- 1School of Mathematics and Statistics, Southwest University, Chongqing, China
- 2Institute of Fundamental and Frontier Sciences, University of Electronic Science and Technology of China, Chengdu, China
- 3Hainan Key Laboratory for Computational Science and Application, Hainan Normal University, Haikou, China
- 4School of Management, Shenzhen Polytechnic, Shenzhen, China
Objective: The aim of this study was to investigate the expression of cyclin-dependent kinase 1 (CDK1) in gastric cancer (GC), evaluate its relationship with the clinicopathological features and prognosis of GC, and analyze the advantage of CDK1 as a potential independent prognostic factor for GC.
Methods: The Cancer Genome Atlas (TCGA) data and corresponding clinical features of GC were collected. First, the aim gene was selected by combining five topological analysis methods, where the gene expression in paracancerous and GC tissues was analyzed by Limma package and Wilcox test. Second, the correlation between gene expression and clinical features was analyzed by logistic regression. Finally, the survival analysis was carried out by using the Kaplan–Meier. The gene prognostic value was evaluated by univariate and multivariate Cox analyses, and the gene potential biological function was explored by gene set enrichment analysis (GSEA).
Results: CDK1 was selected as one of the most important genes associated with GC. The expression level of CDK1 in GC tissues was significantly higher than that in paracancerous tissues, which was significantly correlated with pathological stage and grade. The survival rate of the CDK1 high expression group was significantly lower than that of the low expression group. CDK1 expression was significantly correlated with overall survival (OS). CDK1 expression was mainly involved in prostate cancer, small cell lung cancer, and GC and was enriched in the WNT signaling pathway and T cell receptor signaling pathway.
Conclusion: CDK1 may serve as an independent prognostic factor for GC. It is also expected to be a new target for molecular targeted therapy of GC.
Background
Gastric cancer (GC) is a very common malignant tumor, and its prognosis is relatively poor. In 2015, it was found that the incidence rate of GC was second in all cancers (Chen et al., 2015; Lin et al., 2019; Mottini et al., 2019). Due to the hidden nature of the disease, the early symptoms are not obvious. Most of patients were in the advanced stage at the time of treatment, and the 5-year overall survival (OS) rate was only 28.3% (Siegel and Naishadham, 2012). GC was prone to lymph node metastasis and had strong invasive ability (Cutsem et al., 2015). At present, many studies have focused on identifying new biomarkers for early diagnosis and prognosis prediction of GC (Verma and Sharma, 2018; Ji et al., 2019; Li et al., 2019; Ma et al., 2019; Zheng et al., 2019). However, no widely accepted biomarkers have been found. Therefore, it is very important to identify effective biomarkers for the diagnosis and prognosis of GC (Zou et al., 2016; Zeng et al., 2017, 2018; Zhang et al., 2017; Tang et al., 2018; Xu et al., 2018, 2019).
Cyclin-dependent kinase 1 (CDK1) gene is a cyclin kinase, which can lead to malignant cell proliferation after activation (Ling et al., 2013). It was shown that CDK1 had a positive regulatory effect on the cell cycle of GC, and that its abnormal activation was involved in the malignant transformation of GC. Fu et al. focused on the relationship between the expression of CDC25A and CDK1 and lymph cancer patients. They found that CDC25A and CDK1 were highly expressed in GC tissues with lymph nodes and lowly expressed in GC without lymph nodes. Wang et al. revealed that the high expression of Cyclin B2 and CDK1 in GC patients may indicate that the biological ability of tumor invasion was strong and was related to the low OS rate of patients. Gao et al. (2014) investigated that the downregulation of CDK1 and cyclin B1 expression contributed to oridonin-induced cell cycle arrest at the G2/M phase and growth inhibition in SGC-7901 GC cells. However, so far, no study has proposed and tested CDK1 as an independent prognostic factor for GC, and the molecular mechanism of CDK1 in GC is still unclear. Therefore, the aim of this study is to explore the advantages of CDK1 as an independent prognostic factor for GC from the point of view of statistics and bioinformatics.
In this paper, CDK1 was selected by the intersection of five topological analysis methods in CytoHubba plug-in. The expression of CDK1 in paracancerous tissues and GC tissues was compared, and its correlation with clinical features was studied by non-parametric test. Then, we investigated the prognostic value of CDK1 from analyzing the correlation of its expression with OS by univariate and multivariate Cox analyses and explored the potential biological function of CDK1 using the gene set enrichment analysis (GSEA).
Materials and Methods
Dataset
We collected the set of gene expression profiles of GC from the Gene Expression Omnibus database1. This dataset included 10 GC samples and 10 normal samples. The platform was GPL570 (Affymetrix Human Genome U133 Plus 2.0), and The Cancer Genome Atlas (TCGA) data with corresponding clinical features of GC were downloaded from TCGA database2 that contained 375 tumor tissue samples and 32 paracancerous tissue samples. Gene symbol annotation information was used to match the corresponding probe, gene expression (Workflow Type: HTSeq-FPKM), and related clinical information (Data Type: Clinical Supplement) data (Wang et al., 2016). TCGA database is publicly available and is an open access platform.
Gene Selection
First, the Limma method was used to identify differentially expressed genes (DEGs) between GC tissues and paracancerous tissues. Second, a protein–protein interaction (PPI) network of DEGs was constructed based on the STRING V11 database, and the clustering module of the hub genes was obtained using the Molecular Complex Detection (MCODE) method in Cytoscape software (Shannon et al., 2003). Finally, five topological analysis methods in the CytoHubba plug-in (Chin et al., 2014), including Closeness, Maximal Clique Centrality (MCC), Maximum Neighborhood Component (MNC), Degree, and Edge Percolated Component (EPC), were used to narrow down the gene shortlist further, and the top-ranked genes were compared and selected for downstream analysis.
