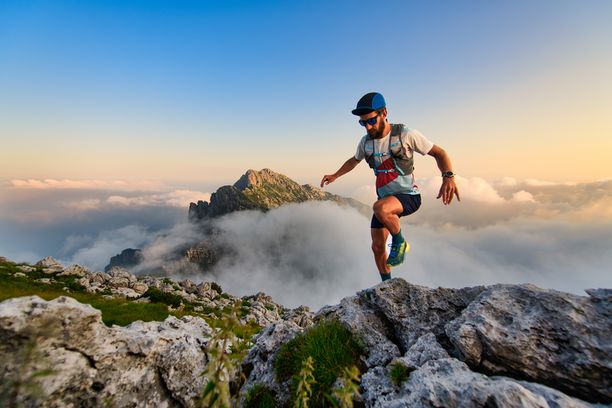
94% of researchers rate our articles as excellent or good
Learn more about the work of our research integrity team to safeguard the quality of each article we publish.
Find out more
ORIGINAL RESEARCH article
Front. Built Environ., 02 September 2024
Sec. Sustainable Design and Construction
Volume 10 - 2024 | https://doi.org/10.3389/fbuil.2024.1463658
This article is part of the Research TopicToward Sustainable Futures: Optimizing Building Energy Consumption for a Greener Built EnvironmentView all 5 articles
The current study investigated the impact of perceived instructional strategies in online design studios on student engagement as a multidimensional construct. Given the rapid shift to online learning during the COVID-19 pandemic, understanding effective teaching strategies and their impacts on student engagement is crucial for enhancing overall student outcomes. The study also examined the role of learning motivation as a mediator in these relationships. A mixed-methods approach was employed, measuring a second-order construct of student engagement based on three reflective dimensions: cognitive, affective, and behavioral. Qualitative interviews with instructors revealed three main instructional strategies utilized in online design studios: learning by doing, learning with interaction, and learning from feedback. The present study analyzed self-report survey responses from 230 undergraduate architecture students across four major universities in Iran, utilizing partial least squares structural equation modeling (PLS-SEM). Based on the results, the perceived learning by doing (PLD) and learning with interaction (PLI) significantly improve student engagement. In contrast, the perceived learning from feedback (PLF) fails to influence student engagement directly. Additionally, intrinsic and extrinsic motivation mediated the impact of PLI and PLF on student engagement. These findings highlight the importance of effective instructional strategies in online design studios. Additionally, the results provide valuable insights for future pedagogical practices in online education, offering guidance for instructors aiming to enhance student outcomes in this dynamic learning environment.
On 11 March 2020, WHO proclaimed the novel coronavirus (COVID-19) epidemic a pandemic (Cucinotta and Vanelli, 2020). In common with other human social activities, COVID-19 has significantly impacted all levels of education (Nicola et al., 2020) and has changed many education delivery methods worldwide (Yasmin, 2022). After the outbreak, some universities around the world ceased educational operations. Still, because there was no end to the pandemic, they had to choose a way to continue their education (Akin, 2002; Muthuprasad et al., 2021). Therefore, Online learning has become the primary alternative to face-to-face learning in many educational institutions (Almahasees et al., 2021). While distance and online education existed before the outbreak, their use was not as widespread as face-to-face classes (Andrade-Vargas et al., 2021), so teachers and students faced challenges along the way (Salahshori et al., 2022; Selvaraj et al., 2021; Al-Mawee et al., 2021), especially in studio-based design courses (Nubani and Lee, 2022). The first experience using online studios in design education dates back to the early 1990s (Akar et al., 2012). In parallel, research in this field began (McCullough et al., 1990; Sheldon et al., 1995; Kvan, 2001). However, online design education research has not kept pace with growing research in other disciplines in scope and number (Güler, 2022; Fleischmann, 2021). Hence, there is a need for more research in this field that can be helpful both in similar critical situations and in the future of design education because the impact of experiences gained during the pandemic on the future of design education cannot be ignored. In the meantime, engagement is critical to student academic achievement (Fredricks et al., 2004) and academic success (Carvalho et al., 2014; Fisher et al., 2018). Due to the distance between students and instructors, engaging them online is exceptionally challenging (Martin et al., 2020; Fisher et al., 2021). A deep understanding of this concept in online learning environments helps to improve their effectiveness. It is imperative to focus on student engagement to ensure an effective online learning environment. Therefore This study focuses on the relationship between perceived instructional strategies and student engagement in online design studios.
A concept known as student engagement has been the subject of numerous studies in education (Vayre and Vonthron, 2016; Karimian Shamsabadi et al., 2022). Also, according to Martin et al. (2020), Kolhe (2017), Kuo et al. (2014), student engagement has been a prominent theme throughout the past two decades of research into online learning. Many studies have explored its relationship to critical educational outcomes, including academic achievement and success (Wang and Degol, 2014; Pietarinen et al., 2014), academic performance (Wang and Holcombe, 2010), Learning satisfaction (She et al., 2021; Gao et al., 2020; Fisher et al., 2018). Studies have also addressed the possible predictors of student engagement, particularly the personality traits of the students (Durón-Ramos et al., 2020; Qureshi et al., 2016; Yeganeh and Kamalizadeh, 2018; Mclaughlan et al., 2021) and contextual and institutional factors (Christenson et al., 2012; Manwaring et al., 2017). Information in Table 1 shows the influence of various factors on student engagement in blended and online learning. However, there is no agreement on student engagement’s definition, dimensions, and measurement (Bond et al., 2020). Despite these challenges, studies consider it a complex and multidimensional construct that links the affective, cognitive, and behavioral factors in an integrated way. Observable behaviors like involvement and participation in learning activities describe behavioral engagement (Fredricks et al., 2004). Affective engagement refers to students’ emotional response to the learning environment, institution, and instructor (Pietarinen et al., 2014; Mohamed, 2021). Students’ cognitive engagement can be defined as their psychological investment in academic activities, their preference for mentally challenging activities, and their use of metacognitive strategies to regulate their cognition while performing tasks (Fredricks et al., 2004; Nazidizaji et al., 2014).
Table 1. An overview of articles discussing predictors of student engagement in online and blended learning.
In previous studies, the motivation of students has been investigated in both traditional and online learning contexts (Deci and Ryan, 1985; Vallerand et al., 1997; Yeganeh and Kamalizadeh, 2018; Boekaerts, 1999; Pintrich, 2002; Chen and Jang, 2010). Also, the effect of learning motivation on academic performance and achievement has been extensively investigated from various perspectives (Castillo-Merino and Serradell-López, 2014). Several theories and concepts have been proposed in the motivation field (Maslow, 1943; Herzberg et al., 1959; Deci and Ryan, 1985). Although not explicitly mentioned, intrinsic and extrinsic motivation are inherent in these theories (Singh et al., 2022). The definition of motivation relies on the distinction between extrinsic and intrinsic motivation (Ryan and Deci, 2000). An inherent tendency toward expiration and learning can describe the learner’s intrinsic motivation. In contrast, extrinsic motivation refers to learning to obtain a different outcome or when external reinforcement, such as rewards or pressure, is present (Ryan and Deci, 2000). At the same time, researchers examined the reciprocal relationship between motivation and engagement (Reeve and Lee, 2014). Appleton et al. (2008) suggested that learning motivation leads to student engagement. Intrinsic and extrinsic motivation predict student engagement. Studies showed the impact of intrinsic motivation on student engagement (Froiland and Worrell, 2016; Saeed and Zyngier, 2012). Oga-Baldwin et al. (2017) demonstrated how promoting autonomy and intrinsic motivation enhanced engagement. In addition, many studies highlight the links between students’ motivation and the learning environment factors (Matthews, 1991; Meece et al., 2003; Zare. et al., 2022).
