- 1Department of Endocrinology, Pingshan District People’s Hospital of Shenzhen, Shenzhen, China
- 2Department of Endocrinology, Pingshan General Hospital of Southern Medical University, Shenzhen, China
- 3Department of Endocrinology, Heilongjiang Provincial Hospital, Harbin, China
Background: The Metabolic score of insulin resistance (METS-IR) has recently been accepted as a reliable alternative to insulin resistance (IR), which was demonstrated to be consistent with the hyperinsulinemic-euglycemic clamp. Few pieces of research have focused on the relationship between METS-IR and diabetes in Chinese. The purpose of this research was to explore the effect of METS-IR on new-onset diabetes in a large multicenter Chinese study.
Methods: At the baseline of this retrospective longitudinal research, 116855 participators were included in the Chinese cohort study administered from 2010 to 2016. The subjects were stratified by quartiles of METS-IR. To assess the effect of METS-IR on incident diabetes, the Cox regression model was constructed in this study. Stratification analysis and interaction tests were applied to detect the potential effect of METS-IR and incident diabetes among multiple subgroups. To verify whether there was a dose-response relationship between METS-IR and diabetes, a smooth curve fitting was performed. In addition, to further determine the performance of METS -IR in predicting incident diabetes, the receiver operating characteristic curve (ROC) was conducted.
Results: The average age of the research participators was 44.08 ± 12.93 years, and 62868 (53.8%) were men. METS-IR were significant relationship with new-onset diabetes after adjusting for possible variables (Hazard ratio [HR]: 1.077; 95% confidence interval [CI]: 1.073-1.082, P < 0.0001), the onset risk for diabetes in Quartile 4 group was 6.261-fold higher than those in Quartile 1 group. Moreover, stratified analyses and interaction tests showed that interaction was detected in the subgroup of age, body mass index, systolic blood pressure, diastolic blood pressure, and fasting plasma glucose, there was no significant interaction between males and females. Furthermore, a dose-response correlation was detected between METS-IR and incident diabetes, the nonlinear relationship was revealed and the inflection point of METS-IR was calculated to be 44.43. When METS-IR≥44.43, compared with METS-IR < 44.43, the trend was gradually saturated, with log-likelihood ratio test P < 0.001. Additionally, the area under receiver operating characteristic of the METS-IR in predicting incident diabetes was 0.729, 0.718, and 0.720 at 3, 4, and 5 years, respectively.
Conclusions: METS-IR was correlated with incident diabetes significantly, and showed a nonlinear relationship. This study also found that METS-IR had good discrimination of diabetes.
Background
Diabetes mellitus (DM) is a chronic epidemic on an unprecedented scale, which is spiraling out of control (1). The International Diabetes Federation (IDF) reported that more than one in 10 adults now have diabetes all over the world. It is forecasted that 537 million people were suffered from DM in 2021. The Western Pacific region accounts for more than a third (38%) of the total number of diabetes, with China accounting for a quarter of the total (2, 3). In recent years, the Chinese Diabetes Society (CDS) has been paying attention to the progression of diabetes in China. The prevalence of diabetes in China is still on the rise, reaching 11.2% in 2017 (4, 5). It is well known that if diabetes is not well managed and treated, this disease will cause damage to multiple organs of the body and leads to complications, such as cardiovascular disease, kidney damage, eye disease, and so on. The direct and indirect costs of diabetes to health services increase continuedly (3, 6). It is a huge challenge to predict future diabetes incidence.
Insulin resistance (IR) is connected with the development and progression of diabetes significantly (7–9). It reduces insulin efficiency in insulin-responsive tissues (muscle, fat, and liver), which conversely causes a decompensated increase in insulin and hyperinsulinemia, and then leads to chronic metabolic disorders (hyperglycemia, hypertension, hyperlipidemia, etc.) and inflammation (10–14). Therefore, it is requisite to detect IR in the early stages of diabetes, and early prevention in non-diabetes patients with metabolic risk is beneficial to reduce the socioeconomic burden of diabetes and other metabolic diseases (15–18).
Accurate assessment of insulin resistance is usually performed with the Euglycaemic-hyperinsulinaemic clamp (EHC) and Homeostatic model assessment for insulin resistance (HOMA-IR). EHC is still the gold standard method of assessing IR. However, it is mostly utilized in research due to the characteristics of being time-consuming, invasive, and high cost, which inhibit its promotion (19–21). Meanwhile, the practical applicability of HOMA-IR is also limited by invasiveness, complexity, and impracticality, especially in resource-poor areas (21, 22). To evaluate IR in large epidemiological studies, several simple formulas have been developed based on readily available and inexpensive biochemical indicators. For example, triglyceride to high-density lipoprotein cholesterol ratio (TG/HDL-C), triglyceride glucose index (TγG index), and triglyceride glucose-body mass index(TγG-BMI) were widely employed as a reliable surrogate marker to estimate IR in clinical practice (23–28). These non-insulin-based indexes offer a simpler and cost-effective option for the identification of IR.
Recently, the Metabolic score for insulin resistance (METS-IR), a newly-developed non-insulin-based metabolic score, consists of fasting plasma glucose (FPG), triglycerides (TG), high-density lipoprotein cholesterol (HDL-C), and body mass index (BMI). It has been demonstrated to be highly consistent with EHC in assessing IR (29).
The relationship between METS-IR and the prevalence of diabetes, however, has received very little investigation, which needs to be further investigated, especially in large epidemiological studies. This research was designed to explore the effect of METS-IR on the incidence of diabetes among a large-scale of Chinese adults.
Methods
Cohort population and study design
Data for this research were collected from a multicenter health check-up program, the Rich Healthcare Group, which was published by Chen et al. at www.Datadryad.org (30). Data on this website is free and public, the providers give all copyright and ownership rights, and there is no interest involved. The detailed research design has been described in previous studies (30). Participants in this cohort underwent two or more follow-up visits between 2010 and 2016 in 11 major Chinese cities, and they were at least 20 years old. At each follow-up visit to the health check center, participants completed a detailed questionnaire and had blood samples collected. According to the report of Chen et al., 211,833 subjects (54.8% males and 45.2% females) were involved in this study (30). Data with missing values (such as weight, height, gender, and FPG) and with an extreme value of BMI (<15 kg/m2 or >55 kg/m2) were excluded at baseline. In addition, participants with a follow-up interval of fewer than 2 years, those who had been diagnosed with diabetes at baseline, and those who could not determine their diabetes status during follow-up were excluded. There was no need to apply for ethical approval due to the secondary data analysis nature of this study. Previous research received approval from the Rich Healthcare Group review board, and this retrospective study conformed with the Helsinki Declaration.
The dataset contains participants’ medical records, which includes demographic and blood biochemical variables: age, gender, height, weight, systolic and diastolic blood pressure (SBP and DBP), history of smoking, history of drinking, family history of the disease, FPG, TG, total cholesterol (TC), low-density lipoprotein cholesterol (LDL-C), HDL-C. BMI (kg/m2) is expressed as a weight (kg) divided by height squared (m2). Fasting venous blood samples were measured on an autoanalyzer, and participants need to be fasting for at least 10 hours. The dependent variable of the research was new-onset diabetes, defined as FPG≥7.00 mmol/L and/or self-reported diabetes during follow-up.
