- 1Escuela Superior Politécnica de Chimborazo (ESPOCH), Sede Orellana, Riobamba, Ecuador
- 2Facultad de Ingeniería, Arquitectura, Universidad Nacional de Chimborazo (UNACH), Riobamba, Ecuador
- 3Department of Civil Engineering, Michael Okpara University of Agriculture, Umudike, Nigeria
- 4Department of Civil Engineering, Kampala International University, Kampala, Uganda
- 5Facultad de Ingenieria, Ingenieria Civil, Universidad Nacional de Chimborazo (UNACH), Riobamba, Ecuador
- 6Department of Civil Engineering, Aditya College of Engineering and Technology, Surampalem, Andhra Pradesh, India
- 7Program in Architecture, Heritage and the City, Universitat Politecnica de Valencia, Valencia, Spain
- 8Facultad de Informatica y Electronica, Escuela Superior Politecnica de Chimborazo (ESPOCH), Riobamba, Ecuador
- 9Facultad de Ciencias, Escuela Superior Politécnica de Chimborazo (ESPOCH), Riobamba, Ecuador
Industrial wastes have found great use in the built environment due to the role they play in the sustainable infrastructure development especially in green concrete production. In this research investigation, the impact of wastes from the industry on the compressive strength of concrete incorporating fly ash (FA) and silica fume (SF) as additional components alongside traditional concrete mixes has been studied through the application of machine learning (ML). A green concrete database comprising 330 concrete mix data points has been collected and modelled to estimate the unconfined compressive strength behaviour. Considering the concerning environmental ramifications associated with concrete production and its utilization in construction activities, there is a pressing need to perform predictive model exercise. Furthermore, given the prevalent reliance of concrete production professionals on laboratory experiments, it is imperative to propose smart equations aimed at diminishing this dependency. These equations should be applicable for use in the design, construction, and performance assessment of concrete infrastructure, thereby reflecting the multi-objective nature of this research endeavour. It has been proposed by previous research works that the addition of FA and SF in concrete has a reduction impact on the environmental influence indicators due to reduced cement use. The artificial neural network (ANN) and the M5P models were applied in this exercise to predict the compressive strength of FA- and SF-mixed concrete also considering the impact of water reducing agent in the concrete. A sensitivity analysis was also conducted to determine the impact of the concrete components on the strength of the concrete. At the end, closed-form equations were proposed by the ANN and M5P with performance indices which outperformed previous models conducted on the same database size. The result of the sensitivity analysis showed that FA is most impactful of all the studied components thereby emphasizing the importance of adding industrial wastes in concrete production for improved mechanical properties and reduced carbon footprint in the concrete construction activities. Also, the M5P and ANN models with R2 of 0.99 showed a potential for use as decisive models to predict the compressive strength of FA- and SF-mixed concrete.
1 Introduction
The compressive strength of concrete is one of its most important properties, indicating its ability to withstand axial loads or pressure (Soleymani and Esfahani, 2019). It is typically measured by subjecting cylindrical or cubic specimens of concrete to a compressive force until failure occurs (Jahangir and Eidgahee, 2021). Compressive strength is the maximum compressive stress that concrete can withstand before failure occurs (Soleymani and Esfahani, 2019). It is usually expressed in megapascals (MPa) or pounds per square inch (psi). Compressive strength is crucial for assessing the structural performance and durability of concrete in various applications, including buildings, bridges, dams, pavements, and other infrastructure projects (Salesa et al., 2017). It is a fundamental parameter used in structural design and quality control of concrete construction (Samad and Shah, 2017). Generally, lower water-cement ratios lead to higher compressive strength because they result in better cement hydration and a denser concrete matrix (Ma et al., 2016). Different types of cement, such as Portland cement, blended cements, or specialty cements, can have varying effects on compressive strength due to differences in chemical composition and fineness (Liew et al., 2017). Aggregate characteristics, including size, shape, gradation, and strength, influence concrete strength (Ting et al., 2021). High-quality aggregates with strong interlocking particles typically contribute to higher compressive strength (Güneyisi et al., 2012). Certain chemical admixtures, such as water reducers, superplasticizers, air-entraining agents, and pozzolanic materials, can affect compressive strength by modifying the properties of fresh and hardened concrete (Guo et al., 2020). Proper curing of concrete is essential for achieving optimal strength development. Factors such as temperature, humidity, duration, and curing methods can significantly impact compressive strength (Sahoo et al., 2021). The proportioning of cement, aggregates, water, and admixtures in the concrete mix, as well as the use of supplementary cementitious materials, influence compressive strength (L et al., 2021). A well-designed mix with proper proportions is essential for achieving desired strength levels. Compressive strength testing of concrete is typically performed on standard specimens (cylinders or cubes) using a compression testing machine (Yang et al., 2019). The specimens are subjected to a gradually applied compressive load until failure occurs, and the maximum load at failure is recorded (Çelik et al., 2022). Compressive strength testing is an essential component of quality control in concrete production (Liu et al., 2017). It helps ensure that concrete meets specified strength requirements for the intended application and provides feedback for adjusting mix designs and production processes as needed (Hossain et al., 2016). The compressive strength of concrete is a fundamental property that depends on various factors related to mix design, materials, curing conditions, and testing procedures (Dong et al., 2015). Understanding and controlling these factors are essential for achieving durable and structurally sound concrete in construction projects (Alaloul et al., 2021). The impact of industrial wastes on the compressive strength of concrete can vary depending on several factors, including the type and characteristics of the waste material, its dosage in the concrete mix, and the curing conditions (Kumar KR. et al., 2021). Different industrial wastes have varying effects on concrete strength. Some industrial by-products, such as fly ash, silica fume, and slag, are commonly used as supplementary cementitious materials (SCMs) and can enhance the compressive strength of concrete when properly utilized (de Matos et al., 2020). Others, like certain types of waste aggregates or sludges, may have a detrimental effect on strength if used inappropriately (Siamardi, 2022). The chemical composition of industrial wastes can influence their interaction with cementitious materials and hydration products in concrete (Bhuva and Bhogayata, 2022). Some industrial by-products contain reactive components that can contribute to the formation of additional cementitious phases, leading to improved strength and durability of concrete (Sun et al., 2022). However, certain contaminants or undesirable constituents in industrial wastes may adversely affect the hydration process and weaken the concrete matrix (Rezazadeh Eidgahee et al., 2022). The proportion of industrial waste incorporated into the concrete mix, as well as the extent to which it replaces conventional materials like cement or aggregates, can significantly impact compressive strength (Chen et al., 2022). Optimal dosage and replacement levels need to be carefully determined through experimental testing to achieve the desired balance between strength enhancement and potential drawbacks (AlShareedah and Nassiri, 2021). The particle size distribution of industrial waste materials can influence their pozzolanic or hydraulic activity and their ability to fill voids in the concrete matrix (Wang A. et al., 2022; Warda et al., 2022; Solouki et al., 2022; Khan and Ali, 2019). Finer particles typically contribute more effectively to strength development by providing nucleation sites for hydration products and improving packing density within the concrete (Xing et al., 2022). Proper curing of concrete is essential to ensure adequate hydration and development of strength (ISO 14040:2006, 2016). The presence of industrial wastes may alter the curing requirements of concrete due to their effects on hydration kinetics and moisture retention (SO 14044:2006, 2016). Adjustments to curing procedures may be necessary to optimize the performance of concrete containing industrial wastes (Kumar M. et al., 2021). In addition to compressive strength, the long-term durability and performance of concrete incorporating industrial wastes should also be considered (Che et al., 2020). Some industrial by-products may impart beneficial properties such as increased resistance to chloride ingress, sulfate attack, or alkali-silica reaction, which can enhance the overall durability of concrete structures over time (Tayeh et al., 2020). The impact of industrial wastes on the compressive strength of concrete is multifaceted and depends on various factors (Sandanayake et al., 2020). Proper selection, characterization, and incorporation of industrial waste materials into concrete mixes, along with careful consideration of mix design parameters and curing practices, are essential to maximize the benefits while minimizing potential drawbacks (Bhanja and Sengupta, 2005). The addition of silica fume and fly ash in concrete can have significant effects on its compressive strength (Nochaiya et al., 2010). Silica fume, also known as microsilica, is a by-product of the silicon and ferrosilicon alloy production process (Mazloom et al., 2004). It consists of very fine particles, usually with an average diameter of less than 1 micron (Seto et al., 2017). When added to concrete, silica fume acts as a pozzolan, reacting with calcium hydroxide (a by-product of cement hydration) to form additional calcium silicate hydrate (C-S-H) gel (Chen et al., 2019). This results in a denser and more refined microstructure of the concrete paste (Khan and Siddique, 2011). The densification of the concrete matrix due to silica fume incorporation leads to improved packing of particles and reduced porosity, resulting in increased compressive strength (Ganesh Kumar et al., 2022). Additionally, the formation of additional C-S-H gel contributes to the strength enhancement (Ashraf et al., 2022). Silica fume also enhances the bond between cement particles and aggregates, which further contributes to the overall strength of the concrete (Thomas