Gene Expression in Paracancerous Tissues and GC Tissues
To compare the gene expression levels in GC and paracancerous tissues, first, the single-gene expression data were extracted using the Limma package, and the data were divided into the high and low expression groups using the median value (samples with the gene expression greater/less than the median value were considered as the high/low expression group). Second, the p-value of Wilcox test was calculated where p < 0.05 indicated that the gene expression was significantly different between GC tissues and paracancerous tissues. Beeswarm package was used to draw scatter plots for differential expression analysis. Finally, in order to prove the conclusion further, the Perl script was used to obtain the paired samples of paracancerous tissues and GC tissues, and the differential expression analysis plot of the paired samples was drawn.
Correlation Analysis Between Gene Expression and Clinical Features
A large amount of clinicopathological information including gender, age, tumor-node-metastasis (TNM) staging, grade, and pathological stage were collected from TCGA dataset. Logistic regression was used to test the correlation between gene expression and the clinical features where a p-value < 0.05 was considered statistically significant.
Survival Analysis
In the survival analysis, all the paracancerous tissue samples were removed, and only the GC tissue samples were retained. Based on the high and low expression groups, Kaplan–Meier was used to draw a survival analysis curve of the selected gene where a p-value of 0.05 was used as the statistical threshold.
Univariate and Multivariate Cox Analyses
Cox proportional hazard models of univariate and multivariate were used to calculate 95% confidence interval (CI) and hazard ratio (HR) where survival package was used for statistical analysis. Univariate Cox analysis model was used to compare the relationship between clinical features and survival rates. Multivariate Cox analysis model was used to evaluate how the gene expression and the clinical factors (gender, age, grade, and stage) affect OS. p < 0.05 was set as the threshold, and the forest boxplot was drawn using the survminer package.
Gene Enrichment Analysis
The data obtained from TCGA were divided into the high and low expression groups according to the expression of target gene for multi-factor GSEA (Subramanian, 2005). GSEA was carried out to explore the gene biological functions. The enrichment results that satisfied two conditions of FDR < 0.05 and p < 0.05 were considered statistically significant.
Results
Selection of CDK1 Gene
Limma identified 1,599 DEGs in the dataset GSE79973 where 1,269 genes were upregulated and 330 genes were downregulated. These DEGs were imported into the STRING V11 database to obtain a TSV file of protein interactions. After the hub genes were calculated by CytoHubba plug-in, one cluster module of hub genes with the highest scoring was obtained that contained 92 nodes/genes and 3,628 edges (Figure 1A). The 92 hub genes were calculated by five topological analysis methods, and the top 10 ranked genes for each method were selected (Table 1), among which CDK1, VCAN, CCNB1, and AURKB were found in the intersection of the results of five methods (Figure 1B). All the four hub genes were upregulated. Besides, CDK1 was found to be ranked first by two topological methods (MNC and EPC) (Table 1). Therefore, in the downstream analysis, we focused on the expression and prognostic value of CDK1 in GC.
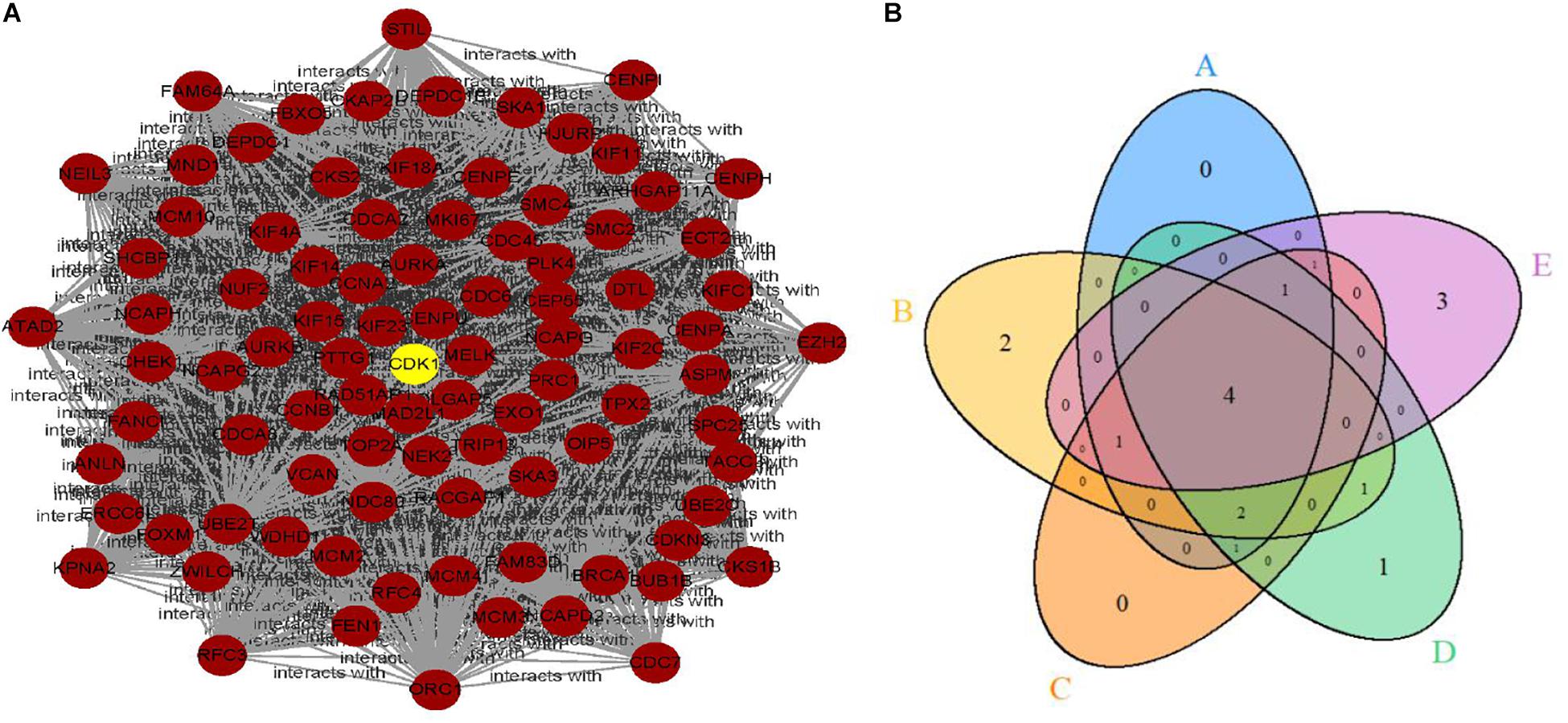
Figure 1. (A) The clustering module of the hub genes with the highest score generated by MCODE. (B) The intersection of the top 10 selected genes of five topological methods.