To further research, missing values and outliers of TG and HDL-C were excluded(n=94,978), and the total number of participants was 116,855 participants (62,868 males and 53,987 females) in the end. The arithmetic Formula of METS-IR was Ln [(2*FPG) + TG]*BMI)/(Ln[HDL-C])(1mmol/L=18mg/dl) (29).
Statistical analysis
Statistical analyses were completed by Empower (R) (www.empowerstats.com) and R-project (version 3.4.3). A two-tailed P value<0.05 indicates statistical significance.
First, all participators were assigned the quartiles of METS-IR. The continuous data under normal distribution were presented in the form of average ± standard deviation, while the skewed distribution was represented by the median (quartile range). One-way analysis and Kruskal-Wallis were utilized for comparing the distribution of normal distribution and skewed distribution between groups. The classified variables were described as numbers (proportions), and comparisons between groups were assessed using the chi-square test.
Subsequently, linear regression analysis was conducted to verify and check the collinearity of variables and report the variance inflation factor (VIF) (31). Variables with VIF larger than 5 were multicollinearity and could not be included in the Cox regression model. Cox proportional hazards model was carried out to analyze the hazard ratio (HR) and 95% confidence interval (CI) for evaluating METS-IR and the risk of new-onset diabetes. The results of three covariate models were demonstrated on the grounds of the recommendations of the STROBE statement to manipulate for possible confounding bias. Covariates with matched hazard ratio change>10% can be added as confounders into the model (32). Crude model: unadjusted, Model I: adjusted for age, gender, smoking status, drinking status, and family history of diabetes, Model II: based on Model I, SBP, DBP, TC, LDL-C, and serum creatinine (SCR) were further adjusted. Further, METS -IR was transformed into a classified variable and trend analysis was calculated for quantifying the stability of the results of regression analysis and observing the nonlinear probability. Moreover, the covariables were converted into the ‘GAM Model’ by the weighted generalized additive model (GAM) to cover the shortage of general linear analysis in the analysis of nonlinearity (33).
Moreover, stratified analysis and interaction testing were applied to analyze the potential effects of METS-IR on incident diabetes in subgroups using multivariable logistic regression models.
The dose-response relationship between METS-IR and incident diabetes was conducted by using a smooth curve fitting and GAM. In the presence of nonlinear correlation, the threshold effect was carried out using a two-piecewise linear regression mode. When the rate between incident diabetes and METS-IR appeared apparent, the inflection point will be calculated automatically by the recursive method.
Furthermore, survival estimates and cumulative diabetes incidence were constructed by the Kaplan-Meier survival analyses, and the log-rank test was used to compute the survival curve functions between METS-IR quartiles.
Additionally, a receiver operating characteristic (ROC) curve was performed to evaluate the performance of METS-IR to predict incident diabetes. Further, ROC was plotted to compare the predictive ability of TG/HDL-C (triglycerides/HDL-c), TγG (Ln[(Glucose*Triglycerides)/2]), TγG-BMI (TyG*BMI) and METS-IR for incident diabetes.
Result
Baseline characteristics and univariate analysis of study participants
As shown in Table 1, the characteristics of the 116,855 research participators at baseline were described. The total study populations were 62,868 men (53.8%) and 53,987 women (46.2%), and the average age of the research participators was 44.08 ± 12.93 years. At a median follow-up of 3.1± 0.94 years, 2,685 participators (2.3%) had new-onset diabetes. Participants in the higher METS-IR quartile group were older than those in the lower quartile groups (Q1-3). BMI, SBP, DBP, FPG, TC, TG, LDL-C, and SCR increased with the increase of the METS-IR quartile, while HDL-C level decreased (all P values < 0.001). By contrast with participators in quartile 1, subjects in the higher quartiles were more current drinkers and fewer current smokers. No significant differences were found in the Family history of diabetes between the METS-IR quartile group. Univariate linear regression analyses were constructed to examine all significant variables in Table 2. And it showed that age, BMI, SBP, DBP, FPG, TC, TG, LDL-C, METS-IR, and family history of diabetes were positively correlated with new-onset diabetes.
The multivariate analysis between METS-IR and the risk of new-onset diabetes
Firstly, variable collinearity diagnostics were conducted to calculate the VIF for each covariate. Covariates were deemed to exhibit substantial multicollinearity and were ineligible for inclusion in the multivariate Cox regression model if their VIF was more than 5. The results and details were listed in Table S1. After that, the outcome of the Cox proportional hazards regression analysis was summarized in Table 3. In the Crude model, METS-IR was a positive correlation with incident diabetes (HR= 1.093, 95%CI:1.089 to 1.097, P < 0.0001). Compared with the crude mode, HR(95% CIs) for diabetes incidence was 1.083 (1.078 to 1.087) in Model I. Furthermore, the HR for the incident diabetes was 1.077 (95% CI: 1.073, 1.082, P<0.0001) in Model II. Remained significant even after the continuous covariates were adopted into the GAM model as curves, and the hazard ratio was 1.085 (95% CI: 1.075 to 1.096, <0.0001), indicating the robustness of the main results. Moreover, when METS-IR was handled as a classified variable into quartiles and using the first quartile as a reference, the trend of incident diabetes increased with METS-IR quartile (P for trend<0.001) in the Crude model. In addition, the risk of incident diabetes was increased with Q4 versus Q1 of METS -IR in Model II (HR 6.261[5.189,7.554], P for trend<0.001). Consistently, there were significantly stronger associations of the METS-IR with incident diabetes.
Kaplan-Meier analysis of diabetes
The comparison of cumulative diabetes incidence between the quartiles of baseline METS-IR in the Kaplan-Meier curve was shown in Figure 1. Cumulative incidence was the highest in quartile 4 and lowest in quartile1 (log-rank test P values < 0.001). It showed that METS-IR Q4 participants had a greater chance of developing incident diabetes than other groups during the follow-up.