et al., 1999). However, excessive amounts of silica fume can lead to a rapid stiffening of the concrete mix, making it challenging to handle and place (Nežerka et al., 2019). Proper mix design and dosage control are essential to harness the beneficial effects of silica fume on compressive strength without compromising workability (Wang et al., 2021a). Fly ash is a by-product of coal combustion in power plants and is composed of fine particles that are similar in size to cement particles (Ghalehnovi et al., 2022). In concrete, fly ash acts as a pozzolan, reacting with calcium hydroxide to form additional C-S-H gel and calcium aluminosilicate hydrate (C-A-S-H) gel (The International Standards Organisation, 2006). This secondary hydration reaction contributes to strength development (Onyelowe and Ebid, 2023). Additionally, fly ash particles can fill voids and improve particle packing within the concrete matrix, resulting in densification and reduced permeability (Onyelowe et al., 2023a). The long-term pozzolanic reaction of fly ash can lead to continued strength gain over time, making concrete more durable (Onyelowe et al., 2023b; Onyelowe et al., 2023c; Onyelowe et al., 2023d; Onyelowe K. C. et al., 2023; Wernet et al., 2016; Huijbregts et al., 2017; Garcia-Troncoso et al., 2022; Dandautiya and Singh, 2019; Teixeira et al., 2016). The effect of fly ash on compressive strength depends on factors such as its chemical composition, fineness, and dosage (Onyelowe et al., 2023c; Onyelowe et al., 2023d; Onyelowe K. C. et al., 2023; Wernet et al., 2016; Huijbregts et al., 2017; Garcia-Troncoso et al., 2022; Dandautiya and Singh, 2019; Teixeira et al., 2016; Panesar et al., 2019; Onyelowe et al., 2022a; Onyelowe et al., 2022b; Onyelowe et al., 2021; Dutta et al., 2018; QUINLAN, 1992; Asteris et al., 2024; Asteris P. G. et al., 2021; Asteris PanagiotisG. et al., 2021; Asteris et al., 2022; Ali et al., 2024; Cavaleri et al., 2022; Armaghani et al., 2021; Zhang et al., 2022; Ali et al., 2023; Faisal Alkayem et al., 2024). In general, moderate levels of fly ash incorporation (typically up to 30% replacement of cement by mass) can lead to significant improvements in compressive strength (Wang L. et al., 2022; Wang et al., 2021b; Wang et al., 2021c; Wang et al., 2020). However, excessive fly ash content may result in slower early-age strength development and may require longer curing periods to achieve desired strength levels (Onyelowe et al., 2023d). Meanwhile the addition of silica fume and fly ash in concrete as illustrated in Figure 1 can enhance compressive strength by promoting densification of the concrete matrix, additional hydration reactions, and improved particle packing (Onyelowe K. C. et al., 2023; Wernet et al., 2016; Huijbregts et al., 2017; Garcia-Troncoso et al., 2022; Dandautiya and Singh, 2019). Proper mix design, dosage control, and curing practices are essential to optimize the beneficial effects of these supplementary cementitious materials on concrete strength (Teixeira et al., 2016; Panesar et al., 2019; Onyelowe et al., 2022a; Onyelowe et al., 2022b; Onyelowe et al., 2021; Dutta et al., 2018; QUINLAN, 1992).
Conversely, the influence of a high-rate water reducing agent (HRWRA) on the compressive strength of concrete containing silica fume and fly ash can vary depending on several factors, including the type and dosage of HRWRA, the characteristics of silica fume and fly ash, and the mix design parameters (Onyelowe et al., 2023d; Onyelowe K. C. et al., 2023; Wernet et al., 2016; Huijbregts et al., 2017). HRWRA is typically added to concrete mixes to improve workability while reducing the water content (AlShareedah and Nassiri, 2021; Teixeira et al., 2016). By dispersing cement particles and reducing interparticle friction, HRWRA allows for better particle packing and improved lubrication, leading to increased workability and easier placement of concrete without the need for additional water 4–8]. This can contribute to better compaction and consolidation of the concrete, potentially enhancing compressive strength (Soleymani and Esfahani, 2019; Jahangir and Eidgahee, 2021; Salesa et al., 2017; Samad and Shah, 2017; Ma et al., 2016). HRWRA can also affect the hydration and pozzolanic reactions of supplementary cementitious materials (SCMs) such as silica fume and fly ash (Sahoo et al., 2021; L et al., 2021; Yang et al., 2019; Çelik et al., 2022; Liu et al., 2017; Hossain et al., 2016). The dispersing action of HRWRA may enhance the distribution of these materials within the concrete matrix, facilitating their interaction with cement hydration products and leading to more complete hydration and pozzolanic reactions (Panesar et al., 2019). This can result in denser microstructures and improved strength properties. The addition of HRWRA may necessitate adjustments to the mix proportions of silica fume and fly ash mixed concrete to maintain desired workability and strength characteristics (Nochaiya et al., 2010; Mazloom et al., 2004; Seto et al., 2017; Chen et al., 2019; Khan and Siddique, 2011; Ganesh Kumar et al., 2022; Ashraf et al., 2022; Thomas et al., 1999). Proper dosage control and optimization of mix designs are essential to achieve the desired balance between workability, strength, and durability (Teixeira et al., 2016). HRWRA can promote early-age strength development by accelerating the hydration reactions of cementitious materials (Onyelowe et al., 2022a). This may lead to higher early compressive strength in silica fume and fly ash mixed concrete, which can be advantageous for reducing formwork time and accelerating construction schedules (Jahangir and Eidgahee, 2021). While HRWRA may enhance early-age strength, its long-term effects on the compressive strength and durability of silica fume and fly ash mixed concrete should be carefully evaluated (Dandautiya and Singh, 2019). Excessive use of HRWRA or improper mix designs may lead to decreased long-term strength and durability due to factors such as increased shrinkage, reduced pore refinement, and potential loss of bond between cementitious materials and aggregates (Güneyisi et al., 2012). Finally, the influence of HRWRA on the compressive strength of concrete containing silica fume and fly ash is complex and depends on various factors. Proper mix design, dosage control, and consideration of both short-term and long-term performance are essential to optimize the benefits of HRWRA while ensuring the desired strength and durability of the concrete (Panesar et al., 2019). More very positive research results have been reported presently on the different advantages of the applying machine learning in solving most civil engineering problems especially those bothering on infrastructure sustainability (Asteris et al., 2024; Asteris P. G. et al., 2021; Asteris PanagiotisG. et al., 2021; Asteris et al., 2022; Ali et al., 2024; Cavaleri et al., 2022; Armaghani et al., 2021; Zhang et al., 2022; Ali et al., 2023; Faisal Alkayem et al., 2024). Among these reports included the research on the application of the newly introduced a20 index in the performance evaluation of machine learning models (Asteris et al., 2024; Asteris P. G. et al., 2021), which has attracted attention as it supports in great extent the previously used indices. Also, previous reports (Wang L. et al., 2022; Wang et al., 2021b; Wang et al., 2021c; Wang et al., 2020), have presented different machine learning models utilized in studying the behaviour of concrete mixed with sustainable admixtures such as fly ash, Pva fiber, phosphorus slag, etc., Producing results that are decisive. However, in this research paper, machine learning models have been reported on the effect of industrial wastes on the compressive strength of hardened concrete under different curing regimes.
2 Research significance
Evaluating the impact of industrial wastes on the compressive strength of concrete using closed-form machine learning algorithms is a research topic with significant implications in both environmental sustainability and civil engineering. Industrial waste disposal is a significant environmental concern. By incorporating these wastes into concrete, you can reduce landfill usage and pollution, contributing to a circular economy. Using industrial wastes as a substitute for traditional concrete components (like cement and aggregates) can reduce the demand for natural resources such as limestone and sand. Cement production is a major source of carbon dioxide emissions. Replacing part of the cement content with industrial by-products like fly ash, slag, or silica fume can lower the overall carbon footprint of concrete production. Understanding how different types of industrial wastes affect the compressive strength of concrete is crucial. It allows engineers to optimize mix designs to achieve the desired strength while incorporating sustainable materials. Closed-form machine learning algorithms can create robust models that predict the compressive strength of concrete based on various input parameters, including the type and amount of industrial waste used. This predictive capability is valuable in both research and practical applications. Cost Reduction: Incorporating industrial waste can reduce the cost of concrete production, particularly if the waste is readily available and would otherwise be expensive to dispose of. Companies that successfully integrate waste materials into high-performance concrete could gain a competitive advantage by offering more sustainable and potentially lower-cost products. The research can lead to new concrete formulations that have enhanced properties or are suitable for specialized applications. It can also provide insights into the long-term durability and environmental performance of such materials. Closed-form machine learning algorithms can analyze large datasets to identify patterns and relationships that might not be apparent through traditional statistical methods. This can lead to new discoveries in material science and civil engineering. The research can contribute to the development of standards and guidelines for using industrial wastes in concrete, helping to ensure safety and performance across the industry. Promoting the use of industrial wastes in concrete can raise awareness about sustainable construction practices among engineers, architects, and builders. Research in this area supports broader societal goals of sustainability and responsible resource management, aligning with global efforts to mitigate environmental degradation and climate change. If the research demonstrates that industrial wastes can be safely and effectively used in concrete, it may influence regulatory bodies to update building codes and material standards, paving the way for wider adoption. This research topic is not only relevant for advancing the field of concrete technology but also for addressing pressing environmental issues. By integrating machine learning with material science, the study could lead to practical, sustainable solutions for the construction industry while contributing to academic knowledge and policy development.
