- 1Department of Biological Sciences, Xi’an Jiaotong-Liverpool University, Suzhou, China
- 2Institute of Ageing and Chronic Disease, University of Liverpool, Liverpool, United Kingdom
- 3Department of Mathematical Sciences, Xi’an Jiaotong-Liverpool University, Suzhou, China
- 4Institute of Integrative Biology, University of Liverpool, Liverpool, United Kingdom
- 5AI University Research Centre, Xi’an Jiaotong-Liverpool University, Suzhou, China
N6-methyladenosine (m6A) is the most abundant post-transcriptional modification in mRNA, and regulates critical biological functions via m6A reader proteins that bind to m6A-containing transcripts. There exist multiple m6A reader proteins in the human genome, but their respective binding specificity and functional relevance under different biological contexts are not yet fully understood due to the limitation of experimental approaches. An in silico study was devised to unveil the target specificity and regulatory functions of different m6A readers. We established a support vector machine-based computational framework to predict the epitranscriptome-wide targets of six m6A reader proteins (YTHDF1-3, YTHDC1-2, and EIF3A) based on 58 genomic features as well as the conventional sequence-derived features. Our model achieved an average AUC of 0.981 and 0.893 under the full-transcript and mature mRNA model, respectively, marking a substantial improvement in accuracy compared to the sequence encoding schemes tested. Additionally, the distinct biological characteristics of each individual m6A reader were explored via the distribution, conservation, Gene Ontology enrichment, cellular components and molecular functions of their target m6A sites. A web server was constructed for predicting the putative binding readers of m6A sites to serve the research community, and is freely accessible at: http://m6areader.rnamd.com.
Introduction
In the exploration of RNA epigenetics, more than 150 types of RNA modification have been identified (Boccaletto et al., 2018). The methylation of adenosine at the N6 position (m6A) is the most prevalent post-transcriptional modification in the mRNA (Meyer and Jaffrey, 2017), which was discovered in a wide range of eukaryotic RNAs (Adams and Cory, 1975) as well as viral RNAs (Gokhale et al., 2016). m6A was considered as a potential mRNA processing regulator in 1970s (Desrosiers et al., 1974), and subsequent studies noticed intensive functions of it (Patil et al., 2018), including cardiac gene expression (Kmietczyk et al., 2019), cell growth, neuronal development (Chen J. et al., 2019), stress response (Engel et al., 2018), translation initiation, and stabilizing junctional RNA (Liu B. et al., 2018).
Similar to other epigenetic modifications, m6A is thought to be dynamic and reversible (Song et al., 2019). It can be installed by methyltransferase (writers) or removed by demethylase (erasers). This internal modification also attracts specific binding proteins, namely readers, which bind selectively to m6A-containing transcripts (Liao et al., 2018). Additionally, m6A performs many functions through interacting with “reader” proteins (Hazra et al., 2019). The most widely studied readers are YT521-B homology (YTH) family of proteins, which possess the evolutionarily conserved YTH domain that recognizes m6A mark. The YTH domain consists of 100–150 residues and adopts alpha/beta fold, with 4–5 alpha helices surrounding a curved six-stranded beta sheet (Zhang et al., 2010). In human, five m6A readers were reported to have the YTH domain, namely YTHDF1,2,3 and YTHDC1,2. However, the YTH domain is not indispensable for m6A readers, a subunit of translation initiation complex factor EIF3 complex, called EIF3A, was reported as an m6A reader lacking YTH domain (Meyer et al., 2015).
The m6A reader YTHDC1 is predominantly found in the nucleus, while YTHDC2 and YTHDF1,2,3 are cytoplasmic (Patil et al., 2016). YTHDC1 and YTHDC2 are unrelated to other members of the YTH family based on amino acid sequence, size or overall YTH domain organization (Patil et al., 2018). By contrast, YTHDF family comprises three paralogs, YTHDF1-3, that share high sequence identity with about 85% of sequence similarity (Hazra et al., 2019). YTHDC1 and three YTHDF proteins contain a single C-terminal YTH domain that binds to m6A marker by a segment rich of proline, glutamate and aspartate. Compared to other YTH domain-containing proteins, whose YTH domains are embedded in low complexity regions, YTHDC2 has a unique multidomain structure (Hazra et al., 2019). N-terminal R3H domain, central DEAH-box helicase domain and helicase associated 2 domain are also found in YTHDC2 apart from the C-terminal YTH domain. Different from the structures of five YTH domain-containing proteins, EIF3 is a large multiprotein complex comprising 13 subunits (Meyer et al., 2015). The EIF3 binding sites are predominantly mapped at the 5′ untranslated region (5′ UTR) (Lee et al., 2015), whereas the binding sites of YTH domain-containing proteins are usually located near the stop codon.
In addition to different cellular locations and structures, m6A readers appear to function through various post-transcriptional control mechanisms to regulate RNAs dynamically. Human YTHDC1 has been demonstrated to participate in RNA splicing by interacting with serine/arginine splicing factor SRSF3, which is involved in exon inclusion and exclusion splicing (Ye et al., 2017). As a putative RNA helicase, YTHDC2 enhances the translation of target RNAs and reduces the abundance of target RNAs (Hsu et al., 2017). YTHDF2 is verified to decrease the stability and control the lifetime of its targeted methylated mRNA transcripts (Du et al., 2016), while YTHDF1 ensures efficient protein expression from their shared regions (Wang et al., 2015). YTHDF3, the third member of YTHDF family, has been proposed to share common targets (about 60%) with both YTHDF1 and YTHDF2 (Shi et al., 2017). This suggests potential coordination in regulating gene expression by YTHDF family proteins. YTHDF3 can promote the function of YTHDF1 by interacting with some ribosomal proteins to facilitate mRNA translation. When associating with YTHDF2, YTHDF3 could participate in mRNA decay. In addition to the five members of YTH family, EIF3A plays an important role in biological processes as well. It can act as both repressor and activator of cap-dependent transcript-specific translation through directly binding to m6A marked mRNA sequence (Lee et al., 2015).
Since the five YTH family proteins (YTHDC1-2 and YTHDF1-3) and EIF3A present distinctive structures and properties, it is worth studying the preferential binding sites in the m6A marked transcripts for each m6A reader.
Single base resolution techniques such as miCLIP (Linder et al., 2015) are developed and are fairly effective on screening m6A sites, and it is usually based on the iCLIP or Par-CLIP approach (Meyer et al., 2015) to identify the binding sites of each m6A reader. As these wet-lab experiments are costly and laborious, computational methods may provide a viable avenue. To date, a large number of RNA methylation sites have been reported, providing sufficient information for effective computational prediction. A huge amount of data extracted from experiments encouraged the establishment of a number of effective m6A site predictors, including WHISTLE (Chen K. et al., 2019), SRAMP (Zhou et al., 2016), BERMP (Huang et al., 2018), and Gene2vec (Zou et al., 2019). However, to our knowledge, the prediction dedicated to the target specificity of the readers is absent. In this project, we constructed a predictor, m6A reader, to distinguish the substrate of each m6A reader. A comprehensive analysis of these readers was then performed, including the analysis of distribution, conservation, GO enrichment, cellular components and molecular functions of their respective epitranscriptome target sites.
Materials and Methods
Collection of m6A Sites and the Target Sites of m6A Readers
The transcriptome-wide m6A sites were collected from 17 different conditions generated from 6 different epitranscriptome profiling approaches of base-resolution or high resolution (Table 1).
In this study, we consider the binding sites of six m6A readers identified by Par-CLIP or iCLIP approaches. Specifically, a total of 16,664 m6A sites located on 4,722 different genes reported by four experiments were considered as the target sites of YTHDC1, and 1,234 sites on 275 genes identified by two experiments were considered as the target sites for YTHDC2. For the three proteins from YTHDF family, three experiments for each reader proposed 25,597, 28,970, and 7,253 target sites located on 6,714, 6,677, and 3,495 genes for YTHDF1, YTHDF2, and YTHDF3, respectively. Two CLIP experiments conducted on HEK2937T cell line discovered 756 sites located in 470 genes on marked RNA transcripts, which are targeted by EIF3A. The testing datasets and training datasets are strictly segregated under all conditions. Detailed information of the target sites of m6A readers analyzed in this study was summarized in Table 2.
Feature Encoding Scheme and Selection
We considered both the conventional sequence-derived features and the genome-derived features.
The sequence-derived features were summarized in the iLearn (Chen Z. et al., 2019; Chen et al., 2020) and BioSeq-Analysis (Liu, 2019; Liu et al., 2019), which can be divided into six different classes. Based on their classification, we chose one method from each class including nucleic acid composition (Lee et al., 2011), binary encoding method (Wu et al., 2015), position-specific tendencies of trinucleotide (He et al., 2018), electron-ion interaction pseudopotentials (He et al., 2019), Autocorrelation and pseudo k-tupler composition (Liu et al., 2015). Also, the chemical property combined with nucleic frequency, which is a popular encoding method in recent years (Bari et al., 2013; Chen et al., 2016a, b, 2017a; Li et al., 2018), was also used in performance testing for m6A reader target prediction.
The genomic features shown in previous projects (Chen K. et al., 2019; Song et al., 2019) are effective in RNA modification prediction. In order to improve the performance of the predictor, 58 mammalian genome features belonging to 9 classes were applied. All the features used were generated by the “GenomicFeatures R/Bioconducter” package using the transcript annotations hg19 TxDb package (Lawrence et al., 2013). The first class involves dummy variables indicating whether the adenosine site overlaps the topological region within the RNA transcript. The second class specifies the relative position of the adenosine site on the region, while the third class tells the length of the target mRNA transcript. Features belonging to the fourth class measure the nucleotide distances to the splicing junction and the nearest neighboring site. The fifth and sixth classes are based on clustering information of modification sites and scores related to conservation (Siepel et al., 2005; Gulko et al., 2015), respectively. The last three feature groups describe RNA secondary structures (Lorenz et al., 2011), genomic properties and attributes of the genes or transcripts, respectively. More details of the genomic features considered in our analysis were presented in Supplementary Table S1.