Expression of CDK1 in Paracancerous Tissues and GC Tissues
Since the median value of the expression level of CDK1 in GC tissues was significantly higher than that in paracancerous tissues, CDK1 was considered to be highly expressed in GC tissues (Figure 2A). This conclusion could also be drawn from the differential expression analysis of paired tissue samples (Figure 2B), where the lines connected the paracancerous tissue and GC tissue of the same patient. Most lines had an upward trend indicating that the expression level of CDK1 was highly expressed in GC tissues.
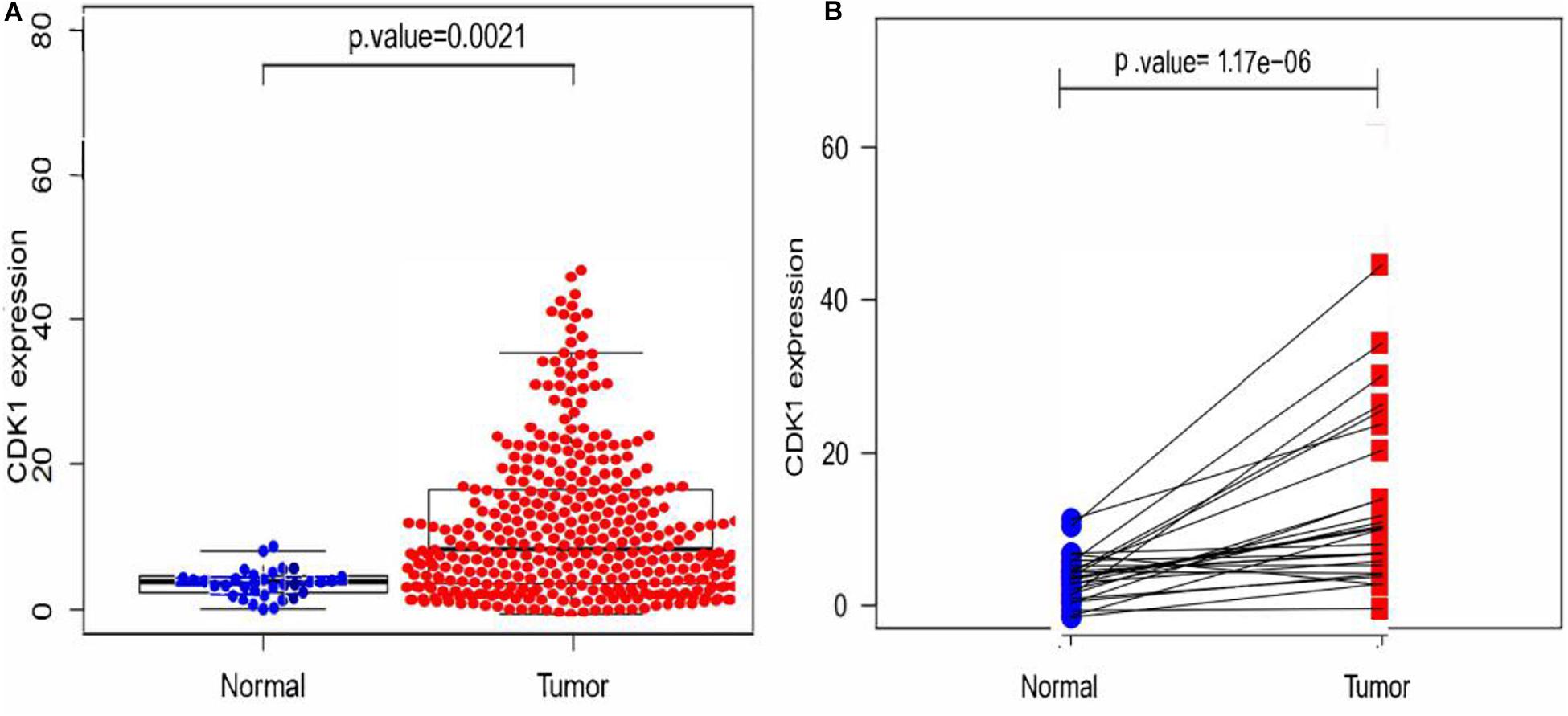
Figure 2. Expression analysis of CDK1. (A) The scatter plot of differential expression analysis of CDK1 in two types of tissues. (B) The differential expression analysis map of paired samples. Blue dots represent the paracancerous tissues, and red dots represent the GC tissues.