Conversely, using industrial wastes in concrete has significant environmental implications, both positive and negative. The practice is gaining attention due to the potential benefits in sustainability and waste management, but it also presents certain challenges. Incorporating industrial wastes like fly ash, blast furnace slag, silica fume, or recycled aggregates into concrete helps divert these materials from landfills, reducing the burden on waste management systems. By reusing waste materials, the demand for new landfill sites is reduced, preserving natural landscapes and decreasing the environmental footprint of waste disposal. Industrial by-products like fly ash or slag can partially replace Portland cement in concrete. Since cement production is highly energy-intensive and a major source of CO₂ emissions, reducing its usage directly lowers the carbon footprint of concrete. Energy Savings: The energy required to process and use industrial wastes is often lower than that needed for producing new raw materials, further reducing the environmental impact. Using industrial wastes reduces the need for virgin raw materials like limestone, sand, and gravel, thereby conserving these natural resources and reducing the environmental degradation associated with their extraction. Incorporating waste materials aligns with the principles of sustainable construction by promoting the use of recycled content and reducing the environmental impacts of new construction projects. Certain industrial wastes can enhance the properties of concrete, such as its durability, strength, and resistance to chemical attacks. For example, fly ash can improve the workability and long-term strength of concrete, potentially leading to longer-lasting structures that require less maintenance and fewer resources over time. By reusing industrial wastes, the potential for pollution from these materials is mitigated. For instance, fly ash or slag, if not used in concrete, may contribute to air and water pollution through leaching or airborne particles. The practice supports the concept of a circular economy, where waste materials are continuously reused and recycled, minimizing environmental impacts and maximizing resource efficiency. Some industrial wastes, such as fly ash from coal-fired power plants, may contain heavy metals or other toxic substances. If not properly managed, there is a risk of leaching, which could contaminate groundwater and soil. Fine particulate matter from industrial wastes, especially during the mixing and curing of concrete, can contribute to air pollution and pose health risks to workers and nearby communities. The long-term performance of concrete containing certain industrial wastes is not always well understood, particularly under varying environmental conditions. If these materials lead to premature degradation of concrete, this could result in increased maintenance and repair activities, offsetting initial environmental benefits. The quality and composition of industrial wastes can vary, leading to inconsistencies in the performance of the concrete. This variability can pose challenges in ensuring the reliability and durability of the structures, which may have environmental and safety implications. Some industrial wastes require significant processing before they can be used in concrete. For example, the grinding of slag or the treatment of fly ash can be energy-intensive, potentially reducing the overall environmental benefits. If industrial wastes need to be transported over long distances to be used in concrete production, the associated emissions from transportation could diminish the environmental advantages. The use of industrial wastes in concrete may face regulatory hurdles, particularly if there is concern about environmental contamination or health risks. Navigating these regulations can be complex and may require additional testing and certification, adding to the environmental and economic costs. There may be resistance from stakeholders or the public to the use of industrial wastes in construction due to concerns about safety, performance, and environmental impact. Overcoming these perceptions requires education and demonstration of the benefits and safety of such practices. Conclusively, the environmental implications of using industrial wastes in concrete are largely positive, contributing to waste reduction, resource conservation, and a lower carbon footprint. However, careful management and thorough assessment of potential risks are essential to maximize the benefits and mitigate any negative impacts. When appropriately handled, the use of industrial wastes in concrete supports the move towards more sustainable and environmentally responsible construction practices.
3 Methodology
3.1 Data collection and preliminary analysis
A total of 331 data sets collected from the experiments were analyzed and divided into three and two groups for ANN and M5P model respectively. The larger group included 225 data used to create models, while the other groups included 73 and 33 data sets used to test and forecasting the models respectively for ANN model. However, for M5P model the total data is segregated into two parts: 70% for training and remaining 30% for testing. The input data set consists of the cement (C), Fine Aggregate (FAg), Coarse Aggregate (CAg), Water (W), Fly Ash (FA), Silica Fume (SF), High-rate water reducing agent (HRWRA), and Curing time (D); compressive strength (fs) of concrete was used as a target. Table 1 shows the statistical characteristics of the data entries with respect to the studied parameters and Table 2 presents the Pearson correlation analysis. The total data was shown in Figure 2 as in the form of histogram.
4 Machine learning models
4.1 Artificial neural networks (ANNs) theory
An ANN functions as a computational system that emulates the processes and analyses undertaken by the human brain. Specifically applied in construction materials (Onyelowe et al., 2022a; Onyelowe et al., 2022b), this machine learning model facilitates various numerical predictions and problem-solving tasks. The architecture of an artificial neural network comprises an input layer, one or more hidden layers, and an output layer. The hidden layer establishes connections through weight, transfer function, and bias to the final output layers. To develop a multilayer feed-forward network for Constructing Engineering, inputs such as proportions, cement (C), Fine Aggregate (Fag), Coarse Aggregate (Cag), Water (W), Fly Ash (FA), Silica Fume (SF), High-rate water reducing agent (HRWRA), and Curing time (D) with maximum compressive strength as the output. The absence of a standardized method for designing network architecture led to a thumb-rule approach, to fix the number of hidden layer neurons (Onyelowe et al., 2021). The next step in optimal network design involved selecting the ideal number of epochs during training, aiming for the minimum Mean Square Error (MSE), and a high R2-value as shown in Figure 3.
From Figure 3, the epochs are fixed as a 1,000. While calculating the MSE and R2 for each increment of epochs default sigmoid activation function was used. Upon designing the optimal architecture, the dataset (comprising a total of 331 data points) was split into training (68% of the data), testing (22% of the data) and forecasting (10% of the data) sets. Multiple transfer functions and ANN structures, featuring different hidden layers and neurons, were assessed to determine the most effective network structure for predicting concrete compression strength (fs). Among the options, a single hidden layer with five neurons was used to for the architecture of ANN model as followed by thumb rule (Dutta et al., 2018). 18 activation function were used with the above proposed ANN architecture such as linear piece, linear piece symmetric, sin symmetric, cos symmetric, sin, linear, threshold, threshold symmetric, sigmoid, sigmoid stepwise, sigmoid symmetric, sigmoid symmetric stepwise, gaussian, gaussian symmetric, gaussian stepwise, Elliot, Elliot symmetric, and cos transfer function emerged as the choice for concrete modified with Silica Fume, and High-rate water reducing agent. This segment of the research focused on utilizing the ANN model to estimate the maximum compressive strength of Silica Fume-containing concrete, considering factors such as C, Fag, Cag, W, FA, SF, HRWRA and D contents following the above said architecture. The architecture of ANNs model is depicted in Figure 4.
4.2 M5P-tree model (M5P) theory
The M5P-tree is a type of algorithm used for solving problems related to predicting numerical values, known as regression problems. It was first introduced in a study (QUINLAN, 1992). This algorithm works like a tree, where each branch represents a different part of the data. In simple terms, the M5P-tree uses a method called linear regression at the end points of its branches. It adapts to different parts of the data by sorting or dividing it into various groups. The M5P model architecture has depicted schematically in Figure 5. The M5P model begins with separating the input data into distinct subsets; each subset has data records with a sharing feature (Figure 5). To overcome the difference within the defined sub-set, linear regression models (LRM) might be employed in this procedure. The information gathered in the preceding stage is then utilized to generate numerous nodes, each of which is segregated accord with the certain attribute (Figure 6A).
