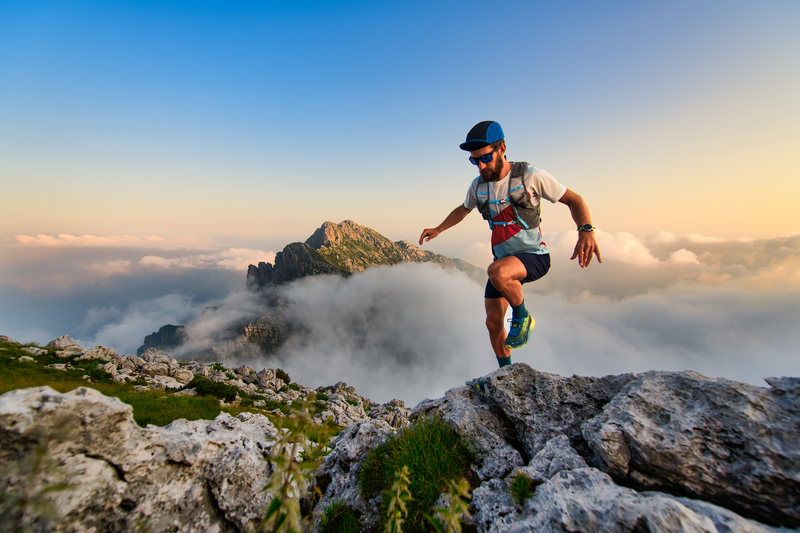
94% of researchers rate our articles as excellent or good
Learn more about the work of our research integrity team to safeguard the quality of each article we publish.
Find out more
ORIGINAL RESEARCH article
Front. Cell Dev. Biol. , 04 November 2014
Sec. Systems Biology Archive
Volume 2 - 2014 | https://doi.org/10.3389/fcell.2014.00059
This article is part of the Research Topic Multi-omic Data Integration View all 12 articles
Objective: To provide a frame to estimate the systemic impact (side/adverse events) of (novel) therapeutic targets by taking into consideration drugs potential on the numerous districts involved in rheumatoid arthritis (RA) from the inflammatory and immune response to the gut-intestinal (GI) microbiome.
Methods: We curated the collection of molecules from high-throughput screens of diverse (multi-omic) biochemical origin, experimentally associated to RA. Starting from such collection we generated RA-related protein-protein interaction (PPI) networks (interactomes) based on experimental PPI data. Pharmacological treatment simulation, topological and functional analyses were further run to gain insight into the proteins most affected by therapy and by multi-omic modeling.
Results: Simulation on the administration of MTX results in the activation of expected (apoptosis) and adverse (nitrogenous metabolism alteration) effects. Growth factor receptor-bound protein 2 (GRB2) and Interleukin-1 Receptor Associated Kinase-4 (IRAK4, already an RA target) emerge as relevant nodes. The former controls the activation of inflammatory, proliferative and degenerative pathways in host and pathogens. The latter controls immune alterations and blocks innate response to pathogens.
Conclusions: This multi-omic map properly recollects in a single analytical picture known, yet complex, information like the adverse/side effects of MTX, and provides a reliable platform for in silico hypothesis testing or recommendation on novel therapies. These results can support the development of RA translational research in the design of validation experiments and clinical trials, as such we identify GRB2 as a robust potential new target for RA for its ability to control both synovial degeneracy and dysbiosis, and, conversely, warn on the usage of IRAK4-inhibitors recently promoted, as this involves potential adverse effects in the form of impaired innate response to pathogens.
Rheumatoid arthritis (RA) is a multifaceted autoimmune, chronic and inflammatory disease with, to date, unclear etiology. As a consequence of its complexity, the definition of efficient and effective therapies remains a remarkable challenge due to the difficulties in controlling side effects and adverse events in relation to known (like genetic susceptibility, Stahl et al., 2010) and emergent (epigenomic factors, Nakano et al., 2012, dysbiosis, Scher and Abramson, 2011) RA-associated con-causes.
Recently, translational research has welcomed into medicine a number of novel perspectives. Among these, sequencing technologies (omic screens) and computational intensive approaches (systems biology) now coagulate into a practice where technology and mathematical modeling serve basic research in the production of selected hypotheses, which testing in vitro, in vivo and ultimately in clinical studies can support medical research and practice (Okada et al., 2014; You et al., 2014). The recent acknowledgment of the importance and complexity of the gut intestinal (GI) microbiome in the onset, progression and regression of RA (Scher and Abramson, 2011; Scher et al., 2012, 2013) and other autoimmune diseases, requires to incorporate the effects on the GI microbiome for any novel therapy. While protocols and medical best practice recommendations become mature in this direction, we propose the use of network approaches and omics from diverse origins (i.e., different biochemical districts/compartments/layers) including genomics, epigenomics, transcriptomics, post-transcriptomics, proteomics, and host-microbiome interface to GI metagenomics, to appropriately monitor the complexity of the disease. The novelty of the present work, therefore, lies not only in its application to RA, but also in the number of omic layers we have used, from genomic to proteomic and including the host-microbiome interface. These novelties allow to draw a single analytical picture of the fragmented molecular information available to date on RA, an easily consultable and extendable reference map for the researchers in the field, and—importantly—a systemic evaluation on the impact of a recently proposed RA therapeutic target (IRAK4), valuable per se and as an exemplar application of this approach. Overall, this work contributes to the general debate about data integration by offering details on our methodology, and to the area of complex inflammatory diseases, by providing specific examples of data choice and operational results.
The datasets used to construct the map are gathered from 13 different sources from databases and literature (Table 1). We included molecules experimentally associated to RA from manual curation of literature sources (core dataset, CD, 377 proteins, Data Sheet 1, Tables S1–S6), and additional molecules and pathways strongly yet not explicitly associated to RA (extended dataset, ED, 4709 proteins, Data Sheet 1, Tables S3A–E, S7–S13). A summary of all datasets and proteins' Uniprot IDs is provided in Data Sheet 1, Table S14. While the core set constitutes a more specific RA map, its extension offers a more systemic and practically usable map, notably in terms of the significance of the statistics that can be run on the extended map. The map presented here assembles genomic, epigenomic, transcriptomic, post-transcriptomic, proteomic, and host-microbiome interface data related to RA, as detailed below, and integrates such information at the functional level of protein-protein interactions (PPIs). The PPI framework is an assessed integrative approach (Hodgman, 2007; Dittrich et al., 2008; Jin et al., 2008; Kim et al., 2010; Iskar et al., 2012) that has already been used in computational biology to understand diseases' pathogenesis (Huang et al., 2009b); to implement tools for the interpretation of inferred gene and protein lists (Berger et al., 2007; Antonov et al., 2009); to prioritize cancer-associated genes (Wu et al., 2012); to predict functional linkages among genes (Lehner and Lee, 2008); to show the implication of protein networks topology in genetics, personal genomics, and therapy (Lee et al., 2013); to implement data integration workflows showcased in obstructive nephropathy in children (Moulos et al., 2011).
The CD is composed of 377 proteins retrieved from six data sources (Data Sheet 1, Tables S1–S6):
1) RA genome-wide association studies (GWAS) gathered and integrated from five different databases (BioGPS (Wu et al., 2009), HuGE (Yu et al., 2008), NHGRI, OMIM, PharmGKB (Klein et al., 2001); see Data Sheet 1, Table S1 for the specific query processes);
2) RA-associated proteins from the Universal Protein Resource (Uniprot) (Consortium, 2010), retrieved using as search parameters “rheumatoid arthritis” and “human” and then manually screened (Data Sheet 1, Table S2);
3) Genes and proteins retrieved from a comprehensive review of the literature, in particular genes appearing in Tables 1, 2 of Review (Mcinnes and Schett, 2011) and cited references (Data Sheet 1, Table S3);
4) Genes that show epigenetic changes in relation to RA, as specified in Trenkmann et al. (2010); Karouzakis et al. (2011) (Data Sheet 1, Table S4);
5) Proteins that are at the interface between the host and the oral microbiome, in particular proteins experimentally known to be differentially expressed in presence of Porphyromonas Gingivalis (Zhou and Amar, 2006), a periodontitis-causing bacterium that has been strongly linked to the insurgence of RA (Mikuls et al., 2012; Scher et al., 2012; Smit et al., 2012; Bingham and Moni, 2013; Ogrendik, 2013; Okada et al., 2013) (Data Sheet 1, Table S5);
6) The key elements of the NF-κB system, the master regulator of inflammation (Oeckinghaus et al., 2011; Smale, 2011; Hayden and Ghosh, 2012) at the center of a complex regulatory interactome (Tieri et al., 2012) prominently implicated in the onset and development of RA (Miagkov et al., 1998; Makarov, 2001; Feldmann et al., 2002; Okamoto, 2006; Roman-Blas and Jimenez, 2006, 2008; Simmonds and Foxwell, 2008; Van Loo and Beyaert, 2011): we included 16 “consensus” proteins that appear at the intersection of the three main NF-κB-related datasets described in Tieri et al. (2012) (Data Sheet 1, Table S6).