Correlation Between CDK1 Expression Level and Clinicopathological Features
According to clinical and pathological information from TCGA, logistic regression analysis showed that CDK1 expression level was significantly correlated with stage (p-value < 0.05 for III vs I and IV vs I) and grade (p-value < 0.05 for III vs I), but not significantly correlated with age and gender (p > 0.05, Table 2).
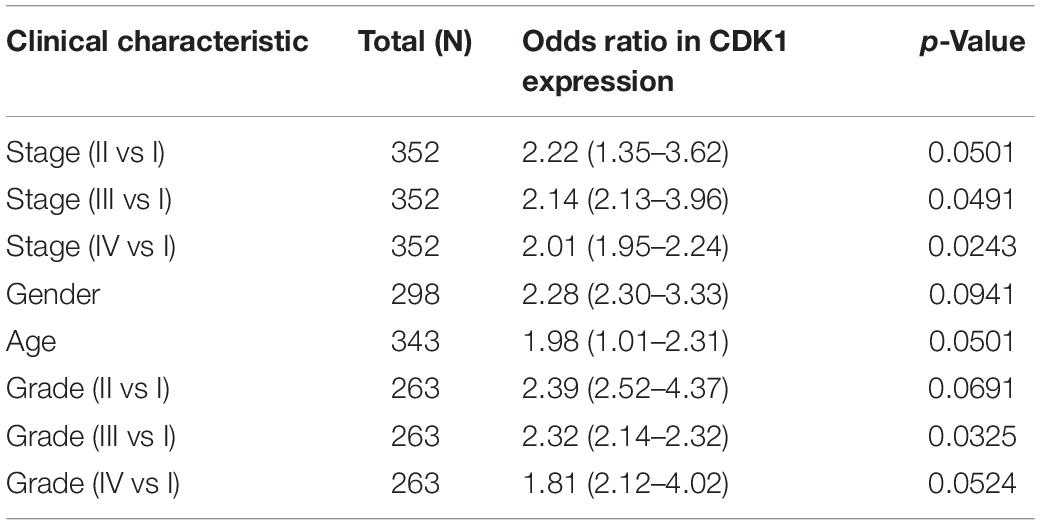
Table 2. Correlation between CDK1 expression and clinicopathological features using logistic regression.
Survival Analysis of CDK1
In the result of survival analysis of CDK1 (Figure 3), p < 0.05 indicated that the survival rates of the high and low expression groups were significantly different. The red line represented the high expression group, and the black line represented the low expression group. It was seen from the result in the figure that the survival rate of the high expression group was significantly lower than that of the low expression group.
Univariate and Multivariate Cox Analyses
The analysis results of the correlation between CDK1 expression and OS as well as other clinical features investigated by Cox models were shown in Table 3. In univariate survival analysis, some factors including age (HR = 1.269, p-value = 0.0056), pathological stage (HR = 1.3350, p-value = 0.0054), T (HR = 1.0975, p-value = 0.0317), N (HR = 1.6707, p-value = 0.0087), M (HR = 2.4306, p-value = 0.0245), and CDK1 expression (HR = 1.2182, p-value = 0.0023) were revealed to be significantly correlated with OS. And the multivariate Cox analysis, described by the forest boxplot (Figure 4), also suggested that age and CDK1 expression were significantly correlated with OS (p-value < 0.05). Therefore, CDK1 may serve as an independent prognostic factor for GC.
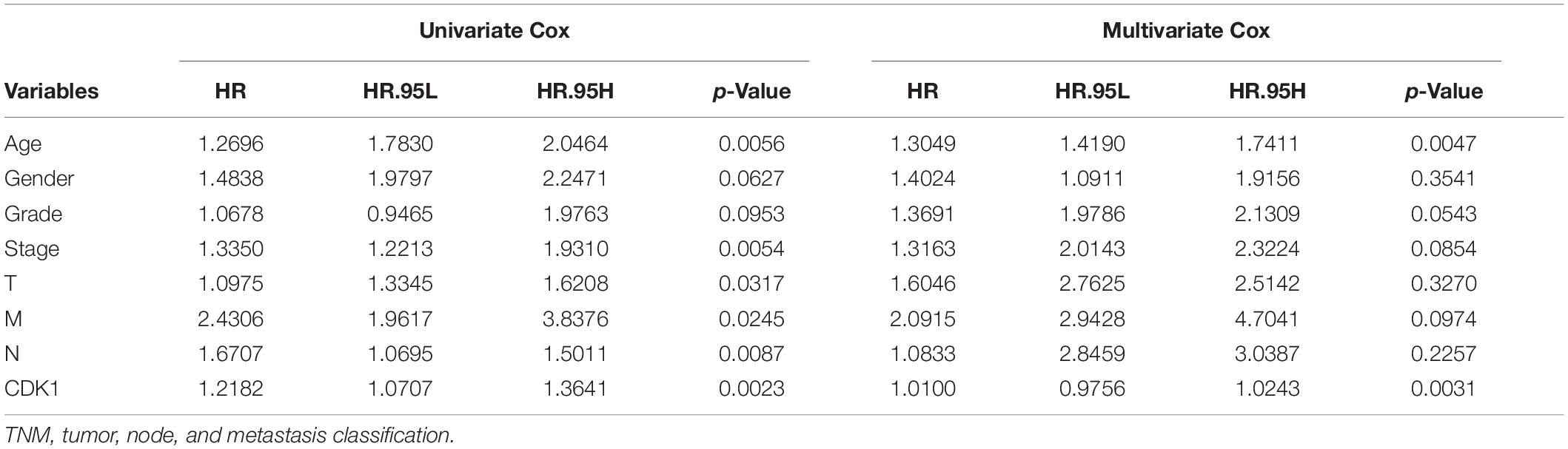
Table 3. Univariate and multivariate Cox analyses of the correlation between CDK1 expression and OS as well as other clinical features.