Feature Selection Technique
With multiple features, the dimension of dataset increases, leading to overfitting, information redundancy or increased computational time. To solve this problem, feature selection is effective in optimizing relevant modeling variables and improving the accuracy of the constructed models. In this study, we performed feature selection using F-score technique (Lin et al., 2014; Dao et al., 2019). Technically, F-score is a wrapper-type feature selection algorithm, used to measure the degree of difference between two real-number data sets. For a given training sample xd, there are n+ positive samples and n− negative samples. The F-score for the i-th feature can be calculated as:
where , and denote the average frequency of the i-th feature in the positive, negative and the whole samples, respectively; and represent the value of the i-th feature of the d-th sequence in the positive and negative samples, respectively. A larger F-score value means better predictive ability of a feature. To demonstrate this relative distinguishing ability of every genomic feature, the computed F-score values were rescaled between 0 and 1, and ranked in the descending order. Referring to this ranking, we used incremental feature selection (IFS) and SVM method to complete the selection process (Chen and Lin, 2006; Lin et al., 2014). Specifically, the feature subset begins with the feature with the highest F-score, and the next feature subset contains the last feature subset and one next feature. AUC values of 5-fold cross-validation were obtained for each feature subset.
Machine Learning Approach and Performance Evaluation
To reduce the bias in the experiment, especially when selecting the polyA RNAs during library preparation, we built separate prediction models using full transcript data and mature mRNA data, respectively. In the mature mRNA predictor, only m6A sites located in exon regions are considered.
Since the positive-to-negative ratio of our datasets was highly unbalanced (1:10), we randomly split the negative data into ten parts and combined with the positive dataset with 1:1 positive-to-negative ratio to avoid the unfavorable choice of machine learning classifiers. Subsequently, 10 models were trained and the average outcome score was reported as the performance of the classifier. For each m6A reader, the target sites identified in different experiments were mixed, and then the predictor was trained with 80% of the total sites before being evaluated by the remaining 20% of sites for independent testing. Specifically, the mature mRNA datasets for YTHDF1-3, YTHDC1-2, EIF3a have 39577, 44025, 11065, 24312, 1245, and 1200 training data, and 9895, 11007, 2767, 6078, 311, and 300 testing data. The full transcript datasets for those m6A readers have 40955, 46352, 11605, 26662, 1970, and 1210 training data, and 10239, 11588, 2901, 6666, 492, and 302 testing data.
Machine learning algorithms have been widely applied in many fields of biological research such as predicting structural and functional properties of biological sequences. We applied Support Vector Machine (SVM) (Chang and Lin, 2011) to compare encoding schemes and approaches. To identify a better algorithm for model construction, we compared multiple machine learning algorithms including SVM, Logistic Regression (LR), Random Forest (RF), and XGBoost.
To validate the model performance, besides 5-fold cross-validation, we also applied the cross-sample test, in which the sites reported from one sample (or condition) were reserved for testing purpose and the sites reported in all other samples (or conditions) were used for training. This testing mode directly evaluates the capability of the prediction approach to detect reader-specific target sites under a single biological condition not profiled previously. Besides, four commonly used performance metrics are used for performance evaluation, including Area under the ROC Curve (AUC) (Bradley, 1997), Precision-Recall Curve (PR AUC) (Keilwagen et al., 2014), accuracy (Acc) (Jin and Ling, 2005) and Mathew’s correlation coefficient (MCC) (Powers, 2008). The formula of Acc and MCC are as follows:
where TP is the number of true positives, TN the number of true negatives, FP the number of false positives and FN the number of false negatives.
Model construction and performance evaluation were conducted in R (Version 3.6.3). Machine learning algorithms were supported by caret package (Kuhn, 2020).
Results and Discussion
Feature Selection
Due to the high reliability and effectiveness in reflecting intrinsic relation to the targets, sequence-derived features have been widely used and achieved high accuracy in extensive researches focusing on the m6A site prediction. However, genome-derived features have been discovering and currently showing a new perspective in feature extraction (Zhou et al., 2016; Chen et al., 2017a). Here, we extracted genome features from 41 bp sequence data. We employed WHISTLE approach to combine both sequence-derived features and genome-derived features to predict the target specificity of m6A readers. To increase robustness and reduce overfitting of the predicter, feature selection was performed, where those most relevant features to the targets were identified.
Initially, all the genomic features were normalized to ensure the equal contribution of each feature. Then the F-score method was applied to allow all features to be ranked accordingly. Combining IFS and SVM, AUC value of 5-fold cross-validation were obtained for each feature subset. By examining AUC scores, the best performance was achieved by the optimal feature subset. The detailed feature selection results were summarized in Supplementary Figures S1–S6 for YTHDF1-3, YTHDC1-2 and EIF3A under both the full transcript and mature mRNA transcript, respectively. For example, it can be observed in Supplementary Figure S6A that, the best performance of EIF3A target prediction was achieved with the top 44 features for the mature mRNA model. Therefore, only the top 44 features were used ultimately to build the mature mRNA prediction models for EIF3A target prediction. Likewise, feature selection in target prediction was conducted for every other reader, and the predictors were constructed in the same way.
Performance Based on Different Features
With the nucleotide encoding methods based on chemical properties, extensive studies have achieved high accuracy in the m6A site prediction. However, for the first time, we explored and compared different sequence encoding schemes for predicting the target specificity of m6A-binding proteins.
For each m6A reader, the target sites identified in different experiments were mixed, and then the predictor was trained with 80% of the total sites before being evaluated by the remaining 20% of sites for independent testing. As a comparison, the performance of 5-fold cross-validation on the training data was also reported. As shown in Supplementary Table S7, m6A reader achieved AUC scores of 0.981 and 0.893 in independent testing under the full transcript and mature mRNA models, respectively. This performance is substantially better than other approaches that did not take advantage of genome-derived features.
Subsequently, we evaluated the capability of the proposed method in identifying the reader-specific target m6A sites under different biological contexts. In this test, the sites generated from each sample were used for independent testing, while all other samples were used for training, so the training sites and the test sites were not reported from the same condition. This is often the real scenario of interest where models are constructed to predict target sites in a new biological context. Besides this cross-condition test, the results of 5-fold cross-validation on the training data were also presented. The detailed evaluation results on every individual sample for every reader are shown in Supplementary Tables S2–S6, with a summary of the cross-condition tests presented in Table 3. It can be seen that our approach achieved a high accuracy with AUC scores of 0.975 and 0.873 under full transcript and mature mRNA models in the cross-condition test. The performance is again substantially better than the competing methods.
Detect Potential Substrate of m6A Readers
In order to further confirm the reliability and efficiency of our predictors, we used our predictors to detect m6A reader binding sites on the unidentified regions. As expected, all m6A readers bind to more than 20% m6A sites, while they bind to less than 10% unmethylated motifs as shown in Figure 1. The binding preference is significant and reasonable, which demonstrated the high discrimination ability of our predictors. Moreover, we compared the previous binding sites of YTHDF family (Figure 2A) and the prediction result of them on unidentified regions (Figure 2B). The wet-lab and prediction result shows that readers in YTHDF family have both common and distinct binding sites, suggesting that the binding sites of YTHDF proteins are not exactly identical. This is not consistent with the conclusion in the previous study that YTHDF proteins bind to identical sites on all m6A mRNAs (Zaccara and Jaffrey, 2020). Our result suggests that YTHDF family proteins have similar functions of mediating degradation of m6A mRNAs, and they also have different functions in mRNA regulation simultaneously. This result is consistent with our GO enrichment analysis, and also partially supports that m6A readers’ effect on downstream processes are much more heterogeneous and context-dependent across transcripts (Zhang et al., 2020). The predicted probabilities for the targeting of each m6A reader are provided on the download page of the website1.
Model Comparison
To discover a better machine learning algorithm for our proposed models, we compared the performance of SVM, LR, RF, and XGBoost on mature mRNA and full transcript data for the prediction of target specificity of six m6A readers. In general, the performances of different machine learning algorithms are all very high (>0.8 for mature mRNA models and >0.9 for full transcript models) and have little difference among them as shown in Supplementary Table S8. Therefore, we decided to use SVM classifier for the predictors.
Characterizing the Target Specificity of m6A Readers
Our result suggests that the substrates of m6A readers can be classified, reflecting the distinct biological characteristics of each m6A reader. We thus explored the distribution, conservation, and functional relevance of the substrates of each m6A reader.
Here, we firstly examined the distribution of binding sites for each reader (Figure 3). High enrichment of YTHDC1 is observed around stop codons and CDSs. However, it can be noticed that the binding abundance of YTHDC1 is relatively lower than members of YTHDF family in stop codons, while it is highly enriched in CDSs. This is consistent with the fact that YTHDC1 is not only targeting to m6A sites at its C terminus but also directly interacting with pre-mRNA splicing factor SRSF3 or SRSF10, which prefers to reside on the upper stream of m6A sites (Roundtree et al., 2017). The spatial association among those proteins implicates the process of recruiting pre-mRNA splicing factors and inducing mRNA splicing outcomes. Surprisingly, YTHDC2 targets are more enriched in CDSs near stop codons than in 3′ UTR, suggesting that YTHDC2 is distinct from other m6A readers. As YTHDC2 is reported to be the largest protein (∼160 kDa) among all YTH family members and with numerous RNA binding domains (e.g., helicase domain and two Ankyrin repeats, Hsu et al., 2017) apart from YTH domain, besides its acknowledged functions of accelerating translation and degradation of mRNAs as an m6A reader, it is possible that there are potential underlying functions independent from m6A-binding remained to be discovered. For instance, the recent study indicated that YTHDC2 as an RNA induced ATPase moves along the RNA from 3′ to 5′ with helicase activity, and interacts with 5′ to 3′ exoribonuclease XRN1 mediated by two Ankyrin repeats (ANK) on YTHDC2 (Wojtas et al., 2017). Remarkably, YTHDF family shows a similar binding distribution in CDSs and 3′ UTRs with peaks at around stop codons of mRNAs. A similar pattern of results was obtained in previous studies suggesting that YTHDFs directly interplay among one another to collaboratively regulate translation and decay of targeted mRNAs in the cytoplasm (Shi et al., 2017). The binding sites of EIF3A are uniquely enriched at 5′UTRs. This is directly in line with previous findings that the HLH motif of EIF3A interacts predominantly with the m6A residues on the 5′UTR, and EIF3A specifically functions to promote cap-independent translation under diverse cellular stresses.