Table 2. RA-associated proteins significantly modified upon MTX therapy release and functional annotation clustering in DAVID.
The extended dataset (ED, that includes CD) is composed of 4709 proteins, which are involved in a broader sense in the onset and development of RA, such as proteins participating in signaling pathways or cascades of recognized importance for RA. This extension provides a more general setting for the molecular framing of RA, and offers a larger network to operate on, with more relevant statistics and analyses, giving account for contributions coming from entities that may have been neglected or that are not experimentally related to RA, but that participate to the inception of the disease. In addition to the proteins of the core dataset, we added eight main subsets, as follows (Data Sheet 1, Tables S3A–E, S7–S13):
3A-B-C-D-E) in retrieving data from Mcinnes and Schett (2011) and references cited there, we considered that some of the key proteins can be “hidden” inside the signaling pathways involved in the disease. In order to take into account such potentially important and usually neglected elements, we expanded subset 3 of CD by a pathway enrichment analysis process, using the genes listed in Mcinnes and Schett (2011) Tables 1, 2. To populate these five subsets, the selected genes have been input in the pathway over-representation analysis (ORA) tool of InnateDB, one of the most comprehensive sources of pathways data available (Lynn et al., 2008; Breuer et al., 2013). Pathway ORA has been performed on InnateDB using hypergeometric distribution for p-value computation and Benjamini–Hochberg correction method for multiple hypothesis testing. All the proteins participating to such over-represented pathways were then included. We retrieved respectively: 39 enriched pathways accounting for 1248 proteins (subset 3A), 14 pathways and 283 proteins (3B), 46 pathways and 1536 proteins (3C), 5 pathways and 472 proteins (3D), and 92 pathways and 1837 proteins (3E), all collected in Data Sheet 1, Tables S3A–E;
7) Genes derived from the transcriptional RA map in Wu et al. (2010) (Data Sheet 1, Table S7);
8) RA-related miRNA-regulated genes: experimentally validated target genes of all miRNAs that are associated to RA in the database miRWalk (Dweep et al., 2011) (search mode: holistic view of validated disease-miRNA interactions; web reference: http://www.umm.uni-heidelberg.de/apps/zmf/mirwalk/disease.html; query keywords: Arthritis AND Rheumatic diseases) (Data Sheet 1, Table S8);
9A,B) gene expression profiles of RA patients and healthy controls were searched on Gene Expression Omnibus (GEO, (Barrett et al., 2011) http://www.ncbi.nlm.nih.gov/geo/) with the query [“rheumatoid arthritis” AND “(synovi* OR blood)”] (i.e., in synovial tissue and/or blood). In order to include only highly consistent information, datasets without pre-treatment samples, with no details about the therapy and no raw data were filtered out. Human PBMCs collected and processed by Affymetrix technology were selected, leaving only one dataset out of the initial 61, GSE7524, which contains transcriptomic profiles of 2 healthy controls, 2 before and 2 after anti-TNFα treatment samples. Affymetrix Human Genome U133A Array was used to measure the expression levels of ~14,500 well-characterized human genes. The raw data were pre-processed using affy package (Gautier et al., 2004) in R (http://www.r-project.org/), normalized using robust multi-array average (rma) (Irizarry et al., 2003) and for multiple probes corresponding to the same gene, the probe with the highest standard variation across all samples was used to represent the gene. Differentially expressed genes [fold-change (Murie et al., 2009) =2] were identified with the comparison between the 2 healthy controls and the 2 before anti-TNFα treatment samples resulting in 646 genes differentially expressed, among which 440 genes (451 proteins) were down-regulated and 206 genes (210 proteins) were up-regulated (Data Sheet 1, Tables S9A,B);
10) Proteins related to the inflammasome, a multiprotein oligomer responsible for activation of inflammatory processes proteins, which is also known to be activated from the bacterium P. Gingivalis, among others, and recognized to play a relevant role in RA (Sidiropoulos et al., 2008; Kolly et al., 2010; Farquharson et al., 2012; Mathews et al., 2013) (Data Sheet 1, Table S10). This set was retrieved using ORA as described in 3A-B-C-D-E;
11) Adenosine receptors and related proteins, known to be involved in RA (Varani et al., 2010, 2011; Vincenzi et al., 2013) and possibly at the basis of the mechanism of action of methotrexate, first-line therapy for the treatment of RA (Stamp et al., 2012) (Data Sheet 1, Table S11). This set was retrieved using ORA as in 3A-B-C-D-E and 10;
12) The large family of G Protein Coupled Receptors (GPCRs) (Hutchings et al., 2010; Lozupone et al., 2012; Maynard et al., 2012; Tremaroli and Backhed, 2012), pertaining to host-microbiome interface proteins (grouped in a separate set from 13 due to their numerosity), retrieved from http://www.iuphar-db.org/DATABASE/ReceptorFamiliesForward?type=GPCR (Sharman et al., 2013) (Data Sheet 1, Table S12);
13) The set of host-microbiome interacting proteins, manually curated from recent reviews (Lozupone et al., 2012; Maynard et al., 2012; Tremaroli and Backhed, 2012), to describe the bridge between innate immunity (altered in RA) and the GI microbiome [known to be involved in immune diseases in general and in RA in particular (Scher and Abramson, 2011)]. Globally this dataset accounts for the Toll-like Receptor family (TLRs), the mucin proteins family, selected Immunoglobulins (Ig) and their receptors, among others (Data Sheet 1, Table S13).
Datasets are integrated at the PPI level as peers to avoid introducing any bias a priori in the network construction and to warrant that these data are connected in a biologically meaningful way. Protein-protein interactions were retrieved in Cytoscape from the Agile Protein Interaction DataAnalyzer database (APID, Prieto and De Las Rivas, 2006) that includes all known experimentally validated protein-protein interactions from BIND, BioGRID, DIP, HPRD, IntAct and MINT databases, accessed via the APID2NET (Hernandez-Toro et al., 2007) plugin. This process lead to the definitions of, respectively, the core interactome (CI, 303 proteins, 597 interactions, high resolution Image S1) and the extended interactome (EI, 3783 proteins, 24457 interactions, high resolution Image S2). Discussion on caveats and choices of original sources can be found in Tieri and Nardini (2013).
Topological analysis was run separately on the main connected component of each interactome (i.e., excluding the proteins for which no PPI was retrieved, i.e., that remained isolated) to evaluate a number of network parameters (Assenov et al., 2008): degree, or connectivity, i.e., the number of nodes linked to the node of interest (number of edges); and betweenness centrality (BC), a measure of the amount of control that a node exerts over the interactions of other nodes in the network. This measure favors nodes that join communities such as dense subnetworks, rather than nodes that lie inside a community, and has been shown to characterize essential proteins (Platzer et al., 2007). All calculated network parameters and rankings are listed in Data Sheet 2, Tables S15, S16 or can be recalculated from the Cytoscape CI_EI.cys (Data Sheet 3) file available at http://www.picb.ac.cn/ClinicalGenomicNTW/RAmultiomic.html.
To simulate the pharmacological treatment, a virtual node knockout experiment has been performed by controlling (manual removal of the nodes and Cytoscape plugin Interference (Scardoni et al., 2014) 20 MTX controlled targets identified in literature (Cutolo et al., 2001; Chan and Cronstein, 2002) present in EI (Data Sheet 2, Table S17). Betweenness centrality (and, to add robustness to the analysis, stress, S, i.e., an alternative centrality functional form) were then re-calculated to assess the impact of such therapy on the topology and hence the functionality of the network. Manual node removal and pharmacological simulation plugin present overlapping results (betweenness: 95.9%, stress: 98.2%, Data Sheet 2, Table S17). The p-values, corrected for multiple testing (threshold 0.05), have been calculated after constructing null betweenness centrality distributions by 1000 random deletions of 20 nodes, as many as the MTX targets (Efron and Tibshirani, 1993). Functional clustering analysis has been then performed (Data Sheet 2, Table S18).