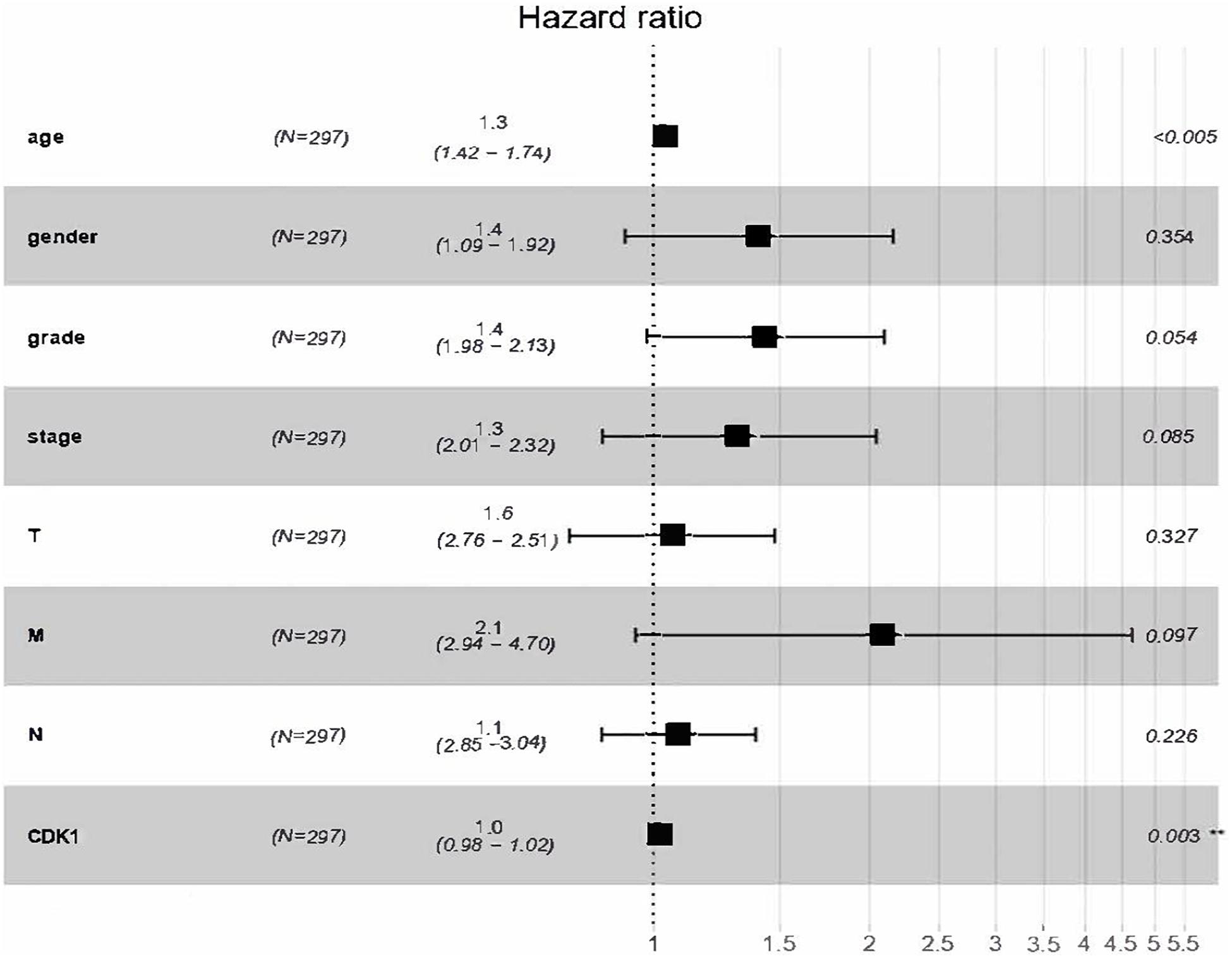
Figure 4. Forest boxplot for multivariate Cox analysis of the correlation between CDK1 expression and OS as well as other clinical features.
Multi-Factor GSEA Enrichment Analysis
Gene set enrichment analysis was performed to explore the potential biological functions of CDK1, and it was used to analyze the high and low expression groups of CDK1 gene. Figure 5 shows the result of Kyoto Encyclopedia of Genes and Genomes (KEGG) pathway analysis with top five pathways positively related to CDK1 expression and top four negatively related pathways. These results implied that CDK1 expression was involved in prostate cancer, small cell lung cancer, and GC and was significantly correlated with the WNT signaling pathway and T cell receptor signaling pathway (Table 4).
Discussion
Gastric cancer is one of the common digestive malignancies that seriously threaten human health. However, the factors affecting the occurrence, development, and prognosis of GC are still unclear. Therefore, it is of great significance to explore the genes related to the prognosis of GC from the gene level and molecule level for the treatment and prognosis assessment of GC.
The Cancer Genome Atlas database contains abundant types of tumor data and complete clinical information, which provides an important resource for the study of GC. In this study, the significant role of CDK1 in the treatment and prognosis of GC was discussed by comprehensive statistical methods. First, CDK1 was selected as the aim gene by combing five topological methods. The results of Limma package and Wilcox test showed that CDK1 was highly expressed in GC samples. Second, logistic regression was used to analyze the correlation between CDK1 expression and the corresponding clinical features. It was concluded that CDK1 expression was significantly correlated with pathological stage and grade, but not with age or gender. Survival analysis using Kaplan–Meier showed that the survival rate of the CDK1 high expression group was significantly lower than that of the low expression group. And the prognostic value of CDK1 was analyzed by univariate and multivariate Cox proportional hazard models. The results showed that CDK1 may be an independent prognostic factor for GC. Finally, GSEA revealed that CDK1 expression was involved in prostate cancer, small cell lung cancer, and GC and was significantly correlated with the WNT signaling pathway and T cell receptor signaling pathway.