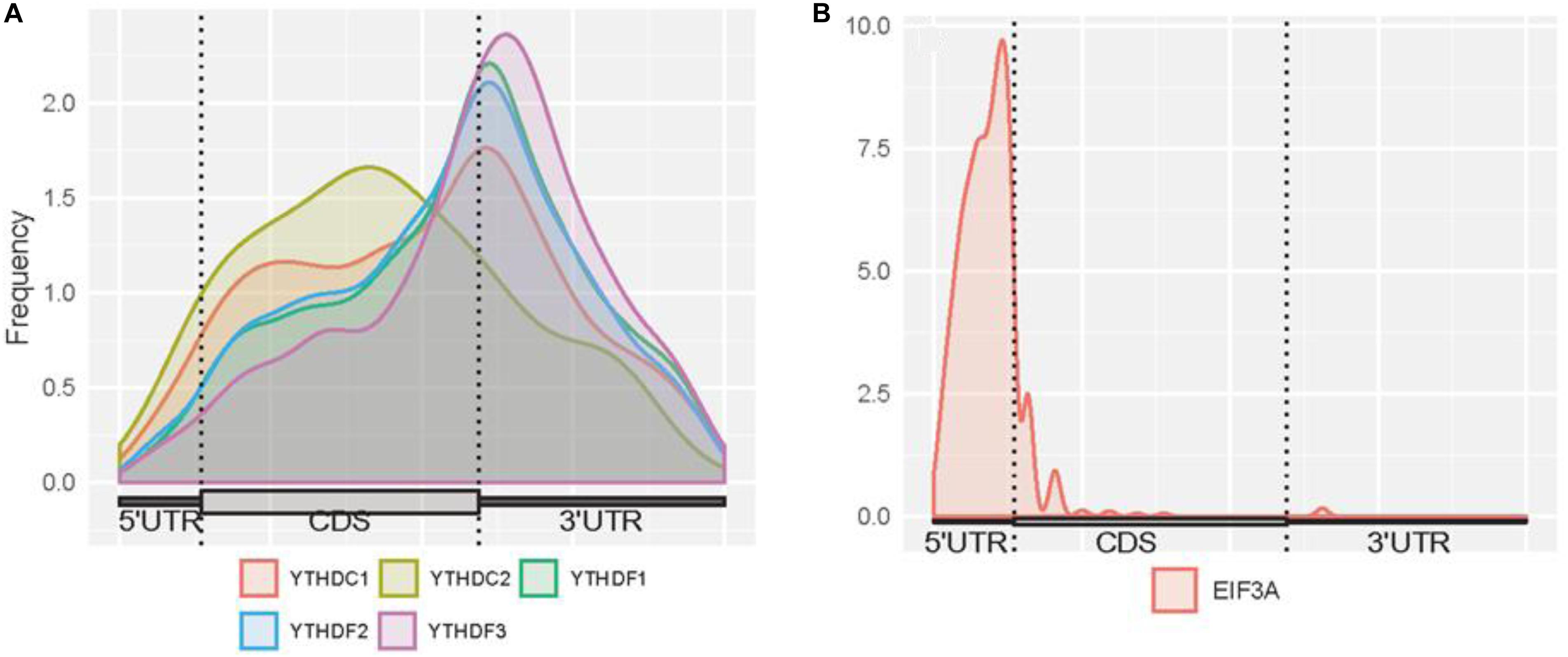
Figure 3. Distribution of m6A readers binding sites on mRNAs. (A) Distribution of the binding sites of YTHDC1, YTHDC2, YTHDF1, YTHDF2, and YTHDF3 on mRNAs. (B) Distribution of the binding sites of EIF3A on mRNAs. The figures were plotted using the Guitar R/Bioconductor package (Cui et al., 2016).
We then compared the conservation of all m6A readers by phastCons score and high conservation ratio (>0.5). As seen in Figures 4A,B, the m6A sites (targeted or not targeted by the studied six readers) are more conservative than unmethylated m6A motifs (DRACH). This suggests that m6A sites and the m6A reader binding sites are more evolutionarily conserved at the gene level, and the occurrence of m6A should be considered of functional importance and maintained under selection pressure. Moreover, the YTH family is more conserved compared with other regulation components, which is similar to the finding that YT521-B homology (YTH) RNA-binding domain in eukaryotes is known to be highly conserved with essential Lys-364, Trp-380, and Arg-478 (Zhang et al., 2010). Additionally, as shown in Figure 4C, compared with EIF3a binding sites and unmethylated sites which are mostly not in 3′ UTR, targets of other m6A readers and other untargeted m6A sites are more correlated with the miRNA binding sites. This result agrees well with existing studies investigated that miRNA targets are more enriched in 3′ UTR and m6A peaks prior to the present of miRNA binding for a majority of the time, suggesting that m6A modification functions to enhance initiation of miRNA biogenesis (Meyer et al., 2012; Alarcón et al., 2015). And the relative low overlapping rate between YTHDC2 binding sites and miRNA binding sites could be explained by multiple RNA-binding domains of YTHDC2. Furthermore, the proportions of overlapping of RNA-binding proteins (RBPs) and each m6A reader’s binding site are calculated. Figure 4D shows that RBPs binding regions overlap with m6A reader binding sites in mRNA more than the other m6A sites, while there are even fewer overlapping regions with unmethylated sites. This is consistent with our knowledge that some RBPs are essential in post-transcriptional control of RNAs including splicing, stabilization, localization and translation of mRNA. In the process of regulating transcription and translation, m6A readers may recruit large numbers of regulators or factors to their targeted RNAs so as to functionally regulate biological processes (Shi et al., 2017).
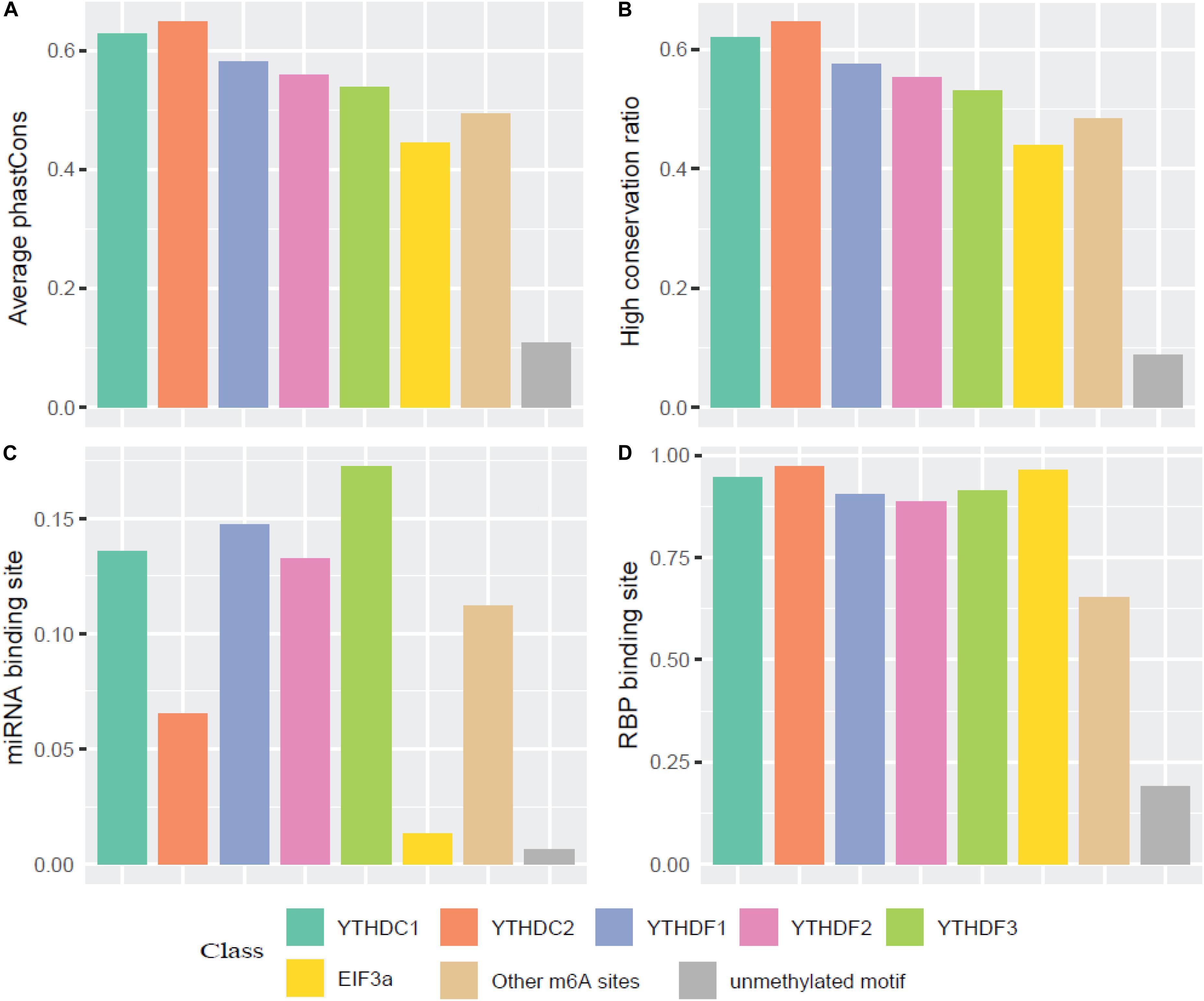
Figure 4. Conservation analysis of the m6A sites targeted by different readers. (A) Average phastCons; (B) High conservation ratio; (C) Frequency of miRNA binding site among the targets of six m6A readers; (D) Frequency of RBP binding site among the targets of six m6A readers.