We further run a comparative analysis between our newly constructed multi-omic map, EI, and TR, that represent an earlier transcriptional-only version (Wu et al., 2010), to highlight the biological mechanisms that have been better emphasized from the usage of multilayer omic data.
Degree was evaluated as the number of edges attached to a node for the undirected networks as EI (and CI) are (i.e., connections among nodes do not indicate directional cause-effect nor temporal relationship). For TR (directed network) proteins and their modified instances (such as MAPKs and phosphorylated-MAPKs) were first considered as one (complex) node, then in-degrees (edges to the node) and out-degrees (edges from the node) of the components (MAPK and phosphorylated-MAPK) were summed up to obtain the undirected degree, after subtracting the number of edges connecting the members of the complex node. To complete the compatibility of the degree defined for undirected maps (and namely EI), given the different sizes of EI and TR, the percentrank of the degree was also computed. The nodes which degree rank was modified by more than 10% between the two networks, were considered as nodes undergoing a transition. A node was defined as accomplished when its % rank degree was preserved, loser when the ranking reduced from TR to EI, climber when it increased from TR to EI (Data Sheet 2, Table S19). From a strictly topological point of view, the threshold that defines a node as accomplished can be set to zero, and hence this definition identifies only the nodes with the same exact degree. From a biological standpoint, and for an informative biological interpretation of the results, it is not necessary to impose the exact matching of the ranking. For this reason we relaxed the threshold and defined as accomplished the nodes that present the same, higher or lower % rank of the degree with ±10% tolerance, as a reasonable compromise.
Biological meaning for climbers and accomplished nodes in the transition TR to EI was assessed by enrichment analysis Enrichr (Chen et al., 2013) see Data Sheet 2, Table S20.
After curating all molecular information (Table 1) we inferred the network from the reconstructed lists with the PPI approach, which consists of connecting nodes (molecules) based on their interactions at the protein level, a broadly assessed approach in computational biology, and already used for RA in both already cited (Okada et al., 2014; You et al., 2014). All following results pertain to the analysis on the extended interactome (EI), more informative for its larger size.
To validate the ability of our network to model the biomolecular aspects of RA, we first simulated a therapeutic approach with MTX (see methods) and compared the results with the major known effects reported in literature (Figure 1A). As a result of the control on 20 MTX targets removal, the network changes its topology (Figure 1B; Data Sheet 2, Table S17), and the functional analysis indicates that 32 molecules which BC significantly altered (Data Sheet 2, Table S17, col. 2) pertain to two main functions [Data Sheet 2, Table S18, DAVID (Huang et al., 2009a)]: regulation of programmed cell death, a known effect of MTX (Spurlock et al., 2011); and metabolic and biosynthetic processes, an alteration known to constitute a side effect of the treatment (Phillips et al., 2003), as well as an area of synergy between host and microbiome (Tremaroli and Backhed, 2012; Devaraj et al., 2013; Winter et al., 2013). Moving down to the gene level, as illustrated in Table 2, Signal Transducers and Activators of Transcription 3 (STAT3) deserves particular attention, as it is a crucial player in the JAK/STAT signaling cascade, at the basis of the signal transduction mechanism for many cytokine receptors, highly activated in RA (Paunovic et al., 2008), and an important member of the host-microbiome interface (Zhou and Amar, 2006), being involved in the host susceptibility/defense against intestinal infections at the mucosal level (Miettinen et al., 2000).
Figure 1. (A) Snapshot of the extended interactome (EI) with nodes highlighted by betweenness centrality (BC), high resolution browsable figure provided in Supplementary Files (Image S2). (B) Zoom on the top ranking BC node (GRB2) and its closer interactome. Pathways relevant in the indication of GRB2 as an RA target, able to control inflammation TGF-β (TGFB1-3), TNF-α (TNF, TNFRS10C), MAPK (MAP4K1, MAPK3), degeneracy EMT (TWIST1-2, CDH1), and dysbiosis (TRL4) are also highlighted. (C) Visual summary of the influence of GRB2 on the RA-affected districts highlight a homeostatic (blue) influence on inflammation, GI microbiome, growth, differentiation. The pie-chart slices' size is proportional to the number of molecules considered in each district. Districts were merged from the total 13 datasets according to biochemical homogeneity in the following 8 categories: Genomic (DNA, Dataset 1); Epigenomic (mDNA, Dataset 4); Transcriptomic (mRNA, Datasets 7, 9A, 9B); Post-transcriptomic (miRNA, Dataset 8); Proteomic (proteins, Dataset 2); Microbiome (Host-microbiome proteins interface, Oral microbiome Datasets 5, 10, 12, 13); Inflammation (6, 3A, 3B, 3C); Others, i.e., Growth, Differentiation (Datasets 3, 11, 3D, 3E).
From a topological point of view, STAT3 presents enhanced betweenness and reduced stress centralities after virtual MTX treatment. This is an unusual topological condition—since there is commonly correlation between stress and betweenness—where, upon perturbation (MTX) a higher fraction of shortest paths converges on STAT3 (gain in betweenness centrality) despite a decrease in their absolute number (loss of stress centrality). This indicates that the networks shrinks and STAT3 becomes more important, a fact that can be translated in biological terms as the compensatory mechanisms induced by the loss of some molecules' presence/activity (MTX targets), which globally force STAT3 to become the molecule through which more numerous (higher betweenness) but less efficient molecular reactions (longer paths, lower stress) occur.
Overall, STAT3, which is already considered a crucial target in RA for its critical role in the T regulatory/helper 17 lymphoid cells [Treg/Th17 balance overabundant in RA (Leipe et al., 2010)] is coherently shown as an indirectly controlled target by MTX explaining the ability of the therapy to rebalance Th17/IL17 ratio (Li et al., 2012).
In conclusion, our map is able to recollect known and yet complex information about the effects of MTX, this represents an important validation of our frame for further simulations. Additionally, our map indicates a clear link between MTX and dysbiosis, which to date has not been explicitly unrevealed, although enterocolitis is a known toxic effect of MTX, linked to the induced nitroxidative stress (Kolli et al., 2008, 2013). This is a critical fact as the known adverse effects of MTX, generally described as immunodepressive, appear to be composed not only by the known oxidative organ stress, but also by an added dysbiosis, possibly mediated by an overload on STAT3.
The topological analysis highlights the striking relevance of Growth factor receptor-bound protein 2 (GRB2) with values of BC more than two-fold (Data Sheet 2, Table S16) compared to the second in rank, the Epidermal growth factor receptor (EGFR). Based on literature, GRB2 is an effective target (Phase I clinical trial, http://www.biopathholdings.com/) for Acute Myeloid Leukemia (AML), Chronic myelogenous leukemia (CML) and Myelodysplastic syndromes (MDS); an important mediator of the oncogenic activities of TGF-β, via epithelial mesenchymal transition (EMT) (Galliher-Beckley and Schiemann, 2008); a crucial player in the host-microbiome interaction of Helicobacter pylori, able to induce host cell scattering and proliferation via the activation of the Ras/MEK/ERK pathway (Mimuro et al., 2002); a marker of RA in synoviocites (Huh et al., 2003). GRB2 is additionally activated by leptin (Pai et al., 2005), abundant in RA (Bokarewa et al., 2003) and able to increase Prevotella intermedia LPS-induced TNF-α production (Kim, 2010). Moreover, another member of the Prevotella genus (P. copri) has recently been liaised to RA (Scher et al., 2013), as a specific marker of GI microbiome dysbiosis associated to the disease. When observed from the network perspective this apparently scattered information fits in a connected map (Figure 1B) and hence builds a robust rationale for considering GRB2 as a target for RA. The activation of proliferative and inflammatory pathways as well as EMT, are hallmarks of RA (You et al., 2014) suggesting that the control on GRB2 as a regulator of such mechanisms is appropriate. Additionally, the control on GRB2 exerted by H. pylori [already proposed in relation to RA (Melby et al., 1999)] and by P. intermedia in the presence of leptin indicate that targeting of GRB2 is not only of relevance to control the phenotypic symptoms of RA (joints degeneracy) but also the recently highlighted dysbiosis that accompany the disease, via the control of the disruptive mechanisms by which pathogens can exert their action on the host (Figure 1C).