There were several literatures using the same dataset in this study (Chen et al., 2020; Chi et al., 2020; Li Z. et al., 2020; Tian et al., 2020; Wang F. et al., 2020; Wu et al., 2020). However, most of them selected multiple genes for GC by some routine methods and did not concentrate on one gene/biomarker. And CDK1 was not identified as a key gene associated with GC in those studies. In view of the important role of CDK1 in the prognosis evaluation of GC, it may become a new target for precise treatment of GC, which is worthy of further study. Some references have shown that the abnormal expression of CDK1 was associated with poor prognosis of some other cancers including colorectal cancer, lung cancer, and pleural mesothelioma. Zhang et al. found that the loss of cytoplasmic CDK1 predicted low survival rate of human lung cancer and induced chemotherapeutic resistance (Chunyu et al., 2011). Linton et al. (2014) revealed through an RNAi-based screen that PLK1, CDK1, and NDC80 may be the potential therapeutic targets in malignant pleural mesothelioma. Sung et al. (2014) showed that high nuclear/cytoplasmic ratio of CDK1 expression predicted poor prognosis in colorectal cancer. Nishida et al. (2015) found that cyclin-dependent kinase activity was related to the prognosis of gastrointestinal tumors. These studies provided theoretical support for CDK1 as a therapeutic target and a new prognostic factor for GC. However, the expression and role of CDK1 in GC are still not fully understood. Therefore, this study evaluated the correlation between CDK1 and prognosis of GC as well as other clinicopathological features from the point of view of statistics and bioinformatics (Wang et al., 2013; Wei et al., 2017a,b, 2018; Su et al., 2018; Zhang et al., 2018; Ding et al., 2019; Shen et al., 2019; Fan et al., 2020; Li H. Y. et al., 2020; Li J. et al., 2020; Tan et al., 2020; Wang H. et al., 2020; Wang Z. et al., 2020) and provided important clues for further exploring the biological function and molecular mechanism of CDK1. In future work, if condition permits, we hope to conduct some experiments to verify the important role of CDK1 in GC from biological point of view.
Data Availability Statement
The original contributions presented in the study are included in the article/supplementary material. Further inquiries can be directed to the corresponding author/s.
Ethics Statement
Ethical review and approval was not required for the study on human participants in accordance with the local legislation and institutional requirements. Written informed consent for participation was not required for this study in accordance with the national legislation and the institutional requirements.
Author Contributions
XZ contributed to data analysis, methodology, and article writing. HM contributed to investigation, figures construction, and validation who contributed equally with XZ. QZ contributed to methodology and validation. JW contributed to supervision and writing of the article. All authors contributed to the article and approved the submitted version.
Funding
This work was supported by the Natural Science Foundation of China (Nos. 61902259 and 11701471) and the Natural Science Foundation of Guangdong Province (Grant No. 2018A0303130084).
Conflict of Interest
The authors declare that the research was conducted in the absence of any commercial or financial relationships that could be construed as a potential conflict of interest.
Footnotes
References
Chen, W., Zheng, R., Baade, P. D., Zhang, S., Zeng, H., Bray, F., et al. (2015). Cancer statistics in China. CA Cancer J. Clin. 66, 115–32. doi: 10.3322/caac.21338
Chen, Y., Chen, W., Dai, X., Zhang, C., and Lu, J. (2020). Identification of the collagen family as prognostic biomarkers and immune-associated targets in gastric cancer. Int. Immunopharmacol. 87:106798. doi: 10.1016/j.intimp.2020.106798
Chi, Y., Wang, H., Wang, F., and Ding, M. (2020). PHTF2 regulates lipids metabolism in gastric cancer. Aging 12, 6600–6610. doi: 10.18632/aging.102995
Chin, C. H., Chen, S. H., Wu, H. H., Ho, C. W., Ko, M. T., and Lin, C. Y. (2014). cytoHubba: identifying hub objects and sub-networks from complex interactome. BMC Syst. Biol. 8:S11. doi: 10.1186/1752-0509-8-S4-S11
Chunyu, Z., Abdel, G. E., and Matthew, P. (2011). Loss of Cytoplasmic CDK1 Predicts Poor Survival in Human Lung Cancer and Confers Chemotherapeutic Resistance. PLoS One 6:e23849. doi: 10.1371/journal.pone.0023849
Cutsem, E. V., Bang, Y. J., Feng-Yi, F., Xu, J. M., Lee, K. W., Jiao, S. C., et al. (2015). HER2 screening data from ToGA: targeting HER2 in gastric and gastroesophageal junction cancer. Gastr. Cancer 18, 476–84. doi: 10.1007/s10120-014-0402-y