This phase enables you to construct a structure as like tree with the roots and leaves presented as top and bottom respectively. New datasets are added to the tree, it progresses from the roots through the nodes until they reach to each leaf (Figure 6B). To estimate errors, the algorithm considers the criteria for dividing the tree at each point. The errors are measured by looking at how much the values in each group differ from the average value. The algorithm decides how to divide the tree based on the attribute that is most likely to reduce the error. This is done by evaluating the impact of each attribute at a specific point in the tree. The error calculation involves looking at the standard deviation, which measures how spread out the values is in a group. As the tree grows, the child nodes (smaller groups) have less variation in values compared to the parent nodes (larger groups). After exploring different ways to structure the tree, the algorithm selects the one that is most likely to reduce errors. However, this process can lead to overfitting, where the model is too closely tailored to the training data. To address this issue, the algorithm goes through a second step called pruning. This involves trimming the branches of the tree and replacing them with simpler linear regression functions. This helps prevent the model from becoming too complex and specialized for the training data. In summary, the M5P-tree algorithm uses linear regression at its branches to predict numerical values. It decides how to structure the tree based on the attributes that are likely to reduce errors. To avoid overfitting, it prunes the tree and replaces some branches with simpler functions. Standard reduction:
In this study, T stands for the group of examples that reach a certain point in the process. Ti refers to the specific examples that fall under the ith product of the potential set, and SD stands for standard deviation. The multi-layer models in this research were implemented using the Weka software. Weka is a free and widely-used open-source platform designed for engineers and scientists. It helps them analyze and create systems and products that have a significant impact on our world.
4.3 Performance measures
Once the model is identified, its performance in predicting compression strength of concrete needs evaluation using a test dataset. Researchers often lack consensus on the best measure for prediction, so accuracy becomes a crucial criterion. To assess quality, we consider minimizing errors, and in this case, mean absolute percentage error (MAPE), root mean square error (RMSE), mean absolute error (MAE), and mean square error (MSE) are chosen. MAE gives an overall accuracy, indicating the degree of spread. It assigns equal weight to all errors, being zero for a good fit and larger for a poor one. Comparisons between prediction methods are made, and the one with the minimum MAE is selected. MSE, providing accuracy measures and indicating spread, assigns additional weight to large errors. RMSE, simply the root of MSE, is measured in the same unit as the predicted variable, offering advantages. MAPE is a relative performance measure, useful for comparing prediction accuracy among different methods. Interpretations include excellent accuracy (less than 10%), good prediction (10%–20%), acceptable prediction (20%–50%), and inaccurate prediction (over 50%). MAPE measures prediction quality independently of the variable’s unit of measurement. Smaller values for MSE, RMSE, MAE, and MAPE indicate better models. Although historically popular, RMSE and MSE are sensitive to outliers, leading some researchers to recommend against their use in predictive accuracy evaluation. MAE, considered a ‘robust’ measure of predictive accuracy, tends to favour procedures with occasional large prediction failures. Estimation procedures often rely on least-squares criteria, and an emphasis on MAE may involve a logical inconsistency. Model selection depends on different criteria, and the choice of an error measure significantly impacts conclusions about predictive methods’ accuracy. When evaluating neural network models, relying solely on coefficients of correlation (r) and determination (R2) can introduce bias. Thus, unbiased statistical criteria such as MSE, RMSE, MAE, and MAPE should complement r and R2 evaluations. Different statistical parameters and error models used for predicting compression strength of concrete are presented in Table 3.
5 Results, discussions and comparison
Overall, as presented in Equations 1–10, 18 ANN models were developed by following the architecture with varying activation functions. Each model performance is validated with the help of performance measures as discussed in the preceding section. Where, the performance measures are calculated and the best five model values are tabulated in Table 4 for training, testing and forecasting. From Table 4, it can be concluded that the sigmoid activation function is predicting the compressive strength of the concrete more accurately as compared with the remaining activation functions. The predicted Vs. experiments plots for training, testing and forecasting are depicted in Figure 7. From Figure 7, it can be concluded that the predicted values are within the 20% of error. In practical terms, for predicting compression strength of concrete, a neural network model with a sigmoid activation function is recommended. This choice yields the least values for R2, MSE, RMSE, MAE, and MAPE, indicating accuracy and good correlation between predicted and targeted compressive strength of concrete. Finally, the number of data Vs. compressive strength of concrete for experimental, ANN-trained, ANN-tested and ANN-forecasted plot was drawn to see the variation among them as depicted in Figure 8. From Figure 8, the experimental and ANN model predicted values are closely matching each other as it revels the prediction accuracy in visual way.
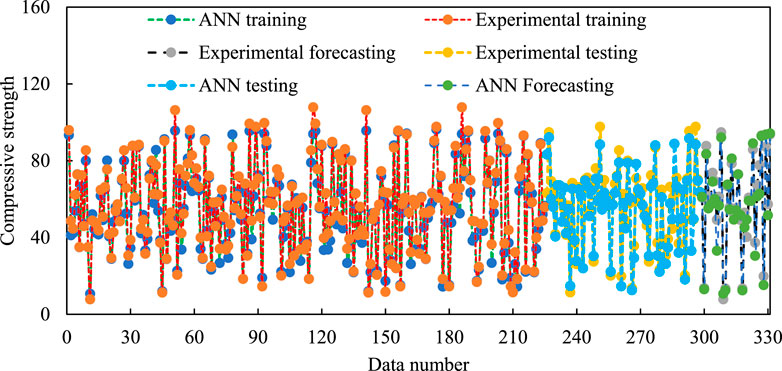
Figure 8. Comparison of training, testing and forecasting for experimental and forecasting based on ANN model.
Similarly, the M5P model was developed using defined user defined parameters. The developed model is validated with the help of performance measures and the calculated performance measures are tabulated in Table 5. From Table 5 yields the least values for MSE, RMSE, MAE, and MAPE and closed to one of r, R2 indicating accuracy and good correlation between predicted and targeted compressive strength of concrete. The predicted Vs. experiments plots for training, testing is depicted in Figure 9. From Figure 9, it can be concluded that the predicted values are within the 20% of error. Finally, the number of data Vs. compressive strength of concrete for experimental, M5P-trained, and M5P-tested plot was drawn to see the variation among them as depicted in Figure 10. From Figure 10, the experimental and ANN model predicted values are closely matching each other as it revels the prediction accuracy in visual way.
6 Closed-form models appraisals
6.1 Model equation for ANN
The fundamental mathematical equation of the ANN connecting the input variables and the output can be expressed as
As a result, the model equation for the output may be formed using the ANN model’s training weights. In this work, a model equation for compressive strength of concrete was created utilising the values of the weights and biases presented in Table 6.
The ANN model equations are developed using Table 5 as presented below:
The compressive strength value produced from Equation 9 is in the [-1, 1] range and must be denormalized as
6.2 Model equation for M5P
The training and testing technique is a most widely used technique to develop the learning algorithms to establish model. For training and testing the model, the train and test datasets are divided into two subsets using random partitioning. The model is trained using 70% of the data and then tested using the remaining 30% for evaluation of desired model. Table 1 provides the mini., max., and Stan. Dev. Values for the total data for each of the individual parameters used to create the M5P model.
The model tree that was created using the M5P technique is displayed in Equations 11–24. The generated equations for the present M5P model in the form of the linear model are shown in Figure 11. The equations have to be used following Figure 11 conditions as represented as M1 to M5.