Given the relevance of RA as a paradigmatic autoimmune disease, a variety of in silico modeling approaches have been devised (Okada et al., 2014; You et al., 2014), and, among those, an early transcriptional only map (hereinafter TR, 302 nodes; Wu et al., 2010). The previous compilation of this simplified version put us in the relatively unique position to be able to quantify the benefit, in terms of information content, of expanding from transcriptional to multi-omic the network modeling of RA. The molecules that gain importance (i.e., have a higher degree) in the multi-omic map versus the TR (climbers, see Methods and Figure 2A) pertain mostly to the MAPK Signaling Pathway (Figure 2B and Data Sheet 2, Table S19). This category is also highly enriched for accomplished nodes, thus validating the importance of this pathway in the disease. However, climbers, all representing genes shared between TR and EI, include molecules known to belong also to the GI interface (SFR, MAP2K4, MAP3K8), absent in the accomplished, implying the importance of the involvement of the host-microbiome interface, not taken into account in the TR map. In particular, Interleukin-1 Receptor Associated Kinase-4 (IRAK4, climber) is known to play a critical role in initiating response to foreign pathogens (Hofman and Vouret-Craviari, 2012) and was recently presented to the American College of Rheumatology (ACR), based on promising results on the control of B-cell-like diffuse large B-cell lymphoma (DLBCL), as a potential treatment for RA (Chaudahry and Al, 2012). In the network perspective, this choice calls for words of cautions. Indeed, while correlating with regression of some aspects of the disease, the control on IRAK4 affects the response to pathogens, and in particular IRAK4 inhibitors impacts on pDCs in RA patients (Chiang et al., 2011), therefore limiting the appropriate and immediate innate host response in case of bacterial infections (Figure 2C).
Figure 2. (A) Multi-omic map (EI) nodes highlighted according to their role in comparison with a transcriptional-only map (TR). In orange, nodes that maintain their role and importance in both EI and TR (accomplished); in red, nodes that gain importance in the multi-omic context, (climbers). (B) Functional analysis of the climber hubs, which highlight the striking significance of MAPK signals. Panel (C) is built in the same way of Figure 1C to permit easy comparison of the two targets. It represents the summary of the influence of IRAK4 on the RA-affected districts, and highlights a homeostatic (blue) influence on inflammation, growth, differentiation as well as transcriptomic and post-transcriptomic districts. However, the microbiome interface response is impaired by IRAK4 inhibition of the innate immune response to pathogens. The pie-chart slices' size is proportional to the number of molecules considered in each district (as in Figure 1).
The aim of the designed framework is to draw hypotheses that can support basic research and further clinical practice. In particular, we here highlight two major areas of application: support in the identification of novel drug targets (exemplified by GRB2); support in the identification of potential contraindication to novel therapies, i.e., support in the design of robust clinical trials (exemplified by IRAK4-inhibitors). While the former application joins other efforts in different clinical areas [such as on diabetes (Liu et al., 2007; Santiago and Potashkin, 2013), in cancer (Hwang et al., 2013), and on glioblastoma (Junhua et al., 2012)], the latter descends from the inclusion of numerous data types, including for the first time to our knowledge, the GI microbiome interface. The results discussed in this article are the output of the knowledge distilled from ~4000 selected molecules and ~15 public databases, a humongous amount of information carefully and often redundantly peer-reviewed by the scientific community. Future and ongoing research and the resulting discoveries will impact on the breadth and possibly on the topology of our map. To take into account these expected (and desirable) events, our map was drawn using open source programs and pathway molecules' standards to allow full map usability, editing and updating by the whole scientific community.
Paolo Tieri built and analyzed the map; XiaoYuan Zhou performed pharmacological simulation; XiaoYuan Zhou and Lisha Zhu run functional and comparative analyses; Christine Nardini analyzed the connection to the GI microbiome; Paolo Tieri and Christine Nardini designed the study, analyzed the results and wrote the manuscript; XiaoYuan Zhou and Lisha Zhu contributed to write and revise the manuscript.
All data are available publicly, our map is publicly available here: http://www.picb.ac.cn/ClinicalGenomicNTW/RAmultiomic.html
The authors declare that the research was conducted in the absence of any commercial or financial relationships that could be construed as a potential conflict of interest.
This project is funded by MoST international cooperation program n. 2013DFA30790, and NSFC n. 31070748, PT collaborated in the frame of the European Commission FP7-PEOPLE-2011-IRSES program 31028760, project ID 294935 “KEPAMOD,” and as CAS fellow grant n. 2011Y1SA04.
The Supplementary Material for this article can be found online at: http://www.frontiersin.org/journal/10.3389/fcell.2014.00059/abstract
Antonov, A. V., Dietmann, S., Rodchenkov, I., and Mewes, H. W. (2009). PPI spider: a tool for the interpretation of proteomics data in the context of protein-protein interaction networks. Proteomics 9, 2740–2749. doi: 10.1002/pmic.200800612
Pubmed Abstract | Pubmed Full Text | CrossRef Full Text | Google Scholar
Assenov, Y., Ramírez, F., Schelhorn, S. E., Lengauer, T., and Albrecht, M. (2008). Computing topological parameters of biological networks. Bioinformatics 24, 282–284. doi: 10.1093/bioinformatics/btm554
Pubmed Abstract | Pubmed Full Text | CrossRef Full Text | Google Scholar
Barrett, T., Troup, D. B., Wilhite, S. E., Ledoux, P., Evangelista, C., Kim, I. F., et al. (2011). NCBI GEO: archive for functional genomics data sets–10 years on. Nucleic Acids Res. 39, D1005–D1010. doi: 10.1093/nar/gkq1184
Pubmed Abstract | Pubmed Full Text | CrossRef Full Text | Google Scholar
Berger, S. I., Posner, J. M., and Ma'ayan, A. (2007). Genes2Networks: connecting lists of gene symbols using mammalian protein interactions databases. BMC Bioinformatics 8:372. doi: 10.1186/1471-2105-8-372
Pubmed Abstract | Pubmed Full Text | CrossRef Full Text | Google Scholar
Bingham, C. O. 3rd., and Moni, M. (2013). Periodontal disease and rheumatoid arthritis: the evidence accumulates for complex pathobiologic interactions. Curr. Opin. Rheumatol. 25, 345–353. doi: 10.1097/BOR.0b013e32835fb8ec
Pubmed Abstract | Pubmed Full Text | CrossRef Full Text | Google Scholar
Bokarewa, M., Bokarew, D., Hultgren, O., and Tarkowski, A. (2003). Leptin consumption in the inflamed joints of patients with rheumatoid arthritis. Ann. Rheum. Dis. 62, 952–956. doi: 10.1136/ard.62.10.952
Pubmed Abstract | Pubmed Full Text | CrossRef Full Text | Google Scholar
Breuer, K., Foroushani, A. K., Laird, M. R., Chen, C., Sribnaia, A., Lo, R., et al. (2013). InnateDB: systems biology of innate immunity and beyond–recent updates and continuing curation. Nucleic Acids Res. 41, D1228–D1233. doi: 10.1093/nar/gks1147
Pubmed Abstract | Pubmed Full Text | CrossRef Full Text | Google Scholar
Chan, E. S., and Cronstein, B. N. (2002). Molecular action of methotrexate in inflammatory diseases. Arthritis Res. 4, 266–273. doi: 10.1186/ar419
Pubmed Abstract | Pubmed Full Text | CrossRef Full Text | Google Scholar
Chaudahry, D., and Al, E. (2012). “Identification of highly potent and selective Interleukin-1 receptor-associated kinase-4 inhibitor for the treatmetn of rheumatic diseases,” in American College of Rheumatology (ACR) Annual Scientific Meeting (Washington, DC).