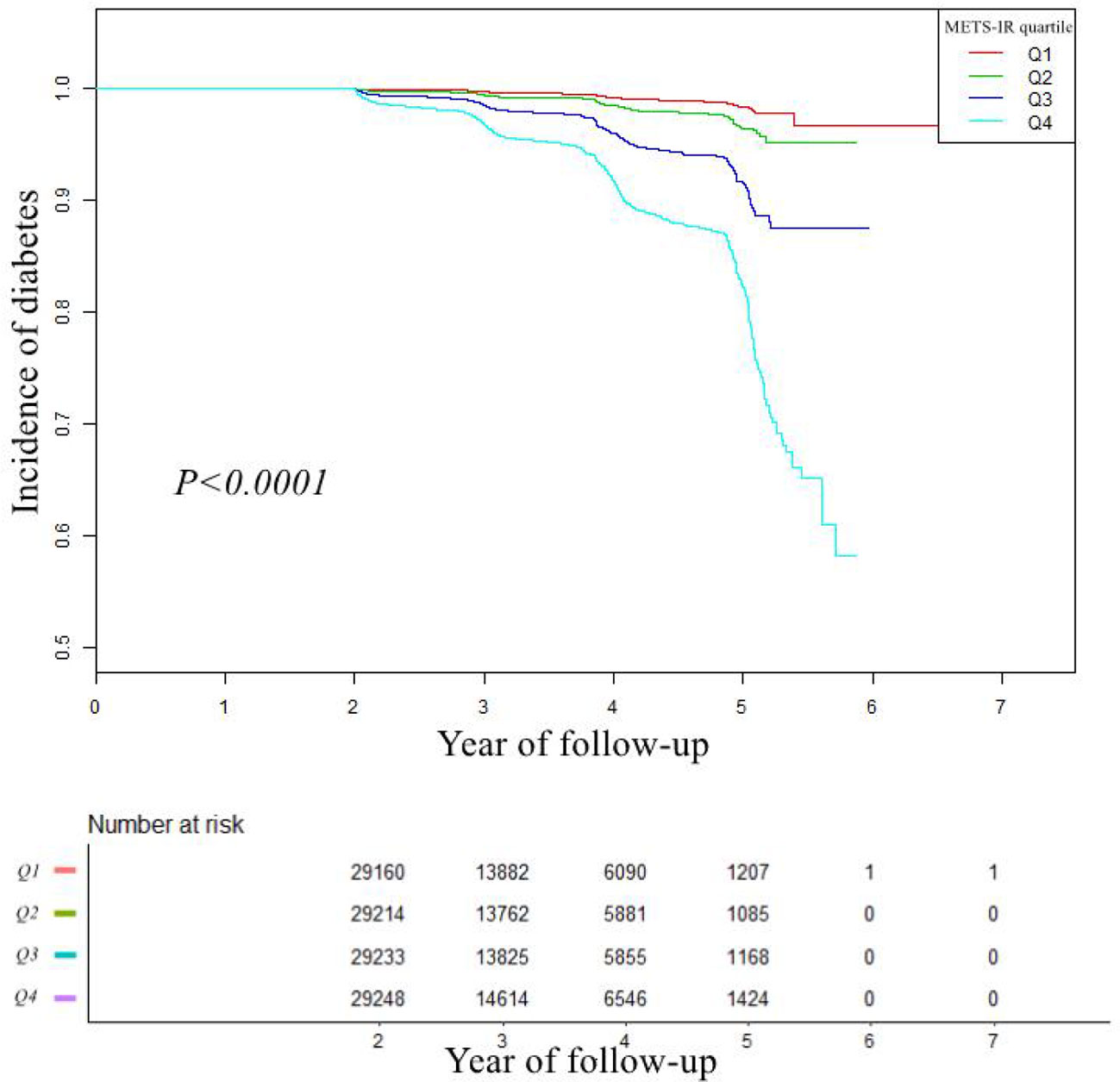
Figure 1 Kaplan–Meier event-free survival curve. Kaplan–Meier analysis of incident of diabetes based on METS-IR quartiles (Log-rank, P < 0.0001). Abbreviation: METS-IR: the metabolic score for insulin resistance.
The analyses of the dose-response and threshold effect
A dose-response study using GAM indicated a non-linear connection between METS-IR and incident diabetes (adjusting age, sex, SBP, DBP, TC, LDL-C, SCR, smoking status, drinking status, and family history of diabetes) in Figure 2. We further explored the inflection point of METS-IR was 44.43 (log-likelihood ratio test P < 0.001, Table 4). When METS-IR < 44.43, the HR was 1.140 (95% CI: 1.126 to 1.154), however, cubic spline smoothing gradually became saturated (HR: 1.051; 95% CI: 1.043 to 1.058) on the right side.
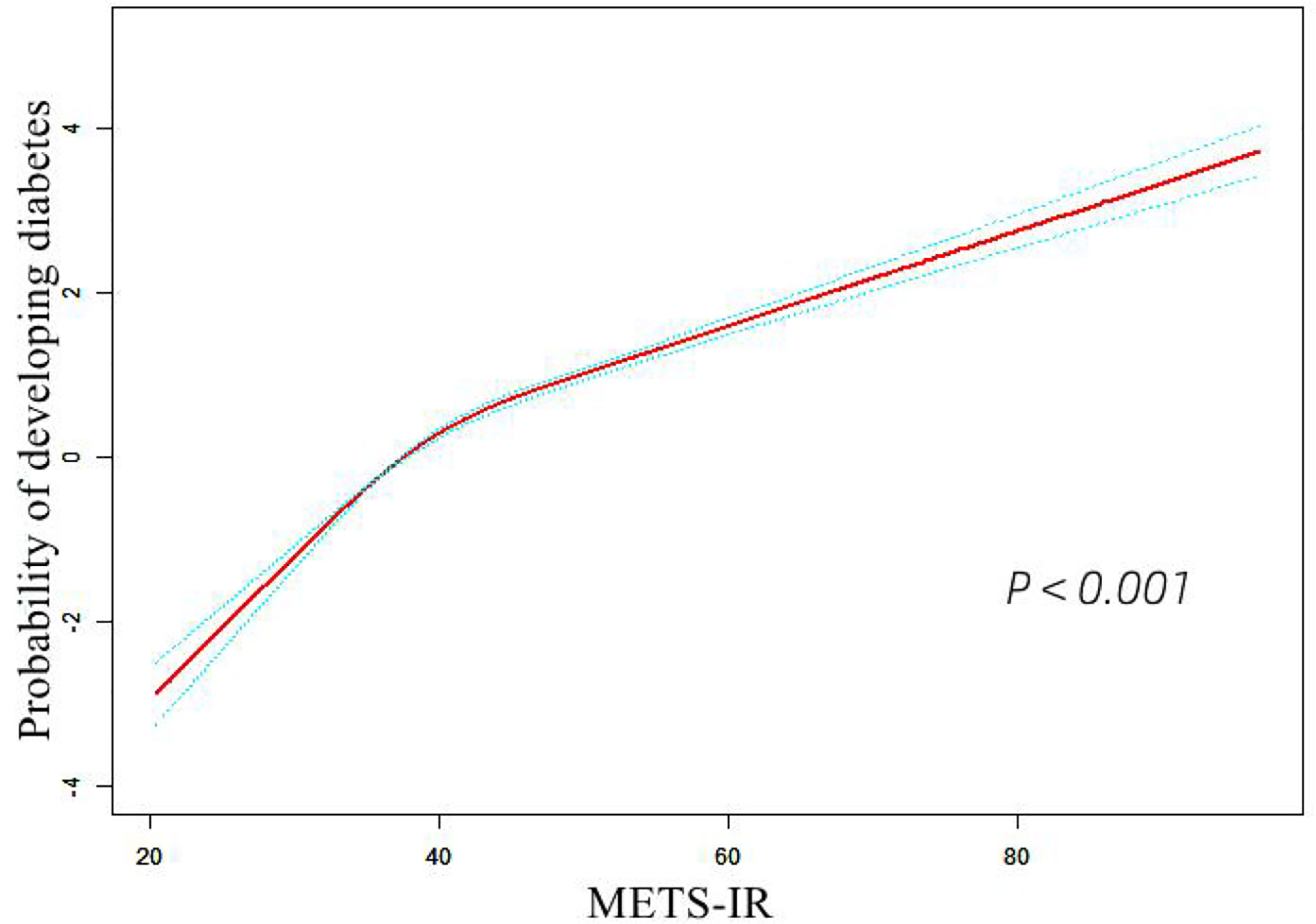
Figure 2 The non-linear relationship between METS-IR and incident of diabetes. Abbreviation: METS-IR: the metabolic score for insulin resistance.