Studio learning is broadly accepted in design education as the dominant delivery method (Crowther, 2013; Park, 2011). The significant factors in design education comprise: the studio environment, The manner of instructor-student communication, and teaching methods and studio management. Some studies investigated the characteristics and role of an effective instructor in the design studio (Goldschmidt et al., 2010), teaching practices (Salama, 2006), training strategies (Quayle, 1985), and teaching and learning strategies (Noels et al., 2019). The literature also has emphasized the importance of instructors’ instructional practices in enhancing students’ learning (Day et al., 2021; Sakhaei et al., 2020). Understanding instructors’ instructional strategies can help assess teaching and instructors (Kendall and Schussler, 2013; Sökmen, 2019; Motie et al., 2023) and reveal how they influence learning outcomes (Young et al., 2003). Instructional strategies are studied under different labels, such as teaching practices (Olivier, 2020), instructional Support (Marchand and Gutierrez, 2012; Maren et al., 2021), instructional practices (Webb et al., 2014), and classroom practices (Smith et al., 2005; Tucker and Rollo, 2006). Instructional strategies as contextual factors influence student engagement (Heilporn et al., 2022; Kahu, 2013; Christenson et al., 2012). Lekwa et al. (2018) divided teacher practices into two categories to understand their impact on student engagement: instructional and behavior management strategies. According to their study, both categories of strategies significantly enhanced students’ engagement with course content. Applying strategies from one domain can affect the strategy’s effectiveness in the other (Wang et al., 2016; Lekwa et al., 2018). Different approaches can be used to measure instructional strategies, including observation, self-report, and perception of students of teaching (Beck and Blumer, 2021; Goharian et al., 2023). Each of these approaches has weaknesses. However, perceptions of students can be used to measure teaching strategies in the classroom and improve teaching strategies (Wubbels et al., 2006; Ghanbari et al., 2022), and assess educational quality (Wang, 2010; Scherer et al., 2016). According to previous studies, Table 2 shows the most important instructional strategies used in the design studios.
The emergence of COVID-19 has transformed the educational system in numerous ways, making online, blended, and distance learning no longer a choice but a necessity. Some of these changes in the education system will persist after the epidemic. Previous studies have investigated the different aspects of online design education during the pandemic and emphasized the critical role of instructional strategies in the effectiveness of online learning (Xiang et al., 2018; Alsuwaida, 2022; Azizibabani et al., 2021). However, they still need to study the instructional strategies used in the online design studio context and their impact on student engagement. This investigation is the first to be conducted in architecture design education. The understanding student engagement concept can provide a deeper insight into the learning process. In different studies, student engagement has been conceptualized as a multidimensional construct with two to eight dimensions (Lanasa et al., 2009; Ashtari et al., 2021; Yeganeh, 2022).
Furthermore, there is evidence that engagement can be considered a higher-order construct (Martin, 2007; Maroco et al., 2016; Hosseini Alamdari et al., 2022). This study presents a model that examines the effect of perceived instructional strategies by students on their engagement. This model assumes that student engagement is a second-order concept characterized by three first-order dimensions: affective, behavioral, and cognitive. Considering the links among instructional strategies, learning motivation, and student engagement that were established separately, this model has a logical mediating relationship; instructional strategies indirectly influence student engagement through motivation (Figure 1)
In this paper, the following research hypotheses are specifically formulated:
H1. Perceived learning by doing (H1a), perceived learning with interaction (H1b), and perceived learning from feedback (H1c), are positively related to student engagement.
H2. Relation between perceived learning by doing (H2a), perceived learning with interaction (H2b), and perceived learning from feedback (H2c), and student engagement mediated by intrinsic motivation.
H3. Relation between perceived learning by doing (H3a), perceived learning with interaction (H3b), and perceived learning from feedback (H3c) and student engagement mediated by extrinsic motivation.
Two groups, university instructors and students, participated in this study. The samples were selected using the cluster sampling method. In the first phase, four universities in Iran were randomly selected. An email outlining the purpose of study was sent to instructors teaching architecture design at these four universities, inviting them to participate in the qualitative phase of the study. In the next phase of the study, an email containing the study’s purpose and a link to the survey was sent to students, inviting them to participate by completing the online questionnaire created using Google Forms.
In the first phase of research, qualitative methods in the form of open-ended interviews were utilized to comprehensively explore teachers’ views and experiences regarding their instructional strategies in online architecture studios. This approach allowed teachers to articulate their experiences in their own language, capturing the nuances of their teaching practices. The qualitative method is particularly effective in exploratory research, especially in contexts like online learning, where there is limited existing information (Cohen et al., 2018), and researchers aim to delve into the experiences and insights of teachers and students (Bdair, 2021; Liu, 2023). The sampling strategy employed was cluster sampling; for the qualitative phase of the research, ten teachers from four universities were selected, comprising six women and four men. In December 2021, data were collected from the qualitative phase. At the time of the interviews, participants had taught three semesters in the online design studio, with teaching experience ranging from two to 14 years. The primary researcher sent emails to teachers inviting them to participate in the study. The email included a detailed description of the study, including its aims and processes, as well as the researchers’ contact information. Recipients interested in participating were invited to reach out to the researchers to schedule an interview. Due to the COVID-19 pandemic, interviews were conducted via Skype to ensure the safety and convenience of all participants. Each interview lasted between 30 and 50 min and was recorded for analysis purposes. Participants were asked five questions during the interview, which can be found in Supplementary Appendix B. The recordings were transcribed verbatim. Two researchers analyzed the data collaboratively following Braun and Clarke’s six-step approach (Braun and Clarke, 2006). The following themes emerged from teachers’ interviews regarding their instructional strategies in the online design studio: (1) learning by doing, (2) learning with interaction, and (3) learning from feedback.
The findings obtained from these qualitative interviews subsequently contributed to the development of the scale for perceived instructional strategies in the following phase of the research, thereby enhancing the overall validity of the research findings.
The sample comprised students from ten online studios. Studios came from four different universities. Cluster sampling was the method used for selecting the sample. Online questionnaires created using Google Forms were distributed to students via email. The demographic characteristics of the study sample are summarized in Table 3. The study participants were 230 architecture undergraduates (68.7% female, 31.3% male). Their ages ranged from 19 to 36 (M = 22.08, SD = 2.95). Participants were spread across five semesters of study (32.6% in the fourth semester, 13% in the fifth semester, 23% in the sixth semester, 18.3% in the seventh semester, and 13.1% in the eighth semester). Students were examined at the end of the course to maximize the ecological validity of the study. They were asked to rate their learning motivation, perception of educational strategies, and engagement in the course.