Chen, E. Y., Tan, C. M., Kou, Y., Duan, Q., Wang, Z., Meirelles, G. V., et al. (2013). Enrichr: interactive and collaborative HTML5 gene list enrichment analysis tool. BMC Bioinformatics 14:128. doi: 10.1186/1471-2105-14-128
Pubmed Abstract | Pubmed Full Text | CrossRef Full Text | Google Scholar
Chiang, E. Y., Yu, X., and Grogan, J. L. (2011). Immune complex-mediated cell activation from systemic lupus erythematosus and rheumatoid arthritis patients elaborate different requirements for IRAK1/4 kinase activity across human cell types. J. Immunol. 186, 1279–1288. doi: 10.4049/jimmunol.1002821
Pubmed Abstract | Pubmed Full Text | CrossRef Full Text | Google Scholar
Consortium, U. (2010). The Universal Protein Resource (UniProt) in 2010. Nucleic Acids Res. 38, D142–D148. doi: 10.1093/nar/gkp846
Pubmed Abstract | Pubmed Full Text | CrossRef Full Text | Google Scholar
Cutolo, M., Sulli, A., Pizzorni, C., Seriolo, B., and Straub, R. H. (2001). Anti-inflammatory mechanisms of methotrexate in rheumatoid arthritis. Ann. Rheum. Dis. 60, 729–735. doi: 10.1136/ard.60.8.729
Pubmed Abstract | Pubmed Full Text | CrossRef Full Text | Google Scholar
Devaraj, S., Hemarajata, P., and Versalovic, J. (2013). The human gut microbiome and body metabolism: implications for obesity and diabetes. Clin. Chem. 59, 617–628. doi: 10.1373/clinchem.2012.187617
Pubmed Abstract | Pubmed Full Text | CrossRef Full Text | Google Scholar
Dittrich, M. T., Klau, G. W., Rosenwald, A., Dandekar, T., and Müller, T. (2008). Identifying functional modules in protein-protein interaction networks: an integrated exact approach. Bioinformatics 24, i223–i231. doi: 10.1093/bioinformatics/btn161
Pubmed Abstract | Pubmed Full Text | CrossRef Full Text | Google Scholar
Dweep, H., Sticht, C., Pandey, P., and Gretz, N. (2011). miRWalk–database: prediction of possible miRNA binding sites by “walking” the genes of three genomes. J. Biomed. Inform. 44, 839–847. doi: 10.1016/j.jbi.2011.05.002
Pubmed Abstract | Pubmed Full Text | CrossRef Full Text | Google Scholar
Efron, B., and Tibshirani, R. (1993). An Introduction to the Bootstrap. New York, NY: Chapman & Hall. doi: 10.1007/978-1-4899-4541-9
Farquharson, D., Butcher, J. P., and Culshaw, S. (2012). Periodontitis, Porphyromonas, and the pathogenesis of rheumatoid arthritis. Mucosal Immunol. 5, 112–120. doi: 10.1038/mi.2011.66
Pubmed Abstract | Pubmed Full Text | CrossRef Full Text | Google Scholar
Feldmann, M., Andreakos, E., Smith, C., Bondeson, J., Yoshimura, S., Kiriakidis, S., et al. (2002). Is NF-kappaB a useful therapeutic target in rheumatoid arthritis? Ann. Rheum. Dis. 61(Suppl. 2), ii13–ii18. doi: 10.1136/ard.61.suppl_2.ii13
Pubmed Abstract | Pubmed Full Text | CrossRef Full Text | Google Scholar
Galliher-Beckley, A. J., and Schiemann, W. P. (2008). Grb2 binding to Tyr284 in TbetaR-II is essential for mammary tumor growth and metastasis stimulated by TGF-beta. Carcinogenesis 29, 244–251. doi: 10.1093/carcin/bgm245
Pubmed Abstract | Pubmed Full Text | CrossRef Full Text | Google Scholar
Gautier, L., Cope, L., Bolstad, B. M., and Irizarry, R. A. (2004). Affy–analysis of affymetrix genechip data at the probe level. Bioinformatics 20, 307–315. doi: 10.1093/bioinformatics/btg405
Pubmed Abstract | Pubmed Full Text | CrossRef Full Text | Google Scholar
Hayden, M. S., and Ghosh, S. (2012). NF-kappaB, the first quarter-century: remarkable progress and outstanding questions. Genes Dev. 26, 203–234. doi: 10.1101/gad.183434.111
Pubmed Abstract | Pubmed Full Text | CrossRef Full Text | Google Scholar
Hernandez-Toro, J., Prieto, C., and De Las Rivas, J. (2007). APID2NET: unified interactome graphic analyzer. Bioinformatics 23, 2495–2497. doi: 10.1093/bioinformatics/btm373
Pubmed Abstract | Pubmed Full Text | CrossRef Full Text | Google Scholar
Hodgman, C. (2007). Integrative biology–the way forward. Brief. Bioinform. 8, 208–209. doi: 10.1093/bib/bbm036
Pubmed Abstract | Pubmed Full Text | CrossRef Full Text | Google Scholar
Hofman, P., and Vouret-Craviari, V. (2012). Microbes-induced EMT at the crossroad of inflammation and cancer. Gut Microbes 3, 176–185. doi: 10.4161/gmic.20288
Pubmed Abstract | Pubmed Full Text | CrossRef Full Text | Google Scholar
Huang, D. W., Sherman, B. T., and Lempicki, R. A. (2009a). Systematic and integrative analysis of large gene lists using DAVID bioinformatics resources. Nat. Protoc. 4, 44–57. doi: 10.1038/nprot.2008.211
Pubmed Abstract | Pubmed Full Text | CrossRef Full Text | Google Scholar
Huang, W., Wang, P., Liu, Z., and Zhang, L. (2009b). Identifying disease associations via genome-wide association studies. BMC Bioinformatics 10(Suppl. 1):S68. doi: 10.1186/1471-2105-10-S1-S68
Pubmed Abstract | Pubmed Full Text | CrossRef Full Text | Google Scholar
Huh, S. J., Paik, D. J., Chung, H. S., and Youn, J. (2003). Regulation of GRB2 and FLICE2 expression by TNF-alpha in rheumatoid synovium. Immunol. Lett. 90, 93–96. doi: 10.1016/j.imlet.2003.07.002
Pubmed Abstract | Pubmed Full Text | CrossRef Full Text | Google Scholar
Hutchings, C. J., Koglin, M., and Marshall, F. H. (2010). Therapeutic antibodies directed at G protein-coupled receptors. MAbs 2, 594–606. doi: 10.4161/mabs.2.6.13420
Pubmed Abstract | Pubmed Full Text | CrossRef Full Text | Google Scholar
Hwang, T. H., Atluri, G., Kuang, R., Kumar, V., Starr, T., Silverstein, K. A., et al. (2013). Large-scale integrative network-based analysis identifies common pathways disrupted by copy number alterations across cancers. BMC Genomics 14:440. doi: 10.1186/1471-2164-14-440
Pubmed Abstract | Pubmed Full Text | CrossRef Full Text | Google Scholar
Irizarry, R. A., Bolstad, B. M., Collin, F., Cope, L. M., Hobbs, B., and Speed, T. P. (2003). Summaries of affymetrix genechip probe level data. Nucleic Acids Res. 31, e15. doi: 10.1093/nar/gng015
Pubmed Abstract | Pubmed Full Text | CrossRef Full Text | Google Scholar
Iskar, M., Zeller, G., Zhao, X. M., Van Noort, V., and Bork, P. (2012). Drug discovery in the age of systems biology: the rise of computational approaches for data integration. Curr. Opin. Biotechnol. 23, 609–616. doi: 10.1016/j.copbio.2011.11.010
Pubmed Abstract | Pubmed Full Text | CrossRef Full Text | Google Scholar
Jin, G., Zhou, X., Wang, H., Zhao, H., Cui, K., Zhang, X. S., et al. (2008). The knowledge-integrated network biomarkers discovery for major adverse cardiac events. J. Proteome Res. 7, 4013–4021. doi: 10.1021/pr8002886
Pubmed Abstract | Pubmed Full Text | CrossRef Full Text | Google Scholar
Junhua, Z., Shihua, Z., Yong, W., Junfei, Z., and Xiang-Sun, Z. (2012). “Identifying mutated core modules in glioblastoma by integrative network analysis,” in Systems Biology (ISB), 2012 IEEE 6th International Conference (Xi'an), 304–309.