Comparatively, the performance of an Artificial Neural Network (ANN) model and an M5P model (a type of model tree) involves analyzing several aspects, including prediction accuracy, interpretability, computational efficiency, and robustness, which formed part of the above results analyses (Asteris et al., 2024). ANNs can model complex, non-linear relationships between inputs and outputs, making them particularly powerful for problems where the relationship is not easily captured by simpler models. With the right architecture and training, ANNs often achieve high prediction accuracy (Rezazadeh Eidgahee et al., 2022). ANNs require a substantial amount of data to train effectively. If the dataset is small or imbalanced, the ANN might overfit or underperform compared to simpler models like M5P. However, k-fold classification and data sorting was applied to overcome the overfitting issue with the ANN. M5P models combine decision trees with linear regression at the leaves, enabling them to capture both non-linear and linear relationships effectively (Onyelowe et al., 2022a). They often perform well on moderately complex datasets and can be surprisingly accurate given their simplicity. M5P models might struggle with highly complex, non-linear relationships where the partitioning of the feature space by the decision tree might not be sufficient to capture the underlying patterns. ANNs are often considered “black-box” models due to their complexity (Onyelowe et al., 2022b). However, recent advancements in interpretability methods (like SHAP values, LIME, and saliency maps) have made it easier to interpret their predictions to some extent. Despite these methods, the internal workings of an ANN remain complex, and it can be challenging to understand how specific input features contribute to the final prediction (Onyelowe et al., 2021). M5P models are highly interpretable. The tree structure provides clear decision paths, and the linear regression models at the leaves offer straightforward relationships between input features and the output. This makes M5P models easier to understand and explain to non-experts. While interpretable, M5P models may oversimplify complex relationships, which could lead to less accurate predictions in certain cases. ANNs, especially deep neural networks, require significant computational resources for training, including powerful GPUs or TPUs. Once trained, they can make predictions relatively quickly (Dutta et al., 2018). The training process can be time-consuming and resource-intensive, especially if the network has many layers or if the dataset is large. Hyperparameter tuning (e.g., optimizing learning rates, number of layers, and neurons) also adds to the computational cost. M5P models are generally more computationally efficient to train and require less computational power compared to ANNs. They are faster to train, particularly on smaller datasets, and their structure allows for quick predictions. While efficient, M5P models might not scale as well as ANNs when dealing with very large or complex datasets, particularly when the underlying data relationships are highly non-linear. ANNs, when properly regularized and trained on large datasets, can generalize well to unseen data. Techniques like dropout, L2 regularization, and data augmentation can improve robustness. ANNs are prone to overfitting, particularly if the model is too complex relative to the amount of training data. They also require careful tuning of hyperparameters to achieve good generalization. M5P models are generally more robust to overfitting than ANNs, particularly when they are pruned correctly. Their inherent simplicity often leads to better generalization on smaller datasets. While robust, M5P models may lack the flexibility needed to capture more complex patterns, leading to poorer generalization on highly complex tasks. Problems where there are complex, non-linear relationships in the data, such as image recognition, natural language processing, or tasks where large amounts of data are available. It is less suited in models with small datasets, where overfitting is a significant concern, or scenarios where model interpretability is critical. M5P is best suited with problems where interpretability is important, and the relationships in the data are either linear or can be well captured by a combination of linear models in different partitions of the feature space and less suited with highly complex tasks with intricate non-linear relationships that are difficult to model with simple linear models or decision trees. With the availability of libraries like TensorFlow, Keras, and PyTorch, implementing ANNs has become more accessible. However, expertise in neural network design, tuning, and training is still required. The complexity of ANN architectures and the need for extensive hyperparameter tuning can make them challenging to implement and optimize effectively. M5P models are relatively easy to implement and do not require as much tuning as ANNs. Tools like Weka provide user-friendly interfaces for building and evaluating M5P models. While easier to implement, the simplicity of M5P models may limit their application to more complex tasks where ANNs would be more appropriate. ANNs are powerful for capturing complex, non-linear relationships, especially when large amounts of data are available. They offer high prediction accuracy but come with higher computational costs and lower interpretability. M5P models strike a balance between interpretability and accuracy, particularly for problems with mixed linear and non-linear relationships. They are computationally efficient and more straightforward to implement but may fall short on tasks requiring deep understanding of complex patterns (Dutta et al., 2018). The choice between ANN and M5P models should be based on the specific requirements of the task, including the need for accuracy, interpretability, computational resources, and the nature of the dataset.
6.3 Sensitivity analysis
Sensitivity analysis was carried out in order to study the contribution of individual variables on the deviator stress using a method reported by Garson (Garson, 1991) which was based on weight configuration. The method essentially involves partitioning the hidden-output connection weights of each hidden neuron into components associated with each input neuron. The detailed procedure for this sensitivity analysis with example is provided in the work of Garson (Asteris et al., 2024) as the same was followed in this article. The final relative importance of each individual input parameter on the output is sown in Figure 12. Form the study of Figure 12 reveals that FA is the major influencing parameter followed by C, Fag, Curing, HRWRA, Cag, W and SF respectively. From the above, it can be seen that sensitivity analysis is an effective method of indicating the physical relationship between inputs with the output.
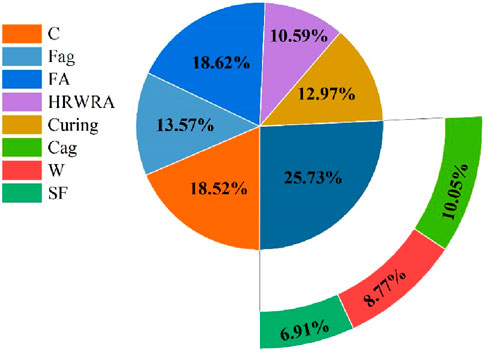
Figure 12. Relative importance (%) of individual input variables on the compressive strength of concrete.
7 Conclusion
The present work is aimed at developing the model equation for the compressive strength of concrete treated with Fly Ash, Silica Fume, High-rate water reducing agent using artificial neural networks and M5P model tree techniques. To obtain the model equations, the independent variables used were cement (C), Fine Aggregate (Fag), Coarse Aggregate (Cag), Water (W), Fly Ash (FA), Silica Fume (SF), High-rate water reducing agent (HRWRA), and Curing time (D). The developed ANN and M5P models and models’ equation for the compressive strength of concrete the following conclusions are put forward.
* The analysis of the plot comparison (targeted versus predicted) shows that the errors are spread consistently along the line in the artificial neural network model. On the other hand, the M5P model tree has a slightly different error distribution, not quite along the line. So, the conclusion is that predictions made with the neural network model are more accurate compared to those made with the M5P model tree technique. This is also supported by various statistical parameters like r, R, MSE, RMSE, MAE, and MAPE.
* Equations were developed for both the ANN and M5P models. However, in the ANN model, forecasting was done using 33 data points out of the total 331. This forecasting effectively predicts the compressive strength of concrete by using only the input parameters during this step.
* A sensitivity analysis was carried out using the weights obtained during the creation of the ANN model. This analysis aimed to understand how the input variables affect the output compressive strength of concrete. The findings show that FA has the greatest impact at 18.62%, followed closely by C at 18.52%.
In general, this paper aims to shed light on using two soft computing techniques to forecast the compressive strength of concrete. This information can be valuable for estimating the compressive strength of concrete treated with Fly Ash, Silica Fume, and a high-rate water reducing agent, saving the cost of conducting expensive experiments.
8 Limitations and future work
There are several limitations and potential areas for future work that should be considered. These are crucial for understanding the scope of the research and identifying opportunities for further investigation. The effectiveness of machine learning models relies heavily on the quality and quantity of data. Limited availability of comprehensive datasets that include various types of industrial wastes and their effects on concrete properties could constrain the model’s accuracy. If certain types of industrial waste are overrepresented in the dataset, the model might be biased, leading to inaccurate predictions for underrepresented waste types. Industrial waste materials can vary significantly in composition depending on the source and processing methods, making it difficult to generalize findings across different waste streams. Closed-form machine learning algorithms might overfit to the training data, particularly if the model is complex relative to the size of the dataset. This could result in poor generalization to new, unseen data. The models developed might be specific to certain types of concrete mixes or waste materials, limiting their applicability to other contexts or regions. Concrete properties are influenced by complex, non-linear interactions between various components. Closed-form models might struggle to capture these interactions, especially if they involve synergistic or antagonistic effects of different industrial wastes. The study might focus on initial compressive strength, but long-term durability and other performance metrics (e.g., resistance to chemical attack, freeze-thaw cycles) are also important. Closed-form models might not adequately predict these long-term effects. The implementation of findings in real-world settings might be challenging. Factors such as the cost of industrial waste processing, availability, and regulatory constraints could limit the practical application of the research. Inconsistent mixing, curing, and environmental conditions during concrete production and application could lead to deviations from predicted compressive strength, affecting the model’s reliability in practical scenarios. While closed-form algorithms can provide quick and interpretable results, they might not capture the complexity of the relationships between variables as effectively as more sophisticated machine learning methods, such as deep learning or ensemble models. Closed-form models typically trade off accuracy for interpretability. While this can be advantageous in understanding the factors affecting compressive strength, it might result in less precise predictions compared to more complex models. However, future research should focus on expanding the dataset to include a wider variety of industrial wastes, mix designs, and environmental conditions. Collaborations with industry partners could help in gathering more diverse and comprehensive data. Integrating long-term performance data, such as durability tests and real-world case studies, can enhance the predictive power of the models and provide a more complete understanding of the impacts. Combining closed-form machine learning algorithms with more advanced techniques, such as neural networks or ensemble methods, could improve the accuracy of predictions while retaining some level of interpretability. Future work could explore models that better capture non-linear interactions between variables, such as polynomial regression, support vector machines, or tree-based methods. Future research could investigate the trade-offs between compressive strength and other properties, such as workability, durability, and environmental impact. Multi-objective optimization techniques could help balance these factors in concrete design. Conducting real-world pilot projects that implement the research findings could provide valuable insights into the practical challenges and effectiveness of using industrial wastes in concrete. Analyzing the economic implications of using industrial wastes in concrete, including lifecycle costs and benefits, could help in understanding the feasibility of large-scale adoption. Future work could contribute to the development of guidelines and standards for incorporating industrial wastes into concrete, ensuring that the research findings are aligned with regulatory requirements and industry practices. Integrating sustainability metrics into the evaluation process, such as carbon footprint reduction or resource efficiency, could help in promoting the adoption of green concrete technologies. Collaborations between material scientists, environmental engineers, and data scientists could lead to more holistic approaches to solving the challenges of incorporating industrial waste into concrete. Investigating new or underutilized waste materials and their potential impact on concrete properties could open up new avenues for sustainable construction practices. While the research on using closed-form machine learning algorithms to evaluate the impact of industrial wastes on concrete compressive strength has significant potential, it is essential to recognize its limitations. Addressing these limitations through future work can lead to more robust, applicable, and sustainable outcomes in the field of concrete technology and environmental engineering.