To explore the association among m6A modification, readers and biological functions, the gene ontology (GO) enrichment analysis was conducted to measure the biological functions of substrates of each reader using DAVID websites (Huang da et al., 2009). The resulting top 10 GO functions related to each m6A readers were illustrated in Figure 5. Interestingly, YTHDC1 is involved in mRNA splicing, mRNA processing and nuclear-transcribed mRNA catabolic process, which is consistent with our understanding of its role of mediating nuclear to cytoplasmic export of nascent m6A-containing mRNAs (Roundtree et al., 2017). The targeting of YTHDC2, shown to accelerate the degradation of mRNA and enhance translation efficiency (Hsu et al., 2017), are more related to nonsense-mediated decay, protein stabilization and translational initiation. YTHDF1 targets are enriched under the GO terms of nuclear-transcribed mRNA catabolic process and translation initiation (Wang et al., 2015), suggesting its function in selectively recruiting of ribosomes and facilitating translation. YTHDF2 and YTHDF3 targets are both associated with proteasome-mediated ubiquitin-dependent protein catabolic process, which corresponds to our knowledge of their regulation in the metabolism of cytosolic m6A-modified mRNAs (Wang et al., 2014; Shi et al., 2017). EIF3A, reported to serve as a driver of specialized translation (Lee et al., 2015), is enriched with gene expression, translation and SRP-dependent co-translational protein targeting to the membrane. Moreover, as summarized in Supplementary Figure S7, six m6A readers show high enrichment in cytosol, cytoplasm, and membrane. Five of them (YTHDC1, YTHDF1-3, and EIF3a) are enriched in nucleus and nucleoplasm. While YTHDC2 is more enriched in extracellular exosome, extracellular matrix and myelin sheath instead of nucleus or nucleoplasm. All six proteins are enriched in the function of protein binding and poly(A) RNA binding, while they each have other specialized functions. This is consistent with analysis above on the enrichment of biological process and previous relevant literature. All gene ontology enrichment results were shown in Supplementary Table S9.
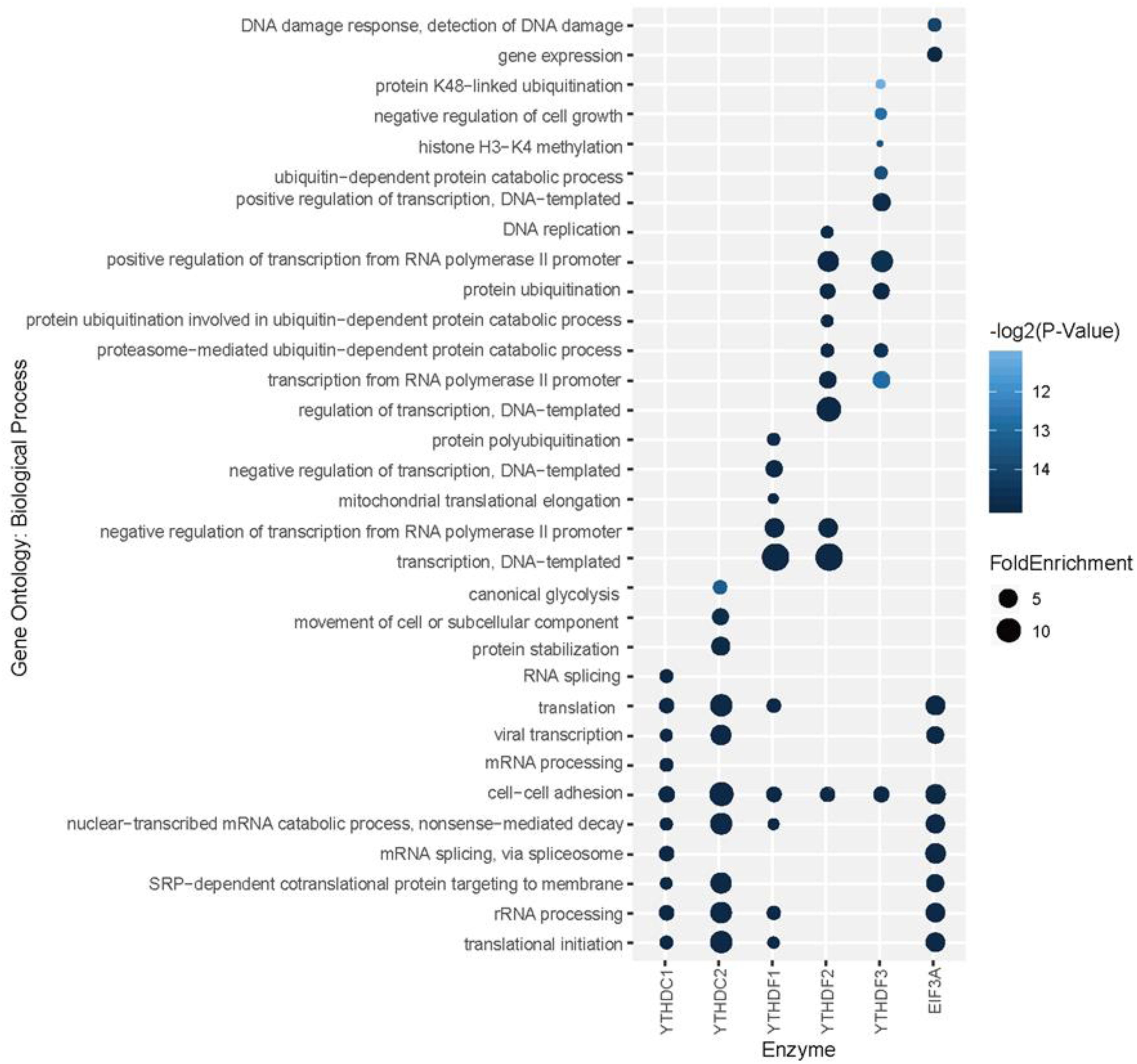
Figure 5. Gene ontology (GO) enrichment analysis for each reader’s substrates. The top 10 GO functions related to each m6A readers are presented.
Additionally, we further confirmed the biological meanings of the substrates of all m6A readers. Based on the results of previous GO enrichment analysis (Chen K. et al., 2018), the most significant p-values of top 10 terms treated with the negative logarithm were firstly added up, and then those computed results of identified targets were compared with those of randomly selected substrates. With the bootstrap sampling approach, substrates were randomly selected and analyzed for 100 times before the results were summarized as proportions and displayed in pie charts. Conceivably, if our results achieved on real data are more biologically meaningful than random permutation, it is possible that our analysis reliably unveiled the true biological functions. Specifically, there are 88, 100, 73, 68, 80, and 100% chances for each reader to be more enriched in biological functions than random permutation as illustrated in Figure 6, suggesting high possibility that our functional prediction for each individual reader is statistically meaningful.
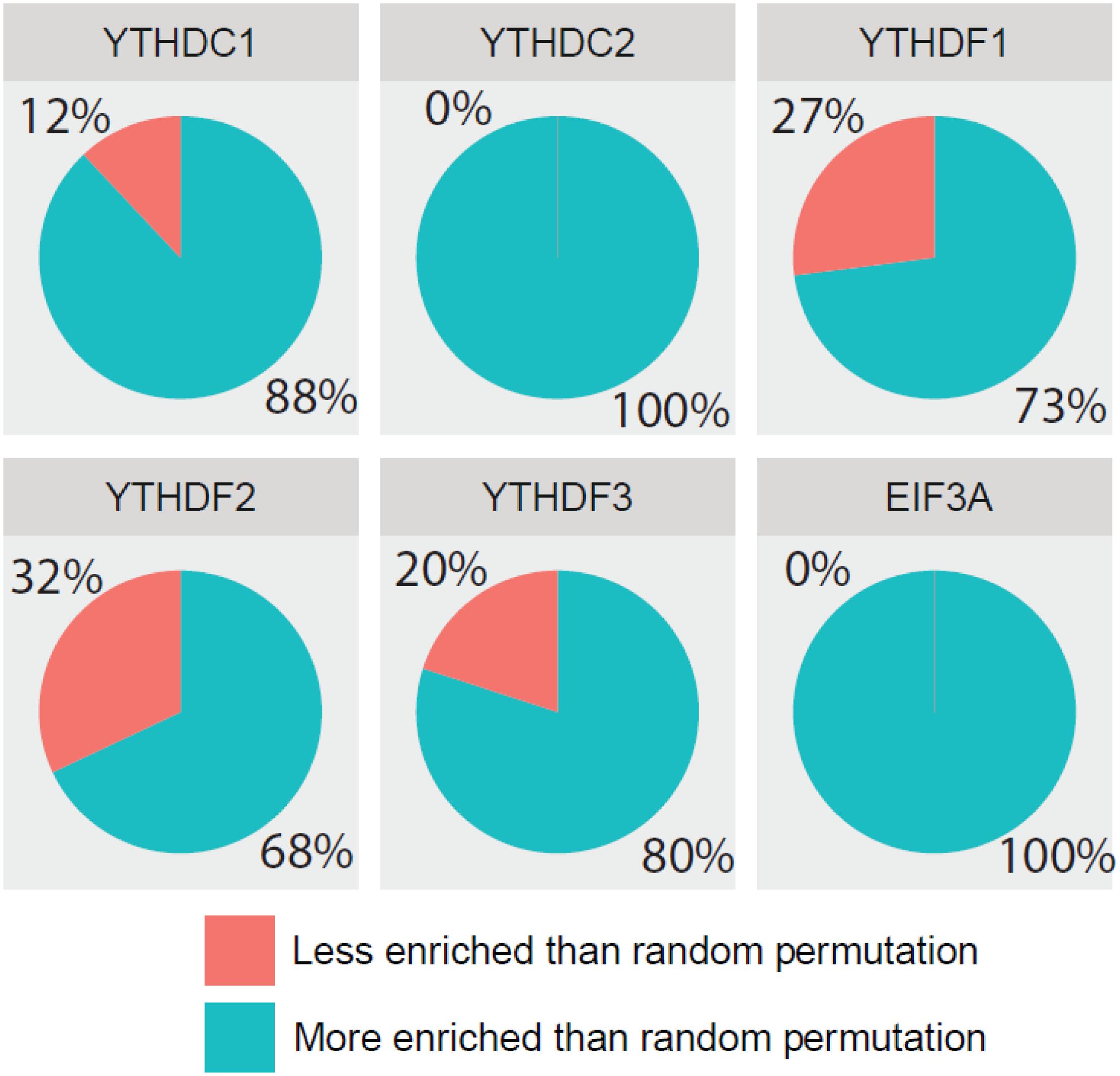
Figure 6. Comparing detection of m6A readers’ targets based on biological significance. The most significant p-values of top 10 GO terms treated with negative logarithm were added up, and those results of identified targets were compared with those of randomly selected substrates. With the bootstrap sampling approach, substrates were randomly selected and analyzed for 100 times before the results were summarized as proportions and displayed in pie charts.