This study used self-administered questionnaires for data collection. Two University professors specializing in design education evaluated the face and content validity of the questionnaire after the first draft was designed. Their feedback was incorporated into the survey instruments. There were two main sections to the survey questionnaire. Several five-point Likert scale questions in the first section of the questionnaire measured perceived instructional strategies, learning motivation, and student engagement. The second section of the survey asked about the student’s demographics, including age, gender, and Experience with an online architectural design studio. The main instrument consisted of three parts:
a) Learning motivation scale: The motivation scale for learning was composed of intrinsic and extrinsic factors. Intrinsic and extrinsic motivation scales were adapted from Intrinsic and Extrinsic Factors subscales, respectively (Ngan and Law, 2015). Intrinsic motivation with six items and extrinsic motivation with five items were adapted from the Intrinsic and Extrinsic Factors subscales (Ngan and Law, 2015), respectively.
b) Perceived instructional strategies: In this study, the scale of perceived instructional strategies was chiefly developed based on theoretical literature and data obtained during the qualitative phase of the study in three sections: (i) Perceived learning by doing (PLD) with five items. (ii) Perceived learning with interaction (PLI) with four items. (iii) Perceived learning from feedback (PLF) with four items.
c) Student engagement was measured with 21 items adapted from (Kong et al., 2003) to examine the three engagement factors with the following dimensions: (i) The affective engagement (ASE) with six items. (ii) The behavioral engagement (BSE) with eight items. (iii) The cognitive engagement (CSE) with seven items. Supplementary Appendix A contains items for learning motivation, perceived instructional strategies, and student engagement.
To analyze the data, SmartPLS 3.0 was used as a partial least squares structural equation modeling (PLS-SEM) tool, capable of assessing measurement models (associations between indicators and related constructs) and structural models (relationships between constructs) simultaneously. PLS-SEM was chosen as the appropriate model for testing small sample sizes (Hair al., 2021) and complex models such as higher-order models (Sarstedt et al., 2019). This paper conceptualizes student engagement as a two-order construct with cognitive, affective, and behavioral dimensions. According to prior studies, reflective-reflective and reflective-formative constructs in PLS-SEM be prominent in various fields (Becker et al., 2012; Sarstedt et al., 2019). According to the literature review, a reflective-reflective second-order construct is presented in the present study. Two commonly used methods for validating and assessing higher-order constructs include the two-stage and repeated indicator approaches. In addition, two versions of the two-stage approach have been proposed, (1) the embedded and (2) the disjoint approach. (Sarstedt et al., 2019). This paper establishes and estimates a path model based on the two-stage disjoint approach. Therefore, there were two significant steps to analyze the two-order path model conceptualized for this article. As a first step, we modeled the path only based on the first-order components of the second-order construct (without considering the second-order construct). Each of these is directly connected to all constructs to which the second-order construct is theoretically associated. We then examined the relationship between first-order components and measurement items via a measurement model assessment. We then recorded the latent variables (LVs) of three components of the second-order construct (BSE, ASE, and CSE). LVs were saved in the original data file and used In step two to analyze the two-order construct. This analysis was performed to estimate the model and validate the hypothesis (Figure 2).
The assessment and interpretation of the PLS model consist of two parts: (1) assessing the reliability and validity of the measurement model and (2) evaluating the structural model. In this paper, the confirmatory factor analysis (CFA) was performed as part of the evaluation process of the measurement model to refine and confirm the items and construct. In the current step, we evaluated three criteria: internal consistency reliability, convergent validity, and discriminant validity (Sarstedt et al., 2019).
This study uses Cronbach’s alpha and composite reliability (CR) to measure reliability. Cronbach’s alpha values above 0.7 indicate admissible reliability (Nunnally, 1978). According to Table 4, Cronbach’s alpha values are between 0.709 and 0.919, which is acceptable. Additionally, the value of the combined reliability is more significant than 0.7 for each variable in Table 4, indicating an excellent internal consistency for the model (Nunnally, 1978). The average variance extracted (AVE) was used to evaluate convergence validity, confirmed for all structures that exceeded the threshold value 0.50.
The discriminant validity of a construct refers to how it distinguishes itself from others. To assess each construct’s discriminant validity, we compared the square root of AVEs with their correlations with other latent constructs (i.e., the Fornell-Larcker criterion). In each construct pair, the square root of the AVE was higher than the correlation with other constructs, which indicates good discriminant validity (Fornell and Larcker, 1981). The discriminant validity test for the constructs can be seen in Table 5. In summary, evaluations of the first-order measurement model conformed to internal consistency reliability, convergent validity, and discriminant validity requirements.
In the next step, the model of second-order measurement was evaluated. According to the conceptual model suggestion, student engagement (SE) has three reflective components: ‘cognitive engagement,’ ‘affective engagement,’ and ‘behavioral engagement.’ Stage two focuses on evaluating the second-order measurement model. Table 6 indicates factor loading, CA, rho_A, CR, and AVE of higher-order construct. Thus, the reflective nature of SE was supported (Table 7).
We also check the model’s fitness before evaluating the structural model. The SRMR value was 0.063, below the recommended value of 0.08, and the NFI value was 0.95, which Hu and Bentler (1999) consider acceptable. Therefore, the model has a good level of fit.
The structural model was tested using the bootstrapping procedure with 5,000 samples in step two. Table 8 presents the results of this analysis. Hair et al. (2014) state that when the t-value exceeds 1.96, the path relationship is assumed to be significant. Two instructional strategies directly affected student engagement (H1b, H1a): PLI and PLD. No direct effect was found for PLF (H1c). It can be concluded that student engagement was positively influenced by factors such as PLI (ßPLI→SE = 0.192, t = 3.065, p = 0.002), PLD (ßPLD→SE = 0.225, t = 3.753, p = 0.000). In contrast, PLF did not directly affect student engagement (ßPLF→SE = 0.073, t = 1.331, p = 0.183 > 0.05), Unlike the proposed H1c. Furthermore, PLF and PLI were significantly associated with IM, with coefficient values of 0.567 (p = 0.000) and 0.178 (p = 0.019), respectively. Also, PLF and PLI were significantly associated with EM, with coefficient values of 0.316 (p = 0.000) and 0.302 (p = 0.000), respectively. Contrary to this, PLD did not affect the EM and IM (ßPLD→EM = 0.052, t = 699, p = 0.485 > 0.05) (ßPLD→IM = 0.002, t = 0.036, p = 0.971 > 0.05).
According to the results of the mediation test, there was a significant indirect effect between the PLF and SE (H3c) (ßPLF→EM→SE = 0.088; t = 3.540; p = .000), and the PLF and SE (H2c) (ßPLF→IM→SE = 0.148; t = 3.793; p = .000) showing that learning motivation mediates the relationship between PLF and SE. In addition, the mediation effects of learning motivation on the relationship between PLI and SE (H3b) (ßPLI→EM→SE = 0.083; t = 3.177; p = .001) and PLI and SE (H2b) (ßPLI→IM→SE = 0.047; t = 1.968; p = .049) revealed in this test. In contrast, IM and EM did not have a mediation effect on the relationship between PLD and SE (H3a, H2a) (ßPLD→EM→SE = 0.014, t = 667, p = 0.505 > 0.05) (ßPLD→IM→SE = 0.001, t = 0.036, p = 0.971 > 0.05).
The effects of different factors on student engagement are summarized in Table 9. Based on Table 9, among instructional strategies, the total effect of PLI on SE (β = 0.321, p = 0.000) was the most significant, followed by PLF (β = 0.309, p = 0.000), PLD (β = 0.270, p < 0.000), respectively.