Data availability statement
The original contributions presented in the study are included in the article/supplementary material, further inquiries can be directed to the corresponding authors.
Author contributions
CL: Data curation, Formal Analysis, Investigation, Project administration, Resources, Software, Supervision, Validation, Visualization, Writing–review and editing, Funding acquisition. CG: Data curation, Formal Analysis, Funding acquisition, Investigation, Project administration, Resources, Software, Visualization, Writing–review and editing, Methodology. KO: Data curation, Formal Analysis, Investigation, Methodology, Project administration, Resources, Software, Visualization, Writing–review and editing, Conceptualization, Supervision, Validation, Writing–original draft. MZ: Conceptualization, Data curation, Formal Analysis, Funding acquisition, Investigation, Methodology, Project administration, Resources, Software, Validation, Visualization, Writing–review and editing. TG: Data curation, Formal Analysis, Investigation, Methodology, Project administration, Resources, Software, Validation, Visualization, Writing–original draft. AA: Data curation, Formal Analysis, Funding acquisition, Investigation, Methodology, Project administration, Resources, Software, Validation, Visualization, Writing–review and editing. NV: Data curation, Formal Analysis, Funding acquisition, Investigation, Methodology, Project administration, Resources, Software, Validation, Visualization, Writing–review and editing. GH: Data curation, Formal Analysis, Funding acquisition, Investigation, Methodology, Project administration, Resources, Software, Validation, Visualization, Writing–review and editing.
Funding
The author(s) declare that no financial support was received for the research, authorship, and/or publication of this article.
Conflict of interest
The authors declare that the research was conducted in the absence of any commercial or financial relationships that could be construed as a potential conflict of interest.
Publisher’s note
All claims expressed in this article are solely those of the authors and do not necessarily represent those of their affiliated organizations, or those of the publisher, the editors and the reviewers. Any product that may be evaluated in this article, or claim that may be made by its manufacturer, is not guaranteed or endorsed by the publisher.
References
Alaloul, W. S., Musarat, M. A., Haruna, S., Law, K., Tayeh, B. A., Rafiq, W., et al. (2021). Mechanical properties of silica fume modified high-volume fly ash rubberized self-compacting concrete. Sustainability 13, 5571. doi:10.3390/su13105571
Ali, B., Mohamed, G., Rebouh, R., Zygouris, N., and Asteris, P. G. (2024). Predicting the shear strength of rectangular RC beams strengthened with externally-bonded FRP composites using constrained monotonic neural networks. Eng. Struct. 313, 118192. doi:10.1016/j.engstruct.2024.118192
Ali, R., Muayad, M., Mohammed, A. S., and Asteris, P. G. (2023). Analysis and prediction of the effect of Nanosilica on the compressive strength of concrete with different mix proportions and specimen sizes using various numerical approaches. Struct. Concr. 24 (3), 4161–4184. doi:10.1002/suco.202200718
AlShareedah, O., and Nassiri, S. (2021). Pervious concrete mixture optimization, physical, and mechanical properties and pavement design: a review. J. Clean. Prod. 288, 125095. doi:10.1016/j.jclepro.2020.125095
Armaghani, D. J., Mamou, A., Maraveas, C., Roussis, P. C., Siorikis, V. G., Skentou, A. D., et al. (2021). Predicting the unconfined compressive strength of granite using only two non-destructive test indexes. Geomechanics Eng. 25 (Number 4), 317–330. doi:10.12989/gae.2021.25.4.317
Ashraf, M., Iqbal, M. F., Rauf, M., Ashraf, M. U., Ulhaq, A., Muhammad, H., et al. (2022). Developing a sustainable concrete incorporating bentonite clay and silica fume: mechanical and durability performance. J. Clean. Prod. 337, 130315. doi:10.1016/j.jclepro.2021.130315
Asteris, P. G., Karoglou, M., Skentou, A. D., Vasconcelos, G., He, M., Bakolas, A., et al. (2024). Predicting uniaxial compressive strength of rocks using ANN models: incorporating porosity, compressional wave velocity, and schmidt hammer data. Ultrasonics 141, 107347. doi:10.1016/j.ultras.2024.107347
Asteris, P. G., Koopialipoor, M., Armaghani, D. J., Kotsonis, E. A., and Lourenço, P. B. (2021a). Prediction of cement-based mortars compressive strength using machine learning techniques. Neural Comput. and Applic 33, 13089–13121. doi:10.1007/s00521-021-06004-8
Asteris, P. G., Lourenço, P. B., Roussis, P. C., Elpida Adami, C., Armaghani, D. J., Cavaleri, L., et al. (2022). Revealing the nature of metakaolin-based concrete materials using artificial intelligence techniques. Constr. Build. Mater. 322, 126500. doi:10.1016/j.conbuildmat.2022.126500
Asteris, P. G., Skentou, A. D., Bardhan, A., Samui, P., and Pilakoutas, K. (2021b). Predicting concrete compressive strength using hybrid ensembling of surrogate machine learning models. Cem. Concr. Res. 145, 106449. doi:10.1016/j.cemconres.2021.106449
Bhanja, S., and Sengupta, B. (2005). Influence of silica fume on the tensile strength of concrete. Cem. Concr. Res. 35, 743–747. doi:10.1016/j.cemconres.2004.05.024
Bhuva, P., and Bhogayata, A. (2022). A review on the application of artificial intelligence in the mix design optimization and development of self-compacting concrete. Mater Today Proc. 65, 603–608. doi:10.1016/j.matpr.2022.03.194
Cavaleri, L., Barkhordari, M. S., Repapis, C. C., Armaghani, D. J., Ulrikh, D. V., and Asteris, P. G. (2022). Convolution-based ensemble learning algorithms to estimate the bond strength of the corroded reinforced concrete. Constr. Build. Mater. 359, 129504. doi:10.1016/j.conbuildmat.2022.129504
Çelik, Z., Bingöl, A. F., and Ağsu, A. S. (2022). Fresh, mechanical, sorptivity and rapid chloride permeability properties of self-compacting concrete with silica fume and fly ash. Iran. J. Sci. Technol. Trans. Civ. Eng. 46, 789–799. doi:10.1007/s40996-021-00676-x
Che, A. R., Ismail, K. N., Ahmad, K. R., and Ibrahim, N. M. (2020). Effects of metakoalin on municipal solid waste incineration (MSWI) bottom ash-cement composite. Mater Sci. Forum 1010, 653–658. doi:10.4028/www.scientific.net/MSF.1010.653
Chen, H., Deng, T., Du, T., Chen, B., Skibniewski, M. J., and Zhang, L. (2022). An RF and LSSVM–NSGA-II method for the multi-objective optimization of high-performance concrete durability. Cem. Concr. Compos 129, 104446. doi:10.1016/j.cemconcomp.2022.104446
Chen, X., Wang, H., Najm, H., Venkiteela, G., and Hencken, J. (2019). Evaluating engineering properties and environmental impact of pervious concrete with fly ash and slag. J. Clean. Prod. 237, 117714. doi:10.1016/j.jclepro.2019.117714
Dandautiya, R., and Singh, A. P. (2019). Utilization potential of fly ash and copper tailings in concrete as partial replacement of cement along with life cycle assessment. Waste Manag. 99, 90–101. doi:10.1016/j.wasman.2019.08.036
de Matos, P. R., Oliveira, J. C. P., Medina, T. M., Magalhães, D. C., Gleize, P. J. P., Schankoski, R. A., et al. (2020). Use of air-cooled blast furnace slag as supplementary cementitious material for self-compacting concrete production. Constr. Build. Mater 262, 120102. doi:10.1016/j.conbuildmat.2020.120102
Dong, B., Qiu, Q., Xiang, J., Huang, C., Sun, H., Xing, F., et al. (2015). Electrochemical impedance interpretation of the carbonation behavior for fly ash–slag–cement materials. Constr. Build. Mater 93, 933–942. doi:10.1016/j.conbuildmat.2015.05.066
Dutta, R. K., Rani, R., and Gnananandarao, T. (2018). Prediction of ultimate bearing capacity of skirted footing resting on sand using artificial neural networks. J. Soft Comput. Civ. Eng. 2 (4), 34–46. doi:10.22115/SCCE.2018.133742.1066.Quinlan
Faisal Alkayem, N., Shen, L., Ali, M., Asteris, P. G., Fu, R., Di Luzio, G., et al. (2024). Prediction of concrete and FRC properties at high temperature using machine and deep learning: a review of recent advances and future perspectives. J. Build. Eng. 83, 108369. doi:10.1016/j.jobe.2023.108369
Ganesh Kumar, B., Muthu, M., Chajec, A., Sadowski, Ł., and Govindaraj, V. (2022). The effect of silica fume on the washout resistance of environmentally friendly underwater concrete with a high-volume of siliceous fly ash. Constr. Build. Mater 327, 127058. doi:10.1016/j.conbuildmat.2022.127058
Garcia-Troncoso, N., Baykara, H., Cornejo, M. H., Riofrio, A., Tinoco-Hidalgo, M., and Flores-Rada, J. (2022). Comparative mechanical properties of conventional concrete mixture and concrete incorporating mining tailings sands. Case Stud. Constr. Mater. 16, e01031. doi:10.1016/J.CSCM.2022.E01031
Ghalehnovi, M., Roshan, N., Taghizadeh, A., Asadi Shamsabadi, E., Ali Hadigheh, S., and de Brito, J. (2022). Production of environmentally friendly concrete incorporating bauxite residue and silica fume. J. Mater Civ. Eng. 34. doi:10.1061/(ASCE)MT.1943-5533.0004060
Güneyisi, E., Gesoğlu, M., Karaoğlu, S., and Mermerdaş, K. (2012). Strength, permeability and shrinkage cracking of silica fume and metakaolin concretes. Constr. Build. Mater 34, 120–130. doi:10.1016/j.conbuildmat.2012.02.017
Guo, Z., Jiang, T., Zhang, J., Kong, X., Chen, C., and Lehman, D. E. (2020). Mechanical and durability properties of sustainable self-compacting concrete with recycled concrete aggregate and fly ash, slag and silica fume. Constr. Build. Mater 231, 117115. doi:10.1016/j.conbuildmat.2019.117115
Hossain, M. M., Karim, M. R., Hasan, M., Hossain, M. K., and Zain, M. F. M. (2016). Durability of mortar and concrete made up of pozzolans as a partial replacement of cement: a review. Constr. Build. Mater 116, 128–140. doi:10.1016/j.conbuildmat.2016.04.147
Huijbregts, M. A. J., Steinmann, Z. J. N., Elshout, P. M. F., Stam, G., Verones, F., Vieira, M., et al. (2017). ReCiPe2016: a harmonised life cycle impact assessment method at midpoint and endpoint level. Int. J. Life Cycle Assess. 22, 138–147. doi:10.1007/s11367-016-1246-y
ISO 14040:2006 (2016). Environmental management: life cycle assessment; Principles and framework. Int. Organ Stand.