Karouzakis, E., Gay, R. E., Gay, S., and Neidhart, M. (2011). Epigenetic deregulation in rheumatoid arthritis. Adv. Exp. Med. Biol. 711, 137–149. doi: 10.1007/978-1-4419-8216-2_10
Pubmed Abstract | Pubmed Full Text | CrossRef Full Text | Google Scholar
Kim, S. J. (2010). Leptin potentiates prevotella intermedia lipopolysaccharide-induced production of TNF-alpha in monocyte-derived macrophages. J. Periodontal Implant Sci. 40, 119–124. doi: 10.5051/jpis.2010.40.3.119
Pubmed Abstract | Pubmed Full Text | CrossRef Full Text | Google Scholar
Kim, T. Y., Kim, H. U., and Lee, S. Y. (2010). Data integration and analysis of biological networks. Curr. Opin. Biotechnol. 21, 78–84. doi: 10.1016/j.copbio.2010.01.003
Pubmed Abstract | Pubmed Full Text | CrossRef Full Text | Google Scholar
Klein, T. E., Chang, J. T., Cho, M. K., Easton, K. L., Fergerson, R., Hewett, M., et al. (2001). Integrating genotype and phenotype information: an overview of the PharmGKB project. Pharmacogenetics research network and knowledge base. Pharmacogenomics J. 1, 167–170. doi: 10.1038/sj.tpj.6500035
Pubmed Abstract | Pubmed Full Text | CrossRef Full Text | Google Scholar
Kolli, V. K., Abraham, P., and Rabi, S. (2008). Methotrexate-induced nitrosative stress may play a critical role in small intestinal damage in the rat. Arch. Toxicol. 82, 763–770. doi: 10.1007/s00204-008-0287-9
Pubmed Abstract | Pubmed Full Text | CrossRef Full Text | Google Scholar
Kolli, V. K., Kanakasabapathy, I., Faith, M., Ramamoorthy, H., Isaac, B., Natarajan, K., et al. (2013). A preclinical study on the protective effect of melatonin against methotrexate-induced small intestinal damage: effect mediated by attenuation of nitrosative stress, protein tyrosine nitration, and PARP activation. Cancer Chemother. Pharmacol. 71, 1209–1218. doi: 10.1007/s00280-013-2115-z
Kolly, L., Busso, N., Palmer, G., Talabot-Ayer, D., Chobaz, V., and So, A. (2010). Expression and function of the NALP3 inflammasome in rheumatoid synovium. Immunology 129, 178–185. doi: 10.1111/j.1365-2567.2009.03174.x
Pubmed Abstract | Pubmed Full Text | CrossRef Full Text | Google Scholar
Lee, Y., Li, H., Li, J., Rebman, E., Achour, I., Regan, K. E., et al. (2013). Network models of genome-wide association studies uncover the topological centrality of protein interactions in complex diseases. J. Am. Med. Inform. Assoc. 20, 619–629. doi: 10.1136/amiajnl-2012-001519
Pubmed Abstract | Pubmed Full Text | CrossRef Full Text | Google Scholar
Lehner, B., and Lee, I. (2008). Network-guided genetic screening: building, testing and using gene networks to predict gene function. Brief. Funct. Genomic Proteomic 7, 217–227. doi: 10.1093/bfgp/eln020
Pubmed Abstract | Pubmed Full Text | CrossRef Full Text | Google Scholar
Leipe, J., Grunke, M., Dechant, C., Reindl, C., Kerzendorf, U., Schulze-Koops, H., et al. (2010). Role of Th17 cells in human autoimmune arthritis. Arthritis Rheum. 62, 2876–2885. doi: 10.1002/art.27622
Pubmed Abstract | Pubmed Full Text | CrossRef Full Text | Google Scholar
Li, Y., Jiang, L., Zhang, S., Yin, L., Ma, L., He, D., et al. (2012). Methotrexate attenuates the Th17/IL-17 levels in peripheral blood mononuclear cells from healthy individuals and RA patients. Rheumatol. Int. 32, 2415–2422. doi: 10.1007/s00296-011-1867-1
Pubmed Abstract | Pubmed Full Text | CrossRef Full Text | Google Scholar
Liu, M., Liberzon, A., Kong, S. W., Lai, W. R., Park, P. J., Kohane, I. S., et al. (2007). Network-based analysis of affected biological processes in type 2 diabetes models. PLoS Genet. 3:e96. doi: 10.1371/journal.pgen.0030096
Pubmed Abstract | Pubmed Full Text | CrossRef Full Text | Google Scholar
Lozupone, C. A., Stombaugh, J. I., Gordon, J. I., Jansson, J. K., and Knight, R. (2012). Diversity, stability and resilience of the human gut microbiota. Nature 489, 220–230. doi: 10.1038/nature11550
Pubmed Abstract | Pubmed Full Text | CrossRef Full Text | Google Scholar
Lynn, D. J., Winsor, G. L., Chan, C., Richard, N., Laird, M. R., Barsky, A., et al. (2008). InnateDB: facilitating systems-level analyses of the mammalian innate immune response. Mol. Syst. Biol. 4, 218. doi: 10.1038/msb.2008.55
Pubmed Abstract | Pubmed Full Text | CrossRef Full Text | Google Scholar
Makarov, S. S. (2001). NF-kappa B in rheumatoid arthritis: a pivotal regulator of inflammation, hyperplasia, and tissue destruction. Arthritis Res. 3, 200–206. doi: 10.1186/ar300
Pubmed Abstract | Pubmed Full Text | CrossRef Full Text | Google Scholar
Mathews, R. J., Robinson, J. I., Battellino, M., Wong, C., Taylor, J. C., Eyre, S., et al. (2013). Evidence of NLRP3-inflammasome activation in rheumatoid arthritis (RA); genetic variants within the NLRP3-inflammasome complex in relation to susceptibility to RA and response to anti-TNF treatment. Ann. Rheum. Dis. 73, 1202–1210. doi: 10.1136/annrheumdis-2013-203276
Maynard, C. L., Elson, C. O., Hatton, R. D., and Weaver, C. T. (2012). Reciprocal interactions of the intestinal microbiota and immune system. Nature 489, 231–241. doi: 10.1038/nature11551
Pubmed Abstract | Pubmed Full Text | CrossRef Full Text | Google Scholar
Mcinnes, I. B., and Schett, G. (2011). The pathogenesis of rheumatoid arthritis. N. Engl. J. Med. 365, 2205–2219. doi: 10.1056/NEJMra1004965
Pubmed Abstract | Pubmed Full Text | CrossRef Full Text | Google Scholar
Melby, K. K., Kvien, T. K., and Glennas, A. (1999). Helicobacter pylori–a trigger of reactive arthritis? Infection 27, 252–255. doi: 10.1007/s150100050022
Pubmed Abstract | Pubmed Full Text | CrossRef Full Text | Google Scholar
Miagkov, A. V., Kovalenko, D. V., Brown, C. E., Didsbury, J. R., Cogswell, J. P., Stimpson, S. A., et al. (1998). NF-kappaB activation provides the potential link between inflammation and hyperplasia in the arthritic joint. Proc. Natl. Acad. Sci. U.S.A. 95, 13859–13864. doi: 10.1073/pnas.95.23.13859
Pubmed Abstract | Pubmed Full Text | CrossRef Full Text | Google Scholar
Miettinen, M., Lehtonen, A., Julkunen, I., and Matikainen, S. (2000). Lactobacilli and streptococci activate NF-kappa B and STAT signaling pathways in human macrophages. J. Immunol. 164, 3733–3740. doi: 10.4049/jimmunol.164.7.3733
Pubmed Abstract | Pubmed Full Text | CrossRef Full Text | Google Scholar
Mikuls, T. R., Thiele, G. M., Deane, K. D., Payne, J. B., O'dell, J. R., Yu, F., et al. (2012). Porphyromonas gingivalis and disease-related autoantibodies in individuals at increased risk of rheumatoid arthritis. Arthritis Rheum. 64, 3522–3530. doi: 10.1002/art.34595
Pubmed Abstract | Pubmed Full Text | CrossRef Full Text | Google Scholar
Mimuro, H., Suzuki, T., Tanaka, J., Asahi, M., Haas, R., and Sasakawa, C. (2002). Grb2 is a key mediator of Helicobacter pylori CagA protein activities. Mol. Cell 10, 745–755. doi: 10.1016/S1097-2765(02)00681-0
Pubmed Abstract | Pubmed Full Text | CrossRef Full Text | Google Scholar
Moulos, P., Valavanis, I., Klein, J., Maglogiannis, I., Schanstra, J., and Chatziioannou, A. (2011). Unifying the integration, analysis and interpretation of multi-omic datasets: exploration of the disease networks of obstructive nephropathy in children. Conf. Proc. IEEE Eng. Med. Biol. Soc. 2011, 3716–3719. doi: 10.1109/IEMBS.2011.6090631