Web Sever for m6A Reader
A web server with a friendly graphical user interface (Figure 7) was constructed to properly share the predictive models we constructed for predicting target specificity of the m6A readers. Users may upload the genome ranges in BED format to the website, and a notification email will be sent to the given email address once the job is finished.
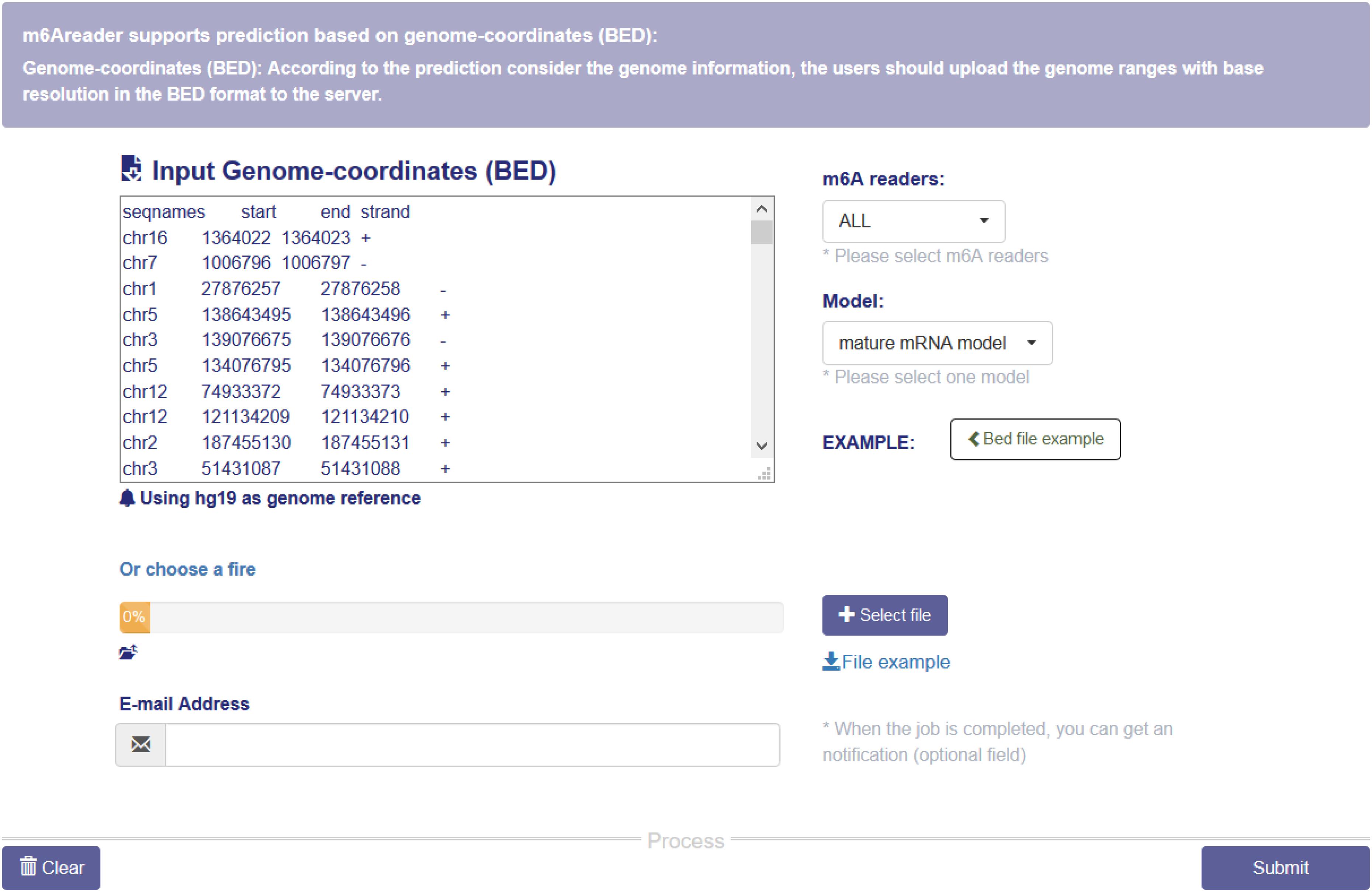
Figure 7. m6A reader web server. The web server takes genome ranges in BED format as the input, and supports prediction for the target sites of six m6A readers (YTHDC1, YTHDC2, YTHDF1, YTHDF2 and YTHDF3 and EIF3A). All the materials used in the project, including the training data and codes, are also available on the website.
Conclusion
With the great breakthroughs made in RNA modification-mediated regulation of gene expression, studies of emerging transcriptome modifications have driven rapid development of the high-throughput sequencing technologies. With the aid of the invention of m6A-seq (Dominissini et al., 2012) and MeRIP-seq (Meyer et al., 2012), transcriptome-wide profiling of m6A is now possible. Based on comprehensive high-throughput sequencing data, MeT-DB (Liu H. et al., 2018) and RMBase (Xuan et al., 2018) were established, providing the site information of RNA modifications. Subsequently, single-based technologies such as m6A-CLIP (Ke et al., 2015) and miCLIP (Linder et al., 2015) were also developed to precisely identify the positions of m6A. Complementary to experimental methods, well-established computational models facilitate the analysis of sequencing data and address the challenges presented in the bioinformatics community by predicting potential RNA methylation sites. The exomePeak R/Bioconductor package (Meng et al., 2013, 2014), MACS algorithm (Zhang et al., 2008) and DRME software (Liu et al., 2016) were introduced to analyze epitranscriptome profiling data, which improved our understanding of RNA methylation. Sequence-based site prediction models such as iRNA(m6A)-PseDNC (Chen W. et al., 2018) and iRNAMethyl (Chen et al., 2015b) applied statistical methods, whereas m6Apred (Chen et al., 2015c), RAM-ESVM (Chen et al., 2017b), and RNAMethPre (Xiang et al., 2016) integrated machine learning approaches, predicting m6A sites in different species’ transcriptome. Furthermore, potential RNA methylation-disease associations have been revealed by m6Avar (Zheng et al., 2018) and m6ASNP (Jiang et al., 2018). With a similar purpose, heterogeneous networks have been used in DRUM (Tang et al., 2019), FunDMDeep-m6A (Zhang et al., 2019b) and Deepm6A (Zhang et al., 2019a), showing a new perspective in studying disease-associated RNA methylation.
In this study, we constructed SVM-based models for the prediction of target specificity of m6A readers (YTHDC1, YTHDC2, YTHDF1, YTHDF2, YTHDF3, and EIF3A). The proposed models rely on 58 genomic features integrated with the sequence features related to chemical properties. After feature selection using the F-score method, those models achieved high prediction performance in 5-fold cross-validation and independent testing. Additionally, we compared the performance of different sequence encoding schemes on each reader’s substrate prediction. As existing m6A base-resolution data suffer from the bias of polyA selection, mature mRNA model was also considered besides the full transcript model. Moreover, we compared different machine learning algorithms and showed that four algorithms all demonstrate high performance with little difference in the prediction of target specificity of m6A readers. We eventually decided to use SVM classifier for our predictors.
It is also worth mentioning that our comprehensive analysis of m6A readers revealed potential regulatory patterns and biological relationships. We showed that m6A reader binding sites on mRNAs were concentrated in CDSs and 3′ UTR near stop codons, which is in line with m6A localization. Although distribution analysis of m6A readers has been conducted in previous studies and suggested similar binding patterns (Xu et al., 2014; Wang et al., 2015; Hsu et al., 2017), the results we presented were substantially enhanced with the incorporation of multiple datasets. Our result shed lights on the post-transcriptional and translational functions of m6A readers on m6A-containing mRNAs with more reliable evidence. Moreover, computed phastCons score and conservation ratio revealed a high conservation of the target sites of m6A readers, suggesting that they are possibly playing necessary or essential roles in regulating m6A-containing mRNAs. This is remarkable since we focused on the conservation of binding sites of m6A readers on mRNAs, rather than the conservation of m6A motifs itself as widely studied currently (Meyer et al., 2012), thus the biologically meaningful relationship between m6A readers and m6A modifications was confirmed. Besides, different from enrichment analysis alone in previous studies (Hsu et al., 2017), we not only unveiled functional relevance through the enrichment of the targets of m6A readers in biological process, cellular components and molecular functions by GO analysis, but also confirmed that reader-regulated sites are more likely to be biologically significant than randomly selected sites. The combination of statistical analysis and GO analysis ensures the robust detection and critical evaluation of the biological functions with a higher degree of confidence. Furthermore, our GO enrichment analysis result is also consistent with the wet-lab experiment and our prediction on unidentified regions that YTHDF proteins have both similar functions and different functions in the m6A mRNA regulation. This supports the conclusion made in previous study that m6A readers’ effect on downstream processes are much more heterogeneous and context-dependent across transcripts (Zhang et al., 2020).