The results of stratified analyses
In addition, this study conducted a stratified analysis to investigate the effects of potential modifications between METS-IR and incident diabetes, including age, gender, sex, age, BMI, SBP, DBP, FPG, and family history of diabetes. (Table 5). Age, BMI, SBP, DBP, and FPG interacted with METS-IR and incident diabetes by interaction tests (all P interaction < 0.05). Nevertheless, there was no significant interaction among different stratifications in gender and family history of diabetes. This suggested that the relationship between METS-IR and diabetes mellitus was not affected by gender and family history, and the combination of certain risk factors with METS-IR may enhance its sensitivity.
The discernibility of METS-IR for diabetes
A time-dependent ROC analysis was performed to assess the predictive efficacy of METS-IR for incident diabetes at various time nodes (Figures 3A–C). The area under the curve (AUC) was 0.729, 0.718, 0.720 at 3, 4, and 5 years, respectively, which revealed a good discriminatory capacity for incident diabetes. In addition, ROC revealed that TG/HDL-C, TγG, TγG-BMI, and METS-IR had AUCs of 0.699, 0.765,0.778, and 0.759, respectively. It seemed that the predictive ability of METS -IR followed by TγG-BMI and was not inferior to TG/HDL-C and TγG (Figure 4). And the cut-off points for the prediction of diabetes with them were shown in Table S2. Therefore, METS-IR can be used to predict incident diabetes during follow-up in Chinese.
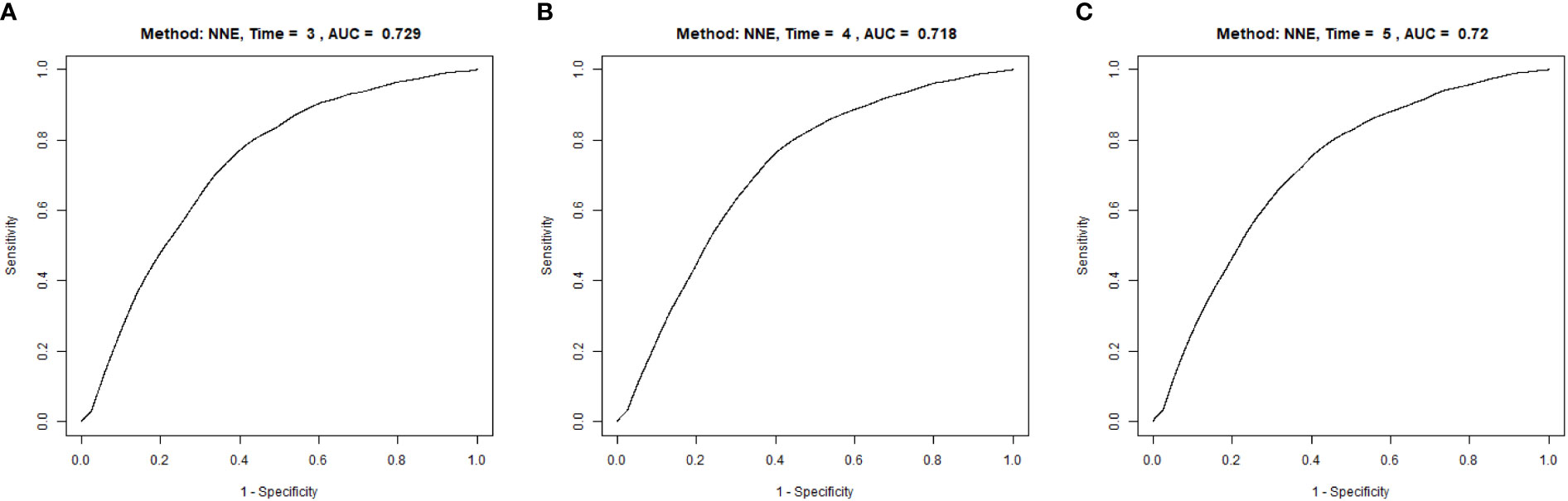
Figure 3 Time-dependent receiver operating characteristic (ROC) curves of METE-IR for diabetes at 3 (A), 4 (B) and 5 years (C). METS-IR, the metabolic score for insulin resistance.
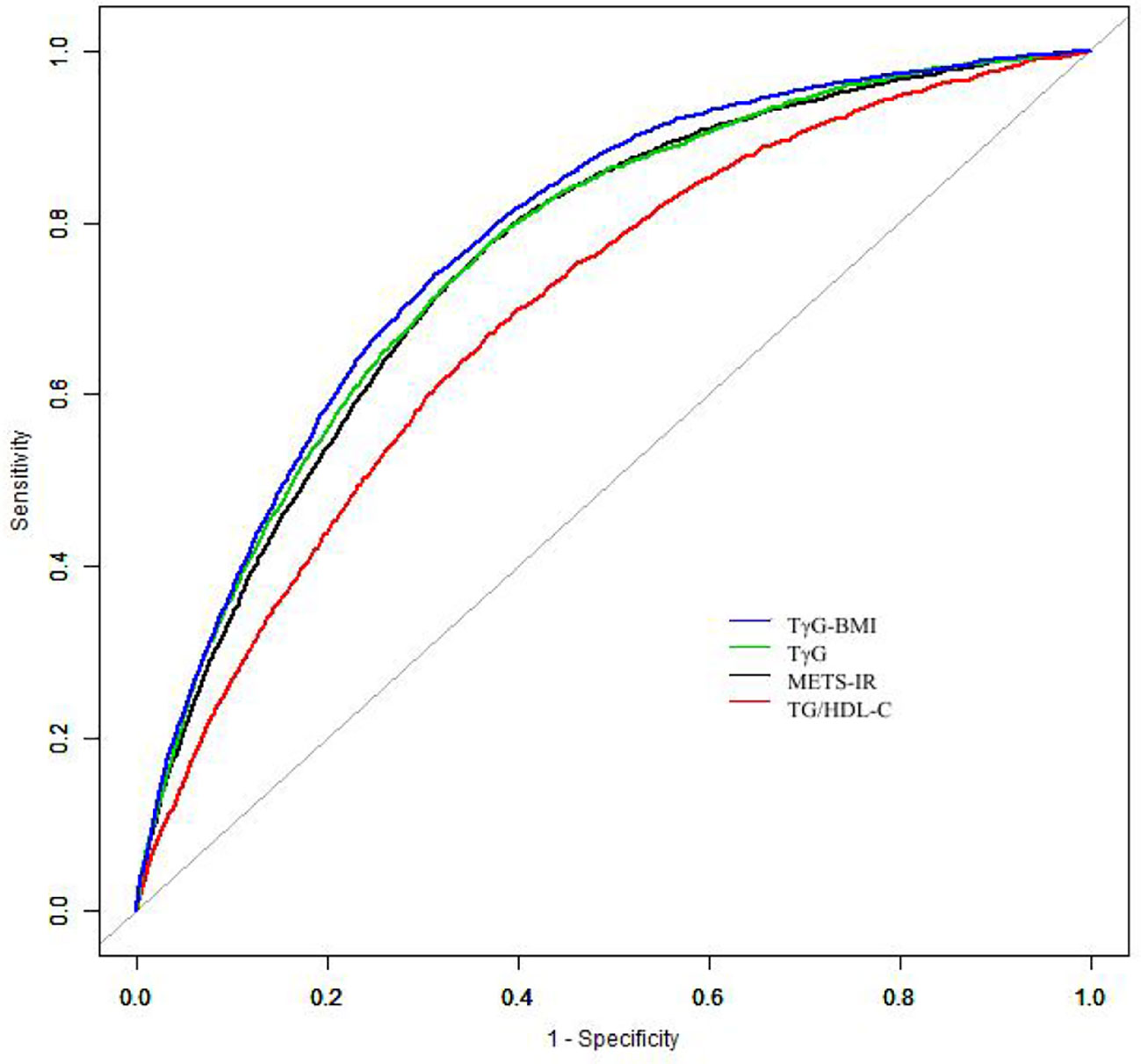
Figure 4 The results of receiver operating characteristics curves. Abbreviations: TG/HDL-C, Triglyceride to high-density lipoprotein cholesterol ratio; TγG index, Triglyceride glucose index; TγG-BMI, TyG*BMI; METS-IR, The Metabolic score of insulin resistance.