Pubmed Abstract | Pubmed Full Text | CrossRef Full Text | Google Scholar
Murie, C., Woody, O., Lee, A. Y., and Nadon, R. (2009). Comparison of small n statistical tests of differential expression applied to microarrays. BMC Bioinformatics 10:45. doi: 10.1186/1471-2105-10-45
Pubmed Abstract | Pubmed Full Text | CrossRef Full Text | Google Scholar
Nakano, K., Whitaker, J. W., Boyle, D. L., Wang, W., and Firestein, G. S. (2012). DNA methylome signature in rheumatoid arthritis. Ann. Rheum. Dis. 72, 110–117. doi: 10.1136/annrheumdis-2012-201526
Pubmed Abstract | Pubmed Full Text | CrossRef Full Text | Google Scholar
Oeckinghaus, A., Hayden, M. S., and Ghosh, S. (2011). Crosstalk in NF-κB signaling pathways. Nat. Immunol. 12, 695–708. doi: 10.1038/ni.2065
Pubmed Abstract | Pubmed Full Text | CrossRef Full Text | Google Scholar
Ogrendik, M. (2013). Rheumatoid arthritis is an autoimmune disease caused by periodontal pathogens. Int. J. Gen. Med. 6, 383–386. doi: 10.2147/IJGM.S45929
Pubmed Abstract | Pubmed Full Text | CrossRef Full Text | Google Scholar
Okada, M., Kobayashi, T., Ito, S., Yokoyama, T., Abe, A., Murasawa, A., et al. (2013). Periodontal treatment decreases levels of antibodies to Porphyromonas gingivalis and citrulline in patients with rheumatoid arthritis and periodontitis. J. Periodontol. 84, 74–84. doi: 10.1902/jop.2013.130079
Pubmed Abstract | Pubmed Full Text | CrossRef Full Text | Google Scholar
Okada, Y., Wu, D., Trynka, G., Raj, T., Terao, C., Ikari, K., et al. (2014). Genetics of rheumatoid arthritis contributes to biology and drug discovery. Nature 506, 376–381. doi: 10.1038/nature12873
Pubmed Abstract | Pubmed Full Text | CrossRef Full Text | Google Scholar
Okamoto, T. (2006). NF-kappaB and rheumatic diseases. Endocr. Metab. Immune Disord. Drug Targets 6, 359–372. doi: 10.2174/187153006779025685
Pubmed Abstract | Pubmed Full Text | CrossRef Full Text | Google Scholar
Pai, R., Lin, C., Tran, T., and Tarnawski, A. (2005). Leptin activates STAT and ERK2 pathways and induces gastric cancer cell proliferation. Biochem. Biophys. Res. Commun. 331, 984–992. doi: 10.1016/j.bbrc.2005.03.236
Pubmed Abstract | Pubmed Full Text | CrossRef Full Text | Google Scholar
Paunovic, V., Carroll, H. P., Vandenbroeck, K., and Gadina, M. (2008). Signalling, inflammation and arthritis: crossed signals: the role of interleukin (IL)-12, -17, -23 and -27 in autoimmunity. Rheumatology (Oxford) 47, 771–776. doi: 10.1093/rheumatology/kem352
Pubmed Abstract | Pubmed Full Text | CrossRef Full Text | Google Scholar
Phillips, D. C., Woollard, K. J., and Griffiths, H. R. (2003). The anti-inflammatory actions of methotrexate are critically dependent upon the production of reactive oxygen species. Br. J. Pharmacol. 138, 501–511. doi: 10.1038/sj.bjp.0705054
Pubmed Abstract | Pubmed Full Text | CrossRef Full Text | Google Scholar
Platzer, A., Perco, P., Lukas, A., and Mayer, B. (2007). Characterization of protein-interaction networks in tumors. BMC Bioinformatics 8:224. doi: 10.1186/1471-2105-8-224
Pubmed Abstract | Pubmed Full Text | CrossRef Full Text | Google Scholar
Prieto, C., and De Las Rivas, J. (2006). APID: agile protein interaction dataanalyzer. Nucleic Acids Res. 34, W298–W302. doi: 10.1093/nar/gkl128
Pubmed Abstract | Pubmed Full Text | CrossRef Full Text | Google Scholar
Roman-Blas, J. A., and Jimenez, S. A. (2006). NF-kappaB as a potential therapeutic target in osteoarthritis and rheumatoid arthritis. Osteoarthritis Cartilage 14, 839–848. doi: 10.1016/j.joca.2006.04.008
Pubmed Abstract | Pubmed Full Text | CrossRef Full Text | Google Scholar
Roman-Blas, J. A., and Jimenez, S. A. (2008). Targeting NF-kappaB: a promising molecular therapy in inflammatory arthritis. Int. Rev. Immunol. 27, 351–374. doi: 10.1080/08830180802295740
Pubmed Abstract | Pubmed Full Text | CrossRef Full Text | Google Scholar
Santiago, J. A., and Potashkin, J. A. (2013). Integrative network analysis unveils convergent molecular pathways in parkinson's disease and diabetes. PLoS ONE 8:e83940. doi: 10.1371/journal.pone.0083940
Pubmed Abstract | Pubmed Full Text | CrossRef Full Text | Google Scholar
Scardoni, G., Montresor, A., Tosadori, G., and Laudanna, C. (2014). Node interference and robustness: performing virtual knock-out experiments on biological networks: the case of leukocyte integrin activation network. PLoS ONE 9:e88938. doi: 10.1371/journal.pone.0088938
Pubmed Abstract | Pubmed Full Text | CrossRef Full Text | Google Scholar
Scher, J. U., and Abramson, S. B. (2011). The microbiome and rheumatoid arthritis. Nat. Rev. Rheumatol. 7, 569–578. doi: 10.1038/nrrheum.2011.121
Pubmed Abstract | Pubmed Full Text | CrossRef Full Text | Google Scholar
Scher, J. U., Sczesnak, A., Longman, R. S., Segata, N., Ubeda, C., Bielski, C., et al. (2013). Expansion of intestinal Prevotella copri correlates with enhanced susceptibility to arthritis. Elife 2:e01202. doi: 10.7554/eLife.01202
Pubmed Abstract | Pubmed Full Text | CrossRef Full Text | Google Scholar
Scher, J. U., Ubeda, C., Equinda, M., Khanin, R., Buischi, Y., Viale, A., et al. (2012). Periodontal disease and the oral microbiota in new-onset rheumatoid arthritis. Arthritis Rheum. 64, 3083–3094. doi: 10.1002/art.34539
Pubmed Abstract | Pubmed Full Text | CrossRef Full Text | Google Scholar
Sharman, J. L., Benson, H. E., Pawson, A. J., Lukito, V., Mpamhanga, C. P., Bombail, V., et al. (2013). IUPHAR-DB: updated database content and new features. Nucleic Acids Res. 41, D1083–D1088. doi: 10.1093/nar/gks960
Pubmed Abstract | Pubmed Full Text | CrossRef Full Text | Google Scholar
Sidiropoulos, P. I., Goulielmos, G., Voloudakis, G. K., Petraki, E., and Boumpas, D. T. (2008). Inflammasomes and rheumatic diseases: evolving concepts. Ann. Rheum. Dis. 67, 1382–1389. doi: 10.1136/ard.2007.078014
Pubmed Abstract | Pubmed Full Text | CrossRef Full Text | Google Scholar
Simmonds, R. E., and Foxwell, B. M. (2008). Signalling, inflammation and arthritis: NF-kappaB and its relevance to arthritis and inflammation. Rheumatology (Oxford) 47, 584–590. doi: 10.1093/rheumatology/kem298
Pubmed Abstract | Pubmed Full Text | CrossRef Full Text | Google Scholar
Smale, S. T. (2011). Hierarchies of NF-κB target-gene regulation. Nat. Immunol. 12, 689–694. doi: 10.1038/ni.2070
Pubmed Abstract | Pubmed Full Text | CrossRef Full Text | Google Scholar
Smit, M. D., Westra, J., Vissink, A., Doornbos-Van Der Meer, B., Brouwer, E., and Van Winkelhoff, A. J. (2012). Periodontitis in established rheumatoid arthritis patients: a cross-sectional clinical, microbiological and serological study. Arthritis Res. Ther. 14, R222. doi: 10.1186/ar4061
Pubmed Abstract | Pubmed Full Text | CrossRef Full Text | Google Scholar
Spurlock, C. F. 3rd., Aune, Z. T., Tossberg, J. T., Collins, P. L., Aune, J. P., Huston, J. W. 3rd., et al. (2011). Increased sensitivity to apoptosis induced by methotrexate is mediated by JNK. Arthritis Rheum. 63, 2606–2616. doi: 10.1002/art.30457