However, this study has a number of limitations that could be improved in the future. Firstly, it has been argued that 4SU PAR-CLIP suffers from U-bias in contrast with UV-254 crosslinking or 6SG crosslinking (Ascano et al., 2012), thus other CLIP techniques are recommended to ensure crosslinking efficiency. Secondly, although data from different experiments were combined to build the predictors and 5-fold cross-validation was used to balance the bias-variance tradeoff, data of YTHDC2 and EIF3A substrates are still limited, which may make overfitting of the models possible. Thus, the analysis and prediction will benefit from other data from wet experiments in the future. Thirdly, as genome-derived features improved the performance of predictors dramatically, this suggests that genomic features carry important characteristics of biological data. Considering only 58 of them were involved in the feature selection procedure, it is worth exploring more genomic features so as to allow more effective features to be selected and reduce the bias as much as possible. In the future, it is expected to see the expanded studies of the enzyme target specificity and functional associations of other RNA modifications, such as m1A and Pseudouridine, on other types of RNAs, such as lncRNA and snRNAs, and in other species, such as mouse and yeast. Additional studies are clearly needed to investigate RNA-sequence-dependent m6A readers other than YTH domain-containing proteins such as FMR1 (Edupuganti et al., 2017). And it could be quite interesting to explore disease-associated RNA modification based on cellular binding patterns of regulatory proteins on modified RNAs.
Data Availability Statement
The datasets presented in this study can be found in online repositories. The names of the repository/repositories and accession number(s) can be found in the article/ Supplementary Material.
Author Contributions
KC conceived the idea, initialized the project, collected and processed the training and benchmark datasets. ZW generated the genomic features. DZ, YW, YZ, and HX built machine learning models. YT and BS designed and built the web server. DZ, YW, and YZ drafted the manuscript. All authors read, critically revised, and approved the final manuscript.
Funding
This work has been supported by the National Natural Science Foundation of China (31671373) and XJTLU Key Program Special Fund (KSF-T-01). This work was partially supported by the AI University Research Centre through XJTLU Key Programme Special Fund (KSF-P-02).
Conflict of Interest
The authors declare that the research was conducted in the absence of any commercial or financial relationships that could be construed as a potential conflict of interest.
Supplementary Material
The Supplementary Material for this article can be found online at: https://www.frontiersin.org/articles/10.3389/fcell.2020.00741/full#supplementary-material
Footnotes
References
Adams, J. M., and Cory, S. (1975). Modified nucleosides and bizarre 5′-termini in mouse myeloma mRNA. Nature 255, 28–33. doi: 10.1038/255028a0
Alarcón, C. R., Lee, H., Goodarzi, H., Halberg, N., and Tavazoie, S. F. (2015). N6-methyladenosine marks primary microRNAs for processing. Nature 519, 482–485. doi: 10.1038/nature14281
Ascano, M., Hafner, M., Cekan, P., Gerstberger, S., and Tuschl, T. (2012). Identification of RNA-protein interaction networks using PAR-CLIP. Wiley Interdiscip. Rev. 3, 159–177. doi: 10.1002/wrna.1103
Bari, A. T. M. G., Reaz, M. R., Choi, H.-J., and Jeong, B.-S. (2013). DNA Encoding for Splice Site Prediction in Large DNA Sequence. Berlin: Springer, 46–58.
Boccaletto, P., Machnicka, M. A., Purta, E., Piatkowski, P., Baginski, B., Wirecki, T. K., et al. (2018). MODOMICS: a database of RNA modification pathways. 2017 update. Nucleic Acids Res. 46, D303–D307. doi: 10.1093/nar/gkx1030
Boulias, K., Toczydlowska-Socha, D., Hawley, B. R., Liberman, N., Takashima, K., Zaccara, S., et al. (2019). Identification of the m(6)Am Methyltransferase PCIF1 reveals the location and functions of m(6)Am in the Transcriptome. Mol. Cell 75, 631.e8–643.e8. doi: 10.1016/j.molcel.2019.06.006
Bradley, A. P. (1997). The use of the area under the ROC curve in the evaluation of machine learning algorithms. Pattern Recogn. 30, 1145–1159. doi: 10.1016/s0031-3203(96)00142-2
Chang, C.-C., and Lin, C.-J. (2011). LIBSVM: a library for support vector machines. ACM Trans. Intell. Syst. Technol. 2, 1–27. doi: 10.1145/1961189.1961199
Chen, J., Zhang, Y. C., Huang, C., Shen, H., Sun, B., Cheng, X., et al. (2019). m(6)A regulates neurogenesis and neuronal development by modulating histone methyltransferase Ezh2. Genom. Proteom. Bioin. 17, 154–168. doi: 10.1016/j.gpb.2018.12.007
Chen, K., Lu, Z., Wang, X., Fu, Y., Luo, G.-Z., Liu, N., et al. (2015a). High-resolution N(6) -methyladenosine (m(6) A) map using photo-crosslinking-assisted m(6) A sequencing. Angew. Chem. Int. Ed. Engl. 54, 1587–1590. doi: 10.1002/anie.201410647
Chen, W., Feng, P., Ding, H., Lin, H., and Chou, K.-C. (2015b). iRNA-Methyl: Identifying N6-methyladenosine sites using pseudo nucleotide composition. Anal. Biochem. 490, 26–33. doi: 10.1016/j.ab.2015.08.021
Chen, W., Tran, H., Liang, Z., Lin, H., and Zhang, L. (2015c). Identification and analysis of the N6-methyladenosine in the Saccharomyces cerevisiae transcriptome. Anal. Biochem. 5:13859. doi: 10.1038/srep13859
Chen, K., Wei, Z., Liu, H., de Magalhaes, J. P., Rong, R., Lu, Z., et al. (2018). Enhancing epitranscriptome module detection from m(6)A-Seq data using threshold-based measurement weighting strategy. BioMed Res. Int. 2018:2075173. doi: 10.1155/2018/2075173
Chen, W., Ding, H., Zhou, X., Lin, H., and Chou, K.-C. (2018). iRNA(m6A)-PseDNC: Identifying N6-methyladenosine sites using pseudo dinucleotide composition. Anal. Biochem. 561-562, 59–65. doi: 10.1016/j.ab.2018.09.002
Chen, K., Wei, Z., Zhang, Q., Wu, X., Rong, R., Lu, Z., et al. (2019). WHISTLE: a high-accuracy map of the human N6-methyladenosine (m6A) epitranscriptome predicted using a machine learning approach. Nucleic Acids Res. 47:e41. doi: 10.1093/nar/gkz074
Chen, W., Feng, P., Tang, H., Ding, H., and Lin, H. (2016a). Identifying 2′-O-methylationation sites by integrating nucleotide chemical properties and nucleotide compositions. Genomics 107, 255–258. doi: 10.1016/j.ygeno.2016.05.003
Chen, W., Tang, H., Ye, J., Lin, H., and Chou, K. C. (2016b). iRNA-PseU: Identifying RNA pseudouridine sites. Mol. Ther. Nucleic Acids 5:e332. doi: 10.1038/mtna.2016.37
Chen, W., Tang, H., and Lin, H. (2017a). MethyRNA: a web server for identification of N(6)-methyladenosine sites. J. Biomol. Struct. Dyn. 35, 683–687. doi: 10.1080/07391102.2016.1157761
Chen, W., Xing, P., and Zou, Q. (2017b). Detecting N6-methyladenosine sites from RNA transcriptomes using ensemble support vector machines. Sci. Rep. 7:40242. doi: 10.1038/srep40242
Chen, Y.-W., and Lin, C.-J. (2006). “Combining SVMs with Various Feature Selection Strategies,” in Feature Extraction: Foundations and Applications, eds I. Guyon, M. Nikravesh, S. Gunn, and L. A. Zadeh (Berlin: Springe), 315–324. doi: 10.1007/978-3-540-35488-8_13
Chen, Z., Zhao, P., Li, F., Marquez-Lago, T. T., Leier, A., Revote, J., et al. (2019). iLearn: an integrated platform and meta-learner for feature engineering, machine-learning analysis and modeling of DNA, RNA and protein sequence data. Brief Bioinform. 21, 1047–1057. doi: 10.1093/bib/bbz041
Chen, Z., Zhao, P., Li, F., Marquez-Lago, T. T., Leier, A., Revote, J., et al. (2020). iLearn: an integrated platform and meta-learner for feature engineering, machine-learning analysis and modeling of DNA, RNA and protein sequence data. Brief Bioinform. 21, 1047–1057. doi: 10.1093/bib/bbz041
Cui, X., Wei, Z., Zhang, L., Liu, H., Sun, L., Zhang, S. W., et al. (2016). Guitar: An R/Bioconductor Package for gene annotation guided transcriptomic analysis of RNA-related genomic features. BioMed Res. Int. 2016:8367534. doi: 10.1155/2016/8367534
Dao, F. Y., Lv, H., Wang, F., Feng, C. Q., Ding, H., Chen, W., et al. (2019). Identify origin of replication in Saccharomyces cerevisiae using two-step feature selection technique. Bioinformatics 35, 2075–2083. doi: 10.1093/bioinformatics/bty943
Desrosiers, R., Friderici, K., and Rottman, F. (1974). Identification of methylated nucleosides in messenger RNA from Novikoff hepatoma cells. Proc. Natl Acade. Sci. U.S.A. 71, 3971–3975. doi: 10.1073/pnas.71.10.3971
Dominissini, D., Moshitch-Moshkovitz, S., Schwartz, S., Salmon-Divon, M., Ungar, L., Osenberg, S., et al. (2012). Topology of the human and mouse m6A RNA methylomes revealed by m6A-seq. Nature 485, 201–206. doi: 10.1038/nature11112
Du, H., Zhao, Y., He, J., Zhang, Y., Xi, H., Liu, M., et al. (2016). YTHDF2 destabilizes m(6)A-containing RNA through direct recruitment of the CCR4-NOT deadenylase complex. Nat. Commun. 7:12626. doi: 10.1038/ncomms12626
Edupuganti, R. R., Geiger, S., Lindeboom, R. G. H., Shi, H., Hsu, P. J., Lu, Z., et al. (2017). N6-methyladenosine (m6A) recruits and repels proteins to regulate mRNA homeostasis. Nat. Struct. Mol. Biol. 24, 870–878. doi: 10.1038/nsmb.3462
Engel, M., Eggert, C., Kaplick, P. M., Eder, M., Roh, S., Tietze, L., et al. (2018). The Role of m(6)A/m-RNA methylation in stress response regulation. Neuron 99, 389.e9–403.e9. doi: 10.1016/j.neuron.2018.07.009
Garcia-Campos, M. A., Edelheit, S., Toth, U., Safra, M., Shachar, R., Viukov, S., et al. (2019). Deciphering the m(6)A code via antibody-independent quantitative profiling. Cell 178, 731e16–747e16.