Discussion
The present large cohort Chinese study demonstrated that there was a positive correlation between METS-IR and the onset of diabetes in Chinese. When adjustments were made for covariates, individuals in the top quartile of METS-IR had a 6.261-fold higher risk of developing diabetes than those in the bottom METS-IR quartile. Meanwhile, the association between METS-IR and incidence diabetes was demonstrated to be nonlinear. Furthermore, the result revealed that the cumulative risk of incident diabetes in Chinese adults increased gradually with the increase of METS-IR. Moreover, ROC analyses suggested the METS-IR had significant discriminatory power for new-onset diabetes at 3 years,4 years, and 5 years. These results indicated that METS-IR can be used to predict the onset of diabetes in healthy individuals during follow-up.
Non-insulin-based metabolic indicators used to evaluate IR have developed into an easier and cost-effective alternative method, which is more suitable for epidemiological studies. The previously widely accepted non-insulin-based IR indicators commonly included FPG, parameters of lipids, and indices of obesity, such as TyG, TyG-BMI, and TG/HDL-C (34–36). Likewise, the METS-IR is a simple and economical indicator that combines FPG, lipid profile, and obesity index. It is reported that TyG (23, 24, 37, 38), TyG-BMI (27, 28), and TG/HDL-C (25) were correlated with the risk of diabetes positively. Consistent with present study, previous studies (29, 39, 40) also identified that there was a positive correlation between METS-IR and incident diabetes. Additionally, a prospective cohort study by Chavolla et al. also proved that METS-IR was superior to the TγG index and TG/HDL ratio in diagnostic performance, but there was no significant difference between METS-IR and TγG-BMI index (29). However, ROC analysis in this research was observed that TG/HDL-C, TγG, TγG-BMI and METS-IR had AUCs of 0.699, 0.765,0.778, and 0.759, respectively, it revealed that the discriminatory power of METS-IR was still superior to TG/HDL ratio, but not TγG. Moreover, Chavolla et al. proved that the effect between METS-IR and diabetes was modulated by age (29). An epidemiological study conducted by 12,107 Chinese participants and subgroup analyses also consistently confirmed that significant associations remained between gender, age, and FPG level in subgroup analyses (39). Interestingly, stratified interaction analysis in this study also found differences in the influence of METS-IR and diabetes among age and baseline FPG subgroups, but there was no interaction observed in the subgroup of gender, suggesting that the correlation between METS-IR and diabetes was robust among men and women. Notably, this study also conducted the dose-response analysis between METS-IR and diabetes and showed that the probability of diabetes gradually increased with the increase of METS-IR, which is consistent with results from a cohort of 12,107 rural Chinese participants (39) and a cohort study of 12,290 non-obese Japanese adults (40). Nonetheless, this study provided additional information that there was a positive correlation between METS-IR and the incidence of DM with a saturation effect, and the inflection point of METS-IR was calculated to be 44.43. When METS-IR ≤44.43, the risk of diabetes increased rapidly with METS-IR, the effect size was 1.140 (95% CI: 1.126-1.154, P < 0.0001); while METS-IR>44.43, the tendency gradually saturated compared with the left side of the inflection point (HR=1.051,95%CI: 1.043,1.058 P<0.0001). A possible explanation for the conflicting results may be the differences in participant selection and covariables, and further studies are needed to check on the result.
The underlying mechanism of METS-IR associated with diabetes has yet to be elucidated. IR has played its pathological mechanism before the onset of diabetes (41). The content of reactive oxygen species (ROS) increases with the increase of blood glucose, which may have a toxic reaction on β-cells, leading to pancreatic β-cell dysfunction, and then causing diabetes (42, 43). During hyperinsulinemia, the efficiency of insulin signaling is inhibited, resulting in reduced glucose uptake from the blood, which increases the risk of diabetes (44). Consecutively, hyperglycemia can induce excessive peroxide production, which eventually leads to impaired insulin secretion and insulin resistance by promoting multiple oxidative stress pathways (45).There is growing evidence about dyslipidemia is a cause of IR (46, 47). Diabetes is a progressive disease, and a study on animal models of diabetes showed β-cell apoptosis accompanied by long-term hyperglycemia/hyperlipemia (glucolipotoxicity) (48). Previous literature indicated that fat accumulation is related to IR, which may provoke metabolic disturbances in the liver, and further affect the homeostasis of blood glucose and lipid (49–51). Adipose tissue contributes to metabolic risk and, in addition to the effects of body mass index, is associated with elevated blood glucose and lower HDL-C (52, 53). Hypertriglyceridemia contributes to a corresponding increase in free fatty acid (FFA) levels, which may impair insulin signaling and induce tissue oxidative stress, giving rise to IR in bone and liver (53, 54). Simultaneously, lower HDL-C reduces its anti-inflammatory effects and its inhibitory effect on LDL-C oxidation (55). Besides, IR is affected by many factors, obesity index is also an important factor in IR (56), which can occur even in people with a normal BMI (57). And BMI has been shown to have a strong association with prediabetes and diabetes in many studies (58–61). Therefore, as one of the important components of the METS-IR model, the obesity index may have a significant impact on the prediction of diabetes by METS-IR. Chavolla and his colleagues found that subjects with high METS-IR index showed increased visceral fat and fasting insulin levels (29). At the same time, in our prospective study, higher levels of BMI, FPG, TC, TG, and LDL-C were observed at higher baseline METS-IR levels, and persons in higher baseline METS-IR levels had higher cumulative incidence of diabetes, which supported METS-IR can be applied to forecast the prevalence of diabetes.
There were still potential limitations that should be considered in this study. First, the occurrence of diabetes was diagnosed only by FPG and self-report in this study, without the use of the 2-hour oral glucose tolerance test (OGTT) and glycated hemoglobin (HbA1c), which may underestimate the incidence of diabetes in the study. However, due to the operation complexity of OGTT, it is not feasible for large cohorts and similar limitations have been observed in previous large population studies (40). Second, due to the limitations of the original cohort data, we could not include several potential confounders and biochemical indicators such as diet, exercise, and plasma insulin. In particular, the lack of plasma insulin limited the ability to explore the concordance between METS-IR and HOMA-IR, and patients with diabetes could not be classified in the study because insulin testing is not a routine physical examination, especially in large epidemiological studies. Additionally, there was a lack of data on the dynamic changes of METS-IR during the follow-up survey, so its correlation with diabetes could not be evaluated in this study. Third, the study population was all Chinese, which may limit the extrapolation of such results to other ethnic groups. Despite these limitations, China has a large population of diabetics (3, 4), and the results of this study are still representative.