In assessing the SEM, the R2 value and Q2 value are the essential criteria for predictive accuracy and relevance. The coefficient of determination (R2) value assesses predictive accuracy. According to Hair et al. (2014), R2 values vary from 0 to 1, with a higher value of R2 indicating a higher predictive accuracy. The SE construct had an R2 value of 0.685 (>0.67), which Henseler et al., 2009 regarded as a significant level of predictive accuracy. While a moderate level of R2 was found for IM and EM (>0.33). The predictive relevance was evaluated using the blindfolding method to calculate the Q2 value. As Henseler et al. (2009) described, Q2 values above zero indicate good reconstruction of the observations and predictive relevance for the model. The results of this study indicate that Q2 values for constructs are above zero, indicating a sufficiently predictive model.
There is limited evidence to support theorized associations between student engagement, perceived instructional strategies, and learning motivation. The current study developed previous research by investigating the relationships among three instructional strategies (learning by doing, learning with peers, and learning from feedback), learning motivation (intrinsic and extrinsic motivation), and student engagement in online studio contexts. This research provides significant insight into the instructional strategies specific to the architecture design online studio that can lead to high levels of student engagement. The study’s results suggest that perceived instructional strategies by the student can play essential roles in student engagement. In the first place, among the instructional strategies examined in this study, perceived learning with interaction (PLI) was the most significant predictor of student engagement. Specifically, our results clarify the need to use learning with interaction as an instructional strategy and emphasize the values of group discussion, teamwork, description of the design process, and interaction through online tools. Therefore, interaction is critical in online studio settings and for engagement in course content. Learning with interaction strategies that increase student communication should be applied in the future. Our result would align with Wang and Eccles’s (2013) research, showing that students’ peer interaction significantly affects their engagement. Our finding also recalls Xu et al. (2020) results indicating that student behavioral and cognitive engagement increase when instructors facilitate online discussion. Intrinsic and extrinsic motivation mediated the relationship between perceived learning with interaction and student engagement. It meant that perceived learning with interaction could predict intrinsic and extrinsic motivation, predicting student engagement. Previously, the neural bases of the relationship between social interaction and intrinsic motivation in educational environments have been confirmed by Clark and Dumas (2015) and how instructors use social strategies to Develop motivation to learn.
In the second place, the direct effect of perceived learning from feedback on student engagement was not significant. Learning motivation fully mediates perceived learning from feedback and student engagement. Specifically, this strategy involved receiving timely and sufficient feedback from the instructor, peer feedback, using drawings and case studies as feedback, and self-feedback. It shows that this strategy does not directly affect student engagement, but it does so indirectly by causing changes in student motivation. One possible explanation for this finding is that feedback may only directly engage students if perceived as helpful and relevant to their learning goals. Therefore, the impact of feedback on student engagement may be mediated by the level of learning motivation, which is influenced by the perceived value and relevance of the feedback. Based on the results, it is clear that as students get feedback that is clear and timely, that helps them realize their mistakes, that helps them learn, that helps them improve design quality, that makes them think, there is a higher probability that they will be motivated in the online studio. Our findings follow the results proposed in the research that feedback can improve intrinsic motivation. Overall, the findings of this study highlight the importance of understanding the complex relationship between feedback, motivation, and engagement in the learning process. By considering these factors, instructors can develop more effective instructional strategies that promote student engagement and learning outcomes.
Finally, perceived learning by doing (PLD) significantly affected student engagement. Specifically, this strategy involved providing step-by-step instructions for designs, analyzing and presenting examples, making physical models, diagrams, and sketches, solving a specific design problem with the instructor, and providing similar assignments for students.
The mediating relationship between learning by doing and student engagement through intrinsic and extrinsic motivations was not confirmed. This finding indicates that the levels of intrinsic or extrinsic motivation did not influence the impact of learning by doing on student engagement. One possible explanation for this finding is that learning by doing is inherently active and engaging for many students because it involves participation, problem-solving, and practical application. This suggests that the act itself can directly enhance engagement, regardless of students’ motivational levels. Moreover, the assignments related to learning by doing in online studios may be challenging, which could limit the impact of this instructional strategy on motivation to learn. Students may feel overwhelmed or discouraged by the difficulty of the assignments, which could limit their motivation to engage in the learning process. Online learning environments often introduce additional cognitive demands, such as navigating technology and managing distractions at home. These factors, combined with the challenges inherent in learning by doing, can increase cognitive load, making it more difficult for students to stay focused and motivated. Finally, if the tasks associated with learning by doing are perceived as monotonous or excessively challenging without direct teacher involvement, students may struggle to maintain their motivation. As a result, the relationship between learning by doing, motivation, and engagement is likely complex and may involve other mediating or moderating variables not accounted for in the study, such as self-efficacy or peer influence or instructor’s supervision. Further research is needed to explore these additional factors and their potential impact on student engagement in online learning contexts.
Consequently, while the PLD strategy effectively promoted student engagement, the lack of a mediating effect through intrinsic and extrinsic motivation suggests that instructional modifications may be needed to optimize its impact on student motivation and engagement.
Several limitations exist in the present study. First, it is difficult to generalize the study’s conclusions due to the inadequate sample size. More students and universities should be selected to make the conclusion more generalizable. Second, in this study, we used a cross-sectional research design in that all variables are self-reported simultaneously in this study. In future research, longitudinal designs can be used to test causal relationships among variables.
Moreover, although self-reported data may provide a view into students’ subjective perceptions and actions that cannot be observed directly (Fredricks and McColskey, 2012), they only provide a limited picture (Kirschner and Van Merrienboer, 2013). Class observations can be used to supplement it. The present study tests the relationships among perceived instructional strategies, motivation, and engagement dimensions. In future research, multilevel analysis can be used to test relationships between instructional strategies and student engagement. While the findings confirmed the impact of instructional strategies on student engagement in online studios, future studies could include additional factors, such as learning outcomes (e.g., performance and satisfaction).
The current study is the first to examine the relationship between perceived instructional strategies in online studios and student engagement. As a result of these findings, it is clear that instructional strategy plays a crucial role in engaging students, both directly and via learning motivation. Based on the results, among the instructional strategy, learning with interaction significantly impacted students’ engagement in architectural design online studios. This study has several theoretical implications. Our findings extend the theoretical framework by demonstrating differential relationships between instructional strategies (e.g., learning with interaction, learning from feedback, and learning by doing) and student engagement. This research also offers several practical implications for establishing how instructors provide instructional strategies to students and emphasizes the importance of motivating students to learn in online studios. Instructors must encourage their students to interact with one another and collaborate while learning.
The study received ethical approval from the Research Ethics Committees of Tarbiat Modares University. In both the qualitative interviews and quantitative surveys, participants were provided with an explanation in easy-to-understand language that comprehensively informed them about the study’s aims and procedures. Participants in the qualitative phase signed an Electronic Consent Statement prior to interview, while those in the quantitative phase provided informed consent by answering a question at the beginning of the online survey. Participation was voluntary, and individuals had the right to withdraw at any time. To ensure participant privacy, all data were kept confidential and used exclusively for research purposes.
The raw data supporting the conclusions of this article will be made available by the authors, without undue reservation.
The studies involving humans were approved by Tarbiat Modares University, Tehran, Iran. The studies were conducted in accordance with the local legislation and institutional requirements. The participants provided their written informed consent to participate in this study.
FB: Conceptualization, Data curation, Investigation, Methodology, Resources, Software, Validation, Visualization, Writing–original draft. MY: Conceptualization, Methodology, Project administration, Supervision, Validation, Writing–review and editing. MB: Investigation, Methodology, Validation, Writing–review and editing.
The author(s) declare that no financial support was received for the research, authorship, and/or publication of this article.