Gokhale, N. S., McIntyre, A. B. R., McFadden, M. J., Roder, A. E., Kennedy, E. M., Gandara, J. A., et al. (2016). N6-methyladenosine in flaviviridae Viral RNA genomes regulates infection. Cell Host Microbe 20, 654–665. doi: 10.1016/j.chom.2016.09.015
Gulko, B., Hubisz, M. J., Gronau, I., and Siepel, A. (2015). A method for calculating probabilities of fitness consequences for point mutations across the human genome. (in English). Nat. Genet. 47, 276–283. doi: 10.1038/ng.3196
Hazra, D., Chapat, C., and Graille, M. (2019). m(6)A mRNA destiny: chained to the rhYTHm by the YTH-Containing Proteins. Genes 10:49. doi: 10.3390/genes10010049
He, J., Fang, T., Zhang, Z., Huang, B., Zhu, X., and Xiong, Y. (2018). PseUI: pseudouridine sites identification based on RNA sequence information. BMC Bioinformatics 19:306. doi: 10.1186/s12859-018-2321-0
He, W., Jia, C., and Zou, Q. (2019). 4mCPred: machine learning methods for DNA N4-methylcytosine sites prediction. Bioinformatics 35, 593–601. doi: 10.1093/bioinformatics/bty668
Hsu, P. J., Zhu, Y., Ma, H., Guo, Y., Shi, X., Liu, Y., et al. (2017). Ythdc2 is an N(6)-methyladenosine binding protein that regulates mammalian spermatogenesis. Cell Res. 27, 1115–1127. doi: 10.1038/cr.2017.99
Huang, H., Weng, H., Zhou, K., Wu, T., Zhao, B. S., Sun, M., et al. (2019). Histone H3 trimethylation at lysine 36 guides m(6)A RNA modification co-transcriptionally. Nature 567, 414–419. doi: 10.1038/s41586-019-1016-7
Huang, Y., He, N., Chen, Y., Chen, Z., and Li, L. (2018). BERMP: a cross-species classifier for predicting m(6)A sites by integrating a deep learning algorithm and a random forest approach. Int. J. Biol. Sci. 14, 1669–1677. doi: 10.7150/ijbs.27819
Huang da, W., Sherman, B. T., and Lempicki, R. A. (2009). Systematic and integrative analysis of large gene lists using DAVID bioinformatics resources. Nat. Protoc. 4, 44–57. doi: 10.1038/nprot.2008.211
Jiang, S., Xie, Y., He, Z., Zhang, Y., Zhao, Y., Chen, L., et al. (2018). m6ASNP: a tool for annotating genetic variants by m6A function. GigaScience 7:giy035. doi: 10.1093/gigascience/giy035
Jin, H., and Ling, C. X. (2005). Using AUC and accuracy in evaluating learning algorithms. IEEE Trans. on Knowl. Data Eng. 17, 299–310. doi: 10.1109/tkde.2005.50
Ke, S., Alemu, E. A., Mertens, C., Gantman, E. C., Fak, J. J., Mele, A., et al. (2015). A majority of m6A residues are in the last exons, allowing the potential for 3′ UTR regulation. Genes Dev. 29, 2037–2053. doi: 10.1101/gad.269415.115
Ke, S., Pandya-Jones, A., Saito, Y., Fak, J. J., Vågbø, C. B., Geula, S., et al. (2017). m(6)A mRNA modifications are deposited in nascent pre-mRNA and are not required for splicing but do specify cytoplasmic turnover. Genes Dev. 31, 990–1006. doi: 10.1101/gad.301036.117
Keilwagen, J., Grosse, I., and Grau, J. (2014). Area under precision-recall curves for weighted and unweighted data. PLoS One 9:e92209. doi: 10.1371/journal.pone.0092209
Kmietczyk, V., Riechert, E., Kalinski, L., Boileau, E., Malovrh, E., Malone, B., et al. (2019). m(6)A-mRNA methylation regulates cardiac gene expression and cellular growth. Life Sci. Allian. 2:e201800233. doi: 10.26508/lsa.201800233
Kuhn, M. (2020). caret: Classification and Regression Training. R package version 6.0-85. Available: https://CRAN.R-project.org/package=caret (accessed March 20, 2020).
Lawrence, M., Huber, W., Pages, H., Aboyoun, P., Carlson, M., Gentleman, R., et al. (2013). Software for computing and annotating genomic ranges. PLoS Comput. Biol. 9:e1003118. doi: 10.1371/journal.pcbi.1003118
Lee, A. S., Kranzusch, P. J., and Cate, J. H. (2015). eIF3 targets cell-proliferation messenger RNAs for translational activation or repression. Nature 522, 111–114. doi: 10.1038/nature14267
Lee, D., Karchin, R., and Beer, M. A. (2011). Discriminative prediction of mammalian enhancers from DNA sequence. Genome Res. 21, 2167–2180. doi: 10.1101/gr.121905.111
Li, J., Huang, Y., Yang, X., Zhou, Y., and Zhou, Y. (2018). RNAm5Cfinder: a Web-server for Predicting RNA 5-methylcytosine (m5C) Sites Based on Random Forest. Sci. Rep. 8:17299. doi: 10.1038/s41598-018-35502-4
Liao, S., Sun, H., Xu, C., and Domain, Y. T. H. (2018). A family of N(6)-methyladenosine (m(6)A) Readers. Genom. Proteom. Bioinf. 16, 99–107. doi: 10.1016/j.gpb.2018.04.002
Lin, H., Deng, E. Z., Ding, H., Chen, W., and Chou, K. C. (2014). iPro54-PseKNC: a sequence-based predictor for identifying sigma-54 promoters in prokaryote with pseudo k-tuple nucleotide composition. Nucleic Acids Res. 42, 12961–12972. doi: 10.1093/nar/gku1019
Linder, B., Grozhik, A. V., Olarerin-George, A. O., Meydan, C., Mason, C. E., and Jaffrey, S. R. (2015). Single-nucleotide-resolution mapping of m6A and m6Am throughout the transcriptome. Nat. Methods 12, 767–772. doi: 10.1038/nmeth.3453
Liu, B. (2019). BioSeq-Analysis: a platform for DNA, RNA and protein sequence analysis based on machine learning approaches. Brief Bioinform. 20, 1280–1294. doi: 10.1093/bib/bbx165
Liu, B., Gao, X., and Zhang, H. (2019). BioSeq-Analysis2.0: an updated platform for analyzing DNA, RNA and protein sequences at sequence level and residue level based on machine learning approaches. Nucleic Acids Res. 47:e127. doi: 10.1093/nar/gkz740
Liu, B., Liu, F., Wang, X., Chen, J., Fang, L., and Chou, K. C. (2015). Pse-in-One: a web server for generating various modes of pseudo components of DNA, RNA, and protein sequences. Nucleic Acids Res. 43, W65–W71. doi: 10.1093/nar/gkv458
Liu, B., Merriman, D. K., Choi, S. H., Schumacher, M. A., Plangger, R., Kreutz, C., et al. (2018). A potentially abundant junctional RNA motif stabilized by m(6)A and Mg(2). Nat. Communi. 9:2761. doi: 10.1038/s41467-018-05243-z
Liu, H., Wang, H., Wei, Z., Zhang, S., Hua, G., Zhang, S. W., et al. (2018). MeT-DB V2.0: elucidating context-specific functions of N6-methyl-adenosine methyltranscriptome. Nucleic Acids Res. 46, D281–D287. doi: 10.1093/nar/gkx1080
Liu, L., Zhang, S.-W., Gao, F., Zhang, Y., Huang, Y., Chen, R., et al. (2016). DRME: count-based differential RNA methylation analysis at small sample size scenario. Anal. Biochem. 499, 15–23. doi: 10.1016/j.ab.2016.01.014
Lorenz, R., Bernhart, S. H., Zu Siederdissen, C. H., Tafer, H., Flamm, C., Stadler, P. F., et al. (2011). ViennaRNA Package 2.0. Algorithms Mol. Biol. 6:26.
Meng, J., Cui, X., Rao, M. K., Chen, Y., and Huang, Y. (2013). Exome-based analysis for RNA epigenome sequencing data. Bioinformatics 29, 1565–1567. doi: 10.1093/bioinformatics/btt171
Meng, J., Lu, Z., Liu, H., Zhang, L., Zhang, S., Chen, Y., et al. (2014). A protocol for RNA methylation differential analysis with MeRIP-Seq data and exomePeak R/Bioconductor package. Methods 69, 274–281. doi: 10.1016/j.ymeth.2014.06.008
Meyer, K. D., and Jaffrey, S. R. (2017). Rethinking m(6)A Readers, Writers, and Erasers. Annu. Rev. Cell Dev. Biol. 33, 319–342. doi: 10.1146/annurev-cellbio-100616-060758
Meyer, K. D., Patil, D. P., Zhou, J., Zinoviev, A., Skabkin, M. A., Elemento, O., et al. (2015). 5′ UTR m(6)A promotes cap-independent translation. Cell 163, 999–1010. doi: 10.1016/j.cell.2015.10.012
Meyer, K. D., Saletore, Y., Zumbo, P., Elemento, O., Mason, C. E., and Jaffrey, S. R. (2012). Comprehensive analysis of mRNA methylation reveals enrichment in 3′ UTRs and near stop codons. Cell 149, 1635–1646. doi: 10.1016/j.cell.2012.05.003
Patil, D. P., Chen, C.-K., Pickering, B. F., Chow, A., Jackson, C., Guttman, M., et al. (2016). m(6)A RNA methylation promotes XIST-mediated transcriptional repression. Nature 537, 369–373. doi: 10.1038/nature19342
Patil, D. P., Pickering, B. F., and Jaffrey, S. R. (2018). Reading m(6)A in the Transcriptome: m(6)A-Binding Proteins. Trends Cell Biol. 28, 113–127. doi: 10.1016/j.tcb.2017.10.001
Powers, D. (2008). Evaluation: from precision, recall and F-Factor to ROC, informedness, markedness & correlation. Mach. Learn. Technol. 2, 37–63.