Although our study had limitations, there were still several advantages. Compared with previous similar studies (29, 39, 40), this study was a large sample size and a multicenter study. Furthermore, the study also conducted a sensitivity analysis by handling METS -IR as a categorical variable and continuous variable to assess the stability of the result.
Conclusion
This study provides additional evidence supporting that METS-IR was connected with incident diabetes in the cohort of Chinese, and there is a non-linear relationship between METS-IR and diabetes. Meanwhile, METS-IR had a good discriminative ability for incident diabetes. METS-IR may be a reliable alternative method for predicting the risk of diabetes in epidemiological investigations.
Data availability statement
The original contributions presented in the study are included in the article/Supplementary Materials. Further inquiries can be directed to the corresponding author.
Ethics statement
The studies involving human participants were reviewed and approved by Rich Healthcare Group. The patients/participants provided their written informed consent to participate in this study.
Author contributions
KF and ZC contributed to the study concept and design, researched and interpreted the data and drafted the manuscript. CH, ZZ and YZ performed the statistical analysis. MX, YT and LF contributed to the discussion. ZC drafted the Manuscript and KF edited the manuscript. All authors read and approved the final the manuscript. All authors contributed to the article and approved the submitted version.
Funding
This study was funded by Heilongjiang Natural Science Foundation Project (LH2019H084), Shenzhen Pingshan District Health System research project (202135), and President’s Fund Project of Shenzhen Pingshan Medical Health Group (Pingshan General Hospital of Southern Medical University).
Acknowledgments
The authors are grateful to all of the doctors and participants who were involved in the Rich Healthcare Group study in China.
Conflict of interest
The authors declare that the research was conducted in the absence of any commercial or financial relationships that could be construed as a potential conflict of interest.
Publisher’s note
All claims expressed in this article are solely those of the authors and do not necessarily represent those of their affiliated organizations, or those of the publisher, the editors and the reviewers. Any product that may be evaluated in this article, or claim that may be made by its manufacturer, is not guaranteed or endorsed by the publisher.
Supplementary material
The Supplementary Material for this article can be found online at: https://www.frontiersin.org/articles/10.3389/fcdhc.2022.1101276/full#supplementary-material
Abbreviations
METS-IR, The Metabolic score of insulin resistance; DM, Diabetes mellitus; IDF, The International Diabetes Federation; CDS, The Chinese Diabetes Society; IR, Insulin resistance; EHC, Euglycaemic-hyperinsulinaemic clamp; HOMA-IR, The homeostatic model assessment for insulin resistance; FPG, Fasting plasma glucose; TG/HDL-C, Triglyceride to high-density lipoprotein cholesterol ratio; TγG index, Triglyceride glucose index; TG, Triglycerides; HDL-C, High-density lipoprotein cholesterol; BMI, Body mass index; SBP, Systolic blood pressure; DBP, Diastolic blood pressure; TC, Total cholesterol; LDL-C, Low-density lipoprotein cholesterol; VIF, Variance inflation factor; HR, Hazard ratio; CI, Confidence interval; SCR, Serum creatinine; GAM, Generalized additive model; ROC, Receiver operating characteristic; AUC, The area under the curve; ROS, Reactive oxygen species; FFA, Free fatty acid; OGTT, Oral glucose tolerance test; HbA1c, Glycated hemoglobin.
References
1. GBD 2017 Risk Factor Collaborators. Global, regional, and national comparative risk assessment of 84 behavioural, environmental and occupational, and metabolic risks or clusters of risks for 195 countries and territories, 1990–2017: a systematic analysis for the global burden of disease study 2017. Lancet (London England). (2018) 392:1923–94. doi: 10.1016/S0140-6736(18)32225-6
2. Sun H, Saeedi P, Karuranga S, Pinkepank M, Ogurtsova K, Duncan BB, et al. IDF diabetes atlas: Global, regional and country-level diabetes prevalence estimates for 2021 and projections for 2045. Diabetes Res. Clin. practice. (2022) 183:109119. doi: 10.1016/j.diabres.2021.109119
3. Magliano DJ, Boyko EJ. IDF diabetes atlas 10th edition scientific committee . IDF DIABETES ATLAS. 10th ed. Brussels: International Diabetes Federation (2021).
4. Jia W, Weng J, Zhu D, Ji L, Lu J, Zhou Z, et al. Standards of medical care for type 2 diabetes in China 2019. Diabetes/metabolism Res. Rev. (2019) 35:e3158. doi: 10.1002/dmrr.3158
5. Prevention D. Clinical guidelines for prevention and treatment of type 2 diabetes mellitus in the elderly in China (2022 edition). Zhonghua nei ke za zhi. (2022) 61:12–50. doi: 10.3760/cma.j.cn112138-20211027-00751
6. Lin X, Xu Y, Pan X, Xu J, Ding Y, Sun X, et al. Global, regional, and national burden and trend of diabetes in 195 countries and territories: an analysis from 1990 to 2025. Sci. Rep. (2020) 10:1–1. an analysis from 1990 to 2025. doi: 10.1038/s41598-020-71908-9
7. James DE, Stöckli J, Birnbaum MJ. The aetiology and molecular landscape of insulin resistance. Nat. Rev. Mol. Cell Biol. (2021) 22:751–71. doi: 10.1038/s41580-021-00390-6
8. Gastaldelli A, Gaggini M, DeFronzo RA. Role of adipose tissue insulin resistance in the natural history of type 2 diabetes: results from the San Antonio metabolism study. Diabetes (2017) 66:815–22. doi: 10.2337/db16-1167
9. Alejandro EU, Gregg B, Blandino-Rosano M, Cras-Méneur C, Bernal-Mizrachi E. Natural history of β-cell adaptation and failure in type 2 diabetes. Mol. aspects Med. (2015) 42:19–41. doi: 10.1016/j.mam.2014.12.002
10. Helmink MA, de Vries M, Visseren FL, de Ranitz WL, de Valk HW, Westerink J. Insulin resistance and risk of vascular events, interventions and mortality in type 1 diabetes. Eur. J. Endocrinology. (2021) 185:831–40. doi: 10.1530/EJE-21-0636
11. Zhao Q, Zhang TY, Cheng YJ, Ma Y, Xu YK, Yang JQ, et al. Impacts of triglyceride-glucose index on prognosis of patients with type 2 diabetes mellitus and non-ST-segment elevation acute coronary syndrome: results from an observational cohort study in China. Cardiovasc. Diabetol. (2020) 19:1–9. doi: 10.1186/s12933-020-01086-5
12. Yaribeygi H, Farrokhi FR, Butler AE, Sahebkar A. Insulin resistance: Review of the underlying molecular mechanisms. J. Cell. Physiol. (2019) 234:8152–61. doi: 10.1002/jcp.27603