The authors declare that the research was conducted in the absence of any commercial or financial relationships that could be construed as a potential conflict of interest.
All claims expressed in this article are solely those of the authors and do not necessarily represent those of their affiliated organizations, or those of the publisher, the editors and the reviewers. Any product that may be evaluated in this article, or claim that may be made by its manufacturer, is not guaranteed or endorsed by the publisher.
Akar, E., Turhan, S., Ozgen, D., Coskun, A., and Oraklibel, R. D. (2012). Online studio in the industrial design education: the implications of kibis as the organizational communication and knowledge management system. Procedia - Soc. Behav. Sci. 47, 771–775. doi:10.1016/j.sbspro.2012.06.732
Akin, O. (2002). Case-based instruction strategies in architecture. Des. Stud. 23 (4), 407–431. doi:10.1016/S0142-694X(01)00046-1
Almahasees, Z., Mohsen, K., and Amin, M. O. (2021). Faculty’s and students’ perceptions of online learning during COVID-19. Front. Educ. 6. doi:10.3389/feduc.2021.638470
Al-Mawee, W., Kwayu, K. M., and Gharaibeh, T. (2021). Student's perspective on distance learning during COVID-19 pandemic: a case study of Western Michigan University, United States. Int. J. Educ. Res. Open 2, 100080. doi:10.1016/j.ijedro.2021.100080
Alsuwaida, N. (2022). Online courses in art and design during the coronavirus (COVID-19) pandemic: teaching reflections from a first-time online instructor. SAGE Open. doi:10.1177/21582440221079827
Andrade-Vargas, L., Estevao-Romeiro, A., Iriarte-Solano, M., Riofrio-Leiva, V., and Yunga-Godoy, D. (2021). Teacher's perceptions, institutional challenges, and educational sustainability during Covid-19 in Ecuador. Heliyon 7 (12), e08596. doi:10.1016/j.heliyon.2021.e08596
Appleton, J., Christenson, S., and Furlong, M. (2008). Student engagement with school: critical conceptual and methodological issues of the construct. Psychol. Sch. 45, 369–386. doi:10.1002/pits.20303
Ashtari, B., Yeganeh, M., Bemanian, M., and Vojdani Fakhr, B. (2021). A conceptual review of the potential of cool roofs as an effective passive solar technique: elaboration of benefits and drawbacks. Front. Energy Res. 9, 738182. doi:10.3389/fenrg.2021.738182
Azizibabani, M., Bemanian, M., and Yeganeh, M. (2021). Investigation of the effects of applying social sustainability components on residential satisfaction. J. Sustain. Archit. Civ. Eng. 29 (2), 49–61. doi:10.5755/j01.sace.29.2.29217
Barratt, J. M., and Duran, F. (2021). Does psychological capital and social support impact engagement and burnout in online distance learning students? Internet High. Educ. 51, 100821. doi:10.1016/j.iheduc.2021.100821
Bdair, I. A. (2021). Nursing students' and faculty members' perspectives about online learning during COVID-19 pandemic: a qualitative study. Teach. Learn. Nurs. 16 (Issue 3), 220–226. doi:10.1016/j.teln.2021.02.008
Beck, C. W., and Blumer, L. S. (2021). The relationship between perceptions of instructional practices and student self-efficacy in guided-inquiry laboratory courses. CBE Life Sci. Educ. 20 (1), ar8. doi:10.1187/cbe.20-04-0076
Becker, J. M., Klein, K., and Wetzels, M. (2012). Hierarchical latent variable models in PLS-SEM: guidelines for using reflective-formative type models. Long. Range Plan. 45, 359–394. doi:10.1016/j.lrp.2012.10.001
Boekaerts, M. (1999). Motivated learning: studying student situation transactional units. Eur. J. Psychol. Educ. 14 (1), 41–55. doi:10.1007/BF03173110
Bond, M., Buntins, K., Bedenlier, S., Zawacki-Richter, O., and Kerres, M. (2020). Mapping research in student engagement and educational technology in higher education: a systematic evidence map. Int. J. Educ. Technol. High. Educ. 17, 2–30. doi:10.1186/s41239-019-0176-8
Braun, V., and Clarke, V. (2006). Using thematic analysis in psychology. Qual. Res. Psychol. 3 (2), 77–101. doi:10.1191/1478088706qp063oa
Carvalho, C., Santos, J., Conboy, J., and Martins, D. (2014). Teachers’ feedback: exploring differences in students’ perceptions. Procedia-Social Behav. Sci. 159, 169–173. doi:10.1016/j.sbspro.2014.12.351
Castillo-Merino, D., and Serradell-López, E. (2014). An analysis of the determinants of students’ performance in E-learning. Comput. Hum. Behav. 30, 476–484. doi:10.1016/j.chb.2013.06.020
Chen, K. C., and Jang, S. J. (2010). Motivation in online learning: testing a model of self-determination theory. Comput. Hum. Behav. 26, 741–752. doi:10.1016/j.chb.2010.01.011
Chen, P. D., Dumford, A., and Guidry, K. (2010). Engaging online learners: the impact of Web-based learning technology on college student engagement. Comput. and Educ. 54, 1222–1232. doi:10.1016/j.compedu.2009.11.008
Christenson S. L., Reschly A. L., and Wylie C. (2012). Handbook of research on student engagement (Springer Science + Business Media). doi:10.1007/978-1-4614-2018-7
Clark, I., and Dumas, G. (2015). Toward a neural basis for peer-interaction: what makes peer-learning tick? Front. Psychol. 6, 28. doi:10.3389/fpsyg.2015.00028
Cohen, L., Manion, L., Morrison, K., and Research, K. (2018). Methods in education. 8th ed. London: Routledge.