Ding, Y., Tang, J., and Guo, F. (2019). Identification of drug-side effect association via multiple information integration with centered kernel alignment. Neurocomputing 325, 211–224. doi: 10.1016/j.neucom.2018.10.028
Fan, M., Yuan, W., Zhao, W., Xu, M., Wang, S., Gao, X., et al. (2020). Joint Prediction of Breast Cancer Histological Grade and Ki-67 Expression Level Based on DCE-MRI and DWI Radiomics. IEEE J. Biomed. Health Inform. 24, 1632–1642. doi: 10.1109/jbhi.2019.2956351
Gao, S. Y., Li, J., Qu, X. Y., and Zhu, N. (2014). Downregulation of Cdk1 and CyclinB1 Expression Contributes to Oridonin-induced Cell Cycle Arrest at G2/M Phase and Growth Inhibition in SGC-7901 Gastric Cancer Cells. Asian Pac. J. Cancer Prev. 15, 6437–6441. doi: 10.7314/apjcp.2014.15.15.6437
Ji, J., Tang, J., Xia, K.-J., and Jiang, R. (2019). LncRNA in Tumorigenesis Microenvironment. Int. J. Mol. Sci. 14, 640–641 doi: 10.2174/157489361407190917161654
Li, H. Y., Zhou, J. X., Sun, H. Y., Qiu, Z. W., Gao, X., and Xu, Y. (2020). CaMeRe: A Novel Tool for Inference of Cancer Metabolic Reprogramming. Front. Oncol. 10:207. doi: 10.3389/fonc.2020.00207
Li, J., Pu, Y., Tang, J., Zou, Q., and Guo, F. (2020). DeepAVP: a dual-channel deep neural network for identifying variable-length antiviral peptides. IEEE J. Biomed. Health Inform. 24, 3012–3019. doi: 10.1109/jbhi.2020.2977091
Li, Y., Zhao, J., Yu, S., Wang, Z., He, X., Su, Y., et al. (2019). Extracellular Vesicles Long RNA Sequencing Reveals Abundant mRNA, circRNA, and lncRNA in Human Blood as Potential Biomarkers for Cancer Diagnosis. J. Clin. Chem. 65, 798–808. doi: 10.1373/clinchem.2018.301291
Li, Z., Liu, Z., Shao, Z., Li, C., and Liu, Y. (2020). Identifying multiple collagen gene family members as potential gastric cancer biomarkers using integrated bioinformatics analysis. PeerJ. 8:e9123. doi: 10.7717/peerj.9123
Lin, M., Li, X., Guo, H., Ji, F., Ye, L., Ma, X., et al. (2019). Identification of Bone Metastasis-associated Genes of Gastric Cancer by Genome-wide Transcriptional Profiling. Curr. Bioinform. 14, 62–69. doi: 10.2174/1574893612666171121154017
Ling, H., Samarasinghe, S., and Kulasiri, D. (2013). Computational experiments reveal the efficacy of targeting CDK2 and CKIs for significantly lowering cellular senescence bar for potential cancer treatment. Bio Syst. 111, 71–82. doi: 10.1016/j.biosystems.2012.12.001
Linton, A., Cheng, Y. Y., Griggs, K., Kirschner, M. B., Gattani, S., Srikaran, S., et al. (2014). An RNAi-based screen reveals PLK1, CDK1 and NDC80 as potential therapeutic targets in malignant pleural mesothelioma. Br. J. Cancer 110, 510–519. doi: 10.1038/bjc.2013.731
Ma, G., Liu, H., Du, M., Zhang, G., Lin, Y., Ge, Y., et al. (2019). A genetic variation in the CpG island of pseudogene GBAP1 promoter is associated with gastric cancer susceptibility. Cancer 125, 2465–2473. doi: 10.1002/cncr.32081
Mottini, C., Napolitano, F., Li, Z., Gao, X., and Cardone, L. (2019). Computer-aided drug repurposing for cancer therapy: Approaches and opportunities to challenge anticancer targets. Semin. Cancer Biol. 25, S1044–579X.
Nishida, T., Matsushima, T., Tsujimoto, M., Takahashi, T., Kawasaki, Y., Nakayama, S., et al. (2015). Cyclin-Dependent Kinase Activity Correlates with the Prognosis of Patients Who Have Gastrointestinal Stromal Tumors. Ann. Surg. Oncol. 22, 3565–3573. doi: 10.1245/s10434-015-4438-y
Shannon, P., Markiel, A., Ozier, O., Baliga, N. S., Wang, J. T., Ramage, D., et al. (2003). Cytoscape: A Software Environment for Integrated Models of Biomolecular Interaction Networks. Abington: Routledge.
Shen, Y., Tang, J., and Guo, F. (2019). Identification of protein subcellular localization via integrating evolutionary and physicochemical information into Chou’s general PseAAC. J. Theoret. Biol. 462, 230–239. doi: 10.1016/j.jtbi.2018.11.012
Su, R., Wu, H., Xu, B., Liu, X., and Wei, L. (2018). Developing a Multi-Dose Computational Model for Drug-induced Hepatotoxicity Prediction based on Toxicogenomics Data. Transact. Comput. Biol. Bioinform 16, 1231–1239. doi: 10.1109/tcbb.2018.2858756
Subramanian, A. (2005). Gene set enrichment analysis: A knowledge-based approach for interpreting genome-wide expression profiles. PNAS 102, 15545–15550. doi: 10.1073/pnas.0506580102
Sung, W. W., Lin, Y. M., Wu, P. R., Yen, H. H., Lai, H. W., Su, T. C., et al. (2014). High nuclear/cytoplasmic ratio of Cdk1 expression predicts poor prognosis in colorectal cancer patients. BMC Cancer 14:951. doi: 10.1186/1471-2407-14-951
Tan, R., Li, H., Huang, Z., Zhou, Y., Tao, M., Gao, X., et al. (2020). Neural Functions Play Different Roles in Triple Negative Breast Cancer (TNBC) and non-TNBC. Scient. Rep. 10:2020.