Jahangir, H., and Eidgahee, D. R. (2021). A new and robust hybrid artificial bee colony algorithm–ANN model for FRP-concrete bond strength evaluation. Compos Struct. 257, 113160. doi:10.1016/j.compstruct.2020.113160
Khan, M., and Ali, M. (2019). Improvement in concrete behavior with fly ash, silica-fume and coconut fibres. Constr. Build. Mater 203, 174–187. doi:10.1016/j.conbuildmat.2019.01.103
Khan, M. I., and Siddique, R. (2011). Utilization of silica fume in concrete: review of durability properties. Resour. Conserv. Recycl 57, 30–35. doi:10.1016/j.resconrec.2011.09.016
Kumar, K. R., Shyamala, G., Awoyera, P. O., Vedhasakthi, K., and Olalusi, O. B. (2021a). Cleaner production of self-compacting concrete with selected industrial rejects-an overview. Silicon 13, 2809–2820. doi:10.1007/s12633-020-00636-6
Kumar, M., Sinha, A. K., and Kujur, J. (2021b). Mechanical and durability studies on high-volume fly-ash concrete. Struct. Concr. 22. doi:10.1002/suco.202000020
L, P. S., Aruna, G., Krishna Rao, A., and Srinivas Kadrekar, K. (2021). Studies on drying shrinkage and water permeability of fine fly ash high performance concrete. Mater Today Proc. 46, 930–933. doi:10.1016/j.matpr.2021.01.069
Liew, K. M., Sojobi, A. O., and Zhang, L. W. (2017). Green concrete: prospects and challenges. Constr. Build. Mater 156, 1063–1095. doi:10.1016/j.conbuildmat.2017.09.008
Liu, J., Wang, X., Qiu, Q., Ou, G., and Xing, F. (2017). Understanding the effect of curing age on the chloride resistance of fly ash blended concrete by rapid chloride migration test. Mater Chem. Phys. 196, 315–323. doi:10.1016/j.matchemphys.2017.05.011
Ma, F., Sha, A., Yang, P., and Huang, Y. (2016). The greenhouse gas emission from Portland cement concrete pavement construction in China. Int. J. Environ. Res. Public Health 13, 632. doi:10.3390/ijerph13070632
Mazloom, M., Ramezanianpour, A. A., and Brooks, J. J. (2004). Effect of silica fume on mechanical properties of high-strength concrete. Cem. Concr. Compos 26, 347–357. doi:10.1016/S0958-9465(03)00017-9
Nežerka, V., Bílý, P., Hrbek, V., and Fládr, J. (2019). Impact of silica fume, fly ash, and metakaolin on the thickness and strength of the ITZ in concrete. Cem. Concr. Compos 103, 252–262. doi:10.1016/j.cemconcomp.2019.05.012
Nochaiya, T., Wongkeo, W., and Chaipanich, A. (2010). Utilization of fly ash with silica fume and properties of Portland cement–fly ash–silica fume concrete. Fuel 89, 768–774. doi:10.1016/j.fuel.2009.10.003
Onyelowe, K. C., and Ebid, A. M. (2023). The influence of fly ash and blast furnace slag on the compressive strength of high-performance concrete (HPC) for sustainable structures. Asian J. Civ. Eng. 25, 861–882. doi:10.1007/s42107-023-00817-9
Onyelowe, K. C., Ebid, A. M., and Ghadikolaee, M. R. (2023b). GRG-optimized response surface powered prediction of concrete mix design chart for the optimization of concrete compressive strength based on industrial waste precursor effect. Asian J. Civ. Eng. 25, 997–1006. doi:10.1007/s42107-023-00827-7
Onyelowe, K. C., Ebid, A. M., and Hanandeh, S. (2023c). The influence of nano-silica precursor on the compressive strength of mortar using Advanced Machine Learning for sustainable buildings. Asian J. Civ. Eng. 25, 1135–1148. doi:10.1007/s42107-023-00832-w
Onyelowe, K. C., Ebid, A. M., and Hanandeh, S. (2023d). Advanced machine learning prediction of the unconfined compressive strength of geopolymer cement reconstituted granular sand for road and liner construction applications. Asian J. Civ. Eng. 2023, 1027–1041. doi:10.1007/s42107-023-00829-5
Onyelowe, K. C., Ebid, A. M., Hanandeh, S., Moghal, A. A. B., Onuoha, I. C., Obianyo, I. I., et al. (2023e). The influence of fines on the hydro-mechanical behavior of sand for sustainable compacted liner and sub-base construction applications. Asian J. Civ. Eng. 25, 623–635. doi:10.1007/s42107-023-00800-4
Onyelowe, K. C., Gnananandarao, T., and Ebid, A. M. (2022b). Estimation of the erodibility of treated unsaturated lateritic soil using support vector machine-polynomial and radial basis function and random forest regression techniques. Clean. Mater. 3, 100039. doi:10.1016/j.clema.2021.100039
Onyelowe, K. C., Gnananandarao, T., Mahdi, H. A., Ghadikolaee, M. R., and Al-Ajamee, M. (2022a). Evaluating the compressive strength of recycled aggregate concrete using novel artificial neural networks. Civ. Eng. J. 8 (8), 1679–1693.
Onyelowe, K. C., Gnananandarao, T., and Nwa-David, C. (2021). Sensitivity analysis and prediction of erodibility of treated unsaturated soil modified with nanostructured fines of quarry dust using novel artificial neural network. Nanotechnol. Environ. Eng. 6 (37), 37–11. doi:10.1007/s41204-021-00131-2
Onyelowe, K. C., Kontoni, D.-P. N., Pilla, S. R. M., Hanandeh, S., Ebid, A. M., Razzaghian Ghadikolaee, M., et al. (2023a). Runtime-based metaheuristic prediction of the compressive strength of net-zero traditional concrete mixed with BFS, FA, SP considering multiple curing regimes. Asian J. Civ. Eng. 25, 1241–1253. doi:10.1007/s42107-023-00839-3
Panesar, D. K., Kanraj, D., and Abualrous, Y. (2019). Effect of transportation of fly ash: life cycle assessment and life cycle cost analysis of concrete. Cem. Concr. Compos. 99, 214–224. doi:10.1016/j.cemconcomp.2019.03.019
Quinlan, J. R. (1992). “Learning with continuous classes,” in Australian joint conference on artificial intelligence, 343–348.