Crowther, P. (2013). Understanding the signature pedagogy of the design studio and the opportunities for its technological enhancement. J. Learn. Des. 6 (3). doi:10.5204/jld.v6i3.155
Cucinotta, D., and Vanelli, M. (2020). WHO declares COVID-19 a pandemic. Acta Biomed. 91 (1), 157–160. doi:10.23750/abm.v91i1.9397
Day, T., Chang, I. C. C., Chung, C. K. L., Doolittle, W. E., Housel, J., and McDaniel, P. N. (2021). The immediate impact of COVID-19 on postsecondary teaching and learning. Prof. Geogr. 73 (1), 1–13. doi:10.1080/00330124.2020.1823864
Deci, E. L., and Ryan, R. M. (1985). Intrinsic motivation and self-determination in human behavior. Berlin: Springer Science and Business Media. doi:10.1007/978-1-4899-2271-7
Durón-Ramos, M. F., Mojica-Gómez, P. A., Villamizar-Gomez, K., and Chacón-Andrade, E. R. (2020). Impact of positive personal traits on university student engagement in Mexico, Colombia, and El Salvador. Front. Genet. 5, 12. doi:10.3389/feduc.2020.00012
Fisher, R., Perényi, Á., and Birdthistle, N. (2018). The positive relationship between flipped and blended learning and student engagement, performance, and satisfaction. Act. Learn. High. Educ. 22, 97–113. doi:10.1177/1469787418801702
Fisher, R., Perényi, Á., and Birdthistle, N. (2021). The positive relationship between flipped and blended learning and student engagement, performance and satisfaction. Act. Learn. High. Educ. 22 (2), 97–113. doi:10.1177/1469787418801702
Fleischmann, K. (2021). Hands-on versus virtual: reshaping the design classroom with blended learning. Arts Humanit. High. Educ. 20 (1), 87–112. doi:10.1177/1474022220906393
Fornell, C., and Larcker, D. F. (1981). Evaluating structural equation models with unobservable variables and measurement error. J. Mark. Res. 18 (1), 39–50. doi:10.2307/3151312
Fredricks, J. A., Blumenfeld, P. C., and Paris, A. H. (2004). School engagement: potential of the concept, state of the evidence. Rev. Educ. Res. 74 (1), 59–109. doi:10.3102/00346543074001059
Fredricks, J. A., and McColskey, W. (2012). “The measurement of student engagement: a comparative analysis of various methods and student self-report instruments,” in Handbook of research on student engagement. Editors S. L. Christenson, A. L. Reschly, and C. Wylie (Springer Science + Business Media), 763–782. doi:10.1007/978-1-4614-2018-7_37
Froiland, J. M., and Worrell, F. C. (2016). Intrinsic motivation, learning goals, engagement, and achievement in a diverse high school. Psychol. Sch. 53 (3), 321–336. doi:10.1002/pits.21901
Gao, B. W., Jiang, J., and Tang, Y. (2020). The effect of blended learning platform and engagement on students’ satisfaction—the case from the tourism management teaching. J. Hosp. Leis. Sport and Tour. Educ. 27, 100272. doi:10.1016/j.jhlste.2020.100272
Ghanbari, S., Yeganeh, M., and Bemanian, M. (2022). Architecture typology of rural plain houses based on formal features, case study:(talesh, Iran). Front. Built Environ. 8, 856567. doi:10.3389/fbuil.2022.856567
Goldschmidt, G., Hochman, H., and Dafni, I. (2010). The design studio “crit”: teacher–student communication. Artif. Intell. Eng. Des. Analysis Manuf. 24, 285–302. doi:10.1017/S089006041000020X
Güler, K. (2022). Structuring knowledge-building in online design education. Int. J. Technol. Des. Educ. 33, 1055–1086. doi:10.1007/s10798-022-09756-z
Hair, J. F., Hult, G. T. M., Ringle, C. M., and Sarstedt, M. (2014). A primer on partial least squares structural equation modeling (PLS-SEM). Sage.
Hair, J. F., Hult, G. T. M., Ringle, C. M., Sarstedt, M., Danks, N. P., and Ray, S. (2021). Partial least squares structural equation modeling (PLS-SEM) using R: a workbook (classroom companion: business). Springer.
Heilporn, G., Lakhal, S., and Belisle, M. (2021). An examination of teachers' strategies to foster student engagement in blended learning in higher education. Int. J. Educ. Technol. High. Educ. 18, 25. doi:10.1186/s41239-021-00260-3
Heilporn, G., Lakhal, S., and Bélisle, M. (2022). Examining effects of instructional strategies on student engagement in blended online courses. J. Comput. Assisted Learn. 38, 1657–1673. doi:10.1111/jcal.12701
Henseler, J., Ringle, C., and Sinkovics, R. (2009). The use of partial least squares path modeling in international marketing. Adv. Int. Mark. 20, 277–319. doi:10.1108/S1474-7979(2009)0000020014
Herzberg, F., Mausner, B., and Snyderman, B. B. (1959). The motivation to work. New York: John Willey and sons.
Hoi, V. N., and Hang, H. L. (2021). The structure of student engagement in online learning: a bi-factor exploratory structural equation modelling approach. J. Comput. Assisted Learn. 37, 1141–1153. doi:10.1111/jcal.12551
Hosseini Alamdari, A., Daneshjoo, K., and Yeganeh, M. (2022). New algorithms for generating isovist field and isovist measurements. Environ. Plan. B Urban Anal. City Sci. 49 (9), 2331–2344. doi:10.1177/23998083221083680
Hu, L. T., and Bentler, P. M. (1999). Cutoff criteria for fit indexes in covariance structure analysis: conventional criteria versus new alternatives. Struct. Equ. Model. A Multidiscip. J. 6 (1), 1–55. doi:10.1080/10705519909540118
Juan, S. (2021). Promoting engagement of nursing students in online learning: use of the student-generated question in a nursing leadership course. Nurse Educ. Today 97, 104710. doi:10.1016/j.nedt.2020.104710
Kahu, E. R. (2013). Framing student engagement in higher education. Stud. High. Educ. 38 (5), 758–773. doi:10.1080/03075079.2011.598505
Karimian Shamsabadi, M., Yeganeh, M., and Pourmahabadian, E. (2022). Urban buildings configuration and pollutant dispersion of PM 2.5 particulate. Front. Sustain. Food Syst. 339. doi:10.3389/fsufs.2022.898549
Kendall, K. D., and Schussler, E. E. (2013). Evolving impressions: undergraduate perceptions of graduate teaching assistants and faculty members over a semester. CBE Life Sci. Educ. 12 (1), 92–105. doi:10.1187/cbe.12-07-0110
Kirschner, P., and Van Merrienboer, J. J. G. (2013). Do learners really know best? Urban legends in education. Educ. Psychol. 48, 169–183. doi:10.1080/00461520.2013.804395
Kolhe, N. (2017). “Innovative tools and techniques to teach architecture,” in International journal of engineering research and technology (India: International Research Publication House. Nagpur), 10, 67–71.
Kong, Q. P., Wong, N. Y., and Lam, C. C. (2003). Student engagement in mathematics: development of instrument and validation of construct. Math. Educ. Res. J. 15, 4–21. doi:10.1007/bf03217366
Kuo, Y. C., Walker, A., Schroder, K., and Belland, B. (2014). Interaction, Internet self-efficacy, and self-regulated learning as predictors of student satisfaction in online education courses. Internet High. Educ. 20, 35–50. doi:10.1016/j.iheduc.2013.10.001
Kvan, T. (2001). The pedagogy of virtual design studios. Automation Constr. 10, 345–353. doi:10.1016/S0926-5805(00)00051-0
Lanasa, S. M., Cabrera, A. F., and Trangsrud, H. (2009). The construct validity of student engagement: a confirmatory factor analysis approach. Res. High. Educ. 50, 315–332. doi:10.1007/s11162-009-9123-1
Lekwa, A., Reddy, L., and Shernoff, E. (2018). Measuring teacher practices and student academic engagement: a convergent validity study. Sch. Psychol. Q. 34, 109–118. doi:10.1037/spq0000268
Liu, Y. (2023). Matches and mismatches between university teachers’ and students’ perceptions of E-learning: a qualitative study in China. Heliyon 9 (6), e17496. doi:10.1016/j.heliyon.2023.e17496
Manwaring, K., Larsen, R., Graham, C., Henrie, C., and Halverson, L. (2017). Investigating student engagement in blended learning settings using experience sampling and structural equation modeling. Internet High. Educ. 35, 21–33. doi:10.1016/j.iheduc.2017.06.002
Marchand, G., and Gutierrez, D. B. A. (2012). The role of emotion in the learning process: comparisons between online and face-to-face learning settings. Internet High. Educ. 15, 150–160. doi:10.1016/j.iheduc.2011.10.001
Maren, S. L., Sigrun, K. E., and Tuomo, V. (2021). Patterns of teachers’ instructional support quality and the association with job satisfaction and collegial collaboration. Educ. Psychol. 41 (10), 1300–1318. doi:10.1080/01443410.2021.1988519
Maroco, J., Maroco, A. L., Campos, J. A. D. B., and Fredricks, J. A. (2016). University student’s engagement: development of the university student engagement inventory (USEI). Psicol. Reflexão Crítica 29, 21. doi:10.1186/s41155-016-0042-8
Martin, A. J. (2007). Examining a multidimensional model of student motivation and engagement using a construct validation approach. Br. J. Educ. Psychol. 77, 413–440. doi:10.1348/000709906X118036
Martin, F., Sun, T., and Westine, C. D. (2020). A systematic review of research on online teaching and learning from 2009 to 2018. Comput. and Educ. 159, 104009. doi:10.1016/j.compedu.2020.104009
Martin, F., Wang, C., and Sadaf, A. (2018). Student perception of helpfulness of facilitation strategies that enhance instructor presence, connectedness, engagement and learning in online courses. Internet High. Educ. 37, 52–65. doi:10.1016/j.iheduc.2018.01.003
Maslow, A. H. (1943). A theory of human motivation. Psychol. Rev. 50 (4), 370–396. doi:10.1037/h0054346
Matthews, D. B. (1991). The effects of school environment on intrinsic motivation of middle-school children. J. Humanist. Educ. Dev. 30, 30–36. doi:10.1002/j.2164-4683.1991.tb00028.x
McCullough M., William J. M., and Patrick P. (1990). The electronic design studio, architectural knowledge and media in the computer era (Cambridge, MA, U.S.A.: MIT Press).