Roundtree, I. A., Luo, G. Z., Zhang, Z., Wang, X., Zhou, T., Cui, Y., et al. (2017). YTHDC1 mediates nuclear export of N(6)-methyladenosine methylated mRNAs. eLife 6:e31311. doi: 10.7554/eLife.31311
Schwartz, S., Mumbach, M. R., Jovanovic, M., Wang, T., Maciag, K., Bushkin, G. G., et al. (2014). Perturbation of m6A writers reveals two distinct classes of mRNA methylation at internal and 5′ sites. Cell Re. 8, 284–296. doi: 10.1016/j.celrep.2014.05.048
Shi, H., Wang, X., Lu, Z., Zhao, B. S., Ma, H., Hsu, P. J., et al. (2017). YTHDF3 facilitates translation and decay of N(6)-methyladenosine-modified RNA. Cell Res. 27, 315–328. doi: 10.1038/cr.2017.15
Siepel, A., Bejerano, G., Pedersen, J. S., Hinrichs, A. S., Hou, M., Rosenbloom, K., et al. (2005). Evolutionarily conserved elements in vertebrate, insect, worm, and yeast genomes. Genome Res. 15, 1034–1050. doi: 10.1101/gr.3715005
Song, Y., Xu, Q., Wei, Z., Zhen, D., Su, J., Chen, K., et al. (2019). Predict epitranscriptome targets and regulatory functions of N (6)-Methyladenosine (m(6)A) Writers and Erasers. Evolu. Bioinf. Online 15:1176934319871290. doi: 10.1177/1176934319871290
Tang, Y., Chen, K., Wu, X., Wei, Z., Zhang, S.-Y., Song, B., et al. (2019). DRUM: inference of disease-associated m(6)A RNA methylation sites from a multi-layer heterogeneous network. Front. Genet. 10:266. doi: 10.3389/fgene.2019.00266
van Tran, N., Ernst, F. G. M., Hawley, B. R., Zorbas, C., Ulryck, N., Hackert, P., et al. (2019). The human 18S rRNA m6A methyltransferase METTL5 is stabilized by TRMT112. Nucleic Acids Res. 47, 7719–7733. doi: 10.1093/nar/gkz619
Vu, L. P., Pickering, B. F., Cheng, Y., Zaccara, S., Nguyen, D., Minuesa, G., et al. (2017). The N(6)-methyladenosine (m(6)A)-forming enzyme METTL3 controls myeloid differentiation of normal hematopoietic and leukemia cells. Nature Med. 23, 1369–1376. doi: 10.1038/nm.4416
Wang, X., Lu, Z., Gomez, A., Hon, G. C., Yue, Y., Han, D., et al. (2014). N6-methyladenosine-dependent regulation of messenger RNA stability. Nature 505, 117–120. doi: 10.1038/nature12730
Wang, X., Zhao, B. S., Roundtree, I. A., Lu, Z., Han, D., Ma, H., et al. (2015). N(6)-methyladenosine modulates messenger RNA translation efficiency. Cell 161, 1388–1399. doi: 10.1016/j.cell.2015.05.014
Wojtas, M. N., Pandey, R. R., Mendel, M., Homolka, D., Sachidanandam, R., and Pillai, R. S. (2017). Regulation of m(6)A Transcripts by the 3′–>5′ RNA Helicase YTHDC2 Is Essential for a Successful Meiotic Program in the Mammalian Germline. Mol. Cell 68, 374.e12–387.e12. doi: 10.1016/j.molcel.2017.09.021
Wu, H., Xu, T., Feng, H., Chen, L., Li, B., and Yao, B., et al. (2015). Detection of differentially methylated regions from whole-genome bisulfite sequencing data without replicates. Nucleic Acids Res. 43:e141.
Xiang, S., Liu, K., Yan, Z., Zhang, Y., and Sun, Z. (2016). RNAMethPre: A Web Server for the Prediction and Query of mRNA m6A Sites. PLoS One 11:e0162707. doi: 10.1371/journal.pone.0162707
Xiao, W., Adhikari, S., Dahal, U., Chen, Y. S., Hao, Y. J., Sun, B. F., et al. (2016). Nuclear m(6)A Reader YTHDC1 Regulates mRNA Splicing. Mol. Cell 61, 507–519. doi: 10.1016/j.molcel.2016.01.012
Xu, C., Wang, X., Liu, K., Roundtree, I. A., Tempel, W., Li, Y., et al. (2014). Structural basis for selective binding of m6A RNA by the YTHDC1 YTH domain. Nat. Chem. Biol. 10, 927–929. doi: 10.1038/nchembio.1654
Xuan, J. J., Sun, W. J., Lin, P. H., Zhou, K. R., Liu, S., Zheng, L. L., et al. (2018). RMBase v2.0: deciphering the map of RNA modifications from epitranscriptome sequencing data. Nucleic Acids Res. 46, D327–D334. doi: 10.1093/nar/gkx934
Ye, F., Chen, E. R., and Nilsen, T. W. (2017). Kaposi’s sarcoma-associated herpesvirus utilizes and manipulates RNA N(6)-Adenosine methylation to promote lytic replication. J. Virol. 91:e00466-17. doi: 10.1128/jvi.00466-17
Zaccara, S., and Jaffrey, S. R. (2020). A unified model for the function of YTHDF proteins in regulating m(6)A-Modified mRNA. Cell 181, 1582.e18–1595.e18. doi: 10.1016/j.cell.2020.05.012
Zhang, S.-Y., Zhang, S.-W., Fan, X.-N., Meng, J., Chen, Y., Gao, S.-J., et al. (2019a). Global analysis of N6-methyladenosine functions and its disease association using deep learning and network-based methods. PLoS Comput. Biol. 15:e1006663. doi: 10.1371/journal.pcbi.1006663
Zhang, S.-Y., Zhang, S.-W., Fan, X.-N., Zhang, T., Meng, J., and Huang, Y. (2019b). FunDMDeep-m6A: identification and prioritization of functional differential m6A methylation genes. Bioinformatics 35, i90–i98. doi: 10.1093/bioinformatics/btz316
Zhang, Z., Chen, L. Q., Zhao, Y. L., Yang, C. G., Roundtree, I. A., Zhang, Z., et al. (2019c). Single-base mapping of m(6)A by an antibody-independent method. Sci. Adva. 5:eaax0250. doi: 10.1126/sciadv.aax0250
Zhang, Y., Liu, T., Meyer, C. A., Eeckhoute, J., Johnson, D. S., Bernstein, B. E., et al. (2008). Model-based analysis of ChIP-Seq (MACS). Genome Biolo. 9:R137. doi: 10.1186/gb-2008-9-9-r137
Zhang, Z., Theler, D., Kaminska, K. H., Hiller, M., de la Grange, P., Pudimat, R., et al. (2010). The YTH domain is a novel RNA binding domain. J. Biol. Chem. 285, 14701–14710. doi: 10.1074/jbc.M110.104711
Zhang, Z., Luo, K., Zou, Z., Qiu, M., Tian, J., Sieh, L., et al. (2020). Genetic analyses support the contribution of mRNA N6-methyladenosine (m6A) modification to human disease heritability. Nat. Genet. doi: 10.1038/s41588-020-0644-z [Epub ahead of print].
Zheng, Y., Nie, P., Peng, D., He, Z., Liu, M., Xie, Y., et al. (2018). m6AVar: a database of functional variants involved in m6A modification. Nucleic Acids Res. 46, D139–D145. doi: 10.1093/nar/gkx895
Zhou, Y., Zeng, P., Li, Y. H., Zhang, Z., and Cui, Q. (2016). SRAMP: prediction of mammalian N6-methyladenosine (m6A) sites based on sequence-derived features. Nucleic Acids Res. 44:e91. doi: 10.1093/nar/gkw104
Keywords: N6-methyladenosine, m6A reader, machine learning (ML), YTH domain, eIF3a
Citation: Zhen D, Wu Y, Zhang Y, Chen K, Song B, Xu H, Tang Y, Wei Z and Meng J (2020) m6A Reader: Epitranscriptome Target Prediction and Functional Characterization of N6-Methyladenosine (m6A) Readers. Front. Cell Dev. Biol. 8:741. doi: 10.3389/fcell.2020.00741
Received: 19 May 2020; Accepted: 16 July 2020;
Published: 11 August 2020.
Edited by:
Yu Xue, Huazhong University of Science and Technology, ChinaReviewed by:
Fuyi Li, Monash University, AustraliaNicolas Reynoird, INSERM U1209 Institut pour l’Avancée des Biosciences (IAB), France
Lin Zhang, China University of Mining and Technology, China
Copyright © 2020 Zhen, Wu, Zhang, Chen, Song, Xu, Tang, Wei and Meng. This is an open-access article distributed under the terms of the Creative Commons Attribution License (CC BY). The use, distribution or reproduction in other forums is permitted, provided the original author(s) and the copyright owner(s) are credited and that the original publication in this journal is cited, in accordance with accepted academic practice. No use, distribution or reproduction is permitted which does not comply with these terms.
*Correspondence: Kunqi Chen, a3VucWkuY2hlbkBsaXZlcnBvb2wuYWMudWs=; a3VucWkuY2hlbkB4anRsdS5lZHUuY24=
†These authors have contributed equally to this work