Rezazadeh Eidgahee, D., Jahangir, H., Solatifar, N., Fakharian, P., and Rezaeemanesh, M. (2022). Data-driven estimation models of asphalt mixtures dynamic modulus using ANN, GP and combinatorial GMDH approaches. Neural Comput. Appl. 34, 17289–17314. doi:10.1007/s00521-022-07382-3
Sahoo, S., Parhi, P. K., and Chandra Panda, B. (2021). Durability properties of concrete with silica fume and rice husk ash. Clean. Eng. Technol. 2, 100067. doi:10.1016/j.clet.2021.100067
Salesa, Á., Pérez-Benedicto, J. Á., Esteban, L. M., Vicente-Vas, R., and Orna-Carmona, M. (2017). Physico-mechanical properties of multi-recycled self-compacting concrete prepared with precast concrete rejects. Constr. Build. Mater 153, 364–373. doi:10.1016/j.conbuildmat.2017.07.087
Samad, S., and Shah, A. (2017). Role of binary cement including Supplementary Cementitious Material (SCM), in production of environmentally sustainable concrete: a critical review. Int. J. Sustain Built Environ. 6, 663–674. doi:10.1016/j.ijsbe.2017.07.003
Sandanayake, M., Gunasekara, C., Law, D., Zhang, G., Setunge, S., and Wanijuru, D. (2020). Sustainable criterion selection framework for green building materials – an optimisation based study of fly-ash Geopolymer concrete. Sustain Mater Technol. 25, e00178. doi:10.1016/j.susmat.2020.e00178
Seto, K. E., Churchill, C. J., and Panesar, D. K. (2017). Influence of fly ash allocation approaches on the life cycle assessment of cement-based materials. J. Clean. Prod. 157, 65–75. doi:10.1016/j.jclepro.2017.04.093
Siamardi, K. (2022). Optimization of fresh and hardened properties of structural light weight self-compacting concrete mix design using response surface methodology. Constr. Build. Mater 317, 125928. doi:10.1016/j.conbuildmat.2021.125928
SO 14044:2006 (2016). Environmental management: life cycle assessment; Principles and framework. Int. Organ Stand.
Soleymani, A., and Esfahani, mohammad R. (2019). Effect of concrete strength and thickness of flat slab on preventing of progressive collapse caused by elimination of an internal column. J. Struct. Constr. Eng. 6, 24–40. doi:10.22065/jsce.2017.98444.1335
Solouki, A., Tataranni, P., and Sangiorgi, C. (2022). Mixture optimization of concrete paving blocks containing waste silt. Sustainability 14, 451. doi:10.3390/su14010451
Sun, Z., Yang, S., Hang, M., Wang, J., and Yang, T. (2022). Optimization design of ultrahigh-performance concrete based on interaction analysis of multiple factors. Case Stud. Constr. Mater 16, e00858. doi:10.1016/j.cscm.2021.e00858
Tayeh, B. A., Ibrahim, O., and Mohamed, O. (2020). Combined effect of lightweight fine aggregate and micro rubber ash on the properties of cement mortar. Adv. Concr. Constr. 10.
Teixeira, E. R., Mateus, R., Camões, A. F., Bragança, L., and Branco, F. G. (2016). Comparative environmental life-cycle analysis of concretes using biomass and coal fly ashes as partial cement replacement material. J. Clean. Prod. 112, 2221–2230. doi:10.1016/j.jclepro.2015.09.124
The International Standards Organisation (2006). Environmental management — life cycle assessment — principles and framework, 1–47. Standard No. 14040).
Thomas, M. D. A., Shehata, M. H., Shashiprakash, S. G., Hopkins, D. S., and Cail, K. (1999). Use of ternary cementitious systems containing silica fume and fly ash in concrete. Cem. Concr. Res. 29, 1207–1214. doi:10.1016/S0008-8846(99)00096-4
Ting, M. Z. Y., Wong, K. S., Rahman, M. E., and Selowarajoo, M. (2021). Prediction model for hardened state properties of silica fume and fly ash based seawater concrete incorporating silicomanganese slag. J. Build. Eng. 41, 102356. doi:10.1016/j.jobe.2021.102356
Wang, A., Huang, M., Chu, Y., Zhu, Y., Liu, K., Guo, L., et al. (2022a). Optimization of mix proportion of basic magnesium sulfate cement-based high-strength coral concrete. Constr. Build. Mater 341, 127709. doi:10.1016/j.conbuildmat.2022.127709
Wang, L., Guo, F., Lin, Y., Yang, H., and Tang, S. W. (2020). Comparison between the effects of phosphorous slag and fly ash on the C-S-H structure, long-term hydration heat and volume deformation of cement-based materials. Constr. Build. Mater. 250, 118807. doi:10.1016/j.conbuildmat.2020.118807
Wang, L., Guo, F., Yang, H., Wang, Y., and Tang, S. (2021c). Comparison of fly ash, Pva fiber, mgo and shrinkage-reducing admixture on the frost resistance of face slab concrete via pore structural and fractal analysis. Fractals 29 (02), 2140002. Special Issue Section on Fractals in Construction Materials. doi:10.1142/S0218348X21400028
Wang, L., Jin, M., Guo, F., Wang, Y., and Tang, S. (2021a). Pore structural and fractal analysis of the influence of fly ash and silica fume on the mechanical property and abrasion resistance of concrete. Fractals 29, 2140003. doi:10.1142/S0218348X2140003X
Wang, L., Jin, M., Guo, F., Wang, Y., and Tang, W. (2021b). Pore structural and fractal analysis of the influence of fly ash and silica fume on the mechanical property and abrasion resistance of concrete. FractalsVol 29 (02), 2140003. Special Issue Section on Fractals in Construction Materials. doi:10.1142/S0218348X2140003X
Wang, L., Yu, Z., Liu, B., Zhao, F., Tang, S., and Jin, M. (2022b). Effects of fly ash dosage on shrinkage, crack resistance and fractal characteristics of face slab concrete. Fractal Fract. 6 (6), 335. doi:10.3390/fractalfract6060335
Warda, M. A., Ahmad, S. S. E., Mahdi, I. M., Sallam, H. E.-D. M., and Khalil, H. S. (2022). The applicability of TOPSIS- and fuzzy TOPSIS-based taguchi optimization approaches in obtaining optimal fiber-reinforced concrete mix proportions. Buildings 12, 796. doi:10.3390/buildings12060796
Wernet, G., Bauer, C., Steubing, B., Reinhard, J., Moreno-Ruiz, E., and Weidema, B. (2016). The ecoinvent database version 3 (part I): overview and methodology. Int. J. Life Cycle Assess. 21, 1218–1230. doi:10.1007/s11367-016-1087-8
Xing, W., Tam, V. W., Le, K. N., Hao, J. L., and Wang, J. (2022). Life cycle assessment of recycled aggregate concrete on its environmental impacts: a critical review. Constr. Build. Mater 317, 125950. doi:10.1016/j.conbuildmat.2021.125950
Yang, J., Huang, J., Su, Y., He, X., Tan, H., Yang, W., et al. (2019). Eco-friendly treatment of low-calcium coal fly ash for high pozzolanic reactivity: a step towards waste utilization in sustainable building material. J. Clean. Prod. 238, 117962. doi:10.1016/j.jclepro.2019.117962
Zhang, H., Nguyen, H., Bui, X. N., Pradhan, B., Asteris, P. G., Costache, R., et al. (2022). A generalized artificial intelligence model for estimating the friction angle of clays in evaluating slope stability using a deep neural network and Harris Hawks optimization algorithm. Eng. Comput. 38 (Suppl. 5), 3901–3914. doi:10.1007/s00366-020-01272-9
Keywords: green concrete, industrial wastes, compressive strength, M5P, ANN, sensitivity analysis
Citation: López Paredes CR, García C, Onyelowe KC, Zuniga Rodriguez MG, Gnananandarao T, Andrade Valle AI, Velasco N and Herrera Morales GC (2024) Evaluating the impact of industrial wastes on the compressive strength of concrete using closed-form machine learning algorithms. Front. Built Environ. 10:1453451. doi: 10.3389/fbuil.2024.1453451
Received: 23 June 2024; Accepted: 12 September 2024;
Published: 03 October 2024.
Edited by:
Rahul V. Ralegaonkar, Visvesvaraya National Institute of Technology, IndiaReviewed by:
Panagiotis G. Asteris, School of Pedagogical and Technological Education, GreeceShengwen Tang, Wuhan University, China
Copyright © 2024 López Paredes, García, Onyelowe, Zuniga Rodriguez, Gnananandarao, Andrade Valle, Velasco and Herrera Morales. This is an open-access article distributed under the terms of the Creative Commons Attribution License (CC BY). The use, distribution or reproduction in other forums is permitted, provided the original author(s) and the copyright owner(s) are credited and that the original publication in this journal is cited, in accordance with accepted academic practice. No use, distribution or reproduction is permitted which does not comply with these terms.
*Correspondence: Kennedy C. Onyelowe, a2VubmVkeWNoaWJ1em9yQGtpdS5hYy51Zw==; Carlos Roberto López Paredes, a29ueWVsb3dlQG1vdWF1LmVkdS5uZw==