Pubmed Abstract | Pubmed Full Text | CrossRef Full Text | Google Scholar
Stahl, E. A., Raychaudhuri, S., Remmers, E. F., Xie, G., Eyre, S., Thomson, B. P., et al. (2010). Genome-wide association study meta-analysis identifies seven new rheumatoid arthritis risk loci. Nat. Genet. 42, 508–514. doi: 10.1038/ng.582
Pubmed Abstract | Pubmed Full Text | CrossRef Full Text | Google Scholar
Stamp, L. K., Hazlett, J., Roberts, R. L., Frampton, C., Highton, J., and Hessian, P. A. (2012). Adenosine receptor expression in rheumatoid synovium: a basis for methotrexate action. Arthritis Res. Ther. 14, R138. doi: 10.1186/ar3871
Pubmed Abstract | Pubmed Full Text | CrossRef Full Text | Google Scholar
Tieri, P., and Nardini, C. (2013). Signalling pathway database usability: lessons learned. Mol. Biosyst. 9, 2401–2407. doi: 10.1039/c3mb70242a
Pubmed Abstract | Pubmed Full Text | CrossRef Full Text | Google Scholar
Tieri, P., Termanini, A., Bellavista, E., Salvioli, S., Capri, M., and Franceschi, C. (2012). Charting the NF-κB pathway interactome map. PLoS ONE 7:e32678. doi: 10.1371/journal.pone.0032678
Pubmed Abstract | Pubmed Full Text | CrossRef Full Text | Google Scholar
Tremaroli, V., and Backhed, F. (2012). Functional interactions between the gut microbiota and host metabolism. Nature 489, 242–249. doi: 10.1038/nature11552
Pubmed Abstract | Pubmed Full Text | CrossRef Full Text | Google Scholar
Trenkmann, M., Brock, M., Ospelt, C., and Gay, S. (2010). Epigenetics in rheumatoid arthritis. Clin. Rev Allergy Immunol. 39, 10–19. doi: 10.1007/s12016-009-8166-6
Pubmed Abstract | Pubmed Full Text | CrossRef Full Text | Google Scholar
Van Loo, G., and Beyaert, R. (2011). Negative regulation of NF-kappaB and its involvement in rheumatoid arthritis. Arthritis Res. Ther. 13, 221. doi: 10.1186/ar3324
Pubmed Abstract | Pubmed Full Text | CrossRef Full Text | Google Scholar
Varani, K., Padovan, M., Govoni, M., Vincenzi, F., Trotta, F., and Borea, P. A. (2010). The role of adenosine receptors in rheumatoid arthritis. Autoimmun. Rev. 10, 61–64. doi: 10.1016/j.autrev.2010.07.019
Pubmed Abstract | Pubmed Full Text | CrossRef Full Text | Google Scholar
Varani, K., Padovan, M., Vincenzi, F., Targa, M., Trotta, F., Govoni, M., et al. (2011). A2A and A3 adenosine receptor expression in rheumatoid arthritis: upregulation, inverse correlation with disease activity score and suppression of inflammatory cytokine and metalloproteinase release. Arthritis Res. Ther. 13, R197. doi: 10.1186/ar3527
Pubmed Abstract | Pubmed Full Text | CrossRef Full Text | Google Scholar
Vincenzi, F., Padovan, M., Targa, M., Corciulo, C., Giacuzzo, S., Merighi, S., et al. (2013). A(2A) adenosine receptors are differentially modulated by pharmacological treatments in rheumatoid arthritis patients and their stimulation ameliorates adjuvant-induced arthritis in rats. PLoS ONE 8:e54195. doi: 10.1371/journal.pone.0054195
Pubmed Abstract | Pubmed Full Text | CrossRef Full Text | Google Scholar
Winter, S. E., Lopez, C. A., and Baumler, A. J. (2013). The dynamics of gut-associated microbial communities during inflammation. EMBO Rep. 14, 319–327. doi: 10.1038/embor.2013.27
Pubmed Abstract | Pubmed Full Text | CrossRef Full Text | Google Scholar
Wu, C., Orozco, C., Boyer, J., Leglise, M., Goodale, J., Batalov, S., et al. (2009). BioGPS: an extensible and customizable portal for querying and organizing gene annotation resources. Genome Biol. 10, R130. doi: 10.1186/gb-2009-10-11-r130
Pubmed Abstract | Pubmed Full Text | CrossRef Full Text | Google Scholar
Wu, C., Zhu, J., and Zhang, X. (2012). Integrating gene expression and protein-protein interaction network to prioritize cancer-associated genes. BMC Bioinformatics 13:182. doi: 10.1186/1471-2105-13-182
Pubmed Abstract | Pubmed Full Text | CrossRef Full Text | Google Scholar
Wu, G., Zhu, L., Dent, J. E., and Nardini, C. (2010). A comprehensive molecular interaction map for rheumatoid arthritis. PLoS ONE 5:e10137. doi: 10.1371/journal.pone.0010137
Pubmed Abstract | Pubmed Full Text | CrossRef Full Text | Google Scholar
You, S., Yoo, S. A., Choi, S., Kim, J. Y., Park, S. J., Ji, J. D., et al. (2014). Identification of key regulators for the migration and invasion of rheumatoid synoviocytes through a systems approach. Proc. Natl. Acad. Sci. U.S.A. 111, 550–555. doi: 10.1073/pnas.1311239111
Pubmed Abstract | Pubmed Full Text | CrossRef Full Text | Google Scholar
Yu, W., Gwinn, M., Clyne, M., Yesupriya, A., and Khoury, M. J. (2008). A navigator for human genome epidemiology. Nat. Genet. 40, 124–125. doi: 10.1038/ng0208-124
Pubmed Abstract | Pubmed Full Text | CrossRef Full Text | Google Scholar
Zhou, Q., and Amar, S. (2006). Identification of proteins differentially expressed in human monocytes exposed to Porphyromonas gingivalis and its purified components by high-throughput immunoblotting. Infect. Immun 74, 1204–1214. doi: 10.1128/IAI.74.2.1204-1214.2006
Pubmed Abstract | Pubmed Full Text | CrossRef Full Text | Google Scholar
Keywords: rheumatoid arthritis, multi-omic data integration, host-microbiome interface, protein-protein interaction, network topology
Citation: Tieri P, Zhou XY, Zhu L and Nardini C (2014) Multi-omic landscape of rheumatoid arthritis: re-evaluation of drug adverse effects. Front. Cell Dev. Biol. 2:59. doi: 10.3389/fcell.2014.00059
Received: 01 July 2014; Accepted: 26 September 2014;
Published online: 04 November 2014.
Edited by:
Radhakrishnan Nagarajan, University of Kentucky, USAReviewed by:
Hong-Wen Deng, Tulane University School of Public Health and Tropical Medicine, New Orleans, USACopyright © 2014 Tieri, Zhou, Zhu and Nardini. This is an open-access article distributed under the terms of the Creative Commons Attribution License (CC BY). The use, distribution or reproduction in other forums is permitted, provided the original author(s) or licensor are credited and that the original publication in this journal is cited, in accordance with accepted academic practice. No use, distribution or reproduction is permitted which does not comply with these terms.
*Correspondence: Paolo Tieri, Istituto per le Applicazioni del Calcolo, Consiglio Nazionale delle Ricerche, Via dei Taurini 19, 00185 Rome, Italy e-mail:cC50aWVyaUBpYWMuY25yLml0;
Christine Nardini, Group of Clinical Genomic Networks, Key Laboratory of Computational Biology, Chinese Academy of Sciences - Max Planck Society Partner Institute for Computational Biology, Shanghai Institutes for Biological Sciences, Shanghai, China e-mail:Y2hyaXN0aW5lLm5hcmRpbmkucnNyY0BnbWFpbC5jb20=
†These authors have contributed equally to this work.
Disclaimer: All claims expressed in this article are solely those of the authors and do not necessarily represent those of their affiliated organizations, or those of the publisher, the editors and the reviewers. Any product that may be evaluated in this article or claim that may be made by its manufacturer is not guaranteed or endorsed by the publisher.
Research integrity at Frontiers
Learn more about the work of our research integrity team to safeguard the quality of each article we publish.