Mclaughlan, R., Pert, Al., and Lodge, J. M. (2021). Productive uncertainty: the pedagogical benefits of Co-creating research in the design studio. Int. J. Art Des. Educ. 40, 184–200. doi:10.1111/JADE.12344
McNeill, K. L. (2009). Teachers' use of curriculum to support students in writing scientific arguments to explain phenomena. Sci. Educ. 93, 233–268. doi:10.1002/sce.20294
Meece, J. L., Herman, P., and McCombs, B. L. (2003). Relations of learner-centered teaching practices to adolescents’ achievement goals. Int. J. Educ. Res. 39, 457–475. doi:10.1016/j.ijer.2004.06.009
Mohamed, K. (2021). A revised experimental method of integrating sustainability into the undergraduate design studio. Int. J. Sustain. Des. 4, 23. doi:10.1504/IJSDES.2021.115464
Motie, M. R., Yeganeh, M., and Bemanian, M. R. (2023). Assessment of greenery in urban canyons to enhance thermal comfort and air quality in an integrated seasonal model. Appl. Geogr. 151 (February 2023), 102861. doi:10.1016/j.apgeog.2022.102861
Muthuprasad, T., Aiswarya, S., Aditya, K. S., and Jha, G. K. (2021). Students' perception and preference for online education in India during COVID -19 pandemic. Soc. Sci. Humanit. Open 3 (1), 100101. doi:10.1016/j.ssaho.2020.100101
Nazidizaji, S., Tomé, A., and Regateiro, F. (2014). Search for design intelligence: a field study on the role of emotional intelligence in architectural design studios. Front. Archit. Res. 3 (4), 413–423. doi:10.1016/j.foar.2014.08.005
Ngan, S. C., and Law, K. M. Y. (2015). Exploratory network analysis of learning motivation factors in e-learning facilitated computer programming courses. Asia-Pacific Educ. Res. 24, 705–717. doi:10.1007/s40299-014-0223-0
Nicola, M., Alsafi, Z., Sohrabi, C., Kerwan, A., Al-Jabir, A., Iosifidis, C., et al. (2020). The socio-economic implications of the coronavirus pandemic (COVID-19): a review. Int. J. Surg. 78, 185–193. doi:10.1016/j.ijsu.2020.04.018
Noels, K. A., Vargas Lascano, D. I., and Saumure, K. (2019). The development of self-determination across the language course. Stud. Second Lang. Acquis. 41, 821–851. doi:10.1017/S0272263118000189
Nubani, L., and Lee, E. (2022). Sense of classroom community in interior design studios: in-person learning versus online learning approaches. J. Interior Des. 47, 51–70. doi:10.1111/joid.12217
Oga-Baldwin, W. L. Q., Nakata, Y., Parker, P. D., and Ryan, R. M. (2017). Motivating young language learners: a longitudinal model of self-determined motivation in elementary school foreign language classes. Contemp. Educ. Psychol. 49, 140–150. doi:10.1016/j.cedpsych.2017.01.010
J. Olivier (2020). Self-directed multimodal learning in higher education. NWU Self-Directed Learning Series (Cape Town: AOSIS), 5.
Park, J. Y. (2011). Design education online: learning delivery and evaluation. Int. J. Art and Des. Educ. 30 (2), 176–187. doi:10.1111/j.1476-8070.2011.01689.x
Pietarinen, J., Soini, T., and Pyhältö, K. (2014). Students’ emotional and cognitive engagement as the determinants of well-being and achievement in school. Int. J. Educ. Res. 67, 40–51. doi:10.1016/j.ijer.2014.05.001
Pintrich, P. (2002). The role of metacognitive knowledge in learning, teaching, and assessing. Theory Into Pract. 41, 219–225. doi:10.1207/s15430421tip4104_3
Quigley, M., Bradley, A., Playfoot, D., and Harrad, R. (2022). Personality traits and stress perception as predictors of students' online engagement during the COVID-19 pandemic. Personality Individ. Differ. 194, 111645. doi:10.1016/j.paid.2022.111645
Qureshi, A., Wall, H., Humphries, J., and Balani, A. (2016). Can personality traits modulate student engagement with learning and their attitude to employability? Learn. Individ. Differ. 51, 349–358. doi:10.1016/j.lindif.2016.08.026
Reeve, J., and Lee, W. (2014). Students’ classroom engagement produces longitudinal changes in classroom motivation. J. Educ. Psychol. 106 (2), 527–540. doi:10.1037/a0034934
Ryan, R. M., and Deci, E. L. (2000). Self-determination theory and the facilitation of intrinsic motivation, social development, and well-being. Am. Psychol. 55 (1), 68–78. doi:10.1037/0003-066X.55.1.68
Saeed, S., and Zyngier, D. (2012). How motivation influences student engagement: a qualitative case study. J. Educ. Learn. 1 (2), 252–267. doi:10.5539/jel.v1n2p252
Sakhaei, H., Yeganeh, M., and Afhami, R. (2020). Quantifying stimulus-affected cinematic spaces using psychophysiological assessments to indicate enhanced cognition and sustainable design criteria. Front. Environ. Sci. 10, 832537S. doi:10.3389/fenvs.2022.832537
Salahshori, A., Eslami, K., Boostani, H., Zahiri, M., Jahani, S., Arjmand, R., et al. (2022). The university students’ viewpoints on e-learning system during COVID-19 pandemic: the case of Iran. Heliyon 8, e08984. doi:10.1016/j.heliyon.2022.e08984
Salama, A. (2006). Learning from the environment: evaluation research and experience based architectural pedagogy. CEBE Trans. 3, 64–83. doi:10.11120/tran.2006.03010064
Sarstedt, M., Hair, J., Cheah, H., Becker, J. M., and Ringle, C. M. (2019). How to specify, estimate, and validate higher-order constructs in PLS-SEM. Australas. Mark. J. (AMJ). 27, 197–211. doi:10.1016/j.ausmj.2019.05.003
Scherer, R., Nilsen, T., and Jansen, M. (2016). Evaluating individual students' perceptions of instructional quality: an investigation of their factor structure, measurement invariance, and relations to educational outcomes. Front. Psychol. 7, 110. doi:10.3389/fpsyg.2016.00110
Selvaraj, A., Vishnu, R., Ka, N., Benson, N., and Mathew, A. J. (2021). Effect of pandemic based online education on teaching and learning system. Int. J. Educ. Dev. 85, 102444. doi:10.1016/j.ijedudev.2021.102444
She, L., Ma, L., Jan, A., Sharif Nia, H., and Rahmatpour, P. (2021). Online learning satisfaction during COVID-19 pandemic among Chinese university students: the serial mediation model. Front. Psychol. 12, 743936. doi:10.3389/fpsyg.2021.743936
Sheldon, D., Bharwani, S., Mitchell, W., and Williams, J. (1995). Requirements for virtual design review. Archit. Res. Q. 1 (2), 80–89. doi:10.1017/s1359135500002785
