Curr. Bioinform. 14, 269–278. doi: 10.2174/1574893613666180703105258
Wang, X., Zhou, X., Liu, J., Liu, Z., Zhang, L., Gong, Y., et al. (2019). Genome-wide investigation of the clinical implications and molecular mechanism of long noncoding RNA LINC00668 and protein-coding genes in hepatocellular carcinoma. Int. J. Oncol. 55, 860–878.
Wantke, F., Focke, M., Hemmer, W., Bracun, R., Wolf-Abdolvahab, S., Götz, M., et al. (2000). Exposure to formaldehyde and phenol during an anatomy dissecting course: sensitizing potency of formaldehyde in medical students. Allergy 55, 84–87. doi: 10.1034/j.1398-9995.2000.00307.x
Wei, R. R., Zhang, M. Y., Rao, H. L., Pu, H. Y., Zhang, H. Z., and Wang, H. Y. (2012). Identification of ADH4 as a novel and potential prognostic marker in hepatocellular carcinoma. Med. Oncol. 29, 2737–2743. doi: 10.1007/s12032-011-0126-3
Weiss, L. A., Shen, Y., Korn, J. M., Arking, D. E., Miller, D. T., Fossdal, R., et al. (2008). Association between microdeletion and microduplication at 16p11.2 and autism. N. Engl. J. Med. 358, 667–675.
Woelber, J. P., Gärtner, M., Breuninger, L., Anderson, A., König, D., Hellwig, E., et al. (2019). The influence of an anti-inflammatory diet on gingivitis. A randomized controlled trial. J. Clin. Periodontol. 46, 481–490. doi: 10.1111/jcpe.13094
Wyganowska-Świątkowska, M., Nowak, A., Paszyńska, E., and Grzech-Lesniak, K. (2018). Ethanol influence on gingival fibroblasts - a real-time in vitro study. AAEM 25, 647–650. doi: 10.26444/aaem/78696
Zhang, S., Divaris, K., Moss, K., Yu, N., Barros, S., Marchesan, J., et al. (2016). The Novel ASIC2 Locus is Associated with Severe Gingival Inflammation. JDR Clin. Trans. Res. 1, 163–170. doi: 10.1177/2380084416645290
Zhang, S., Yu, N., and Arce, R. M. (2020). Periodontal inflammation: integrating genes and dysbiosis. Periodontology 2000 82, 129–142. doi: 10.1111/prd.12267
Zhang, T., Tan, P., Wang, L., Jin, N., Li, Y., Zhang, L., et al. (2017). RNALocate: a resource for RNA subcellular localizations. Nucleic Acids Res. 45, D135–D138.
Zhang, Z. M., Tan, J. X., Wang, F., Dao, F. Y., Zhang, Z. Y., and Lin, H. (2020). Early diagnosis of hepatocellular carcinoma using machine learning method. Front. Bioeng. Biotechnol. 8:254. doi: 10.3389/fbioe.2020.00254
Zhao, J., and Grant, S. F. (2011). Advances in whole genome sequencing technology. Curr. Pharm. Biotechnol. 12, 293–305. doi: 10.2174/138920111794295729
Zhao, T., Hu, Y., Peng, J., and Cheng, L. (2020). DeepLGP: a novel deep learning method for prioritizing lncRNA target genes. Bioinformatics. 36, 4466–4472. doi: 10.1093/bioinformatics/btaa428
Zhu, Z., Zhang, F., Hu, H., Bakshi, A., Robinson, M. R., Powell, J. E., et al. (2016). Integration of summary data from GWAS and eQTL studies predicts complex trait gene targets. Nat. Genet. 48, 481–487. doi: 10.1038/ng.3538
Zou, Q. (2019). Latest machine learning techniques for biomedicine and bioinformatics. Curr. Bioinform. 14, 176–177. doi: 10.2174/157489361403190220112855
Zou, Q., and Ma, Q. (2019). The application of machine learning to disease diagnosis and treatment. Math. Biosci. 320:108305. doi: 10.1016/j.mbs.2019.108305
Keywords: gingivitis, SNPs, genes, summary Mendelian randomization, GWAS, eQTL
Citation: Zhang J, Sun M, Zhao Y, Geng G and Hu Y (2021) Identification of Gingivitis-Related Genes Across Human Tissues Based on the Summary Mendelian Randomization. Front. Cell Dev. Biol. 8:624766. doi: 10.3389/fcell.2020.624766
Received: 01 November 2020; Accepted: 02 December 2020;
Published: 06 May 2021.
Edited by:
Lei Deng, Central South University, ChinaReviewed by:
Hao Lin, University of Electronic Science and Technology of China, ChinaJuan Wang, Inner Mongolia University, China
Copyright © 2021 Zhang, Sun, Zhao, Geng and Hu. This is an open-access article distributed under the terms of the Creative Commons Attribution License (CC BY). The use, distribution or reproduction in other forums is permitted, provided the original author(s) and the copyright owner(s) are credited and that the original publication in this journal is cited, in accordance with accepted academic practice. No use, distribution or reproduction is permitted which does not comply with these terms.
*Correspondence: Guannan Geng, kdou198909@163.com; Yang Hu, huyang@hit.edu.cn
†These authors have contributed equally to this work