13. Krentz AJ. Fortnightly review: insulin resistance. Bmj. (1996) 313:1385–9. doi: 10.1136/bmj.313.7069.1385
14. Ormazabal V, Nair S, Elfeky O, Aguayo C, Salomon C, Zuñiga FA. Association between insulin resistance and the development of cardiovascular disease. Cardiovasc. diabetology. (2018) 17:1–4. doi: 10.1186/s12933-018-0762-4
15. Yoon J, Jung D, Lee Y, Park B. The metabolic score for insulin resistance (METS-IR) as a predictor of incident ischemic heart disease: a longitudinal study among Korean without diabetes. J. personalized Med. (2021) 11:742. doi: 10.3390/jpm11080742
16. Sasaki N, Ozono R, Higashi Y, Maeda R, Kihara Y. Association of insulin resistance, plasma glucose level, and serum insulin level with hypertension in a population with different stages of impaired glucose metabolism. J. Am. Heart Assoc. (2020) 9:e015546. doi: 10.1161/JAHA.119.015546
17. Han T, Lan L, Qu R, Xu Q, Jiang R, Na L, et al. Temporal relationship between hyperuricemia and insulin resistance and its impact on future risk of hypertension. Hypertension. (2017) 70:703–11. doi: 10.1161/HYPERTENSIONAHA.117.09508
18. Baghbani-Oskouei A, Tohidi M, Hasheminia M, Azizi F, Hadaegh F. Impact of 3-year changes in fasting insulin and insulin resistance indices on incident hypertension: Tehran lipid and glucose study. Nutr. Metab. (2019) 16:1–9. doi: 10.1186/s12986-019-0402-3
19. DeFronzo RA, Tobin JD, Andres R. Glucose clamp technique: a method for quantifying insulin secretion and resistance. Am. J. Physiology-Endocrinology And Metab. (1979) 237:E214–23. doi: 10.1152/ajpendo.1979.237.3.E214
20. Muniyappa R, Lee S, Chen H, Quon MJ. Current approaches for assessing insulin sensitivity and resistance in vivo: advantages, limitations, and appropriate usage. Am. J. Physiology-Endocrinology Metab. (2018) 294:E15–E 26. doi: 10.1152/ajpendo.00645.2007
21. Rudvik A, Månsson M. Evaluation of surrogate measures of insulin sensitivity-correlation with gold standard is not enough. BMC Med. Res. methodology. (2018) 18:1–8. doi: 10.1186/s12874-018-0521-y
22. Matthews DR, Hosker JP, Rudenski AS, Naylor BA, Treacher DF, Turner RC. Homeostasis model assessment: insulin resistance and β-cell function from fasting plasma glucose and insulin concentrations in man. Diabetologia. (1985) 28:412–9. doi: 10.1007/BF00280883
23. Wang L, Cong HL, Zhang JX, Hu YC, Wei A, Zhang YY, et al. Triglyceride-glucose index predicts adverse cardiovascular events in patients with diabetes and acute coronary syndrome. Cardiovasc. Diabetol. (2020) 19:1–1. doi: 10.1186/s12933-020-01054-z
24. Park HM, Lee HS, Lee YJ, Lee JH. The triglyceride–glucose index is a more powerful surrogate marker for predicting the prevalence and incidence of type 2 diabetes mellitus than the homeostatic model assessment of insulin resistance. Diabetes Res. Clin. Pract. (2021) 180:109042. doi: 10.1016/j.diabres.2021.109042
25. Lim TK, Lee HS, Lee YJ. Triglyceride to HDL-cholesterol ratio and the incidence risk of type 2 diabetes in community dwelling adults: A longitudinal 12-year analysis of the Korean genome and epidemiology study. Diabetes Res. Clin. Pract. (2020) 163:108150. doi: 10.1016/j.diabres.2020.108150
26. Kim J, Shin SJ, Kim YS, Kang HT. Positive association between the ratio of triglycerides to high-density lipoprotein cholesterol and diabetes incidence in Korean adults. Cardiovasc. Diabetol. (2021) 20:1–10. doi: 10.1186/s12933-021-01377-5
27. Song B, Zhao X, Yao T, Lu W, Zhang H, Liu T, et al. Triglyceride glucose-body mass index and risk of incident type 2 diabetes mellitus in Japanese people with normal glycemic level: A population-based longitudinal cohort study. Front. endocrinology. (2022) 13:907973. doi: 10.3389/fendo.2022.907973
28. Wang X, Liu J, Cheng Z, Zhong Y, Chen X, Song W. Triglyceride glucose-body mass index and the risk of diabetes: a general population-based cohort study. Lipids Health disease. (2021) 20:1–10. doi: 10.1186/s12944-021-01532-7
29. Bello-Chavolla OY, Almeda-Valdes P, Gomez-Velasco D, Viveros-Ruiz T, Cruz-Bautista I, Romo-Romo A, et al. METS-IR, a novel score to evaluate insulin sensitivity, is predictive of visceral adiposity and incident type 2 diabetes. Eur. J. endocrinology. (2018) 178:533–44. doi: 10.1530/EJE-17-0883
30. Chen Y, Zhang XP, Yuan J, Cai B, Wang XL, Wu XL, et al. Association of body mass index and age with incident diabetes in Chinese adults: a population-based cohort study. BMJ Open (2018) 8:e021768. doi: 10.1136/bmjopen-2018-021768
31. Wax Y. Collinearity diagnosis for a relative risk regression analysis: An application to assessment of diet-cancer relationship in epidemiological studies. Stat Med. (1992) 11:1273–87. doi: 10.1002/sim.4780111003
32. Vandenbroucke JP, von Elm E, Altman DG, Gøtzsche PC, Mulrow CD, Pocock SJ, et al. Strobe initiative. strengthening the reporting of observational studies in epidemiology (STROBE): explanation and elaboration. Int. J. surgery. (2014) 12:1500–24. doi: 10.1016/j.ijsu.2014.07.014
33. Zhu F, Chen C, Zhang Y, Chen S, Huang X, Li J, et al. Elevated blood mercury level has a non-linear association with infertility in US women: data from the NHANES 2013–2016. Reprod. Toxicology. (2020) 91:53–8. doi: 10.1016/j.reprotox.2019.11.005
34. Chiang JK, Lai NS, Chang JK, Koo M. Predicting insulin resistance using the triglyceride-to-high-density lipoprotein cholesterol ratio in Taiwanese adults. Cardiovasc. diabetology. (2011) 10:1–6. doi: 10.1186/1475-2840-10-93
35. Ding X, Wang X, Wu J, Zhang M, Cui M. Triglyceride–glucose index and the incidence of atherosclerotic cardiovascular diseases: a meta-analysis of cohort studies. Cardiovasc. Diabetology. (2021) 20:1–3. doi: 10.1186/s12933-021-01268-9
36. Lim J, Kim J, Koo SH, Kwon GC. Comparison of triglyceride glucose index, and related parameters to predict insulin resistance in Korean adults: An analysis of the 2007-2010 Korean national health and nutrition examination survey. PloS One (2019) 14:e0212963. doi: 10.1371/journal.pone.0212963
37. Park B, Lee HS, Lee YJ. Triglyceride glucose (TyG) index as a predictor of incident type 2 diabetes among nonobese adults: a 12-year longitudinal study of the Korean genome and epidemiology study cohort. Trans. Res. (2021) 228:42–51. doi: 10.1016/j.trsl.2020.08.003
38. Chen CL, Liu L, Lo K, Huang JY, Yu YL, Huang YQ, et al. Association between triglyceride glucose index and risk of new-onset diabetes among Chinese adults: findings from the China health and retirement longitudinal study. Front. Cardiovasc. Med. (2020) 7:610322. doi: 10.3389/fcvm.2020.610322