Shi, Y., Tong, M., and Long, T. (2021). Investigating relationships among blended synchronous learning environments, students’ motivation, and cognitive engagement: a mixed methods study. Comput. and Educ. 168, 104193. doi:10.1016/j.compedu.2021.104193
Singh, M., James, P. S., Paul, H., and Bolar, K. (2022). Impact of cognitive-behavioral motivation on student engagement. Heliyon 8, e09843. doi:10.1016/j.heliyon.2022.e09843
Smith, K. A., Sheppard, S. D., Johnson, D. W., and Johnson, R. T. (2005). Pedagogies of engagement: classroom-based practices. J. Eng. Educ. 94, 87–101. doi:10.1002/j.2168-9830.2005.tb00831.x
Sökmen, Y. (2019). The role of self-efficacy in the relationship between the learning environment and student engagement. Educ. Stud. 47, 19–37. doi:10.1080/03055698.2019.1665986
Tucker, R., and Rollo, A. (2006). Teaching and learning in collaborative group design projects. Archit. Eng. Des. Manag. 2, 19–30. doi:10.1080/17452007.2006.9684602
Vallerand, R. J., Fortier, M. S., and Guay, F. (1997). Self-determination and persistence in a real-life setting: toward a motivational model of high school dropout. J. Personality Soc. Psychol. 72 (5), 1161–1176. doi:10.1037/0022-3514.72.5.1161
Vayre, E., and Vonthron, A. M. (2016). Psychological engagement of students in distance and online learning: effects of self-efficacy and psychosocial processes. J. Educ. Comput. Res. 55, 197–218. doi:10.1177/0735633116656849
Walker, K. A., and Koralesky, K. E. (2021). Student and instructor perceptions of engagement after the rapid online transition of teaching due to COVID-19. Nat. Sci. Educ. 50, e20038. doi:10.1002/nse2.20038
Wang, J. C., Morin, A. J., Ryan, R. M., and Liu, W. C. (2016). Students’ motivational profiles in the physical education context. J. Sport Exerc. Psychol. 38 (6), 612–630. doi:10.1123/jsep.2016-0153
Wang, M. T., and Degol, J. (2014). Staying engaged: knowledge and research needs in student engagement. Child. Dev. Perspect. 8 (3), 137–143. doi:10.1111/cdep.12073
Wang, M. T., and Eccles, J. S. (2013). School context, achievement motivation, and academic engagement: a longitudinal study of school engagement using a multidimensional perspective. Learn. Instr. 28, 12–23. doi:10.1016/j.learninstruc.2013.04.002
Wang, M. T., and Holcombe, R. (2010). Adolescents’ perceptions of school environment, engagement, and academic achievement in middle school. Am. Educ. Res. J. 47 (3), 633–662. doi:10.3102/0002831209361209
Wang, T. (2010). A new paradigm for design studio education. Int. J. Art and Des. Educ. 29, 173–183. doi:10.1111/j.1476-8070.2010.01647.x
Webb, N. M., Franke, M. L., Ing, M., Wong, J., Fernandez, C. H., Shin, N., et al. (2014). Engaging with others’ mathematical ideas: interrelationships among student participation, teachers’ instructional practices, and learning. Int. J. Educ. Res. 63, 79–93. doi:10.1016/j.ijer.2013.02.001
Wubbels, T., Brekelmans, M., Den Brok, P. J., and Tartwijk, J. (2006). “An interpersonal perspective on classroom management in secondary classrooms in The Netherlands,” in Handbook of classroom management: research, practice, and contemporary issues. Editors C. M. Evertson, and C. S. Weinstein (Lawrence Erlbaum Associates Publishers), 1161–1191.
Xiang, Y., Song, D., and He, R. (2018). Re-examining ‘learning by doing’: implications from learning style migration. Des. J. 21, 313–330. doi:10.1080/14606925.2018.1444126
Xu, B., Chen, N. S., and Chen, G. (2020). Effects of teacher role on student engagement in WeChat-Based online discussion learning. Comput. and Educ. 157, 103956. doi:10.1016/j.compedu.2020.103956
Yasmin, M. (2022). Online chemical engineering education during COVID-19 pandemic: lessons learned from Pakistan. Educ. Chem. Eng. 39, 19–30. doi:10.1016/j.ece.2022.02.002
Yeganeh, M. (2022). Conceptual and theoretical model of integrity between buildings and city. Sustain. Cities Soc. 59, 102205. doi:10.1016/j.scs.2020.102205
Yeganeh, M., and Kamalizadeh, M. (2018). Territorial behaviors and integration between buildings and city in urban public spaces of Iran׳s metropolises. Front. Archit. Res. 7 (4), 588–599. doi:10.1016/j.foar.2018.06.004
Young, M., Klemz, B., and Murphy, J. (2003). Enhancing learning outcomes: the effects of instructional technology, learning styles, instructional methods, and student behavior. J. Mark. Educ. 25, 130–142. doi:10.1177/0273475303254004
Zare, Z., Yganeh, M., and Dehghan, N. (2022). Environmental and social sustainability automated evaluation of plazas based on 3D visibility measurements. Energy Rep. 8, 6280–6300. doi:10.1016/j.egyr.2022.04.064
Keywords: instructional strategies, student engagement, learning motivation, online design studios, architectural design
Citation: Baigi F, Yeganeh M and Bemanian M (2024) Internet-based infrastructures and online architectural education on design studios: perceived instructional strategies and students engagement. Front. Built Environ. 10:1463658. doi: 10.3389/fbuil.2024.1463658
Received: 12 July 2024; Accepted: 20 August 2024;
Published: 02 September 2024.
Edited by:
Amirhossein Balali, The University of Manchester, United KingdomReviewed by:
David Blanco, Metropolitan University of Technology, ChileCopyright © 2024 Baigi, Yeganeh and Bemanian. This is an open-access article distributed under the terms of the Creative Commons Attribution License (CC BY). The use, distribution or reproduction in other forums is permitted, provided the original author(s) and the copyright owner(s) are credited and that the original publication in this journal is cited, in accordance with accepted academic practice. No use, distribution or reproduction is permitted which does not comply with these terms.
*Correspondence: Mansour Yeganeh, eWVnYW5laEBtb2RhcmVzLmFjLmly
Disclaimer: All claims expressed in this article are solely those of the authors and do not necessarily represent those of their affiliated organizations, or those of the publisher, the editors and the reviewers. Any product that may be evaluated in this article or claim that may be made by its manufacturer is not guaranteed or endorsed by the publisher.
Research integrity at Frontiers
Learn more about the work of our research integrity team to safeguard the quality of each article we publish.