Tang, W., Wan, S., Yang, Z., Teschendorff, A. E., and Zou, Q. (2018). Tumor origin detection with tissue-specific miRNA and DNA methylation markers. Bioinformatics 34, 398–406. doi: 10.1093/bioinformatics/btx622
Tian, Y., Xing, Y., Zhang, Z., Peng, R., and Sun, Y. (2020). Bioinformatics Analysis of Key Genes and circRNA-miRNA-mRNA Regulatory Network in Gastric Cancer. BioMed. Res. Int. 2020, 1–16. doi: 10.1155/2020/2862701
Verma, R., and Sharma, P. C. (2018). Next generation sequencing-based emerging trends in molecular biology of gastric cancer. Am. J. Cancer Res. 8, 207–225.
Wang, F., Xue, Q., Xu, D., Jiang, Y., and Liu, X. (2020). Identifying the hub gene in gastric cancer by bioinformatics analysis and in vitro experiments. Cell Cycle 19, 1–12.
Wang, H., Ding, Y., Tang, J., and Guo, F. (2020). Identification of membrane protein types via multivariate information fusion with Hilbert-Schmidt Independence Criterion. Neurocomputing 383, 257–269. doi: 10.1016/j.neucom.2019.11.103
Wang, J. J. Y., Wang, X. L., and Gao, X. (2013). Non-negative matrix factorization by maximizing correntropy for cancer clustering. BMC Bioinform. 14:107. doi: 10.1186/1471-2105-14-107
Wang, Z., He, W., Tang, J., and Guo, F. (2020). Identification of Highest-Affinity Binding Sites of Yeast Transcription Factor Families. J. Chem. Inform. Model. 60, 1876–1883. doi: 10.1021/acs.jcim.9b01012
Wang, Z., Jensen, M. A., and Zenklusen, J. C. A. (2016). Practical Guide to The Cancer Genome Atlas (TCGA). New York: Springer.
Wei, L., Su, R., Wang, B., Li, X., and Zou, Q. (2018). Integration of deep feature representations and handcrafted features to improve the prediction of N 6-methyladenosine sites. Neurocomputing 324, 3–9. doi: 10.1016/j.neucom.2018.04.082
Wei, L., Wan, S., Guo, J., and Wong, K. K. (2017a). A novel hierarchical selective ensemble classifier with bioinformatics application. Artif. Intell. Med. 83, 82–90. doi: 10.1016/j.artmed.2017.02.005
Wei, L., Xing, P., Zeng, J., Chen, J., Su, R., and Guo, F. (2017b). Improved prediction of protein–protein interactions using novel negative samples, features, and an ensemble classifier. Artif. Intell. Med. 83, 67–74. doi: 10.1016/j.artmed.2017.03.001
Wu, X., Wu, Y., Ye, B., Wu, F., and Wang, P. (2020). High expression of ghrelin and obestatin prepropeptide in tumor tissues predicted adverse overall survival in gastric carcinoma patients. Medicine 99:e20635. doi: 10.1097/md.0000000000020635
Xu, L., Liang, G., Liao, C., Chen, G.-D., and Chang, C.-C. (2018). An Efficient Classifier for Alzheimer’s Disease Genes Identification. Molecules 23:3140. doi: 10.3390/molecules23123140
Xu, L., Liang, G., Liao, C., Chen, G.-D., and Chang, C.-C. (2019). k-Skip-n-Gram-RF: A Random Forest Based Method for Alzheimer’s Disease Protein Identification. Front. Genet. 10:33 doi: 10.3389/fgene.2019.00033
Zeng, X. X., Liu, L., Lu, L. Y., and Zou, Q. (2018). Prediction of potential disease-associated microRNAs using structural perturbation method. Bioinformatics 34, 2425–2432. doi: 10.1093/bioinformatics/bty112
Zeng, X., Liao, Y., Liu, Y., and Zou, Q. (2017). Prediction and Validation of Disease Genes Using HeteSim Scores. Transact. Comput. Biol. Bioinform. 14, 687–695.
Zhang, X., Zou, Q., and Rodriguez-Paton, A. (2017). Zeng XJIAToCB, Bioinformatics. Meta-path methods for prioritizing candidate disease miRNAs. Transact. Comput. Biol. Bioinform. 16, 283–291. doi: 10.1109/tcbb.2017.2776280
Zhang, Z., Song, J., Tang, J., Xu, X., and Guo, F. (2018). Detecting complexes from edge-weighted PPI networks via genes expression analysis. BMC Syst. Biol. 12:40. doi: 10.1186/s12918-018-0565-y
Zheng, R., Liang, J., Lu, J., Li, S., Zhang, G., Wang, X., et al. (2019). Genome-wide long non-coding RNAs identified a panel of novel plasma biomarkers for gastric cancer diagnosis. Gastr. Cancer 22, 631–741.
Keywords: CDK1, prognosis, biomarker, gastric cancer, bioinformatics
Citation: Zhang X, Ma H, Zou Q and Wu J (2020) Analysis of Cyclin-Dependent Kinase 1 as an Independent Prognostic Factor for Gastric Cancer Based on Statistical Methods. Front. Cell Dev. Biol. 8:620164. doi: 10.3389/fcell.2020.620164
Received: 22 October 2020; Accepted: 03 November 2020;
Published: 07 December 2020.
Edited by:
Tao Huang, Chinese Academy of Sciences (CAS), ChinaReviewed by:
Sanyi Tang, Shaanxi Normal University, ChinaChunyu Wang, Harbin Institute of Technology, China
Copyright © 2020 Zhang, Ma, Zou and Wu. This is an open-access article distributed under the terms of the Creative Commons Attribution License (CC BY). The use, distribution or reproduction in other forums is permitted, provided the original author(s) and the copyright owner(s) are credited and that the original publication in this journal is cited, in accordance with accepted academic practice. No use, distribution or reproduction is permitted which does not comply with these terms.
*Correspondence: Jin Wu, wujin@szpt.edu.cn