39. Zhang M, Liu D, Qin P, Liu Y, Sun X, Li H, et al. Association of metabolic score for insulin resistance and its 6-year change with incident type 2 diabetes mellitus. J. Diabetes. (2021) 13:725–34. doi: 10.1111/1753-0407.13161
40. Cai XT, Zhu Q, Liu SS, Wang MR, Wu T, Hong J, et al. Associations between the metabolic score for insulin resistance index and the risk of type 2 diabetes mellitus among non-obese adults: insights from a population-based cohort study. Int. J. Gen. Med. (2021) 14:7729–40. doi: 10.2147/IJGM.S336990
41. Borai A, Livingstone C, Kaddam I, Ferns G. Selection of the appropriate method for the assessment of insulin resistance. BMC Med. Res. methodology. (2011) 11:1–10. doi: 10.1186/1471-2288-11-158
42. Lillioja S, Mott DM, Spraul M, Ferraro R, Foley JE, Ravussin E, et al. Insulin resistance and insulin secretory dysfunction as precursors of non-insulin-dependent diabetes mellitus: prospective studies of pima indians. New Engl. J. Med. (1993) 329(27):1988–92. doi: 10.1056/NEJM199312303292703
43. Robertson RP, Harmon J, Tran PO, Poitout V. β-cell glucose toxicity, lipotoxicity, and chronic oxidative stress in type 2 diabetes. Diabetes. (2004) 53(suppl_1):S119–124. doi: 10.2337/diabetes.53.2007.s119
44. Kolb H, Kempf K, Röhling M, Martin S. Insulin: too much of a good thing is bad. BMC Med. (2020) 18:1–12. doi: 10.1186/s12916-020-01688-6
45. Papachristoforou E, Lambadiari V, Maratou E, Makrilakis K. Association of glycemic indices (hyperglycemia, glucose variability, and hypoglycemia) with oxidative stress and diabetic complications. J. Diabetes Res. (2020) 2020:7489795. doi: 10.1155/2020/7489795
46. Boden G, Lebed B, Schatz M, Homko C, Lemieux S. Effects of acute changes of plasma free fatty acids on intramyocellular fat content and insulin resistance in healthy subjects. Diabetes. (2001) 50:1612–7. doi: 10.2337/diabetes.50.7.1612
47. Rader DJ. Effect of insulin resistance, dyslipidemia, and intra-abdominal adiposity on the development of cardiovascular disease and diabetes mellitus. Am. J. Med. (2007) 120:S12–18. doi: 10.1016/j.amjmed.2007.01.003
48. Ikegami H, Babaya N, Noso S. β-cell failure in diabetes: Common susceptibility and mechanisms shared between type 1 and type 2 diabetes. J. Diabetes Invest. (2021) 12(9):1526–39. doi: 10.1111/jdi.13576
49. Singh RG, Yoon HD, Poppitt SD, Plank LD, Petrov MS. Ectopic fat accumulation in the pancreas and its biomarkers: a systematic review and meta-analysis. Diabetes/Metabolism Res. Rev. (2017) 33:e2918. doi: 10.1002/dmrr.2918
50. Kato KI, Takamura T, Takeshita Y, Ryu Y, Misu H, Ota T, et al. Ectopic fat accumulation and distant organ-specific insulin resistance in Japanese people with nonalcoholic fatty liver disease. PloS One (2014) 9:e92170. doi: 10.1371/journal.pone.0092170
51. Wueest S, Item F, Lucchini FC, Challa TD, Müller W, Blüher M, et al. Mesenteric fat lipolysis mediates obesity-associated hepatic steatosis and insulin resistance. Diabetes. (2016) 65:140–8. doi: 10.2337/db15-0941
52. Abraham TM, Pedley A, Massaro JM, Hoffmann U, Fox CS. Association between visceral and subcutaneous adipose depots and incident cardiovascular disease risk factors. Circulation. (2015) 132:1639–47. doi: 10.1161/CIRCULATIONAHA.114.015000
53. Jiang J, Deng S, Chen YI, Liang S, Ma N, Xu Y, et al. Comparison of visceral and body fat indices and anthropometric measures in relation to untreated hypertension by age and gender among Chinese. Int. J. Cardiol. (2016) 219:204–11. doi: 10.1016/j.ijcard.2016.06.032
54. Bello O, Mohandas C, Shojee-Moradie F, Jackson N, Hakim O, Alberti KG, et al. Black African men with early type 2 diabetes have similar muscle, liver and adipose tissue insulin sensitivity to white European men despite lower visceral fat. Diabetologia. (2019) 62:835–44. doi: 10.1007/s00125-019-4820-6
55. Garvey WT, Kwon S, Zheng D, Shaughnessy S, Wallace P, Hutto A, et al. Effects of insulin resistance and type 2 diabetes on lipoprotein subclass particle size and concentration determined by nuclear magnetic resonance. Diabetes. (2003) 52:453–62. doi: 10.2337/diabetes.52.2.453
56. Barazzoni R, Gortan Cappellari G, Ragni M, Nisoli E. Insulin resistance in obesity: an overview of fundamental alterations. Eating Weight disorders-studies Anorexia Bulimia Obes. (2018) 23:149–57. doi: 10.1007/s40519-018-0481-6
57. Gómez-Ambrosi J, Silva C, Galofré JC, Escalada J, Santos S, Millán D, et al. Body mass index classification misses subjects with increased cardiometabolic risk factors related to elevated adiposity. Int. J. Obes. (2012) 36:286–94. doi: 10.1038/ijo.2011.100
58. Ryoo JH, Park SK, Ye S, Choi JM, Oh CM, Kim SY, et al. Estimation of risk for diabetes according to the metabolically healthy status stratified by degree of obesity in Korean men. Endocrine (2015) 50:650–8. doi: 10.1007/s12020-015-0635-5
59. Ishola AF, Gerstein HC, Engert JC, Mohan V, Diaz R, Anand SS, et al. Longitudinal relationships between glycemic status and body mass index in a multiethnic study: evidence from observational and genetic epidemiology. Sci. Rep. (2016) 6:1–10. doi: 10.1038/srep30744
60. Oti SO, van de Vijver SJ, Agyemang C, Kyobutungi C. The magnitude of diabetes and its association with obesity in the slums of Nairobi, Kenya: results from a cross-sectional survey. Trop. Med. Int. Health (2013) 18:1520–30. doi: 10.1111/tmi.12200
Keywords: metabolic score for insulin resistance, insulin resistance, incident diabetes, relationship, nonlinear
Citation: Chen Z, Huang C, Zhou Z, Zhang Y, Xu M, Tang Y, Fan L and Feng K (2023) A nonlinear associations of metabolic score for insulin resistance index with incident diabetes: A retrospective Chinese cohort study. Front. Clin. Diabetes Healthc. 3:1101276. doi: 10.3389/fcdhc.2022.1101276
Received: 17 November 2022; Accepted: 23 December 2022;
Published: 12 January 2023.
Edited by:
Sueziani Binte Zainudin, Sengkang General Hospital, SingaporeReviewed by:
Ljiljana Lukic, Faculty of Medicine, University of Belgrade, SerbiaZhenwei Wang, Southeast University, China
Copyright © 2023 Chen, Huang, Zhou, Zhang, Xu, Tang, Fan and Feng. This is an open-access article distributed under the terms of the Creative Commons Attribution License (CC BY). The use, distribution or reproduction in other forums is permitted, provided the original author(s) and the copyright owner(s) are credited and that the original publication in this journal is cited, in accordance with accepted academic practice. No use, distribution or reproduction is permitted which does not comply with these terms.
*Correspondence: Kun Feng, ZmVuZ2t1bnN6QDE2My5jb20=